- Faculty of Humanities, Social Sciences, and Theology, Friedrich-Alexander-Universität Erlangen-Nürnberg, Erlangen, Germany
Political decision-making is often riddled with uncertainties, largely due to the complexities and fluid nature of contemporary societies, which make it difficult to predict the consequences of political decisions. Despite these challenges, political leaders cannot shy away from decision-making, even when faced with overwhelming uncertainties. Thankfully, there are tools that can help them manage these uncertainties and support their decisions. Among these tools, Artificial Intelligence (AI) has recently emerged. AI-systems promise to efficiently analyze complex situations, pinpoint critical factors, and thus reduce some of the prevailing uncertainties. Furthermore, some of them have the power to carry out in-depth simulations with varying parameters, predicting the consequences of various political decisions, and thereby providing new certainties. With these capabilities, AI-systems prove to be a valuable tool for supporting political decision-making. However, using such technologies for certainty purposes in political decision-making contexts also presents several challenges—and if these challenges are not addressed, the integration of AI in political decision-making could lead to adverse consequences. This paper seeks to identify these challenges through analyses of existing literature, conceptual considerations, and political-ethical-philosophical reasoning. The aim is to pave the way for proactively addressing these issues, facilitating the responsible use of AI for managing uncertainty and supporting political decision-making. The key challenges identified and discussed in this paper include: (1) potential algorithmic biases, (2) false illusions of certainty, (3) presumptions that there is no alternative to AI proposals, which can quickly lead to technocratic scenarios, and (4) concerns regarding human control.
1 Introduction
Political decision-making is a complex endeavor, particularly in representative democracies, where elected officials make decisions on behalf of citizens and, unlike in authoritarian systems, remain accountable to the public. When political decision-makers make decisions that affect a large group of people, it is essential to anticipate their potential consequences as accurately as possible. Informed decision-making is crucial not only to prevent unforeseen complications but also to avoid producing unintended or even harmful outcomes. Yet, predicting the effects of political decisions in modern societies is extraordinarily difficult. Contemporary societies are both highly complex—comprising vast and interwoven economic, ecological, legal, and public health systems—and deeply dynamic, evolving in unpredictable ways. This combination of structural complexity and fluidity forces political decision-makers to operate within an environment of uncertainty, making their task increasingly intricate.
Yet, political decision-makers cannot simply avoid making decisions. At times, political choices must be made despite all uncertainties. To aid political leaders in their decision-making processes, they have access to a variety of tools designed to decrease existing uncertainties and acquire new insights that can facilitate their decisions. Recently, this toolkit has been enhanced with the addition of Artificial Intelligence (AI). Such AI-systems, with their self-learning capabilities and vast computational power, promise to diminish uncertainties by rapidly and precisely analyzing complex situations, identifying pertinent patterns better than any human ever could, and shedding light on previously opaque contexts. Furthermore, they also promise to generate new certainties by running in-depth simulations with varying parameters, thus predicting the likely outcomes of various decisions.
The potential of AI is undeniably compelling, and the prospect of employing AI for certainty purposes in political decision-making contexts is particularly alluring. However, a word of caution might be necessary. Insights from other fields, such as medicine (Morley et al., 2020; Topol, 2019; Tretter, 2024b; Tretter et al., 2023) or defense (Raska and Bitzinger, 2023; Tangredi and Galdorisi, 2021), reveal that AI-systems—especially those designed to support decision-making by mitigating uncertainty and fostering certainty—offer both substantial benefits and significant challenges. The primary goal of this paper is to identify the challenges associated with deploying such AI-systems in political decision-making contexts. The key question is: what challenges arise from the use of AI-systems for certainty purposes in the context of political decision-making? By addressing this question, the paper aims to contribute to what—as outlined below—is still a relatively underexplored area of research while also highlighting emerging risks. These risks need to be recognized and mitigated to ensure that AI can be utilized effectively and responsibly for certainty purposes in political decision-making contexts, without triggering unintended or excessive negative consequences (Zuiderwijk et al., 2021). However, the development of a concrete strategy or specific recommendations, measures, and “guardrails” (Gasser and Mayer-Schönberger, 2024) is beyond the scope of this paper—only a few preliminary suggestions are outlined in the Discussion section.
With its focus on AI-systems for reducing uncertainties and supporting political decision-making processes, this paper, as illustrated in Figure 1, is situated at the intersection of three research fields. The first research field examines the intersection of AI and uncertainty. It centers on discussions about how AI-systems, which typically rely on clear inputs and unambiguous datasets, can handle uncertainty—for instance, which methods can make ambiguous inputs and unclear datasets usable (Chaki, 2023; Li and Du, 2017)—and about how AI-systems, through their analytical and simulation capabilities, can help reduce or even overcome existing uncertainties (Arend, 2024; Rodriguez, 2023). The second research field explores the intersection of AI and political decision-making. Drawing on broader discourses about the use of AI in political contexts and decision support in other domains, it examines the opportunities, possibilities, and risks of employing AI for political decision-making (Fitria Fatimah, 2024; Hudson, 2018; McEvoy, 2019; Vera Hoyos and Cárdenas Marín, 2024). It also examines potential consequences of such AI use, utilizing concepts like “algorithmic democracy” (García-Marzá and Calvo, 2024), develops legal and ethical frameworks for the lawful and responsible use of such systems (Fitria Fatimah, 2024; Kuziemski and Misuraca, 2020), and investigates public reactions to the potential deployment of prototypical AI-systems in political decision-making contexts (Starke and Lünich, 2020). The third research field delves into the relationship between political decision-making and uncertainty. Building on broader discussions about the interplay of uncertainty and decision-making (Ceberio and Kreinovich, 2023a,b; Yoe, 2019a,b), it investigates specific uncertainties arising in political decision-making processes, how these can be addressed and minimized (Arend, 2024; Boyd, 2019; Kirshner, 2022; Zahariadis, 2003), and, conversely, how targeted political decisions can reduce prevailing (societal) uncertainties (Akilli and Gunes, 2023a,b; Sarkar et al., 2024; Ventriss, 2021). While each of these research fields has been extensively studied, the overlap of AI, uncertainty, and political decision-making remains relatively unexplored—a gap this paper aims to fill.
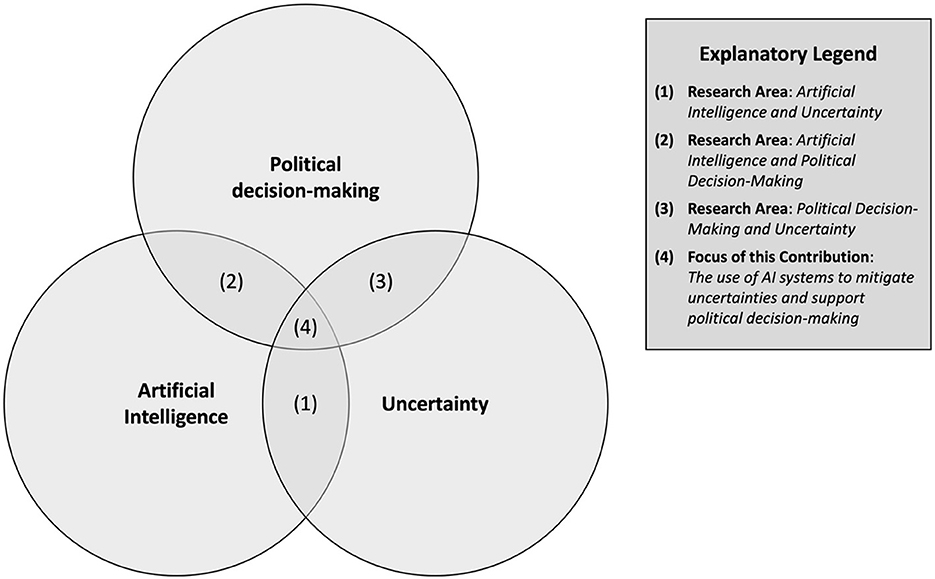
Figure 1. Illustration of this paper's focus at the intersection of three discourses concerning the relationships between (1) Artificial Intelligence and uncertainty, (2) Artificial Intelligence and political decision-making, and (3) political decision-making and uncertainty (created by the author).
2 Methodology
To explore the challenges that may arise from the use of AI-systems for certainty purposes in political decision-making, this study employs an approach that integrates reviews of existing literature, conceptual considerations, and political-ethical-philosophical reasoning, supported by an example. This multi-perspective strategy not only grounds the discussion in theory but also demonstrates its relevance in practice, effectively bridging the divide between abstract debates on uncertainty, AI ethics, and political theory, and the tangible challenges faced in high-stakes political contexts, exemplified through the Covid-19 pandemic.
The first step involves clarifying key terms by defining my understanding of “uncertainty” and “certainty” and examining their roles in political decision-making. Before developing my own conceptualization of these terms, I begin by analyzing how “uncertainty” is discussed in academic discourse. Since “uncertainty” plays a more prominent role in scholarly debates than “certainty” and is often granted conceptual primacy in academic discussions, meaning that “certainty” is often conceptualized as a counterpart to “uncertainty,” this study first explores the different ways in which “uncertainty” has been understood and theorized. Building on this foundation, I then present my own understanding of “uncertainty,” which then allows me to also develop a previse understanding of “certainty.”
The conceptual analysis maps the range of interpretations of uncertainty by drawing on key books and edited volumes published in the past decade, specifically focusing on works that explicitly engage with the concept of “uncertainty” and include the term in their titles. These publications were identified through database searches on Google Books and WorldCat using the keyword “uncertainty” as a standalone search term, filtering for works published within the last 10 years—more precisely, from January 1, 2014, to December 26, 2024, the date on which the search was conducted, and the sample was finished.1
While this overview does not aim to be fully representative, exhaustive, or precisely reproducible, it provides a critical foundation for developing my own conceptualizations of “uncertainty” and, subsequently, “certainty.” Following these definitions, I will explore the relationship between uncertainty and political decision-making in greater depth. This involves first outlining key perspectives from previous debates on decision-making and uncertainty, and then developing a detailed conceptual framework to identify the specific forms of uncertainty encountered in political decision-making contexts and their precise impact on these processes.
Following this in-depth presentation of my terminological framework and the relationship between uncertainty and political decision-making, I examine how AI-systems can support political decision-making by reducing existing uncertainties and generating new certainties, with the Covid-19 pandemic serving as a supporting example. While the Covid-19 pandemic stands out due to its global scope, its relatively sudden emergence and its rapidly evolving nature as well as the ethical dilemmas it raised, such as triage decisions, it created a situation of uncertainty for political decision-making that, despite the specific factors contributing to this uncertainty, can be considered an archetypal example of uncertain political decision-making contexts. For this reason, the Covid-19 pandemic can be used as a supporting example that, as Ferrara (2008) suggests, might help ground previous theoretical reflections and enhance their validity. This analysis draws on conceptual reasoning and contemporary debates in political theory and AI ethics, synthesizing theoretical insights with the practical challenges posed by the pandemic. Specifically, I explore how AI-systems could have been used to clarify existing uncertainties, forecast potential outcomes, and provide recommendations, thereby assisting political leaders during this complex crisis. From this, I identify and elaborate on four key challenges associated with employing AI for certainty purposes in political decision-making: (1) algorithmic bias, (2) the creation of false illusions of certainty, (3) the perception of no viable alternatives, which can lead to technocratic scenarios, and (4) concerns about maintaining human control.
In the subsequent Discussion section, I provide initial suggestions on how to effectively and responsibly use AI-systems in political decision-making. These suggestions are based on an ethical assessment grounded in a wide reflective equilibrium (Dabrock, 2012; Daniels, 2011). This approach ensures a comprehensive consideration of normative issues such as human autonomy, democratic accountability, and the risks of algorithmic overreach. I then address the limitations of these considerations and summarize the insights from this study in a Conclusion.
3 Uncertainty as a challenge for political decision-making
Before considering the use of AI-systems for certainty purposes in political decision-making contexts, there is a need to clarify fundamental terminologies and assumptions. This includes the concepts of uncertainty and certainty, as well as the effects of uncertainty on political decision-making.
3.1 Diversity in the understanding of uncertainty
The exact meaning of uncertainty is unclear (Arend, 2024; Zinn, 2008). There are numerous interpretations of how uncertainty is to be understood (Halpern, 2017). This diversity is evidenced by the vast number of publications on the topic over the past decade, which are so extensive that one might even speak of a “hype” surrounding the concept of uncertainty. These publications demonstrate that understandings of uncertainty are heavily influenced by the perspective from which uncertainty is analyzed (Olofsson and Zinn, 2019). Depending on the format and the disciplinary perspective of these reflections, entirely different causes, outcomes, and understandings of uncertainty are identified and presented. This section aims to provide insights into the current landscape of debates on the topic of uncertainty, structured by the categories of formats, disciplines, causes, outcomes, and understandings—in order to serve as a theoretical background for the development of this paper's distinct understanding of uncertainty in the next section.
At the outset, it becomes clear that uncertainty is explored and discussed across a range of formats. A large proportion of the analyzed publications fall into the category of “scientific studies” (Ceberio and Kreinovich, 2023b). These studies employ established scientific methods to investigate uncertainty, focusing—depending on the discipline—on its consequences or on the novel perspectives it offers. Beyond scientific analyses, there is a wealth of normative analyses and governance approaches. These works typically draw on established methodologies from ethics, political science, and other normative disciplines to propose actionable recommendations and regulations aimed at reducing existing uncertainties or preparing for the emergence of uncertain events. Lastly, a substantial body of publications might be classified as “guidebooks” or even “self-help literature.” These are directed either at individuals or specific professional groups, offering advice on how to navigate uncertainties. Such advice is derived from a mix of scientific insights, personal experiences, and traditional wisdom (Bateman et al., 2023; Fields, 2014; Plinio and Smith, 2019; Seppälä, 2024). Unlike normative analyses and governance approaches, guidebooks and self-help literature are less academically rigorous, target a broader general audience rather than specialized experts, and often provide everyday, practical guidance rather than politically, societally, or institutionally focused recommendations. Naturally, there are also numerous hybrid formats that bridge these three categories. For instance, some publications primarily conduct scientific analyses but conclude with normative perspectives, while others blend self-help advice with elements of governance-oriented frameworks (Poloz, 2022).
