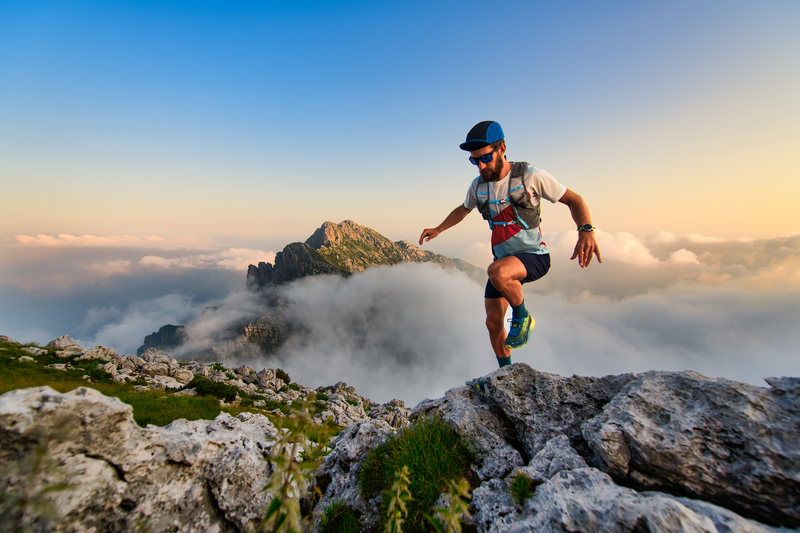
94% of researchers rate our articles as excellent or good
Learn more about the work of our research integrity team to safeguard the quality of each article we publish.
Find out more
ORIGINAL RESEARCH article
Front. Polit. Sci. , 01 August 2023
Sec. Political Science Methodologies
Volume 5 - 2023 | https://doi.org/10.3389/fpos.2023.1024729
Introduction: Although ethnocentrism is one of the fundamental concepts in the social sciences, its study has been impeded by a diversity of conceptualizations and measures. In recent years, a growing number of political scientists and psychologists have undertaken in-depth research into ethnocentrism. In addition, researchers have recently proposed a comprehensive reconceptualization of ethnocentrism and developed a new Ethnocentrism scale. There is strong evidence for this scale's reliability and validity in indexing ethnocentrism, but like most measures in psychology and political science, this scale is based on classical test theory. Item response theory (IRT) is a powerful psychometric technique that can provide a much more sophisticated test of test performance and is currently under-utilized in research.
Methods: We performed IRT to assess the psychometric properties of the Ethnocentrism scale on a sample of 4,187 participants.
Results: The scale's items had strong psychometric properties to capture the ethnocentrism latent construct, particularly in the below average to above average range. Men required marginally lower levels of ethnocentrism to endorse less socially acceptable items than women (items relating to superiority, purity, or exploitativeness). When compared to liberals, conservatives responded more readily to nearly all ethnocentrism items. Given this variation, the IRT approach highlighted that future measurements must adjust for differential item functioning, albeit more for political orientation than gender identity.
Discussion: The findings detail how IRT can enhance measurement in political science and demonstrate the implications for how gender and political ideology may affect the differential performance of items.
Ethnocentrism, the view that one's own ethnic group is of extreme importance, has been a fundamental concept in the social sciences since the early 20th century. Although there have been various attempts to measure ethnocentrism, improving our ability to accurately capture this socio-political attitude remains an important goal. Ludwig Gumplowicz introduced the concept of ethnocentrism in the late 1800s, and William G. Sumner popularized it in the early 1900s (Bizumic et al., 2021). The E-Scale, developed by Adorno et al. (1950), was the first comprehensive measure of ethnocentrism, and since then, researchers have continued to refine and develop instruments to measure it (Beswick and Hills, 1969; Neuliep and McCroskey, 1997; Altemeyer, 1998; Jost and Thompson, 2000; Kinder and Kam, 2009).
In the past 10–15 years, there has been an increasing interest among political science researchers to study ethnocentrism. Kam and Kinder (2007, 2012) and Kinder and Kam (2009) have comprehensively demonstrated the pervasive role of ethnocentrism in US public opinion, such as support for the war on terrorism and opposition to immigration, as well as voting for political candidates, such as the lower likelihood to vote for Obama in the 2008 presidential election. These authors have argued that ethnocentrism, although almost always present, may be activated in particular situations and can exert a powerful effect on public opinion. Similarly, Mansfield and Mutz (2009), Mansfield et al. (2019), Mutz et al. (2020), and Mutz (2021) showed that ethnocentrism, even when controlling for many related variables, exerts a consistent effect on people's opposition to international trade. Further, Valentino et al. (2013, 2018) have demonstrated a substantial effect of ethnocentrism on US participants' support for Donald Trump and on negative perceptions of the cultural and economic effects of immigration. Other recent political science research has investigated the role of ethnocentrism in a variety of contexts, such as consumer preferences in the US (Bankert et al., 2022), sacrifice of civil liberties after terrorist attacks in Germany (Hansen and Dinesen, 2022), support for the Bharatiya Janata Party in India (Ammassari et al., 2022), interethnic trust in 16 African countries (Robinson, 2020), and intergroup relations and policy preferences in Ukraine and Russia (Anderson, 2016).
Political science researchers have recently investigated ethnocentrism using a variety of self-report measures. Kinder and Kam (2009) constructed two measures of ethnocentrism: a cognitive measure that focused on having more favorable stereotypes about ethnic ingroups than ethnic outgroups (e.g., related to hard work, intelligence, and trustworthiness), and an affective measure that focused on affective warmth toward ethnic ingroups over ethnic outgroups. Many political science researchers (Mansfield and Mutz, 2009; Orey and Park, 2012; Sides and Gross, 2013; Valentino et al., 2013; Hainmueller and Hopkins, 2015; Pérez, 2015; Anderson, 2016) have used versions of these measures to investigate ethnocentrism in their research.
Despite the many psychometric strengths and popularity of Kinder and Kam's measure, it is important to acknowledge its limitations and avoid relying solely on any one particular measure of a construct (Monaghan and Bizumic, 2023). First, this approach is typically used to measure preferences for one group within a country (typically the ethnic majority) over two to three ethnic outgroups (typically ethnic minorities), whereas ethnocentrism is related to the belief that one's own ethnic group is better than any other in the world. Second, the two measures assess relative preferences, leading to certain confusion (e.g., a participant who gives the highest rating to the ingroup and outgroups will receive the same score as a participant who gives the lowest rating to the ingroup and outgroups; see Anderson, 2016). Finally, the measures have certain psychometric issues, such as a highly pronounced leptokurtic distribution and lower incremental validity over other existing measures (Bizumic et al., 2021).
Another approach to measuring ethnocentrism is by using specific items that target attitudes toward particular ethnic majorities (e.g., Whites in the US or Canada) and negative attitudes toward specific ethnic minorities (e.g., specific ethnic groups in the US or Canada). Examples of these measures are the E-Scale (Adorno et al., 1950), the Manitoba Ethnocentrism scale (Altemeyer, 1998), and the British Ethnocentrism scale (Warr et al., 1967). These measures are culture-specific, being limited to measuring ethnocentrism within specific cultures. As such, they are not suitable for making cross-cultural comparisons.
Bizumic et al. (2009) and Bizumic and Duckitt (2012) argued that many measures and conceptualisations of ethnocentrism were inconsistent with both the original definition of ethnocentrism and with many research findings. They proposed a reconceptualization of ethnocentrism as ethnic group self-centredness and self-importance. This approach consists of the two broad expressions of intergroup ethnocentrism and intragroup ethnocentrism. Intragroup ethnocentrism comprises the specific attitudes of devotion and group cohesion, whereas intergroup ethnocentrism comprises the specific attitudes of preference, superiority, purity, and exploitativeness.
