- 1Division of Humanities and Social Science, California Institute of Technology, Pasadena, CA, United States
- 2Luskin School of Public Affairs, University of California at Los Angeles, Los Angeles, CA, United States
Does collective identity drive protest participation? A long line of research argues that collective identity can explain why protesters do not free ride and how specific movement strategies are chosen. Quantitative studies, however, are inconsistent in defining and operationalizing collective identity, making it difficult to understand under what conditions and to what extent collective identity explains participation. In this paper, we clearly differentiate between interest and collective identity to isolate the individual level signals of collective action. We argue that these quantities have been conflated in previous research, causing over estimation of the role of collective identity in protest behavior. Using a novel dataset of Twitter users who participated in Black Lives Matter protests during the summer of 2020, we find that contingent on participating in a protest, individuals have higher levels of interest in BLM on the day of and the days following the protest. This effect diminishes over time. There is little observed effect of participation on subsequent collective identity. In addition, higher levels of interest in the protest increases an individuals chance of participating in a protest, while levels of collective identity do not have a significant effect. These findings suggest that collective identity plays a weaker role in driving collective action than previously suggested. We claim that this overestimation is a byproduct of the misidentification of interest as identity.
1. Introduction
In the summer of 2020, protests erupted in the United States in reaction to the murders of Breonna Taylor and George Floyd. Their deaths embodied the systematic racism Black Americans experience in the United States. These protests sparked continued interest in the Black Lives Matter movement's demands for racial justice. Black Lives Matter (BLM) was officially founded by Alicia Garza, Patrisse Cullors, and Opal Tometi as a Black-centered political movement in 2013 in response to the acquittal of George Zimmerman in the shooting of Trayvon Martin in 2012.1 While estimating the exact number of people involved in the 2020 Black Lives Matter protests is difficult, they were likely the largest in American history (Buchanan et al., 2020). According to a poll conducted by Gallup between June 23 and July 6, 2020, 11% of American adults said that they had “participated in a protest about racial justice and inequality” in the past 30 days (Long and McCarthy, 2020), indicating a greater level of expressed support than seen for previous BLM protests. The Gallup data indicate that the racial justice and equality protesters were significantly more diverse than previously, with 18% of Black adults, 20% of Asian adults, 13% of Hispanic adults, and 10% of White adults saying they participated (Olteanu et al., 2015; Fisher, 2020). Formal theory predicts that collective action on this scale should be extraordinarily difficult to organize as it involves a collective good—achieving racial justice in the United States (Olson, 1965). What factors explain the widespread participation in the 2020 Black Lives Matter protests?
Past research posits two explanations to explain why individuals participate in collective action like the 2020 BLM protests. One is that individuals participate because they agree with protesters' desired policy change; this paper calls such alignment “interest”. In the context of the 2020 BLM protests, these interests could be factors like eliminating racial injustice in the United States, stopping policy brutality, or raising awareness about racial discrimination. While early models of collective action suggest interest should not drive participation because it does not affect an individual's benefit from protesting (Olson, 1965), subsequent empirical work has found that interest alignment motivates protest participation (Olsen, 1970; Finkel et al., 1989; Ostrom, 2000).
Another important mechanism thought to enable participation in collective action at this scale is collective identity, the sense of belonging individuals have to a broader community or institution with a shared perception of group status and goals (Polletta and Jasper, 2001). This group status can originate externally, with outsiders grouping individuals together, such as organizers or entrepreneurs using identities such race, ethnicity, religion, gender, or partisanship as mobilization rubrics. Alternatively, this understanding can originate internally, with individuals seeing that there is a shared sense of purpose or shared ideology. Regardless, by definition, collective identity requires that individuals accept status as part of a group and feel a loyalty to enhancing the status of the group as a whole (Turner-Zwinkels and van Zomeren, 2021). By sustaining this sense of belonging and loyalty, working toward the group's goal becomes individually rational and free riding diminishes (Conover, 1988; Chong et al., 2004). Importantly, race in America provides a source of collective identity that has motivated previous episodes of collective action (McClain et al., 2009; Sanchez and Vargas, 2016).
This paper develops a formal model that generates three hypotheses of how these signals of collective action should interact with protest behavior. First, individuals with higher signal values are more likely to protest. Second, individuals should have higher signal values on the day they protest. Finally, going to a protest should increase the signals' value.
The paper also develops measures that distinguish between collective identity and interest expressed in short online texts. The most common method of operationalizing collective identity is via common hashtag or shared imagery (Freelon et al., 2016; Metzger et al., 2016; Driscoll and Steinert-Threlkeld, 2020). This operationalization, however, approximates a quantity closer to topic interest than to collective identity. In the online world, the focus of this paper, we define interest as discussion of relevant topics, while identity is the use of language signifying a sense of belonging (for instance, increased use of plural pronouns such as “we”, “us”, and “them”). Since choosing to identify with a group gives important insights into the individual's perception of themselves as well as the group's status (Shayo, 2009), this explicit version of collective identity should have a stronger alignment with protest participation than interest.
We test these hypotheses using a new panel dataset of 3,040 Twitter accounts of people likely to have joined BLM protests in Los Angeles, Houston, or Chicago. We then use natural language processing techniques, specifically a Reverse Joint Sentiment Topic model, to analyze each of the accounts' 3.8 million tweets from the summer of 2020, generating separate measures of interest and identity. An ordinary least squares model with day and individual fixed effects is then used to help test the hypotheses derived from the formal model. Results show that contingent on participating in a protest, individuals have higher interest levels the day of and the days following the protest, although this effect diminishes over time. There is a similar pattern for identity, but it is on a smaller scale and has lesser statistical significance. In addition, higher interest in BLM-related topics increases an individual's chance of participating in a protest, while collective identity does not have a significant effect. For individuals who protest at least once, interest levels have a higher correlation with protesting than identity.
This article joins a growing body of work using digital trace data to understand mobilization around the BLM movement. Social media data has been used to study public opinion about the Black Lives Matter movement (Dunivin et al., 2022), to trace the subtopics discussed (Ray et al., 2017; Crowder, 2020; Giorgi et al., 2022; Tong et al., 2022), as well as to measure the initiation and dispersion of support through social networks (Jackson and Foucault Welles, 2016; Crowder, 2021). These digital studies join a similarly growing body of scholarship that uses offline data, primarily surveys, to understand opinions toward and participation in the movement. Some scholars examine co-ethnic mobilization in support of Black Lives Matter using other pre-existing organizations (Arora and Stout, 2019). Others have similarly used survey data to look at how the protests might have affected public opinion toward police violence (Reny and Newman, 2021; Shuman et al., 2022). Other studies used administrative data to draw the connection between protests and police violence (Williamson et al., 2018) and ethnography to document how other social movements interact with BLM (Petitjean and Talpin, 2022). As far as we are aware, this paper is the first study to use social media data to study the interaction of protests, collective identity, and interest.
The paper proceeds as follows. Section 2 introduces a model of protests that generates expectations about collective identity and interest. Section 3, describes the research design . Section 4 presents results and Section 5 concludes with a discussion of implications.
2. Collective identity, interest, and protest participation
2.1. The importance of collective identity and interest
Researchers have long struggled to reconcile the reality that large-scale collective action occurs against the theoretical expectation that they should rarely arise since any individuals' contribution to the public good is vanishingly small (Tilly, 1977; Ostrom, 1990; Chong, 1991). This disconnect between theory and reality has led to considerable theorizing about incentives for individual involvement in collective action (Tullock, 1971; Gerber et al., 2008). Instead, motivation can arise from notions of morality, the emotions evoked by collective participation, fear of judgement from the community or having a collective identity (Miller et al., 1981; Johnston and Klandermans, 1995; Jasper, 1997; Stokes, 2003; Sanchez, 2006; Gause, 2022).
Two sources of motivation are particularly prominent: collective identity and interest. Collective identity refers to the extent an individual feels like they belong to a group. It is one of the first concepts used to explain otherwise irrational behavior (Fireman and Gamson, 1977; Teske, 1997). A sense of collective identity provides a private benefit to individuals for participating when they see themselves as part of the group of individuals who would benefit from the policy change a protest seeks. This benefit arises when an individual internalizes the status of a group to which they feel linked (Dawson Michael, 1994; Tate, 1994; McClain et al., 2009).
