- Political Data Science, Technische Universität München, Munich, Germany
The use of AI-generated image captions has been increasing. Scholars of disability studies have long studied accessibility and AI issues concerning technology bias, focusing on image captions and tags. However, less attention has been paid to the individuals and social groups depicted in images and captioned using AI. Further research is needed to understand the underlying representational harms that could affect these social groups. This paper investigates the potential representational harms to social groups depicted in images. There is a high risk of harming certain social groups, either by stereotypical descriptions or erasing their identities from the caption, which could affect the understandings, beliefs, and attitudes that people hold about these specific groups. For the purpose of this article, 1,000 images with human-annotated captions were collected from news agencies “politics” sections. Microsoft's Azure Cloud Services was used to generate AI-generated captions with the December 2021 public version. The pattern observed from the politically salient images gathered and their captions highlight the tendency of the model used to generate more generic descriptions, which may potentially harm misrepresented social groups. Consequently, a balance between those harms needs to be struck, which is intertwined with the trade-off between generating generic vs. specific descriptions. The decision to generate generic descriptions, being extra cautious not to use stereotypes, erases and demeans excluded and already underrepresented social groups, while the decision to generate specific descriptions stereotypes social groups as well as reifies them. The appropriate trade-off is, therefore, crucial, especially when examining politically salient images.
1. Introduction
Vision-to-language technologies have been developed in an effort to improve accessibility for people who are blind or visually impaired (BVI). Examples of such technologies include the automatic alt text, developed on services such as PowerPoint, Outlook, Word, or Facebook to automate image captioning systems. Wu et al. (2017) conclude that automated-image captions assisted people from the BVI community and made them more likely to engage with the content. However, as in many machine learning applications, existing societal pre-judices and human biases can be perpetuated and amplified by these tools (Campolo et al., 2017; Guo and Caliskan, 2021). Less attention has been given to the AI-generated captions of politically salient images. Image captioning technologies combine Natural Language Processing and Computer Vision, and produce a natural description of what the image entails (Luo et al., 2018; Sharma et al., 2018; Barlas et al., 2021). Therefore, these tools also blend the separate biases of each area. Studies of natural language models that investigate religious categories show consistent and strong religious bias, and their analogies enforce stereotypes of different religious groupings, including Atheism, Buddhism, Christianity, Islam, Judaism, and Sikhee (Nadeem et al., 2020; Abid et al., 2021; Guo and Caliskan, 2021; Luccioni and Viviano, 2021). Using state-of-the-art language models like OpenAI's GPT-3 platform and a corresponding programmatic API to automatically complete sentences, Abid et al. (2021) illustrate how the word “Jewish” is analogized to “money” in the test cases, whereas the word “Muslim” is mapped to “terrorist.”
Existing work combines human and machine intelligence in tasks such as image labeling and tagging, with the objective of having “ground truth answers” (Yan et al., 2010; Kamar et al., 2012) and concludes that there is a positive impact when human beings are in the loop as they could assist in overcoming the shortcomings of AI-generated image tags or labels, resulting in an overall user satisfaction. However, while it is possible to have one ground truth when labeling an object in an image, it is often contested on how to decide what is worth describing and how it is being described in an image. This makes the task of generating automated image captions more complex than image tagging.
Moving on to Computer Vision and how it reinforces gender stereotypes, Zhao et al. (2017) showed that images of shopping malls are more likely to caption the presence of women. However, images depicting people in white doctor's/lab coats are most likely to be identified as men (Stangl et al., 2020). The above-mentioned examples are known to merely reflect common stereotypes. Those stereotypes are also evident in controversial public statements made by reporters and politicians, demonstrating the different treatment of social groups seeking asylum. It is pivotal to be aware of how governments give certain social groups preferential treatment, as those distinctions lead to harmful biases that could be adopted by technologies and consequently, amplify existing biases. Recently, the Ukrainian-Russian war reveals how some governments facilitate the entry of Ukrainian refugees and grant them residency, while, in contrast, using drones to identify other “illegal refugees” seeking asylum (Harlan and Zakowiecki, 2022). Therefore, it is important to be aware of those societal biases and develop technologies that are, inclusive of all social groups and, on the other hand, treat different groups equally. Consequently, representational harms caused by technologies should be examined and mitigated.
2. Moving from bias to harms
Research has already discerned that AI is neither impartial nor neutral, emphasizing that “datasets aren't simply raw materials to feed algorithms, but are political interventions” (Crawford and Paglen, 2021). Popular computer vision datasets, such as ImageNet, COCO, and OpenImages underrepresent geographical regions with large populations, particularly in Africa, India, China, and South East Asia (De Vries et al., 2019). Additionally, the classifications are in English, and certain scenes and objects may not be associated with a word in English (De Vries et al., 2019). Wang et al. (2022) highlighted the distinction between datasets used for scientific vs. commercial purposes; those are not the same datasets used to train captioning models.
The technology community and academics have raised concerns about where biases may be present in specific applications. Bias is described in literature as the “reproduction of unjust and harmful social hierarchies” (Crawford and Paglen, 2021), and “disparities in performance” (Friedman and Nissenbaum, 1996) relating to different social groups. There is a common theme within AI to focus on physical appearances when an image depicts women, whereas it focuses on professions when images depict men (Bolukbasi et al., 2016; Zhao et al., 2017; Stangl et al., 2020). Prior research investigates different angles of bias including disability bias (Hutchinson et al., 2020), religion bias (Abid et al., 2021), gender bias (Hendricks et al., 2018; Bhargava and Forsyth, 2019; Tang et al., 2021), racial bias (Zhao et al., 2017), and intersectional bias (Buolamwini and Gebru, 2018; Guo and Caliskan, 2021; Magee et al., 2021). In addition to the complexity of the task, algorithmic bias may exacerbate and duplicate the downstream consequences of real-world racism and sexism and reproduce societal prejudices with respect to an individual's ethnicity, gender, sex, disability, or religion (Buolamwini and Gebru, 2018; O'Neil, 2018; Noble, 2021). It could also under-represent social groups as they are under-sampled or excluded from datasets and consequently over-represent other social groups.
Previous research highlighted that object recognition systems were found to perform relatively poorly on household items, which is more likely to occur in countries with low household incomes. For instance, in the United States, object recognition is around 15–20% more accurate than in Somalia or Burkina Faso. These results are consistent with commercial cloud services for object recognition, provided by Microsoft Azure, Google Cloud Vision, IBM Watson, Amazon Rekognition, Clarifai (De Vries et al., 2019). Moreover, research discovered how computer vision algorithms perform with higher accuracy with lighter skinned groups than darker skinned shades, where the prediction here is almost a random chance (Buolamwini and Gebru, 2018). There is a common theme within AI to focus on physical appearances when an image depicts women, whereas it focuses on professions when images depict men (Bolukbasi et al., 2016; Zhao et al., 2017; Stangl et al., 2020). Several studies discussed how some models predict gender based on the background scene in an image and not based on correct gender evidence, which could lead to “incorrect, and perhaps even offensive predictions.” Hendricks et al. (2018) Consequently, those studies highlight the importance of considering visual evidence of gender and not the context of an image, especially if the background is a “kitchen” or a “snowboard,” avoiding egregious errors (Zhao et al., 2017; Hendricks et al., 2018). Although it is common to measure an algorithm's accuracy in terms of precision, Goodfellow et al. (2016) claim that this doesn't always take into consideration biases present in the training data. Vaswani et al. (2017) emphasize the model's capacity to recognize broad patterns in data, with a preference for low-frequency information over high-frequency information. This is consistent with Gebru et al. (2018)'s concerns, which raised the possibility that the sophistication and specificity of algorithms could not always be the main focus of cutting-edge advancements in AI. For this reason, and in addition to the already-existing studies on bias, research focus is shifting from the notion of bias to more specific and tangible harms, i.e., allocational vs. representational harms (Barocas et al., 2017).
On the one hand, allocational harms focus more on material opportunities or services. For example, these harms involve giving preferential treatment to a specific social group over another or denying certain individuals services because of their race, gender, ethnicity, or religion. By way of illustration, they are predominantly relevant in AI systems, which decide on bank loans, university admissions, employment, or judicial systems.
On the other hand, representational harms “affect the understandings, beliefs, and attitudes that people hold about specific social groups” and thus the standing of those groups within society” (Katzman et al., 2023). Therefore, research on representational harms focuses on whether different social groups are represented equally and whether societies' prejudices are perpetuated and enhanced by technology. For example, object recognition technologies were found to perform worse when asked to recognize household items more likely to be found in countries with low household income (De Vries et al., 2019), which results in unbalanced over-representations of social groups located in high-income countries. Unlike image tagging and object recognition systems, where there is one “correct” answer applied through pre-defined tags to objects at hand, image captioning systems “require a more subjective and contextual choice of choosing what is worth describing” (Wang et al., 2022). Consequently, the exploration of harms is a challenge, as one cannot account for and anticipate all potential harms that could be present in the captions generated. Besides the harm of stereotyping social groups (Zhao et al., 2017; Blodgett et al., 2020; Stangl et al., 2020), Katzman et al. (2023) add the following representational harms:
• Depriving people of the freedom to self-define
• Reifying social groups
• Demeaning social groups
• Erasing social groups
• Alienating Social groups
A balance between those harms needs to be struck, which is intertwined with the trade-off between generating generic vs. specific descriptions. The decision to generate generic descriptions, while being extra cautious not to use stereotypes, erases and demeans excluded and already under-represented social groups, while the decision to generate specific descriptions stereotypes social groups as well as reifies them.