In line with this conceptualization, Bizumic et al. (2009, 2021) and Bizumic (2019) developed the Ethnocentrism Scale, a culture-general measure that assesses a variety of ethnocentric attitudes. This scale served as a basis for research in political science (Sirin et al., 2017; Valentino et al., 2018; Ammassari et al., 2022; Hansen and Dinesen, 2022), though it has primarily been used in psychological research (Greitemeyer, 2012; Harrison, 2012; Cargile and Bolkan, 2013; Huxley et al., 2015; McWhae et al., 2015; Agroskin et al., 2016; Bukhori, 2017; Uhl et al., 2018; Reiss et al., 2019; Bizumic et al., 2022; Sheppard et al., 2023). The Ethnocentrism scale (Bizumic et al., 2009) comprises six bipolar subscales, with an equal number of positively-worded and negatively-worded items measuring each dimension. The Devotion subscale consists of positively worded items that measure strong dedication and loyalty to one's ethnic and cultural group, and negatively worded items measuring a lack of dedication or willingness to sacrifice for the group. The Group Cohesion subscale items measure the belief that one's own cultural or ethnic group should be highly integrated, cooperative, and unified (positively worded), and the belief that one's ethnic group should allow complete individual freedoms and individuality to its members (negatively worded). The Preference subscale items measure a tendency to prefer, like, and trust one's own ethnic group members over others (positively worded) in contrast to having no preference for one's own group over others (negatively worded). The Superiority subscale items measure a belief that one's ethnic group is unique and objectively better than any other group (positively worded) and a belief that one's group is not better than others (negatively worded). The Purity subscale items measure a desire to maintain the purity of one's ethnic group (positively worded) and opposition to mixing, living, and working with those from other groups (negatively worded). Finally, the Exploitativeness subscale items measure a belief that one's ethnic group and its interests should be placed first and above the interests of any other ethnic groups (positively worded), as opposed to the belief that one's group actions should be carried out with equal respect for other cultural or ethnic groups (negatively worded).
The overall scale, subscales, and its reduced versions have repeatedly performed well across cultures and languages (e.g., Bizumic et al., 2009; Agroskin, 2010; Greitemeyer, 2012; Harrison, 2012; Agroskin and Jonas, 2013; Cargile and Bolkan, 2013; Sirin et al., 2017; Valentino et al., 2018). Reflecting research in political science more broadly, all tests of the measure thus far have been based on classical test theory (CTT). Item response theory (IRT) provides many powerful capabilities beyond CTT, with the capacity to advance political science in novel ways. In this study, we use IRT to examine the psychometric properties of the Ethnocentrism scale in greater depth, employing more rigorous tests of performance than previously used with any ethnocentrism measure. In addition, this study investigates how political groups and gender identities respond differently to ethnocentrism statements, even when their level of latent ethnocentrism is the same. Due to the importance of IRT in advancing political science more broadly, we include an expanded discussion of IRT and the analytical steps involved. We focus on modeling appropriate for Likert scales because of their widespread usage in political science. Further, we provide information on an important extension of IRT, differential item functioning (DIF), which identifies how different groups respond to the same items. By highlighting recent advances in the field, we aim to motivate researchers to utilize IRT and DIF to advance political science research.
Political science has traditionally relied on classical test theory (CTT) for evaluating the psychometric properties of measures because CTT provides strong statistical tools based on a well-developed literary base spanning at least a century (Spearman, 1907, 1913; Gulliksen, 1950). CTT is, however, limited in several important aspects. It assumes that all items contribute homogeneous information about the latent construct and that measurement is equally precise at all levels of the latent construct. This disregards an item's ability to provide more information about specific levels of that construct (e.g., more information about low rather than high levels of ethnocentrism). CTT also focuses on scale-level information with insufficient attention to item-level properties (Embretson, 1996; Embretson and Reise, 2000; Ackerman et al., 2012; Reise and Revicki, 2014).
Political scientists are often interested in understanding how items and scales perform across diverse settings and contexts but rely on simplistic estimates of latent constructs based on summing responses to related statements. This approach neglects the unique contributions of each item, by assuming that all statements measure the construct in the same way, and purports measurement error to be the same at all levels of the latent construct. These assumptions can mislead researchers about the accuracy of their findings. Estimates are also temperamental, fluctuating based on the number of scale items and the idiosyncratic properties of the sample under investigation (Embretson, 1996; Reise and Revicki, 2014). This creates a substantial issue, as political scientists are interested in unbiased comparisons of latent (intangible) constructs between independent samples and groups.
There is a growing necessity in political science research for an alternative approach that can address many of these concerns and embodies modern measurement theory and capabilities (Embretson, 1996; Morizot et al., 2009). IRT (Lord and Novick, 1968) provides a useful framework for understanding the breadth of research (e.g., Fraley et al., 2000; Ackerman et al., 2012; Monaghan et al., 2020), addresses many of the criticisms of CTT, and expands the range of potential research questions. IRT has growing usage in social and political science (e.g., Carpini and Keeter, 1993; Treier and Jackman, 2008; Sibley and Houkamau, 2013; Fariss, 2014) and provides the additional capacity to investigate whether individuals in different groups truly vary in their level of ethnocentrism or whether group differences are due to how different populations interpret and value the different survey statements (Embretson, 1996; Reise and Revicki, 2014).
For each item, IRT models the probability of a response being endorsed at each point along the latent construct (θ), given specific item characteristics (Embretson and Reise, 2000; Reise and Revicki, 2014). Along the latent construct, measurement precision (a function of variance) is not treated as constant, instead varying along the latent construct. By modeling the underlying latent construct, individuals within and between samples can be meaningfully compared without comparing them to sample norms. This is also called the sample-independent properties of the IRT model. Barriers to more widespread IRT adoption likely result from the lack of general IRT training in academic institutions, the requirement to run computer-based algorithms to calculate estimates instead of simply summing responses, and the slightly higher technical knowledge required to understand logistic functions and model fitting approaches that are not widely utilized in CTT. Despite these potential barriers, IRT is accessible to all political science researchers.
IRT has been widely applied in education (e.g., Chen et al., 2005), organizational research (e.g., Scherbaum et al., 2006), and is increasingly being applied in psychology (Embretson, 1996; Monaghan et al., 2020; Bizumic et al., 2021; Sivanathan et al., 2021). For example, researchers are using IRT to develop and evaluate more precise and valid scales (Cooke et al., 1999; Edelen and Reeve, 2007; Cooper and Petrides, 2010; Furr, 2011; Ackerman et al., 2012) and to optimally shorten existing ones (Rauthmann, 2013). IRT is also proliferating in social and political science and it helps researchers develop more accurate measures of political constructs such as science curiosity (Kahan et al., 2017) and political knowledge (Carpini and Keeter, 1993), and identify the measurement properties and calibration of existing measures such as Maori Identity and Cultural Engagement (Sibley and Houkamau, 2013). IRT has also been used to model socio-political developments such as the role of changing accountability standards in the identification of political repression (Fariss, 2014) and to better exploit large existing datasets in cross-national investigations of democracy (Treier and Jackman, 2008).
Psychological researchers are also beginning to adopt extensions of IRT, such as utilizing DIF (discussed below) to identify similarities between men and women in their political opinions, reasoning, and labels (Condon and Wichowsky, 2015). In response to the pressure to reduce the length of longer surveys due to costs and potential non-response rates, psychologists have also used computerized adaptive IRT testing to dynamically shorten public opinion and knowledge surveys without reducing measurement precision (Montgomery and Cutler, 2013). Clearly, there is an appetite for IRT in psychological research, and we hope that increased awareness of its benefits will help strengthen research practices.
Given that IRT models have not been widely adopted or understood by many political science researchers, a brief background is presented here. Early IRT models such as one parameter logistic (1PL; from the Thurstone-Lazarsfeld-Lord-Birnbaum tradition) and Rasch models have been applied to model dichotomous data (two possible responses, such as yes/no or correct/incorrect) (Thissen and Steinberg, 2009). The 1PL estimates a location parameter (β; often referred to as item difficulty in dichotomous models) that indicates which level of the latent construct (θ) a participant would require to have a 50% chance of endorsing the item (e.g., giving the correct answer in an ability test). For example, in estimating spelling ability (θ), easier words (e.g., “bee”) will have lower location parameters than harder words (e.g., “phlegm”) because they require less spelling ability to answer correctly (Figure 1A).
Figure 1. Four primary IRT curves. Curves were created using Psych package for R (Revelle, 2014). (A) 2PL item characteristic curves (ICC) that differ only in their threshold/difficulty parameter (β). (B) Item characteristic curves (ICC) that only differ in their slope parameter (α; β = 0). Discrimination labels based on Baker (2001) criteria. (C) Item information curves (IIC) curve, where items 1 and 2 differ in information only, whereas item 3 differs in information and location. (D) GRM category response curve (CRC) for one item with five-categories (disagree strongly to agree strongly). Each curve represents the probability of a response category being endorsed. T = Example threshold parameters, which can vary in both location and spacing based on response information curves. Probability = P(response = 1 ∣ θ).
