- 1Institute of Psychology, Erasmus University Rotterdam, Rotterdam, Netherlands
- 2Department of Child and Adolescent Psychiatry, VU University Medical Center, Amsterdam, Netherlands
- 3Behavioural Science Institute, Radboud University Nijmegen, Nijmegen, Netherlands
One of the major challenges in addiction treatment is relapse prevention, as rates of relapse following treatment remain very high across the main classes of drugs of abuse. Relapse prevention could be improved by a better understanding of the factors that influence treatment outcomes, including better predictors of risk of relapse following treatment. Recent developments in cognitive neuroscience point to neurocognitive measures (i.e., brain-imaging measures during cognitive-task performance) as potential predictors of relapse. These might even be better predictors than self-report measures, such as craving. We first give an overview of the current state of the field, and then discuss the outstanding challenges and future directions in this area of research.
Substance-dependent individuals often relapse, despite their efforts to stay abstinent (1). Substance dependence is therefore characterized as a chronic relapsing disorder (2, 3). For example, after 1–6 months follow-up, 40–80% of the heroin- and cocaine-dependent patients who were in treatment relapse (4–7). To improve treatment and treatment assignment for these patients it is important to gain knowledge about the psychological and biological processes underlying treatment outcome and relapse. The aim of this review is to describe the use of neurocognitive measures in addiction research in relation to the prediction of relapse, and discuss their clinical relevance. Since there is considerable overlap between the various substances of abuse, we attempt to focus on factors which are known to play a role in substance-use disorders in general (i.e., alcohol, cigarette smoking, stimulants, and opiates). Where research on a specific substance is described this is indicated.
Predictors of Substance Relapse: From Self-Report to Neurocognitive Measures
Over the years, various kinds of predictors have been studied in relation to substance-use relapse such as demographic characteristics and other variables such as drug use severity, medical problems, and psychopathology [for reviews see Ref. (8, 9)]. In addition, self-report measures of emotional states such as negative affect (10), and drug-related states such as craving (11–13) have also found to be predictive of substance relapse [contrasting findings: (14, 15)]. However, an important limitation of using self-report measures is that people – and particularly substance-dependent individuals – may have low insight into their motivations and misrepresent their thoughts and feelings, or their reports may be biased due to social desirability (16, 17).
Neurocognitive measures, including neurophysiological measures such as functional magnetic resonance imaging (fMRI) and electroencephalography (EEG) during cognitive-task performance, arguably overcome some of the limitations of self-report measures. During neurocognitive assessments, participants are often unaware of the purpose of the assessment. Automatic, fast cognitive processes that are unavailable to conscious introspection can influence behavior [e.g., Ref. (18)]. These processes cannot be assessed via self-reports, but they can be assessed by neurocognitive psychological assessments. In the last two decades, the use of these neurocognitive assessments to examine neurobiological and cognitive processes underlying addiction has emerged in addiction research (19). Additionally, implicit cognitive and physiological measures hold some promise in predicting drug relapse and may even be better predictors than self-report measures [e.g., Ref. (13, 20–22)]; we will explore this possibility later in this review. Before elaborating upon the association between neurocognitive measures and substance relapse, a short overview of some relevant neurocognitive theories of addiction and supporting empirical evidence will be provided.
Neurocognitive Processes in Addiction
Various recent theories of addiction suggest an imbalance in motivational and cognitive control processes in substance-dependent individuals (23–27). More specifically, it is proposed that substance-dependent individuals have an overactive motivational system that develops as a consequence of repetitive drug use. Repetitive drug use sensitizes the mesolimbic reward system up to a point that merely the perception (and not only the use) of drugs or drug cues becomes salient (28). Because of this incentive salience that is being attributed to drug-related stimuli, attention is automatically oriented to these stimuli, also referred to as attentional bias (24).
A wide range of behavioral studies have confirmed the presence of an attentional bias to substance cues in dependent individuals [for review see Ref. (29)] and its association with self-reported craving has also been supported (30). Accordingly, there has been much interest in investigating the neurobiological substrates of attentional bias. Recent fMRI studies showed that attentional bias to substance cues is associated with activity in prefrontal brain areas such as the anterior cingulate cortex (ACC) and dorsolateral prefrontal cortex (DLPFC) (31–36), other cortical areas including the insula (32, 35, 36) and also subcortical activation in the nucleus accumbens (34) and amygdala (35, 37). It has been suggested that these brain regions play a role in the imbalance between motivational and control processes; that is, the nucleus accumbens and amygdala are evidently involved in the bottom-up process of salience attribution to substance-related stimuli while at the same time top-down attentional resources of the prefrontal executive areas might be impaired or depleted when focusing on cognitive tasks in the presence of distracting drug-related cues (25, 38).