Interest refers to attention to a protest and agreement with the protest's policy goals. Awareness is a necessary precondition to protesting: an individual must know that others desire policy change and are actively working to realize that change (Kurzman, 1996; Wouters, 2019). Awareness is particularly important in the case of spontaneous protests, protests which arise with minimal to no planning from activist organizations (Pearlman, 2021). Just as spatial models of voting predict voters will support a candidate closer to them in ideological space, an individual is more likely to protest when the policy change protesters seek is closer to their desired policy than the status quo (Lohmann, 1994).
In the United States, racial groups are a common source of collective identity, and decades of research analyzes how they affect political participation. Perhaps the earliest quantitative study is Matthews and Prothro (1966). In particular, two survey questions ascertain the closeness Black participants felt to their community and find that increased closeness correlates with increased voting. Subsequent work finds that higher levels of group consciousness in Black Americans correlates with higher levels of participation in collective action (Olsen, 1970; Verba and Nie, 1987). Since political change in favor of minority groups requires interest from members of the majority, much research also seeks to understand how interest conditions involvement in collective action. Surveys of college participants during the Freedom Summer of 1964, for example, find ideological alignment and social embeddedness drive participation (McAdam, 1986). More recently, lab experiments show how the identity of protesters affects support for a protest, with particular focus on America's Black Lives Matter protests (Bonilla and Tillery, 2020; Mitts et al., 2022). Just as during the civil rights movement of the 1950s and 1960s, the rise of the Black Lives Matter movement has led to a surge in interest around police brutality and racial inequality (Freelon et al., 2016; Tillery, 2019).
Protesting due to collective identity means one has internalized the costs and benefits of the group with which one identifies. Interest means that one is motivated to participate even if one's identity is not concordant with a group that is protesting. For example, an individual who has experienced racist treatment may have participated in the 2020 BLM protests from a sense of identification with the larger collectivity that has similarly suffered. Interest drives the individual who is motivated to rectify those injustices regardless of whether they identify as part of the suffering group.
Given these previous findings, collective identity and interest should positively correlate with protest participation. Moreover, since the extent to which they do is likely to vary by factors such as communication technology available, the prevalence of movement organizations to organize protest, the type and intensity of repression a government uses, or the dynamics of protests in nearby places, neither source of motivation should strictly dominate the other. Because of the similar effects of collective identity and interest, the rest of this section refers to the two as signals.
2.2. The model
The following model assumes there are individuals i∈{1, ..., I} and days . In addition, for each individual-day pair we have a collective action signal value for which higher values imply a stronger signal value. This signal could be interest or collective identity. Finally, we also have an indicator on whether or not individual i protests on day t represented by xi, t.
For the original turnout game, we assume that individuals contribute to a public good, such as protesting, when their net utility is non-negative. If a threshold (q) is met then everyone receives the public good (a policy change resulting from a large enough protest), if not, no one does. For the most basic model, we assume that everyone has the same cost (c) of protesting and benefit (β) from the subsequent policy change if enough individuals protest (𝟙). The utility for protesting is thus:
In this case, since everyone is identical, we look for symmetric equilibria. The symmetric equilibria are mixed strategy responses, that is everyone has a probability p of protesting. For a mixed strategy, we need the payoff for protesting to be the same as not protesting. Thus, we have that the cost to protesting must equal the benefit times the probability that the individual is pivotal. Generally, the probability of being pivotal is so small that the benefit must be massive or the cost minuscule.
In our version of the game, individuals have a private individual benefit () from the act of protesting at time t. This private individual level benefit is correlated with their personal signal (either from collective identity or interest). Addition of private signals in this way is taken from the global games literature which studies games in which actions are influenced by the uncertain actions of others (Bueno De Mesquita, 2010; Shadmehr and Bernhardt, 2011; Little, 2016). In that case, each individual's utility function can be rewritten as
For the sake of simplicity, we assume that is normally distributed, however for any known distribution the proof continues in the same manner. Given a cutoff strategy, such that individuals protest if their individual cost is less than some value k*, then we can solve for this cutoff by solving the equation:
In reality, however, the observed measures are noisy signals for identity and interest, so the value is instead
where yt is a daily fixed effect and ϵit is the normally distributed, daily noise given the individual. With this information, we have the probability that the true value is greater than the cutoff increases with the measured value. This probability leads to the first hypothesis: Hypothesis 1 (H1). Individuals who have higher signal values are more likely to participate in protest.
Two more hypotheses explain how these signals should operate on the day of a protest and subsequent days. These hypotheses follow from homophily in social networks (Hegselmann and Krause, 2002; Siegel, 2009). Given some network which represents the contacts of individual i, we have that
Since protesting reinforces identity and interest through interactions with other like-minded individuals (Madestam et al., 2013), it should increase signal production. On the day of a protest, protesting individuals should exhibit higher than usual signal values. Formally: Hypothesis 2 (H2). The act of protesting increases the expected levels of collective action signals observed during that day compared to the non-protesting expectation.
After a protest, signal production should remain elevated. This expectation arises because new connections created by protesting will have higher levels of signals. As a result, given new connections who, on average have higher signal values, the average signal value of an individual's connections will increase. Thus overall signal production about the protest will increase.
Hypothesis 3 (H3). The act of protesting increases the expected levels of the signals of collective action observed for the days following the protest action compared to the non-protesting expectation.
3. Research design
The expectations about collective identity and interest are tested using the 2020 Black Lives Matter Protests in the United States of America. These events are chosen because of the simultaneous importance of collective identity (race) and interest to the protests. The protests are also the largest to have ever occurred in the United States, with over 7,750 in 2,440 locations in every state (Raleigh et al., 2010; Putnam et al., 2020).
Geolocated social media data provide the foundation for analyzing collective identity and interest. First, we select three cities for analysis and find Twitter users we classify as protesters. These accounts are classified as protesters if they were likely at protests in their city based on keywords and location provided from Twitter. We say an individual participated in a particular protest if they are found using this process. We then collected the entire Twitter timeline for each of these protesters for the summer of 2020. In order to measure both signals , we estimated a Reverse Joint Sentiment Topic (RJST) model, a weakly supervised natural language processing model. Finally, we use the results from the RJST model to test the hypotheses. The next subsections explain each step in detail .
3.1. Data collection
We choose to analyze the BLM movements in Los Angeles, Chicago, and Houston. Cities were not chosen for geographic or political reasons, as we do not expect the role of identity to vary based on the location or median preferences of a city. Instead, we chose to focus on three of America's four largest cities because they account for a significant number of protests and participants during the period of this study.2,3
Having determined locations to analyze, the next decision involved data collection. Social media was chosen over participant observation or surveys because they give researchers the ability to observe individuals before, during, and after treatment across disparate locations at much lower cost than in-person studies and do not require researcher foreknowledge of an event. Surveys face difficulties that arise from the spontaneity of these events; they are often not known far enough in advance for a research group to pull together a proposal and get the funding and individuals in place to create an effective survey. In addition, people at a protest are often uninterested in responding to a long list of questions when they are focused on their bigger goal. Finally, it is difficult to sample research subjects for surveys conducted at a protest location in a way that produces a scientifically representative sample.4
These issues in the collection of data can easily lead to biased responses (Westwood et al., 2022). Additionally, survey methods are unable to dynamically track these values over time (Chenoweth et al., 2022). Even in the case of panel data, researchers have at most two or three points for each individual over time. Most importantly, perhaps, is that they rarely have information on the individuals before the first protest and are thus unable to compare how the protest affected them and whether those effects endure. These shortcomings make real time and in-person data collection almost impossible, especially for large scale protests.
By using social media data, we are able to retroactively access the conversations of protesters before they protest, providing a baseline for their activities prior and subsequent to their action. In addition, the nature of the 2020 BLM protests means that we were able to obtain data from a series of protests from the same locations and with the same basic subject matter but over a varying period of time. A major benefit of collecting time series cross section (TSCS) data is the ability to factor out day-specific effects. Finally, there has been significant research connecting the use of social media with protest behavior (Valenzuela, 2013) making it an appropriate venue for this work. Overall, since the generation of social media data occurs outside of the purview of researchers, these sources of bias are reduced.