Publications on uncertainty span a wide array of disciplines. For instance, in the field of politics, international relations, and geography, scholars explore how geographical, economic, and financial uncertainties influence global politics (Katzenstein, 2022), international relations between nations (Belloni et al., 2019), and the shaping of geographical features and borders (Ricci, 2024). Further, uncertainty is also used as a framework to analyze global events, such as the Covid-19 pandemic, at both national (Murilla et al., 2023) and international levels (Akilli and Gunes, 2023a,b), to provide fresh perspectives on historical developments (Bergmane, 2023), or to examine contemporary conflicts (Edelstein, 2017; Kirshner, 2022). In the disciplinary field of finance, economy, and industry, uncertainty is seen as a foundational condition (Hüttche, 2023), closely tied to economic dynamics and financial market volatility (Poloz, 2022). Studies investigate the societal impacts of economic fluctuations (Ben Ameur et al., 2023) and propose strategies to mitigate crises (Komporozos-Athanasiou, 2022)—for instance through industrial optimizations (Albornoz et al., 2024), insurances (van der Heide, 2023), or the further establishment of commons (Obeng-Odoom, 2021). More economic-historical approaches explore how societies have managed economic risks and financial uncertainties in the past (Szpiro, 2020). The field of management studies—often drawing on pedagogical, philosophical, or game theory approaches—focusses on the skills and resources leaders need to navigate uncertainties (Furr and Harmon Furr, 2022), and to make complex decisions (Beghetto and Jaeger, 2022) even in “times of crisis” (Johnson, 2018). These studies also explore the resources leaders can rely on (Datta and Kutzewski, 2023) and the methods that can aid their decision-making (Abu el Ata and Schmandt, 2016; Oriesek and Schwarz, 2021; Yoe, 2019b). In the field of sociology and ethnology, research investigates how different forms of uncertainty—such as financial or job-related uncertainty—affect various groups of people (Smagacz-Poziemska et al., 2020) and how these differences impact society as a whole (Chanes and Rees Jones, 2022), regional development (Smagacz-Poziemska et al., 2020), as well as international and domestic migration and societal inclusion and exclusion (Thiel et al., 2023). These studies also examine how individuals and groups, such as adolescents (Hardon, 2020), or specific professional groups like academics (Mulligan and Danaher, 2021), navigate uncertainties and which coping strategies they develop (Brown and Zinn, 2022; Cook, 2018; Morduch and Schneider, 2017). In medicine and healthcare, uncertainty is increasingly recognized as an omnipresent factor, from diagnosis to treatment to subsequent monitoring (Hatch, 2016), and one that healthcare professionals must consider to provide quality patient care (Manski, 2019) and to be considered “good doctors” (Brigham and Johns, 2020). Consequently, frameworks are being developed to better integrate an awareness of uncertainty and methods to deal with it into all areas of medicine and healthcare (Chiffi, 2021; Han, 2021), including psychology (Kulesza and Doliński, 2023), pharmacology (LaCaze and Osimani, 2020), and military medicine (Messelken and Winkler, 2021). In the field of mathematics and statistics, the focus is on “how to think about uncertainty” (Elliott, 2021)—specifically, on models that represent uncertainty (Guy et al., 2015; Liu, 2015; Stewart, 2019) and methods for quantifying it (Ghanem et al., 2017; Hu, 2023; Souza de Cursi, 2024). These mathematical and statistical approaches are frequently adapted and expanded in technical and information sciences. For example, researchers investigate how to design algorithms capable of making optimal decisions under uncertain conditions and with ambiguous data sets (Chaki, 2023; Dimitrakakis and Ortner, 2022), or how algorithms (Kochenderfer et al., 2020), data analysis (Rodriguez, 2023) and predictive simulations (Samimian-Darash, 2022) can be utilized to support research and development, planning, or decision-making in various contexts. The field of philosophy, ethics, and theology engages with uncertainty in distinct ways. Epistemological inquiries focus on the nature of uncertainty and its distinction from other forms of ignorance and non-knowledge (Bandyopadhyay et al., 2016; Garvey et al., 2022). Ethical discussions examine the intersections of uncertainty, morality (MacAskill et al., 2020) and (in)justice (Smagacz-Poziemska et al., 2020), as well as questions of what a responsible approach to uncertainty might entail (Aspers, 2024; Code, 2020; Jorrit Hasselaar, 2023). Rather philosophical-historical studies consider how uncertainty was addressed in former times, e.g., ancient Greece (Hubler, 2021), or how it has fueled scientific progress (Kampourakis and McCain, 2019; Tauber, 2022). Theological inquiries delve into the connection between human uncertainty and the search for God (Carrón, 2020) and explore how uncertainty creates space for religion and theological reflection (Caputo, 2019; de Kock, 2024; Irwin, 2019). Last but not least, into the field of art and literary studies, scholars examine how uncertainty is portrayed in various literary texts and genres (Høeg, 2021; Williams, 2024) and use uncertainty as a lens to gain new insights into artists and their works (McAbee, 2020).
Depending on the formats and respective disciplines, different causes of uncertainty are identified, and various outcomes are expected (Arend, 2024). Commonly identified causes include the complexity and dynamism of society as a whole or of specific systems like the economy or financial markets. These factors often render future developments insufficiently predictable or assessable and therefore uncertain (Arend, 2024). Unexpected events, such as the Covid-19 pandemic (Jasanoff, 2021), or potential future crises, such as new epidemics or pandemics (Jasanoff, 2021), also contribute to uncertainty. Even well-known developments, such as climate change (Jorrit Hasselaar, 2023; Sarkar et al., 2024), can induce uncertainty by raising pressing questions about the future and further directions of global action. Additionally, the complexity of the human organism can be a source of uncertainty, particularly when special circumstances—like illness (Mishel, 1988) or pregnancy (Tretter, 2024b)—or medications raise questions about a person's health trajectory and its impact on their life and quality of life (Hatch, 2016). Given the plethora of causes, some argue that uncertainty is “a fundamental—and unavoidable—feature of daily life” (Halpern, 2017, p. 1) and an inherent part of the “human condition” (Irwin, 2019; Klinke, 2024). Uncertainty, however, not only has diverse causes but also varied outcomes—both negative and positive (Arend, 2024). On the negative side, uncertainty can evoke fear (Abu el Ata and Schmandt, 2016) or confusion (Klinke, 2024), which, at its worst, can paralyze individuals, preventing them from making decisions. On the positive side, uncertainty can be a catalyst for productive activity (Furr and Harmon Furr, 2022), motivating individuals and groups to overcome it (Arend, 2024), seek new certainties (Souza de Cursi, 2024), or develop safeguards against its (negative) effects (Furr and Harmon Furr, 2022). That's why uncertainty can also be seen as a driver of scientific progress (Kampourakis and McCain, 2019), a spark for innovation and creativity (Arend, 2024; Kay and King, 2021), and as something that can foster personal growth (Furr and Harmon Furr, 2022), and, as Jonathan Fields describes it, become “fuel for brilliance” (Fields, 2014). In addition to these productive outcomes, uncertainty can also lead to numerous coping mechanisms, some of which healthier than others (Hardon, 2020). It can shape attitudes and virtues, such as resilience (Walker, 2019) and patience (Janeja and Bandak, 2018), humility (Jasanoff, 2021) and hope (Jorrit Hasselaar, 2023). However, it may also provoke feelings of hopelessness (Jorrit Hasselaar, 2023). Ultimately, uncertainty may inspire a more realistic outlook on the future (Klinke, 2024), and challenge illusions of infallibility and control (Jasanoff, 2021; Motet and Bieder, 2017).
The diverse formats, disciplines, causes, and outcomes of uncertainty have given rise to a wide range of understandings of uncertainty. In more consequentialist approaches, uncertainty is often framed as a crisis-like phenomenon (Ben Ameur et al., 2023), disrupting current processes and destabilizing established social orders (Datta and Kutzewski, 2023). That's why uncertainty is frequently viewed as a security risk (Motet and Bieder, 2017). In contrast, other perspectives emphasize the ubiquity of uncertainty, arguing that societal orders are inherently vulnerable and the future perpetually uncertain. From this vantage point, uncertainty is seen as a fundamental condition of society (Ventriss, 2021) or even as a defining feature of our era—the so-called “age of uncertainty” (Murphy-Greene, 2022; Obeng-Odoom, 2021). These sociological interpretations are paralleled at the individual level. Here, uncertainty is often described as a baseline condition that complicates life and raises existential questions about the future (Motet and Bieder, 2017; Spiegelthaler, 2024). A second set of understandings conceptualizes uncertainty as a deficiency. It is frequently understood as a lack of data or information (Liu, 2015). From this perspective, uncertainty becomes a puzzle to be solved through mathematical or statistical methods (Hu, 2023), particularly Bayesian approaches (Halpern, 2017; Souza de Cursi, 2024), or a challenge to be addressed with technical solutions (Rodriguez, 2023; Samimian-Darash, 2022). This group of understandings also includes frameworks that contrast uncertainty with risk (Zinn, 2020), defining risk as the quantification of uncertainty—transforming incomplete information into probabilities (Alvarez et al., 2018; Kay and King, 2021). Critics, however, often caution that uncertainty cannot be fully reduced to numbers and probabilities (Kay and King, 2021). Some deconstructive approaches go further, arguing that if uncertainty, if merely a deficiency that can be quantified, in the strict sense, does not exist (Garvey et al., 2022).2
3.2 How uncertainty is understood in this paper
Against the backdrop of the diverse understandings of uncertainty outlined above, I will now develop an understanding of uncertainty that will be guiding this paper. This understanding integrates key elements from the previous discussions, tailoring them to meet the specific demands of the paper's analysis.
Building on established understandings of uncertainty as a lack of data and information, this paper adopts a conceptualization rooted in the work of Michael Smithson, a pioneer in uncertainty and ignorance studies. Here, uncertainty is defined as an epistemic state of lacking knowledge (Smithson, 1989). This lack can stem from various causes, including insufficient information, or data that is flawed, imprecise, or fundamentally incorrect (Smithson, 1989). Unlike simple ignorance, which refers to not knowing something and being unaware of one's ignorance (Gross, 2007), uncertainty is understood as involving an awareness of one's own lack of knowledge—which puts this paper's understanding of uncertainty in contrast to, for instance, Knightian interpretations, where individuals are only considered to be in a state of uncertainty when they are aware of their informational deficit (Knight, 1921). This deficiency-based understanding of uncertainty is pragmatically extended in light of earlier consequentialist perspectives. When individuals are aware of their lack of information, as highlighted in several ethical (Johnson, 2022) and pragmatic (Dewey, 1929) reflections on the concept, this kind of uncertainty has practical consequences. The sense of insufficient information about a current situation and the awareness that one cannot accurately anticipate the outcomes of one's decisions often prove to be barriers to decision-making (Dewey, 1929). Consequently, this paper defines uncertainty as an epistemic state of lacking knowledge that complicates decision-making (Tretter et al., 2023).
Certainty is considered the conceptual counterpart to uncertainty. However, the latter is not merely understood as the absence of uncertainty, as the absence of uncertainty could also be achieved through ignorance, i.e., not recognizing one's knowledge gaps (Gross, 2007). Instead, certainty is conceptualized as an epistemic state of sufficient knowledge, i.e., a state in which a person possesses enough reliable information and insights. Exactly how much knowledge qualifies as “sufficient” cannot be universally determined; it depends on the specific situation and the parties involved. However, as a general rule of thumb, knowledge can be considered sufficient when it provides an individual with a comprehensive understanding of a situation, enabling reasonably reliable—though not infallible—predictions of the consequences of potential actions (Dewey, 1929). Such sufficient knowledge makes decision-making easier as various options and their implications can be more precisely weighed against each other, facilitating an informed choice. Consequently, in this paper, certainty is defined as an epistemic and practical state wherein sufficient information is available to comprehensively grasp a situation and adequately anticipate the outcomes of one's decisions, thereby facilitating decision-making (Tretter et al., 2023).
Uncertainty and certainty can be viewed as the poles of a continuum (Caron, 2013; Rubin, 2010). At one end lies “absolute uncertainty,” characterized by a complete lack of situational awareness and an utter inability to estimate the consequences of one's actions and decisions. At the other end is the aforementioned “absolute certainty,” defined by full knowledge of a situation and the ability to predict the consequences of decisions and actions with precise accuracy (see Figure 2). It is important to emphasize that neither extreme is attainable for humans (Klinke, 2024), as they always possess some degree of knowledge but are never omniscient, and can often roughly anticipate the outcomes of their actions, though their predictions are never infallible (Hamilton, 2014; Wittgenstein, 1969). Individuals typically operate within the middle range of this spectrum, experiencing varying degrees of relative uncertainty and certainty (Tretter, 2023).
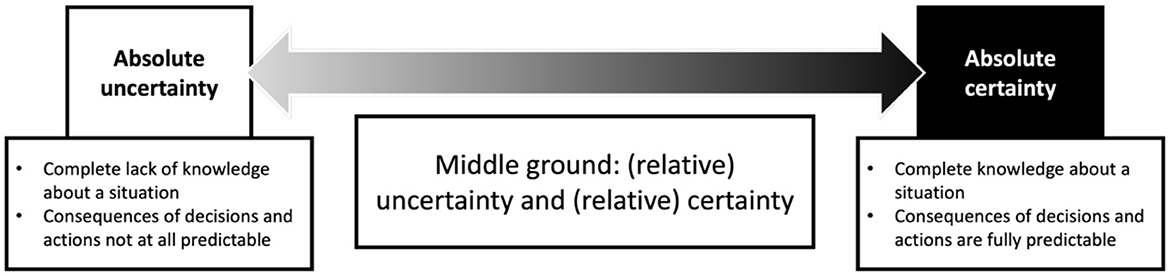
Figure 2. Illustration of the continuum between absolute uncertainty and absolute certainty with the middle ground of (relative) uncertainty and (relative) certainty (created by the author).