The 2PL model extends the 1PL by including a slope parameter (α; often referred to as item information or item discrimination), which estimates each item's ability to differentiate individuals on the latent construct. In dichotomous models, the slope parameter represents the information each item provides about the latent construct and is conceptually similar to factor loadings or item-total correlations in CTT. For example, the spelling ability questions would be better at discriminating participants based on, and therefore provide more information about, their spelling ability than a question about their hair color (Figure 1B). Spelling questions that are more informative are those that are consistently answered correctly by better spellers and incorrectly by poorer spellers. Less informative items are those that are answered correctly by some poorer spellers and incorrectly by some better spellers.
IRT provides several important figures that communicate discrimination and information clearly. The item characteristic curve (ICC; Figures 1A, B) models the logistic relationship between the probability of endorsing a response and the underlying latent construct (in logits, which are often interpreted similarly to Z-score units). From the ICC, the second essential curve is then calculated, the item information curve (IIC; Figure 1C), which models the information each item provides at every location on the latent construct. In Figure 1C, item 2 provides more information about the middle of the latent construct than item 1, item 3 provides more information than items 1 and 2 about higher levels of the latent construct, despite providing less than item 2 overall. IICs can be added together to create the information curve for the entire scale. In contrast to a single standard error of estimate (SE) in CTT, IRT estimates a different SE for each level of the latent construct based on the reciprocal of information; the more we know about a location on the latent construct, the smaller the SE.
CTT's measurement of social and political constructs assumes that measurement precision is constant across the latent construct and for all people, which is often represented with an estimate of internal consistency such as Cronbach's alpha. If the scale's location parameters are not equally distributed along the latent construct, then a single error term is misleading and can result in erroneous conclusions. For example, if the scale provides more accurate estimates at either the high or low end of the latent construct, then the scale might offer poor estimates of the general population. Therefore, you may have two scales with equivalent alpha coefficients, yet they could differ considerably in their capacity to measure ethnocentrism in the general population. To inverse this issue, researchers using IRT can exploit this fact by specifically selecting items that provide useful information in precisely the location they require or to understand how a particular statement is perceived by participants or groups (i.e., where the location parameter sits).
Although standard 1 or 2PL models can be used to investigate dichotomous data, IRT models have been extended to account for polytomous responses (more than two response options), which are frequent in political and social science (e.g., a 7-point Likert scale). Various polytomous IRT models have been developed to account for different types of responses (e.g., ordered vs. unordered nominal response categories). The partial credit model (PCM) (Masters, 1982) awards partial credit for each correct response within a single item and is thus well suited for the assessment of proficiency (for example, awarding partial points to a student for correctly completing each step in working through a mathematics or reasoning problem). Although the PCM assumes each item discriminates equally among participants (1PL), the generalized PCM (GPCM) (Muraki, 1992) relaxes this assumption (2PL). IRT models such as the nominal response model (Bock, 1972) do not assume that response categories have an inherent order, making them well suited for data such as multiple-choice examination style questions, or when responses have no pre-specified order (e.g., “yes”, “no”, “maybe”, “unsure”; “at home”, “at work”, “when traveling”). The capacity to accurately model nominal data is a further strength of IRT over CTT, as summing nominal responses to create a total score is meaningless (De Ayala, 2013).
Likert-type data is commonly used in political science research for self-report measurement and is best analyzed using Samejima's graded response model (GRM) (Samejima, 1969, 1997) due to its underlying assumptions and ease of use (Ackerman et al., 2012). The traditional GRM is a unidimensional 2PL that treats scale response categories as a set of ordinal dichotomies. For example, with a four-category Likert scale (ranging from 0–3), the GRM would have three thresholds: Threshold 1 (β≥1) = 0 vs. 1, 2, 3; Threshold 2 (β≥2) = 0, 1 vs. 2, 3; Threshold 3 (β≥3) = 0, 1, 2 vs. 3. The GRM location parameters represent the level of the latent construct where a person has a 50% probability of responding in any higher category compared to any category lower than the threshold (Figure 1D). The spacing between thresholds is allowed to vary, and higher response categories are assumed to represent a higher level of the latent construct. This matches conceptually with many political science constructs such as ethnocentrism.
Despite some variation in the names used for the GRM equivalent of the standard IRT curves (DeMars, 2010), the category response curve (CRC; akin to the IIC) and its scale-level equivalent are the most widely used curves for interpreting GRM. The CRC models the probability of selecting each response category given the latent construct (Figure 1D). Unlike dichotomous models, where information parameters can be compared to external standards, GRM information parameters (slopes) are compared to other items within the same model. For example, an item with a slope parameter of 3 would be as informative (provide the same precision) as four items with slope parameters of 1.5 (32/1.52 = 4).
IRT is a large-sample technique (Tay et al., 2014). Hulin et al. (1982), Reise and Yu (1990), and Tsutakawa and Johnson (1990) recommend at least 500 participants for accurate parameter estimates in the vast majority of cases. Accurate parameter estimates for polytomous items may be achieved for data with more than 250 participants; however, this is dependent upon the number of response options and the fit of the data to the model (Morizot et al., 2009). We encourage researchers to maximize the sample size before endorsing the IRT.
The two essential assumptions of IRT are unidimensionality and local independence (Embretson and Reise, 2000). As estimations of the latent construct become biased by additional dimensions or subgroups (e.g., ethnic, gender, or age groups), the covariance among the items must be due to a single underlying dimension, the latent construct of interest. Unidimensionality is often assessed by exploratory factor analysis (EFA) and confirmatory factor analysis (CFA) (Tay et al., 2014). Although pure unidimensionality is often unrealistic, if not impossible, given the nature of psychological constructs, the latent dimension must explain most of the variance in the data (Morizot et al., 2009).
IRT requires that subsets of items are not biased by external factors, and that any two items should not be predicted by a common factor beyond the underlying construct all items measure. This ensures that the items are locally independent. If some items are worded similarly but not shared by other items, these similar items can associate beyond the common construct, leading to bias in parameter estimates (Yen, 1993; Chen et al., 1997; Morizot et al., 2009). This bias is called local dependence (LD), which can be assessed using statistical tests such as Pearson's chi-square, the likelihood ratio (Chen et al., 1997), and Yen (1984) Q3, or via residual covariances in CFA. Unfortunately, LD is common in psychological scales and often overlooked (Embretson and Reise, 2000).
There is some variation in the approach that researchers take to evaluating model fit (Edelen and Reeve, 2007; Tay et al., 2014). Although model fit is not always reported in psychological studies on IRT, it is good practice to evaluate all levels of fit (Embretson and Reise, 2000). Model fit can be thought of at the model level, the item level, and the person level. At the model level, the M2 statistics and variants (Maydeu-Olivares and Joe, 2006; Cai and Hansen, 2013; Cai and Monroe, 2014) can be used to establish how well the IRT model as a whole reproduces observations in the data. Among a wide range of standardized statistics, S-X2 is a strong measure of item-level fit under the GRM (Orlando and Thissen, 2000, 2003), but it is also often useful to complement this statistic by visually inspecting the fit between each item's response function and the data. Person-level fit, or the degree to which participant responses fit the model, can be assessed using the Zh statistic (Drasgow et al., 1985). When running GRM models, sample size, unidimensionality, LD, and all levels of model fit should be assessed to indicate model validity.
One of the strengths of IRT is the ability to assess whether groups (e.g., based on gender, ethnicity, or ideology) respond to items or scales differently given equivalent levels of the latent construct, known as DIF. IRT can provide a picture of item-level measurement invariance that is difficult to replicate under CTT. This is because IRT focuses on latent variables (instead of manifest variables), and item parameters are sample-invariant under linear transformations (an approximation can be made by comparing item intercepts within a multigroup CFA while constraining factor loadings to be equal). The ability to detect and reduce bias has facilitated the shift from CTT to IRT in educational settings (Smith and Reise, 1998).
Expanding upon our previous examples, a native Australian participant (known as the reference or baseline group) is more likely to correctly spell a word native to Australia than a Chinese participant with the same latent spelling ability (the comparison or focal group). Focusing on the number of correct responses would be misleading when trying to compare spelling abilities. Substantial DIF suggests that the item performs differently across subgroups (Smith and Reise, 1998; Morizot et al., 2009; Shou et al., 2018) and that raw score differences between groups may be biased. DIF can also be completed at the scale-level of analysis (differential test functioning; DTF) and used to investigate responses between subgroups for whole scales. This is also beneficial because DIF within a scale in opposing directions can cancel out at the scale level (Chalmers et al., 2016).
