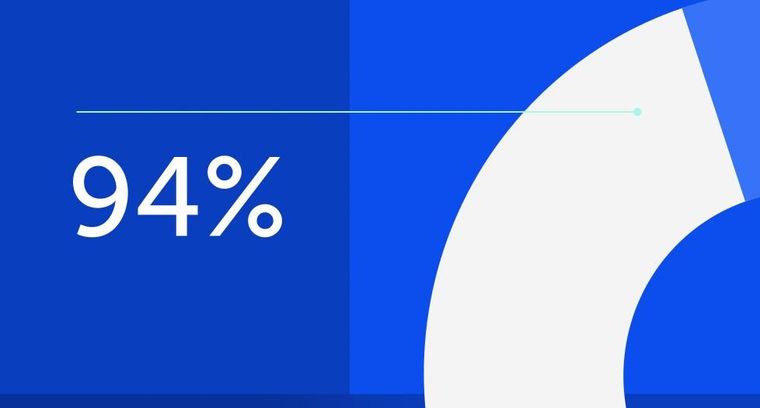
94% of researchers rate our articles as excellent or good
Learn more about the work of our research integrity team to safeguard the quality of each article we publish.
Find out more
ORIGINAL RESEARCH article
Front. Polit. Sci., 20 December 2023
Sec. Politics of Technology
Volume 5 - 2023 | https://doi.org/10.3389/fpos.2023.1238461
This article is part of the Research TopicHumans in the Loop: Exploring the Challenges of Human Participation in Automated Decision-Making SystemsView all 4 articles
This paper draws on three case studies to examine some of the challenges and tensions involved in the use of Autonomous Decision-Making Systems (ADMS). In particular, the paper highlights: (i) challenges around the shifting “locale” of the decision, and the associated consequences for stakeholders; (ii) potential implications for stakeholders from regulation such as the General Data Protection Regulation (GDPR); (iii) the different values that stakeholder groups bring to the “decision” question; (iv) how complex pre-existing webs of stakeholders and decision-making authorities may be disrupted or disempowered by the use of an automated system and the lack of evaluation of possible consequences; (v) how ADMS for non-technical users can lead to circumvention of the boundaries of intended system use. We illustrate these challenges through case studies in three domains: adult social care, aviation, and vehicle driver monitoring systems. The paper closes with recommendations for both practice and policy in the deployment of ADMS.
Autonomous Decision-Making Systems (ADMS) are one example of increasingly complex computing systems that can take in and respond to information, either digitally or physically, producing outputs that can either support or replace human decision-making. ADMS can be defined as “a (computational) process, including AI techniques and approaches, that, fed by inputs and data received or collected from the environment, can generate, given a set of predefined objectives, outputs in a wide variety of forms (content, ratings, recommendations, decisions, predictions, etc.)” (ELI, 2022). ADMS can be used in any field or realm in which a decision may be made: an ADMS has the potential to either augment or be substituted for a human decision or decision-maker. As the following case studies demonstrate, this breadth of application indicates that field- and domain-specific challenges should be carefully evaluated and that generalizable principles should be formulated with care.
While attitudes toward acceptability of ADMS vary by application and individual, there are clear potential benefits of such systems. The individual decision-making capacity of humans is relatively limited, and scales on an individual level: one human can only respond to so many stimuli at once. Conversely, once an ADMS is trained, it is then unlimitedly replicable. Besides computational efficiency, the use of ADMS may present other potential advantages such as safety, cost reductions, efficiency, and accuracy.
However, there are inherent challenges to ADMS. Human decision making is often based on a wide variety of heavily contextual factors, many of which are extremely difficult to distill into discrete data streams and be assigned different weights. Additionally, ADMS may either retain or introduce undesirable errors or bias, which may then be replicated on an ongoing basis until the error is detected. This is a sizeable task—while it may be comparatively simple to see where a physically-embodied system, such as an automated light rail system, has gone wrong, it would be comparatively difficult to uncover a systemic issue such as assigning higher fares to certain passengers using that system. Consequently, there are multiple kinds of harm that may ensue, including both direct physical harm and indirect or non-physical harm. These will vary depending on what outcome the ADMS was built to accomplish, as well as the way in which it was designed to accomplish that goal. A non-physical ADMS error may also result in physical harm, for example, an ADMS recommending that a patient take a certain medication that is contra-indicated. The number of variables therefore makes it problematic to generalize about ADMS except insofar as to note that they should be carefully assessed with a broad understanding of the context, and anticipatory work to understand possible outcomes.
The shortcomings of ADMS and the serious harms they could bring about to humans, in particular in safety critical domains such as healthcare, warfare, finance, and justice, are the main reasons to introduce human beings into the “loop” of these automated systems, which is frequently presented as a crucial means of achieving accountability and oversight. However, the concept of meaningful human control presents us with something of a paradox. On the one hand, by designing systems that can make decisions autonomously, we attempt to reduce or remove human involvement in order to increase safety (avoiding human error); reduce costs (replacing humans with automation); or improve efficiency (predicting the behavior of something). On the other hand, the growing call to supervise autonomous systems in order to achieve ethical goals such as fairness, through human oversight and accountability (e.g., Ozmen Garibay et al., 2023), reintroduces the impacts of human involvement. In fact, meaningful human control presents several challenges too.
Of major concern is the issue of quasi-automation, which essentially entails humans acting simply as a rubber-stamping mechanism in an otherwise completely automated decision-making system (Wagner, 2019). Often companies do not sufficiently train their staff or provide adequate time for making decisions. A key example is when Amazon came under scrutiny in 2018 for its recruiting tool algorithm that ranked candidates and turned out to be biased against women (Dastin, 2018). The algorithm gave applicants a score of 1–5 based on their resume but consistently discriminated against women as it was built on historical data which showed male dominance of the technology industry. Recruiters did not have much agency in choosing who to interview due to the volume of applicants and sparse time for decision-making. It is critical that certain criteria be created to define meaningful human control. The Article 29 Working Party has developed a classification relating to this: “[t]o qualify as human intervention, the controller must ensure that any oversight of the decision is meaningful, rather than just a token gesture. It should be carried out by someone who has the authority and competence to change the decision. As part of the analysis, they should consider all the available input and output data” (Article 29 Data Protection Working Party, 2017, p. 10).
The four questions below, summarize the main challenges for ADMS:
1. Whether an ADMS should be used—does the utility outweigh the inherent risks?
2. If it is acceptable for the system to be used, is human intervention required and under what conditions?
3. If the system is used, what level of human oversight over the operation as a whole is appropriate?
4. If a certain level of oversight is needed, can that oversight feasibly be undertaken by a human?
All four of these challenges are in essence a risk-balancing exercise. For (1), this risk balancing includes consideration of whether and how often human error is likely to occur in practice, and the potential risks between human and ADMS use. For example, many societies have adapted well to traffic lights, and, though they are automated, do not argue for their abolishment in favor of a human signaler. As to (2), this includes a weighing of the type of risks that are likely to arise. In our traffic light example, there is no human at a central control board monitoring the conditions and timing of traffic lights. However, there would almost certainly be a human monitoring the administration of anesthetic during a surgery, even if certain portions of the procedure are automated. The third question of such a risk-balancing exercise refers to a level of recursive checks: how often, to what extent, and by what measures are the ADMS monitored? If a human is not “in the loop” at the time of use, it may still be necessary to perform checks on the system overall. For example, if using an automated weed-killing system by which an ADMS targeted certain plants it identified as weeds rather than all crops in a field, it may still be appropriate to monitor the level of chemicals deployed by the automated system overall. Finally, feasibility of human oversight must also be considered. For instance, it might be impossible for a human to check all data processed by an ADMS. Moreover, engaging with ADMS can affect the ways in which humans make decisions themselves, and can lead to physical and cognitive issues such as deskilling, automation bias, distraction and automation complacency (Parasuraman et al., 1993), among other issues. Such factors have raised concern in computer-aided tasks such as piloting aircraft (Carr, 2015), making medical decisions (Povyakalo et al., 2013) and driving semi-autonomous vehicles (Dunn et al., 2021).
The overall goal of this paper is to articulate the problems of human participation in ADMS. We will focus on three specific domains: social care, aviation, and road vehicles. After describing the methodology, we present the three case studies and finally, drawing on the lessons learned from them, we propose a number of reflections for the design, development and use of ADMS.
The first case study is based on a fieldwork conducted by one of the authors. We focus on an ADMS designed to (a) predict the risk of elderly adults falling (which can be very serious in this population) and (b) offer an enhanced care service to try and prevent such falls. This situation therefore provides an opportunity to examine questions around use, oversight, and monitoring of such systems, and provide draft guidance around what questions should be considered in cases where ADMS has the potential to support and enhance human decision-making.
The second and third case studies are both based on desk research. In the second, we examine a notorious event, the crashes of two Boeing 737 MAX which caused the deaths of 346 people. Among the main causes of the accidents was the Maneuvering Characteristics Augmentation System (MCAS), a software system designed to manage the aircraft's stabilizer. While many ADMS use big data or machine learning aspects, the MCAS does not, and is an interesting example of an algorithm designed to manage the aircraft's stabilizer, without human supervision. In the third case study, we examine Driver Monitoring Systems (DMS), which are integrated into partially-autonomous vehicles to ensure that the driver is adequately supervising the vehicle's automated functions.
The adult social care sector in England is undergoing what many commentators refer to as a “crisis” (Alderwick et al., 2019; Dowling, 2021). The crisis is generally regarded as arising from an imbalance between supply and demand in care services. An aging population with increasing comorbidities demands additional care; yet social care organizations—local authorities and private care providers–struggle to meet their needs due to decades of budget cuts under governmental “austerity” policies, and high attrition rates for underpaid social care workers (Hamblin, 2020; Wright, 2020; Dowling, 2021; The King's Fund, 2023).
Faced with these challenges, over the past two decades policymakers and sector associations have proposed various forms of digital technology as one possible remedy (Skills for Care, 2015; Hamblin, 2020; IIPC, 2021; DHSC, 2022; Wright and Hamblin, 2023). Recently, these proposals have turned toward data analytics and the use of ADMS. A repository of case studies on “AI in social care” in England, hosted by the country's digital transformation directorate, shows that many applications center around leveraging ADMS to facilitate preventative care programs (NHS England, n.d.).