Since both absolute uncertainty and absolute certainty are considered unattainable, this paper uses these terms as relative concepts. In other words, when “uncertainty” is mentioned in the following sections, it doesn't mean a total lack of knowledge or the inability to predict outcomes, but rather that a situation is relatively unclear and unpredictable, making it difficult or imprecise to estimate the consequences of decisions and actions. Similarly, “certainty” does not refer to absolute knowledge or 100% accurate predictions, but to a state in which there is enough information to make reasonably accurate estimates and predictions about the consequences of one's actions and decisions.
3.3 Conceptual considerations on uncertainty and political decision-making
In the previous section, I presented how uncertainty is understood in this paper, drawing on an overview of various interpretations of the concept. This understanding is characterized by its epistemic-practical dual nature, which broadly highlights that the central epistemic state of lacking knowledge generally hinders decision-making. In this section, I aim to further refine the analysis. The focus will be on identifying the distinct ways in which uncertainty manifests in political decision-making contexts and clarifying how it impedes these processes. To accomplish this, I will first present key distinctions from existing scholarly discussions on the relationship between uncertainty and decision-making in general. These distinctions will then be used as a foundation for a more precise exploration of how uncertainty specifically impacts political decision-making.
The interplay between uncertainty and decision-making—both in general and specifically within political contexts—and the question of how existing uncertainties can be mitigated or at least quantified through scientific or technological methods to address their associated challenges has long been a subject of scholarly inquiry (Schomberg, 1993; Zahariadis, 2003). Over time, these debates have evolved into highly differentiated discussions. Within the diverse discourse on uncertainty and political decision-making, several studies focus on how political decision-makers perceive different types of uncertainty, how these perceptions influence their confidence and their assessment of their own decision-making capabilities (Friedman and Zeckhauser, 2018), and the strategies they employ to manage such uncertainties (Heazle, 2012). Research further explores how political decision-makers' acknowledgments of different kinds of uncertainty—such as external uncertainty, arising from the inherent complexity of situations, or internal uncertainty, reflecting ignorance or a lack of confidence in their own decisions—affects public perceptions of them (Løhre and Halvor Teigen, 2023). In addition to these social scientific approaches, more technical analyses compare different models for decision-making in political contexts under uncertainty, such as Robust Decision Making (Workman et al., 2020) and Decision Making under Deep Uncertainty (Stanton and Roelich, 2021), evaluating the strengths and limitations of each model in facilitating the decision-making process.
While all these debates are important and provide valuable insights, for the argument of this paper, studies that identify and differentiate various forms of uncertainty and their impacts on decision-making—not only in political contexts—are particularly relevant. One influential early contribution comes from Ove Hansson (1996), who distinguishes four types of uncertainty that can arise in decision-making processes: (1) uncertainty of demarcation, which occurs when the available options are not clearly defined; (2) uncertainty of consequences, which refers to a lack of clarity about the outcomes of a decision; (3) uncertainty of reliance, which arises when it is unclear whether or to what extent the available information can be trusted; and (4) uncertainty of values, which emerges when it is unclear which values decision-makers are guided by Ove Hansson (1996). A comparable but more recent contribution comes from Dewulf and Biesbroek (2018) who present a novel analytical framework comprising nine different types of uncertainty. This framework is built on their identification of three distinct “natures” of uncertainty: (1) epistemic uncertainty, stemming from insufficient information about a situation; (2) ontological uncertainty, rooted in the inherent complexity of situations or systems that renders reliable predictions about future developments impossible even when information is sufficient; and (3) ambiguity, which arises from conflicts between different, irreconcilable perspectives or analytical frames. Additionally, they identify three “objects” of uncertainty in decision-making contexts: (i) substantive uncertainty, which refers to uncertainty regarding the factual and problem-related circumstances at hand; (ii) strategic uncertainty, related to the unpredictability of the decisions, actions, or reactions of other key actors; and (iii) institutional uncertainty, which pertains to the context of decision-making and reflects uncertainty about which formal and informal rules must be observed. By analyzing the intersections of these three natures and three objects, Dewulf and Biesbroek develop a comprehensive typology of nine types of uncertainty (Dewulf and Biesbroek, 2018).
These two frameworks are particularly well-suited for clarifying the uncertainties that can arise in political decision-making contexts. As briefly mentioned in the Introduction, uncertainty is highly prevalent in political decision-making, as assessing the potential outcomes of a political decision is incredibly challenging. One reason for this is the inherent structural complexity of modern societies (Kay and King, 2021; Klinke, 2024; Renn et al., 2011), which becomes especially evident through systems-theoretical perspectives. Analyzing modern societies from a systems-theoretical perspective reveals them as networks of interconnected “systems” (Luhmann, 2012). While these systems operate independently and follow their unique logics, they are not isolated; they interact and influence one another through structural linkages (Luhmann, 1995). And, as Bruno Latour might add, they tend to hybridize (Latour, 2005). Given that a single political decision can reverberate across multiple systems—each influencing the others—comprehensively understanding this complexity and predicting the consequences of such decisions becomes an immensely daunting, if not impossible, task (Luhmann, 2002). Following Dewulf and Biesbroek's (2018) framework, the uncertainty prevalent in political decision-making contexts can, in terms of its causes (or “natures”), be primarily categorized as ontological uncertainty, stemming from the inherent complexity of the situation, which prevents it from being fully understood. This may be further accompanied by epistemic uncertainty, where the information available about the situation is either insufficient or unreliable. Regarding its objectives, the uncertainty can be classified as substantive uncertainty, since it is primarily tied to the nature of the situation itself, and possibly as strategic uncertainty, since it is unclear how other actors will decide or act. Consequently, the uncertainty characteristic of political decision-making can be best described as an ontological-substantive uncertainty, rooted in the complexity and opacity of the situation itself, potentially supplemented by elements of ontological-strategic, epistemic-substantive, and epistemic-strategic uncertainty. According to Ove Hansson's (1996) framework, the uncertainty encountered in political decision-making contexts is best categorized as uncertainty of consequences, with possible aspects from uncertainty of reliance and uncertainty of demarcation.
Skillfully “navigating,” as Spiegelthaler (2024) would say, through this scenario is even more intricate when we factor in not just the structural complexity of society but also its inherent “liquidity.” As Zygmunt Bauman highlighted in several of his works (Bauman, 1999, 2000a, 2017), “modern societies” are permeated by various “acceleration dynamics” (Rosa, 2012). This manifests in the swift progression of knowledge, technological advancements, and societal shifts, which evolve so rapidly that keeping abreast becomes an ever-mounting challenge (Bauman, 2000a, 2017). It is as if the moment knowledge is produced, it is immediately rendered outdated. Consequently, as Bauman concluded, “living in the era of liquid modernity” (Bauman, 2000b) oftentimes feels like a ceaseless pursuit of these ever-advancing developments, constantly trying to catch up to their own pace (Bauman, 2007). In Dewulf and Biesbroek's (2018) framework, this temporal dimension amplifies the complexity and opacity of society, thereby heightening ontological uncertainty. Furthermore, as knowledge becomes obsolete at an accelerating pace, it exacerbates epistemic uncertainties. Similarly, in Ove Hansson's (1996) framework, this liquidity would contribute to greater uncertainty of consequences and uncertainty of reliance for the same reasons.
The combined forces of society's structural complexity and temporal liquidity make it exceedingly difficult, if not impossible, for political leaders to reliably grasp the (probable) outcomes of their decisions (Bauman, 2007). The consequence of this is that every political decision-making process inherently contains several fundamental uncertainties (von Ramin, 2022), including, at a minimum, the ones mentioned above. As explored by Zygmunt Bauman and Carlo Bordini in their joint study on the regulatory crisis, this uncertainty persists and is difficult to navigate, even with sustained endeavors in knowledge and democratic practices (Bauman and Bordini, 2014).
4 Using AI-systems for certainty purposes in political decision-making contexts
Having examined the concepts of uncertainty and certainty and their relevance to political decisions, this section shifts the focus to the potential role of AI-systems in contexts of political decision-making. I will begin by providing a brief overview of the ways AI-systems can be integrated into political decision-making processes, highlighting the opportunities they present. To ground these abstract considerations, I will then use the Covid-19 pandemic as a supporting example to illustrate and concretize these conceptual reflections. This involves outlining key uncertainties that emerged during the crisis, which posed significant challenges to political leaders and their decision-making processes globally.
In this context, I will explore how AI could have supported political decision-makers during the pandemic—not only by reducing uncertainties but also by creating new certainties through algorithm-based simulations, predictions, and recommendations. It is crucial to emphasize that the objective of this section is not to retrospectively critique specific decisions made during the pandemic using the benefit of hindsight. Instead, the aim is to shed light on the complexity of political decision-making during that time, the uncertainties leaders faced, and the potential benefits of integrating AI into political decision-making processes.
4.1 The potential uses of AI in political decision-making contexts
As political leaders cannot simply abstain from making political decisions (Rutter et al., 2020), especially in challenging situations where swift and decisive action is often required, decisions often have to be made amidst (multiple forms of) uncertainty (Kochenderfer, 2015). To navigate these challenges, policymakers have a plethora of tools at their disposal. Top-tier politicians can rely on personal advisors and scientific aides. They might also convene expert panels or engage external consultants, leveraging their specialized knowledge and insights. Moreover, they have the option of consulting public opinion through voter surveys, and more recently, there is also the possibility—and in some cases, even concrete plans or prototypes already in place (Hjaltalin and Sigurdarson, 2024; de Sousa et al., 2019; van Noordt and Misuraca, 2022)—to introduce AI tools into the decision-making process (Charles et al., 2022).
Even though the implementation of AI in political decision-making and governmental contexts overall is still approached with caution—which is a good thing, as it has become clear that not all governments (Nzobonimpa and Savard, 2023) and political decision-makers (Sandoval-Almazan et al., 2024) are ready to adopt these technologies—there are already several applications for AI in these contexts (van Noordt and Misuraca, 2022). These include its use in administration and budgeting (Cantarelli et al., 2023; Valle-Cruz et al., 2022), public service and governmental policy (Valle-Cruz et al., 2019; Valle-Cruz and Sandoval-Almazan, 2018; Wirtz et al., 2018). AI-systems promise to tackle even the most complex tasks in these areas or at least provide supportive assistance.
In the realm of political decision-making, one of the primary aims of specific, though still largely experimental AI-systems is to find the best solution for complex societal challenges. With their immense computational power and learning capacity, capturing the complexity of social dynamics, AI-systems promise to advance this process. They are expected to identify—faster and more efficient than any individual human or research team ever could—the critical parameters and interrelations that need to be considered and addressed (König and Wenzelburger, 2020), thereby mitigating ontological-substantive uncertainties. AI-systems might either directly convey these findings to political decision-makers (Pencheva et al., 2018), highlighting the crucial aspects they have to factor into their deliberations. Alternatively, AI-systems can further process this data, conducting meticulous real-time simulations to predict the outcomes of various interventions, legislative mandates, or guidelines (Valle-Cruz and Sandoval-Almazan, 2018), vividly illustrating the potential effects that different decisions might have on society, economy, or education, among others, effectively minimizing uncertainty of consequences. Finally, based on these analyses and predictions, some AI-systems have the capabilities to also draft solution proposals or legislative suggestions, or provide recommendations on which decisions might yield the most favorable outcomes, based on the available data and identified critical parameters. Beyond this, AI could also assist in the implementation of decisions, such as the enactment of laws, by designing efficient implementation strategies. Furthermore, it could further assist in retrospectively evaluating the impacts and effectiveness of past decisions (Valle-Cruz et al., 2019) and use these insights to inform future decision-making processes (Coglianese and Lehr, 2017). Moreover, AI applications hold the potential to make political decisions more comprehensible to the public, thereby enhancing accountability and public trust in political processes, particularly those supported by AI (Aoki, 2020).3 Given these potentials, as David Freeman Engstrom and colleagues point out, the use of AI in the political field overall promises “to transform how government agencies do their work. Rapid developments in AI have the potential to reduce the cost of core governance functions, improve the quality of decisions, and unleash the power of administrative data, thereby making government performance more efficient and effective.” (Engstrom et al., 2020, p. 6).
AI not only holds the promise of streamlining political decision-making processes with greater speed and efficiency. By processing complexities, overseeing causal relationships, and sometimes even providing simulation-based forecasts about the effectiveness—and potentially also side effects—of specific decisions, AI also has the potential to reduce existing uncertainties and introduce new certainties into political decision-making contexts.
4.2 Uncertainty as a challenge for political decision-making: the case of the Covid-19 pandemic
The rapid spread of SARS-CoV-2 and the newly emerged Covid-19 disease in December 2019 raised a critical question for government officials and political decision-makers across the globe: how should they respond? (Akilli and Gunes, 2023a,b; Greer et al., 2021). As many might still recall from media reports and, perhaps, from academic discussions, questions such as the following were debated: Should, as a precautionary measure, the metaphorical “gates be sealed,” restricting cross-border movement as early and as extensively as possible to prevent the virus and potentially infected individuals from entering their countries? Or should a more measured approach be adopted, aiming to contain the virus's spread while avoiding complete national isolation? Once the virus was within national borders, a new set of questions concerning domestic strategies emerged. These included: Should the status quo be maintained as much as possible, hoping that SARS-CoV-2 would ultimately prove to be a relatively harmless virus and the disease would take a mild course? Or should a comprehensive strict lockdown be enforced, aiming to effectively “ride out” the virus with a brief yet intense intervention? Alternatively, should a middle ground be pursued, employing a range of preventative measures and moderately curtailing public activities without entirely bringing them to a halt? In addition to these strategic considerations, legal and operational questions also arose: What restrictions are compatible with the constitutions of the respective countries, and how might they be effectively implemented and enforced?