From the universe of social media platforms, Twitter is best suited for this research. It is widely and frequently used (Duggan and Smith, 2013). In addition, it is used both to coordinate political activities and to discuss everyday events, providing a holistic picture of individuals (Boyd et al., 2010). Twitter has also emerged as a primary tool used by social movement organizers to engage individuals in collective action (Clark-Parsons, 2022). Importantly for this study, while only 13.5 percent of the United States population is Black, they make up 25 percent of users on Twitter (Brock, 2012), which allows us to more heavily weigh the population for whom this movement is most likely to be salient. In addition, there has already been substantial research using Twitter use to study the BLM movement (Cox, 2017; Ince et al., 2017; Freelon et al., 2018) which provide references to compare our results with. Researchers have also used Twitter to study protests across the globe, in autocracies and democracies (Burns and Eltham, 2009; Rahimi, 2011; Steinert-Threlkeld, 2017; Larson et al., 2019), for the study of the Black Lives Matter movement in the United States (Ray et al., 2017; Hsiao, 2021), and for the study of feminist social movements like MeToo (Clark-Parsons, 2022). Finally, Twitter was easily accessible via two APIs.5
There are, however, concerns about measuring collective identity using social media data. The nature of the data means that we do not have access to relevant sociodemographic information which would ideally be used in determining collective identity strength. In addition, an account must have geotagged at least one tweet from one of the study's three cities to be included, so findings are most applicable to other Twitter users who geotag their tweets. Some existing research finds that users with geotagged tweets are statistically different than those who do not (Karami et al., 2021), but work which analyzes protest finds no difference between those who geotag and those who do not (Steinert-Threlkeld et al., 2022). Finally, it is worth noting that in this case we select on the dependent variable: only individuals who protest at least once are in this dataset. Future work should include a baseline of non-protesters as well, though for this paper this selection is not problematic since we are specifically concerned about the signals for people who protest.
This paper operationalizes a protester as anyone who uses keywords related to the Black Lives Matter movement from Los Angeles, Houston, or Chicago during a subsample of those cities' summer 2020 protests. Selecting on keywords generates accurate estimates of the number of people who protest (Sobolev et al., 2020). Table 1 provides a sample of tweets associated with protesters. 6
These tweets and the associated users were found using the Version 2 Twitter API and the Python package TwitterAPI.7,8 These tools allow us to enter a time period, location bounding box around the protest city, and keywords to search for and return the desired information for all tweets that meet the criteria. For this project, we requested the author ID, time the tweet was written, geolocation information (which can be in the form of coordinates, a bounding box, or a city name), public metrics (likes, retweets, etc.), entities (hashtags, mentions, symbols, and URLs), and the tweet text. We choose protests listed in the Crowd Counting Consortium (Chenoweth and Pressman, 2017). From Los Angeles, we choose 14 protests from which we draw 2,348 protesters, from Houston we have 273 protesters from 8 protests , and from Chicago we have 391 protesters from 24 protests (see Supplementary Tables 1.2–1.4).
Next, we downloaded all available tweets from each protester from May 20th 2020 until October 1st 2020 using the package gatherTweet (Kann et al., 2023). 9 We again used the Version 2 Twitter API and TwitterAPI to pull the entire timeline for all of these accounts. These tweets provide the conversations of all the selected individuals from five days before the murder of George Floyd through the end of the summer. Figure 1 shows the number of tweets we collected on each day from each city. While there are significantly more tweets from Los Angeles than the other two cities—a result of larger protests in Los Angeles than the other two cities—when we look at the distribution of tweets they follow similar patterns. These approximate similarities between the cities provides preliminary support for the assumption that we can pool the protests from the three cities in our analysis. Supplementary Tables 1.2–1.4 show summary statistics for the protests.
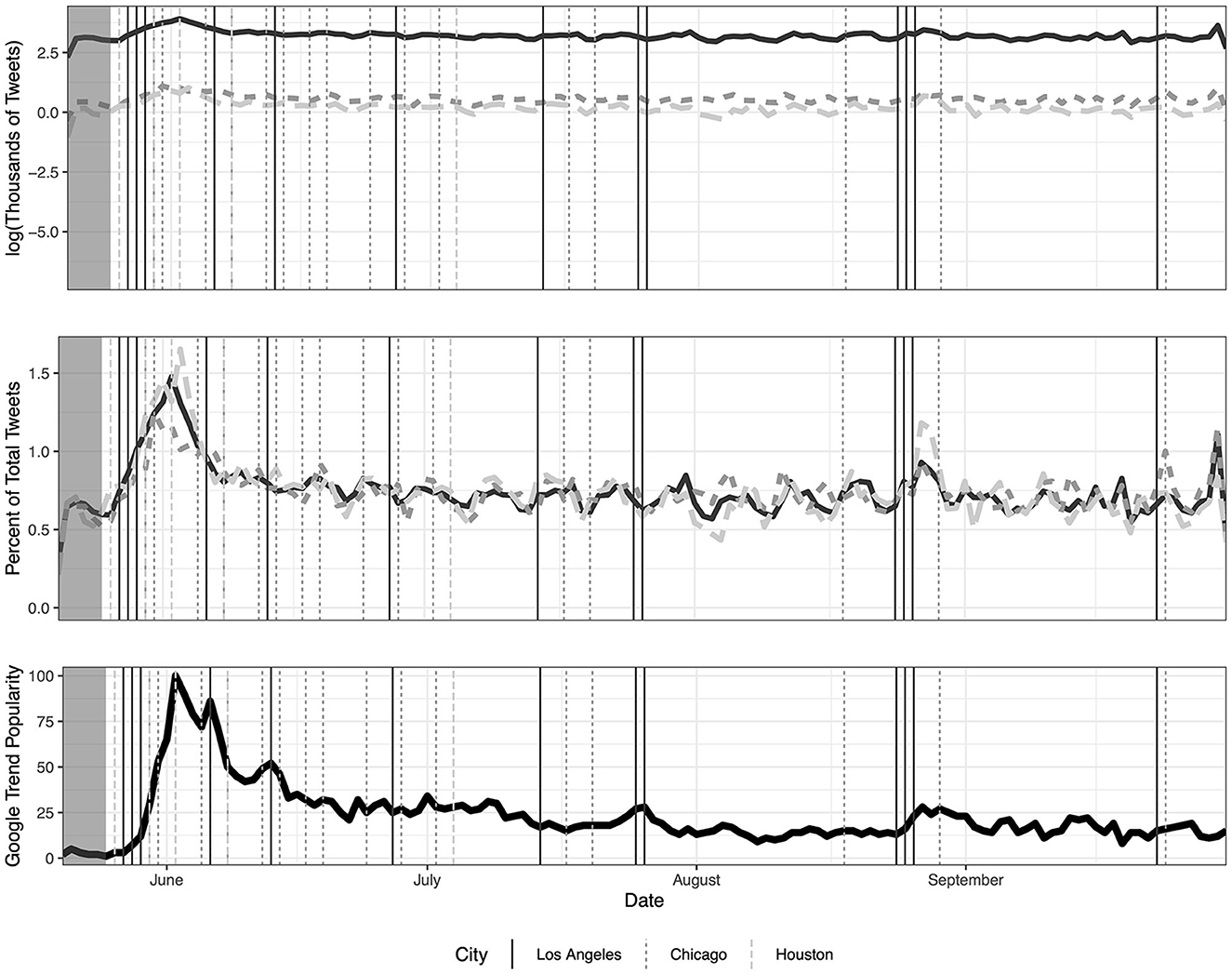
Figure 1. Overview of tweets collected for the summer of 2020. The top panel shows the total tweets collected, the middle panel shows the percent of tweets for each state collected on a date and the bottom panel shows the Google Trends data for the keyword “BLM” in the country as a whole as well as vertical lines for protests which were investigated in this paper. The grey area represents the time before the murder of George Floyd.
Each protester's tweet history is then combined into a single dataset which is used for subsequent analysis. The collective identity and interest estimates, explained starting in Section 3.3, are then assigned to each tweet.