In this case study, we draw from a 5-month field study on the implementation of such an ADMS within a social care organization in England. We begin by providing further context on the project, namely: the role of local authorities in adult social care and high-level descriptions of the system and the preventative care program. Then, we introduce the tension that arose during the study on determining the appropriate form and extent of human involvement in the ADMS.
“Adult social care” refers to a suite of care services for those 18 years or older who struggle with essential daily activities—such as eating, washing, and socializing—due to a physical or mental impairment or illness (NAO, 2021). In England, the 152 local authorities have devolved responsibility for the delivery of care services. “Service users” can receive their care either directly from the local authority or, more commonly, from private providers and volunteer or charitable organizations who have been commissioned by the local authority.
For the field study, a member of our research team enlisted as a participant observer on a team responsible for the implementation of an ADMS within the adult social services department of a local authority in England. The project team was composed of individuals from three different organizations: an operations consultancy, a technology provider, and the local authority. Responsibilities for different aspects of the project were distributed across these groups. For instance, the technology provider was subcontracted by the consultancy to help deliver the technology underlying the ADMS, while the consultancy managed the design and implementation of the preventative care program. Acting as the client, the local authority retained ultimate decision-making authority, but, of course, its decisions were influenced by the guidance of actors within the two other organizations.
The objective of the project was to implement an ADMS, jointly developed by the consultancy and technology provider, that would support a preventative care program focused on reducing the rate of falls among older adults in the community. Falls among older adults present a significant challenge to England's health and social care systems. Each year, around 30% of older adults living at home fall at least once, and these incidents impact an individual's quality of life and health, as well as adding substantial costs to health and social care services (NICE, 2013).
The ADMS is formed of two components: a natural language processing (NLP) framework and a machine learning (ML) model. As an input, the ADMS analyses case notes written by social care practitioners. Case notes are unstructured text data that social care practitioners create during their interactions with service users. The NLP framework—created by the technology provider—would extract “risks” from the case notes. For example, if the NLP framework identifies the word “fall” in a case note, it would indicate that this particular service user has a fall risk. The NLP framework is shielded behind intellectual property protection; in effect, only the technology provider has insight into its structure and functioning. This process then results in a “master risk table”: a structured data set that presents occurrences of risks for each service user. Using different combinations of risks as features, a binary classification algorithm was trained on this structured data set to create a model to predict whether a service user is likely to have a fall risk appear in their case notes in the next 9 months. The model calculates a probability score for each service user, and those with a score over a set threshold are identified to be at risk of falling.
Once the ADMS identifies service users who are at risk of falling, they are allocated to the local authority's new falls prevention program. After a series of preliminary checks to ensure that non-eligible individuals are not contacted—which include identifying and excluding those who are deceased, in residential care, or under the age of 65—the list of service users are shared with a call center team. Members of this team are non-clinical practitioners: while they do not have a background in health or social care, they have experience performing this role for similar public health programs. This team contacts each service user to conduct an over-the-phone assessment, asking a series of predefined questions; the purpose of these assessments is to gain a better understanding of the factors that lead to an individual being at risk of falling. Based on this discussion, the call center team member would allocate the individual to an appropriate prevention service, such as exercise classes for mobility and strength or a home safety assessment.
Using this case, we explore the complexities of human involvement in ADMS. To structure our discussion, we draw from the UK General Data Protection Regulation's (UK GDPR) provision on automated decision-making. Apart from guidance on automated decision-making, UK GDPR of course includes extensive requirements on data privacy. The project team navigated several processes, including: negotiating data sharing agreements across multiple organizations; drafting data protection impact assessments; communicating privacy-related decisions to service users; and implementing several modes of data quality evaluation. These tasks take up considerable time and expertise and highlight the complicated tasks practitioners must perform when following data protection regulation in the public sector.
Despite these efforts, UK GDPR, and in particular Article 22 (1), generate several ambiguities that practitioners must contend with. We argue that these difficulties are not restricted to any one group, but are relevant to all organizations hoping to comply with this provision. In fact, scholars such as Binns and Veale (2021) have previously argued that Article 22 (1) is limited by several such complications. Through our case study, we observe two of the ambiguities identified by Binns and Veale (2021), lending an empirical basis to their conceptual analysis.
In this section, we first provide a brief description of Article 22 (1), underlining the restrictions it imposes on the use of automated decision-making. Leveraging the work of Binns and Veale (2021), we point to two ambiguities found when interpreting the regulation: the notion of singular decision, and deciding on what form human involvement will take. The remainder of the section explores those two points in further detail, drawing on empirical data from our case study.
Article 22 (1) of the UK GDPR delineates the bases for the lawful use of automated decision-making and profiling. Specifically, it states:
The data subject shall have the right not to be subject to a decision based solely on automated processing, including profiling, which produces legal effects concerning him or her or similarly affects him or her (ICO, 2018).
While on initial review the provisions laid out in Article 22 (1) seem fairly categorical, serious questions are left to discretion when interpreting this guidance in practice. These ambiguities rose to prominence throughout our case, emphasized by the conflicting interpretations of two aspects of Article 22 (1) held by different members of the project team: what decision is being automated? and what form should human involvement take?
Article 22 (1) specifies that individuals have the right to not be subject to a solely automated decision which has a legally significant effect. This language, particularly the notion of “a decision,” seems to imply that there is a unitary point at which a decision is made that can be considered in isolation: for example, deciding whether an individual is eligible for a loan or if a person should be interviewed for a new role. Yet, as the following discussion elaborates, locating the specific decision (Binns and Veale, 2021) that may be considered automated proves in practice to be challenging. In real applications of ADMS such as this, these systems sit within complex processes composed of “upstream” and “downstream” decision points.
Throughout the project, team members frequently reminded one another that the ADMS is not deciding what sort of care someone should receive. That decision is always left to a practitioner, made through the over-the-phone assessment conversation.
Yet, by broadening the perspective to focus on the entire process, other significant decisions that have the potential to be automated become apparent. One alternative framing, brought up several times by a practitioner closely involved with the project's data privacy considerations, is that sharing the predictions made by the ADMS with the call center team constitutes a decision. This view brought out questions of whether data sharing has a “legally significant effect” on an individual and assurances that data sharing agreements between organizations adequately cover this transfer of information. Meanwhile, moving further “upstream” in the process highlights other decisions that must be considered; for example, whether an individual is eligible for services in the first place. From this vantage point, measures for meaningful human control must move further up in the process, and the concern becomes less one of preserving practitioner expertise than of equitable access to services.
These examples highlight how the location and boundaries of the “decision” can take many different shapes, each formulation bringing with it a different set of questions and considerations. When the decision under question is what care a person should receive, anxieties over practitioner expertise take center stage. But, once the lens shifts toward whether the decision is one of data sharing or eligibility for services, questions of privacy, data sharing, and access gain prominence.
The next ambiguity emerges from Article 22's statement that decisions shall not be solely automated. An immediate implication of this requirement is that there must be some form of meaningful human control over the outcomes of a decision-making process. In our case study, this prompted significant debate over what constitutes proportionate human involvement. While this question presents several interesting directions for research, such as what mechanisms of control satisfy the requirement that human involvement is “active and not just a token gesture” (ICO, 2018), they are not the focus of our present discussion. Instead, we use this provision and the discussion it engenders on mechanisms for meaningful human control to explore a different complexity. ADMS works to categorize people: in this case, service users are allocated into “high” or “low” risk subgroups. For both high and low risk service users, UK GDPR mandates that decisions should not be based solely on automated processing. Yet, as the following discussion illustrates, this requirement points to an impracticality when deploying ADMS that operate at the population level.
When working to satisfy this guideline, the project team considered two routes for meaningful human control. The first would involve a practitioner in the local authority manually reviewing the list of people predicted by the ADMS to be at risk of falling. This practitioner would review the case notes of each individual and either confirm or reject the ADMS' output. Others proposed to use a set of exclusion criteria to identify people predicted by the ADMS but who are not in fact eligible for the program. For example, using Excel, they would check whether the list of predictions included any people who were in a care home or are deceased; if an individual met either of these criteria, he or she would not be contacted by the call center and would therefore be excluded from the program. At the time of writing, the ADMS predictions are disseminated as an Excel spreadsheet which includes the service user's personal information, the probability estimate generated by the ML model, and the top 15 most locally important features for each prediction.
As these proposed interventions show, the focus was primarily on ensuring that people who are not eligible for the preventative care program are not erroneously included. In other words, these interventions sought to limit the number of false positives made by the ADMS.
But false positives are only one side of the story: in classification tasks such as this, ADMS also produces another type of error: false negatives. In this case, that means people who are actually at risk of falling but are not predicted as such by the ADMS. Although UK GDPR would require each individual, regardless of his or her estimated level of risk, to be subject to some form of active human involvement, this provision proves infeasible when ADMS generate probability estimates for thousands of service users. How organizations should account for this provision when deploying ADMS that operate at the population level thus remains an open question.
Article 22 (1) of UK GDPR presents significant complications to organizations attempting to deploy ADMS. Some of these challenges are amplified when the system in question operates at the population level, as highlighted by the requirement that human involvement must be active across all predictions. By connecting the conceptual discussion of Binns and Veale (2021) to the on-the-ground work of practitioners in a real-world organization, we show how such complications are not merely speculative or isolated to one particular organization, but are in fact challenges that many organizations planning to deploy ADMS will have to contend with.
As a second case study, we examine the story of the two fatal crashes of Boeing 737 MAX: the Lion Air 610 on October 29, 2018 and Ethiopian 302, on March 10, 2019. The MCAS system (Maneuvering Characteristics Augmentation System), a flight control software designed to prevent stalls, is known to have been the main cause of the two plane crashes. Aviation industry is usually regarded as a model for safety. However, in this particular case, the Boeing 737 MAX example is useful for reflecting on how AI systems should and should not be implemented. Mongan and Kohli (2020) draw five lessons from the 737 MAX disaster, and apply these to the implementation of AI in medicine in general and radiology specifically.
In the opinion of the present authors, the lessons learned from the Boeing MCAS case can more broadly provide relevant insights on the design, development and deployment of ADMS in general.