Other theories suggest that the ability to control drug use behaviors is reduced in drug-dependent individuals, particularly in conditions that deplete cognitive recourses, like craving or cue-exposure (38, 39). Several studies indeed reported that cognitive control processes, which have their neural basis in regions of the prefrontal cortex, are impaired in substance-dependent people (25, 40, 41). Two specific cognitive control processes (i.e., inhibitory control and error-processing) may be particularly involved in the continuation of substance use. Inhibitory control is crucial when one would like to control substance use by implementing the inhibition of inappropriate behavior, while error-processing is involved in monitoring ongoing behavior to prevent future mistakes. A recent review of neuroimaging studies (41) into inhibitory control and error-processing suggests that substance dependence is associated with reduced brain activation during inhibitory control and error-processing in the ACC, inferior frontal gyrus (IFG), and DLPFC. In addition, event-related potentials such as the N2 and error-related negativity (ERN), reflecting brain activation associated with inhibitory control and error-processing respectively, seem to be reduced in substance-dependent patients. These findings implicate that cognitive control processes of substance-dependent people may be dysfunctional, thereby contributing to the lack of control over substance-related behaviors.
In sum, both drug-related motivational processes as well as dysfunctions in cognitive control may contribute to compulsive drug use behavior and this might explain why substance-dependent individuals cannot control their drug use and often relapse after a period of abstinence (23, 38, 39). Below, we will discuss studies that have prospectively examined neurocognitive measures and their association with substance-use outcomes. Note that all studies described below report effects on group level; the clinical relevance for individual patients will be discussed later in this review.
Neurocognitive Predictors of Treatment Outcome and Relapse
Well-established research on the role of cognitive and neurobiological processes in addiction has resulted in an increased focus on neurocognitive measures as predictors of treatment outcome and relapse. On the behavioral level, results have mainly showed an association between attentional bias and treatment outcome in substance dependency [although some results have been inconsistent; for a recent review see Ref. (42)]. To the best of our knowledge, only two fMRI studies have used an attentional bias paradigm to examine whether brain-activity related to attentional bias was associated with substance relapse (36, 43). Other fMRI studies have examined whether cue-reactivity to substance-related stimuli might predict substance-use outcomes [(44, 45), contrasting findings: (46); see Table 1].
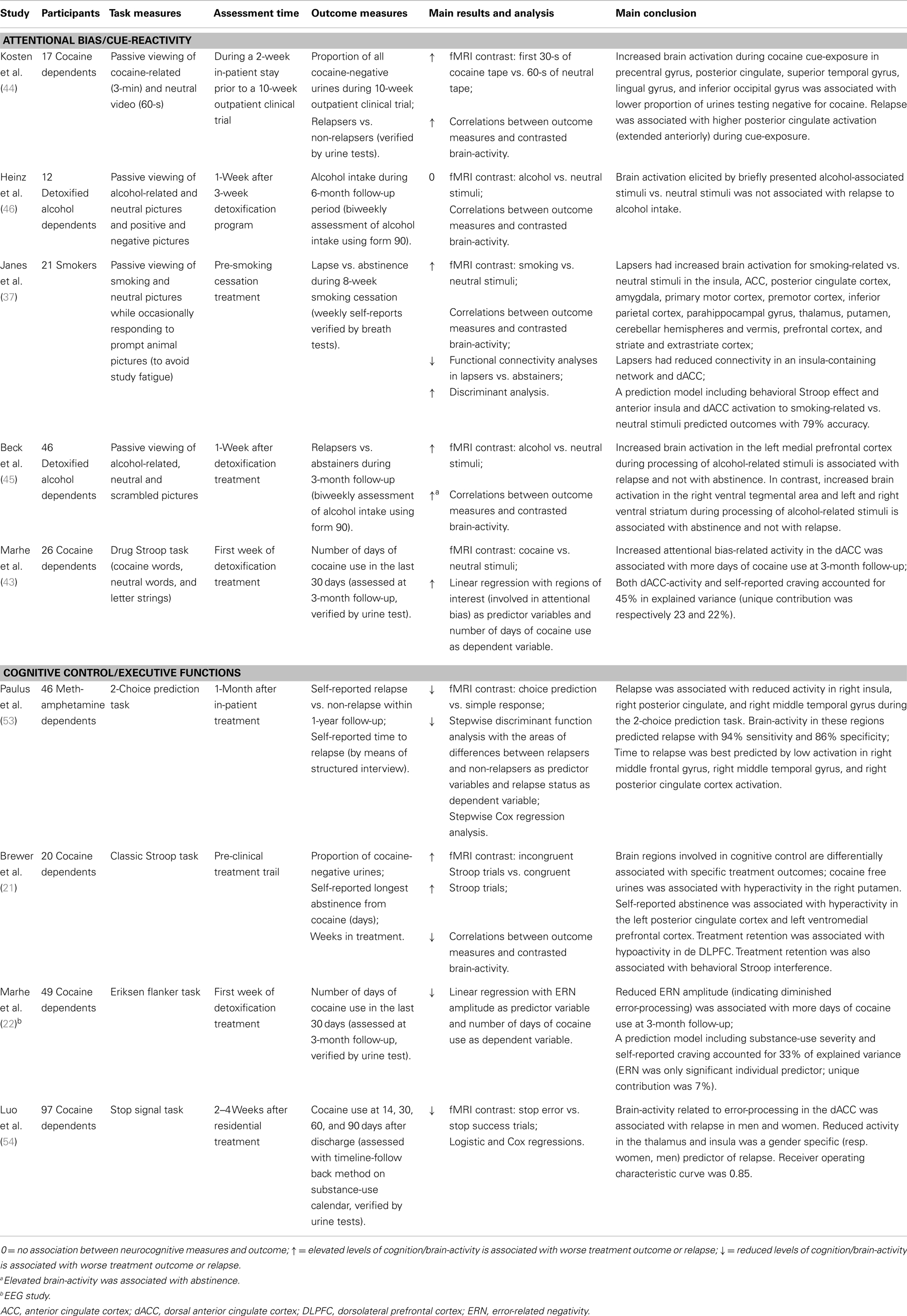
Table 1. Overview of studies described in the present review investigating neurocognitive predictors of substance relapse after treatment.
Overall, the cue-reactivity studies show that enhanced brain-activity during substance cue-exposure in prefrontal, sensory, motor, and limbic (sub)cortical areas is associated with substance relapse [(44, 45), cf. (46)]. Note that results in alcohol dependent patients are inconsistent. Heinz et al. (46) found no relation between neural cue-reactivity and alcohol intake after treatment. In contrast, Beck et al. (45) found that increased prefrontal brain-activity (during passive viewing of alcohol cues) was associated with relapse after treatment while increased activity in the ventral tegmental area and ventral striatum were associated with abstinence after treatment, indicating that different brain processes (cognitive control vs. reward system) are differently associated with treatment outcome in alcohol dependents.