3.2. Ethical considerations
The collection and analysis of the data was reviewed and approved by the Institutional Review Board at the California Institute of Technology. In this study, we did not ask Twitter users for permission to observe their Twitter history or use this data in our analysis. This approach is consistent with other work using similar social media data. By joining Twitter and using a public account, individuals accept the Twitter terms of use that specifically state that their content is public information. There is an additional concern, however, that use of Twitter data in research or publishing tweets with identifying information could put users at risk. Though public tweets are available to anyone by definition, users may expect that their public tweets will remain within their individual social sphere. Thus, if researchers expose the views of vulnerable individuals in their research, it could lead to harassment or retaliation. This concern is particularly acute when the topic is polarizing and contentious or the individuals in question belong to a group that has a history of suffering exploitation. A final concern comes from using the geolocation information provided. Users choose how much of their location to share, a setting that can be changed for each tweet individually or for the account as a whole, but they may not realize others see location information.
This study uses four strategies to mitigate these risks. First, the social media data collected is analyzed and presented at the aggregate level—we do not present nor publish individual tweets along with identifying information. Second, we do not attempt to discover the true identities of the users. Thirdly, upon publication we will share only the tweet identification numbers, consistent with the terms of academic use of these data. Finally, location is only used for city assignment. We do not use higher resolution spatial information and do not request geolocation information when downloading each protester's previous tweets.
3.3. Reverse joint sentiment topic analysis
This paper's raw data is 3,810,307 tweets. In order to test the hypotheses, we need to find a way of reducing the dimensionality of our text data. We do this by classifying the tweets as belonging to certain clusters. Specifically, we use a Reverse Joint Sentiment Topic Model (RJST) as presented in Lin et al. (2011) to define each tweet by a lower dimension topic and sentiment. RJST works by finding clusters of words that are used frequently together in order to define groupings. RJST, while based on a Latent Dirichlet Allocation (LDA) model, includes a second latent layer that allows us to account for additional structure that the simple LDA model may overlook. A detailed discussion of RJST, our results, and the diagnostics regarding topic selection and validation can be found in Supplementary Section 2.1.
The final model used generates 5 topics and 3 sentiments for a total of 15 groupings. Table 2 shows the list of author-generated labels for each group. For each tweet, there is a probability measure θ which represents the proportion of the tweet belonging to each topic. Within each document and topic, there is a probability measure π which represents the distribution of sentiment within each topic in the document. Thus, by multiplying the probability measures we are able to get a value for how much of each tweet is in each topic sentiment pair (for instance θ1π12 is how much the tweet is in Topic1Sentiment2). These values will be important for analyzing the content of the tweets going forward. In addition, we label the four senTopics which begin with “BLM” as the relevant topics for the analysis; these topics will form the foundation for our analysis.
The validity of these labels is tested in multiple ways, the details of which are presented in Supplementary Section 2.4. First, is the distribution of the topics over time: the topics labeled as related to BLM clearly follow the same pattern as the Google Trend data on the topic. Supplementary Figure 2.3 shows this concordance. Next, we look at the percent related to BLM the tweets are which were found using keyword and location information and compare it to the distribution of those in the individuals timelines in general. The results, seen in Supplementary Figure 2.4 show that those tweets we know are related to BLM score high while the overall tweets are distributed much lower. Finally, we took a sample of 800 tweets and had four individuals rate the percent they believe the tweet is related to BLM, the results can bee seen in the Supplementary Figure 2.5. The correlation between the RJST result and the average hand labeling is 80%. Overall, these three tests lead us to be confident in the RJST model accurately labeling the relevance of tweets to the BLM movement.
3.4. Operationalizing the hypotheses
3.4.1. Measurement
Every tweet for every individual is given an interest and collective identity score. Given that on day t individual i tweets N times, for each n∈{1, ..., N}, there is a topic distribution and a sentiment distribution for each topic in each tweet . In order to get the senTopic distribution, we multiply the sentiment distribution by the corresponding element in the topic distribution. These tweet-level measures are then aggregated to estimate the individuals' daily interest and collective identity scores.
In order to calculate the interest score for each individual on each day we first calculate tweet-level interest scores. For each tweet, we take the mean of the sums of the senTopic distributions multiplied by a BLM indicator:
This calculation estimates the percentage of the tweet discussing BLM. Specifically, for our data we have that δℓ, k = 1 for the pairs (1, 1), (1, 2), (4, 2), (4, 3) and is zero for the rest. This suggests that these four senTopics indicate discussion of BLM while the rest are unrelated. The score for each tweet in our data set is the sum of the BLM scores:
In order to get the daily score, we take the average score for the day:
This value represents how much of an individual's daily Twitter production is devoted to discussion of BLM—their daily interest.
In order to find individuals' collective identity scores, we look at the levels of explicit group belonging in the topic-related tweets and call this variable . This value is found by first categorizing the percent of the pronouns in each tweet that are plural, cn, t, i∈(0, 1). This tweet level value is a representation of how closely an individual identifies with the subject matter of the tweet. We then take the weighted average, using the interest score over the tweets for each day, to observe to what extent the individual discusses the topic of the protests as part of the group rather than as the individual. Weighting by interest score is necessary to capture identity relevant to the BLM protests as opposed to other manifestations of collective identity, i.e., a tweets such as “We are sad the NBA playoffs have been canceled” expresses collective identity but is not about the protests and therefore receives a score of 0 for collective identity. Equation (11) shows this calculation.
For each tweet in the dataset, we label individuals as having protested for those days in which their tweets are originally collected. For all other protests, we mark the individuals as not protesting. This binary variable is the most straightforward we use. Supplementary Tables 1.2–1.4 show the protest dates, the number of protests drawn, and the estimated size of each protest.
These daily scores are our values of interest as we proceed.
3.4.2. Example tweet calculations
In order to clarify the process above, we now show how the values are calculated for three tweets in our data. Table 3 shows these tweets.
Reading these tweets, it is clear that tweets 1 and 2 are related to Black Lives Matter while tweet 3 discusses COVID-19. We therefore expect 1 and 2 to be high on the interest score and 3 to be low. Tweet 1 should also score high on collective identity—the user is identifying with the group claiming, “People are angry, as we should be” (emphasis added). On the other hand, Tweet 2 is more observational, so it should score lower for collective identity. Finally, tweet 3 is not related to BLM, but there is a high level of collective identity with respect to being a Houstonian. Ideally, the algorithm should down weight this tweet after applying the weighting.
The RJST model outputs the percent that each tweet falls into each topic and within each topic, and each sentiment. Table 4 shows the scores for each of the three example tweets. The BLM related sentiment topic pairs are bolded. From looking at the distributions, we can see that Tweet 1 is related to the city news category while Tweet 2 is related to the George Floyd/Breonna Taylor topic as well as the police violence one. Tweet 3 is almost entirely related to Covid. These characterizations are sensible when looking at the content of the tweets and these examples give confidence in the reliability of the topic modeling. Summing the distributions in the BLM labeled topics provides the tweet level interest value (yitn). The tweet level identity scores are also as expected—tweets 1 and 3 are high while tweet 2 is low.
Assuming these three tweets came from a single day, and they were the user's only tweets for the day, the daily interest and identity scores are calculated as:
Both of these scores make sense when looking at the three tweets chosen. About 2/3 of the tweets are clearly related to BLM. In addition, of the tweets that are related to BLM, TweetID 1 has what would be considered a strong collective identity score while the other is weak. The identity values of non-BLM related tweets should barely come into play.
3.4.3. Testing the hypotheses
How these account signal values— and —correlate with protest attendance provides the test for the paper's three hypotheses.
To test Hypothesis 1, we create a prediction of whether an individual protests based on their signal values. A logit model with day and individual fixed effects provides this prediction. First, we segment the data to only include days in which protests occurred—this is to prevent null results on the days in which protests do not occur. We then run the model solving for:
The value and significance of α1 and α2 indicate the effect of the levels of these signals on protesting.
For Hypotheses 2 and 3, we run a time and individual fixed effect OLS model with indicators for the relative date of the tweet compared to a protest event the individual participated in if the relative date is between –4 and 4 days inclusive. Thus, given that an individual protests at time τ we are solving for:
The values for α1 − 5 represent the change in signal value if the individual protests at relative time 0 compared to the counterfactual that they did not protest. Statistically significant positive values for α4 will provide evidence in support of Hypothesis 2. If α3 is positive and statistically significant, this provides evidence in support of Hypothesis 3.
4. Results
Interest strongly supports all three hypotheses. In addition, collective identity supports hypotheses 1 and 2, although the magnitude of the results are smaller. In order to verify that any significant result is not spurious, we also create two placebo tests by setting the protest day to 10 days prior and subsequent to the actual protest. Table 5 shows the OLS results, while the Supplementary Tables 3.2–3.5 show placebo tests.
