- 1Universidad del Norte, Barranquilla, Colombia
- 2Department of Political Sciences and International Relations, Universidad del Norte, Barranquilla, Colombia
- 3Department of Systems Engineering and Computing, Barranquilla, Colombia
This study explores the profound effects of information and communication technologies (ICT) on contemporary democracy. Focusing on the 2020 US presidential election, this research investigates how Twitter/X structures online communities with specific socialization patterns and ways to construct the truth. The rise of these platforms has sparked debate with split conclusions over whether they are enhancing or undermining democratic processes. Rather than keep digging within this unsolved discussion, this study moves the focus of inquiring toward how ICT affects the very existence of subjects in democracy. This means transitioning from defining ICT solely by its utility as a separate technology that affects behavior to seeing how subjects are entangled in the virtual world created by ICT. Methodologically, the users’ practices on the web are mapped using computational sciences metrics. This study employed Twitter’s Stream API to assemble a dataset encompassing tweets that featured keywords. The descriptive analytics are executed utilizing the Python programming language. In conducting sentiment analysis, this research employed the Twitter-roBERTa-base model. To develop a comprehensive analysis of large interaction datasets, we propose a novel methodology leveraging large language models to automate the classification process. The analysis reveals how algorithmically driven virtual interactions create a “mixed reality,” where virtual and real-world dynamics intersect, leading to increased polarization and the erosion of democratic deliberation. Trump’s defeat marked a collision between users who took to the streets under the banner of a conspiracy theory, which had gained traction as an alternative virtual truth through acclamation as practice, and citizens who use the practice of deliberation over empirical results of the electoral process. This study not only provides empirical evidence on the impact of ICT on democracy but also introduces innovative computational techniques for analyzing large-scale social media data.
1 Introduction
The impact of technology on every aspect of life worldwide has generated significant uncertainty in diverse issues such as economic prosperity, climate change, medical advancements, and military conflicts. Are these advances genuinely solving problems and propelling humanity forward, or are they exacerbating issues and leading us toward disaster? This uncertainty is also prevalent in politics, where public perceptions range from utopian to dystopian, often overlapping in a confusing and disorganized manner (Thiele, 2020). The situation is particularly concerning for democratic systems, where the once solid foundation constructed on a basic consensus reveals not only its fragility but gives freeway to radical dissensus among citizens. Currently, the corresponding decline in social welfare and the deterioration of international peace remind also us of the historical bond between these phenomena and democracy since the 18th century, making the matter more pressing.
The increasing uncertainty, confusion, and urgency in politics have led to numerous studies on the current and future impacts of information and communication technologies (ICT) on democracy. This research has expanded our understanding of how social media reshape information consumption, transform communication models, and influence political outcomes. Central to these discussions are effects of on citizens’ attitudes and behaviors and whether it ultimately strengthens or undermines democratic systems. Findings, however, remain divided, with scholars highlighting both the negative and positive impacts of ICT on democratic processes.
One prominent focus within these studies is the role of digital platforms in disseminating political information. Barash (2022) underscores how openness and communicative freedom among ICT users can foster public expression and generate demand for more democratic spaces. In contrast, Vosoughi et al. (2018) find that false information spreads more rapidly on these platforms, amplifying misinformation’s influence on public opinion. Similarly, Pariser (2011), Iyengar and Hahn (2009), and Ohme (2021) argue that algorithm-driven “filter bubbles” create echo chambers of political content tailored to users’ preferences, reinforcing biases and ideological silos.
Further analyses examine whether online interactions create an “echo chamber” effect or facilitate a “national conversation” (Barbera et al., 2015), getting mixed and inconclusive results. Studies by Weismueller et al. (2022) indicate that emotionally charged content is more likely to be shared, especially content expressing positive emotions. Meanwhile, research by Thiele (2020), Jackson et al. (2020), and Fileborn and Loney-Howes (2019) highlights ICT’s role in empowering social and political movements—such as Iran’s Twitter revolution in 2009 and the #MeToo movement—by providing platforms that bypass traditional media gatekeepers. This shift toward a more open information environment has led some to question whether ICT facilitates a new form of “demos,” akin to the ancient Greek assembly, by fostering a virtual agora that promotes participatory democracy (Mounk, 2018). However, scholars like Sunstein (2001) and Barbera et al. (2015) warn that echo chambers may instead create fragmented digital spaces, driving polarization, fostering intolerance, and undermining democratic norms through the rapid spread of fake news.
These debates extend to ICT’s effects on specific political outcomes. A consensus acknowledges social media’s influence in lowering participation barriers for elections and protests through user-generated content (Zhuravskaya et al., 2020). Nevertheless, perspectives diverge on the implications: some argue that ICT strengthens democratic engagement by challenging dominant narratives, as seen in movements like #BlackLivesMatter (Tufecki, 2017). Others contend that ICT also enables manipulative tactics, which can favor populist candidates and fuel extremism (Shahin, 2023; Schwartz et al., 2022; Adler and Drieschova, 2021). Here, literature review yields neither conclusive evidence nor definitive conclusions.
Whether the focus is on information consumption, communication models, or political outcomes; efforts to distill the essence of ICT through its influence on citizens’ attitudes and behavior encounter a persistent ambiguity. This inconclusiveness underscores a deeper tension within political theory: the dual capacity of ICT to both liberate and constrain democratic engagement. Rather than keep digging within these split conclusions, this study moves the focus of inquiring toward how ICT affects the very existence of subjects in democracy. This means transitioning from defining ICT solely by its utility as a separate technology that affects behavior to seeing how subjects are entangled in the virtual world created by ICT. In this approach, continuity emerges where there was once a separation between individuals as inner “subjects” and technology as outer “objects.” It is through what the subject “does” in this reality–virtuality continuum that it comes to understand itself and its Being. The political Being manifests in the subject through its actions in social media that, in turn, constitute him.
Drawing on Heidegger’s classic metaphor of the workman using a hammer, this juncture offers an apt opportunity to examine the political entanglement of democracy with ICT. In Being and Time (Heidegger, 2008), Heidegger illustrates how, in the act of hammering, the hammer becomes an extension of the workman’s hand, only recognized as a distinct object when it fails to function as expected. In a similar way, the ICT research referred to above has focused on utility of social media in these current moments when society experiences increasing uncertainty about capacity of ICT to ensure political behavior in ways compatible with democracy as it was initially presumed. However, it is precisely through the “broken” hammer that the workman, seeking answers, discovers the broader context of his workshop—his Being-in-the-world (Heidegger, 2008). Hence, this original contribution of article relies on exploring how subjects exist in democracy (what they do in the continuum) entangled with ICT, thereby illuminating the current Being of democracy itself in digital times.