We set out to an overview of what happened and highlight open questions around human involvement focusing on the interactions with the flight control software and beyond. As we shall see, the explanations of the accidents include other factors and issues emerging from failures at different levels of granularity: leadership, governance, engineering, risk analysis and safety culture (Sullenberger, 2019).
The context is that of market competition between Boeing and Airbus, the two largest aircraft manufacturers in the world. In December 2010, Airbus announced the launch of the A320neo family and in August 2011 Boeing responded with the launch of the 737 MAX family (Herkert et al., 2020). The 737 MAX is an update of the latest 737NG, a model dating back to the 60s (Herkert et al., 2020). The most substantial change to the old model concerns the engines, which have been replaced with more fuel-efficient engines. However, the new engines are larger than the old ones and consequently have had to be mounted in a higher and more forward position on the wing. This change created an aerodynamic stability problem, which was discovered in the late flight testing phase: the high risk of a stall in certain flight conditions. The engineers decided to solve this hardware problem by developing a flight control software—the MCAS (Gates, 2019a,b). The MCAS can be described as a closed-loop system that activates automatically during manual flight, without the pilots being aware of it, and repeatedly adjusts the plane's stabilizer every time the Angle of Attack (AOA) sensor signals a dangerous up-pitch inclination of the plane's nose. The AOA sensor measures the angle formed between the direction of the air during flight and the nose of the aircraft. If the angle increases to the point of stall, in some cases, it could lead to a crash. The MCAS task is to bring the plane nose down in order to avoid a stall situation.
The MAX passed the certification procedures by the US FAA and entered service in May 2017. What happened then is well known. In 2019 the planes were grounded and Boeing announced the suspension of production. The accident investigations led to the discovery that the triggering cause was the malfunction of the AOA sensor and highlighted serious safety flaws at numerous stages: in the design of the MCAS; in the assessment of the risks associated with its operation; in the certification procedures; and, above all, in the omission of relevant information to pilots and flight companies (Herkert et al., 2020).
In November 2020, the Boeing 737 returned to service after being grounded for around 20 months (The Boeing Company, n.d.). During this period, the US company claims to have made improvements to the 737 MAX, including MCAS; the pilot training procedure; and the process for returning the aircraft to service.
With respect to MCAS, the main updates consist of increased levels of protection in response to the flight control system weaknesses:
• “Measurements from two Angle of Attack (AOA) sensors will be compared.
• Each sensor will submit its own data to the airplane's flight control computer.
• MCAS will only be activated if both sensors agree.
• MCAS will only be activated once.
• MCAS will never override the pilot's ability to control the airplane using the control column alone” (The Boeing Company, n.d.).
To overcome the problem of possible incorrect readings from the AOA sensor, since all 737 MAX aircraft have two AOA sensors, the new MCAS software is activated only if the values of both sensors are in agreement. If there is a discrepancy, a flashing light warns the pilots. In addition, while in the previous version, the MCAS started automatically and repeatedly every time the sensor reads the data, in the updated version it corrects only once and without disabling the control column, therefore allowing the pilot to always take control (The Boeing Company, n.d.).
In January 2021, the US charged Boeing with fraud against the Federal Aviation Administration's Aircraft Evaluation Group (FAA AEG) for failing to provide relevant information about MCAS during certification procedure and in pilot manuals and training guidance. According to Acting Assistant Attorney General David P. Burns of the Justice Department's Criminal Division: “Boeing's employees chose the path of profit over candor by concealing material information from the FAA concerning the operation of its 737 Max airplane and engaging in an effort to cover up their deception. This resolution holds Boeing accountable for its employees' criminal misconduct, addresses the financial impact to Boeing's airline customers, and hopefully provides some measure of compensation to the crash-victims' families and beneficiaries.” (Burns in The United States Department of Justice, 2021). However, the company was able to avoid going on trial, by agreeing to pay $2.5 bn, including $500 m to the families of those killed, and promising to tighten up its compliance procedures (Leggett, 2023).
The Boeing 737 MAX story demonstrates that human involvement with ADMS, in this case the MCAS, is not just about those who have to interact with it (i.e., the pilots). The involvement of people begins earlier in the design phase and it is also affected by non-technological factors. As pointed out by Sullenberger (2019) “Accidents are the end result of a causal chain of events, and in the case of the Boeing 737 MAX, the chain began with decisions that had been made years before, to update a half-century old design”. Among the many failures are: the competition with Airbus that prompted Boeing's managers and engineers to try and use software to remediate a hardware problem; flawed design with grave errors in safety; the lack of funds and resources in the FAA which led it to increasingly delegate certification work to Boeing itself; the conflict of interests of Boeing technical flight pilots (Boeing's employees) responsible for the risk assessment evaluation and successful certification of their employer's product. As Chang, Lee, and Mas argue, the Boeing affair is not a case of a “computer bug” but of a scandal originating out of economic interests (Chang et al., 2019). In this respect it bears comparison with the Challenger Space shuttle disaster (Werhane, 1991).
In relation to MCAS, a number of design issues can be highlighted which may also be relevant to ADMS in general. According to the accident investigations, the main triggering event was malfunction of the AOA sensor rather than a malfunction of the MCAS software. The software would—in theory—have done its job correctly, but its “decision” was based on the wrong data. As pointed out by Mongan and Kohli (2020), “such a failure illustrates that the output of an AI system is only as good as its inputs”. To date it is not known what caused the malfunction of the sensor, but the crucial question is: why did the engineers rely on just one sensor reading for such a critical function, in so doing violating the principles of security, especially that of redundancy? This is even more egregious because it was known MAX 737 planes had two AOA sensors, and despite the fact that “the black box data provided in the preliminary investigation report shows that readings from the two sensors differed by some 20 degrees not only throughout the flight but also while the airplane taxied on the ground before takeoff” (Gates, 2019b).
Related to this design issue is the authority given to the MCAS, in other words, the excessive controlling power of the system compared to the pilots. The system was allowed to tilt by 2.5 degrees (in an earlier version the tilt variation was 0.6 degrees, but then was modified because this was not enough). According to Sullenberger (2019), “Boeing designers also gave MCAS too much authority, meaning that they allowed it to autonomously move the horizontal stabilizer to the full nose-down limit”.
Not only was this authority conferred on the MCAS system, but moreover, it was designed to activate independently, and repeatedly, without the pilots being aware of it. In fact, the pilots were designedly unaware of the existence of MCAS—a deliberate omission on Boeing's part. According to Travis “MCAS was not disclosed to pilots in order to preserve the fiction that the 737 MAX was just an update of an earlier 737 model which served as a way to circumvent the more stringent FAA certification requirement for a new airplane” (Herkert et al., 2020). Finally, in the original version of MCAS the ability to disable the stabilization system using the conventional control levers was removed, in this way preventing pilots from quickly overriding the system. According to Boeing's engineers, the authority given to MCAS was nothing exceptional. It should have been activated in the background, only in special emergency situations. However, the problem is not just one of transparency, but of safety risk assessment: the Boeing engineers did not question what happens if this system fails? How can this be remedied? In fact, they did, but in the wrong way. They did not categorize MCAS failure as a critical “because they assumed that pilot action would be the ultimate safeguard” (Sullenberger, 2019).
In this case, it can be seen that the failure to anticipate possible poor outcomes led to an increase in overall risk.
Related to the risks associated with the failure of the MCAS is the question of how to manage an emergency situation. So here the question is not what happens if the MCAS stops working but what pilots can do in such a critical situation?
This is a matter of human factors in emergency situations. As pointed out by Sullenberger (2019), besides activating the MCAS, “in both 737 MAX accidents, the failure of an AOA sensor quickly caused multiple instrument indication anomalies and cockpit warnings. And because in this airplane type the AOA sensors provide information to airspeed and altitude displays, the failure triggered false warnings simultaneously of speed being too low and also of speed being too fast. The too slow warning was a ‘stick-shaker' rapidly and loudly shaking the pilots' control wheel. The too fast warning was a ‘clacker', another loud repetitive noise signalizing overspeed”. Of course individual characteristics can make the difference in these situations. Indeed, according to other experts, the case of the Boeing 737 crashes is also a matter of pilot training. Indeed, Langewiesche (2021) points out that crew performance was critical in these accidents. Sullenberger (2019) goes on saying that he recreated in a flight simulator the cockpit situation with all warnings of the accident flights and concludes “Even knowing what was going to happen, I could see how crews could have run out of time and altitude before they could have solved the problems”. It is clear that in such a situation it can be difficult to manage and solve the problem.
In conclusion, for ADMS in general human understandability is paramount, even more so in safety critical situations where there is little time to work with the system.
In the third case study we examine how over-reliance on automated systems can occur not only at the governance, engineering, or technical user level but also at the consumer level. ADMS can capitalize on consumer desire to offload difficult tasks to machines and can exhibit system design that allows the user to have peace of mind by gaming compliance of supervision without actually complying in the intended manner. One such example is misleading the Driver Monitoring Systems (DMS) on semi-autonomous vehicles.
The automotive industry has been striving to make driving safer through the development of Advanced Driver Assistance System (ADAS) and autonomous vehicle technologies such as Adaptive Cruise Control (ACC) and Automatic Lane Keeping Systems (ALKS). These technologies have already shown some success in preventing accidents as well as helping drivers practice safer habits (Isaksson-Hellman and Lindman, 2016; Spicer et al., 2018; Lyu et al., 2019; Gouribhatla and Pulugurtha, 2022). As their capabilities increase and they burgeon on true self-driving capabilities, the temptation arises to use the system not merely as a driving assist or safety aide but as a replacement for the driving task itself. Because ADAS capabilities are still limited, supervision of these vehicles is required by the driver. However, monitoring the vehicle and the environment can be fatiguing, making it difficult to remain vigilant, even for eager operators (Körber et al., 2015; Arakawa et al., 2019; Vogelpohl et al., 2019; Huang et al., 2021). Long-term studies have shown that increased familiarity and experience with an ADAS can lead to more distracted driving behaviors and more time with eyes off the road (Dunn et al., 2021; Morando et al., 2021; Reagan et al., 2022). ADAS functions may also make drivers more likely to speed (Monfort et al., 2022).