The appropriate trade-off is, therefore, crucial, especially when examining politically salient images. This paper examines AI-generated captions of politically salient images, and argues that generating overly generic descriptions is as harmful as creating overly specific captions. The questions we are attempting to address are: What potential trade-offs should we take into account, and how do we handle all those various biases and harms?
In order to demonstrate the various dimensions and complexity of having those trade-offs, we have chosen exemplary images that serve as examples to illustrate various biases and harms.
3. Methods
This paper does not seek to introduce a technical solution, but investigates the AI-generated image captions of some exemplary politically salient images to understand and evaluate potential representational harms. On that account, the aim is to pave the road for deeper explorations and offer insights on the possible mitigation strategies of captioning political images. One thousand images under the “politics” category and their human-annotated captions were gathered from various news agencies, such as Al Arabiya, Al Jazeera, BBC, CNN, The Guardian, India Today, and the New York Times. In order to generate the AI captions, Microsoft's Cognitive Services was used. The images were uploaded to the Vision Studio with the most recent available version by December 2021. The human annotated captions do not describe the image, but rather its context. Therefore, they are not used as the benchmark of what an image should include. Prior research also states how crowd-sourced “human-centric annotations on people's images contain a wealth of information beyond the image content” (Otterbacher et al., 2019) and introduces “reporting bias,” which is the “discrepancy between what exists and what people mention” (Misra et al., 2015). However, for the purpose of this paper, human-annotated captions serve to demonstrate what a comparatively more detailed caption could include. Recent studies show the tendency to take image labels and tags as a benchmark to measure whether the AI-generated captions were able to identify important objects (Wang et al., 2022; Katzman et al., 2023).
These images weren't picked at random; rather, they have a significant impact on how our study is understood. Rather than being chosen for their simplicity or complexity, they are chosen for their potential to offer significant new insights into larger socio-political challenges. The selected examples in this article do not purport to be representative of all potential cases. Instead, they serve as illustrations of the various forms of harm that may occur. They are meant to illustrate both the possible risks and advantages that automated captioning may present. Therefore, rather than making exhaustive generalizations, our main objective is to use these scenarios to show some potential dangers.
The following section delves deeper to link those harms with politically salient images. Subsequently, we focuses on the harm of erasing social groups or their artifacts and landmarks from captions. Following that is a short discussion about potential trade-offs between the harms and generic vs. specific captions.
4. Results
4.1. Harms of describing politically salient images
Mittelstadt et al. (2016) claim that algorithms pose challenges that cannot always be attributed to clear failures; some effects are questionable and yet appear ethically neutral, as they do not clearly harm anyone. However, this is because AI systems may change how we conceptualize the world and its social and political structures (Floridi, 2014). According to Friedman and Nissenbaum (1996) bias can arise from
1. pre-existing social values,
2. “technical constraints” and,
3. context of use.
When linking those three biases with representational harms, one can argue that a harm such as erasure derive from technological constraints, conscious or unconscious social biases, and the use of technology for tasks it is not designed to support. Therefore, Friedman and Nissenbaum (1996) argue that technical biases stem from either technological constraints, i.e., the description of an image is wrong, or conscious design decisions, “which favor particular groups without an underlying driving value” (Mittelstadt et al., 2016). This paper argues that unconscious design results in the erasure of social groups that are already under-represented in reality, which could amplify existing political oppression and uneven power structures. Excluding social groups, their artifacts, or their landmarks might contribute to their existing marginalization and imply that those social groups have a lower standing compared to other social groups recognized by the model. Therefore, the harm of demeaning social groups or erasing them could be a result of pre-existing social values from which a technology emerges. It is important to state that those harms could stem from unconscious behavior due to the inconclusiveness of a dataset, which leads to the second bias type - technical constraints. Calders and Žliobaitė (2013) present three types of scenarios for why data models could lead to “discriminatory decision procedures.” The first type is concerned with incorrect labels resulting from historical biases. The second type deals with cases when particular data groups are under- or over-represented. The third type focuses on incomplete data due to attributes hidden for reasons of privacy or sensitivity. The three types are predominantly relevant when examining politically salient images.
When focusing on political images and their captions, we highlight the difference between innocuous and obnoxious errors, discussed in the following sections. Lastly, it is vital to delve into the context of using image captions and whether it is appropriate for AI to caption politically salient images (Friedman and Nissenbaum, 1996). To illustrate the trade-off between having generic vs. specific AI-generated captions, we show the attributes that are not mentioned in an image, yet are important for understanding it. Figure 1 depicts the Russian president, Vladimir Putin, sitting at a long table far from the French president, Emmanuel Macron. From a political perspective, the long and giant table can be interpreted to reflect the distanced and problematic diplomatic relations between both countries. The AI-generated captions correctly described the picture. However, certain nuances pivotal to understanding the picture are missing. For example, the table could be more specifically described as giant or long.
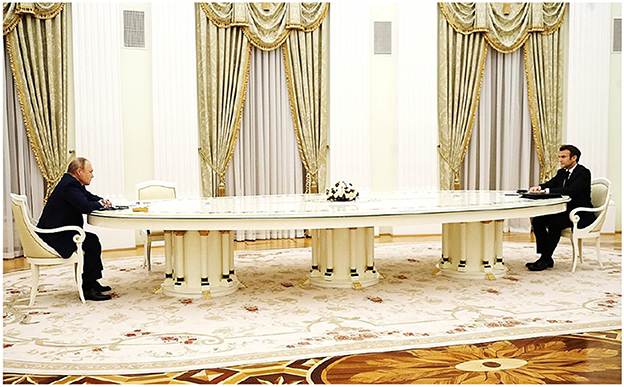
Figure 1. AI caption: men with suits sitting at a table. Human-annotated caption: Russian President Vladimir Putin (L) meets French President Emmanuel Macron (R) on February 07, 2022 in Moscow, Russia. Image taken by Anadolu Agency and can be found under: https://www.businessinsider.com/macron-thinks-the-worst-is-yet-to-come-in-ukraine-after-putin-call-2022-3.
There may be one-off examples for which the model was not able to recognize certain objects. However, this paper provides a socio-technical perspective on potential representational harms that could result from failing to capture certain attributes depicted in politically salient images.
4.1.1. Flatness of the description
Floridi (2014) distinguish between intended and unintended system failures. Dysfunction describes the system's failure to operate as intended, whereas malfunction alludes to unintended harms and consequences. Mittelstadt et al. (2016) add that the mere difference between the system malfunctioning and negative side-effects is that malfunction is avoidable. Hence, image captioning could be designed to generate generic captions while avoiding malfunction and the resulting stereotypes. However, with generic descriptions, especially those of politically salient images, other harms emerge that erase and demean social groups. An example of a generic description is: “The picture includes a man with a face.” This generic caption definitely fails to show that the depicted man is more than just a face. The man's identity is being hidden or erased for the sake of avoiding “malfunction.” Generic descriptions may lead individuals to be described in an inaccurate way, because of how simplified models and classes are used (Barocas, 2014), leading to inconclusive and flat descriptions that can reduce people to just a face.
4.1.2. Right captions, wrong contexts
This section discusses how captioning political images could lead to insensitive captions that erase the lived realities of some social groups. According to Burrell (2016), Machine Learning presents unique challenges, since achieving the intended or “correct” behavior does not always mean the absence of errors or harms. While the AI-generated caption is not mistaken when describing the following picture as people flexing their muscles in Figure 2, the main message of this picture is ignored. In this context and by diminishing the situation to just one man flexing his muscles, the main purpose of this political image is lost. The wounds visible in the picture, which are passed over by the model entirely, erases the struggle and torture these men endured.
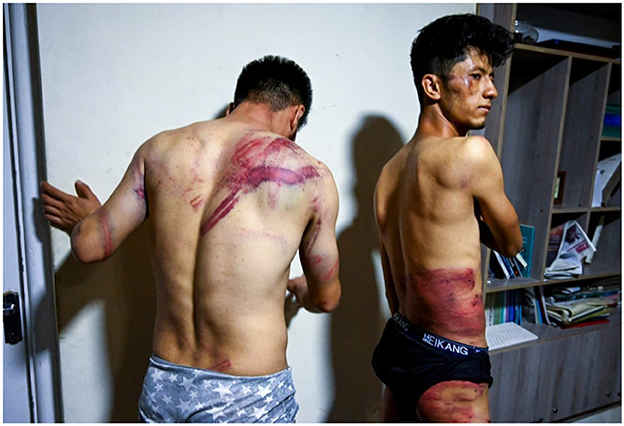
Figure 2. AI caption: a man flexing his muscles. Human-annotated caption: “Journalists Neamat Naqdi and Taqi Daryabi show their wounds in their newspaper office after being beaten and detained for hours by Taliban fighters for covering a protest in Kabul.” Image taken by Wakil Kohsar and can be found under: https://www.theguardian.com/artanddesign/gallery/2021/sep/10/twenty-photographs-of-the-week?CMP=share_btn_tw&page=with:img-16#img-16.