Comparisons between groups require the same underlying metric. This is achieved by constraining one or more non-DIF items to be equivalent (the common scale for θ), known as anchor items. There are several methods for identifying anchor items (Kopf et al., 2015), and selecting an anchor item that has significant DIF may artificially inflate other DIF parameters (known as contamination) (Wang and Yeh, 2003). If there is a theoretical reason for one or more items to be equivalent across groups, then these items can be the anchor. An empirical approach is to first run a DIF analysis on all items without an anchor, assuming all items to be invariant. Items with non-significant overall, information, and threshold DIF are strong candidates for anchors. The DIF/DTF analysis is then re-run with the anchor items and equivalent metrics for θ, estimating all items freely in regard to that anchor (SD and M for the focal group latent construct are set to the previously estimated values).
DTF/DIF provides the ability to investigate an array of interesting new research questions and overcome many methodological flaws that currently exist. For example, scales can be developed that provide unbiased estimates across different social and political groups so that changes in test scores reflect true differences in the underlying construct or to identify appropriate measurement corrections for existing scales. Although the differences in item parameters are themselves an interesting area of investigation, IRT can be used to understand how readily groups endorse statements or actions, or how informative topics are of each group's identity or position.
This study aimed to advance contemporary conceptualizations of ethnocentrism and its measurement by conducting a technically robust IRT analysis of the Ethnocentrism scale (Bizumic et al., 2009). Importantly, we emphasize the steps involved and the benefits of IRT to motivate researchers to utilize these techniques in their own research, specifically for Likert-scale data given its ubiquity in political psychological research. First, IRT identified the information and location for each scale item and the subscales as a whole. In doing so, we provide novel information about how different aspects of ethnocentrism map onto the underlying construct. Second, we investigated whether there were any measurement differences on the Ethnocentrism scale due to gender and political ideology using DIF and DTF. Males, on average, tend to obtain higher scores on the Ethnocentrism scale than females, and conservatives tend to obtain higher scores on the Ethnocentrism scale than liberals (Bizumic et al., 2009). Through DTF, we aimed to determine whether these group differences are the result of measurement artifacts or true differences in ethnocentrism.
Participants (N = 4,227) completed on-line measures of ethnocentrism and demographics at http://www.YourMorals.org, a data collection and feedback website for a range of psychological constructs. This study was exempt from ethics approval because it utilized an existing dataset. As IRT estimates response level information and the sample size was large, we set a high item completion rate for inclusion in the study, removing the data from 40 participants who did not complete at least 90% of the Ethnocentrism scale items. The final sample consisted of 4,187 participants (2,590 men) who had a mean age of 54.87 (SD = 15.81). Of these participants, 2,192 described themselves as politically liberal, 355 as moderate, and 598 as conservative (1,042 described themselves as other or did not respond).
Ethnocentrism was measured by the 36-item Ethnocentrism scale (Bizumic, 2019). This scale contains three positively- and three negatively-worded items for each of the six subscales. Intragroup ethnocentrism was measured by the Devotion (α = 0.87) and Group Cohesion (α = 0.81) subscales. Intergroup ethnocentrism was measured by the Preference (α = 0.89), Superiority (α = 0.89), Purity (α = 0.90), and Exploitativeness (α = 0.90) subscales. Respondents were asked to indicate how much they agreed with items on a 9-point Likert scale ranging from Very Strongly Disagree (1) to Very Strongly Agree (9).
We used both CTT and IRT approaches to provide a comprehensive understanding of the psychometric properties of the Ethnocentrism scale. We used Lavaan (Rosseel, 2012), a package for R (version 3.5.1), to conduct CFA on the Ethnocentrism scale. We decided to use CFA to provide initial evidence on the unidimensionality of each subscale and LD assumptions because it provides a more stringent test than EFA. We assessed unidimensionality, LD, and person-fit before fitting unidimensional 2PL GRMs using expectation maximization to each subscale. To achieve this we used the “mirt” package (Chalmers, 2012) for R and IRTPRO (Cai et al., 2011). Model fit was conducted using the C2 variant of M2 statistic developed for ordinal data (Cai and Monroe, 2014). Item fit was conducted using the S-X2 item-fit statistic. We then expanded these models to investigate DIF and DTF associated with groups related to gender and political ideology.
In line with the qualitative labels endorsed by Ackerman et al. (2012), we will refer to the underlying latent continuum for each subscale (θ) as: well below average (θ < −2.0 logits), below average (−2.0 logits < θ < −1.0 logits), average (−1.0 logits < θ < 1.0 logits), above average (1.0 logits < θ < 2.0 logits), and well above average (θ > 2.0 logits).
We employed widely recommended cleaning and screening protocols (Tabachnick and Fidell, 2007; Enders, 2010) at the item-level of analysis. Missing data (there was <1% missing data from any item) were missing completely at random. We imputed the missing data using ordinal multiple imputation and retained the fifth dataset. The sample substantially exceeded 500 participants per response, allowing for stable IRT parameter estimates and acceptable standard errors. Further, the power to reject bad models (RMSEA = 0.08) and accept good models (RMSEA = 0.06; MacCallum et al., 1996) was excellent for the proposed CFA (βpower > 0.99).
We evaluated the Ethnocentrism scales' six-factor structure using weighted least squares means and variance adjusted estimation. Each ethnocentrism item loaded onto its respective first-order factor (representing the six basic dimensions), which in turn loaded onto its respective second-order factor (representing intergroup and intragroup dimensions). This model has been shown to fit numerous data sets well and to be superior to other plausible hierarchical models in the past (Bizumic et al., 2009). To remove the influence of method variance due to the direction of item wording, we estimated a latent construct that loaded onto all negatively-worded items. The ability of the model to reproduce the observations in the data was assessed using widely accepted fit indices (Kline, 2011): Non-Normed Fit Index (NNFI) and Comparative Fit Index (CFI) >0.90 indicating the model is likely specified adequately (Bentler and Bonett, 1980; Bentler, 1992), RMSEA <0.06 (Browne and Cudeck, 1992) and SRMR values <0.08 (Hu and Bentler, 1999) suggesting the model is acceptably close to the “perfect” model. We de-emphasized the Chi-squared statistic (χ2) as its accuracy may decline in large samples (see Kline, 2011). The model fitted the data well, χ2(569) = 7,771.93, p < 0.01, CFI = 0.922, NNFI = 0.914, SRMR = 0.059, RMSEA = 0.055, CI95% = [0.054–0.056]. These results suggest that the factor structure of the Ethnocentrism scale is reproducible and provide initial evidence for the IRT assumptions.
We continued to test the IRT assumptions for each subscale. The original Scree test (Cattell, 1966) and non-graphical interpretations (Raîche et al., 2013) supported a clear single factor for each subscale (the ratios of the first and second eigenvalues were all above 3.44). A single component, principal components analysis (PCA), accounted for approximately 62% of the variance in the data, with all item loadings exceeding 0.65. When considering the PCA results (internal consistencies alone are misleading indicators of unidimensionality), internal consistencies and average inter-item correlations were further suggestive of a single dimension underlying each subscale (see Supplementary material 1A for item statistics and correlations).
We ran an initial GRM (using the expected a-posteriori estimation method) for each subscale, constrained one to two non-DIF items for the subscale as anchors, and examined person-fit statistics using Zh fit statistics (Drasgow et al., 1985). Data from participants with Zh values <-2.00 were considered unlikely response patterns (Embretson and Reise, 2000) and were removed from the subscales measuring devotion (N = 66, 1.5%), cohesion (N = 127, 3%), preference (N = 204, 4.87%), superiority (N = 70, 1.67%), purity (N = 117, 2.79%), and exploitativeness (N = 116, 2.77%). The standardized LD statistic (G2) (Chen et al., 1997) based on the likelihood ratio statistics (interpreted similarly to χ2), needs to be considered in relation to the number of response categories, items, and sample size (Christensen et al., 2017). We compared Q3 estimates to the average Q3 values for each subscale (see Supplementary material 1A for LD statistics), which suggested that there were several item pairs with mildly elevated LD. As a result, these items were monitored for signs of artificially elevated parameter estimates.
We then tested model fit by comparing a parsimonious version of the GRM that estimates a single slope parameter for each item with a full GRM that estimates unique slopes (C2 interpreted in line with χ2). The full GRM for each subscale fitted the data significantly better than the parsimonious version: devotion, = 526.81, p < 0.001; group cohesion, = 877.68, p < 0.001; preference, = 1,121.39, p < 0.001; superiority = 280.83, p < 0.001; purity, = 553.51, p < 0.001; and exploitativeness, = 371.38, p < 0.001. Therefore, we proceeded with full GRM models given their superior fit.