Analysis of both signals support Hypothesis 1. In Table 6, the average partial effects are displayed for the logit model using both identity and interest as well as the two independently. City fixed effects are included in the table due to their significance. There were no additional significant terms when interactions were included. The model was also evaluated using a truncated version of the model—only using individuals who tweeted during a significant number of protests—but this truncation did not change the results. The combined model shows that changing an individual's interest from 0 to 1 causes a 9% increase in the probability that they protest, while changing the identity score from 0 to 1 has a 1.4% increase in the probability of protesting.
In the regression with interest as the dependent variable, where interest is what percent of an individual's daily tweets are in the topics labeled as about the BLM movement, we see significant positive results the day before, day of, and 2 days after the protest. Following this, the results are not statistically significant. In addition, the F statistic is significant at the 0.01 level, indicating a good fit of the model. This result suggests that individuals spend about 1.4% more of their Twitter time discussing BLM the day before they protest than they would if they were not going to protest. On the day of a protest, their interest level is on average 6.7% more relevant than it would be otherwise (supporting Hypothesis 2 for interest) and 10% more relevant the day after (supporting Hypothesis 3). By 2 days after, there is still an increase (3.4%), but the interest level is returning back to non-protesting levels. While we see that in the location-pooled model there is a sustained increase 3 and 4 days after the protest, when including interaction terms for protest location, this result varied by location. Supplementary Section 3.2 shows the significant results for the fully interacted model. As the average amount the sample talks about BLM in the time period ranges from about 20-60%, we view these results as substantially significant in addition to statistically significant.
In addition to the interest-level dynamics related to the hypotheses, it is interesting to note that before protesting, interest levels have a small increase. On the day of the protest, interest levels increase substantially. This trend continues through the day after the protest, after which the results begin to dissipate. When the same test is ran for a placebo protest date 10 days before the real protest, none of the results are significant. When the test is run around relative day 10 there are still some slight increases on days 8 and 9 (1.4% and 1.3% , respectively), but these values are only significant at the 0.1 level. Overall, the results combined with the placebo test supports both Hypotheses 2 and 3 for the interest signal.
For collective identity, there is a 1.6% increase of it the day of protests. This result is significant at the 0.05 level. While this increase is approximately that of interest, the placebo test produces null results. There are no significant results for the rest of the protest-relative days. In Figure 2, we plot the coefficient values around the date of protest and report the 95% confidence interval.
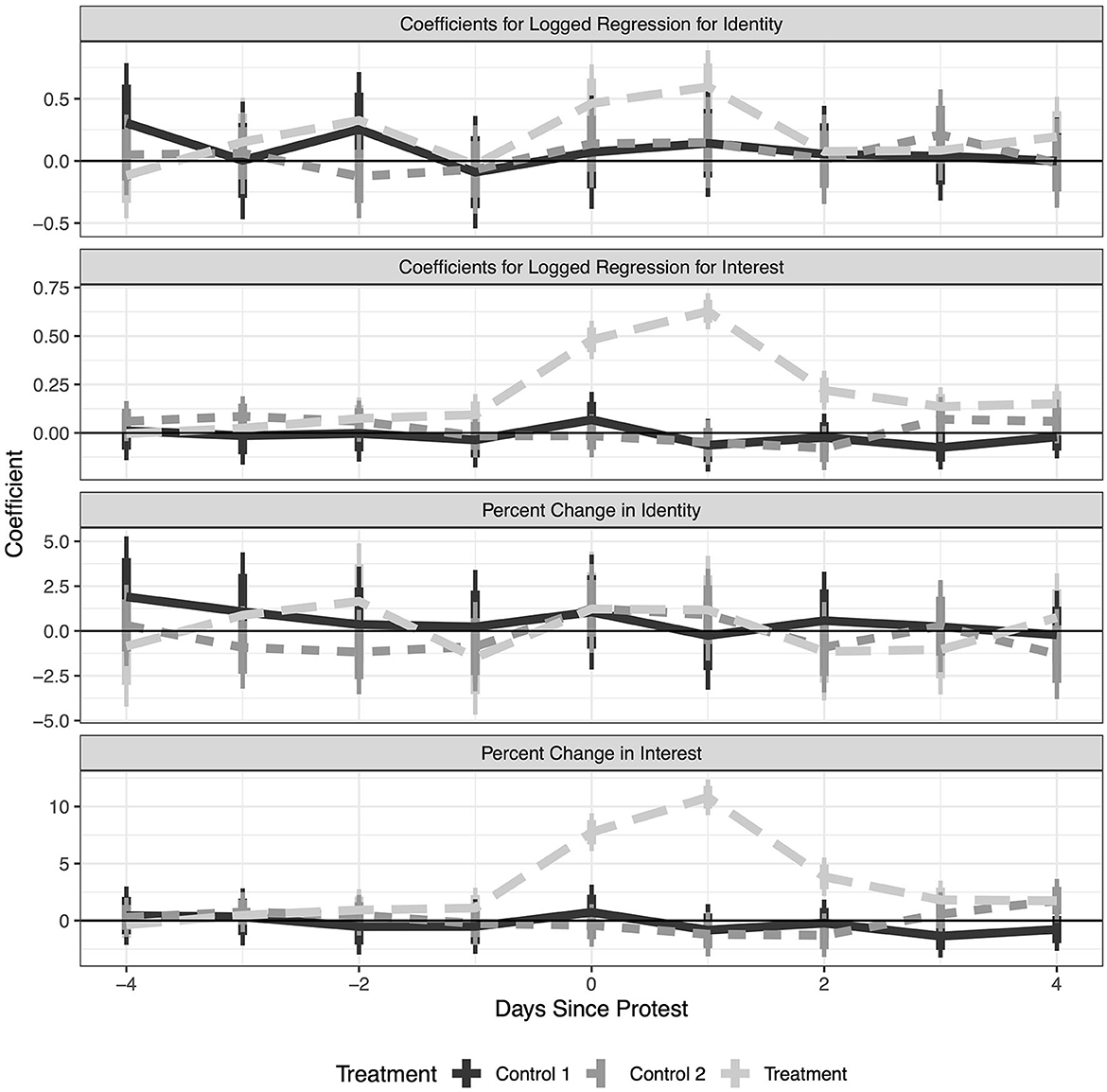
Figure 2. Changes in interest and identity when protesting. The thick error bars are the 90% confidence interval while the thinner one is 99%. The scales of the plots are different. The first and second are the coefficients for the log OLS, while less intuitively interpretable, they reflect a similar trend to the third and fourth which reflect a percent change in interest or identity. These results visually represent the regression information found in Table 5.
5. Discussion
This paper contributes to the collective action literature by distinguishing between collective identity and interest as similar but separate motivations for individuals deciding whether or not to protest. An individual may protest because part of their identity is aligned with a larger collective, such as an occupational or racial group, and this alignment increases the perceived private benefit of protesting. An individual may also protest when their interests are closer to the policy change toward which protesters push. This distinction is especially important for studies using digital trace data since collective identity has been operationalized with hashtags or images. This paper develops and applies a weakly supervised topic model to to 3.8 million tweets from Black Lives Matter protesters in Los Angeles, Chicago, and Houston, allowing for the decomposition of individuals' motivations into collective identity and interest components. A series of regression models and placebo tests suggest that interest more strongly explains protest participation than collective identity. These results suggest that previous work which finds collective identity drives protest mobilization does so because of the measurement conflation of interest with collective identity.
6. Discussion
Several features of this paper's research design could explain this provocative result. One is the unique nature of the 2020 Black Lives Matter protests. Extensive news media coverage of racial injustice and policy brutality drove strong interest in the protests, so collective identity was not needed to mobilize participation in protests. In addition, if individuals from a group frequently protest and collective identity drives their protest, then when members of other groups join a protest it is more likely due to interest than the new participants' sense of collective identity. In other words, 2020 was not the first time, even recently, that Black Americans had protested police brutality; it is the first time in a long time they were joined by large numbers of individuals from other racial groups (Fisher, 2020; Fisher and Rouse, 2022).
