- Tecnologico de Monterrey, Monterrey, Mexico
False information flows through social media are a worldwide phenomenon, affecting Latin America in particular, where more than 70% of internet users report growing concern regarding the circulation of fake news, especially through social media platforms. Although the academic literature has studied how governments may be incentivized to disseminate false information, it has concentrated in the electoral context mostly. In this sense, the impact of disinformation on mass mobilization patterns has been less explored, although it is relevant: manipulation through social media can reduce citizens' inclination to protest by directly influencing their preferences or beliefs about the government, acting as a preventive digital repression tool. In fact, governments have efficiently used it in the short run; however, it has not been established if this strategy could work in the long run. Hence, this article explores the long-term relationship between manipulation by the government through social media and the occurrence of mass mobilization to determine whether they are cointegrated. Drawing on the most recent literature on digital repression, manipulation, and mass mobilization and by applying a panel cointegration methodology in nine countries in Latin America (2000–2021), we find that the variables are cointegrated. In addition, we explore the causal (Granger) effect and find a unidirectional link that goes from social media manipulation to mass mobilization.
Introduction
Disinformation (false information created and spread with knowledge of its inaccuracies) is a potent tool that could be used to manipulate and possibly control someone's behavior (Bastick, 2021). In a political environment, manipulation through disinformation (i.e., spreading fake news through social media) could be a potent tool for a politician or government intending to stay in power. The academic literature has extensively analyzed the effects of digital manipulation (through social media) on voting behavior (Pascal Zachary, 2020), in particular, the need for politicians to influence potential voters through cues and signals that convince them to vote in a desired way (Bradshaw and Howard, 2018; Wilson, 2019). In the Cambridge Analytica scandal, using specific targeting through Facebook, false statements were presented to social media users as facts. Once in power, politicians could also be willing to perform some kind of manipulation to assure reelection (Matusitz and Simi, 2021).
Beyond elections, to what end would a politician or a government be interested in manipulating its own population? Intuitively and in plain terms, this could be because the government wishes to conceal sensitive information, often related to negative performance, or to blame political opponents while crediting supported parties with respect to issue positions (Jackson, 2017; Wardle, 2017; Balod and Hameleers, 2021). All in all, controlling public opinion could be crucial for avoiding legitimacy erosion. Moreover, in face of an active and engaged civil society, the government may wish to avoid episodes of protest or mass mobilization developing under a logic of accountability.
The latter is not a minor issue, especially in regions like Latin America, where protests have increased in recent decades (Bellinger and Arce, 2011). Episodes such as the mass mobilizations in Ecuador, Chile, and Colombia in 2019 (Aravena Lavín, 2022) and the constant protests in Nicaragua (Martí i Puig and Serra, 2020) empirically demonstrate that “street politics” are (or should be) an important concern for politicians, especially as such movements can be source of constant political mobilization and power and have successfully toppled governments in the past (Silva, 2015).
As mass mobilization emerges, a government could call on direct or offline repression to contain it. However, doing so is very costly: (1) the government needs to deploy police and military forces and (2) it then needs to contain the consequences of the repression, which will create high unpopularity once it is witnessed by the citizenry, especially if the mass mobilization is peaceful. This could make direct repression counterproductive, revealing the government as incompetent and highly repressive. Furthermore, repression could spur further dissent, ultimately failing to serve its intended purpose of dissuasion (Goldstone and Tilly, 2009).
Due to these counterproductive consequences of direct repression, it would seem to be more desirable for a government to prevent protests from occurring in the first place. If governments were to follow this strategy, what alternative mechanisms might they use?
Thanks to the emergence of social media, subtler means of influencing the production and consumption of information can be used by politicians and governments. These tools could frame information in a way that disincentivizes people from engaging in public demonstrations in the first place. For example, in response to social unrest, the government could portray key protesters as destabilizers of public order to prevent people from joining their cause. According to this logic, social media could be deployed as a preventive digital tool for repression: through social media, the government can spread false information to manipulate public opinion and prevent protests from beginning in the first place.
It has been shown that the latter strategy works over the short run for specific episodes of discontent and to influence near-term electoral outcomes (Bradshaw and Howard, 2018). This article investigates the relationship between the manipulation of information by the government through social media and mass mobilization to evaluate its effectiveness over the long run. This is an especially valuable question where governments around the world have dramatically increased their efforts to manipulate information on social media.
The remainder of this article is organized as follows: the next section develops a theoretical framework that links literature from political science, communications, and sociology to understand why popular movements arise, how governments respond, and how we can understand the role of manipulation through social media within these dynamics. After this, we present a literature review discussing the main contributions on this topic, concentrating on Latin America. The following section applies a panel cointegration methodology to study the possible long run relationships between those variables. Data are drawn from the Varieties of Democracy project and cover nine Latin American countries from 2000 to 2021. The paper finds a positive relationship between manipulation through social media and mass mobilization over the long run, which implies that increasing levels of manipulation through social media increases the occurrence of mass mobilization. Finally, a conclusion is provided that discusses the possible explanations of these findings and proposes new avenues for research.
Under what contexts and why do people mobilize?
Do people mobilize in democracies or in autocracies?
In general terms, people take the streets in demonstrations, strikes, and sit-ins to obtain a benefit (material or otherwise), to show disagreement with a policy or specific event, or to call for compliance with a demand (Brancati, 2014; Zysberg, 2018; Choi, 2020). Thus, mobilization could occur in either a democratic or an autocratic environment, as no government could pre-empt the populace by always filling every societal demand. While in closed autocracies, social protest is uncommon (Trejo, 2014); the capacity to organize mass mobilizations is present in both electoral autocracies and democracies.
The existence of a vibrant civil society cannot be solely ascribed to a specific type of regime. Academic studies have found that protests are ever-present, regardless of the nature of the regime they are under (democratic or electoral autocracy); however, the governments have varied responses to protests (Carey, 2006). The common nature of protests is easily understood: it is virtually impossible to agree on every issue, and civil society actors take the streets to manifest their discontent. In democracies, people have the freedom to mobilize, and it could be argued that this freedom demonstrates a level of democratic attainment; in this sense, mass mobilizations could be a proxy for the freedom of expression allowed in a society. Furthermore, under democracy, institutional arrangements could influence mass mobilizations, such that protesters in democratic countries with a weak legislature find it difficult to present their demands to the government. This could make them more inclined to protest than those in countries with a strong legislature (Nam, 2007). In electoral autocracies, on the other hand, mass mobilization could be among the few available tools used to demand radical change.
Latin America has experienced increased protests and civil unrest over the last decade (Justino and Martorano, 2016); the heterogeneity of the economies and political systems in this region indicates that civil society is using protests for various reasons that are not necessarily linked to the type of regime. That is, we can empirically observe protest events that occurred in countries with different sociopolitical characteristics. For example, in 2019, Colombia and Chile witnessed massive mobilizations begun by students, labor groups, and apolitical groups, while in Nicaragua Ortega's autocratic consolidation was protested. Here we should note in these episodes that in all three, people used the streets to canalize their concerns and make the government accountable. These actions can be effective: protests in the region have led to the ouster of presidents in many countries: in particular, between 1992 and 2016, 15 presidents in nine countries were removed in the region (Pérez-Liñán, 2014). Given the capacity for mobilization and the region's heterogeneity, Latin America can provide an interesting area to explore the dynamics of mass mobilization.
Motivations
Participation in a mobilization or protest is a conscious action, undertaken to gain some benefit at some cost. Thus, wanting to participate in a protest or having a reason for doing so is a precondition, as the participation cannot exist without a specific motivation, whatever that might be. Regarding specific motivations, the literature has distinguished between the nature of a motivation and its reach. Thus, there are two different types of motivations: instrumental and expressive (Walgrave et al., 2013).
The motivations for and benefits obtained from protesting are various, including desired change in a specific policy, the rewarding feeling of supporting a friend, or even receiving payment for showing support of a specific cause; hence, it could be useful to think about protest in terms of an instrumental action (performed to obtain something specific, often external) as well as of a mechanism for channeling emotion (to express an inner emotion: anger, empathy, support, or even feeling better). The literature holds that people tend to participate out of a mixture of internal and external orientations: the participants at protests' participants tend to share interests and values (instrumental motives) as well as feelings and emotions (expressive motives).
Further, protesters can be motivated by individual or collective motives (Van Zomeren and Spears, 2009). In other words, people can make claims or express their emotions or ideologies in their own name or in the name of a group. The distinction between individual and collective motives is gradated and not absolute, as most protest participants probably both want to improve their own lives, as well as sharing their grievances with others (Zoll et al., 2018). It is thus a matter of degree whether motives are individual or collective. Both individual and collective motives are permeated instrumental and emotional dimensions, leading to four distinct types of motivation: instrumental-collective (our interests), instrumental-individual (my interests), expressive-collective (our grievances), and expressive individual (my grievances).
Contextualizing mobilization in Latin America in the last two decades
Latin America is a vibrant region in terms of its social movements and mass mobilization events. Since this paper covers a period of 21 years (2000–2021), known as the post-neoliberal period (Munck, 2020), this section aims to contextualize the region to better understand the dynamics it faces.