Because of ADAS capability limitations, at any given moment it must be determined if the vehicle is adequately able to navigate the environment and if the driver is in a state to handle the driving task. Naturally, determining these things is difficult for two reasons: (1) It is an unsolved problem for a vehicle to accurately estimate its own ability in any given environment (Michelmore et al., 2018; Stocco et al., 2020), and (2) it is difficult to measure current driver distraction or fatigue to understand if the driver is in a state to safely conduct the vehicle (Albadawi et al., 2022). In this case study, we will focus on the second issue of using ADMS in the form of DMS to estimate driver readiness.
Driver inattention of ADAS functions has already been considered a major contributing factor in a couple of notable crashes (NTSB, 2017, 2020). For this reason, DMS are becoming a common feature on vehicles with Level 2 (L2) and Level 3 (L3) autonomous driving features (as defined by SAE International, 2021). DMS can vary in what they measure and how they perform measurements. In research settings, DMS often measure physiological responses of the driver by using Electrocardiogram (ECG) or Galvanic Skin Response (GSR) techniques. However, these methods usually involve attaching sensors to the human's skin and thus are not done in production systems (Begum, 2013). In production vehicles, popular methods include checking seat weight, checking seat belt connectivity, monitoring eye gaze, feeling pressure of a hand on the wheel, or using skin conductance to check if a hand is on the wheel (United Nations, 2021; Gross, 2022). Overall, DMS have several notable benefits including:
• Detecting if the driver is not safely supervising the vehicle, potentially protecting vehicle occupants and other road users from unsafe driving.
• Detecting a medical emergency of the driver and maneuvering the vehicle to a safe location.
• Allowing the driver time to take over when the system anticipates it will need to disengage soon.
• Recording user state to help with accident scenario investigations.
However, DMS are not created equally and the underlying assisted driving functions may not be able to perform all of the above functions (including emergency maneuvers) in all situations (Capallera et al., 2019; Monticello, 2023). Both regulators and automakers have promoted DMS as a solution to permitting imperfect autonomous systems in vehicles. The idea is that they will enforce drivers to maintain the legally required supervision over L2 systems even if the driver over-trusts system capabilities. According to Rule 150 of the UK Highway Code, the driver is always responsible when using driver assistance systems and must have full control over the systems at all times (Gov.uk, 2022, 2023). The Alliance for Automotive Innovation (2023) has proposed that DMS should be a standard feature of all L2 systems, however, the recommendation allows for rudimentary torque-based steering detection as a form of DMS. As ADAS advance, the United Nations has created provisions to require DMS on L3 vehicles (United Nations, 2021). The UK Law Commission found that experts express concerns about proving the safety of assisted driving features due to the infancy of DMS currently found in vehicles (UK Department for Transport, 2021). In the United States, a bill has been introduced that, if passed, would require all new vehicles with ADAS functions to have a DMS installed as early as 2027 (Congress.gov, 2021).
Analyzing the role of human involvement in DMS we draw out four main takeaways:
1. ADMS aimed at non-technical users do not guarantee user understanding of the system, potentially leading to unsafe scenarios.
2. ADMS should be avoided if possible in situations where there is little oversight and the operator has incentive to trick or override the system.
3. DMS are currently rudimentary and may not function appropriately even for users attempting to comply.
4. DMS raise privacy concerns that must be considered by automakers and communicated to users.
Even a simple ADAS can be convoluted to understand for non-technical users or require dedicated learning on the part of the operator (Orlovska et al., 2020). If this learning does not occur in a controlled training environment, it will occur during system operation. DMS do not have a driver's mental model of the ADAS system, and thus they believe that the user understands the ADAS capabilities. This leads to the assumption that a user is a safe operator as long as users are attentive to the system. However, a user may be monitoring the ADAS while also expecting it to perform maneuvers of which it is not capable. This can lead to dangerous situations that leave the user little time to respond to vehicle deficiencies. More importantly, ADAS vary greatly in their capabilities and reliability (Monticello, 2023). Nevertheless, to the average user all systems may appear similar or the user may simply not be aware of the vehicle's capabilities (Harms et al., 2020). This can lead to situations of over-trust if the user is accustomed to a more advanced system and switches to a vehicle with a less advanced system.
In the case of DMS, drivers have incentives to mislead the system into believing they are attentive. The drivers have bought the system to make the driving task easier. However, monitoring the system can still be a fatiguing process, leading the user to offload as much work to the system as possible. It has been found through a long-term study of driver gaze behavior that Tesla drivers exhibit significantly less attentiveness to the road when using Autopilot systems, even compared to vehicles with ACC and ALKS where this phenomenon also exists (Morando et al., 2021). This is likely due to the increased perceptions around the capabilities of Tesla Autopilot. Tesla uses steering wheel motion-based recognition to detect if the driver is monitoring the vehicle. However, in the context of detecting distracted driving behavior in young drivers in Germany, authors found that motion-based recognition is not enough to detect and classify distracting behaviors (Jannusch et al., 2021). Instead, camera and acoustic based systems are necessary. Ultimately, it seems that eye gaze and vision based systems (as proposed by the UN regulation on L3 systems) are sufficiently more difficult to trick and may be a practicable compromise between the easily mislead torque-based systems and potentially intrusive multimodal systems that would analyse all driver behaviors and emotions (Gross, 2022).
Lack of oversight at the user level can also provide incentive to mislead DMS. For personally-owned vehicles, there is no managerial oversight of operator behavior like there would be in an industrial context (such as bus or tram driving) and in many cases there are no passengers in the vehicle that the driver might otherwise feel an increased obligation to be especially safe around (Rosenbloom and Perlman, 2016). For this reason, the driver may feel the desire to trick the DMS even if it presents a safety risk. This is not to say there should be centralized oversight from insurance agencies, government, or automotive companies on personally-owned ADAS systems, but rather that DMS designers should recognize this lack of oversight and make the systems more difficult to mislead.
An open question with DMS is what data is needed to perform adequate monitoring and accurately quantify driver attention. For steering wheel detection methods, having one hand on the wheel is hardly an indication that the driver is not on their smartphone or other device (NTSB, 2017). Steering wheel methods alone simply do not provide enough information to properly understand driver distraction (Jannusch et al., 2021). The American Automobile Association (AAA) found that camera-based DMS were able to detect driver distraction 50 s sooner than steering wheel DMS (Gross, 2022). Likewise, steering wheel DMS users were able to perform 5.65 min of continuous distracted driving before the system alerted the driver to pay attention, compared to 2.25 min for camera-based DMS users.
A further knowledge limitation of DMS is that they do not understand the intricacies of driver engagement with other activities and thus they grant a fixed amount of time when requesting a driver control takeover in situations where the ADAS can no longer operate. For L3 systems, the UN has proposed that the driver has 10 s to take over before the vehicle performs an emergency maneuver (United Nations, 2021). However, the amount of time that a driver will actually need during a transition period to gain situational awareness can vary greatly (Li et al., 2018; Morales-Alvarez et al., 2020; Huang and Pitts, 2022). More time for the driver to prepare improves the situational awareness and safety of the driver (Tan and Yiqi, 2022). The required time largely depends on how attentive the driver is in the moments leading up to the transition period, the complexity of the environment, and the driver's stress during the transition (Agrawal and Peeta, 2021). The UK Law Commission found that many experts suggest that 10 s is too short of a time for a transition (UK Department for Transport, 2021). Transitions from the automation can be either planned (e.g., a highway exit) or unplanned (e.g., an emergency maneuver) (United Nations, 2021). In the situation of the unplanned transitions, it is important the driver quickly gains situational awareness since the vehicle may not be able to give the driver much advance notice before they need to take over. For L2 systems, there is no regulated transition time since the driver is supposed to remain situationally aware at all times. This can lead to quick disengagements by the system that leave even an attentive driver needing to react quickly to control the vehicle. For this reason, it is critical that DMS on L2 systems are sophisticated enough to ensure drivers are continually paying attention.
Going beyond information limitations, DMS also suffer accuracy limitations even when presented with extensive data on driver emotion, gaze, and interaction with other objects. Although they work relatively well (even working through sunglasses in the case of gaze detection), their accuracy is not 100%. For fatigue detection, advanced research-grade systems using image-based methods only achieve around 70–98% accuracy and the vehicle motion-based methods achieve around 72–98% accuracy (Albadawi et al., 2022). The authors also found accuracy of these systems can vary depending on facial characteristics, skin colors, and illumination changes. For this reason, it may be necessary to combine both camera- and motion-based DMS to understand if the driver behaviors identified with camera measures are truly affecting the driving and attention level (Jannusch et al., 2021).
Finally, let us consider the potential accuracy of a DMS. If we assign a system distraction alert to be a positive value, then our best and worst cases can be represented by false positives and false negatives, respectively. In the best case, the system thinks the driver is not paying attention when they are (false positive) and this results in alerts that annoy the driver and cause them to take unnecessary action to assure the car of their attention. This can lead to frustration, lack of automated function use, and potentially general distrust of autonomy that can arise from poor failure rates of autonomous systems (Shahrdar et al., 2019). However in the worst case, the system thinks the driver is attentively supervising the system when they are not (false negative). A false negative situation could lead to crashes if the system reaches a road scenario it is not prepared to handle, and the driver is not paying attention to circumvent the hazard in time.
Finally, any discussion of camera-based systems should consider privacy concerns. We must take steps to ensure that DMS, and particularly more advanced ones that have yet to reach the consumer market, are designed to prioritize user privacy. The first step involves considering what data DMS store and who has access to that data. There has been legislation stating that processing of footage should remain on the vehicle.1 This regulation is a good step, but is aimed at DMS involving eye gaze monitoring. However, future systems may record driver emotion as well as driver interactions with vehicle interfaces or other objects. Concerning driver emotion, there emerge issues with accuracy of the system and how the data is used. Imagine emotion detection is used to understand user interface frustrations and better improve the user experience (provided that the user can opt out of sharing these metrics). If the system inaccurately measures emotion in these cases, there is no penalty to the user. However, imagine DMS are used to determine insurance prices. There is already some user acceptance of sharing vehicle emotion recognition data with insurers (Mangano et al., 2023). Emotion detection accuracy becomes much more important because it would affect the user's financial stability and ability to get good insurance rates. Can these systems be verified to be equally good at emotion detection for those of all backgrounds, vocal tones, and skin tones (Albadawi et al., 2022)? Can emotion be reliably determined in general from facial expression alone? We caution automakers and users to consider the above accuracy concerns before accepting these technologies.