The operationalization of collective identity and interest may also partially explain this paper's findings. Interest is assumed to reflect Twitter users' discussion of certain topics. This paper's topic model uses a dimension reduction technique, the authors inferred the topic of the dimensions, and then an account was determined to have interest based on tweets containing at least one of four topics. The results could therefore be driven by the authors' inference of interest as opposed to tweet authors' true interest. For example, it is possible that the interest topics reflect accounts' sense of perceived injustice, outrage, or other feelings that motivate action more than interest (Pearlman, 2018). Collective identity is then determined from the percent of pronouns that are first-person plural in the interest topics. This measurement is direct but identity is often a latent attribute of an individual, so the use of these pronouns may not mean that an author merges their identity with a collective's.
Despite these limitations, these results build on previous quantitative, non-social media research into identity and collective action in several ways. Collective identity is salient during the mobilization process in authoritarian settings (Pfaff, 1996; Pearlman, 2018). This contrast with the 2020 BLM protests suggests that identity may be less salient in settings where citizens have other means of organizing. In settings such as the United States, identity may therefore not be an axis on which to build boundary-spanning movements (Wang et al., 2018). The difficulty of mobilizing around identity is further heightened when the identity is race and there are prevailing biases against the group mobilizing (Manekin and Mitts, 2022).
Future research should proceed along three avenues. In order to further validate the results found in this paper, measuring interest and collective identity for other social movements should be performed. Other movements, such as the Yellow Vests in France, have different contexts and can be used to ascertain the generality of this paper's results. The second extension is to include individuals who did not protest as a baseline in order to measure differences in the interest and collective identity of those who protest and those who do not. Third, previous studies show that identity motivates changes in online behavior (Munger, 2016; Siegel and Badaan, 2020; Taylor et al., 2022). This paper's results suggest that collective identity is less important in changing offline protest behavior . Future work should continue to explore the differential effects of identity.
This paper provides a framework in which to study protest movements and individual signals of collective action. It enables the contextualization of much of the previous quantitative work on the subject and takes a step toward unifying it into a unified conversation. While there is clear future work to be done, this paper provides a first step in these efforts.
Data availability statement
The raw data supporting the conclusions of this article will be made available by the authors, without undue reservation.
Ethics statement
The studies involving human participants were reviewed and approved by Institutional Review Board at the California Institute of Technology. Written informed consent for participation was not required for this study in accordance with the national legislation and the institutional requirements.
Author contributions
CK, SH, ZS-T, and RA: research design and paper writing and editing. CK and SH: software development and data collection. CK: data preprocessing and analysis. CK, ZS-T, and RA: interpretation of results. CK and RA: project management. All authors contributed to the article and approved the submitted version.
Funding
SH's work on this project in 2021 was supported by a Summer Undergraduate Byrant Family Research Fellowship from Caltech.
Acknowledgments
We thank the Google Cloud Research Credits Program for providing credits for our use of the Google Cloud Platform for data collection and analysis. Thanks to the audience and discussants at the 2022 Midwest Political Science Association Annual Meeting for comments. Thanks also to Marisa Abrajano, William Hobbs, Melina Much, Danny Ebanks, Jacob Morrier, and Sabrina Hameister for their valuable feedback.
Conflict of interest
The authors declare that the research was conducted in the absence of any commercial or financial relationships that could be construed as a potential conflict of interest.
Publisher's note
All claims expressed in this article are solely those of the authors and do not necessarily represent those of their affiliated organizations, or those of the publisher, the editors and the reviewers. Any product that may be evaluated in this article, or claim that may be made by its manufacturer, is not guaranteed or endorsed by the publisher.
Supplementary material
The Supplementary Material for this article can be found online at: https://www.frontiersin.org/articles/10.3389/fpos.2023.1185633/full#supplementary-material
Footnotes
1. ^For more details on the on BLM movement and racial inequality in the United States please refer to Bunyasi and Smith (2019).
2. ^New York City is excluded because the amount of data would have introduced significant data storage issues and computational complexities.
3. ^According to the Crowd Counting Consortium, these cities make up 14% of the protesters and 2% of the protests . Houston made up 9% of the people but only 0.2% of the protests while Los Angeles and Chicago were both about 2% and 1% for protesters and protests , respectively.
4. ^Twitter is a biased sample of Americans (Mitchell et al., 2021), so this paper's results are most applicable for the subset of Americans on Twitter.
5. ^Past tense is used here because Twitter has become much less generous with sharing data since Elon Musk became its owner.
6. ^While we refer to each Twitter account as an individual , it is possible an account is actually for an organization. The differentiation between individual and organization is beyond the scope of this project.
7. ^https://developer.twitter.com/en/docs/twitter-api
8. ^https://github.com/geduldig/TwitterAPI
9. ^The data was collected roughly a year after the protests occurred, in that time if people delete their tweets or accounts the tweets will not show up in our dataset. In addition, some accounts are set to private. Those tweets and accounts will also not show up in our set . We are aware of no research quantifying this decay rate, but studies using Twitter and Facebook in China, Colombia, and Uganda have found no differences in results when comparing this paper's method to data collected in real time (Morales, 2021; Boxell and Steinert-Threlkeld, 2022; Chang et al., 2022).
References
Arora, M., and Stout, C. T. (2019). Letters for black lives: co-ethnic mobilization and support for the black lives matter movement. Polit. Res. Q. 72, 389–402. doi: 10.1177/1065912918793222
Bonilla, T., and Tillery, A. B. (2020). Which identity frames boost support for and mobilization in the #BlackLivesMatter movement? An experimental test. Am. Polit. Sci. Rev. 114, 947–962. doi: 10.1017/S0003055420000544
Boxell, L., and Steinert-Threlkeld, Z. (2022). Taxing dissent: the impact of a social media tax in Uganda. World Dev. 158, 105950. doi: 10.1016/j.worlddev.2022.105950
Boyd, D., Golder, S., and Lotan, G. (2010). “Tweet, tweet, retweet: conversational aspects of retweeting on Twitter,” in 2010 43rd Hawaii International Conference on System Sciences (IEEE), 1–10.
Brock, A. (2012). From the Blackhand side: Twitter as a cultural conversation. J. Broadcast. Electron. Media 56, 529–549. doi: 10.1080/08838151.2012.732147
Buchanan, L., Bui, Q., and Patel, J. K. (2020). Black Lives Matter may be the largest movement in U.S. history. New York, NY: The New York Times.
Bueno De Mesquita, E. (2010). Regime change and revolutionary entrepreneurs. Am. Polit. Sci. Rev. 104, 446–466. doi: 10.1017/S0003055410000274
Bunyasi, T. L., and Smith, C. W. (2019). Stay Woke: A People's Guide to Making All Black Lives Matter. New York, NY: NYU Press.
Burns, A., and Eltham, B. (2009). “Twitter free Iran: an evaluation of Twitter's role in public diplomacy and information operations in Iran's 2009 election crisis.” in Communications Policy and Research Forum (Sydney).
Chang, K.-C., Hobbs, W. R., Roberts, M. E., and Steinert-Threlkeld, Z. C. (2022). COVID-19 increased censorship circumvention and access to sensitive topics in China. Proc. Natl. Acad. Sci. U.S.A. 119. doi: 10.1073/pnas.2102818119
Chenoweth, E., Hamilton, B. H., Lee, H., Papageorge, N. W., Roll, S. P., and Zahn, M. V. (2022). Who Protests, What Do They Protest, and Why? National Bureau of Economic Research.
Chenoweth, E., and Pressman, J. (2017). Crowd Counting Consortium. Available online at: crowdcounting.org (accessed January, 2021).
Chong, D. (1991). Collective Action and the Civil Rights Movement. Chicago, IL: University of Chicago Press.
Chong, D., Rogers, R., and Tillery, A. B. (2004). “Reviving group consciousness.” in The Politics of Democratic Inclusion (Philadelphia, PA: Temple University Press), 45–74.
Clark-Parsons, R. (2022). Networked Feminism: How Digital Media Makers Transformed Gender Justice Movements. Berkeley, CA: University of California Press.
Conover, P. J. (1988). The role of social groups in political thinking. Brit. J. Polit. Sci. 18, 51–76.