In smokers, Janes et al. (36) found that both behavioral attentional bias for smoking-related words (measured with a Stroop task outside of the scanner) along with reactivity of the brain to smoking cues were predictive of smoking relapse. In addition, anterior insula and dorsal ACC (dACC) activation strongly correlated with respectively larger interference of drug-related words and low accuracy during the Stroop task, suggesting that these regions might represent the neural correlates of attentional bias that may be important for identifying individuals at risk of relapse. This idea is supported by a recent study showing that in cocaine-dependent patients, increased dACC-activity related to attentional bias for cocaine stimuli (measured with a drug Stroop task) was associated with relapse to cocaine use after treatment (43). Thus, it seems that the dACC – involved in salience detection and conflict monitoring (47–50) – plays an important role in relapse risk. It has been suggested that hyperactivity in the dACC reflects enhanced conflict in the presence of emotionally salient distracters, such as substance-related stimuli. Hence, increased dACC-activity in response to substance cues might reflect that patients at risk of relapse need more top-down resources to focus on cognitive tasks when substance-related cues are present as distractors during the task. This implies that relapse-vulnerable individuals have a reduced ability to control their substance-related cognitions, regulated by the dACC, and consequently might experience difficulties in controlling their substance-use behavior.
Studies examining the association between cognitive control processes (e.g., inhibitory and attentional control, behavior monitoring) and relapse following treatment have generally found that impaired cognitive functioning is associated with a higher risk of relapse [for recent reviews see Ref. (51, 52)]. Only a few studies have examined whether brain-activity related to performance on cognitive tasks is associated with substance relapse. Paulus and colleagues (53) were the first to report that brain-activity during a simple two-choice task (measured with fMRI) can predict relapse in methamphetamine dependence. This indicated that relapse vulnerability was associated with reduced activation in a brain network related to decision-making (e.g., DLPFC, parietal, temporal, and insular cortex). An fMRI study in cocaine-dependent patients found that behavioral interference on the classical Stroop task and associated brain activations in prefrontal and striatal regions are predictive of treatment outcome, which indicates that impaired attentional control may be a marker of relapse risk (21). Two recent studies have examined the association between brain-activity during error-processing and relapse in cocaine-dependent patients [(22, 54), see also Ref. (55)]. These studies found that reduced brain-activity during error-processing is associated with cocaine use after treatment. More specifically, Luo et al. (54) found that reduced thalamus, insula, and dACC-activity, measured with fMRI, was predictive of relapse to cocaine. Marhe et al. (22) examined the ERN, an event-related potential reflecting the automatic detection of an error. Results showed that ERN amplitudes are associated with increased cocaine use 3 months after detoxification treatment. These findings suggest that underactive error-related brain-activity might be a marker of relapse risk (55).