Placed in the continuum of reality–virtuality, our study opens the subject–object intertwined relations explaining how machine intelligence works to influence, change, or alter the previous democratic political patterns of socialization in the real world. Rather than just taking for granted the inputs and outputs received and produced by ICT as an external object, this article figures out—through machine-learning algorithms and natural language inference techniques—the process of how network interactions shape “our reality” altering our pre-existing one. The outcome of using these platforms is a mixed reality, which emerges from the overlap between the algorithmically mediated virtual reality and the real reality produced by intersubjective relations. Shortly, by combining political science and engineering, we see how machine intelligence from the virtual world impacts real-world democratic practices. This situation creates a mixed reality where emotional and alternative truths from the virtual world fuel polarization and mass mobilization in the real world. It is, in other words, an updated way of saying that transformations in how we exist within democracy reshape our very being in democracy.
The theoretical and methodological proposal embedded in this interdisciplinary approach is based on a case study. It studies data collected from Twitter/X communities formed after the 2020 US presidential election. Within this frame, the central problem of article is to explain how ICT practices and logic deployed by Twitter/X communities in that electoral juncture contribute to erode American democracy. The “co-retweets tree” analysis reveals the formation of homogenous user clusters. These clusters contribute to the radicalization of ideas, polarization among groups, and the proliferation of fake news. We hold that in this US election, virtual spaces supplanted deliberation as the primary democratic practice, with virtual interactions simultaneously constraining discussion, magnifying voting, and undermining negotiation, thereby generating aggregative polarizing truths. In this characteristic mixed reality scenario, the clash between “deliberation truth” and “acclamation truth” created an epistemic crisis that favors Trump’s populism and republican radicalization, fueling a spiral of protest in support and a counterprotest opposing them.
At first glance, selecting the 2020 US presidential election and Twitter/X as a case study might seem controversial, given that democratic competition in mixed reality is widespread worldwide. However, there are specific junctural, historical, and methodological reasons behind this choice. First, the period surrounding the election was marked by the intense use of platforms and political misinformation, perhaps as any other. The 2020 US presidential election highlighted, for the first time, the negative effects virtual communities—especially Twitter/X, which is highly popular in politics—could have, even in well-established and mature democracies. This brought the American public and citizens all over vis-à-vis with these challenges.
Second, the United States is crucial for understanding democracy in a mixed reality. It has been the epicenter of democracy in modernity and the hub of transformative technologies in Silicon Valley in the contemporary world. Erosion in US democracy, as a global reference, affects democracies in both the Global North and South. Additionally, the way the impact of ICT in US politics is assessed could help determine its potential use in the future. Finally, from a methodological perspective, selecting a case facilitates access to relevant data and allows for its in-depth analysis through innovative computer science metrics making precise theoretical and methodological contributions possible.
We aimed to contribute theoretically and methodologically to political science and introduce methodological innovations in computational science for this type of research. On the one hand, we contribute to the theoretical critical theory debate in political science by identifying and tracing the new discursive and behavioral virtual practices that are changing political reality and eroding democracy. We move beyond theoretical conjecture by offering empirical evidence on how relationships mediated by algorithms alter intersubjective relations, replacing deliberation with acclamation as the communicative action in democracy. Due to computational techniques, this research provides accurate metrics of the practices involved in this virtual space. Although Milgram et al. (1994) and Zuboff (2018) have previously referenced “mixed reality” to describe the continuum of reality and virtuality, neither has fully identified the distinct social practices that reveal the Being in each—one in reality, the other in virtuality—nor the complex overlaps and their implications for democracy that exist in ubiquity.
On the other hand, while this study utilizes well-known computational science techniques for parts of the analysis, it also introduces important methodological innovations to complete others. The research constructs a classic co-retweet network to analyze the creation and behavior of communities and conducts a sentiment analysis to measure the degree of polarization and radicalization within clusters. However, given the necessity for a precise instrument to detect misinformation stories, this research diverges from the use of traditional machine-learning algorithms and natural language inference techniques. Based on the rise of large language models, it proposes a stance detection approach with multiple agents, combined with a straightforward methodology to find the most optimal prompts for the task. Consequently, the contribution extends beyond political science to include advancements in computational techniques. Existing computational studies analyzing cluster formation typically rely on the manual classification of a subset of interactions to establish a model baseline. To develop an optimal prompt strategy that facilitates comprehensive analysis of large interaction datasets, a novel methodology leveraging large language models to automate the classification process is proposed.
Our argument develops in five sections. Building on computational science methods, the first section details the source, metrics, and techniques used to access, collect, classify, and analyze Twitter/X data for the research. The second section situates the 2020 US presidential election within a critical theory framework, introducing the concept of mixed reality to understand the challenging democratic struggle in this new form of a continuous spectrum of reality. The third section explains how the Twitter/X algorithm constructs two sharply differentiated communities— @realDonaldTrump vs. @JoeBiden—characterizing their structure, practices, and the attitudes of their members. Here, the community constructions reveal the way algorithmic relations constitute the “Being,” this is how it alters the previous structure powers behind the democratic discourse and how new identities and political realities produce radicalization and polarization. The fourth section analyzes how these differences make one community more prone to sharing fraud narratives, assessing the challenges in terms of democratic erosion. In the final section, the conclusion and implications are presented.
2 Methodology and techniques
The practices on the web are mapped using computational sciences methodologies and metrics. This study employed Twitter’s Stream API, specifically the “Spritzer” variant, to assemble a dataset encompassing tweets that featured keywords associated with the 2020 US Presidential Elections. The identification of keywords is facilitated using study by Chen et al. (2022). The temporal scope of the tweets spans from November 3 to 23, 2020, a period notable for spreading misinformation within the social network, as documented by Kennedy et al. (2022). Subsequently, the dataset utilized in this investigation comprised 150,569 original tweets and 727,085 retweets generated from 475,629 distinct Twitter/X users.
The descriptive analytics are executed utilizing the Python programming language, leveraging scientific libraries such as Pandas and NumPy. Topological analyses of the networks are conducted using the Python Library NetworkX. Furthermore, the construction of a graphical representation of the overall network topology and sub-community networks is accomplished through the utilization of Gephi, with the ForceAtlas-2 algorithm.
In conducting sentiment analysis, this research employed the Twitter-roBERTa-base model, fine-tuned specifically for sentiment analysis using the TweetEval benchmark. The sentiment analysis is executed with a sample of 1 to 10 tweets from a randomly selected subset of users within each designated group, encompassing both inside and outside mentions of each community. Approximately 1,000 tweets were retrieved per group, facilitating a comprehensive evaluation of sentiment patterns across the four groups.
In order to analyze the false stories and fake news in our case study, a new dataset is constructed using data from the US 2020 presidential election dataset. This dataset comprises 35,076 Twitter/X users, categorized into two communities based on the co-retweet network: 19,570 users (49%) associated with the Trump community and 15,506 users (39%) associated with the Biden community. Users not belonging to a community were discarded. Each user in the dataset is represented by several key attributes, including tweet IDs, follower count, network centrality, and other relevant variables.