Cox, J. M. (2017). The source of a movement: making the case for social media as an informational source using Black Lives Matter. Ethnic Racial Stud. 40, 1847–1854. doi: 10.1080/01419870.2017.1334935
Crowder, C. (2020). Following radical and mainstream African-American interest groups on social media: an intersectional analysis of Black organizational activism on Twitter. Natl. Rev. Black Polit. 1, 474–495. doi: 10.1525/nrbp.2020.1.4.474
Crowder, C. (2021). When #BlackLivesMatter at the women's march: a study of the emotional influence of racial appeals on instagram. Polit. Groups Ident. 11, 55–73. doi: 10.1080/21565503.2021.1908373
Dawson Michael, C. (1994). Behind the Mule: Race and Class in African-American Politics. Princeton, NJ: Princeton University Press.
Driscoll, J., and Steinert-Threlkeld, Z. C. (2020). Social media and Russian territorial irredentism: some facts and a conjecture. Post Soviet Aff. 36, 101–121. doi: 10.1080/1060586X.2019.1701879
Dunivin, Z. O., Yan, H. Y., Ince, J., and Rojas, F. (2022). Black Lives Matter protests shift public discourse. Proc. Natl. Acad. Sci. U.S.A. 119, e2117320119. doi: 10.1073/pnas.2117320119
Finkel, S. E., Muller, E. N., and Opp, K.-D. (1989). Personal influence, collective rationality, and mass political action. Am. Polit. Sci. Rev. 83, 885–903.
Fireman, B., and Gamson, W. A. (1977). Utilitarian logic in the resource mobilization perspective. CRSO Working Paper 153. Ann Arbor, MI.
Fisher, D. R. (2020). The Diversity of the Recent Black Lives Matter Protests is a Good Sign for Racial Equity. Brookings.
Fisher, D. R., and Rouse, S. M. (2022). Intersectionality within the racial justice movement in the summer of 2020. Proc. Natl. Acad. Sci. U.S.A. 119, e2118525119. doi: 10.1073/pnas.2118525119
Freelon, D., McIlwain, C., and Clark, M. (2018). Quantifying the power and consequences of social media protest. New Media Soc. 20, 990–1011. doi: 10.1177/1461444816676646
Freelon, D., Mcllwain, C. D., and Clark, M. (2016a). Beyond the Hashtags: #Ferguson, #Blacklivesmatter, and the Online Struggle for Offline Justice. Center for Media & Social Impact, American University. Available online at: https://ssrn.com/abstract=2747066
Gause, L. (2022). Costly protest and minority representation in the United States. Polit. Sci. Polit. 55, 279–281. doi: 10.1017/S1049096521001591
Gerber, A. S., Green, D. P., and Larimer, C. W. (2008). Social pressure and voter turnout: evidence from a large-scale field experiment. Am. Polit. Sci. Rev. 102, 33–48. doi: 10.1017/S000305540808009X
Giorgi, S., Guntuku, S. C., Himelein-Wachowiak, M., Kwarteng, A., Hwang, S., Rahman, M., et al. (2022). “Twitter corpus of the #BlackLivesMatter movement and counter protests: 2013 to 2021,” in Proceedings of the International AAAI Conference on Web and Social Media, 1228–35.
Hegselmann, R., and Krause, U. (2002). Opinion dynamics and bounded confidence: models, analysis and simulation. J. Artif. Soc. Soc. Simul. 5.
Hsiao, Y. (2021). Evaluating the mobilization effect of online political network structures: a comparison between the Black Lives Matter network and ideal type network configurations. Soc. Forces 99, 1547–1574. doi: 10.1093/sf/soaa064
Ince, J., Rojas, F., and Davis, C. A. (2017). The social media response to Black Lives Matter: how Twitter users interact with Black Lives Matter through hashtag use. Ethnic Racial Stud. 40, 1814–1830. doi: 10.1080/01419870.2017.1334931
Jackson, S. J., and Foucault Welles, B. (2016). #Ferguson is everywhere: initiators in emerging counterpublic networks. Inform. Commun. Soc. 19, 397–418. doi: 10.1080/1369118X.2015.1106571
Jasper, J. M. (1997). The Art of Moral Protest: Culture, Biography, and Creativity in Social Movements. Chicago, IL: University of Chicago Press.
Johnston, H., and Klandermans, B. (1995). Social Movements and Culture: Social Movements, Protest, and Contention, Vol. 4. Minneapolis, MN: University of Minnesota Press.
Kann, C., Hashash, S., Steinert-Threlkeld, Z., and Alvarez, R. (2023). Gathertweet: a Python package for collecting social media data on online events. J. Comput. Commun. 11, 172–193. doi: 10.4236/jcc.2023.112012
Karami, A., Kadari, R. R., Panati, L., Nooli, S. P., Bheemreddy, H., and Bozorgi, P. (2021). Analysis of geotagging behavior: do geotagged users represent the Twitter population? ISPRS Int. J. Geoinform. 10, 373. doi: 10.3390/ijgi10060373
Kurzman, C. (1996). Structural opportunity and perceived opportunity in social-movement theory: the Iranian Revolution of 1979. Sociol. Rev. 61, 153–170.
Larson, J. M., Nagler, J., Ronen, J., and Tucker, J. A. (2019). Social networks and protest participation: evidence from 130 million Twitter users. Am. J. Polit. Sci. 63, 690–705. doi: 10.1111/ajps.12436
Lin, C., He, Y., Everson, R., and Ruger, S. (2011). Weakly supervised joint sentiment-topic detection from text. IEEE Trans. Knowledge Data Eng. 24, 1134–1145. doi: 10.1109/TKDE.2011.48
Little, A. T. (2016). Communication technology and protest. J. Polit. 78, 152–166. doi: 10.1086/683187
Lohmann, S. (1994). The dynamics of informational cascades: the monday demonstrations in Leipzig, East Germany, 1989-91. World Polit. 47, 42–101.
Long, S., and McCarthy, J. (2020). Two in Three Americans Support Racial Justice Protests. The Gallup Organization.
Madestam, A., Shoag, D., Veuger, S., and Yanagizawa-Drott, D. (2013). Do political protests matter? Evidence from the Tea Party movement. Q. J. Econ. 128, 1633–1685. doi: 10.1093/qje/qjt021
Manekin, D., and Mitts, T. (2022). Effective for whom? Ethnic identity and nonviolent resistance. Am. Polit. Sci. Rev. 116, 161–180. doi: 10.1017/S0003055421000940
Matthews, D. R., and Prothro, J. W. (1966). Negroes and The New Southern Politics. New York, NY: Harcourt, Brace & World.
McAdam, D. (1986). Recruitment to high-risk activism: the case of freedom summer. Am. J. Sociol. 92, 64–90.
McClain, P. D., Johnson Carew, J. D., Walton, E. Jr., and Watts, C. S. (2009). Group membership, group identity, and group consciousness: measures of racial identity in American politics? Annu. Rev. Polit. Sci. 12, 471–485. doi: 10.1146/annurev.polisci.10.072805.102452
Metzger, M. M., Bonneau, R., Nagler, J., and Tucker, J. A. (2016). Tweeting identity? Ukrainian, Russian, and #Euromaidan. J. Comp. Econ. 44, 16–40. doi: 10.1016/j.jce.2015.12.004
Miller, A. H., Gurin, P., Gurin, G., and Malanchuk, O. (1981). Group consciousness and political participation. Am. J. Polit. Sci. 25, 494–511. doi: 10.2307/2110816
Mitchell, A., Shearer, E., and Stocking, G. (2021). News on Twitter: Consumed by Most Users and Trusted by Many. Technical report, Pew Research Center.
Mitts, T., Phillips, G., and Walter, B. F. (2022). Studying the impact of ISIS Propaganda Campaigns. J. Polit. 84, 1220–1225. doi: 10.1086/716281
Morales, J. S. (2021). Legislating during war: conflict and politics in Colombia. J. Public Econ. 193, 104325. doi: 10.1016/j.jpubeco.2020.104325
Munger, K. (2016). Tweetment effects on the tweeted: experimentally reducing racist harassment. Polit. Behav. 39, 629–649. doi: 10.1007/s11109-016-9373-5
Olteanu, A., Weber, I., and Gatica-Perez, D. (2015). Characterizing the demographics behind the #BlackLivesMatter movement. arXiv preprint arXiv:1512.05671. doi: 10.48550/arXiv.1512.05671
Ostrom, E. (1990). Governing the Commons: The Evolution of Institutions for Collective Action. Cambridge: Cambridge University Press.