Most of the abovementioned studies indicate that cognitive and motivational processes are associated with relapse vulnerability. This is only a first step toward clinical implementation, and current findings need to be interpreted with caution since group results are not necessarily valid on individual results. Different methodologies and designs hamper a direct comparison between these studies. Further, it is not known whether the results found in one substance-dependent group (e.g., cocaine-dependent patients) can be generalized to other substances. In addition, the treatment settings are quite diverse. However, if these findings will stand after replication and provide more accurate information on individual-level prediction, they might eventually help to identify substance-dependent patients that are at risk of relapse into substance use. Obviously, this is not only the case for neurocognitive predictors, but for all predictors of relapse, including demographical, self-report, or behavioral measures.
Are Neurocognitive Measures Better Predictors of Relapse than Self-Report Measures?
It is clear that neurocognitive measures such as fMRI and – to a lesser degree – EEG are relative expensive and time consuming, limiting their daily use in clinical practice. Therefore, in order to advocate the use of neurocognitive measures in clinical practice, there should be a clear advantage compared to inexpensive and more feasible self-report predictors of relapse such as self-reported craving and substance-use severity. One hypothetical advantage could be that neurocognitive measures explain relapse better than self-report measures – or at least explain additional variance in predicting relapse over and above self-report measures.
Current relapse prediction studies addressing motivational and cognitive control processes provide some preliminary indications for this. Some of the studies addressing motivational aspects found that brain-activity during substance cue-exposure was associated with relapse, whereas self-reported craving and substance-use severity were not associated with relapse (44–46). Marhe et al. (43) also found that the association between attentional bias-related brain-activity and relapse persisted when controlling for self-reported substance-use severity. However, self-reported craving and attentional bias-related brain-activity contributed equally to the prediction of cocaine relapse (i.e., craving explained 23% and dACC-activity explained 22% of the variance). In addition, studies addressing cognitive control also show additional benefit of neurocognitive measures above self-reported measures. Paulus and colleagues (53) reported that substance-use severity was not associated with methamphetamine relapse, while brain-activity during decision-making was. Brewer et al. (21) showed that brain activation during Stroop interference was more strongly related to treatment outcome than self-reported craving. Additionally, another study (22) showed that brain-activity during error-processing was a unique predictor of cocaine relapse, over and above substance-use severity and self-reported craving.
Hence, some relapse prediction studies indicate that neurocognitive measures might make a unique contribution to the prediction of relapse and may even be able to better predict outcomes than self-report measures such as craving. In addition, regarding the specific role of subjective craving, results suggest that the relationship between neurocognitive measures and relapse is not accounted for or mediated by craving [e.g., Ref. (42)]. Speculatively, they reflect two processes that might both explain variance in relapse risk.
Limitation Concerning the Use of Neurocognitive Measures in Clinical Practice and Suggestions for Future Research
Neurocognitive methods provide us with crucial information of how brain responses are related to clinical outcomes. Although there are some indications that neurocognitive measures could be relevant in clinical practice there are some issues that need further research before these measures can be applied in a clinical setting. Obviously, one of the biggest challenges is moving from group-level associations with treatment outcomes/relapse to individual-level prediction of such outcomes, for example by using receiver operating characteristic analyses (53, 54). This technique provides information on the sensitivity and specificity of predictors, which need to be sufficient enough to contribute to treatment planning for an individual patient.
In the long run, neurocognitive techniques such as fMRI could be used to investigate individual risk profiles for example with the use of machine-learning approaches (56). However, in the short term it is not feasible and cost-effective to scan every patient in substance-use treatment programs using fMRI. Although the same problems are true for EEG, it is arguably a more cost-effective and more accessible neuroimaging tool that could be implemented in treatment programs more easily. The idea to use EEG as a diagnostic instrument has gained interest specifically for ERP components that have adequate psychometric properties, such as the ERN (57, 58). Future studies should be carried out to examine whether routine assessment of for example ERN amplitudes in cocaine-dependent patients [see Ref. (22)] could identify patients vulnerable for relapse. Additionally, treatment programs could be tailored to the patient’s need to improve outcomes. For example, by providing specific training programs to improve cognitive [e.g., Ref. (59)] and/or brain functions [e.g., Ref. (60)].