To investigate the prevalence of election fraud narratives across different levels of network, a stratified sampling strategy is implemented. Users in each community (@realDonaldTrump vs. @JoeBiden) are categorized into four groups based on their network centrality, ranging from low to high. Within each centrality group, 500 users were randomly selected. For each selected user, a minimum of two and a maximum of five tweets are randomly sampled. This resulted in a final dataset of 15,488 tweets from 6,000 users, ensuring representation across various levels of the network and providing a diverse range of perspectives on election fraud claims.
To develop an effective prompt for classifying tweets according to their stance on election fraud, a two-step approach is employed. First, a preliminary small sample of tweets is collected using the previously described sampling strategy. This sample served as a basis for discussion and analysis, providing insights into the types of content and language used in tweets related to election fraud. Second, a separate test dataset is created through manual annotation. Eighty seven tweets are carefully examined and assigned one of three labels: “support,” “against,” or “neutral,” reflecting the tweet’s stance on election fraud. The limited size of the test dataset is due to the time-consuming nature of manual annotation.
Following a thorough analysis of the collected tweets, five distinct prompts are designed. Each prompt, while employing different words and approaches, aimed to effectively identify the stance of a tweet regarding election fraud. To evaluate the efficacy of these prompts, we leveraged state-of-the-art large language models (LLMs) provided by OpenAI. These models are selected due to their accessibility, advanced capabilities, and sophisticated reasoning abilities, making them ideal for stance detection tasks. Specifically, we tested the prompts using GPT-4-turbo, GPT-4, GPT-3.5-turbo, and GPT-3.5-turbo augmented with the COLA strategy outlined by Lan et al. (2023). This variety of approaches allowed for a comprehensive assessment of performance of each prompt across different LLMs and strategic implementations.
Simple accuracy is selected as the primary evaluation metric for comparing the performance of different combinations of models and prompts. This choice is made after preliminary analysis revealed no substantial differences in performance when using F1 macro or micro averaging. After classifying sampled tweets of each user using the best-performing model and prompt, the overall stance of a user toward election fraud can be determined. This is achieved by calculating the percentage of tweets classified as “support,” “neutral,” or “against” for each user. These percentages are calculated using the following formulas:
Finally, for analyzing the distribution of users in each different centrality group, percentiles and box plots are employed.
3 2020 US presidential election, mixed reality, and critical theory
Website Navigation is one of the most widespread social practices of our time. This modest yet absorbing practice is behind the deep transformation of our Being-in-the-world. In January 2020, 87% of the US population was connected to the internet (Kemp, 2020), spending an average of 48.7% of their waking hours in front of a screen (Guttmann, 2023). Its ever-increasing popularity stems from its functionality in mixed reality. Political reality and our daily lives occur neither in the real world nor in virtual reality but in a mixed reality where physical and digital objects coexist (Milgram et al., 1994). Navigation, in contrast to immersion, allows real-time interaction with both worlds within this new blended environment (Milgram et al., 1994). The gift of ubiquity that users experience in this new overlapping reality, which becomes more naturalized with technological advances, presents significant challenges for how people perceive their world, and alters the parameters of democracy.
Before the development of ICT, schools of thought like critical theory understood reality as a social construction, leading them to focus on social practices. For Habermas, discursive practices are central to how we interpret the world, as no knowledge is possible without language, given that language structures thought (Habermas, 1984). The Frankfurt School holds that deliberation allows the construction of a rational, moral, and objective order (Cortina, 2009). Thus, the dialogue of deliberation enables individuals to mutually and rationally adjust their initial approaches under the principle of the best argument. In addition, the consensus reached through deliberation shifts imposition from one-sided to joint acceptance. Moreover, finally, deliberation reaches objectivity because the consensus establishes what a specific community thinks is the truth under precise circumstances of time and space (Cortina, 2009).
Following the same vein, Elster (1998) has identified four intersubjective practices for decision-making in modern societies: negotiation, voting, representation, and deliberation. In a representative democracy, deliberation precedes the other three. For result of an election to be legitimate, there must be deliberation among candidates and voters. Elections must be competitive to be valid. Given the urgency to form a government every 4 years, representative democracy builds a majority by aggregating citizens’ preferences through free voting after deliberations. Once representatives are elected, Congress applies a similar formula to achieve consensus: deliberation, plus voting, and, additionally, negotiation.
What ICT alters is the intersubjective relations through which human beings construct reality in the real world. It introduces new interactions based on algorithms and machine intelligence, characteristics of virtual reality.
The computational processes with which these platforms [such as Twitter/X] operate fracture the intersubjective encounters between different users that characterize deliberation in the real world. Through machine learning—a process that machines increasingly execute without supervision and automatically—user navigation data is grouped according to similarities, identifying data structures and patterns even without labeling (TIBCO, 2023).
As a result, the deliberative communities that characterize politics in the real world are replaced by homogenous subgroups called echo chambers. These echo chambers hinder encounters with alterity, or people who hold different opinions. The automatic clustering of users based on their navigation similarities intensifies the subjective character of reality, reduces the possibilities of encountering opposing views, insulates these communities, and reinforces the opinions of their members through sameness and repetition (Sunstein, 2001; Pariser, 2011; Barbera et al., 2015).
In the virtual world, the replacement of encounters with clustering strips users of agency, reducing them to mere patients who are allocated to the group where they fit best. Rather than being subjects capable of forming their views and making decisions while dealing with differences, automated machine learning treats users as things grouped according to opinions and decisions that can be anticipated algorithmically (Zuboff, 2018). The substitution of subject-citizens deliberation by user-objects clustering makes virtual realities more susceptible to manipulation.
Nevertheless, in a political world that experiences reality as a blend—a continuous integration of the real and virtual worlds—establishing what is really true becomes problematic. The practice of navigating these interconnected realities allows for real-time interactions between both. In this mixed reality, there is a clash between intersubjective methods of validating ideas and algorithmic ones.
4 Twitter/X algorithm and the constriction of plurality
A total of 150,569 original tweets and 727,085 retweets from 475,629 distinct Twitter/X users discussing the results of the 2020 US presidential elections were identified on the social network from 3 November to 23 November 2020. Upon examining this extensive material, which encompasses users’ reactions from Election Day and the subsequent 20 days, a striking division between two distinct groups emerges: those supporting @realDonaldTrump and those supporting @JoeBiden.