While this harm could arise simply from a technical constraint, wherein the algorithm is simply not trained and designed to recognize wounds, it could also be a symptom of using block-lists to avoid describing cruel scenes. However, Calders and Žliobaitė (2013) emphasize that incompleteness of data as well as “underlying relations between different variables is not sufficient to remove the sensitive attribute,” which results in generic captions that fail to capture the political and social realities of certain social groups. Barlas et al. (2021) investigate the image tagger's fairness criteria by asking participants whether they would prefer human-generated or AI-generated image tags. They conclude that the human and AI-generated tags were deemed to be equally unfair across all images. Political correctness was one of the ten dimensions used in the study to define fairness. Participants favored AI-generated tags in this dimension because they gave more conservative word selections. The question is whether using conservative wording and eliminating objectionable tags from the database is a solution to the underlying harms or merely a workaround (Barlas et al., 2021). Removing offensive tags from the database could create a more appropriate tag and, consequently, avoid a failure to exhibit political correctness. However, such limitations also lead to harms, as in the context of captioning politically salient images. Those limitations could erase and demean social groups and their lived realities. In another illustration, the AI-generated caption does not mention the rubbish bin depicted in the image and describes the following image as a person carrying a bag (Figure 3). To understand the hidden political meaning behind this image, the rubbish bin should be described. By specifying that there is a rubbish bin and that this person has his/her hand inside it, could shed light on the pension reform problems of the global north and how some seniors struggle to maintain a decent living and therefore, have to search for glass or plastic bottles in the bin for their refund.
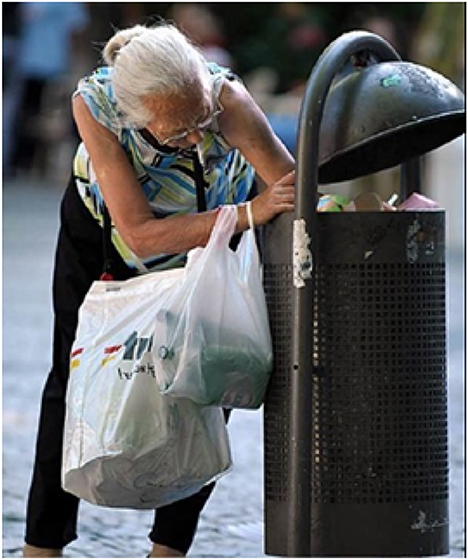
Figure 3. AI-generated caption: a person carrying a bag. Human-annotated caption: “Many pensioners and unemployed people in Berlin are turning to an unusual means of supplementing their meager incomes: collecting discarded deposit bottles. They can return them to stores or supermarkets for a few cents per bottle.” Image taken by Martin Schutt and can be found under: https://www.spiegel.de/international/germany/pensioners-in-berlin-collecting-deposit-bottles-to-supplement\discretionary-income-a-823409.html#fotostrecke-6edec5e1\discretionary-0001\discretionary-0002\discretionary-0000-000000078383.
4.1.3. When the system is wrong
The next example concerns what happens when the caption is simply wrong and therefore malfunctions. “Unethical algorithms can be thought of as malfunctioning software-artifacts that do not operate as intended” (Mittelstadt et al., 2016). We argue that the captions' malfunctioning when describing the realities of children in the global south leads to unethical, harmful algorithms. In the context of describing different social groups, Figure 4 illustrates how a caption can misrepresent the realities of children. It is clear that some attributes, linked to the identities of social groups in underdeveloped countries, are being overlooked and mistaken for objects that are predominantly found in more developed countries (De Vries et al., 2019). Figure 4 shows how the system mistakes the pot for drums and mistakes the actions of those children for playing instead of describing that the children are waiting in line. The AI-generated caption denigrates the lived experiences depicted in this image and hence, fails to acknowledge the injustices those children are facing, such as malnutrition and poverty, leading to a noxious error. While it is important to highlight that the task of image captions is to merely describe what is depicted by an image and not to interpret it, failing to generate a caption that describes those children could result in their erasure and imply that they have a lower standing within society.
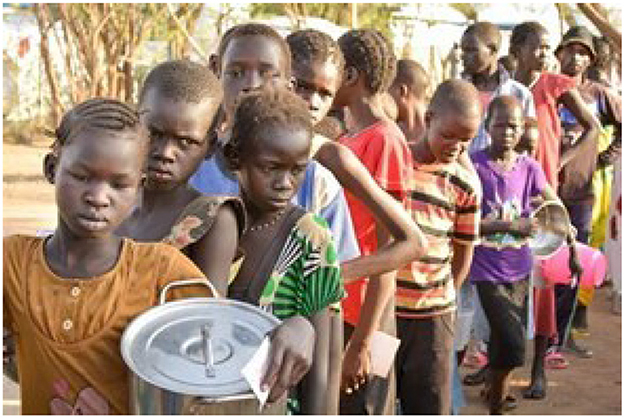
Figure 4. AI-caption: a group of children playing drums. Human-annotated caption: “South Sudanese refugee children line up for breakfast at a reception centre in Kakuma after fleeing fighting in their country. Most arrived desperately hungry.” Image taken by World Vision and can be found under: https://www.huffpost.com/archive/ca/entry/children-humanitarian-crisis_b_10131362.
Another demeaning example of a wrong caption is mistaking a stick for a gun and therefore, hallucinating objects that are not present in the image. Captioning the presence of a gun in a picture containing a possible dark-skinned child is a loaded error which enforces societal prejudices about black people and violence. This hallucination has far-reaching harm and could be tied to the historical institutionalization of discrimination in data that affects popular Western understandings, beliefs, and attitudes about the African community and therefore, has an impact on their status within society. Whilst this hallucination is most likely to be a one-off error, it is harmful because of the pre-existing historical evidence of black people being associated with more violence than white people (Buolamwini and Gebru, 2018; Magee et al., 2021). Therefore, the intersection of being black and the hallucination of a gun leads to a more harmful and complex socio-technical challenge than any picture depicting a white person, when focusing on politically salient images.
4.1.4. When the system is specific
It is worthwhile to state that the AI-generated captions were also able to generate more specific captions. In Figure 5, the AI-generated caption did a better job of describing the image than the human-annotated caption, which focused on the context and the story behind this picture. The caption, mistakenly, describes a hut as the Kasubi Tombs, which is a landmark in Uganda and considered an important spiritual and political place for the Ganda people in Kampala. This proves that AI is able to identify and describe important landmarks located in African countries, and therefore, include sites that are important for the identity of those social groups, as well as recognizing the landmark's outstanding universal value. Nonetheless, huts are an integral part of society and are of high value to the community as a whole. Therefore, it is important to be able to recognize huts and to examine whether this incident was just a one-off error due to technical failure or a sign of systematic error. In the case of a systematic error, this is dangerous as it might lead to erasing an essential part of the African culture and identity, as huts provide shelter and protection for specific communities.
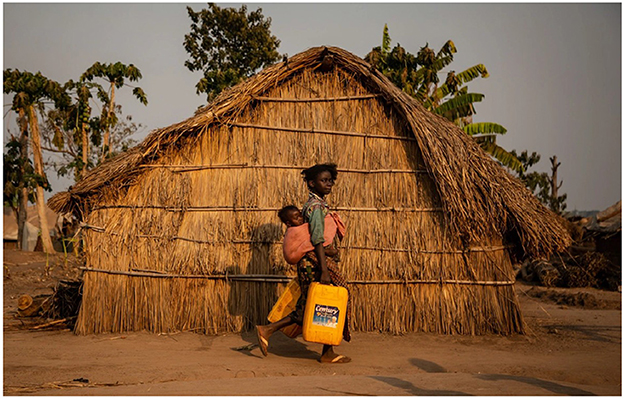
Figure 5. AI-caption: a man carrying a child with Kasubi Tombs in the background. Human-annotated caption: “Even before the most recent displacement crisis, nearly 1.3 million people were displaced in the country or across its borders.” Central Africa Image taken by Tom Peyre-Costa and can be found under: https://www.aljazeera.com/gallery/2021/3/18/families-forced-into-a-deadly-spiral-in-central-african-republic.
4.1.5. When the system is right
The AI-generated captions were also able to describe image just as well as the human-annotated caption. Generally the human-annotated captions relied primarily on background stories from where the picture was taken. In addition to that, the AI-generated caption was able to also generate specific captions that captures the essence of the activity depicted in images. For instance, the AI generated captions were able to describe a man's turban, his white beard, and his background. Given the importance of the turban's meaning for some communities, the caption did a decent job, including it in the description because this headdress is a tradition and sign of nobility in specific social groups. A further example is recognizing headscarves, which is a sign of identity.
Furthermore, the model was also able to describe the activities of the people depicted in the images. The AI-generated caption correctly describes an image as a group of people fetching water (Figure 6). Although the image scenery is predominantly familiar in the global south, the model was able to understand that this act and behavior can be described as “fetching water.” The caption was able to capture the essence of what this group was doing and use the same verb as the human-annotated caption. Moreover, the AI-generated caption described the scene better than the human-annotated caption, where background information was used in the human-annotated caption.
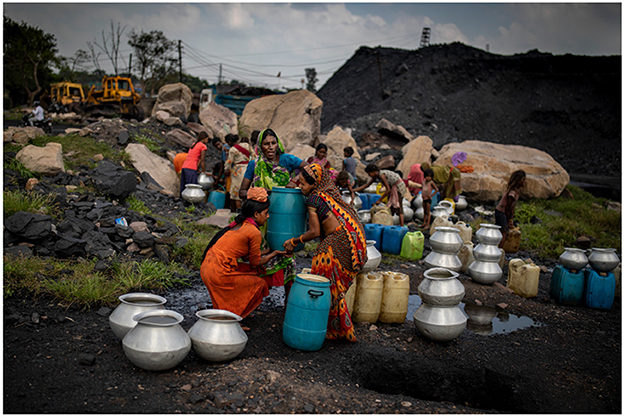
Figure 6. AI-caption: a group of people fetching water. Human-annotated caption: “Members of coal workers' community fetch drinking water from a pipe at a coal depot near an open-cast mine in Dhanbad.” Image taken by Altaf Qadri and can be found under: https://www.aljazeera.com/gallery/2021/11/1/photos-climate-crisis-saved-by-coal-far-from-cop26-another-reality-in-india.