After 2000, several countries in Latin America witnessed the rise of left-of-center governments because of mass mobilizations and protests (Prevost et al., 2012). The intensification of such events was a response to the austerity measures imposed by neoliberal governments, especially since the 1980s. Consequently, people took to the streets to demand better quality of life and the respect of basic rights, especially challenging privatization measures imposed during those years. In this sense, the streets became a scenario in which people could keep their governments accountable. Examples of these episodes may include: the War on Water in Bolivia (2000), the Forajidos protests in Ecuador (2005), the “Corralito” in Argentina (2001), or the generalized protests to impede the instauration of a trade agreement between the USA and Latin America.
Once Latin America entered the Pink Tide, people started to protest demanding third generation rights, i.e., focused on cultural and environmental rights mostly. In terms of important episodes of mass mobilization, we may include anti-extractivism demands in Ecuador, Panama, Colombia, Bolivia, and Peru. Notably, the feminist movements staged important mobilization campaigns demanding abortion legalization and the expansion of women's rights; these protests were especially visible in Argentina, Chile, and Mexico. Although protests demanding third generation rights were prominent, political, civil, and socioeconomic themed mobilizations continued to occur, including protests against Maduro's regime in Venezuela (2014, 2019), general protests in Colombia, Chile and Ecuador in 2019, protests in Peru (2022) or protests in Nicaragua (2018).
An important change in mobilization patterns started to occur because of the availability of technological tools, which became an important asset for protests' organizers, for the government that aims at containing them, as well as for staging important repertoires of collective action. Among these technological tools, an Internet's penetration of 78% is key to understanding these new dynamics as it creates a potent resource for organization both online and offline. In fact, using the Internet, especially social media, for political purposes significantly increases protest chances (Valenzuela et al., 2016).
Regarding the potential of the Internet as a repertoire of collective action, the feminist movement staged a coordinated campaign through social media platform to make a dance performance of the feminist hymn “El violador eres tú” (You are the rapist), an original song written by Las Tesis organization that attained important international attention and positioned Chile as a reference of the feminist movement. Similar initiatives take place locally, through the promotion of hashtags in X, to visibilize specific demands. For example, #PaseDeLista1al43 was used for network gatekeeping purposes in the Ayotzinapa disappearance case in Mexico in 2014 (Galarza Molina, 2019).
In terms of how the government may use digital tools to counteract protesters' online and offline activities, the following section will offer an overview of such dynamics.
How does the government respond when people mobilize?
When people in a country stage mass mobilizations or protests, the government may take a variety of responses: call for dialogue to address demands and assign them to pertinent institutions, ignore the protests until the people disperse or the movement weakens, or repress protesters using one of several mechanisms. The literature recognizes that there are a range of possible responses to protests, depending on the nature of the regime (O'Brien and Deng, 2017): while a democratic regime may be more likely to make concessions in the face of a protest mobilization, they will also tend to make use of subtle forms of social control that falls short of direct repression, due to the costs involved. Autocratic governments, on the other hand, are more likely to directly repress protests against incumbents that claim legitimacy based on the person of the leader (Keremoǧlu et al., 2022).
Whatever response the government employs, its main objective is to control the effects of protests, as their mere existence could signal a shortcoming to the government's performance. Johnson and Thyne (2018) and others have shown that protests signal the illegitimacy of a regime, which, in turn, incentivizes coups. Thus, governments are interested in portraying themselves in a positive light, as this provides various benefits: increased chance of reelection, strengthening the incumbent's party, and/or guaranteeing governance in general. In all these scenarios, it is assumed that the government wishes to stay in power.
The literature indicates that when governments feel threatened by protests, they will use all the tools at their disposal to remain in power and maintain the status quo (Regan and Henderson, 2002; Carey, 2006). In some cases, the government may select a physical repression tool, but in others, it may choose a digital repression tactic, or a combination of both. Governments' responses to dissent reflect the nature of their regime and the potential costs of the policy options they choose, their impact on future dissent as well as their capacity to implement digital or direct repression (Franklin, 2009; Sullivan, 2016). For example, in her study on Syria, Gohdes (2020) demonstrates higher levels of Internet accessibility granted by the government are significantly and substantially associated with a more targeted strategy of regime physical violence (direct repression). In contrast, where Internet access is limited or shut down, the Syrian government employs a significantly more indiscriminate campaign of physical violence.
The most efficient, although costly tool (in terms of resources needed and impact to the government's popularity), for controlling mass mobilization is direct/analog repression. Repression has been used by democratic and autocratic governments (although at different levels); it often consists of deploying police or military forces in the streets where the protests are occurring or of incarcerating key members of social movements. Its moves are all directed at undermining resistance in general. It is precisely because the dissent is threatening that governments repress protesters to maintain their authority.
The latter assumption is grounded in studies focusing on government coercion, often referred to as the “threat-response theory” (Earl et al., 2003). On this understanding, governments employ higher levels of repression to contain increased levels of mass mobilization. This theory builds on the assumption that governments respond to overt collective challenges to the regime by engaging in repressive behavior to control or eliminate the challengers (e.g., Pion-Berlin, 1989; Davenport, 1995; Earl et al., 2003). The violent repression of the Nicaraguan protesters in 2018–2019 illustrates the latter goal.
Empirical evidence shows that repression, conceived of in such a way, could be counterproductive for governments, raising serious criticisms, both internally and internationally, and could raise serious doubts on the legitimacy of the government. More specifically, governments must consider two types of costs. The first are production costs (including salaries to soldiers, uniforms, and equipment) and, on the other hand, transaction costs (the political and economic consequences derived from repression). Here, it can be observed that transaction costs can be especially sensitive, as the government could face international condemnation or economic sanctions as a result.
Governments may not only consider the visible challenges, but also the hidden or potential ones to better anticipate and prevent the emergence of significant collective mobilizations. They can achieve this by closely observing their citizens, recognizing the signs that indicate the formation of the most severe collective challenges. Some examples of observable indicators include those mentioned in studies like Davenport (1995) and Earl et al. (2003), while unobservable or latent probabilities of challenges can be detected through research such as Herreros and Criado (2009), Nordas and Davenport (2013), and Danneman and Ritter (2014).
Governments recognize that the outcome of repression that directly targets overt collective challenges is highly unpredictable (e.g., Davenport et al., 2005). At the same time, the repression of those who are not directly engaged in challenges is costly, as it involves large resource allocations, the possible sacrifice of human life, and potential political backlash. Governments must balance the costs of repression designed to weaken or eliminate the development of a challenge to their power against (1) the probability of a threat being realized as an overt collective challenge and (2) the probable costs of dealing with those challenges, should they materialize.
Another dimension to consider is the nature of the protest, i.e., the level of violence that the protesters use. If the protesters are violent, the likelihood of repression increases, and governments tend to follow a somewhat linear path, meeting protester violence with state violence (Carey, 2006; Franklin, 2009), but they may also consider other attributes of a protest, such as the number of its participants (DeNardo, 1985; Earl et al., 2003; Biggs, 2018), the nature of its demands (Regan and Henderson, 2002), and differences in protest tactics or from “normal” dissent (Davenport, 1995). However, repression is not an automatic response to protests, even violent ones, as it is risky and costly (Pierskalla, 2010).
Thus, due to these high costs, governments may wish to act before protesters take to the streets, for two reasons: governments will save the cost of direct repression, and the government will not be questioned. Here, the ideal situation would be that the government behaves as expected (and meets the demands) but when the government is not able to do so, it could employ other, less costly strategies to prevent the emergence of mass mobilizations.
In this sense, digital repression tools are available for both democracies and non-democracies, and although non-democratic governments, such as China, frequently employ surveillance technologies, the key users of these technologies are liberal democracies, such as the United States. Furthermore, democracies engage in almost all kinds of digital repression tools (Earl et al., 2022).
When direct repression is too costly: using social media as a preventive digital repression tool
Digital repression is costly, but less costly when employed to prevent a mass mobilization from occurring. Governments have important reasons to avoid using direct repression when controlling mass mobilizations. However, they need to create alternative strategies to contain the emergence of protests. The literature on state repression includes work by a growing body of scholars who focus on analyzing the role of social media in the dynamics of repressing mass mobilization.
Broadly speaking, governments can draw upon digital media to contain mass mobilization through information coercion and through information channeling. In information coercion, governments can develop means of controlling information by limiting access or content and through the use of overt or covert mechanisms. For example, governments can use digital surveillance to harvest intelligence to target coercion. While traditional (analog) surveillance is often used against specific targets or smaller groups of targets, digital repression allows surveillance to happen at scale when done at an internet backbone or at the level of an internet service provider, which provides regimes with unprecedented windows into public discontent, social movements, and even lower-level state functionaries whose incompetence or corruption may incite social unrest.
In information channeling, governments can influence the production and consumption of information using social media. As direct/analog repression is very costly, it is recognized that unnoticed acts or those done in ways that make the connection to the protest harder to discern are likely more practical and effective; in this realm, social media has enormous potential. As with information coercion, both overt and covert mechanisms could be employed. Overt strategies would include government-linked accounts posting distracting information and/or flooding online spaces or hashtags with irrelevant material, and covert strategies include creation of disinformation and/or misrepresentations that would influence the perception of key topics by social media users. These strategies are considered preventive digital repression tools given their potential for containing the emergence of protests.
Taking these various approaches into account, it is preferable for governments to create mechanisms to hinder mobilizations before they occur, as this would allow them to avoid two sets of related costs: (1) the costs of containing the protest through direct repression and (2) the costs of containing the negative image of the government that protests create through preventive digital repression. Governments have developed a range of mechanisms that take the costs into consideration.