These two Twitter/X communities can be observed after building what Finn et al. (2014) referred to as a co-retweeted network. This “network is constructed as an undirected weighted graph connecting highly visible accounts that audience members have retweeted during some real-time event” (Finn et al., 2014). When a single user retweets content from two or more different accounts, they endorse the content and reveal their thematic affinity. In a co-retweet network analysis, the nodes represent different users who create content with thematic affinity. The size and the number assigned to the nodes within a graph establish their network position (Van den Heuvel and Sporns, 2013). The edges convey information about the links between nodes. A node with more links to other nodes indicates greater centrality for that user and the content they create within the network. A wider link between two users signifies greater affinity in their tweets. The more connections (edges) a content creator (node) has with other high-weight nodes, the more influential they are considered within the network. In general terms, the distinction and measurement of nodes and edges helps establish the centrality of nodes, assigning a measure of influence to each node based on both the quantity and quality of its connections to other nodes (Bihari and Pandia, 2015). In Figure 1, for example, node 2 exhibits high centrality within the network, as indicated by its size and the number of edges. Additionally, nodes 2 and 3 demonstrate a strong thematic affinity, as reflected in the broadness of the connecting edge.
When the automated machine-learning process runs and users engage on Twitter/X regarding the US presidential election results, the anticipated plural discussion among 475,629 distinct users becomes elusive. Out of 1,616 distinct communities, the top two—@realDonaldTrump (the pink one) and @JoeBiden (the green one)—dominated, encompassing 88 percent of all nodes. @realDonaldTrump held 49 percent with 19,570 nodes, while @JoeBiden had 39 percent with 15,506 nodes (see Figure 2). This co-retweet network graph illustrates how this clustering restricts deliberation between the two groups, with relative few important nodes, thereby promoting insular interactions. The narrowing of voices is driven by users’ preference for being followers, primarily through retweeting, rather than generating original content. In this scenario, a discussion involving 475,629 Twitter/X users was largely shaped by 39,842 individuals who created similar content, which was then amplified by 727,085 retweet-votes from the remaining users. Twitter/X clusters are mainly communities of followers instead of discussants.
Rather than remaining neutral, Twitter/X has begun “organizing our mind” (Hardt and Negri, 2000) through a recommendation algorithm that distills approximately 500 million daily tweets into a select few top tweets (Twitter/X, 2023). In the context of the 2020 US presidential election, the tweets appearing on users’ timelines were chosen based on the likelihood of future user interactions and engagement with trending tweets within their communities (Twitter/X, 2023). Politically, this translates into the creation and reinforcement of insular communities and confrontational scenarios.
So, how does it work? In essence, the algorithm first fetches the top 1,500 tweets from a massive pool, evenly split between users followed and unfollowed. In-network tweets are predicted using logistic regression for engagement likelihood, whereas out-of-network tweets are sourced through social graph analysis. These candidates are then ranked by a neural network that optimizes positive engagement using a 48 M-parameter model. Finally, the feed is curated by applying heuristics and filters, excluding tweets from blocked or muted accounts (Twitter/X, 2023).
Critics might argue—with a high degree of validity—that this article confirms that Twitter/X algorithms operate as intended and publicly proclaimed. After all, such platforms are not digital public infrastructures; they were not designed to promote democratic deliberation but to collect user data and serve advertisements. However, this analysis focuses less on the platform as an “external object” and more on its entanglement with subjects, revealing how it alters public deliberation through the algorithm’s commercialized bias, ultimately affecting the agency of citizens who become users in its presence. It is crucial to interrogate the algorithmic effects on subjects within a commercialized public sphere.
4.1 Communities’ radicalization
In the 2020 US presidential election, Twitter/X contributed to the increased radicalization of the electoral contest. Users were not only automatically sorted according to their preferences but also retweeted posts and content creators that aligned with their views, often without prior deliberation. This created a virtual environment in which users became more radicalized as their initial preferences were repeatedly confirmed. Ideas were reinforced through repetition, and individuals became emotionally engaged with their community through the act of retweeting, which served as a form of voting without dialogical discussions.
An analysis of the co-retweet network depicted in Figure 2 reveals that the communities surrounding Donald Trump and Joe Biden are both endogamous and highly radicalized, with Trump’s community being more so. When examining the 20 most mentioned users within each cluster, Trump’s community shows that 70% of mentions are directed within its own group, with only 30% directed outside. Of these, 6% are directed toward the opposing community, and 24% to unspecified groups. This pattern of reinforcing messages and repeatedly mentioning specific users within the community, while neglecting outsiders, is also evident in Biden’s community, though to a lesser degree: 53% of mentions are directed within the community, and 47% are directed outside, with 16% toward the opposing community and 31% to others (see Table 1).
Mentions in social networks do more than include specific names and messages; they also engage community members with those contents and influencers through the act of retweeting. A sentiment analysis of these mentions highlights the positive sentiment scores associated with mentions occurring both within and outside each community (see Figure 3). Initial findings indicate that the 75th percentile value for sentiment scores in outside communities is significantly lower than that within the inside community. This suggests that mentions directed toward users within one’s own community carry a more positive sentiment than those directed outside the community. Additionally, an intra-community analysis reveals a slight positivity difference between the Trump and Biden communities, with Trump’s community showing a higher positive sentiment. On a scale from 0.0 to 1.0 where the former means the lower positive sentiment and the latter the maximum, Trump registered 0.58, while Biden 0.38. In both cases, but more pronounced in Trump’s, retweeting fosters strong emotional identification among users with their communities, creating a culture of approval and acclamation toward influencers and messages. In this context, radicalization intensifies, as emotional attachment creates barriers that prevent questioning certain names and ideas.
The network analysis reveals why Trump’s community is more endogamous and radicalized than Biden’s. Trump’s community exhibits a greater number of edges, which indicates stronger thematic affinity among its nodes, and includes more users with intense connections (see Figure 2). Specifically, Trump’s community comprises 19,570 nodes and 253,294 edges, with an average degree of 25.88. In contrast, Biden’s community has 15,506 nodes and 101,520 edges, with an average degree of 13.09. These higher numbers and averages in Trump’s community suggest it is larger and more densely interconnected than Biden’s. Additionally, Donald Trump is significantly more influential within his community than Biden is within his. Tables 2, 3 display the top 10 most influential users in the co-retweet network, based on eigenvector-weighted centrality. With a weighted centrality of 0.65, @realDonaldTrump surpasses @JoeBiden’s 0.57. Furthermore, Trump’s node almost triples the influence of the next most influential account within his community, which has a mere 0.22 centrality weight (see Table 2). In contrast, Biden’s community displays more plurality, with @KamalaHarris holding a centrality weight of 0.52 and others close behind (see Table 3). Shortly, Trump’s community of acclamation is bigger, thicker, more intense, and more centered in his figure than Bidens.