Besides captioning people fetching water, the model was also able to generate a decent caption, given a complex environment. Figure 7 is described by the AI-generated caption as: “A person and a child riding on a vehicle with a child on the back.” This is a reasonable caption and the model did a decent job; however, certain nuances are missing as the human-generated caption is slightly different. The human-generated caption described the vehicle as a cart. Although the words cart and vehicle are synsets, their meaning is slightly different. A vehicle is defined as a piece of “mechanized equipment,” which gives the wrong impression when mistaking a cart for a vehicle. Describing the following means of transportation as a cart leads to a better understanding of the image.
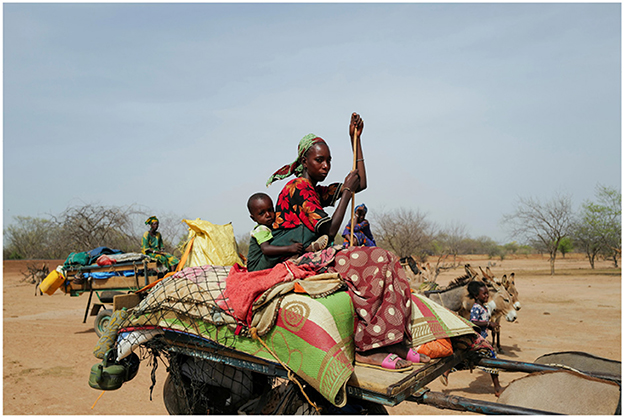
Figure 7. AI caption: a person and a child riding on a vehicle with a child on the back. Human-annotated caption: “A Fulani nomadic tribe member sits on a cart as she travels in the Barkedji-Dodji Forest, an area which is part of the Great Green Wall of the Sahara and the Sahel.” Image taken by Zohra Bensemra and can be found under: https://www.aljazeera.com/gallery/2021/7/29/senegalese-plant-circular-gardens-in-green-wall-defence.
There could be several reasons why the AI-generated caption chose to describe this transportation mode as a vehicle. On the one hand, it could simply be a symptom of a technical constraint, as the cart is obscured in the image. On the other hand, it could also mean that the model is not familiar with carts and therefore, labels it as a vehicle, something which fits better with a predominantly Western life-style.
The next case demonstrates a scenario in which the model makes a predictable mistake; however, it also leads us to question whether this mistake is appropriate or not. The caption confuses burqas with raincoats, which is understandable, but could also potentially be observed as hostile discrimination against people wearing burqas (Figure 8).
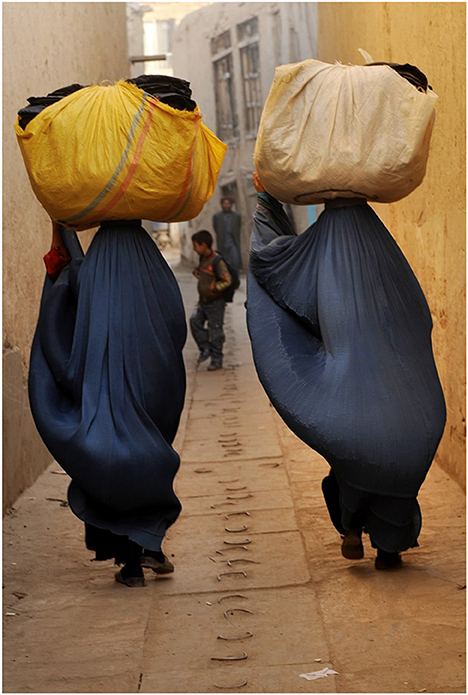
Figure 8. AI-caption: a couple of people wearing blue and yellow raincoats. Human-annotated caption: “Afghan women clad in burqas carry secondhand clothes to wash before trying to sell them.” Image taken by Farzana Wahidy and can be found under: https://www.theguardian.com/culture/gallery/2021/sep/15/photographic-print-sale-to-raise-funds-for-afghans-female-journalists?CMP=share_btn_tw&page=with:img-5#img-5.
Moreover, more transparency is needed to understand on which basis the model chooses to caption guns. There were instances where images contained guns, but the caption mentions the gun only in the picture depicting non-European social groups. When describing and comparing images, the model deemed different aspects as important and decided differently on what is worth mentioning. It is important to investigate whether systematic differences between different social groups and identities occur in these cases of captioning political images.
The next section discusses erasure in terms of the impact of representational harms when captioning politically salient images, highlighting why erasure is harmful and dangerous to under-sampled and under-represented social groups.
4.2. Addressing the trade-offs
When examining political images and their AI-generated captions, the representational harm of erasure is most notable for ignoring or failing to recognize social groups' important attributes, artifacts, or landmarks, which could lead to dehumanization and discrimination. Katzman et al. (2023) identify examples where omitting words from captions could lead to harmful erasure. These are summarized as follows:
• When the caption is too vague
• When the system ignores important aspects of an image
• When the entity & group identity are not named
• When the model consistently misnames objects, artifacts, or landmarks belonging to specific social groups
Those categories aim to “capture instances in which identity is critical to appropriately understanding the context of the image, but the model does not provide it, and in doing so, erases the relevance of that group identity” (Katzman et al., 2023). To compare the above mentioned types of erasure, Katzman et al. (2023) examine the landmarks, attributes, and artifacts of social groups mentioned in the human-annotated captions, but not the AI-generated captions. Another measurement approach to capture erasure is through noting the presence or absence of important attributes. For example, when a machine learning model is able to identify a Bible but fails to recognize a Quran or a Torah, it shows that the training data was biased .
The following sections link the above-mentioned types of erasure with their potential consequences for social groups.
4.2.1. Too vague
Captions can become vague when the human-annotated caption identifies people or named entities which are not mentioned by the AI-generated caption, or when the description is more general and vague than needed. As an example of vague captions, Katzman et al. (2023) mention women suffragists described as walking instead of marching, which undermines the reality of those women. When examining political images and the potential harm of erasure, it is crucial to consider the trade-off between generating generic vs. specific descriptions.
The pattern observed from the collected images and their captions is that the model tends to generate generic captions and describe all social groups as one homogeneous entity. By way of illustration, people demonstrating and holding signs, regardless of the social group and their setting, were predominantly captioned as: “A group of people holding signs,” “A group of people in clothing,” “A large crowd of people,” and “A group of people holding flags.”
Demonstration images are captioned vaguely, even when they take place in different countries and for different causes. The social groups depicted are located in Brazil, Costa Rica, Ethiopia, Scotland, Sudan, Tunisia, the United States, and the West Bank. As seen from signs visible in the crowds, the events vary from demonstrations about abortion laws, climate change, and oppressive regimes to protests about COVID-19 regulations. However, all those protesters and their objectives are captioned simply as a group of people holding signs or flags.
Although there are existing technologies capable of reading the signs as well as identifying flags of countries, it is clear that this is not a technological constraint but merely a choice to adhere to generic descriptions in this context. The choice of description level, such as captioning sign content and identifying flags, has two different sides. On the one hand, the model is neutral and objective, as it does not identify any content for any social group, no matter their differences, representing all social groups equally. Having neutral and vague captions could be beneficial as it could be risky if the model interfered with the content and identified the main reasons that protesters are on the streets. Political images are very sensitive and controversial in nature. If protesters are depicted in one image, the caption might be biased toward/against the demonstrations and therefore, risk showing semantic preference for one social group over the other, thus promoting opinion. Consequently, the threat of treating one opinion as having lower social standing could perpetuate the belief that one ideology is superior to the other.
On the other hand, generic description erases the demonstrators' identity and fails to acknowledge their causes. In some of those pictures, individuals are in hostile situations where they are willing to risk their lives for what they believe in. By treating all social groups as one homogeneous entity, the captions fail to recognize human differences and therefore, fails to recognize the right to protest and freedom of expression.
4.2.2. Ignoring order
Moving on to the recognition of political figures, the general observation was that the model was able to recognize prominent politicians. In addition to recognizing them, the model was able to accurately give a detailed description of what the image depicts. The model was able to mention the names of prominent political figures from the global South and North equally. For example, recognizing Samia Sululu, the president of Tanzania, and captioning that she is wearing a red scarf. However, the interesting pattern to observe, and to further examine, is how politicians are ordered in a given caption. When several politicians from different nations are depicted in one image, the order of politicians does not align with the order mentioned in the human-annotated captions. The norm with human-annotated captions is to name politicians standing from left to right, which has been adopted by several news agencies. However, the model randomly orders the names of politicians in a caption. It is important to examine whether the order of politicians visible in an image is necessary to understand the political context. For instance the AI-generated caption mentions politicians standing in the second row and leaves out prominent politicians standing in the first row from the caption. Giving the name of a politician standing at the back of the picture as the first name gives viewers the impression that this person is the center of attention and therefore, might deceive users, leading them to understand, and interpret political relationships differently. An essential design decision to examine is whether the order of politicians displayed in photos is important for people using image captions and their understanding of the image. People who are blind or visually impaired, as well as other users, must be involved in these inquiries.
4.2.3. Consistent misnaming
The next erasure topic is the consistent mis-naming of attributes or artifacts belonging to a specific social group. It is concerned with identifying systematic errors and not just one-time-off incidents. Systematic errors can be measured by gathering a great deal of data to be able to conclude an in-depth analysis and examination to identify which social groups are being systematically harmed by the consistent misapplication of their belongings. In addition to that, it is also important to survey those social groups and understand the impact of this systematic error on their political and social standing within society.