For the costs related to direct repression, preventing the need for mass mobilization means that the police or military do not need to be deployed, and important resources will be saved. Because direct repression is only applied once a mobilization is already taking place, it is used as a post-mobilization mechanism. On the other hand, a second set of costs influences both the pre-mobilization government's image and the post-mobilization government's image, in the sense that through preventive digital repression, the government can redirect and control the narrative of the protest or, ideally, could prevent the protest from occurring at all.
In this sense, digital repression is a tool that allows governments to save the negative costs of mass mobilization and direct repression, however, digital repression has specific costs, specifically those related to implementing the technological platforms needed for surveillance, bots acquisition and servers. However, as digital repression could be aimed at preventing mass mobilizations from occurring in the first place, it could be a mechanism to prevent bigger costs in the future, i.e., costs related to direct repression, the government's image, and possible reelections.
Given that the ideal outcome for governments is to avoid the emergence of mass mobilization, it is safe to assume that governments tend to employ strategies that allow them to control or redirect mobilization episodes before they occur. The following section examines the patterns of use of such mechanisms and strategies in Latin America.
Social media and mass mobilization: evidence from Latin America
Manipulation of public opinion over social media platforms is a critical threat to public life. Around the world, a range of government agencies and political parties are exploiting social media platforms to spread junk news and disinformation and to exercise censorship and control, undermining trust in the media and the public. Latin America is not an exception to these trends. In fact, recent studies have shown that concern regarding the risk of false information circulating through the internet is highest in the Latin America and the Caribbean region (74.2% of internet users); while it is far lower in South Asia (32.2%) and Central Asia (38.1%) (Knuutila et al., 2022).
Latin America features high levels of political participation. In 2019, Ecuador, Chile, Colombia, and other countries witnessed protests demanding better opportunities and economic equality. These grievances were exacerbated by the dynamics of the pandemic, which posed more challenges to a situation that already seemed to be at its limit (Busso and Messina, 2020). Even before the pandemic, Latin America faced several crises that could be used to explain the constant existence and relevance of mass mobilization (Grigera, 2022). What the COVID crisis showed was the potential of social media to manipulate public opinion and to affect, among other things, mass mobilization patterns. More concretely, governments used specific strategies to control and repress mass mobilization: censorship and mass surveillance measures, manipulation through disinformation campaigns and internet censorship.
Regarding manipulation through disinformation campaigns, Piña-García and Espinoza (2022) and other authors have shed light on how the Mexican government employed coordinated Twitter campaigns to manipulate online discourse during the pandemic. In other countries, such as Ecuador, the government sought to inhibit emerging of protests by enacting a state of emergency that prohibited mass mobilization through the coordination of online campaigns that amplified these messages and restrictions on the freedom of the media (Edgell et al., 2020). Furthermore, Bradshaw et al. (2020) report that governments deploy organized social media manipulation through the coordination of fake accounts, troll farms, or creating and expanding political propaganda supporting the government. Specific findings for Ecuador, Colombia, Peru, and Bolivia suggest that social media manipulation is directed toward creating narratives to burnish the image of the governments, a useful strategy for disincentivizing mass mobilization. More concretely, López-Paredes and Carrillo-Andrade (2022) argue in a study of Ecuador's October 2019 protests, that governmental Twitter accounts were instrumental in disseminating conflicting narratives and authorities used storytelling to shape processes as legitimate or not through video, text, and image interaction.
Following this line of thought, Carter and Carter (2021) find that propaganda diminishes the rate of protest: by increasing the level of pro-regime propaganda by one standard deviation, autocrats can reduce the odds of protest the following day by 15%. The half-life of this effect is between 5 and 10 days, and very little of the initial effect persists after 1 month. This temporal persistence is remarkably consistent with the findings for campaign advertisements in democracies. Although it may not be centered in social media, Deibert et al. (2010) argue that online propaganda interestingly translates historical offline tendencies.
Munger et al.'s (2019, p. 815) study of the La Salida/anti-Maduro movement showed that governments may “advance many competing narratives that [address] issues unrelated to the opposition's criticism” in the hopes of flooding social media platforms and diverting attention from information that cannot be directly suppressed. In Mexico, so-called Peñabots promote fake trends to distract and drown out government criticism (Porup, 2015). Elections and social movements may cross over, as occurred when Peñabots sought to distract from the emergence of the #YoSoy132 movement around the 2012 Mexican general elections (Baker, 2015). Governments may also seek to drown out messages that they oppose by flooding digital spaces with spam. In Mexico, Suárez-Serrato et al. (2016) report an influx of spam tweets in response to the #YaMeCanse hashtag protesting government complacency in the disappearance of more than 40 student activists.
Regarding censorship and mass surveillance, the 2019 mass protests in Ecuador witnessed a clear-cut censorship toward independent media and citizens that were reporting human rights violations committed by the government during the October strike. Specifically, On October 9, Pichincha Universal radio received a directive from the Presidency's Communication Secretariat to indefinitely align with the public radio signal. This decision was later superseded by a judicial request for broadcasting suspension issued by the Telecommunications Regulation and Control Agency as a provisional precautionary measure. Additionally, the radio station's premises were reportedly raided on allegations of “incitement to discord among citizens.” This incident reflects a complex interplay between governmental control and legal measures affecting media outlets, highlighting potential challenges to freedom of expression (CIDH, 2020).
Strategies such as Internet censorship are evidenced in the region as well. For example, Ecuador's data from V-Dem reveals a nuanced stance on internet censorship, media control, and journalist harassment. There are indicators that depict selective blocking of politically sensitive sites. Similarly, media censorship suggests a routine of indirect censorship, highlighting a tightening grip on press freedom over the years. While some journalists face pressure to halt publications when their content annoys powerful actors, others can practice journalism freely in the long run. In essence, Ecuador's data underscores a complex and evolving landscape, with positive shifts tempered by ongoing concerns about routine media censorship and selective internet content blocking (Puyosa and Chaguaceda, 2017).
Using manipulation as a mechanism to contain mobilization: how does it work?
Manipulation through digital strategies is a tool used by governments, but the relationship between social media and protest has been explored to determine whether the use of social media inhibits or fosters protest. Existing academic studies trying to assess this relationship often focus on specific scenarios fixed in the short run. For example, Valenzuela et al. (2016), examining Latin America as a whole, find that using social media for political purposes significantly increases the tendency to join protests, as the second strongest predictor. Additionally, the use of social media reduces the protest gaps associated with individuals' age, gender, psychological engagement with politics, and recruitment networks. Furthermore, Salzman (2015) and others confirm that the presence of a strong relationship between social media use and protest participation. Similarly, Masías et al. (2018) study the impact of social networking sites and confirm interplay between the use of these sites and participation in protests.
The specific effects of manipulation using social media have been generally explored in the context of elections. For Latin America, case studies have been conducted relating the use of social media for manipulation for elections—for example, Abdin (2019) analyzes Brazil; Ackerman et al. (2020) study Ecuador; Aguilar (2018) studies El Salvador. While these studies find that manipulation using social media is a potent tool for winning an election, Saldivar et al. (2022) challenge this; drawing on an analysis of the use of Twitter during the 2017/2018 presidential elections in Paraguay, they conclude that this network did not correlate with elections results.
Studies of manipulation through social media and the persistence of protests are relatively scarce. The reasoning for considering a relationship here is that political propaganda can reduce citizens' inclination to protest by directly influencing their preferences or beliefs regarding the government. However, this relationship has only been explored for specific junctures and for the short run. In this sense, digital suppression strategies offered by the Internet in general, and by social media in particular, might be able to hinder mobilization through specific mechanisms.
The first mechanism is related to the development of positive narratives for the government, which is key to conceal critical problems that could be occurring. By doing so, the government could prevent the emergence of mobilization but only momentarily: if the problem persists it would be impossible to contain it only through misinformation and manipulation. Gunitsky (2015) argues that autocratic regimes proactively subvert and co-opt social media for their benefit by selectively divulging information that reinforces the government popularity. Rather than being an obstacle, social media is increasingly used as a tool for counter-mobilization, discourse framing, preference divulgence, and elite coordination.
A second mechanism in which digital tools play an important role in containing mobilization is by discouraging its participation through the enactment of specific laws against freedom of assembly. This strategy could be used during sensitive episodes, such as the COVID 19 emergency, where several countries imposed lockdown restrictions and specifically prohibited mass protests. Bethke and Wolff (2020) suggest that restrictions imposed during the COVID-19 pandemic, which primarily target the freedom of assembly, are less effective in justifying politically motivated restrictions. The resurgence of mass protests in various countries indicates that broad COVID-19-related restrictions may lead to widespread resistance and are challenging to sustain in the long run, which means that the political response to the pandemic is unlikely to result in a direct and lasting closure of civic space.
Derived from the latter, a more specific mechanism employed by governments consists in targeting specific possible protesters through harassment techniques such as exposing their private lives with the aim of discrediting them. On a study on Greta Thunberg that sheds light on how activists could be negatively targeted by digital repression tools, argue that “narratives discredit Thunberg through personal attacks (questioning her mental abilities), her alleged associations (with antifa and Soros), and allegations that she is manufactured or a hoax” (Dave et al., 2020, p. 1).