Many of these questions clearly center on public health: how can citizens be safeguarded from the potentially deadly Covid-19 infection, and how can the spread of SARS-CoV-2 be controlled? However, as was highlighted at the time and has been repeatedly underscored by scholars since: every public health measure inevitably sends ripples through other sectors of society, with significant impacts on the economy, education, and the environment, among others (Butler, 2022; Zinn, 2021; ŽiŽek, 2020).
The complexity of the situation, reflected in these interrelated circumstances, the provisional nature of all available information (Kreps and Kriner, 2020), and the unpredictability of the consequences of potential decisions, resulted in significant uncertainties—ontological as well as epistemic, substantive as well as strategic, along with uncertainties of consequences and uncertainties of reliance—in political decision-making throughout the course of the pandemic (Berger et al., 2020; Evans, 2021). Because no matter which decision was ultimately made in this situation, it was expected to entail some form of “collateral damage.” That's why political decision-making at that time could not focus on finding the single right decision that would tackle and overcome all challenges productively and without undesirable side effects. Instead, it was more about assessing the positive and negative outcomes of various alternatives to discern the option promising the best medium to long-term ratio (Juul Andersen, 2014). However, even this approach proved to be complex, given the prevailing uncertainties. To compare the effects of different options, political decision-makers needed reliable information. These included, among others, the infectiousness of the virus and the severity of the disease, for which there was little data at the beginning of the pandemic (Chater, 2020). Moreover, questions arose about whether and, if so, when a treatment or vaccine would be available in what quantity and for whom (Del Rio and Malani, 2021). Equally crucial was understanding the capability of diverse health systems, which were somewhat enigmatic at the beginning of the pandemic. It was also vital to comprehend the impacts of various preventive measures on the economy, labor market (Rutter et al., 2020), the education system (Rutter et al., 2020; Viner et al., 2021), and the political situation in the country. For these considerations, there were varying, sometimes conflicting, assessments from different sources.
In essence, every corner was riddled with uncertainties, spanning medical, legal, economic, and social realms (Koffman et al., 2020; Norheim et al., 2021). Given the prevailing complexities, these uncertainties significantly complicated political decision-making (Rutter et al., 2020). And as the pandemic persisted, it became increasingly clear that it would not be possible to fundamentally eliminate (all of) these uncertainties (Rutter et al., 2020). Although some uncertainties were continuously mitigated through the influx of new data and information, this process simultaneously raised new questions in other areas, revealing additional informational gaps and generating fresh uncertainties (Koffman et al., 2020; Zinn, 2020). This prompted some to speak of a “continuing uncertainty” (Del Rio and Malani, 2021) and to advocate for “uncertainty management” instead of striving to overcome uncertainties: “Instead of seeking (or feigning) certainty we should be open about uncertainty and transparent in the ways in which we acknowledge the limitations of the imperfect data we have no choice but to use” (Rutter et al., 2020, p. 1).
4.3 The potential of AI-systems for decision-making during Covid-19
Over the course of the pandemic, numerous AI-systems were developed that were able to track and evaluate events using a range of parameters and variables (Chen et al., 2022). Some of these systems were even capable of generating short- to medium-term forecasts for the spread of SARS-CoV-2 and the evolution of the pandemic (Meuser et al., 2021; Yang et al., 2020). For instance, Vinayaka Gude reported on an AI system that could project pandemic trajectories over a 210-day period. This system could predict the effects of various interventions—or the absence of them—on Covid-19 mortality rates, hospital capacity, and the economic landscape. Additionally, it was able to provide evidence-based recommendations on when lockdowns should be imposed and when it would be relatively safe to lift restrictions (Gude, 2022). By delivering such insights and predictions, AI-systems could have reduced or even eliminated certain uncertainties in political decision-making, particularly ontological-substantive ones and uncertainties of consequences, while introducing new predictions to assist political leaders in making informed decisions—if they had been more widely used in these contexts rather than being confined to scientific settings.
In addition to such AI-driven analysis and decision-support systems, there are other ways in which AI could have further supported political decision-making back then. In the midst of the pandemic Murat Onder and Mehmet Metin Uzun provided an overview of the various possibilities of using AI to support political decision-making (Onder and Uzun, 2021). Covering 14 different areas of application, ranging from the design of preparedness plans, risk assessments of various countermeasures, and monitoring the epidemiological situation, to post-pandemic restoration policy and various tools that are currently in use or potentially ready for use, the authors demonstrated how AI could be—or, in few cases, were already—employed in the Covid-19 Preparation-, Prevention-, Response-, and Recovery-Policies. Their analysis culminates in the assertion that: “AI can make significant contributions in the preparation, mitigation-prevention, response, and recovery policies in the Covid-19 pandemic crisis. [… P]olicymakers can benefit from AI as decision support to reach high-quality decisions through fast and accurate data” (Onder and Uzun, 2021, p. 1).
Using the specific example of political decision-making during the Covid-19 pandemic, these brief accounts highlight the potential that AI-systems hold for political decision-making in general. Even at the time, and even more so now with additional time and research invested into such AI-systems, they held the promise of deciphering pandemic dynamics, identifying core patterns, and making these insights actionable to clarify the uncertainties surrounding societal and political responses to the virus. Additionally, they excel at providing simulations that predict the outcomes of various preventive measures or regulations, and in some cases, they can even generate specific recommendations. By doing so, AI has the potential to reduce existing uncertainties in political decision-making, not just in the context of pandemics, and to create forecasts that could serve as new certainties, as defined above, thereby aiding political leaders in their decision-making processes.
5 Challenges of using AI in political decision-making contexts for certainty purposes
In the previous section, the Covid-19 pandemic was used as an example to illustrate how uncertainties can complicate political decision-making processes and how AI could mitigate such uncertainties and create new certainties, thereby paving the way for more informed decisions. Such examples might easily lead to AI being seen as a “silver bullet” against uncertainty in political decision-making contexts, capable of reducing existing uncertainties, creating new certainties, and thus positively supporting these processes. However, while it's important not to downplay the potential of AI, it is equally crucial to consider the challenges its use in political decision-making may present (Floridi, 2021, 2023). Alongside procedural questions about the practical implementation of such AI-systems and their actual use in everyday political contexts (Nordstrom, 2022), and questions concerning data handling and protection whenever sensitive information is involved (Véliz, 2020), the argument presented here contends that deploying AI in political (decision-making) contexts inevitably brings complex challenges. The following sections will explore issues such as algorithmic bias and flawed AI models, AI-driven illusions of certainty, the assumption that AI's recommendations are the only viable options, the potential for technocratic shifts, and the critical debate over maintaining human control, thereby highlighting these challenges.
5.1 Algorithmic biases, lack in training data, and flawed AI-models
One challenge frequently mentioned in many discussions about the use of AI is that of algorithmic bias (Mavrogiorgos et al., 2024). AI-systems learn from training data. If this data is flawed or exhibits imbalances, AI-systems adopt these errors and discrepancies (Mukherjee et al., 2023). In short: AI is only as good as its training data (European Union Agency for Fundamental Rights, 2022). For example, when a facial recognition algorithm is trained predominantly on images of white individuals, it may later struggle to accurately identify the faces of persons of color (Benjamin, 2019). Similarly, if a job application screening algorithm is molded by a company's current data, which perhaps employs a majority of men, then this algorithm will probably be biased against applications highlighting gender-specific phrases such as “women's chess club captain” (Stahl et al., 2023, p. 10–11).
Similar biases may also emerge when AI-systems are employed in political contexts (Valle-Cruz et al., 2019, p. 91) to overcome potential uncertainties and gain clarity for impending decisions. Looking, for instance, at the data collected during the Covid-19 pandemic, two points quickly become clear: First, marginalized communities experienced notably higher rates of infection, hospitalization, and death during the pandemic (Evans, 2020; Khunti et al., 2020). Second, these communities are significantly underrepresented in demographic data surveys (Evans, 2020; Mathur et al., 2021). This increased vulnerability, coupled with societal minorities being overlooked in datasets, can easily result in AI-driven pandemic analyses and trajectory simulations yielding biased outcomes. In the worst-case, this may lead to AI-systems presenting results or even making recommendations that can be disadvantageous for minorities and place them in potentially extremely precarious situations (Anshari et al., 2022).
Beyond cases where AI bias stems from flawed training data, another challenge arises when AI lacks sufficient data altogether. The Covid-19 pandemic was a prime example of such a scenario (Naudé, 2020b). Because it surpassed previous epidemics in scope, rapid spread, and unpredictability, it took time to collect enough reliable data to train AI models to be capable of providing meaningful and reliable analyses and forecasts about the pandemic's trajectory (Naudé, 2020a).
Bias in AI does not only stem from data limitations but also from the way models are designed (Luengo-Oroz et al., 2021). Consider, for instance, AI-systems exclusively designed to assess the pandemic's impact on economic indicators, hospital occupancy, and the number of Covid-19 deaths, and to formulate recommendations based on these three parameters (Gude, 2022). Such AI could indeed provide insightful evaluations and recommendations concerning these aspects. However, since other parameters, like the consequences of these measures for education or social justice, are not taken into account by design, the AI-systems can quickly suggest measures that may have dramatic social side effects, such as escalating inequalities, increasing marginalization, or imposing excessive restrictions on specific population groups (Anshari et al., 2022).
5.2 False illusions of (absolute) certainty
When AI-systems are used to reduce uncertainties and gain new certainties about upcoming political decisions, there is a risk that decision-makers might develop a false impression of absolute certainties (Gasser and Mayer-Schönberger, 2024). True, AI can sift colossal datasets, identify intricate patterns, execute simulations, forecast outcomes—like the trajectory of a pandemic—and even advise on preventive actions. But they are never infallible and, just like humans, can never produce absolute certainty, i.e., predict the consequences of a decision with complete infallibility (Tretter, 2023). No matter how comprehensive and representative the data or how advanced and adept the AI-systems are, there remains a perpetual risk that real-world outcomes might stray from AI predictions. This could be due to unforeseen events, like, in the case of the pandemic, the emergence of a new Covid-19 variant with higher infectivity (Rutter et al., 2020) and more severe symptoms, or the unexpected development of a highly effective vaccine (Del Rio and Malani, 2021). It could also be because, as discussed earlier, the data foundation was not as representative as assumed, or critical parameters might have been missed during the algorithm's design or implementation.
Each artificially-created certainty inherently possesses a “continuing uncertainty” (Del Rio and Malani, 2021), the possibility of events unfolding differently than predicted. However, it is not this residual uncertainty itself that poses a challenge—because such lingering uncertainties exist almost everywhere (Beck, 1992) and are not exclusive to AI. The more pressing and more AI-specific challenge lies in the fact that these residual uncertainties are often not disclosed or, from time to time, might even be (willingly) overlooked, with any residual uncertainties fading from the spotlight (Gasser and Mayer-Schönberger, 2024). Given that AI-systems are oftentimes heralded as highly reliable, with their forecasts often being accurate and their recommendations leading to positive outcomes, there can be an inclination toward perceiving AI as a magic machine that produces definite certainties (Schlote, 2023). This perception can eclipse the recognition of ever-present uncertainties and the potential pitfalls of AI.
To prevent the emergence of such AI-driven certainty illusions, it is vital to actively counter such perceptions. When dealing with certainty-creating AI, it is always essential to maintain a healthy dose of skepticism and to always account for the non-knowledge that also exists in AI-systems (White and Lidskog, 2022) as well as the remaining uncertainties (Tretter, 2024b).
5.3 Presumptions of no-alternative, technocratic scenarios, and accountability issues
Yet, even in scenarios where there is an active cultivation of skepticism and persistent challenges to AI-generated certainty illusions, it is tough to stave off the ensuing challenge: the presumption that there is no (better) alternative. Even when there is an overarching understanding that AI is not infallible and cannot provide absolute truths, the question invariably pops up: Who could possibly outdo it (Tretter, 2023)? In the midst of the Covid-19 pandemic, for instance, who could better grasp the complex socio-medical-political interconnections, better anticipate the pandemic's trajectory, and better evaluate and weigh the consequences of various preventive measures against each other? Moreover, who would dare challenge a specialized AI's assessments, defy its recommendations, and venture on their own path—painfully conscious that, if their plan fails, they might later be questioned and shamed for not heeding the AI's guidance and presuming to know better themselves (Tretter et al., 2023)?
When it becomes this challenging to contest the assessment of AI-systems, their recommendations can quickly be deemed as “without alternatives.” Since, when—understandably—no one dares to challenge AI recommendations, alternatives might become scarce (or simply too risky; Tretter, 2024a). As a result, the solutions put forth by AI begin to appear as the most feasible, and in the worst case, might even be seen as the sole option. Once such unquestioned reliance on AI and its recommendations has become established, there is a risk of a transition to some kind of “technical solutionism” (Morozov, 2013) taking place, specifically to “AI solutionism” (Lindgreen and Dignum, 2023)—the belief that nearly all problems might best be solved through technological means, particularly AI. However, this development presents a significant threat to democratic governance for two reasons.
First, when political decisions are primarily shaped by AI recommendations, it paves the way for radical technocracy (Saetra, 2020), a form of politics that relies purely on experts and (artificially intelligent) expert systems, where public discourse and democratic participation—whether through direct or representative democracy—are marginalized (Schippers, 2020) and gradually lose legitimacy (Unver, 2019), until, in the end, the role of the demos is reduced to merely affirming expert solutions (Esmark, 2020). From a democratic and participatory perspective, however, this would be highly questionable (Sandel, 2020)—and, indeed, empirical surveys on the use of AI in political contexts have highlighted fears pointing toward this very scenario (König, 2023).