Ostrom, E. (2000). Collective action and the evolution of social norms. J. Econ. Perspect. 799, 137–158. doi: 10.1257/jep.14.3.137
Pearlman, W. (2018). Moral identity and protest cascades in Syria. Brit. J. Polit. Sci. 48, 877–901. doi: 10.1017/S0007123416000235
Pearlman, W. (2021). Mobilizing from scratch: large-scale collective action without preexisting organization in the Syrian uprising. Comp. Polit. Stud. 54, 1786–1817. doi: 10.1177/0010414020912281
Petitjean, C., and Talpin, J. (2022). Tweets and doorknocks. Differentiation and cooperation between Black Lives Matter and community organizing. Perspect. Polit. 20, 1275–1289. doi: 10.1017/S1537592722001049
Pfaff, S. (1996). Collective identity and informal groups in revolutionary mobilization: East Germany in 1989. Soc. Forces 75, 91–117.
Polletta, F., and Jasper, J. M. (2001). Collective identity and social movements. Annu. Rev. Sociol. 27, 283–305. doi: 10.1146/annurev.soc.27.1.283
Putnam, L., Chenoweth, E., and Pressman, J. (2020). The Floyd Protests Are the Broadest in U.S. History—and Are Spreading to White, Small-Town America. Washington, DC: The Washington Post — Monkey Cage.
Rahimi, B. (2011). The agonistic social media: cyberspace in the formation of dissent and consolidation of state power in postelection Iran. Commun. Rev. 14, 158–178. doi: 10.1080/10714421.2011.597240
Raleigh, C., Linke, A., Hegre, H., and Karlsen, J. (2010). Introducing ACLED-armed conflict location and event data. J. Peace Res. 47, 651–660. doi: 10.1177/0022343310378914
Ray, R., Brown, M., Fraistat, N., and Summers, E. (2017). Ferguson and the death of Michael Brown on Twitter: #BlackLivesMatter, #TCOT, and the evolution of collective identities. Ethnic Racial Stud. 40, 1797–1813. doi: 10.1080/01419870.2017.1335422
Reny, T. T., and Newman, B. J. (2021). The opinion-mobilizing effect of social protest against police violence: evidence from the 2020 George Floyd protests. Am. Polit. Sci. Rev. 115, 1499–1507. doi: 10.1017/S0003055421000460
Sanchez, G. R. (2006). The role of group consciousness in political participation among Latinos in the United States. Am. Polit. Res. 34, 427–450. doi: 10.1177/1532673X05284417
Sanchez, G. R., and Vargas, E. D. (2016). Taking a closer look at group identity: the link between theory and measurement of group consciousness and linked fate. Polit. Res. Q. 69, 160–174. doi: 10.1177/1065912915624571
Shadmehr, M., and Bernhardt, D. (2011). Collective action with uncertain payoffs: coordination, public signals, and punishment dilemmas. Am. Polit. Sci. Rev. 105, 829–851. doi: 10.1017/S0003055411000359
Shayo, M. (2009). A model of social identity with an application to political economy: nation, class, and redistribution. Am. Polit. Sci. Rev. 103, 147–174. doi: 10.1017/S0003055409090194
Shuman, E., Hasan-Aslih, S., van Zomeren, M., Saguy, T., and Halperin, E. (2022). Protest movements involving limited violence can sometimes be effective: evidence from the 2020 Black Lives Matter protests. Proc. Natl. Acad. Sci. U.S.A. 119, e2118990119. doi: 10.1073/pnas.2118990119
Siegel, A. A., and Badaan, V. (2020). #No2Sectarianism: experimental approaches to reducing sectarian hate speech online. Am. Polit. Sci. Rev. 114, 837–855. doi: 10.1017/S0003055420000283
Siegel, D. A. (2009). Social networks and collective action. Am. J. Polit. Sci. 53, 122–138. doi: 10.1111/j.1540-5907.2008.00361.x
Sobolev, A., Joo, J., Chen, K., and Steinert-Threlkeld, Z. C. (2020). News and geolocated social media accurately measure protest size variation. Am. Polit. Sci. Rev. 114, 1343–1351. doi: 10.1017/S0003055420000295
Steinert-Threlkeld, Z., Chan, A., and Joo, J. (2022). How state and protester violence affect protest dynamics. J. Polit. 84, 798–813. doi: 10.33774/apsa-2019-bv6zd-v3
Steinert-Threlkeld, Z. C. (2017). Spontaneous collective action: peripheral mobilization during the Arab spring. Am. Polit. Sci. Rev. 111, 379–403. doi: 10.1017/S0003055416000769
Stokes, A. K. (2003). Latino group consciousness and political participation. Am. Polit. Res. 31, 361–378. doi: 10.1177/1532673X03031004002
Tate, K. (1994). From Protest to Politics: The New Black Voters in American Elections. Cambridge, MA: Harvard University Press.
Taylor, S. J., Muchnik, L., Kumar, M., and Aral, S. (2022). Identity effects in social media. Nat. Hum. Behav. 7, 27–37. doi: 10.1038/s41562-022-01459-8
Teske, N. (1997). Political Activists in America: The Identity Construction Model of Political Participation. Cambridge University Press.
Tillery, A. B. (2019). What kind of movement is Black lives matter? The view from Twitter. J. Race Ethnicity Polit. 4, 297–323. doi: 10.1017/rep.2019.17
Tong, X., Li, Y., Li, J., Bei, R., and Zhang, L. (2022). What are people talking about in #BlackLivesMatter and #StopAsianHate? Exploring and categorizing Twitter topics emerging in online social movements through the latent dirichlet allocation model. arXiv preprint arXiv:2205.14725. doi: 10.1145/3514094.3534202
Turner-Zwinkels, F. M., and van Zomeren, M. (2021). Identity expression through collective action: how identification with a politicized group and its identity contents differently motivated identity-expressive collective action in the U.S. 2016 presidential elections. Pers. Soc. Psychol. Bull. 47, 499–513. doi: 10.1177/0146167220933406
Valenzuela, S. (2013). Unpacking the use of social media for protest behavior: the roles of information, opinion expression, and activism. Am. Behav. Sci. 57, 920–942. doi: 10.1177/0002764213479375
Verba, S., and Nie, N. H. (1987). Participation in America: Political Democracy and Social Equality. Chicago, IL: University of Chicago Press.
Wang, D., Piazza, A., and Soule, S. A. (2018). Boundary-spanning in social movements: antecedents and outcomes. Annu. Rev. Sociol. 44, 167–187. doi: 10.1146/annurev-soc-073117-041258
Westwood, S. J., Grimmer, J., Tyler, M., and Nall, C. (2022). Current research overstates American support for political violence. Proc. Natl. Acad. Sci. U.S.A. 119, e2116870119. doi: 10.1073/pnas.2116870119
Williamson, V., Trump, K.-S., and Einstein, K. L. (2018). Black Lives Matter: evidence that police-caused deaths predict protest activity. Perspect. Polit. 16, 400–415. doi: 10.1017/S1537592717004273
Keywords: protest behavior, collective identity, Twitter, social media, BLM
Citation: Kann C, Hashash S, Steinert-Threlkeld Z and Alvarez RM (2023) Collective identity in collective action: evidence from the 2020 summer BLM protests. Front. Polit. Sci. 5:1185633. doi: 10.3389/fpos.2023.1185633
Received: 13 March 2023; Accepted: 26 June 2023;
Published: 18 July 2023.
Edited by:
Yuan Wang, City University of Hong Kong, Hong Kong SAR, ChinaReviewed by:
Lorien Jasny, University of Exeter, United KingdomRonni Michelle Greenwood, University of Limerick, Ireland
Copyright © 2023 Kann, Hashash, Steinert-Threlkeld and Alvarez. This is an open-access article distributed under the terms of the Creative Commons Attribution License (CC BY). The use, distribution or reproduction in other forums is permitted, provided the original author(s) and the copyright owner(s) are credited and that the original publication in this journal is cited, in accordance with accepted academic practice. No use, distribution or reproduction is permitted which does not comply with these terms.
*Correspondence: Claudia Kann, Y2thbm4mI3gwMDA0MDtjYWx0ZWNoLmVkdQ==