Finally, another mechanism consists of shutting down the internet in specific locations to prevent communication among people, discouraging “real life” mobilization. By using the Pakistani case study, Wagner (2018) suggests that effectively preventing mobilization is crucial for short-term Internet shutdowns, while negating the presence of “others” is more pertinent for explaining long-term shutdowns.
These three mechanisms result in two significant outcomes that shed light on why manipulation can impede mobilization in the short term. The initial outcome is that manipulation via social media, aimed at restricting access and controlling content, can lead to a reduction in coordination and mobilization as individual expression (one's ability of communicating with other people) is stifled (Earl et al., 2022). For instance, Syria utilized spear-phishing attacks to collect data on dissidents abroad and discredit them, while Jordan engaged in hacking activities targeting non-governmental organization websites and the shutdown of activist Facebook pages, hence affecting possible collective action initiatives (Moss, 2018).
The second outcome involves information channeling, wherein oppressors can shape the overall information landscape. This is achieved by fostering a specific perspective, altering topics, or creating the illusion of widespread support for a specific viewpoint. Information channeling hinders individuals from encountering information related to causes they might otherwise support, as well as activities like protests they would have otherwise participated in Earl et al. (2022). In China, city- and county-level governments primarily implement information channeling, such as flooding online spaces with non-political, highly positive content from local government social media accounts before periods of heightened risk of collective action (Lu and Pan, 2021). In fact, authors such as King et al. (2017) argue that the “Chinese regime's strategy is to avoid arguing with skeptics of the party and the government, with the goal of distracting the public and changing the subject.”
While there is evidence linking manipulation of social media to the containment of mass mobilizations in the short term, a thorough exploration of this relationship in the long run is lacking. The long-term dynamics of this connection could be influenced by several factors.
Firstly, the imposition of restrictions, control, and channeling in the digital space has the potential to give rise to new forms of digital divides (Keremoğlu and Weidmann, 2020). This, in turn, can amplify grievances among the populace, fostering increased motivations for mobilization. It is crucial to recognize that these consequences may unfold over an extended period, shaping the evolving landscape of political dynamics.
Furthermore, the resilience of civil society plays a pivotal role in counteracting manipulated information propagated by the government. The ability of individuals and organizations within civil society to critically assess and challenge such misinformation serves as a check against the sustained impact of manipulation over time (Brookes and Waller, 2023).
Lastly, the effectiveness of digital repression tools in the long run may be limited due to two significant reasons. Firstly, individuals must remain engaged in internet use to receive manipulated messages, which is not guaranteed under all circumstances. Additionally, people may devise strategies to access nonmanipulated information, undermining the efficacy of digital repression tools over time (Roberts, 2018).
As we delve into the complexities of this relationship, it becomes evident that a comprehensive understanding requires consideration of both short-term mechanisms and the enduring dynamics that shape the long-term impact of social media manipulation on mass mobilizations. Consequentially, the following section coordinates them using a dataset drawn from Varieties of Democracy (V-Dem) and employing a panel cointegration methodology. Our hypothesis is that manipulation strategies are less effective in the long run, especially as reality cannot be permanently concealed through manipulation and disinformation due to the abovementioned mechanisms.
Dataset
The empirical analysis is based on data extracted from V-Dem. We use measures of dissemination of false information and mass mobilization:
a. Government Dissemination of False Information Domestic Index (v2smgovdom). This variable responds to the question: How often do the government and its agents use social media to disseminate misleading viewpoints or false information to influence its own population? We refer to this variable as NEWS. This variable takes values on a scale from 0 to 4, where zero is the highest level of manipulation, and four is the lowest level. The data set presents a transformed variable that has a continuous scale (where a lower number indicates higher levels of manipulation) using a measurement model (https://v-dem.net/data/the-v-dem-dataset/). We use this continuous version of the variable in the analysis.
b. Mass Mobilization Index (v2cagenmob). This variable responds to the question: Over the past year, how frequent and large have mass mobilization events been? This variable is continuous and scales from low to high (with the higher bound indicating higher levels of mass mobilization). We will refer to this variable as MOB.
The data correspond to a subset of n = 9 countries followed for T = 21 years from 2000 to 2021. All countries belong to the Latin American region, namely: Mexico, Colombia, Brazil, Bolivia, Honduras, Venezuela, Chile, Ecuador, and Paraguay. The V-Dem database shows data for all Latin American countries, however, not all countries have information for all years. For that reason, we take the sample from all countries with complete data during the studied period to have a panel with no omitted variables.
This sample conforms a panel dataset of dimension 9 × 21 and it is publicly available from the V-Dem website (https://www.v-dem.net/data/the-v-dem-dataset/). Figures 1 and 2 show the evolution of both variables over time.
Econometric methodology
The objective of this study is to investigate possible long run relationships between mass mobilization and government dissemination of false information. To determine such links, we use the well-known panel cointegration methodology and causality analysis for our panel data set.
Panel data methodologies are widely used in empirical research related to economics and political science issues. One of the reasons of such popularity is the small size of samples of interesting databases. For example, V-Dem dataset is one of the main sources of statistical data related to democracy and for some interesting variables (the two of them that we use in the analysis are good examples) we have continuous and complete annual data for only a subset of the complete sample. Because of the latter, time series or cross-sectional techniques are not adequate to study the impact of one variable on the other.
Using a panel data approach brings some important advantages. For example, we can seize the increment in the sample size and consider the heterogeneity of the data, making the cointegration analysis more robust.
Because our objective is to study the long-term dynamics between MOB and NEWS, we include in the panel all Latin American countries that present sufficient data to allow for a panel and cointegration analysis. What are the implications of cointegration relationships in our data? Two variables that are not stable (non-stationary) can potentially share common trends in the long run that are stable (stationary). We establish in this section that it is indeed the case for our panel.
The main feature of the data that can affect the consistency of cointegration and causality analysis is the presence of cross-sectional dependence. In the next section, we perform a test to contrast the null hypothesis of no cross-sectional dependence in the data. Then, we study stationarity of the panel so we can proceed with the cointegration and Granger causality analysis.
Cross-sectional dependence
We begin by examining the existence of cross-sectional dependence in the panel dataset. The presence of cross-sectional dependence (a situation in which time series for different cross-section units are correlated) has pervasive effects on the estimation process using panel data, causing stationarity and cointegration estimation to be inconsistent. Because our panel is relatively small (N < T), we employ the Breusch–Pagan Lagrange Multiplier Test (Breusch and Pagan, 1980) for cross-sectional dependence. This test contrasts the null hypothesis of no cross-sectional dependence using the residuals of the estimation of one time–series regression for each cross-sectional unit. Table 1 shows the results of the test applied to the panel data set. In the second column we show the LM test statistic (a higher value of this statistic indicates higher cross-sectional correlation) and the p-value associated with it.
We can emphatically reject the null hypothesis of no-cross-section dependence for both time series using 10%, 5%, and 1% level of significance. The presence of cross-sectional dependence is because of unobserved factors common to all units that affect them. For example, technological evolution would be a good candidate for a common condition across the sample we are using that could cause this cross-sectional dependence. To deal with cross-sectional dependence, we use only cross dependence robust stationarity and cointegration tests.
Unit root tests
To study cointegration in the panel it is a prerequisite that the variables are not stationary. We require to perform the unit root test to contrast the null hypothesis of the presence of unit roots. Given that our dataset presents cross-section dependence, we need to use robust non-stationarity tests.
We use the panel unit root tests proposed by Breitung (2000), Hadri (2000), Levin et al. (2002), and Im et al. (2003), and the Fisher type test of Choi (2001) to test the non-stationarity of the variables. All tests are performed using the demeaned version of the data to account for cross-sectional dependence. Additionally, we employ Herwartz and Siedenburg (2008), which is robust to cross-sectional dependence, Demetrescu and Hanck (2012), and Herwartz et al. (2017). The results are presented in Tables 2, 3.
The first column presents the 2 variables in levels and their corresponding first and second differences while the other columns present the unit root test statistic and their corresponding p-values. All tests contrast the null hypothesis of non-stationarity except for the Demetrescu and Hanck (DH) test.
The Breitung and the Herwartz and Siedenburg (HS) tests explicitly account for cross-section dependence, while the other tests are performed using demeaned versions of the data as suggested by Pedroni (1999). The tests presented in Table 3 are also robust to heteroskedasticity in the panel.
We can emphatically conclude that both variables are non-stationary, and this result is robust to the presence of cross-section dependence and possible heteroskedasticity in the panel. The fact that the variables have a unit root allows us to explore long term relationships using cointegration analysis. The implication of these results related to the data structure is that the mean and variance of the variables are not constant over time, making us to expect that after an external shock affects the data, it takes a lot of time for the two variables to return to their equilibrium levels.
Cointegration analysis
Because the two variables are non-stationary, we test whether they are cointegrated by performing the cointegration analysis in four tests. The first three, drawn from Kao (1999), Pedroni (1999), and Westerlund (2005), share the common null hypothesis that the two variables are not cointegrated by testing that eit is not stationary. These tests are termed residual-based tests.
In general, we consider the following model:
for i = 1, .N and t = 1, …, T. MOBit and NEWSit are integrated I(1) variables (see Tables 2, 3): that is, the variables have a unit root.
In Equation 1, we include country-specific fixed effects and time trends when possible: ci+δit = zitγi.