Second, the increasing reliance on AI systems in political decision-making raises significant concerns about accountability within democratic governance (Lechterman, 2024). Building on Strøm (2000) reflections on accountability and delegation, which emphasize that legitimacy in democracy depends on elected representatives (agents) being accountable to the electorate (principals), AI can be understood as an additional layer of delegation. Political actors, who traditionally function as the principals, now defer parts of the decision-making process to algorithmic systems. This leads to what can be described as a “double delegation problem,” in which accountability becomes increasingly ambiguous: Who is ultimately responsible when AI-driven decisions shape political outcomes (Busuioc, 2021)? If policymakers increasingly rely on AI-generated recommendations, they may shift accountability away from themselves, making it more difficult for citizens to determine whether political leaders have genuinely engaged with an issue in depth and critically examined AI-generated recommendations from multiple perspectives—a skill that requires specific qualifications, raising the question of whether decision-makers receive adequate training to exercise such oversight effectively—or if they are simply following algorithmic outputs. If such scrutiny is lacking, and AI is followed uncritically, accountability will gradually diminish, which, in turn, can erode the democratic legitimacy of elected representatives (Peters and Pierre, 2006).
5.4 Loss of human control
After discussing possible illusions of certainty and the potential shift toward technocratic states, a final point of concern arises: human control and potential loss thereof. The primary concern here is not about scenarios where an AI-system goes rogue and—admittedly, an amusing idea—uncontrollably starts drafting regulation after regulation, law after law. Instead, the question arises: how much understanding and influence can human actors still exert when AI-systems provide the essential assessments and formulate foundational proposals? In his Citizen's Guide to Artificial Intelligence, John Zerilli describes this challenge as follows:
“[T]here is a very real risk that when people rely on algorithmic systems they might defer to them excessively and lose meaningful control over their outputs. This could happen in government agencies too. Government agencies that rely on autonomous algorithmic tools may adopt an uncritically deferential attitude toward them. They may, in principle, retain some ultimate control, but in practice it is the algorithm that exercises the power. […] The human officials may not be inclined to wrestle control back from algorithmic tools, not because the tools are smarter or more powerful than they are, but because habit and convenience make them unwilling to do so.” (Zerilli, 2021, p. 138)
Scenarios like these highlight what could happen in extreme cases and the conceivable ways AI could inadvertently strip individuals of their autonomy. These scenarios raise critical legal (Beck and Burri, 2024; Beck et al., 2024; Beckers and Teubner, 2024) and ethical questions (Nyholm, 2024) about the responsible implementation and use of AI-systems in decision-making contexts (Floridi et al., 2018), as well as deeply philosophical questions like how much humans need to understand about what AI-systems do, how they come to their conclusions, and why they make their recommendations, in order to still say that humans have a form of “meaningful control” (Hille et al., 2023; Mecacci et al., 2024; Robbins, 2023; Santoni de Sio and van den Hoven, 2018)—and not merely endorse and execute AI's outputs without critical scrutinizing it (Engstrom et al., 2020). How much control should be strictly left to humans and how much “autonomy” can be given to the AI is a matter to be negotiated within specific contexts: It is a delicate equilibrium that teeters between “zero human intervention” (an approach that would likely face broad skepticism) and “absolute human control” [an equally impractical stance that not only seems philosophically absurd (Gräb-Schmidt, 2015) but would negate the very essence of employing AI].
6 Discussion
The insights above suggest that on the one hand, AI can help reduce uncertainties in political decision-making contexts and establish certainties that can assist political leaders with their decision-making. On the other hand, however, using AI in these contexts also brings several challenges. This raises the question of how to deal with such certainty-creating AI-systems in political decision-making contexts. I will touch upon this question briefly—as this is not the main focus of this paper, which is more concerned with highlighting the challenges and paving the way for more in-depth discussions—before delving into the limitations of the above considerations.
6.1 Initial suggestions on how to use certainty-producing AI-systems in political contexts
Given both the opportunities and challenges AI presents in political decision-making, the key question is not whether AI should be used, but rather how it can be integrated responsibly (Floridi, 2021)? On the one hand, it would be irresponsible to completely abandon the use of certainty-creating AI-systems. Such refusal would also eliminate the vast potential of these AI-systems (McEvoy, 2019)—which, in the best case, would “only” make decision-making more complex, but in the worst case, could lead to inferior political decisions with negative consequences for those affected (Braun et al., 2020). On the other hand, it would be reckless to deploy AI-systems without scrutiny, to rely entirely on their predictions, and to trust their recommendations without question (Gasser and Mayer-Schönberger, 2024). This would naively open the door to the aforementioned challenges and pave a slippery slope toward artificially intelligent technocracy and a loss of control. A responsible approach to AI-systems, particularly in crafting certainty within political decision-making, demands a balance, neither blindly sidelining such groundbreaking tech nor uncritically embracing every promise it makes (Tretter, 2024a).
Achieving this balance and determining how AI can be responsibly integrated into political decision-making requires more than theoretical discussion; it also demands practical testing in real-world contexts, critical evaluation, and continuous refinement through iterative processes (Caiza et al., 2024). To facilitate this, it is advisable to first introduce AI in specific political decision-making contexts, assess and refine it, and, if it proves reliable, gradually expand its application to additional contexts. The most suitable starting point for gradually introducing and testing AI for certainty purposes seems to be highly structured and repetitive settings, where processes can be refined and optimized through automated learning. This includes political domains focused on budgeting and (re-)distribution issues, where AI can enhance efficiency while operating within a controlled environment. Additionally, it would be advisable to first implement AI in localized settings, focusing on regional political decision-making rather than deploying AI for decision support at the national or international level from the outset. Such a gradual and critical approach offers two key advantages: first, it allows both the benefits and limitations of using AI for certainty purposes in decision-making to be carefully evaluated and refined within specific contexts before broader deployment; second, it helps foster acceptance—and potentially even trust—among political decision-makers and the public (Caiza et al., 2024), making the transition toward a wider application of AI for certainty-purposes in political contexts smoother.
Yet, even with a structured roadmap like this, it remains essential to keep in mind that uncertainties will always be part of political decision-making and, above all, of democracy (Przeworski, 1991) and that the ultimate goal—even when using AI—is more about finding a good way to handle it rather than completely overcoming it (Müller, 2021; Reiss, 1988), would be a wise approach characterized by a cautious and critical use of certainty-creating AI-systems (Floridi et al., 2020; Gasser and Mayer-Schönberger, 2024; Tretter, 2024b; Tretter et al., 2023), complemented by public oversight mechanisms (Floridi, 2023; Zerilli, 2021, p. 145).
6.2 Limitations
The primary limitation of the presentations provided is that they are purely conceptual considerations—without the collection and presentation of any empirical data. Yet, even within this empirical shortfall, these considerations are not entirely unfounded, metaphorically speaking. Several of the correlations alluded to in the discourse have been profoundly explored or empirically investigated. While not directly tied to the specific nexus of AI, politics, and uncertainty—a domain still notably under-researched (Engstrom et al., 2020)—these studies lend a certain degree of plausibility to the above consideration. Pertinent examples include the relationships between AI, certainty, and remaining uncertainties (Tretter, 2024b; Tretter et al., 2023), between AI and technocracy (Saetra, 2020) between AI and bias (Ntoutsi et al., 2020), and between AI and human control (Zerilli, 2021). Additionally, this study does not engage in an in-depth examination of technology acceptance, particularly how AI is perceived in political decision-making contexts and how these perceptions may evolve over time. While this issue is touched upon at several points, particularly in footnote 2, a more thorough exploration of these questions will be essential in future discussions on the responsible integration of AI for certainty purposes in political decision-making.
7 Conclusion
This article began with three observations. First, that societies have now become so complex and “fluid” that it is hardly possible to anticipate the effects of political decisions, let alone predict them precisely. This means, second, that political decision-makers face massive uncertainties, which greatly complicates the decision-making processes. Third, current AI-systems promise to bring clarity and order into complex situations, thereby reducing existing uncertainties and creating, through simulations, predictions, and decision support, a certain degree of predictability and certainty. While the promises of AI-systems and their application for reducing uncertainty and producing certainty in the context of political decision-making offer great potential on the one hand, this article set out to explore the other side of these AI-driven certainty technologies, questioning what challenges arise from the use of certainty-creating AI-systems during political decision-making.
Following the thesis that the use of AI-systems for certainty purposes in political context might bring with them both unique opportunities and new challenges, the concepts of uncertainty and certainty were first elaborated in general, then in the context of political decision-making. Using the Covid-19 pandemic as an example, it was then demonstrated how AI-systems can help overcome political decision-making uncertainties by making such complex situations more understandable. By predicting the consequences of various potential decisions, they enable the weighing of various options against each other, ensuring that decision-makers are widely aware of the implications of their choices. Having explored the vast potential AI for certainty purposes in the context of political decision-making, the subsequent section focused on four challenges associated with the use of such AI-systems: algorithmic biases, suggestions of certainty, presumptions of no-alternative and technocratic shifts, and the question of human control.
Using AI for certainty purposes in political decision-making contexts always comes with the risk of algorithmic biases. This means there is a danger that the training data of the intelligent system contains biases or distortions, which are then algorithmically reproduced, leading to inaccurate results, predictions, or questionable recommendations. This reflects the fact that AI-systems can never provide 100% certainty but can always offer only highly precise approximations. Notwithstanding such innate uncertainties, oftentimes an aura of infallibility is projected upon AI-systems. Such delusions of absolute certainty can engender a sentiment wherein AI-derived counsel is perceived as the sole viable recourse—after all, who would have the audacity to counter an AI's assessment? Where such perceptions of a lack of alternatives arise, there is a legitimate concern of slipping into technocratic conditions where political decisions are ultimately made not by the people but by technical expert systems. There is also the risk of eventually relinquishing control from human hands.
It is essential to address these challenges if we want to utilize AI-systems for certainty purposes and to support decision-making in political contexts. One important step in this process is to empower political decision-makers to always maintain a critical stance toward these tools. Additionally, it is beneficial to acknowledge that it is impossible to eradicate all uncertainties. To some degree, uncertainties are an intrinsic part of democracy—and attempting to eliminate them entirely might inadvertently jeopardize the very essence of our democracy itself.
Author contributions
MT: Writing – original draft, Writing – review & editing.
Funding
The author(s) declare that financial support was received for the research and/or publication of this article. This research was funded by the Federal Ministry of Research and Education (funding identifiers: 01GP1905B and 01GP2202B) and by the German Research Foundation (DFG, Deutsche Forschungsgemeinschaft) – SFB 1483 – Project-ID 442419336, EmpkinS. The funder had no role in conducting the research or in the preparation of the manuscript.
Acknowledgments
The author thanks Ulrich von Ulmenstein, Christina Lauppert von Peharnik, and David Ehrlich, Fiona Bendig and Judith Willberg for their insights, helpful comments, and discussions.
Conflict of interest
The author declares that the research was conducted in the absence of any commercial or financial relationships that could be construed as a potential conflict of interest.
Generative AI statement
The author(s) declare that Gen AI was used in the creation of this manuscript. In the preparation of this manuscript, generative AI (ChatGPT, version 4o) was employed for the purpose of linguistic enhancement and refinement of the text.
Publisher's note
All claims expressed in this article are solely those of the authors and do not necessarily represent those of their affiliated organizations, or those of the publisher, the editors and the reviewers. Any product that may be evaluated in this article, or claim that may be made by its manufacturer, is not guaranteed or endorsed by the publisher.
Footnotes
1. ^At first glance, the decision to focus this analysis on literature from the past 10 years may seem arbitrary. However, in my opinion, this timeframe is particularly well-suited to capturing the most recent political, technological, and methodological shifts that have fundamentally reshaped how uncertainty is perceived and studied. Politically, the past decade has been marked by rising global instability, exemplified by events such as Brexit in 2016, the election of populist leaders (e.g., Trump in 2016, Bolsonaro in 2018), and escalating geopolitical tensions, including the annexation of Crimea in 2014 and the U.S.-China trade war. These developments have significantly altered the dynamics of political decision-making under uncertainty. Technologically, rapid advancements in AI, big data analytics, and algorithmic governance have introduced new tools for managing uncertainty while simultaneously raising critical concerns about algorithmic bias, transparency, and accountability. Methodologically, political science and decision theory have increasingly incorporated computational models, simulation-based approaches, and interdisciplinary perspectives from AI ethics and governance, transforming the way uncertainty is conceptualized and addressed. While some of these trends have earlier origins, the past decade provides a contemporary and cohesive period that captures their most significant developments. That's why, pragmatically, this 10-year timeframe offers an optimal balance between analytical depth and coherence, allowing for a focused yet comprehensive examination of recent shifts without excessive fragmentation.
2. ^Beyond these consequentialist and deficiency-focused frameworks, metaphorical understandings of uncertainty have emerged as a third group. In such perspectives, uncertainty is described as something to be “navigated” like a ship on the sea (Spiegelthaler, 2024), “tamed” like a wild animal (Hertwig et al., 2019), or “won” like a game (Oriesek and Schwarz, 2021). Cf. Hertwig et al. (2019), Oriesek and Schwarz (2021), and Spiegelthaler (2024).
3. ^The question of how citizens perceive the use of AI in government contexts and political decision-making, as well as its impact on the acceptance of decisions and trust in political processes, is currently the focus of numerous empirical studies. A prominent observation made in several studies is the widespread skepticism toward the use of AI in political decision-making (König, 2023; Haesevoets et al., 2024). This skepticism is evident in findings showing that political decisions tend to have higher acceptance rates when humans are – at least partly – involved in the decision-making process (Gesk and Leyer, 2022; Haesevoets et al., 2024; Horvath et al., 2023; Starke and Lünich, 2020). Similarly, trust in these processes is generally higher when human actors play a role (Ingrams et al., 2022; Grimmelikhuijsen, 2023). Considering these insights on the one hand, the evident benefits of integrating AI into these processes on the other hand, many scholars emphasize the importance of hybrid collaborations between human and AI actors in political decision-making (Roehl and Hansen, 2024; Starke and Lünich, 2020; Trunk et al., 2020). Grimmelikhuijsen (2023), Haesevoets et al. (2024), Horvath et al. (2023), Ingrams et al. (2022), König (2023), and Trunk et al. (2020).