4.2 Network polarization
The common ground that fosters deliberation and enables negotiation in real-world democracy diminishes with the loss of the few bridge nodes that connect two highly differentiated communities on social networks. In the polarized environment of the Twitter/X network, while acclamation within homogenous groups intensifies, the number of bridge nodes and their audiences decline. Only a minor segment, constituting 1%, engages in retweeting content from both Trump and Biden communities (see Figure 2). During the 2020 US presidential election, Twitter/X users active in both communities were exceedingly rare. Twitter/X’s recommendation algorithm effectively isolated these communities, fueling radicalization within them and exacerbating polarization across the network.
As previously noted, Figure 3 illustrates that the 75th percentile value for positive sentiment in external communities is significantly lower than that within the internal community. Currently, Figure 4 presents the negative sentiment scores related to mentions within and outside each community. It reveals that the low positive sentiment scores for external communities in Figure 3 reflect not indifference, but polarization. Using the median as a benchmark, it is evident that mentions directed outside the community tend to exhibit a greater degree of negativity than those within the community (see Figure 4). This dual-scope sentiment analysis provides insights into the emotional dimensions characterizing inter-community and intra-community interactions. Although pure authors constitute only 12% of these communities, the sentiment conveyed in their statements spreads to other users via retweets.
Overall, in the 2020 US presidential election, the dialogical debate integral to democratic deliberation in the real world was seriously undermined by the emergence of large echo chambers within the virtual world. These echo chambers emerge from highly centralized communities with dense interconnected nodes—isolated environments that foster dynamics of radicalization within the community and polarization toward those outside. Both communities were susceptible to radicalization and polarization, with mentions of the same users circulating within and strong negative sentiments directed at those outside, while positive sentiments were reserved for insiders. The bridges between the Trump and Biden communities were obstructed by mutual hostility.
Moreover, in these insular environments characterized by distrust and a lack of deliberation, positive sentiments from influential users within the community paved the way for the creation of shared ideas that diverged from reality but were positioned as truth. Given that the Trump community exhibited stronger levels of centrality and endogamy, it not only scored higher in radicalization and polarization than Biden’s community but was also more prone to believe fake news and conspiracy theories as we will see.
5 Fraud narratives, alternative truth, and democratic erosion
Conspiracy theories have long been intertwined with US politics, and they have become almost expected in the rhetoric of figures like Donald Trump. As Axelrod (2022) notes, Trump leaned into fraud allegations well before his reelection bid, declaring in August 2020 that “the only way we are going to lose this election is if the election is rigged.” Trump’s history of alleging fraud dates back even further when he claimed that his TV show “The Apprentice” was unfairly denied an Emmy. What was truly unprecedented in American democracy, however, was an outgoing president inciting a riot under the guise of electoral fraud, which resulted in the ransacking of the Capitol in a bid to prevent the certification of the newly elected president by Congress.
This situation was unprecedented because it marked the first democratic contest at the presidential level that played out in a mixed reality, where the mechanisms for establishing the validity of facts in the real world did not match with the narrative being driven on virtuality. When Georgia officials certified the election results in favor of Biden, when the press reported Trump’s improper requests to state officials, and when Attorney General William Barr dismissed claims of widespread voter fraud; simultaneously, on Twitter/X, @realDonaldTrump’s community retweeted thousands of baseless messages about election wrongdoing.
Trump’s personal misinterpretation of the electoral results led to the alignment of nearly the entire Republican Party. It also incited his followers to take to the streets, rallying under the banner of electoral fraud. This reaction of massifying subjectivity can be understood through the lens of how virtual reality constructs alternative truths.
In a democracy, truth emerges through dialogic discussion within a framework of constitutional and legal provisions. These provisions ensure the integrity of procedures and the credibility of the authorities involved. Deliberation, on the other hand, enables rational adjustment and moral alignment, guided by the principle of the best argument and the achievement of consensus. The result is an objective truth, in the sense that depicts a reality aligned with the views of a specific political community. This truth is grounded in empirical evidence debated through dialogue and is morally sound, as it is upheld by a consensus that is not imposed but freely agreed upon (Cortina, 2009).
In contrast, in virtual insular environments like the one of @realDonaldTrump vs. @JoeBiden the absent of deliberation undermines the sense of veracity of the intersubjective construction of reality. It legitimized an acclamation truth that results not only from the automatic machine patterns of centrality but also from the way users navigate in those conditions. In algorithmic communities instantaneously formed by users’ affinities such as Twitter/X, a post becomes a trend in the community as it receives more votes, this is retweets, comments, and likes. In other words, in acclamation communities, ideas gain legitimacy by elevating the vote to an absolute measure and dismissing deliberation. This approach is possible because the algorithm ensures the community’s homogeneity from the start, making further discussion unnecessary. Thus, acclamation truth is essentially an aggregative truth that gains a sense of reality through vote. This vote reflects the resonance of an idea that has been algorithmically parameterized as truth by its potential popularity. Acclamation can account for Weismueller et al. (2022) observation that emotionally charged content is more likely to be shared on platforms than content supported by well-founded arguments. Trump’s electoral fraud conspiracy theory, freed from the intersubjective bounds of deliberation and consensus-building, was positioned to appear not as fake news but as an alternative truth. This truth, paraphrasing Arendt (2024), seemed even more powerful because it claimed to expose the “real truth” hidden by the hypocrisy of arguments and corrupt democratic compromises. It was one more personal, more intuitive, more from the guts.
The discursive practice of acclamation tends to be more susceptible to embracing conspiracy theories, especially when centrality values are higher. For instance, within the @realDonaldTrump community, 75% of users demonstrate a support level of 33.33% or more for the election fraud narrative, while only 1.32% of tweets oppose this theory (see Figure 5).
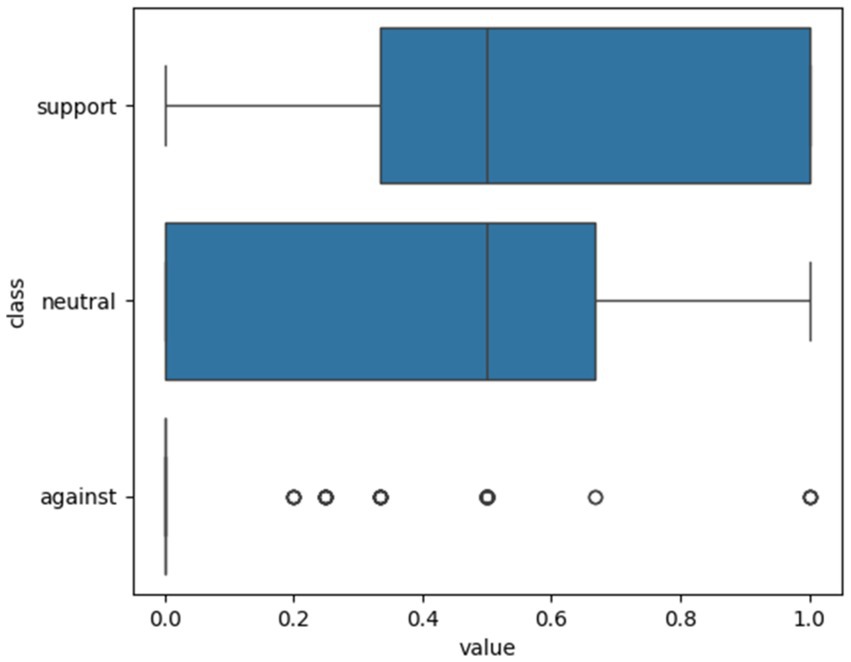
Figure 5. Distribution of the percentage of tweets inside the trump community that hold a stance of support, neutrality, or against electoral fraud story.