Kao (1999) and Pedroni (1999) implement several types of tests (DF t, ADF t and PP t) to determine whether eit is non-stationary. Westerlund (2005) makes use of a variance ratio test for the same null hypothesis and the statistics are computed by testing for a unit root in the predicted residuals using the following model in Equation 2:
Following the regression in Equation 3:
for the ADF t-tests, p is the optimal number of lags selected by an information criterion.
These three tests are performed using the STATA command xtcointtest (StataCorp, 2021). For the latter three tests, we demean the data to account for dependence in the cross-sections and the results are presented in Table 4.
The three tests contrast the null hypothesis of no cointegration. The first column of Table 4 presents the name of the available statistics used for each test while the other columns present the values of those statistics and their corresponding p-values. The Kao (1999) test rejects the null hypothesis of no cointegration in four out of five statistics, the Pedroni (1999) test rejects the null in all cases. Finally, the variance ratio Westerlund (2005) test also rejects the null hypothesis. According to the residual based cointegration tests, we can emphatically reject the null hypothesis of no cointegration and conclude that the variables share common trends in the long run.
To make the analysis robust, we also use the cointegration Westerlund test (Westerlund, 2007) that is a correction-based cointegration test for panel data. It is explicitly robust to cross-sectional dependence and one of its attractive features is that it has good small-sample properties and high power relative to the residual based tests presented in Table 4. The test is based on the following model:
In this model, can be interpreted as the system's equilibrium relationship and measures the speed at which the system return to equilibrium. If αi < 0 there exists error correction and the test concludes that the two variables are cointegrated. We can state the null hypothesis of no cointegration in the panel as H0:αi = 0 ∀i. In this case, there is no error correction and then no cointegration relationships are present. Note that the test statistics assume that αi are equal ∀i, therefore, they contrast the null against the alternative hypothesis that the entire panel is cointegrated. Table 5 presents the results for the two panel test statistics Pτ and Pα which are estimated using the STATA command xtwest (Persyn and Westerlund, 2008) as follows. The first step is to regress ΔMOBit and MOBi, t−1 onto dt, the lags of ΔMOBit and the contemporaneous and lagged values of ΔNEWSi, t. Note that those regressions yield the projection errors and . In a second step, those estimated errors are used to estimate α and its standard error. Then, the two statistics are defined as:
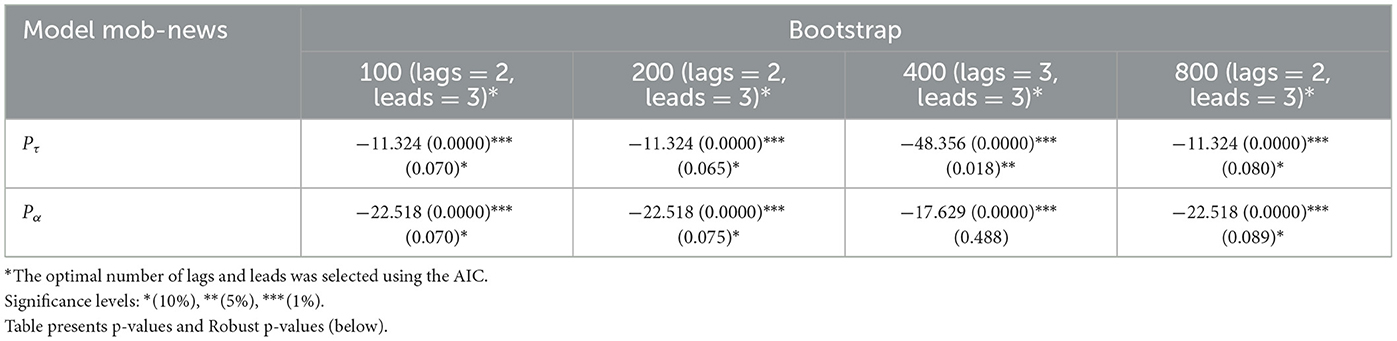
Table 5. Cointegration tests (Westerlund, 2007).
It is assumed that eit in Equation (4) is independent across i. Westerlund (2007) proposed a bootstrap approach to handle the cross-dependence of the panel by obtaining a bootstrap sample of the data to get robust error correction tests and p-values. We consider asymptotic and cross-section dependence robust bootstrap p-values and Table 5 shows the empirical results. First column shows the two test statistics in Equations 5 and 6, and the other four columns the value of the statistics jointly with the non-robust and robust p-values. We can see that, when considering cross-sectional dependence, we can reject the null hypothesis of no cointegration at the 10% level of significance for the entire panel.
In summary, the four cointegration tests used emphatically suggest cointegration in the panel, implying that there exists a long-term relationship between the variables. More precisely, for MOB and NEWS, which are non-stationary variables, there exists a linear combination that is stationary. One of the most important implications of the latter result is the identification of a Granger relationship between the two variables in at least one direction, that is, that we can use one variable to better predict the other. This aspect of the panel is explored in the following section.
Granger causality tests
One of the main implications of the existence of cointegration relationships is the existence of Granger causality link in at least one direction. We study the Granger relationships in our model using Dumitrescu and Hurlin (2012) panel Granger causality test. This is a robust test under cross-sectional dependence and has good properties under small samples, based on the following model:
For i = 1, …, n; t = 1, …, T. DMOBi, t and DNEWSi, t−k are the first-order differenced versions of the original variables, which are stationary. The coefficients in the model are assumed to be heterogenous, that is, they can vary across countries.
The test examines the null hypothesis H0:βi1 = ⋯ = βik = 0, ∀i = 1, …, N, which corresponds to the absence of causality for all individuals in the panel. In this case, the alternative hypothesis is H1:βi1 = ⋯ = βik = 0, ∀i = 1, …, N1 and H1:βi1≠⋯≠βik≠0, ∀i = N1+ 1, …, N.
To compensate for cross-sectional dependence, we use the bootstrap method developed by Dumitrescu and Hurlin (2012) to compute robust p-values. The test runs the individual regressions implied by the specifications (7) and (8) and performs F tests of the K linear hypothesis βi1 = ⋯ = βik = 0 to compute Wald statistics for each individual Wi. Then, the test considers the average Wald statistic The procedure of the test is based on two statistics, and which are defined in Equations 9 and 10, as follows:
We perform this test using the STATA command “xtgcause” (Lopez and Weber, 2017), and the results are presented in Table 6.
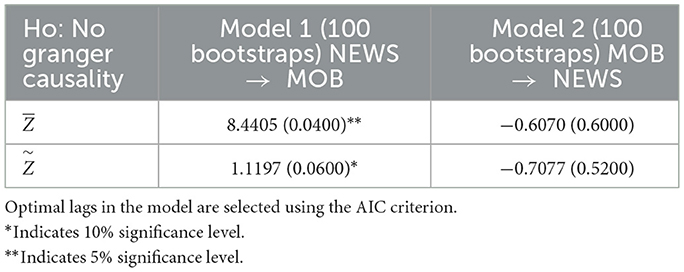
Table 6. Granger causality tests (Dumitrescu and Hurlin, 2012).
The first column of Table 6 presents the two statistics, and Model 1 corresponds to Equation (7) and model 2 corresponds to Equation (8). Both models are estimated using a procedure of 100 bootstraps to apply the resample strategy and the optimal value of K (optimal number of lags) is selected using the AIC criterion. The two last columns present the statistics values and their corresponding p-values. For model 1, we can emphatically reject the null hypothesis of no Granger causality while for model 2, we cannot reject the null hypothesis.
In summary, we cannot reject the null hypothesis that NEWSit causes (in Granger's terms) MOBit. On the other hand, we can clearly reject the null hypothesis that MOBit causes (in Granger's terms) NEWSit. Hence, we find a unidirectional Granger relationship between the variables. That is, MOBit can be better predicted using past values of both MOBit and NEWSit rather than using solely past values of MOBit. A causal inference analysis is necessary to study the mechanisms that might explain this relation, and that opens the venue for future research.
In the following section we estimate the cointegration relationship found. The empirical analysis shows that both variables have a direct (positive) relation in the long run, supporting the hypothesis stated in the study.
Estimation of long run relationships
We estimate the cointegrated panel using three models that allow us to consider potential features of the panel that can affect the estimators. Problems of endogeneity, serial correlation, cross-sectional dependence, and heterogeneity are considered in the following models to make the estimation of the panel robust. We explain each model in more detail in this section and present the estimation results.
Following Kao and Chiang (2000), we estimate the long run relationship of “news” on “mass mobilization” with the widely known Fully Modified Panel Dynamic Least squares (FMOLS) estimator. We estimate the fully modified OLS to the following cointegrated model, specified in Equations 11 and 12, using the correction in Pedroni (2000):
Where uit and εit are stationary. FMOLS methodology can account for heterogeneity across individuals in the panel and potential serial-correlation problems when estimating cointegrated panels. Also, FMOLS is more suitable than OLS because it accounts for endogeneity problems, making the estimators consistent and unbiased (Tugcu, 2018).
This type of estimation allows to extract the long run information while considering heterogeneity in the short run dynamics and in the fixed effects among the members of the panel (Pedroni, 2000). Also, this model has good small sample properties that makes it suitable given the structure of our panel data.
We seize the flexibility of FMOLS estimation to control for another variable in the model by including one additional covariate. We use the civil liberties index (v2x_civlib) from V-Dem that responds to the question: “To what extent is civil liberty respected?”. We refer to this variable as LIB. The two FMOLS models are estimated using the STATA command xtcointreg (Khodzhimatov, 2018).