Ultimately, we argue that the presence of DMS is not inherently sufficient for ensuring safety of vehicles with ADAS. We highlight that average users might have incentives to circumvent or control ADMS through input manipulation. Any system will have its limitations, and this is not to say that DMS are fatally flawed. As previously mentioned works demonstrate, ADAS and DMS have certainly helped reduce crashes and have made drivers think twice about their attention. Going forward, careful regulation of DMS is critical. UN regulation on L3 systems provides an excellent step of promoting DMS designed to measure at least two metrics, meaning they are less likely to be misled (United Nations, 2021). However, the lack of application to L2 systems raises a concern that automakers may be encouraged to remain at L2 certification. A company could lack L3 certification by not installing emergency maneuver capabilities on the vehicle but still making the vehicle's autonomy increasingly capable in diverse situations. These advanced capabilities combined with intelligent branding could encourage users to treat the L2 system as if it were an L3 or L4 system. In this way, an automaker could avoid having sophisticated DMS while also providing advanced driving features that their customers want. L2 systems will likely be around for a long while, and regulators and automakers together must appreciate that a safer future requires thinking critically about how attention and conformance is measured.
To structure our reflections, we draw on the Guiding Principles on automated decision systems drafted by the European Law Institute (ELI, 2022). The ELI principles are an attempt to offer ADM operators a coherent governing framework, drawing on existing EU legal provisions, which—according to the authors—are often “scattered in different pieces of legislation,” “partial in their scope,” “unharmonised,” “sector specific” and “their implementation is still uncertain in practice, may be unfeasible or too costly, or may become significantly complex” (ELI, 2022).
In the Table 1, we list and briefly describe the ELI principles and reflect on their application to the three case studies.
Table 1. Column 1 and 2 of the table provides the number and the title of the guiding principle, respectively; column 3 contains a short description of each principle, directly taken from ELI (2022) and finally, in column 4, we offer our reflections on each principle with respect to the three case studies.
In the following section, we offer a few recommendations for practice in the design, development and deployment of ADMS.
Reducing the focus of human involvement to the interaction between humans and the ADMS alone can make us lose sight of other and perhaps more important aspects influencing the use of autonomous decision making systems. These devices cannot be considered independently from their ecosystem of relationships and preconceptions. As Mindell argues: “we must deeply grasp how human intentions, plans, and assumptions are always built into machines. Every operator, when controlling his or her machine, interacts with designers and programmers who are still present inside it - perhaps through design and coding done many years before” (Mindell, 2015—emphasis ours). It is critical, therefore, that when assessing the design and performance of an automated system, these inbuilt assumptions, networks of relationships, and overall contexts are taken into account. Avoiding an overly narrow focus on the locality of the decision that the system is purportedly making, could help foster a wider awareness of the range of inputs—and therefore of possible outputs—of the system.
In the first case, the crisis of the adult social care sector in the UK (and the related need to reduce costs) has led to an ADMS being designed to prevent the risk fall in old adults. The deployment of the ADMS system coincides with the creation of the preventative care program. In this case the system was not meant to replace a critical function previously performed by humans or improve the accuracy or the efficiency of an existing service (e.g., reducing the number of false negatives during the identification process of beneficiaries). A comparison between a human-based and a machine based preventative program is not possible, because the preventative care program did not exist before the project. However, one could wonder whether the preventative care program could have existed without an ADMS. Would a human based system have been able to perform a preventative care program? In which ways does the AI system outperform humans in this task? We believe that these are important questions to address when introducing AI in sensitive domains such as social care.
In the second case, Boeing's market competition with Airbus led to an attempt to solve a hardware problem with a software solution. In this case automation originates out of a problematic situation and becomes bolted on to the situation as a fix rather than in the form of added value (e.g., a system providing a new feature or capability). In this case, economic drivers have led to an automated decision making system being applied to a situation for which it is a poor “fit”, demonstrating that when automation is used as a “patch” to remedy a complex situation, there may be unforeseen consequences.
In the third case, DMS are an attempt to solve an underlying deficiency in driving algorithms (the inability to safely perform L3 or L4 driving) by promising that the human will closely supervise the system. Rather than providing value to the driver, they are used as an easier alternative to safe algorithms and some DMS are made as simple as possible to avoid the cost of creating more effective ones. However, there will always be changing edge cases in autonomous driving systems and human-vehicle teaming needs to be better at adapting to these changes (Lee et al., 2023). Adding an attention monitor is hardly sufficient for ensuring that the driver accurately understands the system and where it is most likely to need help. Full autonomy is a myth (Mindell, 2015) and the question is therefore not whether autonomy is possible but why we want full autonomy. What is the purpose? What are the benefits and the risks? While we acknowledge the benefits brought about by ADMS, attention should be paid when ADMS are used as fixes.
Depending on the application domain, the use of ADMS can result in a range of harms. For critical applications those harms might be very serious indeed. To mitigate these harms (i) all critical application systems should be subject to risk assessment before use, in order to identify application domain risks and mitigation policies, (ii) risk assessment should be transparent and auditable, and (iii) risk assessment should determine the level of human oversight that is applied to every system.
Risk Assessment is a well-known method for discovering and mitigating risks, and hence improving safety. Ethical Risk Assessment (ERA) is not new either; it is essentially what research ethics committees do. But the idea of extending the envelope of safety risk assessment of intelligent systems to encompass ethical risks is new. Given the growing awareness of the ethical risks of intelligent systems in recent years, ERA offers a powerful method for systematically identifying and mitigating the ethical, societal and environmental risks associated with the use of robots and AI.
British Standard BS861 Guide to the ethical design and application of robots and robotic systems, sets out a method for ethical risk assessment (BSI, BS8611:2023, 2023). BS8611 defines an ethical harm as “anything likely to compromise psychological and/or societal and environmental well-being”. An ethical hazard as “a potential source of ethical harm” and an ethical risk as the “probability of ethical harm occurring from the frequency and severity of exposure to a hazard”. ERA thus extends the envelope of risk assessment to include ethical harms, hazards and risks, in addition to physical risks. For examples of ethical risk assessment following BS8611 of robot toys see (Winfield et al., 2022).
The first two case studies analyzed in this paper demonstrate a failure somehow related to design, risk assessment and certification procedures. In the preventive care program case, as far as we know, the local authority used the UK Government Algorithmic Transparency Reporting Standard (Algorithmic Transparency Recording Standard, 2021) to conduct a risk assessment of the ADMS before it was deployed, but after it was developed. We do not have insight into how the technology provider conducts their own risk analyses, but they supported the local authority in drafting the transparency report. The local authority also conducted data protection impact assessments to evaluate privacy risks, which are mandated by GDPR.
However, neither the risk assessment carried out by the local authority, nor the risk analyses conducted by the technology provider highlighted the false negative problem. AI systems are never 100% accurate because of errors and because the existence of false positives and false negatives is not a novelty, especially in the medical field. Testing the software not just for its functionality but at the level of integration and system level can be useful for identifying emerging errors and problems during the development phase. Moreover, independent expert evaluations, such as certification procedures are also important, although they are not currently required in preventative care, but only in some safety-critical sectors such as nuclear, aerospace and medical. In fact, the GDPR is the only piece of UK legislation that addresses ADMS specifically and cross-sectorally. However, there are a variety of other laws that may apply in practice, depending on both the party employing the ADMS, and in which sector they propose to do so. For example, ADMS employed in the public sector would be subject to the GDPR in terms of data protection, as well as equality law stemming from the Public Sector Equality Duty, and the common law of judicial review (Edwards et al., 2021). Specific sectors including medicine also have independent agencies, guidelines and regulation, such as the Medicines and Healthcare products Regulatory Agency, which is under the Department of Health and Social Care. While such sectoral regulators may increase the specificity of their address of ADMS, all original laws and regulations laid down without such specificity apply to ADMS as well. These regulations require specific contextual and legal interpretation beyond the scope of this paper, such as, for example, how the definition of “defect” in the Product Liability Directive applies to ADMS. The UK's March 2023 White Paper on AI, entitled “A Pro-Innovation approach to AI regulation,” confirms that the UK does not, at this time, plan to create legislation specific to AI, nor to create an AI-specific regulator. While AI and ADMS are not inherently identical, this does signal that there does not appear to be incoming legislation that might cover an overlap between the two.
The situation is different in the Boeing 737 MAX scenario. Notwithstanding stringent risk assessment procedures and mandatory certification, safety assurance was nonetheless very poor. This was mainly due to the way the people responsible for it performed their duties. We have already mentioned how production pressures, financial drivers, outsourcing the certification works, and conflict of interests had a role in the disaster.
However, the Boeing case examined in the article shows that the problem is not the technology itself, but once again the human in and on the loop. Whether or not specific regulations, standards, safety risk assessment, certification bodies, oversight measures, or codes of ethics exist, the misconduct of humans is a different area than technological issues. What are the remedies? The solution cannot be technology or process-oriented, but must be human-oriented. A possible way out is to encourage and protect whistle-blowers. As pointed out by Sullenberger (2019): “whistle-blower protection must be strong and effective, and if it is not strong enough, we must strengthen it”. Other scholars have suggested considering the Boeing 737 MAX case from the perspective of engineering ethics: “to strengthen the voice of engineers within large organizations” and to ensure “broader focus on moral courage in engineering ethics education” (Herkert et al., 2020).
The DMS case study shows that during deployment drivers have incentives to mislead the system with dangerous consequences for safety. Were these flaws not identified during the risk assessment phase?
Overall risk assessments are fundamental tools for balancing risks and benefits and therefore to decide whether or not to use an ADMS. In particular, they can help answer the question: What if the ADMS system does not work? In fact, as pointed out by Mongan and Kohli (2020) “There is a natural tendency to assume that systems intended to improve safety will do just that, and that their worst-case failure will be the absence of the additional safety the system is supposed to provide”. In all the cases analyses, in particular the Boeing case, it can be seen that the failure to anticipate possible poor outcomes led to an increase in overall risk.