In contrast, within the @JoeBiden community, 50% of users produce tweets with a neutrality level of 66.66%. Additionally, 75% of users oppose the electoral fraud narrative at a level of 50%, and none support the conspiracy theory (see Figure 6).
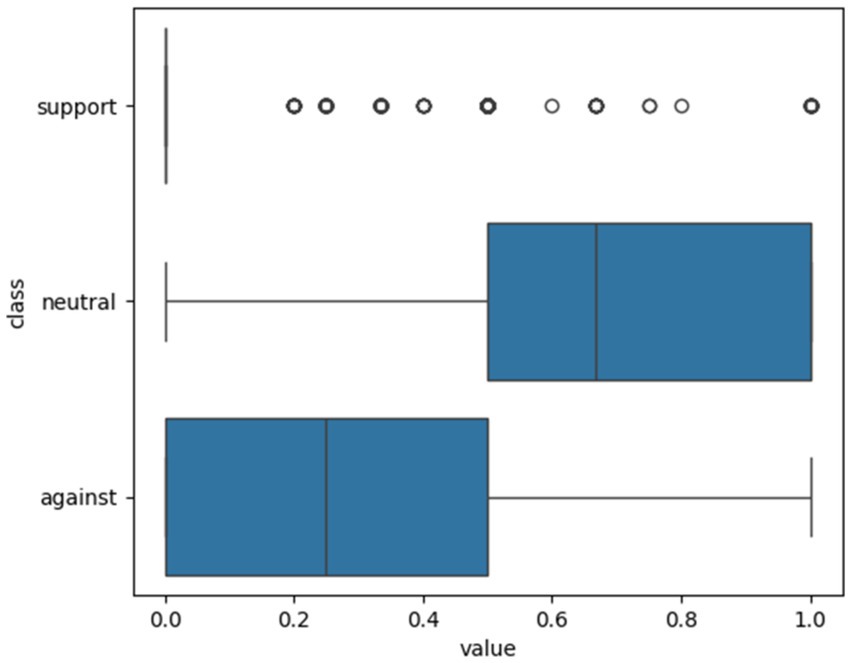
Figure 6. Distribution of the percentage of tweets inside the Biden community that hold a stance of support, neutrality, or against electoral fraud story.
Even more interestingly, an analysis of support percentages across centrality ranks within the Trump community, from rank 4 (the highest) to rank 1 (the lowest), reveals distinct patterns. Among the 50% of users in the highest centrality groups—those in ranks 3 and 4—over 60% of their tweets support the fraud theory. In contrast, among the 50% of users in the lowest centrality ranks—ranks 1 and 2—support drops to 50%. In short, to gain followers, influencers tend to become more radicalized (see Figure 7).
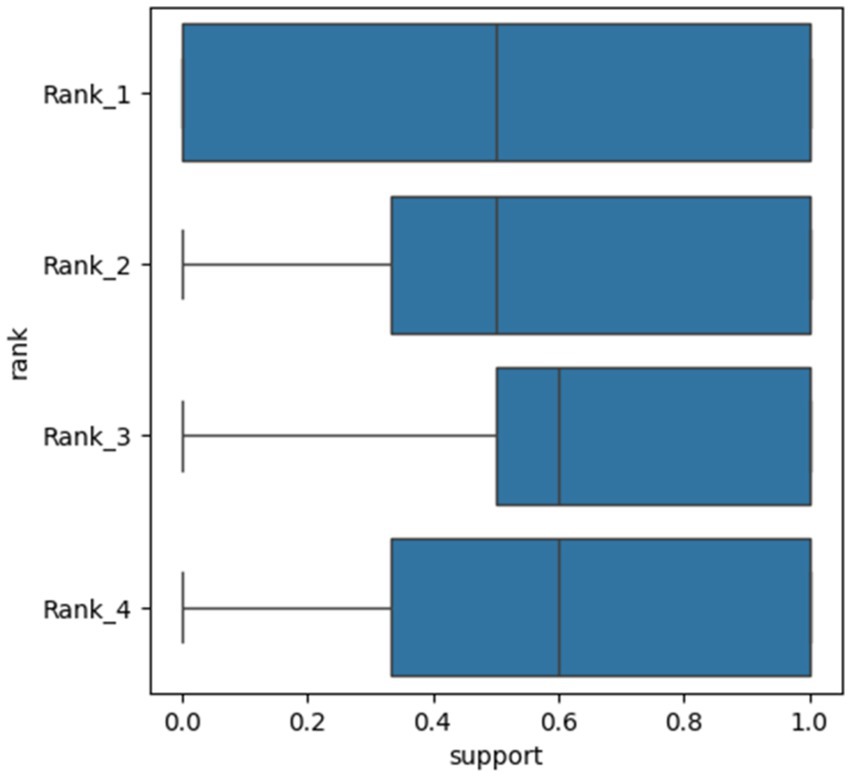
Figure 7. Distribution of support stance percentage inside the Trump community by centrality groups. Groups are organized as ranks, from the lowest to the highest.
Figure 8 illustrates the distribution of anti-fraud stance percentages within the Biden community, categorized by network centrality. In Rank 1, the 25th and 50th percentiles are identical, indicating that 50% of users have a 0% anti-fraud stance. The 75th percentile is at 50%, meaning that 75% of users with the lowest centrality show an anti-fraud stance of 50% or less. This pattern remains consistent in Ranks 2 and 3, though there is a shift in the 50th percentile, showing that 50% of users currently have more than a 25% anti-fraud stance. Rank 4, representing the highest centrality group, continues this trend, with the 50th percentile increasing slightly to 33.33%, while the 75th percentile remains at 50%.