The Ethnocentrism scale captured all six domains with strong coverage across the latent continua, principally differentiating responses within the average to high level of latent construct ranges. Item and test information curves for all subscales are displayed in the left panel of Figure 2 (item parameters and SE are in Supplementary material 1B). All items captured a similar range on the latent construct, providing the most information from the below average to above average range (θ ≈ −1.5 to 2 logits). Compared to the other subscales, the Superiority and Purity Subscales contained items that captured more of the above-average range than the other subscales (θ ≈ 2 to 3 logits).
Figure 2. Subscale IRT figures; item (left) and scale (right) information curves as functions of their respective latent constructs. Exploit, Exploitativeness.
Items differed in the type of information they provided about ethnocentrism (the left panels of Figure 2), capturing either high information about a narrow range, or less information about a wider range of the latent construct. For example, items 6 (devotion) and 35 (exploitativeness) captured more range at the positive end of their respective subscales, having relatively weaker associations with the latent construct compared to the other items in their subscales. On the other hand, item 30 provided the most information about the above average range of the Purity subscale, whereas items 2 and 3 provided the most information about the devotion latent construct, and items 10 and 12 about the cohesion latent construct. These findings suggest that, at the item level, most items tended to discriminate well between the participants at most levels of the latent construct, but that they did not discriminate well at very low levels of the latent construct—possibly because the items are relatively strongly worded and might be rejected by both those who are very low and those who are extremely low on the latent construct. Accordingly, the measure would benefit from including more items that do discriminate better between these individuals, such as item 14.
At the subscale level of analysis (the right panels of Figure 2), all subscales captured information in a similar range on the latent construct, from the below average to the above average range (θ ≈ −1 to 2.5 logits, and approximately θ ≈ −0.50 to 2.5 logits for the Superiority and Purity Subscales). Therefore, the Ethnocentrism scale is most precise within this range which corresponds to the smallest standard errors. All scales showed a rapid increase in SE in the well below average range (θ <−2 logits), implying that measurement errors increased quickly. The standard error for each point on the latent constructs can be seen in Figure 2. Although SE, therefore reliability, should be conceptualized as varying at each point on the latent construct, we also estimated the overall marginal reliability (integration using the prior density function), which was excellent for all subscales: Devotion = 0.88, Cohesion = 0.87, Preference = 0.92, Superiority = 0.88, Purity = 0.88, and Exploitativeness = 0.89.
We subsequently ran DIF to determine the effect of gender and political ideology on participants' responses to the scales. We compared item parameters between the subgroups using Wald tests (χ2) to identify whether DIF occurred and the source of that variance—either threshold or information parameters (p < 0.001, adjusted for multiple comparisons). DIF statistics are reported in Supplementary material 1C. The item with the least DIF in each subscale was used as the short anchor, and we investigated DTF by comparing scale information curves between subgroups (Figures 3, 4).
Figure 3. DTF figures for the effect of gender identity on each subscale. Scale information curves (left) and expected values (right) DTF figures are provided. Expected DTF figures plot the total raw score (y-axis) for each level of the respective underlying latent construct (x-axis) by gender identity.
Figure 4. DTF figures for the effect of political ideology on each subscale. Scale information curves (left) and expected values (right) DTF figures are provided. Expected DTF figures plot the total raw score (y-axis) for each level of the respective underlying latent construct (x-axis) by political ideology. Exploit, Exploitativeness.
We first investigated whether individuals who identify as men or women responded differently on each subscale given equivalent levels of the latent construct (DTF; Figure 3). The subscales provided more information (the left panel of Figure 3), thus differentiating respondents better for men than women in the average range (θ ≈ −1.0 to 2.0 logits). On the other hand, these subscales provided marginally more information about women than men in the well above average range (θ ≈ 2.0 to 4.0 logits). The right panel of Figure 3 displays the relationship between raw subscale scores (y-axis) and the underlying latent construct (θ; x-axis). For the same level of latent construct in the average to above average range (θ ≈ 0.0 to 3.0 logits), men were also more likely to obtain marginally higher scores than women. DTF was most prominent on the Superiority and Purity Subscales.
DTF for political ideology—differentiating conservatives from liberals—suggested that all subscales, besides the Devotion subscale, provided more information for conservatives than for liberals in the low average (θ ≈ −2) to above average (θ ≈ 2) range. Although the Devotion Subscale provided more information about conservatives in the low average range (θ ≈ −2), it provided more information about liberals in the above average range (θ ≈ −2). This resulted in similar amounts of information about both political ideologies overall (Figure 4 left panel). Thresholds for liberals were consistently higher than for conservatives, indicating that liberals required less of the latent construct to endorse response categories. The right panels of Figure 4 demonstrate that this effect was strongest in the average to well above average range. However, the size of this difference varied between subscales, with the smallest effect seen for the Preference subscale. Overall, the results suggest that, given the same level of the underlying construct, response styles across the Ethnocentrism scale differ as a function of gender and political ideology.
This study utilized IRT techniques to expand our understanding of ethnocentrism, the Ethnocentrism scale's psychometrics (Bizumic et al., 2009), and provide a framework for political scientists to follow to conduct GRM-based IRT analyses in future research. Below, we discuss how IRT provides a contemporary understanding of ethnocentrism measurement and ongoing considerations and advancements for IRT in political science.
An initial CFA supported the original factor structure proposed by Bizumic et al. (2009), suggesting the shortened 36-item scale's structure is replicable and appropriate for subsequent analyses in similar populations. IRT assumptions of unidimensionality were satisfied, although due to the small number of items, LD was identified between several items, most notably in the Superiority and Group Cohesion subscales. Unfortunately, the nature of psychological scales, comprising numerous closely related and overlapping items, inevitably results in LD (Embretson and Reise, 2000). IRT is relatively robust to mild-moderate LD, yet researchers should be mindful of possible parameter inflations.
We evaluated the six ethnocentrism subscales using unidimensional IRT models. Items within the six subscales varied substantially in their IRT parameters, with several items having relatively flat information curves. As a result, one may be tempted to shorten these scales, particularly in the Group Cohesion and Preference subscales. When selecting items for scale shortening using IRT, researchers should consider both the location and the amount of information that items provide, because relatively flat information curves may still capture areas of the latent construct neglected by the other items. For example, the items “It is absolutely vital that all true members of my ethnic or cultural group forget their differences and strive for greater unity and cohesion” (item 8) and “I would probably be quite content living in a cultural or ethnic group that is very different to mine” (item 18) had relatively flat curves but still provide information about those who are very low on ethnocentrism—in fact, more than the items that have more peaked curves.
Overall, the information curves suggested that the subscales provide the most information about the below average to above average range (θ ≈ −1.0 to 2.5 logits), with higher information curves for the intergroup ethnocentrism subscales. Future researchers refining this measure could consider more items that would differentiate those who are generally low on the latent ethnocentrism construct, increasing measurement precision (reducing the standard error) in this range.
Researchers interested in developing a psychometrically robust scale using IRT can employ information curves to select items that provide comparable reliability across the desired range of theta (construct of interest). For instance, when developing an abbreviated version of the Ethnocentrism scale, items could be selected from each subscale that provide the most information in the middle of the latent trait. Table 1 presents a six-item abbreviated version of the Ethnocentrism scale, which incorporates an item from each subscale that provides the most information in the middle of each latent trait, as displayed in Figure 2 (these are items 2, 10, 15, 24, 30, and 31). This abbreviated version can be used by researchers to investigate ethnocentrism with only six items and concentrate on the general range of ethnocentrism. On the other hand, if researchers are specifically interested in items measuring higher levels of ethnocentrism, when developing an abbreviated version of the scale, they may consider items that offer more information at the higher end of the theta range, such as item 6 for devotion, item 27 for purity, and item 35 for exploitativeness. These items, however, provide less information in the general range but achieve greater measurement precision above approximately 3 logits. In this way, researchers can maximize measurement precision within the desired range. Applying information curves and the item selection criteria detailed here can aid in the development of more reliable and valid measures of socio-political constructs.