A possible systematic error requiring further investigation is that of religious clothing which has been misnamed as costumes or dresses (Figure 9), which could be degrading and demeaning for those affected. In another observation (Figure 10), neither the human-annotated caption nor the AI-generated caption mention that this man is wearing a traditional headpiece, rather calling it a hat. Whereas this caption did a decent job describing the man, there is a trade-off when choosing to generate a generic description. Potential harms need to be explored when representing indigenous groups; perhaps those groups depicted in images would find it offensive and demeaning to describe their traditional headgear as just a hat.
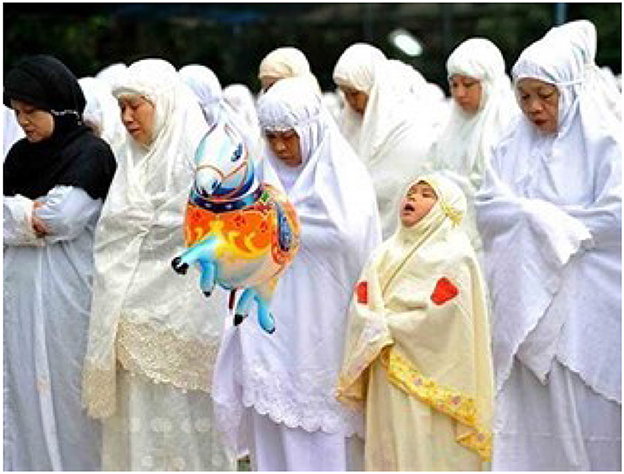
Figure 9. AI-caption: a group of women in white dresses. Human-annotated caption: “Indonesian Muslim women pray in Jakarta.” Image taken by Adek Berry and can be found under: https://www.theguardian.com/world/gallery/2009/nov/27/islam-religion.
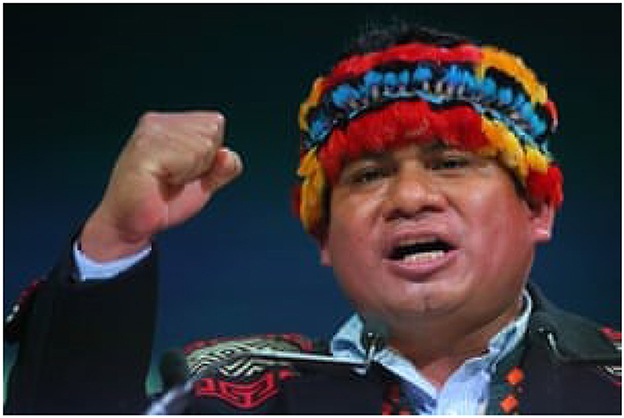
Figure 10. AI-caption: a man wearing a hat. Human-annotated caption: “Tuntiak Katan, vice-coordinator of the Indigenous Organizations of the Amazon Basin.” Image taken by Robert Perry and can be found under: https://www.theguardian.com/environment/gallery/2021/nov/02/cop26-global-leaders-begin-talks-in-pictures.
4.2.4. Group identity not used
Another theme of erasure is when a group's identity is mentioned in the human-annotated caption but not in the AI-generated one. Here the focus is on traditional clothing. Specifically, the model should recognize that the clothing depicted is special and, therefore, the caption provides a more specific description that is important for social groups' identities as well as the general understanding of the image. It is important that the exemplary model (Figure 11) was able to recognize traditional clothing, in which the AI-generated caption correctly described certain images as a group of people in traditional clothing.
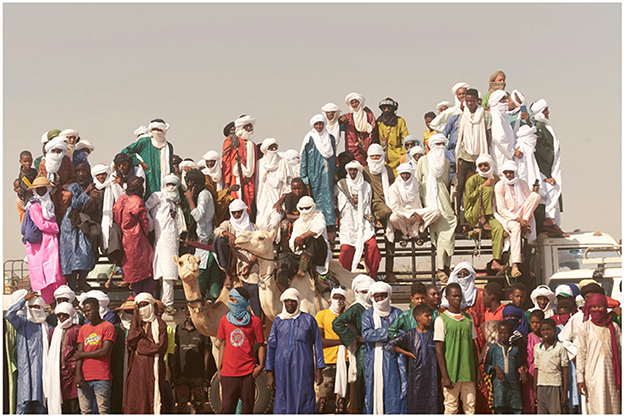
Figure 11. AI-caption: a group of people in traditional dress. Human-annotated caption: “Spectators watch the camel race.” Image taken by Michele Cattani and can be found under: https://www.aljazeera.com/gallery/2021/9/21/ingall-niger-hosts-camel-race-sahara-desert.
However, other captions illustrate different scenarios, in which human-annotated captions mention “traditional” clothing, whereas the AI-generated caption does not mention the word traditional. It is important to highlight that in some cases, when group identity is not relevant, this may be innocuous. The model successfully describe traditional clothing, in some instances, by recognizing garments.
4.2.5. Named entity not named
The next erasure incident occurs when a named entity is mentioned in the human-annotated caption, but not the AI-generated caption. It is important to emphasize that, “often, Machine Learning researchers omit identity data in an attempt to remain universally objective or to avoid bias” (Abid et al., 2021; Bennett et al., 2021). However, remaining universally objective could also lead to harmful consequences, thus worsening inequities for already marginalized people. The next observation links several erasure incidents and highlights how ignoring social groups and consistently misnaming their belongings, as well as the conscious or unconscious decision not to name an entity, are highly intertwined.
An observation worth noting and investigating is the mis-naming of Yazidi temples as caves as well as the failure to recognize the Lalish Temple (Figure 12). Describing Yazidi temples as caves is not just demeaning but also enforces and amplifies existing discrimination toward this social group. Whereas not recognizing the landmarks or temples of other social groups might be innocuous, erasing this particular temple is harmful due to the underlying political motivations of certain countries, which attempt to eradicate the Yazidi. Therefore, not identifying their temples and especially the Lalish temple, which according to the UNESCO all worshippers from around the world visit, puts Yazidi identity in danger.
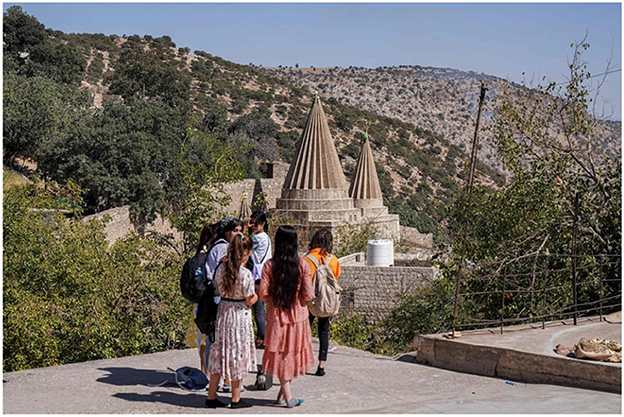
Figure 12. AI-caption: a group of people standing on a stone path with a building on the side. Human-annotated caption: “People walk toward the Lalish temple.” Image taken by Ismael Adnan and can be found under: https://www.theguardian.com/world/gallery/2021/oct/11/yazidis-visit-holiest-temple-during-autumn-assembly-in-pictures.
Figure 13 divulges the sensitivity needed to describe images of people who are persecuted and oppressed. From a mere technical perspective, one has to state that the model did a decent job describing the setting of the images and captioning the temple as a cave. From a socio-technical perspective, however, there are underlying harms in failing to recognize such a landmark, which amplifies injustices these social groups face.
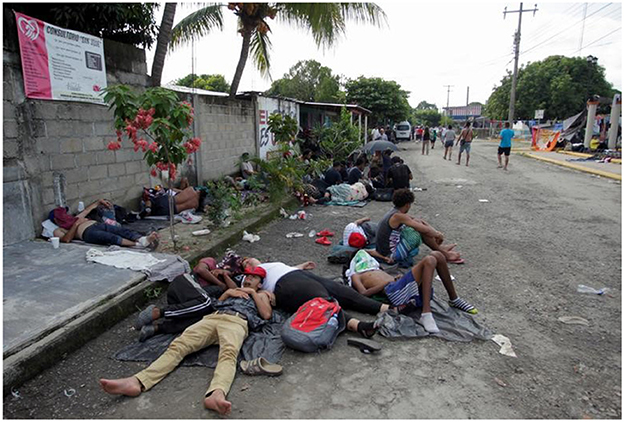
Figure 13. AI caption: a group of people lying on the beach. Human-annotated caption: “Migrants rest as they take part in a caravan heading to Mexico City, in Nuevo Milenio Valdivia, Mexico.” Image taken by Daniel Becerril and can be found under: https://www.reuters.com/news/picture/migrant-caravan-limps-north-through-mexi-idUSRTXJK8A19.
Therefore, the attempt to remain universally objective in order to avoid bias can be harmful to politically persecuted/marginalized social groups, and this neutrality, in return, amplifies political injustices. Training AI to be universally objective may generate relatively “correct” but generic descriptions, which could impose systematic erasure and unconsciously participate in further endangering, for example, Yazidis. In this case, image captions should be used to safeguard artifacts and landmarks that are important for specific groups. Therefore, those who train AI should strive to equally represent various social groups and their belongings.
5. Discussion
The trade-offs between the harms and the trade-off between the description level (generic vs. specific captions) are closely tied to the application purposes of the system (Barocas et al., 2021). The context of describing political images differs significantly from captioning images depicting nature or landmarks, as there is a higher risk of harming social groups, either by employing stereotypical descriptions or erasing their identities from the caption.