In the social care case, what could be considered a meaningful form of human control? As discussed in Section 2.1, a human team checking the ADMS outputs by reviewing the case notes of each individual was not considered as a viable option because “disproportionate” and not in line with the project goals (it would be non-sense to exploit the preventive capabilities of ADMS and then to ask a human to repeat the process manually). This control task could have been better performed by a software specifically designed for that purpose. In this case, the human is forcefully out of the loop due to its limited capabilities with respect to the AI based system. In certain cases, meaningful human control should be identified during the ADMS design process rather than once the service is developed.
Moreover, as the Boeing case demonstrates, human factors play a role in meaningful human control. The human factor should be studied in normal conditions as well as in emergency situations. Considering the human factor in an emergency situation, such as the event of an accident caused by the failure of an automated system, can be vital for specific application domains in which humans are supposed to take back control from AI systems. As pointed out by Sullenberger (2019): “[w]e must make sure that everyone who occupies a pilot seat is fully armed with the information, knowledge, training, skill, experience and judgement they need to be able to be the absolute master of the aircraft and all its component systems, and of the situation, simultaneously and continuously throughout a flight”. Moreover, concerning the DMS, can we consider that ensuring the driver has a hand on the wheel is meaningful monitoring and control over the vehicle? First, the vehicle should more clearly explain what attention is expected of the driver when the system is engaged. Next, for the user to have meaningful control over the vehicle even in an attentive state, system visualizations are important. There should be real-time explanations for how the DMS perceives the driver and real-time explanations for how the ADAS is perceiving the environment so the user can better understand vehicle perception and capabilities. In this way, there is open dialogue between the two parties controlling the vehicle leading to safe environment understanding and response.
The DMS case illustrates that human control cannot be assumed to be a constant, fixed factor, especially in non-technical and non-work settings. Humans will not provide the same effort and supervision as each other and their individual supervision will vary with time. Likewise, drivers will experience passive fatigue while monitoring the autonomous functions which may mean their attention is reduced at certain points. It is important that the DMS be designed to accommodate the lowest reasonable level of human control so that unsafe situations are not created. There should additionally be more standardized forms of driving monitoring so there is less variation across vehicles in how the driver is being monitored. This will lead to more consistent and manageable expectations for the driver.
The problem of false negatives and false positives is a problem of accuracy of inputs as well as a problem of ADMS. The old database adage of “garbage in, garbage out” applies exponentially to automated decision makers, where the accuracy of the output may be to a safety-critical system, or to a life-changing medical intervention. The quality of the data should therefore be of key importance when planning the deployment of an automated system—while humans are often able to extrapolate from context or supplement incomplete information from prior knowledge, this is not a facility yet available to the generality of automated systems. Consequently, designers need to allow for these flaws and plan for the highest possible data integrity and legibility.
IEEE Standard 7001-2021 on Transparency of Autonomous Systems defines transparency as “the transfer of information from an autonomous system or its designers to a stakeholder, which is honest, contains information relevant to the causes of some action, decision or behavior and is presented at a level of abstraction and in a form meaningful to the stakeholder.” (Winfield et al., 2021; IEEE, 2022). The same standard defines explainability as transparency that is accessible to non-experts. Transparency is defined with respect to five different categories of stakeholders: users, the general public and bystanders, safety certification agencies, incident/accident investigators and lawyers/expert witnesses. The standard sets out 5 normative levels of transparency for each stakeholder group.
Common to the first two cases reviewed in Section 2 is the lack of transparency and explainability. According to Mongan and Kohli “people working with AI need to be made aware of the system's existence and must be trained on its expected function and anticipated dysfunction” (Mongan and Kohli, 2020). They are referring to professionals. In the Boeing case, neither pilots nor flight companies knew about MCAS; the flight control system was not included in operations manuals and pilots had not been trained on possible failures of the system. Knowing about MCAS and how to handle possible failures could have saved many lives. However, it would have been irrelevant for passengers to be aware of MCAS as of the many other technological systems at work in an airplane. In the other case study, the practitioners in the adult care program were aware of the ADMS. However, because of the technology provider's IP protection it makes it difficult to understand clearly how the system works, although it has an explainability mechanism in place (e.g., locally important features). For instance, we are not 100% sure whether the system takes account of context. For example, if the word “fall” was used in a note that read “Not considered to be at risk for fall”—would the system still pick it up as a fall risk?
Moreover, in this case, the people directly involved, or maybe affected, by the decision making system were not the practitioners, but the care service beneficiaries, namely adults at risk of falling. They should have known that the decision concerning the possibility to be part of a care service or not was made by an AI-based system, as were all people evaluated, selected, treated etc. by an AI based system, whether for financial, medical, job or educational purposes.
Transparency is not only relevant for understanding how an intelligent system works, but also for challenging the outcomes of decisions made by such systems (OECD, 2019). DMS function in a simple enough fashion that telling the user to look at the road is mostly sufficient for explaining how the system perceives the user. However, for future systems that may include emotion detection and object recognition it will be increasingly important that the DMS communicates to the user how it perceives them. The system may have inaccuracies that will be frustrating if the user cannot understand why the vehicle is alerting them and how they can modify their behavior to comply. Perhaps the system has malfunctioned and is getting a bad reading from the camera, perhaps the user is wearing a hat that obstructs the camera's view, perhaps the user is holding a drink that the system perceives to be a smartphone. Being able to understand how the camera perceives the driver can help the driver have agency to modify their behavior to comply or to account for system inadequacies. In the example mentioned previously where emotion data is used to potentially raise insurance rates or canceling a policy, there needs to be a way to verify that the system has interpreted the situation accurately as well as a way for a user to file an appeal for an agent to evaluate the case, which would help insurance rate determination better align with the aforementioned Article 22. For safety certification agencies, a more explainable mode of the DMS could be helpful for understanding how well the system is perceiving the user in real time. Additionally, it should be transparent how the user's data is being handled and where it is going for privacy reasons and peace of mind. It should be transparent to users if camera and audio are being recorded. If the user knew that the vehicle was monitoring their emotion, they may be more conscious of their behaviors.
ADMS may present advantages in terms of costs, efficiency, and safety. However, they are not risk free, making it extremely important to be very clear about the goal of the automation of a decision making process. In this paper, we have discussed three case studies concerning different applications of ADMS in different domains: administration, engineering, and non-technical user. In all three case studies, the main problems concerned the connection between humans and the system, in particular common issues such as design flaws, failure of safety assessment and certification, and human factors. Moreover, we have shown that oversight of these systems is not easily defined or implemented. Finally, we believe that there should be more interactions among ADMS developers, operators, users and third parties affected by the decisions during the risk assessment phases. In particular, the results obtained from risk assessment procedures should be made publicly available to all relevant stakeholders. This brings back the issue of how to solve the clash between the need to protect intellectual property for developers and the need to fully understand how the system is working for operators and other parties.
The datasets presented in this article are not readily available because we did not receive consent from participants to make the field study research data publicly available, due to the confidential nature of the research site. Requests to access the datasets should be directed to dHlsZXIucmVpbm11bmRAamVzdXMub3guYWMudWs=.
The studies involving humans were approved by Computer Science Departmental Research Ethics Committee (reference number CS_C1A_22_027) University of Oxford. The patients/participants provided their written informed consent to participate in this study. The studies were conducted in accordance with the local legislation and institutional requirements. The participants provided their written informed consent to participate in this study.
PS contributed to conception of the manuscript, wrote Sections 2.2 through 2.2.2 and Sections 3.2.1, 3.2.3, and 3.2.5. TR wrote Sections 2.1 through 2.1.4. BH wrote Sections 2.3 through 2.3.3. AW wrote Section 3.2.2. CT wrote Section 3.2.4. KG wrote Section 1 and 1.2. AJ contributed to the introduction. MJ and LK contributed to the manuscript conception. All authors contributed to manuscript revision. They have read and approved the submitted version.
This research was funded in whole by RoboTIPS: Developing Responsible Robots for the Digital Economy, which is an EPSRC Established Career Fellowship awarded to MJ (Award reference EP/S005099/1). For the purpose of Open Access, the author has applied a CC BY public copyright licence to any Author Accepted Manuscript (AAM) version arising from this submission.
MJ received financial support from RoboTIPS. TR's doctoral studies are partly funded by the consultancy involved in Case 1, the company name has been retracted to preserve the anonymity of participants in accordance with the University of Oxford's Central University Research Ethics Committee (CUREC).
The remaining authors declare that the research was conducted in the absence of any commercial or financial relationships that could be construed as a potential conflict of interest.
All claims expressed in this article are solely those of the authors and do not necessarily represent those of their affiliated organizations, or those of the publisher, the editors and the reviewers. Any product that may be evaluated in this article, or claim that may be made by its manufacturer, is not guaranteed or endorsed by the publisher.
1. ^European Union Supplementing Regulation (EU) 2019/2144 of the European Parliament and of the Council by laying down detailed rules concerning the specific test procedures and technical requirements for the type-approval of motor vehicles with regard to their driver drowsiness and attention warning systems and amending Annex II to that Regulation [2021] OJ 1 2639/01.
Agrawal, S., and Peeta, S. (2021). Evaluating the impacts of situational awareness and mental stress on takeover performance under conditional automation. Transport. Res. 83, 210–225. doi: 10.1016/j.trf.2021.10.002
Albadawi, Y., Takruri, M., and Awad, M. (2022). Areview of recent developments in driver drowsiness detection systems. Sensors 25, 2069. doi: 10.3390/s22052069
Alderwick, H., Tallack, C., and Watt, T. (2019). What Should be Done to Fix the Crisis in Social Care? The Health Foundation. Available online at: https://www.health.org.uk/publications/long-reads/what-should-be-done-to-fix-the-crisis-in-social-care (accessed May 22, 2023).
Algorithmic Transparency Recording Standard (2021). Available online at: https://www.gov.uk/government/publications/algorithmic-transparency-template (accessed May 22, 2023).
Alliance for Automotive Innovation (2023). Level 2 Driver Monitoring Principles. Available online at: https://www.autosinnovate.org/drivermonitoring (accessed July 6, 2023).
Arakawa, T., Hibi, R., and Fujishiro, T. (2019). Psychophysical assessment of a driver's mental state in autonomous vehicles. Transport. Res. 124, 587–610. doi: 10.1016/j.tra.2018.05.003
Article 29 Data Protection Working Party (2017). Guidelines on Automated Individual Decision-Making and Profiling for the Purposes of Regulation 2016/679. Available online at: https://ec.europa.eu/newsroom/article29/itemdetail.cfm?item_id=612053 (accessed July 6, 2023).