Another limitation is that all studies use different measures of treatment outcome/relapse (e.g., self-reported use or abstinence, urine screens, time to relapse) which makes it difficult to compare the present results. Using the same (multiple) outcome measures across studies would certainly advance the field, also beyond neurocognitive studies.
Also, current prediction studies using neurocognitive measures have not addressed the role of response inhibition, which is another important index of cognitive control. Some evidence comes from cross-sectional studies showing that ex-substance users have increased brain-activity during inhibitory control (assessed with Go-Nogo) compared to current users, suggesting that response inhibition might underlie recovery from substance dependence [smokers: (34); cocaine users: (61)]. Future studies should address the predictive role of response inhibition in prospective designs.
Finally, it is of theoretical as well as clinical importance that studies investigating the predictive value of neurocognitive processes of addiction include self-report and behavioral measures in prediction models. It is important to test whether the unique contribution of these relatively “new” measures is high enough in addition to well-established, more easily administered measures. Ideally, relapse prediction models should be multifactorial and should include (socio)demographic, psychological, physiological, and cognitive variables. Of course, large sample sizes are needed to accomplish sufficient power (62).
Conclusion
Neuroimaging research has yielded important information on neurocognitive mechanisms of substance dependence in relation to treatment processes and outcome. Results have shown that neurocognitive measures can provide information on relapse vulnerability over and above the information gained from self-report measures such as craving on a group level. However, regarding clinical utility it is important that all prediction studies report the sensitivity and specificity of neurocognitive relapse predictors. This will allow gaining more knowledge on the suitability of neurocognitive measures for individual risk taxation, necessary for implementation in clinical settings.
Conflict of Interest Statement
The authors declare that the research was conducted in the absence of any commercial or financial relationships that could be construed as a potential conflict of interest.
References
1. American Psychiatric Association. Diagnostic and Statistical Manual of Mental Disorders. 4th ed. Washington, DC: American Psychiatric Press (1994).
2. Leshner AI. Addiction is a brain disease, and it matters. Science (1997) 278:45–7. doi: 10.1126/science.278.5335.45
3. McLellan AT, Lewis DC, O’Brien CP, Kleber HD. Drug dependence, a chronic medical illness: implications for treatment, insurance, and outcomes evaluation. J Am Med Assoc (2000) 284(13):1689–95. doi:10.1001/jama.284.13.1689
4. Hättenschwiler J, Rüesch P, Hell D. Effectiveness of inpatient drug detoxification: links between process and outcome variables. Eur Addict Res (2000) 6:123–31. doi:10.1159/000019024
5. Gossop M, Stewart D, Browne N, Marsden J. Factors associated with abstinence, lapse or relapse to heroin use after residential treatment: protective effect of coping responses. Addiction (2002) 97:1259–67. doi:10.1046/j.1360-0443.2002.00227.x
6. Day E, Strang J. Outpatient versus inpatient opioid detoxification: a randomized controlled trial. J Subst Abuse Treat (2011) 40:56–66. doi:10.1016/j.jsat.2010.08.007
7. Gossop M, Green L, Phillips G, Bradley B. What happens to opiate addicts immediately after treatment: a prospective follow up study. Br Med J (1987) 294:1377–80. doi:10.1136/bmj.294.6584.1377
8. Stark MJ. Dropping out of substance abuse treatment: a clinically oriented review. Clin Psychol Rev (1992) 12:93–116. doi:10.1016/0272-7358(92)90092-M
9. Poling J, Kosten TR, Sofuoglu M. Treatment outcome predictors for cocaine dependence. Am J Drug Alcohol Abuse (2007) 33:191–206. doi:10.1080/00952990701199416