To overcome the problem of cross-section dependence, a second model is estimated following Pesaran (2006) and considering the pooled common correlated effects (CCE) estimator in the following specification, showed in Equations 13 and 14.
The common factors in the model can be approximated with the averages of the endogenous and exogenous variables, and then the model to be estimated in Equation 15 is the following:
where and are the cross-sectional averages of MOB and NEWS, respectively.
For robustness, allowing for the dynamic behavior of the panel, we consider the following model in Equation 16 (Dynamic CCE), which that allows weak cross-section dependence (Chudik and Pesaran, 2015):
with E(λi) = λ.
The model to be estimated in Equation 17 is the following:
where .
These models are estimated using the STATA command xtdcce2 (Ditzen, 2018), the optimal number of lags pT is determined using the AIC and the results are presented in Table 7.
We find that, for the three models considered, the coefficient is negative and statistically significant, implying that there exists a direct relationship between NEWS and MOB over the long run. That is, an increment (reduction) in the level of government dissemination of false information to manipulate leads to an increment (reduction) in the levels of mass mobilization in the long run. The relation in the long run between the two variables is positive (recall that the variable NEWS, as defined in the dataset, is such that a lower value indicates higher levels of manipulation) and we can see that, even when controlling for the variable LIB, FMOLS estimation is consistent in the sign of the parameter, statistically significant and is closer to CCE regressions estimations.
Table 7 shows the estimated long-run parameters for each model and their corresponding significance statistics (all coefficients are statistically significant at the 5% level).
We found that the effect of NEWS on MOB is positive (direct) in the long run.
Conclusions
This paper investigates the long-term relationship between manipulation of information by the government and mass mobilization. After establishing that the manipulation of information can be used as a tool of preventive digital repression and identifying the lack of academic literature on the matter, the article provides a novel approach to disentangling the relationship between these two variables are related. Studying their relationship in the long run is key, as social media offers an almost imperceptible mechanism of affecting the dynamics of mass mobilization. The latter is crucial for governments, as controlling a mobilization before it emerges is less costly than trying to contain it after it has begun.
Using a dataset containing information on nine Latin American countries from 2000 to 2021 from Varieties of Democracy (2022), which deploys the most recent, transparent, and well-constructed indicators measuring democracy, we employ a novel methodology and find that the variables NEWS and MOB are cointegrated, meaning that a long-term relationship exists between them: they have a common tendency. This relationship is positive, such that when manipulation increases, mass mobilization increases too, implying that manipulation of mass mobilization is only efficacious in the short run, and over the long run, and manipulation is not effective. Using three estimation methods, we find that an increase in NEWS leads to a small but statistically significant increment in MOB.
The cointegration relationship between the variables implies that at least one of them causes, in the Granger sense, the other. In this specific case, we found that, for our data, the Granger relation goes from manipulation through social media to mass mobilization. That is, MOBit can be better predicted using past values of both MOBit and NEWSit rather than using solely past values of MOBit. This opens the venue for a profound causal inference analysis between the variables in future research.
The results that we obtained could be explained by the dynamics of manipulation and mobilization in the short run vs. over the long run. When the government manipulates information to win an election, for example, citizens do not have sufficient time or will to corroborate information and, in those cases, manipulation could have its intended effect. For containing protests, in the short run, the government could employ manipulation through social media to develop a favorable narrative and contain the protesters. However, when citizens realize that their grievances and demands are not being responded to, preventive digital repression tools do not work.
That is, some issues cannot be hidden from the citizenry. This is evident when, in extreme cases, economic hardships affect the population, leading people to take the streets regardless of the government's strategy to hide the situation and redirect the conversation. The spread of protests in Latin America in 2019 and during the COVID crisis exemplifies the latter: although governments sought to discredit protesters to prevent the emergence of generalized social unrest, people mobilized against austerity measures.
Furthermore, using social media for manipulation could backfire, as users could counteract the intent of the government to establish favorable narratives. Fact-checking agencies have proliferated worldwide to combat disinformation spread by the government and other actors as well. Finally, it is important to consider that the effects we found for the long run could be explained by the inherent dynamics of social media: although online use has grown recently, we should recall that offline dynamics matter, in the sense that mobilizing messages do not always transcend certain publics and/or spaces.
This paper opens venues for future research. The effects of government manipulation on mass mobilization can also be investigated through the consideration of other channels, unlike from social media. Finally, the exact mechanisms to explain why social media disinformation is less effective over time is not explored in this study but could reward attention in the future.
Data availability statement
Publicly available datasets were analyzed in this study. This data can be found at: https://v-dem.net/data/the-v-dem-dataset/.
Author contributions
AJ-G: Conceptualization, Formal analysis, Investigation, Methodology, Validation, Writing – original draft, Writing – review & editing. JA-L: Conceptualization, Data curation, Formal analysis, Investigation, Methodology, Software, Validation, Writing – original draft, Writing – review & editing.
Funding
The author(s) declare that no financial support was received for the research, authorship, and/or publication of this article.
Conflict of interest
The authors declare that the research was conducted in the absence of any commercial or financial relationships that could be construed as a potential conflict of interest.
Publisher's note
All claims expressed in this article are solely those of the authors and do not necessarily represent those of their affiliated organizations, or those of the publisher, the editors and the reviewers. Any product that may be evaluated in this article, or claim that may be made by its manufacturer, is not guaranteed or endorsed by the publisher.
References
Abdin, L. (2019). “Bots and fake news: the role of whatsApp in the 2018 Brazilian presidential election,” in Conference Preceedings ISCS. Available online at: https://iscs-conference.com/wp-content/uploads/2019/10/ISCS_2019ConferenceProceedings.pdf
Ackerman, J. M., Cabo de la Vega, A., de Casado, F., Chávez, V. G., Hernández Zubizarreta, J., Luque, A., et al. (2020). La democracia de los trolls y el asalto a la democracia: análisis del proceso electoral en Ecuador y los elementos irradiadores que lo conforman a través de sus redes sociales. Ediciones UTM; Observatorio Euromediterráneo de Democracia y Espacio Público, Universidad Rey Juan Carlos.
Aguilar, A. E. (2018). Twitter herramienta de campaña?: una mirada a la red social el día de las elecciones para diputaciones y concejos municipales 2018 en El Salvador. Real. Reflex. 18, 86–103.
Aravena Lavín, P. (2022). The impact of social networks on the protests in Chile, Ecuador and Colombia during 2019. Asian J. Lat. Am. Stud. 35, 59–82. doi: 10.22945/ajlas.2022.35.2.59
Balod, H. S. S., and Hameleers, M. (2021). Fighting for truth? The role perceptions of Filipino journalists in an era of mis- and disinformation. Journalism 22, 2368–2385. doi: 10.1177/1464884919865109
Bastick, Z. (2021). Would you notice if fake news changed your behavior? An experiment on the unconscious effects of disinformation. Comput. Hum. Behav. 2020:106633. doi: 10.1016/j.chb.2020.106633
Bellinger, P. T., and Arce, M. (2011). Protest and democracy in Latin America's market era. Polit. Res. Q. 64, 688–704. doi: 10.1177/1065912910373557
Bethke, F. S., and Wolff, J. (2020). COVID-19 and shrinking civic spaces: patterns and consequences [COVID-19 und das Schrumpfen zivilgesellschaftlicher Handlungsspielräume: Muster und Konsequenzen]. Z. Friedens Konfliktforschung 9, 363–374. doi: 10.1007/s42597-020-00038-w
Biggs, M. (2018). Size matters: quantifying protest by counting participants. Soc. Methods Res. 47, 351–383. doi: 10.1177/0049124116629166
Bradshaw, S., Bailey, H., and Howard, P. N. (2020). Industrialized Disinformation: 2020 Global Inventory of Organized Social Media Manipulation. Oxford, UK: Programme on Democracy & Technology. Available online at: demtech.oii.ox.ac.uk
Bradshaw, S., and Howard, P. N. (2018). The global organization of social media disinformation campaigns. J. Int. Aff. 71, 23–32. Available online at: https://www.jstor.org/stable/26508115
Brancati, D. (2014). Pocketbook protests: explaining the emergence of pro-democracy protests worldwide. Comp. Polit. Stud. 47, 1503–1530. doi: 10.1177/0010414013512603
Breitung, J. (2000). “The local power of some unit root tests for panel data,” in Advances in Econometrics, Volume 15: Nonstationary Panels, Panel Cointegration, and Dynamic Panels, ed. B. H. Baltagi (Amsterdam: JAI Press), 161–178. doi: 10.1016/S0731-9053(00)15006-6
Breusch, T., and Pagan, A. (1980). The Lagrange multiplier test and its application to model specification in econometrics. Rev. Econ. Stud. 47, 239–254. doi: 10.2307/2297111
Brookes, S., and Waller, L. (2023). Communities of practice in the production and resourcing of fact-checking. Journalism 24, 1938–1958. doi: 10.1177/14648849221078465
Busso, M., and Messina, J. (2020). The Inequality Crisis: Latin America and the Caribbean at the Crossroads. Washington, DC: Inter-American Development Bank. doi: 10.18235/0002629
Carey, S. C. (2006). The dynamic relationship between protest and repression. Polit. Res. Q. 59, 1–11. doi: 10.1177/106591290605900101
Carter, E. B., and Carter, B. L. (2021). Propaganda and protest in autocracies. J. Confl. Resolut. 65, 919–949. doi: 10.1177/0022002720975090
Choi, I. (2001). Unit root tests for panel data. J. Int. Money Finance 20, 249–272. doi: 10.1016/S0261-5606(00)00048-6
Choi, S. Y. (2020). When protests and daily life converge: the spaces and people of Hong Kong's anti-extradition movement. Crit. Anthropol. 40, 277–282. doi: 10.1177/0308275X20908322
Chudik, A., and Pesaran, M. H. (2015). Common correlated effects estimation of heterogeneous dynamic panel data models with weakly exogenous regressors. J. Econom. 188, 393–420. doi: 10.1016/j.jeconom.2015.03.007
CIDH (2020). CIDH Presenta Observaciones de su Visita a Ecuador. Available online at: https://www.oas.org/es/cidh/prensa/comunicados/2020/008.asp (accessed January 5, 2024).