Begum, S. (2013). “Intelligent driver monitoring systems based on physiological sensor signals: a review,” in 16th International IEEE Conference on Intelligent Transportation Systems (ITSC 2013) (The Hague: IEEE), 282–289. doi: 10.1109/ITSC.2013.6728246
Binns, R., and Veale, M. (2021). Is that your final decision? Multi-stage profiling, selective effects, and Article 22 of the GDPR. Int. Data Privacy Law 11, 319–332. doi: 10.1093/idpl/ipab020
BSI BS8611:2023. (2023). Robots and Robotic Devices, Guide to the Ethical Design and Application of Robots and Robotic Systems. British Standards Institute.
Capallera, M., Meteier, Q., de Salis, E., Angelini, L., Carrino, S., Khaled, O. A., et al. (2019). “Owner manuals review and taxonomy of ADAS limitations in partially automated vehicles,” in Proceedings of the 11th International Conference on Automotive User Interfaces and Interactive Vehicular Applications.
Carr, N. (2015). The Glass Cage: How Our Computers Are Changing Us. New York, NY: Norton, W. W. and Company, Inc.
Chang, A., Lee, D., and Mas, K. (2019). The Real Reason Boeing's New 737 Max Crashed Twice” —Vox [Webzine]. Voxmedia. Available online at: https://www.vox.com/videos/2019/4/15/18306644/boeing-737-max-crash-video (accessed May 31, 2023).
Congress.gov (2021). S.1406 - 117th Congress (2021-2022): Stay Aware For Everyone Act of 2021. Congress.gov, Library of Congress (2021). Available online at: https://www.congress.gov/bill/117th-congress/senate-bill/1406 (accessed July 6, 2023).
Dastin, J. (2018). Amazon Scraps Secret AI Recruiting Tool That Showed Bias against Women. Reuters. Available online at: www.reuters.com/article/us-amazon-com-jobs-automation-insight-idUSKCN1MK08G (accessed July 6, 2023).
DHSC (2022). Build Back Better: Our Plan for Health and Social Care. Available online at: https://www.gov.uk/government/publications/build-back-better-our-plan-for-health-and-social-care/build-back-better-our-plan-for-health-and-social-care (accessed July 6, 2023).
Dunn, N. J., Dingus, T. A., Soccolich, S., and Horrey, W. J. (2021). Investigating the impact of driving automation systems on distracted driving behaviors. Accid. Anal. Prev. 156, 106152. doi: 10.1016/j.aap.2021.106152
Edwards, L., Williams, R., and Binns, R. (2021). “Legal and regulatory frameworks governing the use of automated decision making and assisted decision making by public sector bodies,” in The Legal Education Foundation Workshop Briefing Paper. Available online at: https://research.thelegaleducationfoundation.org/wp-content/uploads/2021/07/FINAL-Legal-and-Regulatory-Frameworks-Governing-the-use-of-Automated-Decision-Making-and-Assisted-Decision-Making-by-Public-Sector-Bodies-1.pdf
ELI (2022). Guiding Principles for Automated Decision-Making in the EU. ELI Innovation Paper. European Law Institute. Available online at: https://www.europeanlawinstitute.eu/fileadmin/user_upload/p_eli/Publications/ELI_Innovation_Paper_on_Guiding_Principles_for_ADM_in_the_EU.pdf (accessed May 22, 2023).
Gates, D. (2019a). Investigators Find New Clues Pointing to Potential Cause of 737 MAX Crashes as FAA Details Boeing's Fix. The Seattle Times. Available online at: https://www.seattletimes.com/business/boeing-aerospace/investigators-find-new-clues-to-potential-cause-of-737-max-crashes-as-faa-details-boeings-fix/ (accessed May 22, 2023).
Gates, D. (2019b). Flawed Analysis, Failed Oversight: How Boeing and FAA Certified the Suspect 737 MAX Flight Control System. The Seattle Times. Available online at: https://www.seattletimes.com/business/boeing-aerospace/failed-certification-faa-missed-safety-issues-in-the-737-max-system-implicated-in-the-lion-air-crash/ (accessed May 22, 2023).
Gouribhatla, R., and Pulugurtha, S. S. (2022). Drivers' behavior when driving vehicles with or without advanced driver assistance systems: a driver simulator-based study. Transport. Res. Interdiscipl. Perspect. 13, 100545. doi: 10.1016/j.trip.2022.100545
Gov.uk (2022). The Highway Code. Available online at: http://www.gov.uk/guidance/the-highway-code/general-rules-techniques-and-advice-for-all-drivers-and-riders-103-to-158 (accessed July 6, 2023).
Gov.uk (2023). Medicines and Healthcare Products Regulatory Agency. Available online at: https://www.gov.uk/government/organisations/medicines-and-healthcare-products-regulatory-agency#:~:text=The%20Medicines%20and%20Healthcare%20products%20Regulatory%20Agency%20regulates%20medicines%2C%20medicalof%20Health%20and%20Social%20Care (accessed July 6, 2023).
Gross, A. (2022). Face It: Only One Type of Driver Monitoring System Works, but It's Not Foolproof. AAA Newsroom. Available online at: http://www.newsroom.aaa.com/2022/02/face-it-only-one-type-of-driver-monitoring-system-works-but-its-not-foolproof/ (accessed July 6, 2023).
Hamblin, K. (2020). Care System Sustainability: What Role for Technology? An Evidence Review. Sustainable Care Paper 3, CIRCLE. Sheffield: University of Sheffield.
Harms, I. M., Bingen, L., and Steffens, J. (2020). Addressing the awareness gap: a combined survey and vehicle registration analysis to assess car owners' usage of ADAS in fleets. Transport. Res. Part A 134, 65–77. doi: 10.1016/j.tra.2020.01.018
Herkert, J., Borenstein, J., and Miller, K. (2020). The Boeing 737 MAX: lessons for engineering ethics. Sci. Eng. Ethics 26, 2957–2974. doi: 10.1007/s11948-020-00252-y
Huang, G., and Pitts, B. J. (2022). Takeover requests for automated driving: the effects of signal direction, lead time, and modality on takeover performance. Accid. Anal. Prev. 165, 106534. doi: 10.1016/j.aap.2021.106534
Huang, P., Li, J., Lan, C., and Lu, J. (2021). “Research on driver's passive fatigue under the condition of autonomous driving based on eye movement and ECG,” in Proceedings Volume 12058, Fifth International Conference on Traffic Engineering and Transportation System (ICTETS 2021) (Chongqing).
ICO (2018). Automated Decision-Making and Profiling. Information Commissioner's Office. Available online at: https://ico.org.uk/for-organisations/uk-gdpr-guidance-and-resources/individual-rights/automated-decision-making-and-profiling/ (accessed July 6, 2023).
IEEE (2022). “Standard for Transparency of Autonomous Systems,” in IEEE Std 7001-2021 (IEEE), 1–54. doi: 10.1109/IEEESTD.2022.9726144
IIPC (2021). Research on Adoption of Technology in Social Care. Institute of Public Care. Available online at: https://ipc.brookes.ac.uk/publications/building-the-evidence-base-for-tech-innovation-in-adult-social-care (accessed May 22, 2023).
Isaksson-Hellman, I., and Lindman, M. (2016). “Using insurance claims data to evaluate the collision-avoidance and crash-mitigating effects of collision warning and brake support combined with adaptive cruise control,” in 2016 IEEE Intelligent Vehicles Symposium (IV) (IEEE Press), 1173–1178. doi: 10.1109/IVS.2016.7535538
Jannusch, T., Shannon, D., Voller, M., Murphy, F., and Mullins, M. (2021). Cars and distraction: how to address the limits of Driver Monitoring Systems and improve safety benefits using evidence from German young drivers. Technol. Soc. 66, 101628. doi: 10.1016/j.techsoc.2021.101628
Körber, M., Congel, A., Zimmermann, M., and Bengler, K. (2015). Vigilance decrement and passive fatigue caused by monotony in automated driving. Proc. Manuf. 3, 2403–2409. doi: 10.1016/j.promfg.2015.07.499
Langewiesche, W. (2021). What Really Brought Down the Boeing 737 Max? The New York Times Magazine. Available online at: https://www.nytimes.com/2019/09/18/magazine/boeing-737-max-crashes.html (accessed July 6, 2023).
Lee, J., Rheem, H., Lee, J. D., Szczerba, J. F., and Tsimhoni, O. (2023). Teaming with your car: Redefining the driver–automation relationship in highly automated vehicles. J. Cogn. Eng. Decis. Making 17, 49–74. doi: 10.1177/15553434221132636
Leggett, T. (2023). 737 Max Crashes: Boeing Says Not Guilty to Fraud Charge. BBC News. Available online at: https://www.bbc.com/news/business-64390546 (accessed May 22, 2023).
Li, S., Blythe, P., Guo, W., and Namdeo, A. (2018). Investigation of older driver's takeover performance in highly automated vehicles in adverse weather conditions. IET Intell. Transp. Syst. 12, 1157–1165. doi: 10.1049/iet-its.2018.0104
Lyu, N., Deng, C., Xie, L., Wu, C., and Duan, Z. (2019). A field operational test in China: exploring the effect of an advanced driver assistance system on driving performance and braking behavior. Transport. Res. 65, 730–747. doi: 10.1016/j.trf.2018.01.003
Mangano, G., Ferrari, A., Rafele, C., Vezzetti, E., and Marcolin, F. (2023). Willingness of sharing facial data for emotion recognition: a case study in the insurance market. AI Soc. 1–12. doi: 10.1007/s00146-023-01690-5
Michelmore, R., Kwiatkowska, M., and Gal, Y. (2018). Evaluating uncertainty quantification in end-to-end autonomous driving control. arXiv [Preprint]. arXiv: 1811.06817.