Several patterns were observed after looking at the gathered images and their AI-generated captions. There is an overall tendency toward generating more generic descriptions and, therefore, choosing not to stereotype social groups and not deny them the opportunity to self-define. It should be noted that the effort to generate equal captions for all different social groups is evident. However, preferring overly generic description creates different harm levels for different groups. The motivation to treat all social groups in a neutral and equal way might be in return dehumanizing and exclusionary, as the context and the living situations of social groups in the global south and the global north differ significantly.
Generic captions, therefore, more accurately describe the living conditions of those in the global north. This is caused by very particular contextual and historical factors that are difficult for the model to caption. Nevertheless, generic description amplifies the harm of erasure beyond what the computer vision model exhibits, as the political and social realities of specific social groups are misrepresented, particularly if they are from the global south.
The following examples illustrate the sensitivity of captioning politically salient images and highlight the differences between innocuous and obnoxious AI hallucinations of objects that are not depicted in an image.
Figure 13 shows people lying on the street. The AI captions it as: “A group of people lying on the beach.” This hallucination of a beach is harmful, as it erases the sufferings of migrants who are resting on the streets and therefore, is insensitive. Figure 14 shows a person standing in rubbish with storks in the background. The AI captions this as follows: “A person standing on a rocky beach with many birds.” Although the caption included birds, it describes an overly relaxed and luxurious lifestyle, thus losing authentic contact with reality.
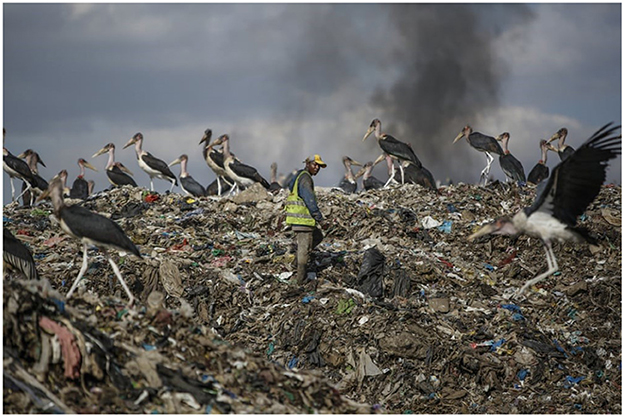
Figure 14. AI caption: a person standing on a rocky beach with many birds. Human-annotated caption: “A man who scavenges recyclable materials for a living walks past Marabou storks feeding on a mountain of rubbish amid smoke from burning trash at Dandora, the largest dump in the capital, Nairobi.” Image taken by Brian Inganga and can be found under: https://www.theguardian.com/artanddesign/gallery/2021/sep/10/twenty-photographs-of-the-week?CMP=share_btn_tw&page=with:img-18#img-18.
There are also cases where the model does not hallucinate but generates overly generic descriptions. It is important to note that not all generic captions are harmful. However, in the case of politically salient images, it is essential to examine unnecessary vagueness and focus on the different harm levels affecting different social groups. More detailed captions are able to identify surfers and beaches whereas, more details are needed to capture aspects that extinguish image contexts. Describing images that depict beaches and people with a generic caption such as “A group of people on a beach” (Figure 15) is not in itself wrong. However, the fact that the model chooses to unite all social groups with the same or similar captions promotes the erasure of social realities by ignoring important objects in an image that could specify the situation and are important to viewers' understanding. By way of illustration, the model could be trained to identify life-jackets or life-boats (Figure 16), which will indicate a significant change in the description and thus relate more to the realities of certain groups.
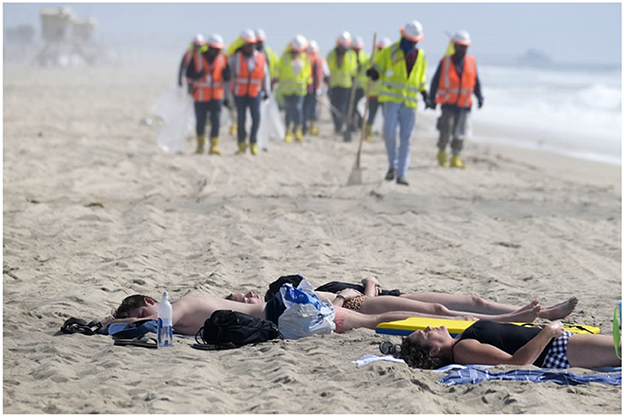
Figure 15. AI caption: a group of people lying on the beach. Human-annotated caption: “People sunbathe as workers clean the contaminated beach.” Image taken by Ringo HW Chiu and can be found under: https://www.theguardian.com/news/gallery/2021/oct/12/columbus-defaced-turkish-mosaics-guard-tuesday-best-photos.
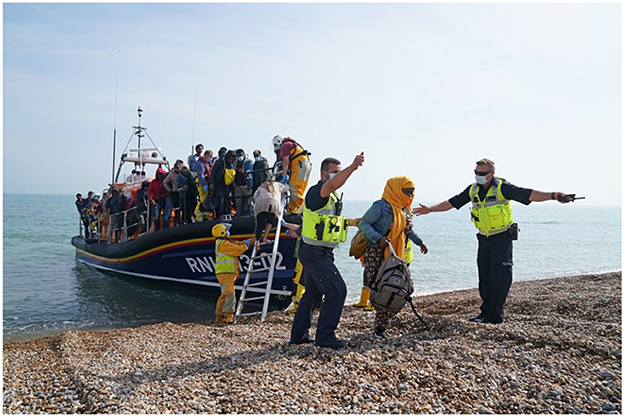
Figure 16. AI caption: a group of people on a beach. Human-annotated caption: “People are brought ashore from a lifeboat at Dungeness in Kent. Hundreds of refugees and migrants crossed the Channel this week after the weather improved.” Image taken by Gareth Fuller and can be found under: https://www.theguardian.com/artanddesign/gallery/2021/sep/10/twenty-photographs-of-the-week?CMP=share_btn_tw&page=with:img-18#img-18.
Consequently, it is inappropriate to describe different lifestyles equally and without the necessary specificity, as one cannot equate surfing or sunbathing with refuge and, therefore, the following captions might be misleading. Figures 15, 16 show how the same caption or similar descriptions affect social groups unevenly. The generic description trivializes misrepresents the cruel lived experiences of refugees. Suffice to say, generic descriptions of images in the context of politically salient images are as harmful as having captions that are too specific and stereotypical. Deciding on an appropriate trade-off between those harms is a complex task, which requires a socio-technical approach going forward. This plays a pivotal role when examining political scenes and the role of technology within those spaces.
According to Friedman and Nissenbaum (1996), AI developers are responsible for creating appropriate solutions for diverse contexts governed by the different ethical codes of diverse groups. There is a growing tendency in literature that supports the development of collaborative ethical frameworks for AI systems (Mittelstadt et al., 2016). This growing tendency is also found in non-profit organizations such as Algorithmic Justice League, whose mission is to lead a “cultural movement toward equitable and accountable AI.”
However, those movements and NGOs fall short when it comes to dealing with powerful countries, as mentioned by the AI Now report, published in 2019 (Campolo et al., 2017). The report covers the incident that occurred when Microsoft funded an Israeli company, AnyVision, a surveillance company that uses facial recognition technology to track the movements of Palestinians. After public upheaval, Microsoft then opened an investigation to assess the company's compliance with its own Responsible AI Standard. This shows that we must not just focus on the technology but the broader political circumstances within which AI is developed.
Efforts to regulate and standardize AI ethics, such as the EU AI ethics regulations which emphasize the importance of human-in the loop guidelines, fall short as they are not always feasible and suitable to all applications. Furthermore, those ethical standards and regulations are predominantly developed by corporations, organizations, and countries of the global north (Greene et al., 2019; Krupiy, 2020; Schiff et al., 2020). Crawford and Paglen (2021) present evidence on the politics behind training sets and their potential harms to representation and self-identification. For example, ImageNet is a widely used and “critical asset for computer vision research,” allowing online labor platforms such as Amazon Mechanical Turk to label huge quantities of images and therefore, enforce their own views on how images should be labeled and viewed. Further illustrations show how, for research purposes, race and gender labels are being crowd-sourced using Amazon's Mechanical Turk (Zhao et al., 2021). Research has also covered how image description datasets such as Flickr30K and MS COCO “show a high degree of variation in the ways that crowd-workers talk about the world,” making various inferences about people depicted in images, especially their ethnicity and demographic related attributes (Misra et al., 2015). Several taxonomies have been introduced to serve as a reference point for how people should be described (Otterbacher et al., 2019). Scholars have suggested adopting group fairness approaches, addressing questions such as “what's worth reporting” in an image, in terms of sensitive attributes, and “is group fairness respected?” (Van Miltenburg et al., 2018).
Creating image caption technologies that are universal and can serve people worldwide is a challenge. While such technologies have increased accessibility for e.g., the BVI community, developers still bear great responsibility, particularly in ensuring that caption systems work fairly everywhere—regardless of the socioeconomic status or cultural background of the people depicted. Therefore, there has to be a common understanding that non-inclusive algorithms can have severe consequences for individuals, social groups, and even whole societies. The choices designers make must be transparent, fair, and inclusive.