10. Shiffman S, Waters AJ. Negative affect and smoking lapses: a prospective analysis. J Consult Clin Psychol (2004) 72:192–201. doi:10.1037/0022-006X.72.2.192
11. Weiss RD, Griffin ML, Mazurick C, Berkman B, Gastfriend DR, Frank A, et al. The relationship between cocaine craving, psychosocial treatment, and subsequent use. Am J Psychiatry (2003) 160:1320–5. doi:10.1176/appi.ajp.160.7.1320
12. Preston KL, Vahabzadeh M, Schmittner J, Lin J, Gorelick DA, Epstein DH. Cocaine craving and use during daily life. Psychopharmacology (2009) 207:291–301. doi:10.1007/s00213-009-1655-8
13. Marhe R, Waters AJ, Van de Wetering BJM, Franken IHA. Implicit and explicit drug-related cognitions during detoxification treatment are associated with drug relapse: an ecological momentary assessment study. J Consult Clin Psychol (2013) 81(1):1–12. doi:10.1037/a0030754
14. Weiss RD, Griffin ML, Hufford C. Craving in hospitalized cocaine abusers as a predictor of outcome. Am J Drug Alcohol Abuse (1995) 21:289–301. doi:10.3109/00952999509002698
15. Bordnick PS, Schmitz JM. Cocaine craving: an evaluation across treatment phases. J Subst Abuse (1998) 10:9–17. doi:10.1016/S0899-3289(99)80136-9
16. Hammersley R. A digest of memory phenomena for addiction research. Addiction (1994) 89:283–93. doi:10.1111/j.1360-0443.1994.tb00890.x
17. Marissen MAE, Franken IHA, Blanken P, van den Brink W, Hendriks VM. The relation between social desirability and different measures of heroin craving. J Addict Dis (2005) 24:91–103. doi:10.1300/J069v24n04_07
18. Fazio RH, Olson MA. Implicit measures in social cognition: their meaning and uses. Annu Rev Psychol (2003) 54:297–327. doi:10.1146/annurev.psych.54.101601.145225
19. Garavan H, Brennan K, Hester R, Whelan R. The neurobiology of successful abstinence. Curr Opin Neurobiol (2013) 23(4):668–74. doi:10.1016/j.conb.2013.01.029
20. Marissen MAE, Franken IHA, Waters AJ, Blanken P, van den Brink W, Hendriks VM. Attentional bias predicts heroin relapse following treatment. Addiction (2006) 101:1306–12. doi:10.1111/j.1360-0443.2006.01498.x
21. Brewer JA, Worhunsky PD, Carroll KM, Rounsaville BJ, Potenza MN. Pretreatment brain activation during Stroop task is associated with outcomes in cocaine-dependent patients. Biol Psychiatry (2008) 64:998–1004. doi:10.1016/j.biopsych.2008.05.024
22. Marhe R, van de Wetering BJM, Franken IHA. Error-related brain activity predicts cocaine use after treatment at 3-month follow-up. Biol Psychiatry (2013) 73(8):782–8. doi:10.1016/j.biopsych.2012.12.016
23. Field M, Cox WM. Attentional bias in addictive behaviors: a review of its development, causes, and consequences. Drug Alcohol Depend (2008) 97:1–20. doi:10.1016/j.drugalcdep.2008.03.030
24. Franken IHA. Drug craving and addiction: integrating psychological and neuropsychopharmacological approaches. Prog Neuropsychopharmacol Biol Psychiatry (2003) 27:563–79. doi:10.1016/S0278-5846(03)00081-2
25. Goldstein RZ, Volkow ND. Dysfunction of the prefrontal cortex in addiction: neuroimaging findings and clinical implications. Nat Rev Neurosci (2011) 12:652–69. doi:10.1038/nrn3119
26. Volkow ND, Fowler JS, Wang G-J. The addicted human brain viewed in the light of imaging studies: brain circuits and treatment strategies. Neuropharmacology (2004) 47(Suppl 1):3–13. doi:10.1016/j.neuropharm.2004.07.019
27. Wiers RW, Bartholow BD, van den Wildenberg E, Thush C, Engels RCME, Sher KJ, et al. Automatic and controlled processes and the development of addictive behaviors in adolescents: a review and a model. Pharmacol Biochem Behav (2007) 86:263–83. doi:10.1016/j.pbb.2006.09.021
28. Robinson TE, Berridge KC. The neural basis of drug craving: an incentive-sensitization theory of addiction. Brain Res Rev (1993) 18:247–91. doi:10.1016/0165-0173(93)90013-P
29. Cox WM, Fadardi JS, Pothos EM. The Addiction-Stroop test: theoretical considerations and procedural recommendations. Psychol Bull (2006) 132:443–76. doi:10.1037/0033-2909.132.3.443
30. Field M, Munafo MR, Franken IHA. A meta-analytic investigation of the relationship between attentional bias and subjective craving in substance abuse. Psychol Bull (2009) 135:589–607. doi:10.1037/a0015843