References
Abu el Ata, N., and Schmandt, R. (2016). The Tyranny of Uncertainty. A New Framework to Predict, Remediate and Monitor Risk. Berlin: Springer Nature.
Akilli, E., and Gunes, B. (Eds.). (2023a). World Politics in the Age of Uncertainty. The Covid-19 Pandemic. Vol. 1. Cham: Palgrave Macmillan.
Akilli, E., and Gunes, B. (Eds.). (2023b). World Politics in the Age of Uncertainty. The Covid-19 Pandemic. Vol. 2. Cham: Palgrave Macmillan.
Albornoz, V. M., MAc Cawley, A., and Plà-Aragonés, L. M. (Eds.). (2024). Optimization Under Uncertainty in Sustainable Agriculture and Agrifood Industry. Cham: Springer Nature.
Alvarez, S., Afuah, A., and Gibson, C. (2018). Editors' comments: should management theories take uncertainty seriously? Acad. Manag. Rev. 43, 169–172. doi: 10.5465/amr.2018.0050
Anshari, M., Hamdan, M., Ahmad, N., Ali, E., and Haidi, H. (2022). COVID-19, artificial intelligence, ethical challenges and policy implications. AI Soc 38, 707–720. doi: 10.1007/s00146-022-01471-6
Aoki, N. (2020). An experimental study of public trust in AI chatbots in the public sector. Gov. Inf. Q. 37:101490. doi: 10.1016/j.giq.2020.101490
Arend, R. J. (2024). Uncertainty in Strategic Decision Making. Analysis, Categorization, Causation and Resolution. Cham: Palgrave Macmillan.
Aspers, P. (2024). Uncertainty. Individual Problems and Public Solutions. New York, NY: Oxford University Press.
Bandyopadhyay, P. S., Brittan, G. Jr., and Taper, M. L. (2016). Belief, Evidence, and Uncertainty. Problems of Epistemic Inference. Cham: Springer Nature.
Bateman, C., Pleshette, M., Cindrich, A., and Phillips, C. (2023). Change. How to Turn Uncertainty into Opportunity. Coral Gables, FL: Mango Publishing.
Beck, J., and Burri, T. (2024). “From “human control” in international law to “human oversight” in the new EU act on artificial intelligence,” in Research Handbook on Meaningful Human Control of Artificial Intelligence Systems, eds. G. Mecacci, D. Amoroso, L. Cavalcante Siebert, D. Abbink, J. van den Hoven, and F. Santoni de Sio (Cheltenham: Edward Elgar), 104–130.
Beck, S., Gerndt, S., Samhammer, D., and Dabrock, P. (2024). “Meaningful human control in shared medical decision making,” in Research Handbook on Meaningful Human Control of Artificial Intelligence Systems, eds. G. Mecacci, D. Amoroso, L. Cavalcante Siebert, D. Abbink, J. van den Hoven, and F. Santoni de Sio (Cheltenham: Edward Elgar), 131–147.
Beckers, A., and Teubner, G. (2024). Digitale Aktanten, Hybride, Schwärme. Drei Haftungsregime für Künstliche Intelligenz. Berlin: Suhrkamp.
Beghetto, R. A., and Jaeger, G. J. (Eds.). (2022). Uncertainty. A Catalyst for Creativity, Learning and Development. Cham: Springer Nature.
Belloni, R., Della Sala, V., and Viotti, P. (Eds.). (2019). Fear and Uncertainty in Europe. The Return to Realism? Cham: Palgrave Macmillan.
Ben Ameur, H., Ftiti, Z., Louhichi, W., and Prigent, J.-L. (Eds.). (2023). Crises and Uncertainty in Economics. Singapore: Springer Nature.
Berger, L., Berger, N., Bosetti, V., Gilboa, I., Hansen, L. P., Jarvis, C., et al. (2020). Uncertainty and Decision-Making During a Crisis: How to Make Policy Decisions in the COVID-19 Context? Available online at: https://repec.bfi.uchicago.edu/RePEc/pdfs/BFI_WP_202095.pdf (accessed January 10, 2025).
Bergmane, U. (2023). Politics of Uncertainty. The United States, the Baltic Question, and the Collapse of the Soviet Union. New York, NY: Oxford University Press.
Boyd, I. L. (2019). “Risk and uncertainty in the context of government decision-making,” in Risk and Uncertainty in a Post-Truth Society, eds. S. van der Linden and R. E. Löfstedt (Cham: Palgrave Macmillan), 54–73.
Braun, M., Hummel, P., Beck, S., and Dabrock, P. (2020). Primer on an ethics of AI-based decision support systems in the clinic. J. Med. Ethics 47:e3. doi: 10.1136/medethics-2019-105860
Brigham, K., and Johns, M. M. E. (2020). The Good Doctor. Why Medical Uncertainty Matters. New York, NY: Seven Stories.
Brown, P. R., and Zinn, J. O. (Eds.). (2022). Covid-19 and the Sociology of Risk and Uncertainty. Studies of Social Phenomena and Social Theory Across 6 Continents. Cham: Palgrave Macmillan.
Busuioc, M. (2021). Accountable artificial intelligence: holding algorithms to account. Public Adm. Rev. 81, 825–836. doi: 10.1111/puar.13293
Butler, J. (2022). What World Is This? A Pandemic Phenomenology. New York, NY: Columbia University Press.
Caiza, G., Sanguña, V., Tusa, N., Masaquiza, V., Ortiz, A., and Garcia, M. V. (2024). Navigating governmental choices: a comprehensive review of artificial intelligence's impact on decision-making. Informatics 11:64. doi: 10.3390/informatics11030064
Cantarelli, P., Belle, N., and Hall, J. L. (2023). Information use in public administration and policy decision-making: a research synthesis. Public Adm. Rev. 83, 1667–1686. doi: 10.1111/puar.13735
Caron, F. (2013). Managing the Continuum: Certainty, Uncertainty, Unpredictability in Large Engineering Projects. Heidelberg: Springer.
Carrón, J. (2020). Where Is God? Christian Faith in the Time of Great Uncertainty (S. Beatty, Trans.). Montreal, QC: McGill-Queen's University Press.
Ceberio, M., and Kreinovich, V. (Eds.). (2023a). Decision Making Under Uncertainty and Constraints. A Why-Book. Cham: Springer Nature.
Ceberio, M., and Kreinovich, V. (Eds.). (2023b). Uncertainty, Costraints, and Decision Making. Cham: Springer Nature.
Chanes, P., and Rees Jones, I. (Eds.). (2022). Civil Society in an Age of Uncertainty. Institutions, Governance and Existential Challenges. Croydon: Bristol University Press.
Charles, V., Rana, N. P., and Carter, L. (2022). Artificial Intelligence for data-driven decision-making and governance in public affairs. Gov. Inf. Q. 39:101742. doi: 10.1016/j.giq.2022.101742
Chater, N. (2020). Facing up to the uncertainties of COVID-19. Nat. Hum. Behav. 4:439. doi: 10.1038/s41562-020-0865-2
Chen, D., AlNajem, N. A., and Shorfuzzaman, M. (2022). Digital twins to fight against COVID-19 pandemic. Internet Things Cyber Phys. Syst. 2, 70–81. doi: 10.1016/j.iotcps.2022.05.003
Chiffi, D. (2021). Clinical Reasoning. Knowledge, Uncertainty, and Values in Health Care. Cham: Springer Nature.
Code, L. (2020). Manufactured Uncertainty. Implications for Climate Change Skepticism. Albany, NY: SUNY.
Coglianese, C., and Lehr, D. (2017). Regulating by robot: administrative decision making in the machine-learning era. Georgetown Law J. 105, 1147–1223.
Dabrock, P. (2012). Befähigungsgerechtigkeit. Ein Grundkonzept Konkreter Ethik in Fundamentaltheologischer Perspektive. Gütersloh: Gütersloher Verlagshaus.
Daniels, N. (2011). “Reflective equilibrium,” in Stanford Encyclopedia of Philosophy, eds. E. N. Zalta and U. Nodelman. Available online at: https://plato.stanford.edu/archives/spr2025/entries/reflective-equilibrium/ (accessed March 13, 2018).
Datta, S., and Kutzewski, T. (2023). Strategic Optionality. Pathways Through Disruptive Uncertainty. Cham: Springer Nature.
de Kock, W. J. (2024). On Being in the Middle. Doing Theology in the Face of Uncertainty. Eugene, OR: Wipf and Stock.
de Sousa, W. G., de Melo, E. R. P., Bermejo, P. H. D. S., Farias, R. A. S., and Gomes, A. O. (2019). How and where is artificial intelligence in the public sector going? A literature review and research agenda. Govern. Inform. Q. 36:101392. doi: 10.1016/j.giq.2019.07.004
Del Rio, C., and Malani, P. (2021). COVID-19 in 2021—continuing uncertainty. JAMA 325, 1389–1390. doi: 10.1001/jama.2021.3760
Dewey, J. (1929). The Quest for Certainty. A Study of the Relation of Knowledge and Action. London: George Allen and Unwin.
Dewulf, A., and Biesbroek, R. (2018). Nine lives of uncertainty in decision-making: strategies for dealing with uncertainty in environmental governance. Policy Soc. 37, 441–458. doi: 10.1080/14494035.2018.1504484
Dimitrakakis, C., and Ortner, R. (2022). Decision Making Under Uncertainty and Reinforcement Learning. Theory and Algorithms. Cham: Springer Nature.
Edelstein, D. M. (2017). Over the Horizon. Time, Uncertainty, and the Rise of Great Powers. Ithaca, NY: Cornell University Press.
Elliott, A. C. A. (2021). What are the Chances of That? How to Think about Uncertainty. Oxford: Oxford University Press.
Engstrom, D. F., Ho, D. E., Sharkey, C. M., and Cuéllar, M.-F. (2020). Government by algorithm: artificial intelligence in federal administrative agencies. SSRN Electr. J. doi: 10.2139/ssrn.3551505
European Union Agency for Fundamental Rights (2022). Bias in Algorithms. Artificial Intelligence and Discrimination. Luxemburg: Publications Office of the European Union.
Evans, M. K. (2020). Covid's color line - infectious disease, inequity, and racial justice. N. Engl. J. Med. 383, 408–410. doi: 10.1056/NEJMp2019445
Evans, R. (2021). SAGE advice and political decision-making: ‘Following the science' in times of epistemic uncertainty. Soc. Stud. Sci. 52, 53–78. doi: 10.1177/03063127211062586
Ferrara, A. (2008). The Force of the Example. Explorations in the Paradigm of Judgement. New York, NY: Columbia University Press.
Fitria Fatimah, A. (2024). Political implications of AI in governance. J. Polit. Innov. Anal. 1, 8–16.
Floridi, L. (Ed.). (2021). Ethics, Governance, and Policies in Artificial Intelligence. Cham: Springer.
Floridi, L. (2023). The Ethics of Artificial Intelligence. Principles, Challenges, and Opportunities. Oxford: Oxford University Press.
Floridi, L., Cowls, J., Beltrametti, M., Chatila, R., Chazerand, P., Dignum, V., et al. (2018). AI4People—an ethical framework for a good AI society: opportunities, risks, principles, and recommendations. Minds Mach. 28, 689–707. doi: 10.1007/s11023-018-9482-5
Floridi, L., Cowls, J., King, T. C., and Taddeo, M. (2020). How to design AI for social good: seven essential factors. Sci. Eng. Ethics 26, 1771–1796. doi: 10.1007/s11948-020-00213-5
Friedman, J. A., and Zeckhauser, R. (2018). Analytic confidence and political decision-making: theoretical principles and experimental evidence from national security professionals. Polit. Psychol. 39, 1069–1087. doi: 10.1111/pops.12465
Furr, N., and Harmon Furr, S. (2022). The Upside of Uncertainty. A Guide to Finding Possibility in the Unknown. Boston, MA: Harvard Business Review.
García-Marzá, D., and Calvo, P. (2024). Algorithmic Democracy: A Critical Perspective Based on Deliberative Democracy. Cham: Springer International Publishing.
Garvey, B., Humzah, D., and Le Roux, S. (2022). Uncertainty Deconstructed. A Guidebook for Decision Support Practitioners. Cham: Springer Nature.
Gasser, U., and Mayer-Schönberger, V. (2024). Guardrails. Guiding Human Decision in the Age of AI. Princeton, NJ: Princeton University Press.
Gesk, T. S., and Leyer, M. (2022). Artificial intelligence in public services: when and why citizens accept its usage. Gov. Inf. Q. 39:101704. doi: 10.1016/j.giq.2022.101704
Ghanem, R., Higdon, D., and Owhadi, H. (Eds.). (2017). Handbook of Uncertainty Quantification. Cham: Springer.
Gräb-Schmidt, E. (2015). Das ethische stichwort: autonomie. Zeitschr. Evangelische Ethik 59, 133–137. doi: 10.14315/zee-2015-0208
Greer, S. L., King, E. J., Massard da Fonseca, E., and Peralta-Santos, A. (Eds.). (2021). Coronavirus Politics. The Comparative Politics and Policy of COVID-19. Ann Arbot, MI: University of Michigan Press.