Existing social hierarchies that undermine the equal representation of disadvantaged social groups must be dismantled by improving transparency criteria for image caption development. Beyond the influences of human subjectivity, the controversial nature of political images increases captioning complexity due to the multiplicity of interpretations. Computer Vision systems often have biases and limits to their ability to represent the world's complexity as well as the intersectionality of people's lives. As a result, representational harms amplify injustices faced by marginalized social groups (Keyes, 2018; Bennett and Keyes, 2020). Crawford et al. emphasize that object recognition algorithms are mostly designed and developed in a white, western and middle class context (Crawford and Paglen, 2021), failing to recognize common household objects that are more often found in “non-Western countries or in low-income communities” (De Vries et al., 2019). We argue that, in addition to object recognition algorithms, image caption models also reflect the prevalence of western dynamics and realities. The captions of politically salient images, because they are generic, fail to describe the political realities of social groups in under-developed countries. These captions may be misleading, deceiving, or inefficacious.
Hence, representational harms must be examined further to assess their impact on two exemplary groups. The first consists of people within the BVI community living in the global south, as academic research on this topic mostly includes BVI people in high-income countries (Salisbury et al., 2017; Bennett et al., 2021; Stangl et al., 2021). Therefore, it is important to include the political needs and wants of the BVI community in different global regions (Mozur, 2019), and to start a debate on the desired caption cultures. The second social group who are affected by captioning systems are those depicted in images. These various social groups can be affected by representational harms, as they might be under-sampled in datasets, misrepresented, or excluded from captioning systems. Little to no research has engaged with the harms caused by captions to the people in the images themselves.
5.1. Future challenges
Datasets should be continuously updated to capture the fast changing pace of the real world. Climate change also has unprecedented consequences in the domain of computer vision. Figure 17 illustrates how the AI-generated caption understandably mistakes foam for snow. The chemical foam is caused by industrial and domestic pollution in India. Whether the AI distinction between foam and snow is currently technically feasible or not is not the main point. The aim is to shed light on the need to keep an eye on our rapidly-changing earth as a result of human activity. The realities of various social groups are subject to change, which emphasizes the fact that datasets need to capture this dynamic nature as well.
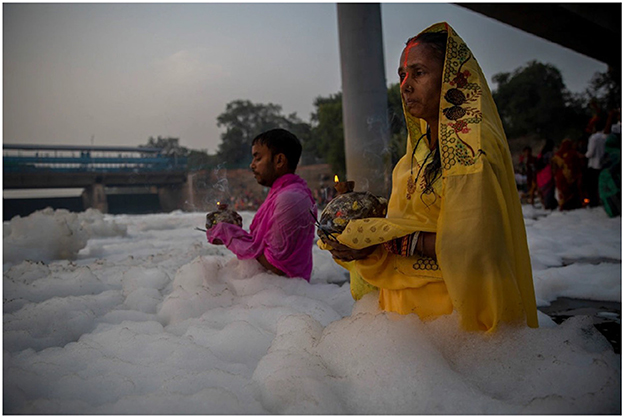
Figure 17. AI-generated caption: a person and a child in the snow. Human-annotated caption: “Hindu devotees perform rituals in Yamuna river, covered by chemical foam caused by industrial and domestic pollution, during Chhath Puja festival.” Image taken by Altaf Qadri and can be found under: https://www.aljazeera.com/gallery/2021/11/11/india-hindus-yamuna-river-pollution-chhath-puja.
6. Conclusion
Current automated image captioning solutions are still not robust enough to be used to describe critical and grievous political images, as those captions could be very insensitive and harmful to under-represented social groups. Further investigations need to explore the limits of image captions and their accompanying representational harms to different social groups. Therefore, we must emphasize that the claim that image captions have reached human parity falls short when describing politically salient images, and makes it seem like image captioning is a solved problem. When corporations define their tasks and train models on non-inclusive data, the technology fails to include under-sampled and underrepresented social groups. In order to really achieve human parity when captioning politically salient images, one has to think of the task as a strong AI problem, perhaps requiring Artificial General Intelligence to be able to make the expected moral decisions. Unfortunately, this is currently unattainable. Scholars are introducing theories such as userism (Hegelich, 2022) to emphasize that mere technological solutions fall short when dealing with socio-technical problems. The expectation that AI will capture historical content to do justice to all social groups is unrealistic. Because of the multifaceted nature of politically salient pictures, overgeneralized demands will be impossible to meet from a political standpoint. It may suffice for a model to understand that sensitive content, such as a wound, is evident, to recognize where things could go wrong, and to warn users that this image requires human review. AI Technology is still developing and this maturity level adds additional complexity. However, when examining politically salient images, the representational harms outweigh the benefits of simple and inexpensive AI programs. Consequently, one has to question whether the current state of AI could, and if so, should, even solve this problem.
Data availability statement
The original contributions presented in the study are included in the article/Supplementary material, further inquiries can be directed to the corresponding author.
Ethics statement
Written informed consent was not obtained from the individual(s) for the publication of any potentially identifiable images or data included in this article because images are publicly available on newspaper websites and images are cited in the article accordingly.
Author contributions
All authors listed have made a substantial, direct, and intellectual contribution to the work and approved it for publication.
Conflict of interest
The authors declare that the research was conducted in the absence of any commercial or financial relationships that could be construed as a potential conflict of interest.
Publisher's note
All claims expressed in this article are solely those of the authors and do not necessarily represent those of their affiliated organizations, or those of the publisher, the editors and the reviewers. Any product that may be evaluated in this article, or claim that may be made by its manufacturer, is not guaranteed or endorsed by the publisher.
Supplementary material
The Supplementary Material for this article can be found online at: https://www.frontiersin.org/articles/10.3389/fpos.2023.1245684/full#supplementary-material
References
Abid, A., Farooqi, M., and Zou, J. (2021). “Persistent anti-muslim bias in large language models,” in Proceedings of the 2021 AAAI/ACM Conference on AI, Ethics, and Society (New York, NY), 298–306. doi: 10.1145/3461702.3462624
Barlas, P., Kyriakou, K., Guest, O., Kleanthous, S., and Otterbacher, J. (2021). To “see” is to stereotype: Image tagging algorithms, gender recognition, and the accuracy-fairness trade-off. Proc. ACM Hum. Comput. Inter. 4, 1–31. doi: 10.1145/3432931
Barocas, S. (2014). “Data mining and the discourse on discrimination,” in Data Ethics Workshop, Conference on Knowledge Discovery and Data Mining (New York, NY), 1–4.
Barocas, S., Guo, A., Kamar, E., Krones, J., Morris, M. R., Vaughan, J. W., et al. (2021). “Designing disaggregated evaluations of ai systems: Choices, considerations, and tradeoffs,” in Proceedings of the 2021 AAAI/ACM Conference on AI, Ethics, and Society (New York, NY), 368–378. doi: 10.1145/3461702.3462610
Barocas, S., Crawford, K., Shapiro, A., and Wallach, H. (2017). “The problem with bias: Allocative versus representational harms in machine learning,” in 9th Annual Conference of the Special Interest Group for Computing, Information and Society (New York, NY).
Bennett, C. L., Gleason, C., Scheuerman, M. K., Bigham, J. P., Guo, A., and To, A. (2021). “‘it's complicated: Negotiating accessibility and (MIS) representation in image descriptions of race, gender, and disability,” in Proceedings of the 2021 CHI Conference on Human Factors in Computing Systems (New York, NY), 1–19. doi: 10.1145/3411764.3445498
Bennett, C. L., and Keyes, O. (2020). What is the point of fairness? disability, ai and the complexity of justice. ACM SIGACCESS Accessib. Comput. 5, 1. doi: 10.1145/3386296.3386301
Bhargava, S., and Forsyth, D. (2019). Exposing and correcting the gender bias in image captioning datasets and models. arXiv preprint arXiv:1912.00578.
Blodgett, S. L., Barocas, S., Daumé, I. I. I. H, and Wallach, H. (2020). Language (technology) is power: A critical survey of “bias” in nlp. arXiv preprint arXiv:2005.14050. doi: 10.18653/v1/2020.acl-main.485
Bolukbasi, T., Chang, K.-W., Zou, J. Y., Saligrama, V., and Kalai, A. T. (2016). “Man is to computer programmer as woman is to homemaker? debiasing word embeddings,” in Proceedings of the 30th International Conference on Neural Information Processing Systems (New York, NY), 4356–4364.
Buolamwini, J., and Gebru, T. (2018). “Gender shades: Intersectional accuracy disparities in commercial gender classification,” in Conference on Fairness, Accountability and Transparency (PMLR) 77–91.
Burrell, J. (2016). How the machine “thinks”: Understanding opacity in machine learning algorithms. Big Data Soc. 3, 2053951715622512. doi: 10.1177/2053951715622512
Calders, T., and Žliobaitė, I. (2013). “Why unbiased computational processes can lead to discriminative decision procedures,” in Discrimination and Privacy in the Information Society: Data Mining and Profiling in Large Databases. Berlin: Springer. 43–57. doi: 10.1007/978-3-642-30487-3_3
Campolo, A., Sanfilippo, M. R., Whittaker, M., and Crawford, K. (2017). Ai Now 2017 Report. AI Now Institute.
Crawford, K., and Paglen, T. (2021). Excavating ai: The politics of images in machine learning training sets. AI Soc. 36, 1105–1116. doi: 10.1007/s00146-021-01301-1
De Vries, T., Misra, I., Wang, C., and Van der Maaten, L. (2019). “Does object recognition work for everyone?,” in Proceedings of the IEEE/CVF Conference on Computer Vision and Pattern Recognition Workshops 52–59.
Floridi, L. (2014). The Fourth Revolution: How the Infosphere is Reshaping Human Reality. Oxford: OUP Oxford.