DTF analyses suggested that men required marginally less of more socially unacceptable latent constructs (superiority, purity, and exploitativeness) to endorse scale items. Therefore, men more readily endorsed items such as “We need to do what's best for our own people and stop worrying so much about what the effect might be on other peoples” and “Our culture would be much better off if we could keep people from different cultures out”. This effect was not present for more socially acceptable and passive subscales which emphasize ingroup unity and preference (devotion, group cohesion, and preference). One explanation for this effect is that men are, on average, higher in the personality construct of antagonism (inverse of agreeableness; Weisberg et al., 2011). In conjunction with dominant and aggressive actions being more socially acceptable in men, a man might be more willing and able, on average, to express more socially unacceptable ethnocentric ideas, such as rejection or exploitation of ethnic outgroups, when compared to a woman with the same level of ethnocentrism. The raw scale scores the latent construct similarly between genders, suggesting only a minimal adjustment is necessary to achieve measurement equivalence.
Conservatives more readily endorsed all ethnocentrism subscales than liberals, and therefore, required lower levels of the ethnocentrism latent construct to endorse ethnocentric statements. The effect was smallest for the Group Cohesion and Preference subscales, with more substantial effects on the other subscales (Figure 4). It is likely that among liberals, it is less socially desirable to express relatively strong ethnocentric statements. Opposing ethnocentrism might further act to signal your group membership to others who identify as liberals. Ethnocentric viewpoints, however, may align more closely with some conservative values and, as a result, be more readily endorsed to express ingroup membership given the same strength of personal ethnocentrism (e.g., “We need to do what's best for our own people, and stop worrying so much about what the effect might be on other peoples” and “It is better for people from different ethnic and cultural groups not to marry.”).
The differences in raw means between liberals and conservatives need to be adjusted to account for the differences in responding, as not doing so would bias any conclusions drawn from the analyses. To quantify this difference, the largest DIF was seen for the Devotion subscale. Liberals would score substantially lower (with a raw score of approximately 15) despite being as devoted to their ethnic group as conservatives (with an equivalent raw score of approximately 22; Figure 4). Ongoing social and political psychological research should also investigate whether there might be similar DTF between ethnic, national, or cultural groups. This finding demonstrates one of the strengths of IRT and emphasizes that differences between conservatives and liberals must take into account how ideological differences influence responses to psychological instruments.
Psychological research and theory focus largely on understanding latent constructs. Claims about any construct or theory are rendered meaningless if based upon poor measurement practices because any conclusions drawn from the data will either be biased or invalid. Therefore, strong measurement practices are axiomatic to developing valid social or political theory. Unfortunately, poor measurement practices often underpin psychological research, a practice that likely formed a key factor in the psychological replication crisis. For example, Flake et al. (2017) reviewed a sample of articles from the Journal of Personality and Social Psychology (JPSP), a leading psychology journal, and found that most of the sampled articles reported poor psychometric practices. For example, the authors of the articles reported only face validity and Cronbach's alpha as evidence of the scale's validity. One half of the articles used measures for which there was no previously published psychometric evidence. Given the pervasiveness and cost of poor measurement practices, IRT provides much needed robust psychometric tools upon which stronger social and political science theory can be built.
When investigating the results from the current study, LD indicated possible parameter inflation in two of the subscales, which is likely a result of the length of each subscale. This is not uncommon given the nature of psychological research, and potential solutions include creating testlets (although this is troublesome with non-binary data; Embretson and Reise, 2000) or using Multiple-Chain Monte Carlo (MCMC) estimation. The Bayesian MCMC approach can be used to estimate an additional parameter to account for the LD, resulting in parameter correction (Bradlow et al., 1999). The best approach is to reduce the possibility of LD occurring in the first place through careful item selection, monitoring of possible testing factors (such as fatigue), and administering a large item pool to allow for the final selection of non-LD items.
Further, our interpretation of DTF was based on analyzing the size of the effect on the DTF plots. Our approach could be complemented with a quantification of effect size, which, unlike in CTT, is often difficult to estimate in IRT. Recently, Meade (2010), Chalmers et al. (2016), and Chalmers (2022) proposed two methods for quantifying the size of the DTF between the subgroups. The signed DTF estimates the systematic bias resulting from one subgroup scoring higher consistently across θ, thus providing the overall curve differences on θ. The unsigned DTF estimates overall separation at a particular θ, reporting the average area between the curves to represent variations in DTF along the latent construct. Given the utility of these effect estimates and ongoing research into establishing benchmarks and qualitative labels, further studies should consider implementing and validating these effect sizes when they become more widely available and implemented.
The 9-point Likert scale resulted in a thin distribution of scores across response categories, reducing the number of data points in extreme categories (1 and 9) and therefore reducing the accuracy of parameter estimates. One method is to collapse the two most extreme categories (i.e., merging 1 with 2, and 8 with 9) and compare item descriptive statistics and internal consistencies for equivalence. Further, the optimum number of response categories can be identified by measuring item separation (indexed measurement) by person separation (Piquero et al., 2002). From an IRT perspective, each response category should become the most probable response category at a location on the latent construct continuum. If this does not occur, this category can be considered unnecessary and removed. Despite this, wider Likert scales are often recommended for socio-political attitudes given the capacity for individuals to accurately express themselves. For example, Altemeyer (1998) implemented a 9-point scale for his Right-Wing Authoritarianism scale because it tended to produce a more reliable scale than those with fewer response options. Future researchers, however, may need to re-evaluate this recommendation considering our findings. Nevertheless, more research is needed before we can be clear on these ideas related to socio-political attitudes, such as ethnocentrism and authoritarianism.
IRT provides interesting, nuanced, and precise modeling of participant responses that complements the existing array of multivariate techniques, which are ubiquitous within political science. For example, IRT allows for the accurate calibration of the relationship between items and latent constructs, whereas latent structural modeling identifies the broader structure of constructs and the relationship between them. IRT can also be seamlessly used to extract precise estimates of each participant's latent construct, which can then be fed into standard multivariate models such as multiple regression, moderation, and mediation. Additionally, stronger assessment measures can be developed to provide better differentiation, more targeted assessment (e.g., information curves in the center for the general population or at the extremes for advantaged or disadvantaged populations), and less biased estimates. IRT can also allow social and political science researchers to ask new and exciting questions of the data. Researchers might be interested in the endorsement of specific content, such as the strength of a particular social or political ideology that is required to endorse or reject a belief or behavior (difficulty/intercept parameter). Alternatively, IRT can provide more contextually sensitive or fine-grained information regarding how individuals or groups vary in the expression of their beliefs despite having the same level of the underlying latent construct.
A range of considerations need to be taken into account once DIF has been identified. Inevitably, we will find DIF if we look closely or compare enough subgroups. DIF itself is not necessarily troublesome because it often cancels out at the scale level or has a minimal influence on estimates. If substantial DTF is identified, then researchers will most commonly remove DIF items from the item pool when enough non-DIF items remain to accurately capture the construct. If there are not enough non-DIF items remaining, then other approaches include: (a) splitting the measure to create different versions of the scale (e.g., a politically-left and a politically-right version of the scale); (b) equating the groups using anchor items; or (c) introducing different scoring rubrics for each group (e.g., different weightings or an item score adjustment). Nevertheless, these approaches still require the classification of individuals into groups, which will then define their scoring metric. This is troublesome for participants whose identity or response profile does not neatly match their assigned group (Smith and Reise, 1998).
Although we introduced the basic procedure of IRT and DIF tests, there are a range of advanced techniques and extensions that could enhance social and political research. For example, under the Bayesian IRT framework, researchers could incorporate prior research findings when specifying the IRT model and the differences between groups. This approach would allow the accumulation of evidence for research questions of interest. In addition, although the maximum likelihood method only allows for the detection of the difference between groups, Bayesian approaches allow researchers to accumulate evidence for the null hypothesis, that is, that there is no difference between groups. Readers who are interested in more advanced Bayesian IRT techniques can consult texts specific to this purpose (Fox, 2010).
An important extension to standard IRT techniques is multidimensional IRT, which models θ for several constructs at the same time. This has many advantages, such as relaxing the assumption of unidimensionality, which may reduce some of the difficulties seen with LD, and can address questions on the nature of the construct when managing subscales through compensatory vs. non-compensatory models. Although beyond the scope of the current guide, there are several strong texts on multidimensional IRT extensions (Reckase, 2009). These extensions also include increasing measurement efficiency through adaptive testing, where items are sequentially administered to each participant based on their previous response. Items are selected to specifically target the participant's estimated construct with increasing precision until a minimum acceptable error rate is reached (Chalmers, 2016; Monaghan and Bizumic, 2023).