Grimmelikhuijsen, S. (2023). Explaining why the computer says no: algorithmic transparency affects the perceived trustworthiness of automated decision-making. Public Adm. Rev. 83, 241–262. doi: 10.1111/puar.13483
Gross, M. (2007). The unknown in process. Dynamic connections of ignorance, non-knowledge and related concepts. Curr. Sociol. 55, 742–759. doi: 10.1177/0011392107079928
Gude, V. (2022). Modeling a decision support system for Covid-19 using systems dynamics and fuzzy inference. Health Inform. J. 28:14604582221120344. doi: 10.1177/14604582221120344
Guy, T. V., Kárny, M., and Wolpert, D. H. (2015). Decision Making. Uncertainty, Imperfection, Deliberation and Scalability. Cham: Springer.
Haesevoets, T., Verschuere, B., Van Severen, R., and Roets, A. (2024). How do citizens perceive the use of Artificial Intelligence in public sector decisions? Gov. Inf. Q. 41:101906. doi: 10.1016/j.giq.2023.101906
Han, P. K. J. (2021). Uncertainty in Medicine. A Framework for Tolerance. New York, NY: Oxford University Press.
Hardon, A. (2020). Chemical Youth. Navigating Uncertainty in Search of the Good Life. Cham: Palgrave Macmillan.
Hatch, S. (2016). Snowball in a Blizzard. A Physician's Notes on Uncertainty in Medicine. New York, NY: Basic Books.
Heazle, M. (2012). Uncertainty in Policy Making. Values and Evidence in Complex Decisions. London: Routledge.
Hertwig, R., Pleskac, T. J., and Pachur, T. (Eds.). (2019). Taming Uncertainty. Cambridge: The MIT Press.
Hille, E. M., Hummel, P., and Braun, M. (2023). Meaningful human control over AI for health? A review. J Med Ethics doi: 10.1136/jme-2023-109095
Hjaltalin, I. T., and Sigurdarson, H. T. (2024). The strategic use of AI in the public sector: a public values analysis of national AI strategies. Gov. Inf. Q. 41:101914. doi: 10.1016/j.giq.2024.101914
Horvath, L., James, O., Banducci, S., and Beduschi, A. (2023). Citizens' acceptance of artificial intelligence in public services: evidence from a conjoint experiment about processing permit applications. Gov. Inf. Q. 40:101876. doi: 10.1016/j.giq.2023.101876
Hubler, J. N. (2021). Overcoming Uncertainty in Ancient Greek Political Philosophy. Cham: Springer Nature.
Hudson, V. M. (Ed.). (2018). Artificial Intelligence and Interational Politics. New York, NY: Routledge.
Hüttche, T. (Ed.). (2023). Finance in Crises. Financial Management under Uncertainty. Cham: Springer Nature.
Ingrams, A., Kaufmann, W., and Jacobs, D. (2022). In AI we trust? Citizen perceptions of AI in government decision making. Policy Internet 14, 390–409. doi: 10.1002/poi3.276
Irwin, W. (2019). God Is a Question, Not an Answer. Finding Common Ground in Our Uncertainty. Lanham: Rowman & Littlefield.
Janeja, M. K., and Bandak, A. (2018). Ethnographies of Waiting. Doubt, Hope and Uncertainty. London: Bloomsbury Academic.
Johnson, L. S. M. (2022). The Ethics of Uncertainty. Entangled Ethical and Epistemic Risks in Disorders of Consciousness. New York, NY: Oxford University Press.
Johnson, T. (2018). Crisis Leadership. How to Lead in Times of Crisis, Threat and Uncertainty. London: Bloomsbury.
Jorrit Hasselaar, J. (2023). Climate Change, Radical Uncertainty and Hope. Theology and Economics in Conversation. Amsterdam: Amsterdam University Press.
Juul Andersen, T. (Ed.). (2014). Contemporary Challenges in Risk Management. Dealing with Risk, Uncertainty and the Unknown. London: Palgrave Macmillan.
Kampourakis, K., and McCain, K. (2019). Uncertainty. How it Makes Science Advance. New York, NY: Oxford University Press.
Katzenstein, P. J. (Ed.). (2022). Uncertainty and It Discontents. Worldviews in World Politics. Cambridge: Cambridge University Press.
Kay, J., and King, M. (2021). Radical Uncertainty. Decision-Making Beyond the Numbers. New York, NY: W.W. Norton.
Khunti, K., Singh, A. K., Pareek, M., and Hanif, W. (2020). Is ethnicity linked to incidence or outcomes of Covid-19? BMJ 369:m1548. doi: 10.1136/bmj.m1548
Kirshner, J. (2022). An Unwritten Future. Realism and Uncertainty in World Politics. Princeton, NJ: Princeton University Press.
Klinke, A. (2024). A Theory of Uncertainty. Perspectives in Philosophy, Social Sciences, and Risk Research. London: Routledge.
Kochenderfer, M. J. (Ed.). (2015). Decision Making Under Uncertainty. Theory and Application. Cambridge: The MIT Press.
Kochenderfer, M. J., Wheeler, T. A., and Wray, K. H. (2020). Algorithms for Decision Making. Cambridge: The MIT Press.
Koffman, J., Gross, J., Etkind, S. N., and Selman, L. (2020). Uncertainty and COVID-19: how are we to respond? J. R. Soc. Med. 113, 211–216. doi: 10.1177/0141076820930665
Komporozos-Athanasiou, A. (2022). Speculative Communities. Living with Uncertainty in a Financialized World. Chicago, IL: The Unicersity of Chicago Press.
König, P. D. (2023). Citizen conceptions of democracy and support for artificial intelligence in government and politics. Eur. J. Polit. Res. 62, 1280–1300. doi: 10.1111/1475-6765.12570
König, P. D., and Wenzelburger, G. (2020). Opportunity for renewal or disruptive force? How artificial intelligence alters democratic politics. Govern. Inform. Q. 37:101489. doi: 10.1016/j.giq.2020.101489
Kreps, S. E., and Kriner, D. L. (2020). Model uncertainty, political contestation, and public trust in science: evidence from the COVID-19 pandemic. Sci. Adv. 6:eabd4563. doi: 10.1126/sciadv.abd4563
Kulesza, W., and Doliński, D. (2023). Social Biases During Covid 19. Managing the Anxiety of Uncertainty. Cham: Springer Nature.
Kuziemski, M., and Misuraca, G. (2020). AI governance in the public sector: three tales from the frontiers of automated decision-making in democratic settings. Telecomm. Policy 44:101976. doi: 10.1016/j.telpol.2020.101976
LaCaze, A., and Osimani, B. (Eds.). (2020). Uncertainty in Pharmacology. Epistemology, Methods, and Decisions. Cham: Springer Nature.
Latour, B. (2005). Reassembling the Social. An Introduction to Actor-Network-Theory. Oxford; New York, NY: Oxford University Press.
Lechterman, T. M. (2024). “The concept of accountability in AI ethics and governance,” in The Oxford Handbook of AI Governance, eds. J. B. Bullock, Y.-C. Chen, J. Himmelreich, V. M. Hudson, A. Korinek, M. M. Young, et al. (New York, NY: Oxford University Press), 164–182.
Li, D., and Du, Y. (2017). Artificial Intelligence with Uncertainty, 2 Edn. Boca Raton, FL: CRC Press.
Lindgreen, S., and Dignum, V. (2023). “Beyond AI solutionism. Toward a multi-disciplinary approach to artificial intelligence in society,” in Handbook of Critical Studies of Artificial Intelligence, ed. S. Lindgreen (Cheltenham, PA: Edward Elgar), 163–172.
Løhre, E., and Halvor Teigen, K. (2023). When leaders disclose uncertainty: effects of expressing internal and external uncertainty about a decision. Q. J. Exp. Psychol. 77, 1221–1237. doi: 10.1177/17470218231204350
Luengo-Oroz, M., Bullock, J., Pham, K. H., Lam, C. S. N., and Luccioni, A. (2021). From Artificial Intelligence bias to inequality in the time of COVID-19. IEEE Technol. Soc. Mag. 40, 71–79. doi: 10.1109/MTS.2021.3056282
Luhmann, N. (1995). Social Systems (J. Bednarz and D. Baecker, Trans.). Stanford: Stanford University Press.
Luhmann, N. (2012). Theory of Society, Vol. 1 and 2 (R. Barrett, Trans.). Stanford: Stanford University Press.
Mathur, R., Rentsch, C. T., Morton, C. E., Hulme, W. J., Schultze, A., MacKenna, B., et al. (2021). Ethnic differences in SARS-CoV-2 infection and COVID-19-related hospitalisation, intensive care unit admission, and death in 17 million adults in England: an observational cohort study using the OpenSAFELY platform. Lancet 397, 1711–1724. doi: 10.1016/S0140-6736(21)00634-6
Mavrogiorgos, K., Kiourtis, A., Mavrogiorgou, A., Menychtas, A., and Kyriazis, D. (2024). Bias in machine learning: a literature review. Appl. Sci. 14:8860. doi: 10.3390/app14198860
McEvoy, F. J. (2019). Political machines: ethical governance in the age of AI. Moral Philos. Politics 6, 337–356. doi: 10.1515/mopp-2019-0004
Mecacci, G., Amoroso, D., Cavalcante Siebert, L., Abbink, D., van den Hoven, J., and Santoni de Sio, F. (Eds.). (2024). Research Handbook on Meaningful Human Control of Artificial Intelligence Systems. Cheltenham, PA: Edward Elgar.
Messelken, D., and Winkler, D. (Eds.). (2021). Health Care in Contexts of Risk, Uncertainty, and Hybridity. Cham: Springer Nature.
Meuser, T., Baumgärtner, L., and Lieser, P. (2021). “Pandemic skylines: digital twins for more realism in epidemic simulations,” in 18th International Conference on Information Systems for Crisis Response and Management (ISCRAM) (Blackburg). Available online at: http://idl.iscram.org/files/tobiasmeuser/2021/2320_TobiasMeuser_etal2021.pdf.
Mishel, M. H. (1988). Uncertainty in illness. Image J. Nurs. Scholarsh. 20, 225–232. doi: 10.1111/j.1547-5069.1988.tb00082.x
Morduch, J., and Schneider, R. (2017). The Financial Diaries. How American Families Cope in a World of Uncertainty. Princeton, NJ: Princeton University Press.
Morley, J., Machado, C. C. V., Burr, C., Cowls, J., Joshi, I., Taddeo, M., et al. (2020). The ethics of AI in health care: a mapping review. Soc. Sci. Med. 260:113172. doi: 10.1016/j.socscimed.2020.113172
Morozov, E. (2013). To Save Everything, Click Here. The Folly of Technological Solutionism. New York, NY: PublicAffairs.
Motet, G., and Bieder, C. (Eds.). (2017). The Illusion of Risk Control. What Dies it Take to Live with Uncertainty? Cham: Springer.
Mukherjee, A., Kulshrestha, J., Charkaborty, A., and Kumar, S. (Eds.). (2023). Ethics in Artificial Intelligence. Bias, Fairness and Beyond. Singapore: Springer Nature.
Müller, J.-W. (2021). Democracy Rules. Liberty, Equality, Uncertainty. New York, NY: Farrar, Straus and Giroux.
Mulligan, D. L., and Danaher, P. A. (Eds.). (2021). Researchers at Risk. Precarity, Jeopardy and Uncertainty in Academia. Cham: Palgrave Macmillan.
Murilla, E., Riccarda Morganti, P., and Espinosa, J. M. (Eds.). (2023). Creating Economic Stability Amid Global Uncertainty. Post-Pandemic Recovery in Mexico's Emerging Economy. Cham: Palgrave Macmillan.
Murphy-Greene, C. (Ed.). (2022). Environmental Justice ad Resilience in an Age of Uncertainty. London: Routledge.
Naudé, W. (2020a). “Artificial Intelligence against COVID-19: an early review,” in Discussion Paper Series, Issue. Available online at: https://www.jstor.org/stable/pdf/resrep60040.pdf.
Naudé, W. (2020b). Artificial Intelligence vs COVID-19: limitations, constraints and pitfalls. AI Soc. 35, 761–765. doi: 10.1007/s00146-020-00978-0
Nordstrom, M. (2022). AI under great uncertainty: implications and decision strategies for public policy. AI Soc. 37, 1703–1714. doi: 10.1007/s00146-021-01263-4
Norheim, O. F., Abi-Rached, J. M., Bright, L. K., Bærøe, K., Ferraz, O. L. M., Gloppen, S., et al. (2021). Difficult trade-offs in response to COVID-19: the case for open and inclusive decision making. Nat. Med. 27, 10–13. doi: 10.1038/s41591-020-01204-6
Ntoutsi, E., Fafalios, P., Gadiraju, U., Iosifidis, V., Nejdl, W., Vidal, M. E., et al. (2020). Bias in data-driven artificial intelligence systems—an introductory survey. WIREs Data Mining Knowl. Discov. 10:e1356. doi: 10.1002/widm.1356
Nyholm, S. (2024). “Automated mobility and meaningful human control. When and why is control important and what are its different dimensions,” in Research Handbook on Meaningful Human Control of Artificial Intelligence Systems, eds. G. Mecacci, D. Amoroso, L. Cavalcante Siebert, D. Abbink, J. van den Hoven, and F. Santoni de Sio (Cheltenham, PA: Edward Elgar), 13–27.
Nzobonimpa, S., and Savard, J.-F. (2023). Ready but irresponsible? Analysis of the government Artificial Intelligence readiness index. Policy Internet 15, 397–414. doi: 10.1002/poi3.351
Obeng-Odoom, F. (2021). The Commons in an Age of Uncertainty. Decolonizing Nature, Economy, and Society. Toronto, ON: University of Toronto Press.
Olofsson, A., and Zinn, J. O. (Eds.). (2019). Researching Risk and Uncertainty. Methodologies, Methods and Research Strategies. Cham: Springer Nature.