31. Hester R, Garavan H. Neural mechanisms underlying drug-related cue distraction in active cocaine users. Pharmacol Biochem Behav (2009) 93:270–7. doi:10.1016/j.pbb.2008.12.009
32. Luijten M, Veltman DJ, den Brink WV, Hester R, Field M, Smits M, et al. Neurobiological substrate of smoking-related attentional bias. Neuroimage (2011) 54:2374–81. doi:10.1016/j.neuroimage.2010.09.064
33. Luijten M, Veltman DJ, Hester R, Smits M, Pepplinkhuizen L, Franken IHA. Brain activation associated with attentional bias in smokers is modulated by a dopamine antagonist. Neuropsychopharmacology (2012) 37(13):2772–9. doi:10.1038/npp.2012.143
34. Nestor L, McCabe E, Jones J, Clancy L, Garavan H. Differences in “bottom-up” and “top-down” neural activity in current and former cigarette smokers: evidence for neural substrates which may promote nicotine abstinence through increased cognitive control. Neuroimage (2011) 56:2258–75. doi:10.1016/j.neuroimage.2011.03.054
35. Vollstädt-Klein S, Loeber S, Richter A, Kirsch M, Bach P, von der Goltz C, et al. Validating incentive salience with functional magnetic resonance imaging: association between mesolimbic cue reactivity and attentional bias in alcohol-dependent patients. Addict Biol (2012) 17:807–16. doi:10.1111/j.1369-1600.2011.00352.x
36. Janes AC, Pizzagalli DA, Richardt S, deB Frederick B, Chuzi S, Pachas G, et al. Brain reactivity to smoking cues prior to smoking cessation predicts ability to maintain tobacco abstinence. Biol Psychiatry (2010) 67:722–9. doi:10.1016/j.biopsych.2009.12.034
37. Janes AC, Pizzagalli DA, Richardt S, deB Frederick B, Holmes AJ, Sousa J, et al. Neural substrates of attentional bias for smoking-related cues: an fMRI study. Neuropsychopharmacology (2010) 35:2339–45. doi:10.1038/npp.2010.103
38. Goldstein RZ, Volkow ND. Drug addiction and its underlying neurobiological basis: neuroimaging evidence for the involvement of the frontal cortex. Am J Psychiatry (2002) 159:1642–52. doi:10.1176/appi.ajp.159.10.1642
39. Garavan H, Hester R. The role of cognitive control in cocaine dependence. Neuropsychol Rev (2007) 17:337–45. doi:10.1007/s11065-007-9034-x
40. Hester R, Garavan H. Executive dysfunction in cocaine addiction. Evidence for discordant frontal, cingulate, and cerebellar activity. J Neurosci (2004) 24:11017–22. doi:10.1523/JNEUROSCI.3321-04.2004
41. Luijten M, Machielsen MWJ, Veltman DJ, Hester R, de Haan L, Franken IHA. A systematic review of ERP and fMRI studies investigating inhibitory control and error-processing in substance dependence and behavioral addictions. J Psychiatry Neurosci (2013). doi:10.1503/jpn.130052. [Epub ahead of print].
42. Field M, Marhe R, Franken IHA. The clinical relevance of attentional bias in substance use disorders. CNS Spectr (2013). doi:10.1017/S1092852913000321. [Epub ahead of print].
43. Marhe R, Luijten M, van de Wetering BJM, Smits M, Franken IHA. Individual differences in anterior cingulate activation associated with attentional bias predicts cocaine use after treatment. Neuropsychopharmacology (2013) 38(6):1085–93. doi:10.1038/npp.2013.7
44. Kosten TR, Scanley BE, Tucker KA, Oliveto A, Prince C, Sinha R, et al. Cue-induced brain activity changes and relapse in cocaine-dependent patients. Neuropsychopharmacology (2006) 31:644–50. doi:10.1038/sj.npp.1300851
45. Beck A, Wüstenberg T, Genauck A, Wrase J, Schlagenhauf F, Smolka MN, et al. Effect of brain structure, brain function, and brain connectivity on relapse in alcohol-dependent patients. Arch Gen Psychiatry (2012) 69(8):842–53. doi:10.1001/archgenpsychiatry.2011.2026
46. Heinz A, Wrase J, Kahnt T, Beck A, Bromand Z, Grüsser SM, et al. Brain activation elicited by affectively positive stimuli is associated with a lower risk of relapse in detoxified alcoholic subjects. Alcohol Clin Exp Res (2007) 31(7):1138–47. doi:10.1111/j.1530-0277.2007.00406.x
47. Seeley WW, Menon V, Schatzberg AF, Keller J, Glover GH, Kenna H, et al. Dissociable intrinsic connectivity networks for salience processing and executive control. J Neurosci (2007) 27:2349–56. doi:10.1523/JNEUROSCI.5587-06.2007