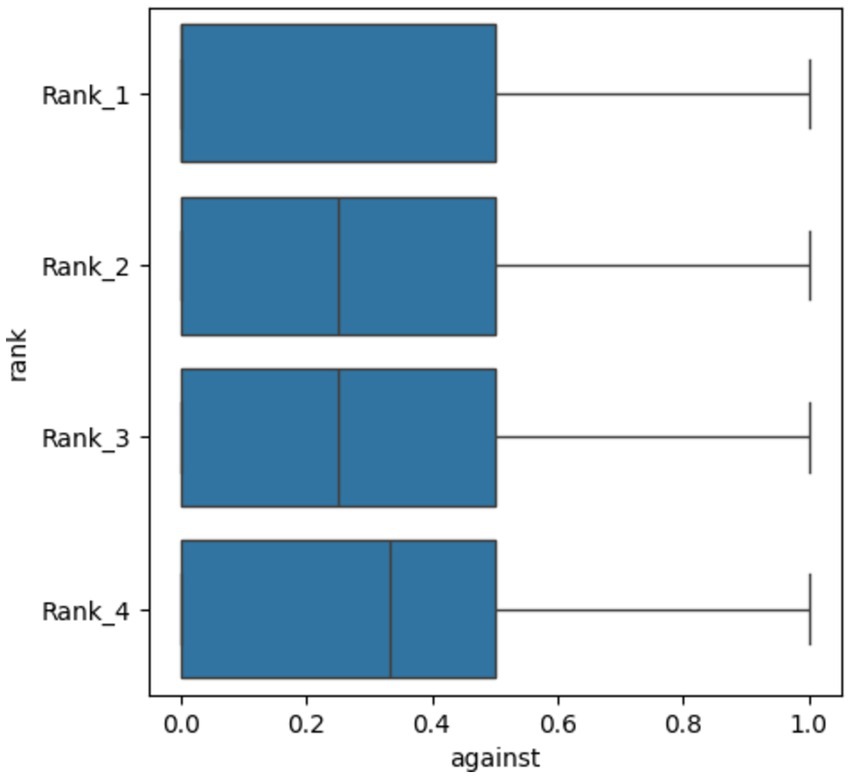
Figure 8. Distribution of against stance percentage inside Biden community by centrality groups. Groups are organized as ranks, from the lowest to the highest centrality.
6 Conclusion
The impact of ICT on politics is a subject of intense debate in political science, with perspectives ranging from utopian to dystopian. An analysis of Twitter/X communities during the 2020 US presidential election reveals that these platforms may have undermined American democracy. While some argue that these platforms allowed more ordinary citizens to engage directly and in real-time with the political process and its key figures, reality fell short of creating a modern-day virtual agora. The vast number of users did not translate into a broader plurality of voices enriching democratic discourse. Instead, users were algorithmically sorted into clusters based on their initial preferences, leading to isolated communities. As a result, among the 1,616 different communities identified, the @realDonaldTrump and @JoeBiden communities accounted for 88% of all nodes. What could have been a pluralistic deliberation was quickly transformed by algorithmic processes into conditions ripe for radicalization and polarization. In other words, the decline of traditional media editors has not liberated content from the monopoly of the media, nor has it returned the voice to ordinary people. Instead, it has merely concentrated these voices into clusters of like-minded individuals, making them more susceptible to influence under the logic of e-commerce.
The assault on the Capitol, which shocked the world, did not stem from a surreal story, though its bizarre chapters and bombastic figures might suggest otherwise. Instead, it was the inevitable denouement of an election process that ran in two separate realities: one virtual, where the persons entered as users and were algorithmically clustered like objects; and the other real, where individuals participated as citizens deliberating within institutional frameworks. What might be dismissed as surreal was, in fact, a clash within a mixed reality, where two different modes of Being-in-the-world—and thus two different approaches to establishing truth—came into conflict. This polarized society arises from the ubiquity in which it resides—a state no longer celebrated as a religious miracle, but rather as the defining condition of existence that shapes the very Being of a fractured democracy. Trump’s defeat marked a collision between users who took to the streets under the banner of a conspiracy theory, which had gained traction as an alternative truth through acclamation as practice, and citizens who use the practice of discussion over empirical results of the electoral process.
The empirical evidence presented in this study indicates that virtual insular environments promote radicalization and polarization. In these networks, communities with higher levels of centrality in their nodes, such as @realDonaldTrump, are more likely to adopt conspiracy theories, effectively “emancipating” themselves from empirical evidence. High centrality appears to be closely associated with the absolutization of the vote and the severe restriction of deliberation, reinforcing acclamation as a means of establishing truth.
Once again, 4 years after the 2020 presidential election, the United States reelected Donald Trump in a campaign marred by criminal convictions and assassination attempts, overshadowing the election much as allegations of fraud did previously. Perhaps the most troubling parallel, however, was that the campaign unfolded in the same virtual realm—driven by identical technologies and algorithms—reproducing the same patterns of behavior and user practices as before.
Algorithm-driven social media is not only influencing US elections but shaping electoral processes worldwide. To align ICT with democratic principles, civil society, governments, and international organizations must seriously debate the regulation of content on these platforms. This delicate discussion must balance the right to accurate information with the protection of free speech, while also addressing the creation of insular communities prone to radicalization and polarization. Simultaneously, educational initiatives should prioritize digital literacy, equipping citizens to critically evaluate information and resist manipulation.
Data availability statement
Publicly available datasets were analyzed in this study. This data can be found at: https://github.com/dgop92/the-crisis-of-the-liberal-international-order-repo.
Ethics statement
Ethical approval was not required for the study involving human data in accordance with the local legislation and institutional requirements. Written informed consent was not required, for either participation in the study or for the publication of potentially/indirectly identifying information, in accordance with the local legislation and institutional requirements. The social media data was accessed and analyzed in accordance with the platform's terms of use and all relevant institutional/national regulations.
Author contributions
GM: Conceptualization, Formal analysis, Funding acquisition, Investigation, Project administration, Writing – original draft, Writing – review & editing. AS: Conceptualization, Data curation, Formal analysis, Investigation, Methodology, Software, Supervision, Validation, Writing – review & editing. DP: Data curation, Methodology, Software, Visualization, Writing – review & editing.
Funding
The author(s) declare that financial support was received for the research, authorship, and/or publication of this article. Sources of funding received for this research come from Universidad del Norte’s Grant.
Conflict of interest
The authors declare that the research was conducted in the absence of any commercial or financial relationships that could be construed as a potential conflict of interest.
Publisher’s note
All claims expressed in this article are solely those of the authors and do not necessarily represent those of their affiliated organizations, or those of the publisher, the editors and the reviewers. Any product that may be evaluated in this article, or claim that may be made by its manufacturer, is not guaranteed or endorsed by the publisher.