Danneman, N., and Ritter, E. H. (2014). Contagious rebellion and preemptive repression. J. Conflict Resolut. 58, 254–279. Available online at: http://www.jstor.org/stable/24545638
Dave, A., Boardman Ndulue, E., Schwartz-Henderson, L., and Weiner, E. (2020). Targeting Greta Thunberg: A Case Study in Online Mis/Disinformation (No. 11). The German Marshall Fund of the United States.
Davenport, C. (1995). Multi-dimensional threat perception and state repression: an inquiry into why states apply negative sanctions. Am. J. Polit. Sci. 39, 683–713. doi: 10.2307/2111650
Davenport, C., Johnston, H., and Mueller, C. (2005). Repression and Mobilization. Minneapolis, MN: University of Minnesota Press.
Deibert, R., Palfrey, J., Rohozinski, R., and Zittrain, J., (eds.). (2010). Access Controlled: The Shaping of Power, Rights, and Rule in Cyberspace. Cambridge, MA: The MIT Press.
Demetrescu, M., and Hanck, C. (2012). A simple nonstationary-volatility robust panel unit root test. Econ. Lett. 117, 10–13. doi: 10.1016/j.econlet.2012.04.067
DeNardo, J. (1985). Power in Numbers: The Political Strategy of Revolt and Rebellion. Princeton, NJ: Princeton University Press.
Ditzen, J. (2018). Estimating dynamic common-correlated effects in stata. Stata J. 18, 585–617. doi: 10.1177/1536867X1801800306
Dumitrescu, E.-I., and Hurlin, C. (2012). Testing for Granger non-causality in heterogeneous panels. Econ. Model. 29, 1450–1460. doi: 10.1016/j.econmod.2012.02.014
Earl, J., Maher, T., and Pan, J. (2022). The digital repression of social movements, protest, and activism: a synthetic review. Sci. Adv. 8:eabl8198. doi: 10.1126/sciadv.abl8198
Earl, J., Soule, S. A., and McCarthy, J. D. (2003). Protest under fire? Explaining the policing of protest. Am. Soc. Rev. 68, 581–606.
Edgell, A., Grahn, S., Lachapelle, J., Lührmann, A., and Maerz, S. (2020). An Update on Pandemic Backsliding: Democracy Four Months After the Beginning of the COVID-19 Pandemic. V-Dem Institute, 24. Available online at: https://www.v-dem.net/media/publications/v-dem_policybrief-24_update-pandemic-backsliding_200702.pdf (accessed January 5, 2024).
Franklin, J. C. (2009). Contentious challenges and government responses in Latin America. Polit. Res. Q. 62, 700–714. doi: 10.1177/1065912908322405
Galarza Molina, R. (2019). Networked gatekeeping and networked framing on twitter protests in mexico about the ayotzinapa case. Int. Multidiscip. J. Soc. Sci. 8, 235–266. doi: 10.17583/rimcis.2019.4637
Gohdes, A. (2020). Repression technology: internet accessibility and state violence. Am. J. Pol. Sci. 64, 488–503. doi: 10.1111/ajps.12509
Goldstone, J. A., and Tilly, C. (2009). “Threat (and opportunity): Popular action and state response in the dynamics of contentious action,” in Silence and Voice in the Study of Contentious Politics, eds. R. Aminzade et al. (New York, NY: Cambridge University Press), 179–194.
Grigera, J. (2022). Adding insult to injury: the COVID-19 Crisis strikes Latin America. Dev. Change 53, 1335–1361. doi: 10.1111/dech.12740
Gunitsky, S. (2015). Corrupting the cyber-commons: social media as a tool of autocratic stability. Perspect. Polit. 13, 42–54. doi: 10.1017/S1537592714003120
Hadri, K. (2000). Testing for stationarity in heterogeneous panel data. Econom. J. 3, 148–161. doi: 10.1111/1368-423X.00043
Herreros, F., and Criado, H. (2009). Social trust, social capital and perceptions of immigration. Polit. Stud. 57, 337–355. doi: 10.1111/j.1467-9248.2008.00738.x
Herwartz, H., Maxand, S., and Walle, Y. (2017). Heteroskedasticity-Robust Unit Root Testing for Trending Panels. Center for European, Governance and Economic Development Research Discussion Papers 314, University of Goettingen, Department of Economics. Available online at: http://econpapers.repec.org/paper/zbwcegedp/314.htm (accessed January 5, 2024).
Herwartz, H., and Siedenburg, F. (2008). Homogenous panel unit root tests under cross sectional dependence: finite sample modifications and the wild bootstrap. Comp. Stat. Data Anal. 53, 137–150. doi: 10.1016/j.csda.2008.07.008
Im, K. S., Pesaran, M. H., and Shin, Y. (2003). Testing for unit roots in heterogeneous panels. J. Econ. 115, 53–74. doi: 10.1016/s0304-4076(03)00092-7
Jackson, D. (2017). Distinguishing Disinformation from Propaganda, Misinformation, and Fake News: National endowment for democracy. National Endowment for Democracy. Available online at: https://www.ned.org/issue-brief-distinguishing-disinformation-from-propaganda-misinformation-and-fake-news/ (accessed January 5, 2024).
Johnson, J., and Thyne, C. L. (2018). Squeaky wheels and troop loyalty: how domestic protests influence Coups d'état, 1951–2005. J. Confl. Resolut. 62, 597–625. doi: 10.1177/0022002716654742
Justino, P., and Martorano, B. (2016). “Inequality, distributive beliefs and protests: a recent story from Latin America,” in HiCN Working Papers 218, Households in Conflict Network. Available online at: https://ideas.repec.org/p/hic/wpaper/218.html (accessed March 4, 2024).
Kao, C. (1999). Spurious regression and residual-based tests for cointegration in panel data. J. Econom. 90, 1–44. doi: 10.1016/S0304-4076(98)00023-2
Kao, C., and Chiang, M. H. (2000). “On the estimation and inference of a cointegrated regression in panel data,” in Nonstationary Panels, Panel Cointegration, and Dynamic Panels (Advances in Econometrics), ed. B. Baltagi (Amsterdam: JAI Press), 161–178. doi: 10.1016/S0731-9053(00)15007-8
Keremoǧlu, E., Hellmeier, S., and Weidmann, N. (2022). Thin-skinned leaders: regime legitimation, protest issues, and repression in autocracies. Polit. Sci. Res. Methods 10, 136–152. doi: 10.1017/psrm.2021.19
Keremoğlu, E., and Weidmann, N. B. (2020). How dictators control the internet: a review essay. Comp. Polit. Stud. 53, 1690–1703. doi: 10.1177/0010414020912278
Khodzhimatov, R. (2018). XTCOINTREG: Stata Module for Panel Data Generalization of Cointegration Regression Using Fully Modified Ordinary Least Squares, Dynamic Ordinary Least Squares, and Canonical Correlation Regression Methods. Statistical Software Components. Chestnut Hill, MA: Boston College Department of Economics.