An additional IRT extension can be used for unipolar latent continuums. Political ideology at the individual level of analysis can be seen as bipolar in that both ends of the continuum are meaningful, representing either strong “left” or “right” wing views. On the other hand, political oppression at the societal level of analysis might be conceptualized as unipolar if we are estimating either the presence or absence of oppression. A range of alternative IRT models are being developed to handle non-normal latent continuums, such as when the latent continuum is skewed (Woods, 2006; Wall et al., 2015) and for unipolar constructs (Lucke, 2015). This is a promising area of development, given that many social and political constructs could be conceptualized as unipolar; however, this work is still in its infancy with ongoing research into how to evaluate model fit, ensure the accuracy of parameters (where future Bayesian approaches might also be beneficial), and utilize these models for DIF (Reise et al., 2018).
A modern approach to psychometric analysis will continue to strengthen psychological research, overcoming many psychometric issues that currently exist and opening new and exciting research directions currently limited by a reliance on classical testing frameworks. We demonstrated the strengths of this approach through a guided analysis of the Ethnocentrism scale, highlighting that the scale was more precise between the below average and above average ranges (θ ≈ −1 to 2.5 logits) on the latent construct continuums. Further, DTF showed that subscale scores differ between political ideologies and genders for the same level of the latent construct. This article highlights the dangers of relying solely on CTT analysis and outlines the many benefits provided through IRT for future psychological research.
The data analyzed in this study is subject to the following licenses/restrictions: This data is not owned by the authors of the article, they are owned by www.yourmorals.org. As a result, the data is not available to be hosted publicly. Requests to access these datasets should be directed to www.yourmorals.org.
I confirm that the contents of the manuscript are consistent with the APA ethical principles. The dataset was collected by www.yourmorals.org, which was approved by the Institutional Review Board at the University of Southern California. All participants provided informed consent surrounding engaging in the research and with the distribution of the results through publication. The patients/participants provided their written informed consent to participate in this study.
CM contributed by initiating and designing the study and was the primary researcher involved in writing and analyzing the data. BB contributed to the identification and sharing of the data, to reviewing versions of the manuscript, and to the ethnocentrism theory aspects of the manuscript. All authors contributed to the study's conception and design, read, and approved the final manuscript.
We would like to thank Dr. Ravi Iyer at the University of Southern California for providing the data for the current study. We gratefully acknowledge Dr. Yiyun Shou for her guidance and expertise in IRT, which have been invaluable to this work.
The authors declare that the research was conducted in the absence of any commercial or financial relationships that could be construed as a potential conflict of interest.
All claims expressed in this article are solely those of the authors and do not necessarily represent those of their affiliated organizations, or those of the publisher, the editors and the reviewers. Any product that may be evaluated in this article, or claim that may be made by its manufacturer, is not guaranteed or endorsed by the publisher.
The Supplementary Material for this article can be found online at: https://www.frontiersin.org/articles/10.3389/fpos.2023.1024729/full#supplementary-material
Ackerman, R. A., Donnellan, M. B., and Robins, R. W. (2012). An item response theory analysis of the Narcissistic Personality Inventory. J Pers Assess. 94, 141–155. doi: 10.1080/00223891.2011.645934
Adorno, T. W., Frenkel-Brunswik, E., Levinson, D. J., and Sanford, R. N. (1950). The Authoritarian Personality. New York, NY: Harper.
Agroskin, D. (2010). Out of control: How and why does perceived lack of control lead to ethnocentrism? Rev. Psychol. 17, 79–90. Available online at: https://psycnet.apa.org/record/2010-26179-001
Agroskin, D., and Jonas, E. (2013). Controlling death by defending ingroups—Mediational insights into terror management and control restoration. J. Exp. Soc. Psychol. 49, 1144–1158. doi: 10.1016/j.jesp.2013.05.014
Agroskin, D., Jonas, E., Klackl, J., and Prentice, M. (2016). Inhibition underlies the effect of high need for closure on cultural closed-mindedness under mortality salience. Front. Psychol. 7, 1583. doi: 10.3389/fpsyg.2016.01583
Altemeyer, B. (1998). The other “authoritarian personality.” Adv. Exp. Soc. Psychol. 30, 47–92. doi: 10.1016/S0065-2601(08)60382-2
Ammassari, S., Fossati, D., and McDonnell, D. (2022). “Supporters of India's BJP: Distinctly Populist and Nativist,” in Government Oppos. 1–17. doi: 10.1017/gov.2022.18
Anderson, C. C. (2016). Ethnocentrism in Russia and Ukraine. Doctor of Philosophy. University of Iowa.
Bankert, A., Powers, R., and Sheagley, G. (2022). Trade politics at the checkout lane: Ethnocentrism and consumer preferences. Polit. Sci. Res. Methods. 11, 605–612. doi: 10.1017/psrm.2022.40
Bentler, P. M. (1992). On the fit of models to covariances and methodology to the Bulletin. Psychol. Bull. 112, 400–404. doi: 10.1037/0033-2909.112.3.400
Bentler, P. M., and Bonett, D. G. (1980). Significance tests and goodness of fit in the analysis of covariance structures. Psychol. Bull. 88, 588–606. doi: 10.1037/0033-2909.88.3.588
Beswick, D. G., and Hills, M. D. (1969). An Australian ethnocentrism scale. Aust. J. Psychol. 21, 211–225. doi: 10.1080/00049536908257791
Bizumic, B. (2019). Ethnocentrism: Integrated Perspectives. 1st edition. Abingdon, UK: Routledge. doi: 10.4324/9781315642970-1
Bizumic, B., and Duckitt, J. (2012). What is and is not ethnocentrism? A conceptual analysis and political implications. Polit. Psychol. 33, 887–909. doi: 10.1111/j.1467-9221.2012.00907.x
Bizumic, B., Duckitt, J., Popadic, D., Dru, V., and Krauss, S. A. (2009). cross-cultural investigation into a reconceptualization of ethnocentrism. Eur. J. Soc. Psychol. 39, 871–899. doi: 10.1002/ejsp.589
Bizumic, B., Gunningham, B., and Christensen, B. K. (2022). Prejudice towards people with mental illness, schizophrenia, and depression among mental health professionals and the general population. Psychiat. Res. 317, 114817. doi: 10.1016/j.psychres.2022.114817
Bizumic, B., Monaghan, C., and Priest, D. (2021). The return of ethnocentrism. Polit. Psychol. 42, 29–73. doi: 10.1111/pops.12710
Bock, R. D. (1972). Estimating item parameters and latent ability when responses are scored in two or more nominal categories. Psychometrika. 37, 29–51. doi: 10.1007/BF02291411
Bradlow, E. T., Wainer, H., and Wang, X. A. (1999). Bayesian random effects model for testlets. Psychometrika. 64, 153–168. doi: 10.1007/BF02294533
Browne, M. W., and Cudeck, R. (1992). Alternative ways of assessing model fit. Sociol. Methods Res. 21, 230–258. doi: 10.1177/0049124192021002005
Bukhori, B. (2017). Educational environment, ethnocentrism, and prejudice towards Indonesian Chinese. ANIMA Indones. Psychol. J. 32, 109–115. doi: 10.24123/aipj.v32i2.589
Cai, L., Du Toit, S. H. C., and Thissen, D. (2011). IRTPRO: Flexible, multidimensional, multiple categorical IRT modeling [Computer software]. Chic IL Sci Softw Int.
Cai, L., and Hansen, M. (2013). Limited-information goodness-of-fit testing of hierarchical item factor models. Br. J. Math. Stat. Psychol. 66, 245–276. doi: 10.1111/j.2044-8317.2012.02050.x
Cai, L., and Monroe, S. A. (2014). New statistic for evaluating item response theory models for ordinal data. CRESST Report 839. National Center for Research on Evaluation, Standards, and Student Testing (CRESST).