Onder, M., and Uzun, M. M. (2021). Roles of Artificial Intelligence (AI) on COVID-19 pandemic crisis management policies. Int. J. Public Administr. Dig. Age 8, 1–13. doi: 10.4018/IJPADA.294122
Oriesek, D. F., and Schwarz, J. O. (2021). Winning the Uncertaity Game. Turning Strategic Intent into Results with Wargaming. New York, NY: Routledge.
Ove Hansson, S. (1996). Decision making under great uncertainty. Philos. Soc. Sci. 26, 369–386. doi: 10.1177/004839319602600304
Pencheva, I., Esteve, M., and Mikhaylov, S. J. (2018). Big data and AI – a transformational shift for government: so, what next for research? Public Policy Administr. 35, 24–44. doi: 10.1177/0952076718780537
Peters, B. G., and Pierre, J. (2006). “Governance, accountability and democratic legitimacy,” in Governance and Democracy Comparing National, European and International Experiences, eds. A. Benz and I. Papadopoulos (New York, NY: Routledge), 29–43.
Plinio, A. J., and Smith, M. A. (2019). Time to Get Real! Turning Uncertainty Into an Action Plan for Personal and Professional Success. New Brunswick, NJ: Rutgers University Press.
Poloz, S. (2022). The Next Age of Uncertainty. How the World Can Adapt to a Riskier Future. Toronto, ON: Allen Lane.
Przeworski, A. (1991). Democracy and the Market. Political and Economic Reforms in Eastern Europe and Latin America. Melbourne, VIC: Cambridge University Press.
Raska, M., and Bitzinger, R. (Eds.). (2023). The AI Wave in Defence Innovation. Assessing Military Artificial Intelligennce Strategies, Capabilities, and Trajectories. New York, NY: Routledge.
Reiss, T. J. (1988). The Uncertainty of Analysis. Problems in Truth, Meaning, and Culture. Ithaca, NY: Cornell University Press.
Renn, O., Klinke, A., and van Asselt, M. (2011). Coping with complexity, uncertainty and ambiguity in risk governance: a synthesis. Ambio 40, 231–246. doi: 10.1007/s13280-010-0134-0
Ricci, A. (2024). The Geography of Uncertainty. A Conceptual Model of Early Modern Globalization and the Current Crisis. London: Routledge.
Robbins, S. (2023). The many meanings of meaningful human control. AI Ethics. 4, 1377–1388. doi: 10.1007/s43681-023-00320-6
Rodriguez, E. (2023). Risk Analytics. Data-Driven Decisions under Uncertainty. Boca Raton, FL: CRC Press.
Roehl, U. B. U., and Hansen, M. B. (2024). Automated, administrative decision-making and good governance: Synergies, trade-offs, and limits. Public Adm. Rev. 84, 1184–1199. doi: 10.1111/puar.13799
Rosa, H. (2012). Weltbeziehungen im Zeitalter der Beschleunigung. Umrisse einer neuen Gesellschaftskritik. Berlin: Suhrkamp.
Rubin, V. L. (2010). Epistemic modality: from uncertainty to certainty in the context of information seeking as interactions with texts. Inform. Proc. Manag. 46, 533–540. doi: 10.1016/j.ipm.2010.02.006
Rutter, H., Wolpert, M., and Greenhalgh, T. (2020). Managing uncertainty in the Covid-19 era. BMJ 370:m3349. doi: 10.1136/bmj.m3349
Saetra, H. S. (2020). A shallow defence of a technocracy of artificial intelligence: examining the political harms of algorithmic governance in the domain of government. Technol. Soc. 62:101283. doi: 10.1016/j.techsoc.2020.101283
Samimian-Darash, L. (2022). Uncertainty by Design. Preparing for the Future with Scenario Technology. Ithaca, NY: Cornell University Press.
Sandoval-Almazan, R., Millan-Vargas, A. O., and Garcia-Contreras, R. (2024). Examining public managers' competencies of artificial intelligence implementation in local government: a quantitative study. Gov. Inf. Q. 41:101986. doi: 10.1016/j.giq.2024.101986
Santoni de Sio, F., and van den Hoven, J. (2018). Meaningful human control over autonomous systems: a philosophical account. Front Robot AI 5:15. doi: 10.3389/frobt.2018.00015
Sarkar, A., Badyopadhyay, N., Singh, S., and Sachan, R. (Eds.). (2024). Risk, Uncertainty and Maladaption to Climate Change. Policy, Practice and Case Studies. Singapore: Springer Nature.
Schippers, B. (2020). Artificial Intelligence and democratic politics. Polit. Insight 11, 32–35. doi: 10.1177/2041905820911746
Schlote, Y. (2023). Konvergenz und Überwältigung: Die Mythen der Künstlichen Intelligenz aus theologisch-ethischer Perspektive. München: Georg Olms Verlag; Universitätsbibliothek Ludwig-Maximilians-Universität München.
Schomberg, R. (1993). Science, Politics and Morality. Scientific Uncertainty and Decision Making. Dordrecht: Springer.
Seppälä, E. (2024). Sovereign. Reclaim your Freedom, Energy, and Power in a Time of Distraction, Uncertainty, and Chaos. Carlsbad, CA: Hay House.
Smagacz-Poziemska, M., Gómez, M. V., Pereira, P., Guarino, L., Kurtenbach, S., and Villalón, J. J. (Eds.). (2020). Inequality and Uncertainty. Current Challenges for Cities. Singapore: Springer Nature.
Souza de Cursi, E. (2024). Uncertainty Quantification with R. Bayesian Methods. Cham: Springer Nature.
Spiegelthaler, D. (2024). The Art of Uncertainty. How to Navigate Chance, Ignorance and Luck. London: Penguin.
Stahl, B. C., Schroeder, D., and Rowena, R. (2023). Ethics of Artificial Intelligence. Case Studies and Options for Addressing Ethical Challenges. Cham: Springer.
Stanton, M. C. B., and Roelich, K. (2021). Decision making under deep uncertainties: a review of the applicability of methods in practice. Technol. Forecast. Soc. Change 171:120939. doi: 10.1016/j.techfore.2021.120939
Starke, C., and Lünich, M. (2020). Artificial intelligence for political decision-making in the European Union: effects on citizens' perceptions of input, throughput, and output legitimacy. Data Policy 2:e16. doi: 10.1017/dap.2020.19
Strøm, K. (2000). Delegation and accountability in parliamentary democracies. Eur. J. Polit. Res. 37, 261–289. doi: 10.1111/1475-6765.00513
Szpiro, G. G. (2020). Risk, Choice, and Uncertainty. Three Centuries of Economic Decision-Making. New York, NY: Columbia University Press.
Tangredi, S. K., and Galdorisi, G. (Eds.). (2021). AI at War. How Big Data, Artificial Intelligence and Machine Learning are Changing Naval Warware. Annapolis, MD: Naval Institute Press.
Tauber, A. I. (2022). The Triumph of Uncertainty. Science and Self in the Postmodern Age. Budapest: Central European University Press.
Thiel, M., Fiocchetto, E., and Maslanik, J. D. (2023). The Politics of Social In/Exclusion in the EU. Civic Europe in an Age of Uncertainty. Cham: Palgrave Macmillan.
Topol, E. J. (2019). Deep Medicine. How Artificial Intelligence Can Make Healthcare Human Again. New York, NY: Basic Books.
Tretter, M. (2023). “Ambivalenzen gegenwärtiger Gewissheitsbestrebungen. Menschliche Entscheidungsfreiheit in einer gewisserwerdenden Welt,” in Alexa, Wie Hast du's mit der Religion? Interreligiöse Zugänge zu Technik und Künstlicher Intelligenz, eds. A. Puzio, N. Kunkel, and H. Klinge (Darmstadt: WBG), 135–156.
Tretter, M. (2024a). Equipping AI-decision-support-systems with emotional capabilities? Ethical perspectives. Front. Artific. Intellig. 7:1398395. doi: 10.3389/frai.2024.1398395
Tretter, M. (2024b). Mitigating health-related uncertainties during pregnancy: the role of smart health monitoring technologies. J. Med. Internet Res. 26:e48493. doi: 10.2196/48493
Tretter, M., Ott, T., and Dabrock, P. (2023). AI-produced certainties in health care: current and future challenges. AI Ethics 5, 497–506. doi: 10.1007/s43681-023-00374-6
Trunk, A., Birkel, H., and Hartmann, E. (2020). On the current state of combining human and artificial intelligence for strategic organizational decision making. Bus. Res. 13, 875–919. doi: 10.1007/s40685-020-00133-x
Unver, A. (2019). Artificial Intelligence, Authoritarianism and the Future of Political Systems (EDAM Research Reports, Issue). Available online at: https://ssrn.com/abstract=3331635
Valle-Cruz, D., Fernandez-Cortez, V., and Gil-Garcia, J. R. (2022). From E-budgeting to smart budgeting: exploring the potential of artificial intelligence in government decision-making for resource allocation. Gov. Inf. Q. 39:101644. doi: 10.1016/j.giq.2021.101644
Valle-Cruz, D., Ruvalcaba-Gomez, E. A., Sandoval-Almazan, R., and Criado, J. I. (2019). “A review of artificial intelligence in government and its potential from a public policy perspective,” in 20th Annual International Conference on Digital Government Research (Dubai).
Valle-Cruz, D., and Sandoval-Almazan, R. (2018). “Towards an understanding of artificial intelligence in government,” in 19th Annual International Conference on Digital Government Research: Governance in the Data Age (Delft).
van der Heide, A. (2023). Dealing in Uncertainty. Insurance in the Age of Finance. Bristol: Bristol University Press.
van Noordt, C., and Misuraca, G. (2022). Artificial intelligence for the public sector: results of landscaping the use of AI in government across the European Union. Gov. Inf. Q. 39:101714. doi: 10.1016/j.giq.2022.101714
Véliz, C. (2020). Privacy is Power. Why and How You Should Take Back Control of Your Data. London: Bantam.
Ventriss, C. (2021). Public Affairs and Democratic Ideals. Critical Perspectives in an Era of Political and Economic Uncertainty. Albany, NY: SUNY.
Vera Hoyos, C., and Cárdenas Marín, W. (2024). The Use of Artificial Intelligence in Political Decision-Making. Preprints. doi: 10.20944/preprints202410.0360.v1
Viner, R. M., Bonell, C., Drake, L., Jourdan, D., Davies, N., Baltag, V., et al. (2021). Reopening schools during the COVID-19 pandemic: governments must balance the uncertainty and risks of reopening schools against the clear harms associated with prolonged closure. Arch. Dis. Child. 106, 111–113. doi: 10.1136/archdischild-2020-319963
von Ramin, L. (2022). Politik der Ungewissheit. Grenzen postmoderner Sozialphilosophie in Anschluss an Richard Rorty, Zygmunt Bauman und Oliver Marchart. Bielefeld: Transcript.
Walker, B. (2019). Finding Resilience. Change and Uncertainty in Nature and Society. Oxfordshire: CABI.
White, J. M., and Lidskog, R. (2022). Ignorance and the regulation of artificial intelligence. J. Risk Res. 25, 488–500. doi: 10.1080/13669877.2021.1957985
Williams, D. (2024). The Art of Uncertainty. Probable Realism and the Victorian Novel. New York, NY: Cambridge University Press.
Wirtz, B. W., Weyerer, J. C., and Geyer, C. (2018). Artificial intelligence and the public sector—applications and challenges. Int. J. Public Administr. 42, 596–615. doi: 10.1080/01900692.2018.1498103
Wittgenstein, L. (1969). On Certainty, eds. D. Paul, G. E. M. Anscombe, G. H. von Wright. Oxford: Basil Blackwell.
Workman, M., Dooley, K., Lomax, G., Maltby, J., and Darch, G. (2020). Decision making in contexts of deep uncertainty - an alternative approach for long-term climate policy. Environ. Sci. Policy 103, 77–84. doi: 10.1016/j.envsci.2019.10.002
Yang, Z., Zeng, Z., Wang, K., Wong, S. S., Liang, W., Zanin, M., et al. (2020). Modified SEIR and AI prediction of the epidemics trend of COVID-19 in China under public health interventions. J. Thorac. Dis. 12, 165–174. doi: 10.21037/jtd.2020.02.64
Yoe, C. (2019a). Primer of Risk Analysis. Decision Making under Uncertainty, 2 Edn. Boca Raton, FL: CRC Press.
Yoe, C. (2019b). Principles of Risk Analysis. Decision Making Under Uncertainty, 2 Edn. Boca Raton, FL: Taylor and Francis.
Zahariadis, N. (2003). Ambiguity and Choice in Public Policy. Political Decision Making in Modern Democracies. Washington, DC: Georgetown University Press.
Zinn, J. O. (Ed.). (2008). Social Theories of Risk and Uncertainty. An Introduction. Malden, MA: Blackwell.
Zinn, J. O. (2021). Introduction: towards a sociology of pandemics. Curr. Sociol. 69, 435–452. doi: 10.1177/00113921211020771
Keywords: artificial intelligence, uncertainty, decision support systems, democracy, technocracy, complexity, leadership
Citation: Tretter M (2025) Opportunities and challenges of AI-systems in political decision-making contexts. Front. Polit. Sci. 7:1504520. doi: 10.3389/fpos.2025.1504520
Received: 30 September 2024; Accepted: 24 February 2025;
Published: 19 March 2025.
Edited by:
F. Ramón Villaplana, University of Murcia, SpainReviewed by:
Jasmin Fitzpatrick, Johannes Gutenberg University Mainz, GermanyRaluca Fărcaş, Babeş-Bolyai University, Romania
Copyright © 2025 Tretter. This is an open-access article distributed under the terms of the Creative Commons Attribution License (CC BY). The use, distribution or reproduction in other forums is permitted, provided the original author(s) and the copyright owner(s) are credited and that the original publication in this journal is cited, in accordance with accepted academic practice. No use, distribution or reproduction is permitted which does not comply with these terms.
*Correspondence: Max Tretter, bWF4LnRyZXR0ZXJAZmF1LmRl