King, G., Pan, J., and Roberts, M. (2017). How the Chinese Government fabricates social media posts for strategic distraction, not engaged argument. Am. Polit. Sci. Rev. 111, 484–501. doi: 10.1017/S0003055417000144
Knuutila, A., Neudert, L.-M., and Howard, P. N. (2022). “Who is afraid of fake news? Modeling risk perceptions ofmisinformation in 142 countries,” in Harvard Kennedy School (HKS) Misinformation Review (Cambrigde, MA). doi: 10.37016/mr-2020-97
Levin, A., Lin, C.-F., and Chu, C.-S. J. (2002). Unit root tests in panel data: asymptotic and finite-sample properties. J. Econom. 108, 1–24. doi: 10.1016/S0304-4076(01)00098-7
Lopez, L., and Weber, S. (2017). Testing for granger causality in panel data. Stata J. 17, 972–984. doi: 10.1177/1536867X1801700412
López-Paredes, M., and Carrillo-Andrade, A. (2022). “Legitimation by digital discourses: the case of the indigenous protest in Ecuador, October 2019,” in Cases on Developing Effective Research Plans for Communications and Information Science, eds M. Carrillo-Durán, and M. Pérez Pulido (Hershey, PA: IGI Global), 235–250. doi: 10.4018/978-1-6684-4523-5.ch012
Lu, Y., and Pan, J. (2021). Capturing clicks: how the chinese government uses clickbait to compete for visibility. Political Commun. 38, 23–54. doi: 10.1080/10584609.2020.1765914
Martí i Puig, S., and Serra, M. (2020). Nicaragua: de-democratization and Regime Crisis. Lat. Am. Polit. Soc. 62, 117–136. doi: 10.1017/lap.2019.64
Masías, V., Hecking, T., and Hoppe, U. (2018). Social networking site usage and participation in protest activities in 17 Latin American countries. Telemat. Inform.35, 1809–1831. doi: 10.1016/j.tele.2018.05.010
Matusitz, J., and Simi, D. (2021). U.S. government deception in the Luis Posada scandal: Information manipulation theory. Lat. Am. Policy 1, 57–68. doi: 10.1111/lamp.12210
Moss, D. (2018). The ties that bind: Internet communication technologies, networked authoritarianism, and ‘voice' in the Syrian diaspora. Globalizations 15, 265–282. doi: 10.1080/14747731.2016.1263079
Munck, R. (2020). Social movements in latin america: paradigms, people, and politics. Lat. Am. Perspect. 47, 20–39. doi: 10.1177/0094582X20927007
Munger, K., Bonneau, R., Nagler, J., and Tucker, J. A. (2019). Elites tweet to get feet off the streets: measuring regime social media strategies during protest. Polit. Sci. Res. Methods 7, 815–834. doi: 10.1017/psrm.2018.3
Nam, T. (2007). Rough days in democracies: comparing protests in democracies. Eur. J. Polit. Res. 46, 97–120. doi: 10.1111/j.1475-6765.2006.00645.x
Nordas, R., and Davenport, C. (2013). Fight the Youth: Youth Bulges and State Repression. Am. J. Polit. Sci. 57, 926–940. doi: 10.1111/ajps.12025
O'Brien, K. J., and Deng, Y. (2017). Preventing protest one person at a time: psychological coercion and relational repression in China. China Rev. 17, 179–201.
Pascal Zachary, G. (2020). Digital Manipulation and the Future of Electoral Democracy in the U.S. IEEE Trans. Technol. Soc. 1, 104–112. doi: 10.1109/TTS.2020.2992666
Pedroni, P. (1999). Critical values for cointegration tests in heterogeneous panels with multiple regressors. Oxf. Bull. Econ. Stat. 61, 653–670. doi: 10.1111/1468-0084.61.s1.14
Pedroni, P. (2000). Fully modified OLS for heterogeneous cointegrated panels. Adv. Econome. 15, 93–130. doi: 10.1016/S0731-9053(00)15004-2
Pérez-Liñán, A. (2014). A two-level theory of presidential instability. Latin Am. Polit. Soc. 56, 34–54. doi: 10.1111/j.1548-2456.2014.00220.x
Persyn, D., and Westerlund, J. (2008). Error-correction–based cointegration tests for panel data. Stata J. 8, 232–241. doi: 10.1177/1536867X0800800205
Pesaran, M. H. (2006). Estimation and inference in large heterogeneous panels with a multifactor error structure. Econometrica 74, 967–1012. doi: 10.1111/j.1468-0262.2006.00692.x
Pierskalla, J. H. (2010). Protest, deterrence, and escalation: the strategic calculus of government repression. J. Confl. Resol. 54, 117–145.
Piña-García, C. A., and Espinoza, A. (2022). Coordinated campaigns on Twitter during the coronavirus health crisis in Mexico. Tapuya: Lat. Am. Sci. Technol. Soc. 5:2035935. doi: 10.1080/25729861.2022.2035935
Pion-Berlin, D. (1989). Latin American national security doctrines: hard and softline themes. Armed Forces Soc. 15, 411–429. doi: 10.1177/0095327X8901500305
Porup, J. (2015). How Mexican Twitter Bots Shut Down Dissent. Motherboard Tech by Vice. Retrieved from: https://www.vice.com/en_us/article/z4maww/how-mexican-twitter-bots-shut-down-dissent (accessed January 20, 2024).
Prevost, G., Campos, C. O., and Vanden, H. E. (Eds.). (2012). Social Movements and Leftist Governments in Latin America: Confrontation or Co-Optation? Chicago, IL: University of Chicago Press.
Puyosa, I., and Chaguaceda, A. (2017). Five Political Regimes in Latin America, Internet Freedom and Mechanisms of Control. Retos, n. 14. Quito: Universidad Politécnica Salesiana. Available online at: http://revistas.ups.edu.ec/index.php/retos/article/view/14.2017.01 (accessed January 5, 2024).
Regan, P. M., and Henderson, E. A. (2002). Democracy, threats and political repression in developing countries: are democracies internally less violent? Third World Q. 23, 119–136.
Roberts, M. E. (2018). Censored: Distraction and Diversion inside China's Great Firewall. Princeton, NJ: Princeton University Press. doi: 10.23943/9781400890057
Saldivar, J., Parra, C., Laconich, M., and Cernuzzi, L. (2022). The electoral success of social media losers: a study on the usage and influence of Twitter in times of elections in Paraguay. SN Soc. Sci. 2:98. doi: 10.1007/s43545-022-00392-x
Salzman, R. (2015). Technologies of the body and communication practices in Latin America. J. Lat. Am. Commun. Res. 5, 72–85. Available online at: http://journal.pubalaic.org/index.php/jlacr/issue/view/14
Silva, G. E. (2015). Social movements, protest, and policy. Eur. Rev. Lat. Am. Caribb. Stud. 100, 27–39. doi: 10.18352/erlacs.10122
StataCorp (2021). Stata 17 Longitudinal-Data/Panel-Data Reference Manual. College Station, TX: Stata Press.
Suárez-Serrato, P., Roberts, M. E., Davis, C. A., and Menczer, F. (2016). On the influence of social bots in online protests - preliminary findings of a mexican case study. Soc. Informat.
Sullivan, C. M. (2016). Undermining resistance: mobilization, repression, and the enforcement of political order. J. Confl. Resolut. 60, 1163–1190. doi: 10.1177/0022002714567951
Trejo, G. (2014). The ballot and the street: an electoral theory of social protest in autocracies. Perspect. Politics 12, 332–352. doi: 10.1017/S1537592714000863
Tugcu, C. T. (2018). “Panel data analysis in the energy-growth nexus (EGN),” in The Economics and Econometrics of the Energy-Growth Nexus, ed. A. N. Menegaki (Amsterdam: Elseiver), 255–271. doi: 10.1016/B978-0-12-812746-9.00008-0
Valenzuela, S., Somma, N. M., Scherman, A., and Arriagada, A. (2016). Social media in Latin America: deepening or bridging gaps in protest participation? Online Inf. Rev. 40, 695–711. doi: 10.1108/OIR-11-2015-0347
Van Zomeren, M., and Spears, R. (2009), Metaphors of protest: a classification of motivations for collective action. J. Soc. Issues 65, 661–679. doi: 10.1111/j.1540-4560.2009.01619.x
Wagner, B. (2018). Understanding internet shutdowns: a case study from Pakistan. Int. J. Commun. 12:22. Available online at: https://ijoc.org/index.php/ijoc/article/view/8545/2465
Walgrave, S., Van Laer, J., Verhulst, J., and Wouters, R. (2013). Why People Protest: Comparing Demonstrators' Motivations Across Issues and Nations (Working paper), pp. 1–42. Retrieved from: webh01.ua.ac.be (accessed January 23, 2024).
Wardle, C. (2017). Fake News. It 's Complicated. First Draft. Available online at: https://medium.com/1st-draft/fake-news-its-complicated-d0f773766c79 (accessed January 5, 2024).
Westerlund, J. (2005). New simple tests for panel cointegration. Econom. Rev. 24, 297–316. doi: 10.1080/07474930500243019
Westerlund, J. (2007). Testing for error correction in panel data. Oxf. Bull. Econ. Stat. 69, 709–748. doi: 10.1111/j.1468-0084.2007.00477.x
Wilson, R. (2019). Cambridge Analytica, Facebook, and Influence Operations: A Case Study and Anticipatory Ethical Analysis. Reading: Academic Conferences International Limited.
Zoll, F., Specht, K., Opitz, I., Siebert, R., Piorr, A., Zasada, I., et al. (2018). Individual choice or collective action? Exploring consumer motives for participating in alternative food networks. Int. J. Consum. Stud. 42, 101–110. doi: 10.1111/ijcs.12405
Keywords: disinformation, manipulation, Latin America, mass mobilization, cointegration
Citation: Alcántara-Lizárraga JÁ and Jima-González A (2024) Digital manipulation and mass mobilization over the long run: evidence from Latin America. Front. Polit. Sci. 6:1296004. doi: 10.3389/fpos.2024.1296004
Received: 17 September 2023; Accepted: 19 February 2024;
Published: 12 March 2024.
Edited by:
Sevasti Chatzopoulou, Roskilde University, DenmarkReviewed by:
Francisco Sánchez, University of Salamanca, SpainSebastian Vallejo Vera, Western University, Canada
Copyright © 2024 Alcántara-Lizárraga and Jima-González. This is an open-access article distributed under the terms of the Creative Commons Attribution License (CC BY). The use, distribution or reproduction in other forums is permitted, provided the original author(s) and the copyright owner(s) are credited and that the original publication in this journal is cited, in accordance with accepted academic practice. No use, distribution or reproduction is permitted which does not comply with these terms.
*Correspondence: Alexandra Jima-González, alexandra.jg@tec.mx
†ORCID: José Ángel Alcántara-Lizárraga orcid.org/0000-0003-2785-3233
Alexandra Jima-González orcid.org/0000-0002-9719-3069