Monfort, S. S., Reagan, I. J., Cicchino, J. B., Hu, W., Gershon, P., Mehler, B., et al. (2022). Speeding behavior while using adaptive cruise control and lane centering in free flow traffic. Traffic Inj. Prev. 23, 85–90. doi: 10.1080/15389588.2021.2013476
Mongan, J., and Kohli, M. (2020). Artificial intelligence and human life: five lessons for radiology from the 737 MAX disasters. Radiol. Artif. Intell. 2, e190111. doi: 10.1148/ryai.2020190111
Monticello, M. (2023). Ford's BlueCruise Ousts GM's Super Cruise as CR's Top-Rated Active Driving Assistance System. Consumer Reports. Available online at: https://www.consumerreports.org/cars/car-safety/active-driving-assistance-systems-review-a2103632203/ (accessed July 6, 2023).
Morales-Alvarez, W., Sipele, O., Léberon, R., Tadjine, H. H., and Olaverri-Monreal, C. (2020). Automated driving: a literature review of the take over request in conditional automation. Electronics 9, 2087. doi: 10.3390/electronics9122087
Morando, A., Gershon, P., Mehler, B., and Reimer, B. (2021). A model for naturalistic glance behavior around Tesla Autopilot disengagements. Accid. Anal. Prev. 161, 106348. doi: 10.1016/j.aap.2021.106348
NAO (2021). The Adult Social Care Market in England. London. Available online at: https://www.nao.org.uk/wp-content/uploads/2021/03/The-adult-social-care-market-in-England.pdf
NHS England (n.d.). AI in Social Care. NHS Transformation Directorate. Available online at: https://transform.england.nhs.uk/ai-lab/explore-all-resources/understand-ai/ai-adult-social-care/ (accessed May 22, 2023).
NICE (2013). Falls in Older People: Assessing Risk and Prevention. Available online at: https://www.nice.org.uk/guidance/cg16122 (accessed May 22, 2023).
NTSB (2017). Collision Between a Car Operating With Automated Vehicle Control Systems and a Tractor- Semitrailer Truck Near Williston, Florida: May 7, 2016. Washington, DC: National Transportation Safety Board. Available online at: https://www.ntsb.gov/investigations/AccidentReports/Reports/HAR1702.pdf
NTSB (2020). Collision Between a Sport Utility Vehicle Operating With Partial Driving Automation and a Crash Attenuator Mountain View, California March 23, 2018. Washington, DC: National Transportation Safety Board. Available online at: https://www.ntsb.gov/news/events/Pages/2020-HWY18FH011-BMG.aspx
OECD (2019). Recommendation of the Council on Artificial Intelligence. Paris: Organisation for Economic Co-operation and Development, Tech. Rep.
Orlovska, J., Wickman, C., and Söderberg, R. (2020). Design of a data-driven communication framework as personalized support for users of ADAS. Proc. CIRP 91, 121–126. doi: 10.1016/j.procir.2020.02.156
Ozmen Garibay, O., Winslow, B., Andolina, S., Antona, M., Bodenschatz, A., Coursaris, C., et al. (2023). Six human-centered artificial intelligence grand challenges. Int. J. Hum. Comput. Interact. 39, 391–437. doi: 10.1080/10447318.2022.2153320
Parasuraman, R., Molly, R., and Singh, I. L. (1993). Performance consequences of automation induced complacency. Int. J. Aviat. Psychol. 3, 1–23. doi: 10.1207/s15327108ijap0301_1
Povyakalo, A. A., Alberdi, E., Strigini, L., and Ayton, P. (2013). How to discriminate between computer aided and computer hindered decisions: a case study in mammography. Med. Decis. Making 33, 98–107. doi: 10.1177/0272989X12465490
Reagan, I. J., Cicchino, J. B., Teoh, E. R., Reimer, B., Mehler, B., and Gershon, P. (2022). Behavior change over time when driving with adaptive cruise control. Proc. Hum. Factors Ergon. Soc. Annu. Meet. 66, 352–356. doi: 10.1177/1071181322661191
Rosenbloom, T., and Perlman, A. (2016). Tendency to commit traffic violations and presence of passengers in the car. Transport. Res. 39, 10–18. doi: 10.1016/j.trf.2016.02.008
SAE International (2021). SAE Levels of Driving AutomationTM. Available online at: http://www.sae.org/blog/sae-j3016-update (accessed July 6, 2023).
Shahrdar, S., Menezes, L., and Nojoumian, M. (2019). “A survey on trust in autonomous systems,” in Intelligent Computing. SAI 2018. Advances in Intelligent Systems and Computing, Vol. 857, eds K. Arai, S. Kapoor, and R. Bhatia (Cham: Springer). doi: 10.1007/978-3-030-01177-2_27
Skills for Care (2015). 6 Reasons Why Data and Information is Important When Running a Successful Social Care Business. https://www.skillsforcare.org.uk/Documents/NMDS-SC-and-intelligence/Informatics/6-reasons-why-data-is-important.pdf22 (accessed July 6, 2023).
Spicer, R., Vahabaghaie, A., Bahouth, G., Drees, L., Martinez von Bülow, R., and Baur, P. (2018). Field effectiveness evaluation of advanced driver assistance systems. Traffic Inj. Prev. 19 (Supp. 2), S91–S95. doi: 10.1080/15389588.2018.1527030
Stocco, A., Weiss, M., Calzana, M., and Tonella, P. (2020). “Misbehaviour prediction for autonomous driving systems,” in Proceedings of the ACM/IEEE 42nd International Conference on Software Engineering (ICSE '20) (New York, NY: Association for Computing Machinery), 359–371. doi: 10.1145/3377811.3380353
Sullenberger, C. B. (2019). ‘Sully'. My Testimony Today Before the House Subcommittee on Aviation. Sully's Blog. Available online at: https://www.sullysullenberger.com/my-testimony-today-before-the-house-subcommittee-on-aviation/ (accessed July 6, 2023).
Tan, X., and Yiqi, Z. (2022). The effects of takeover request lead time on drivers' situation awareness for manually exiting from freeways: A web-based study on level 3 automated vehicles. Accid. Anal. Prev. 168, 106593. doi: 10.1016/j.aap.2022.106593
The Boeing Company (n.d.). The 737 MAX MCAS Software Enhancement. Available online at: https://www.boeing.com/commercial/737max/737-max-software-updates.page#/overview (accessed May 29 2023).
The King's Fund (2023). A History of Social Care Funding Reform in England. The King's Fund. Available online at: https://www.kingsfund.org.uk/audio-video/short-history-social-care-funding (accessed May 22, 2023).
The United States Department of Justice (2021). Boeing Charged with 737 Max Fraud Conspiracy and Agrees to Pay over $2, 5. Billion [An official website of the United States government]. Office of Public Affairs News. Available online at: https://www.justice.gov/opa/pr/boeing-charged-737-max-fraud-conspiracy-and-agrees-pay-over-25-billion (accessed May 22, 2023).
UK Department for Transport (2021). Safe Use of Automated Lane Keeping System on GB Motorways: Call for Evidence. GOV.UK. Available online at: https://www.gov.uk/government/calls-for-evidence/safe-use-of-automated-lane-keeping-system-on-gb-motorways-call-for-evidence (accessed July 6, 2023).
United Nations (2021). Addendum 156 – UN and Regulation No, 157. Uniform Provisions Concerning the Approval of Vehicles with Regard to Automated Lane Keeping Systems (2021). Available online at: https://unece.org/sites/default/files/2021-03/R157e.pdf
Vogelpohl, T., Kühn, M., Hummel, T., and Vollrath, M. (2019). Asleep at the automated wheel—sleepiness and fatigue during highly automated driving. Accid. Anal. Prev. 126, 70–84. doi: 10.1016/j.aap.2018.03.013
Wagner, B. (2019). Liable, but not in control? Ensuring meaningful human agency in automated decision-making systems. Policy Int. 11, 104–122. doi: 10.1002/poi3.198
Werhane, P. H. (1991). Engineers and management: the challenge of the challenger incident. J. Bus. Ethics 10, 605–616. doi: 10.1007/BF00382880
Winfield, A. F. T., van Maris, A., Winkle, K., Jirotka, M., Salvini, P., Webb, H., et al. (2022). “Ethical risk assessment for social robots: case studies in smart robot toys,” in Towards Trustworthy Artificial Intelligent Systems. Intelligent Systems, Control and Automation: Science and Engineering, vol 102, eds M. I. A. Ferreira, and M. O. Tokhi (Cham: Springer).
Winfield, F. T., Serena, B., Louise, A. D., Takashi, E., Helen, H., Naomi, J., et al. (2021). IEEE P7001: a proposed standard on transparency. Front. Robot. 8, 665729. doi: 10.3389/frobt.2021.665729
Wright, J. (2020). Technology of Social Care: Review of the UK Policy Landscape. Sustainable Care Paper, Vol. 2. Sheffield: University of Sheffield.
Keywords: autonomous decision making systems, human oversight, Boeing 737 MAX MCAS, predictive algorithm for social care, driver monitoring systems
Citation: Salvini P, Reinmund T, Hardin B, Grieman K, Ten Holter C, Johnson A, Kunze L, Winfield A and Jirotka M (2023) Human involvement in autonomous decision-making systems. Lessons learned from three case studies in aviation, social care and road vehicles. Front. Polit. Sci. 5:1238461. doi: 10.3389/fpos.2023.1238461
Received: 11 June 2023; Accepted: 06 November 2023;
Published: 20 December 2023.
Edited by:
Ben Wagner, Delft University of Technology, NetherlandsReviewed by:
Francesco Mureddu, The Lisbon Council for Economic Competitiveness and Social Renewal, BelgiumCopyright © 2023 Salvini, Reinmund, Hardin, Grieman, Ten Holter, Johnson, Kunze, Winfield and Jirotka. This is an open-access article distributed under the terms of the Creative Commons Attribution License (CC BY). The use, distribution or reproduction in other forums is permitted, provided the original author(s) and the copyright owner(s) are credited and that the original publication in this journal is cited, in accordance with accepted academic practice. No use, distribution or reproduction is permitted which does not comply with these terms.
*Correspondence: Pericle Salvini, cGVyaWNsZS5zYWx2aW5pQGNzLm94LmFjLnVr
Disclaimer: All claims expressed in this article are solely those of the authors and do not necessarily represent those of their affiliated organizations, or those of the publisher, the editors and the reviewers. Any product that may be evaluated in this article or claim that may be made by its manufacturer is not guaranteed or endorsed by the publisher.
Research integrity at Frontiers
Learn more about the work of our research integrity team to safeguard the quality of each article we publish.