48. Botvinick MM, Cohen JD, Carter CS. Conflict monitoring and anterior cingulate cortex: an update. Trends Cogn Sci (2004) 8:539–46. doi:10.1016/j.tics.2004.10.003
49. Kerns JG, Cohen JD, MacDonald AW III, Cho RY, Stenger VA, Carter CS. Anterior cingulate conflict monitoring and adjustments in control. Science (2004) 303:1023–6. doi:10.1126/science.1089910
50. Egner T, Etkin A, Gale S, Hirsch J. Dissociable neural systems resolve conflict from emotional versus nonemotional distracters. Cereb Cortex (2008) 18:1475–84. doi:10.1093/cercor/bhm179
51. Garavan H, Weierstall K. The neurobiology of reward and cognitive control systems and their role in incentivizing health behavior. Prev Med (2012) 55(Suppl):S17–23. doi:10.1016/j.ypmed.2012.05.018
52. Sofuoglu M, Devito EE, Waters AJ, Carroll KM. Cognitive enhancement as a treatment for drug addictions. Neuropharmacology (2013) 64:453–63. doi:10.1016/j.neuropharm.2012.06.021
53. Paulus MP, Tapert SF, Schuckit MA. Neural activation patterns of methamphetamine-dependent subjects during decision making predict relapse. Arch Gen Psychiatry (2005) 62:761–8. doi:10.1001/archpsyc.62.7.761
54. Luo X, Zhang S, Hu S, Bednarski SR, Erdman E, Farr OM, et al. Error processing and gender-shared and -specific neural predictors of relapse in cocaine dependence. Brain (2013) 136:1231–44. doi:10.1093/brain/awt040
55. Marhe R, Franken IHA. Error-related brain activity as a biomarker for cocaine relapse. Neuropsychopharmacology (2014) 39(1):241. doi:10.1038/npp.2013.245
56. Hart H, Chantiluke K, Cubillo AI, Smith AB, Simmons A, Brammer MJ, et al. Pattern classification of response inhibition in ADHD: toward the development of neurobiological markers for ADHD. Hum Brain Mapp (2013). doi:10.1002/hbm.22386. [Epub ahead of print].
57. Hajcak G. What we’ve learned from our mistakes: insights from error-related brain activity. Curr Dir Psychol Sci (2012) 21:101–6. doi:10.1177/0963721412436809
58. Hoffmann S, Falkenstein M. Predictive information processing in the brain: errors and response monitoring. Int J Psychophysiol (2012) 83(2):208–12. doi:10.1016/j.ijpsycho.2011.11.015
59. Houben K, Wiers RW, Jansen A. Getting a grip on drinking behavior. Psychol Sci (2011) 22(7):968–75. doi:10.1177/0956797611412392
60. Li X, Hartwell KJ, Owens M, Lematty T, Borckardt JJ, Hanlon CA, et al. Repetitive transcranial magnetic stimulation of the dorsolateral prefrontal cortex reduces nicotine cue craving. Biol Psychiatry (2013) 73(8):714–20. doi:10.1016/j.biopsych.2013.01.003
61. Connolly CG, Foxe JJ, Nierenberg J, Shpaner M, Garavan H. The neurobiology of cognitive control in successful cocaine abstinence. Drug Alcohol Depend (2012) 121:45–53. doi:10.1016/j.drugalcdep.2011.08.007
Keywords: neurocognitive processes, addiction, substance relapse, clinical relevance, attentional bias, cognitive control
Citation: Marhe R, Luijten M and Franken IHA (2014) The clinical relevance of neurocognitive measures in addiction. Front. Psychiatry 4:185. doi: 10.3389/fpsyt.2013.00185
Received: 10 September 2013; Accepted: 23 December 2013;
Published online: 10 January 2014.
Edited by:
Filippo Passetti, University of Cambridge, UKReviewed by:
Agnes J. Jasinska, National Institute on Drug Abuse, USALuigi Janiri, Università Cattolica del Sacro Cuore, Italy
Frank Ryan, Imperial College London, UK
Copyright: © 2014 Marhe, Luijten and Franken. This is an open-access article distributed under the terms of the Creative Commons Attribution License (CC BY). The use, distribution or reproduction in other forums is permitted, provided the original author(s) or licensor are credited and that the original publication in this journal is cited, in accordance with accepted academic practice. No use, distribution or reproduction is permitted which does not comply with these terms.
*Correspondence: Reshmi Marhe, Institute of Psychology, Erasmus University Rotterdam, P.O. Box 1738, 3000 DR Rotterdam, Netherlands e-mail:ci5tYXJoZUBkZWJhc2N1bGUuY29t