Friedman, B., and Nissenbaum, H. (1996). Bias in computer systems. ACM Trans. Inf. Syst. 14, 330–347. doi: 10.1145/230538.230561
Gebru, T., Morgenstern, J., Vecchione, B., Vaughan, J. W., Wallach, H., Daum, I. I. I., et al. (2018). Datasheets for datasets. arXiv preprint arXiv:1803.09010.
Greene, D., Hoffmann, A. L., and Stark, L. (2019). “Better, nicer, clearer, fairer: A critical assessment of the movement for ethical artificial intelligence and machine learning,” in Proceedings of the 52nd Hawaii International Conference on System Sciences. doi: 10.24251/HICSS.2019.258
Guo, W., and Caliskan, A. (2021). “Detecting emergent intersectional biases: Contextualized word embeddings contain a distribution of human-like biases,” in Proceedings of the 2021 AAAI/ACM Conference on AI, Ethics, and Society 122–133. doi: 10.1145/3461702.3462536
Harlan, C., and Zakowiecki, P. (2022). Poland builds a border wall, even as it welcomes ukrainian refugees. Available online at: https://www.washingtonpost.com/world/2022/04/13/poland-refugees-wall-belarus/ (accessed September 8, 2023).
Hegelich, S. (2022). Der nutzerismus: Eine ideologie mit totalitrem potential. Available online at: https://www.heise.de/meinung/Der-Nutzerismus-Eine-Ideologie-mit-totalitaerem-Potential-7268404.html (accessed September 8, 2023).
Hendricks, L. A., Burns, K., Saenko, K., Darrell, T., and Rohrbach, A. (2018). “Women also snowboard: Overcoming bias in captioning models,” in Proceedings of the European Conference on Computer Vision (ECCV) 771–787. doi: 10.1007/978-3-030-01219-9_47
Hutchinson, B., Prabhakaran, V., Denton, E., Webster, K., Zhong, Y., and Denuyl, S. (2020). Unintended machine learning biases as social barriers for persons with disabilitiess. ACM SIGACCESS Access. Comput. 9, 1. doi: 10.1145/3386296.3386305
Kamar, E., Hacker, S., and Horvitz, E. (2012). “Combining human and machine intelligence in large-scale crowdsourcing,” in AAMAS. International Foundation for Autonomous Agents and Multiagent Systems (IFAAMAS).
Katzman, J., Wang, A., Scheuerman, M., Blodgett, S. L., Laird, K., Wallach, H., et al. (2023). Taxonomizing and measuring representational harms: A look at image tagging. arXiv preprint arXiv:2305.01776. doi: 10.1609/aaai.v37i12.26670
Keyes, O. (2018). “The misgendering machines: Trans/HCI implications of automatic gender recognition,” in Proceedings of the ACM on Human-Computer Interaction 2, 1–22. doi: 10.1145/3274357
Krupiy, T. T. (2020). A vulnerability analysis: Theorising the impact of artificial intelligence decision-making processes on individuals, society and human diversity from a social justice perspective. Comput. Law Secur. Rev. 38, 105429. doi: 10.1016/j.clsr.2020.105429
Luccioni, A., and Viviano, J. (2021). “What's in the box? an analysis of undesirable content in the common crawl corpus,” in Proceedings of the 59th Annual Meeting of the Association for Computational Linguistics and the 11th International Joint Conference on Natural Language Processing (Volume 2: Short Papers) 182–189. doi: 10.18653/v1/2021.acl-short.24
Luo, R., Price, B., Cohen, S., and Shakhnarovich, G. (2018). “Discriminability objective for training descriptive captions,” in Proceedings of the IEEE conference on computer vision and pattern recognition 6964–6974. doi: 10.1109/CVPR.2018.00728
Magee, L., Ghahremanlou, L., Soldatic, K., and Robertson, S. (2021). Intersectional bias in causal language models. arXiv preprint arXiv:2107.07691.
Misra, I., Zitnick, C. L., Mitchell, M., and Girshick, R. B. (2015). Learning visual classifiers using human-centric annotations. CoRR, abs/1512.06974.
Mittelstadt, B. D., Allo, P., Taddeo, M., Wachter, S., and Floridi, L. (2016). The ethics of algorithms: Mapping the debate. Big Data Soc. 3, 2053951716679679. doi: 10.1177/2053951716679679
Mozur, P. (2019). One month, 500,000 face scans: How china is using ai to profile a minority. The New York Times 14.
Nadeem, M., Bethke, A., and Reddy, S. (2020). Stereoset: Measuring stereotypical bias in pretrained language models. arXiv preprint arXiv:2004.09456. doi: 10.18653/v1/2021.acl-long.416
Noble, S. (2021). Algorithms of oppression: How search engines reinforce racism. Science 374, 542–542. doi: 10.1126/science.abm5861
O'Neil, C. (2018). Weapons of Math Destruction: How Big Data Increases Inequality and Threatens Democracy. London: Penguin Books.
Otterbacher, J., Barlas, P., Kleanthous, S., and Kyriakou, K. (2019). “How do we talk about other people? Group (un) fairness in natural language image descriptions,” in Proceedings of the AAAI Conference on Human Computation and Crowdsourcing 106–114. doi: 10.1609/hcomp.v7i1.5267
Salisbury, E., Kamar, E., and Morris, M. (2017). “Toward scalable social alt text: Conversational crowdsourcing as a tool for refining vision-to-language technology for the blind,” in Proceedings of the AAAI Conference on Human Computation and Crowdsourcing 147–156. doi: 10.1609/hcomp.v5i1.13301
Schiff, D., Biddle, J., Borenstein, J., and Laas, K. (2020). “What's next for ai ethics, policy, and governance? a global overview,” in Proceedings of the AAAI/ACM Conference on AI, Ethics, and Society 153–158. doi: 10.1145/3375627.3375804
Sharma, P., Ding, N., Goodman, S., and Soricut, R. (2018). “Conceptual captions: A cleaned, hypernymed, image alt-text dataset for automatic image captioning,” in Proceedings of the 56th Annual Meeting of the Association for Computational Linguistics 2556–2565. doi: 10.18653/v1/P18-1238
Stangl, A., Morris, M. R., and Gurari, D. (2020). “Person, shoes, tree is the person naked? What people with vision impairments want in image descriptions,” in Proceedings of the 2020 CHI Conference on Human Factors in Computing Systems 1-13. doi: 10.1145/3313831.3376404
Stangl, A., Verma, N., Fleischmann, K. R., Morris, M. R., and Gurari, D. (2021). “Going beyond one-size-fits-all image descriptions to satisfy the information wants of people who are blind or have low vision,” in Proceedings of the 23rd International ACM SIGACCESS Conference on Computers and Accessibility 1–15. doi: 10.1145/3441852.3471233
Tang, R., Du, M., Li, Y., Liu, Z., Zou, N., and Hu, X. (2021). “Mitigating gender bias in captioning systems,” in Proceedings of the Web Conference 633–645. doi: 10.1145/3442381.3449950
Van Miltenburg, E., Elliott, D., and Vossen, P. (2018). “Talking about other people: an endless range of possibilities,” in Proceedings of the 11th International Conference on Natural Language Generation 415–420. doi: 10.18653/v1/W18-6550
Vaswani, A., Shazeer, N., Parmar, N., Uszkoreit, J., Jones, L., Gomez, A. N., et al. (2017). “Attention is all you need,” in Advances in Neural Information Processing Systems 5998–6008.
Wang, A., Barocas, S., Laird, K., and Wallach, H. (2022). “Measuring representational harms in image captioning,” in 2022 ACM Conference on Fairness, Accountability, and Transparency 324–335. doi: 10.1145/3531146.3533099
Wu, S., Wieland, J., Farivar, O., and Schiller, J. (2017). “Automatic alt-text: Computer-generated image descriptions for blind users on a social network service,” in Proceedings of the 2017 ACM Conference on Computer Supported Cooperative Work and Social Computing 1180–1192. doi: 10.1145/2998181.2998364
Yan, T., Kumar, V., and Ganesan, D. (2010). “Crowdsearch: Exploiting crowds for accurate real-time image search on mobile phones,” in Proceedings of the 8th International Conference on Mobile systems, Applications, and Services 77–90. doi: 10.1145/1814433.1814443
Zhao, D., Wang, A., and Russakovsky, O. (2021). “Understanding and evaluating racial biases in image captioning,” in Proceedings of the IEEE/CVF International Conference on Computer Vision 14830–14840. doi: 10.1109/ICCV48922.2021.01456
Keywords: AI-generated image captions, representational harms, inclusion, responsible AI, AI harms frontiers
Citation: Sarhan H and Hegelich S (2023) Understanding and evaluating harms of AI-generated image captions in political images. Front. Polit. Sci. 5:1245684. doi: 10.3389/fpos.2023.1245684
Received: 23 June 2023; Accepted: 01 September 2023;
Published: 20 September 2023.
Edited by:
Domenico Marino, Mediterranea University of Reggio Calabria, ItalyReviewed by:
Zlatko Hadzidedic, International Center for Minority Studies and Intercultural Relations, BulgariaSeung-Youn Oh, Bryn Mawr College, United States
Copyright © 2023 Sarhan and Hegelich. This is an open-access article distributed under the terms of the Creative Commons Attribution License (CC BY). The use, distribution or reproduction in other forums is permitted, provided the original author(s) and the copyright owner(s) are credited and that the original publication in this journal is cited, in accordance with accepted academic practice. No use, distribution or reproduction is permitted which does not comply with these terms.
*Correspondence: Habiba Sarhan, aGFiaWJhLnNhcmhhbkB0dW0uZGU=