References
Adler, E., and Drieschova, A. (2021). The epistemological challenge of truth subversion to the Liberal international order. Int. Organ. 75, 359–386. doi: 10.1017/S0020818320000533
Axelrod, T. (2022) A timeline of Donald Trump's election denial claims, which republican politicians increasingly embrace from 2016 to today, Trump made election criticism a key part of his campaigns. ABC News. Available at: https://abcnews.go.com/Politics/timeline-donald-trumps-election-denial-claims-republican-politicians/story?id=89168408
Barash, R. E. (2022). Social media as a factor forming social and political attitudes, the Russian context. Monitoring of Public Opinion: Economic and Social Changes. 2, 430–453. doi: 10.14515/monitoring.2022.2.1980
Barbera, P., Jost, J. T., Nagler, J., Tucker, J. A., and Bonneau, R. (2015). Tweeting from left to right: is online political communication more than an Echo chamber? Psychol. Sci. 26, 1531–1542. doi: 10.1177/0956797615594620
Bihari, A., and Pandia, M. K. (2015). “Eigenvector centrality and its application in research professionals' relationship network,” in International Conference on Futuristic Trends on Computational Analysis and Knowledge Management (ABLAZE), Greater Noida, India, 2015, 510–514.
Chen, E., Deb, A., and Ferrara, E. (2022). #Election2020: the first public twitter dataset on the 2020 US presidential election. J. Comput. Soc. Sci. 5, 1–18. doi: 10.1007/s42001-021-00117-9
Cortina, A. (2009). Las Políticas Deliberativas de Jurger Habermas: Virtualidades y Límites. Rev. Estud. Polít. 144, 169–193.
Elster, J. (1998). “Introduction” in Deliberative democracy, compiled by Jon Elster. ed. J. Elster (Cambridge: Cambridge University Press), 13–33.
Fileborn, B., and Loney-Howes, R. (2019). #MeToo and the politics of social change. London: Palgrave Macmillan.
Finn, S., Mustafaraj, E., and Metaxas, P. T. (2014). The co-retweeted network and its applications for measuring the perceived political polarization. Proceedings of the 10th International Conference on Web Information Systems and Technologies 2, 276–284. doi: 10.6084/M9.FIGSHARE.1383259
Guttmann, A. (2023). Time spent with digital vs. traditional media in the U.S., 2011-2025. Statista. Available at: https://www.statista.com/statistics/565628/time-spent-digital-traditional-media-usa/ (Accessed May 23, 2024).
Habermas, J. (1984). The theory of communicative action, I. Reason and the rationalization of society. Boston: Beacon Press.
Iyengar, S., and Hahn, K. (2009). Red media, blue media: evidence of ideological selectivity in media use. J. Commun. 59, 19–39. doi: 10.1111/j.1460-2466.2008.01402.x
Jackson, S. J., Bailey, M., and Foucault Welles, B. (2020). #HashtagActivism: networks of race and gender justice. Cambridge: MIT Press.
Kemp, S. (2020). Digital 2020: the United States of America : Datareportal Available at: https://datareportal.com/reports/digital-2020-united-states-of-america#:~:text=There%20were%20288.1%20million%20internet,%25)%20between%202019%20and202020.
Kennedy, I., Wack, M., Beers, A., Schafer, J., Garcia-Camargo, I., Spiro, E. S., et al. (2022). Repeat spreaders and election Delegitimization. Election. J. Quant. Descript. 2, 1–49. doi: 10.51685/jqd.2022.013
Lan, X., Gao, C., Jin, D., and Li, Y. (2023). Stance detection with collaborative role-infused llm-based agents. arXiv preprint arXiv:2310.10467
Milgram, P., Takemura, H., Utsumi, A., and Kishino, F. (1994). Augmented reality: a class of displays on the reality-Virtuality continuum. Proc. SPIE Int. Soc. Opt. Eng. 2351, 282–292.
Mounk, Y. (2018). “The people vs” in Democracy. Why our freedom is in Danger & how to save it (Cambridge: Harvard University Press).
Ohme, J. (2021). Algorithmic social media use and its relationship to attitude reinforcement and issue-specific political participation – the case of the 2015 European immigration moventments. J. Inform. Technol. Polit. 18, 36–54. doi: 10.1080/19331681.2020.1805085
Pariser, E. (2011). The filter bubble: how the new personalized web is changing what we read and how we think. New York: Penguin Press.
Schwartz, S. A., Nelimarkka, M., and Larsson, A. O. (2022). Populist platform strategies: a comparative study of social media campaigning by Nordic right-wing populist parties. Inform. Commun. Soc. 26, 1–19. doi: 10.1080/1369118X.2022.2147397
Shahin, S. (2023). Affective polarization of a protest and a Counterprotest: million MAGA March v. Million Moron March. Am. Behav. Sci. 67, 735–756. doi: 10.1177/00027642221091212
Thiele, L. P. (2020). Politics of technology-specialty grand challenge. Front. Polit. Sci. 2, 1–3. doi: 10.3389/fpos.2020.00002
TIBCO. (2023). What is unsupervised learning? Available at: https://www.tibco.com/es/reference-center/what-is-unsupervised-learning (Accessed November 18, 2023).
Tufecki, Z. (2017). Twitter and tear gas: the power and fragility of networked protest. New Haven: Yale University Press.
Twitter/X. (2023). Twitter's recommendation algorithm. Available at: https://blog.twitter.com/engineering/en_us/topics/open-source/2023/twitter-recommendation-algorithm
Van den Heuvel, M. P., and Sporns, O. (2013). Network hubs in the human brain. Trends Cogn. Sci. 17, 683–696. doi: 10.1016/j.tics.2013.09.012
Vosoughi, S., Roy, D., and Aral, S. (2018). The spread of true and false news online. Science 359, 1146–1151. doi: 10.1126/science.aap9559
Weismueller, J., Harrigan, P., Coussement, K., and Tessitore, T. (2022). What makes people share political content on social media? The role of emotion, authority and ideology. Comput. Hum. Behav. 129:107150. doi: 10.1016/j.chb.2021.107150
Zhuravskaya, E., Petrova, M., and Enikolopov, R. (2020). Political effects of the internet and social media. Annu. Rev. Econ. 12, 415–438. doi: 10.1146/annurev-economics-081919-050239
Keywords: US democracy, US election, ICT—information and communication technologies, social networks, critical theory, democracy
Citation: Morales G, Salazar A and Puche D (2025) From deliberation to acclamation: how did Twitter’s algorithms foster polarized communities and undermine democracy in the 2020 US presidential election. Front. Polit. Sci. 6:1493883. doi: 10.3389/fpos.2024.1493883
Edited by:
Adolfo A. Abadía, ICESI University, ColombiaReviewed by:
Sulaimon Adigun Muse, Lagos State University of Education LASUED, NigeriaTiago Silva, University of Lisbon, Portugal
Copyright © 2025 Morales, Salazar and Puche. This is an open-access article distributed under the terms of the Creative Commons Attribution License (CC BY). The use, distribution or reproduction in other forums is permitted, provided the original author(s) and the copyright owner(s) are credited and that the original publication in this journal is cited, in accordance with accepted academic practice. No use, distribution or reproduction is permitted which does not comply with these terms.
*Correspondence: Gustavo Morales, Z3ZlZ2FzQHVuaW5vcnRlLmVkdS5jbw==