Cargile, A. C., and Bolkan, S. (2013). Mitigating inter-and intra-group ethnocentrism: Comparing the effects of culture knowledge, exposure, and uncertainty intolerance. Int. J. Intercult. Relat. 37, 345–353. doi: 10.1016/j.ijintrel.2012.12.002
Carpini, M. X. D., and Keeter, S. (1993). Measuring political knowledge: Putting first things first. Am. J. Polit. Sci. 37:1179–1206. doi: 10.2307/2111549
Cattell, R. B. (1966). The Scree test for the number of factors. Multivar. Behav. Res. 1, 245–276. doi: 10.1207/s15327906mbr0102_10
Chalmers, R. P. (2012). MIRT: A multidimensional item response theory package for the R environment. J. Stat. Softw. 48, 1–29. doi: 10.18637/jss.v048.i06
Chalmers, R. P. (2016). Generating adaptive and non-adaptive test interfaces for multidimensional item response theory applications. J. Stat. Softw. 71, 1–38. doi: 10.18637/jss.v071.i05
Chalmers, R. P. (2022). A unified comparison of IRT-based effect sizes for DIF investigations. J. Educ. Meas. 60, 318–350. doi: 10.1111/jedm.12347
Chalmers, R. P., Counsell, A., and Flora, D. B. (2016). It might not make a big DIF: Improved differential test functioning statistics that account for sampling variability. Educ. Psychol. Meas. 76, 114–140. doi: 10.1177/0013164415584576
Chen, C. M., Lee, H. M., and Chen, Y. H. (2005). Personalized e-learning system using item response theory. Comput. Educ. 44, 237–255. doi: 10.1016/j.compedu.2004.01.006
Chen, W.- H., and Thissen, D. (1997). Local dependence indexes for item pairs using item response theory. J. Educ. Behav. Stat. 22, 265–289. doi: 10.3102/10769986022003265
Christensen, K. B., Makransky, G., and Horton, M. (2017). Critical values for Yen's Q3: Identification of local dependence in the Rasch model using residual correlations. Appl. Psychol. Meas. 41, 178–194. doi: 10.1177/0146621616677520
Condon, M., and Wichowsky, A. (2015). Same blueprint, different bricks: Reexamining the sources of the gender gap in political ideology. Polit. Groups Ident. 3, 4–20. doi: 10.1080/21565503.2014.992793
Cooke, D. J., Michie, C., Hart, S. D., and Hare, R. D. (1999). Evaluating the screening version of the Hare Psychopathy Checklist—Revised (PCL: SV): An item response theory analysis. Psychol. Assess. 11, 3–13. doi: 10.1037/1040-3590.11.1.3
Cooper, A., and Petrides, K. V. A. (2010). Psychometric analysis of the trait emotional intelligence questionnaire–short form (TEIQue–SF) using item response theory. J. Pers. Assess. 92, 449–457. doi: 10.1080/00223891.2010.497426
De Ayala, R. J. (2013). The Theory and Practice of Item Response Theory. New York: Guilford Publications.
DeMars, C. (2010). Item Response Theory. New York: USA: Oxford University Press. doi: 10.1093/acprof:oso/9780195377033.001.0001
Drasgow, F., Levine, M. V., and Williams, E. A. (1985). Appropriateness measurement with polychotomous item response models and standardized indices. Br. J. Math. Stat. Psychol. 38, 67–86. doi: 10.1111/j.2044-8317.1985.tb00817.x
Edelen, M. O., and Reeve, B. B. (2007). Applying item response theory (IRT) modeling to questionnaire development, evaluation, and refinement. Qual. Life Res. 16, 5–18. doi: 10.1007/s11136-007-9198-0
Embretson, S. E. (1996). The new rules of measurement. Psychol. Assess. 8, 341–349. doi: 10.1037/1040-3590.8.4.341
Embretson, S. E., and Reise, S. P. (2000). Item Response Theory for Psychologists. Mahwah, NJ: Lawrence Erlbaun Associates.
Fariss, C. J. (2014). Respect for human rights has improved over time: Modeling the changing standard of accountability. Am. Polit. Sci. Rev. 108, 297–318. doi: 10.1017/S0003055414000070
Flake, J. K., Pek, J., and Hehman, E. (2017). Construct validation in social and personality research: Current practice and recommendations. Soc. Psychol. Personal Sci. 8, 370–378. doi: 10.1177/1948550617693063
Fox, J. P. (2010). Bayesian Item Response Modeling: Theory and Applications. Berlin: Springer Science and Business Media. doi: 10.1007/978-1-4419-0742-4
Fraley, R. C., Waller, N. G., and Brennan, K. A. (2000). An item response theory analysis of self-report measures of adult attachment. J. Pers. Soc. Psychol. 78, 350. doi: 10.1037/0022-3514.78.2.350
Furr, M. (2011). Scale Construction and Psychometrics for Social and Personality Psychology. London, UK: SAGE Publications Ltd. doi: 10.4135/9781446287866
Greitemeyer, T. (2012). Boosting one's social identity: Effects of social exclusion on ethnocentrism. Basic Appl. Soc. Psychol. 34, 410–416. doi: 10.1080/01973533.2012.712013
Gulliksen, H. (1950). Theory of Mental Tests. New York, NY: John Wiley and Sons. doi: 10.1037/13240-000
Hainmueller, J., and Hopkins, D. J. (2015). The hidden American immigration consensus: A conjoint analysis of attitudes toward immigrants. Am. J. Polit. Sci. 59, 529–548. doi: 10.1111/ajps.12138
Hansen, C. N., and Dinesen, P. T. (2022). Terrorism activates ethnocentrism to explain greater willingness to sacrifice civil liberties: evidence from Germany. Polit. Sci. Res. Methods. 2022, 1–8. doi: 10.1017/psrm.2022.5
Harrison, N. (2012). Investigating the impact of personality and early life experiences on intercultural interaction in internationalised universities. Int. J. Intercult. Relat. 36, 224–237. doi: 10.1016/j.ijintrel.2011.03.007
Hu, L., and Bentler, P. M. (1999). Cutoff criteria for fit indexes in covariance structure analysis: Conventional criteria versus new alternatives. Struct. Equ. Model Multidiscip. J. 6, 1–55. doi: 10.1080/10705519909540118
Hulin, C. L., Lissak, R. I., and Drasgow, F. (1982). Recovery of two- and three-parameter logistic item characteristic curves: a monte carlo study. Appl. Psychol. Meas. 6, 249–260. doi: 10.1177/014662168200600301
Huxley, E., Bizumic, B., and Kenny, A. (2015). “The role of ethnocentrism in the relationship between openness to experience and ethnic prejudice,” in Ethnic and cultural identity: Perceptions, discrimination and social challenges 85–101.
Jost, J. T., and Thompson, E. P. (2000). Group-based dominance and opposition to equality as independent predictors of self-esteem, ethnocentrism, and social policy attitudes among African Americans and European Americans. J. Exp. Soc. Psychol. 36, 209–232. doi: 10.1006/jesp.1999.1403
Kahan, D. M., Landrum, A., Carpenter, K., Helft, L., and Hall Jamieson, K. (2017). Science curiosity and political information processing. Polit. Psychol. 38, 179–199. doi: 10.1111/pops.12396
Kam, C. D., and Kinder, D. R. (2007). Terror and ethnocentrism: Foundations of American support for the war on terrorism. J. Polit. 69, 320–338. doi: 10.1111/j.1468-2508.2007.00534.x
Kam, C. D., and Kinder, D. R. (2012). Ethnocentrism as a short-term force in the 2008. American presidential election. Am. J. Polit. Sci. 56, 326–340. doi: 10.1111/j.1540-5907.2011.00564.x
Kinder, R., and Kam, C. D. (2009). Us Versus Them: Ethnocentric Foundations of American Public Opinion. University of Chicago Press.
Kline, R. B. (2011). Principles and Practice of Structural Equation Modeling. New York, NY: Guilford Press.
Kopf, J., Zeileis, A., and Strobl, C. (2015). Anchor selection strategies for DIF analysis: review, assessment, and new approaches. Educ. Psychol. Meas. 75, 22–56. doi: 10.1177/0013164414529792
Lord, F. M., and Novick, M. R. (1968). Statistical Theories of Mental Test Scores. Charlotte, NC: Information Age Publishing.
Lucke, J. R. (2015). “Unipolar item response models,” in Handbook of item response theory modeling: Applications to Typical Performance Assessment (New York, NY, US: Routledge/Taylor and Francis Group) 272–84.
MacCallum, R. C., Browne, M. W., and Sugawara, H. M. (1996). Power analysis and determination of sample size for covariance structure modeling. Psychol. Methods. 1, 130–149. doi: 10.1037/1082-989X.1.2.130
Mansfield, E. D., and Mutz, D. C. (2009). Support for free trade: Self-interest, sociotropic politics, and out-group anxiety. Int. Organ. 63, 425–457. doi: 10.1017/S0020818309090158
Mansfield, E. D., Mutz, D. C., and Brackbill, D. (2019). Effects of the Great Recession on American attitudes toward trade. Br. J. Polit. Sci. 49, 37–58. doi: 10.1017/S0007123416000405
Masters, G. N. A. (1982). Rasch model for partial credit scoring. Psychometrika. 47, 149–174. doi: 10.1007/BF02296272
Maydeu-Olivares, A., and Joe, H. (2006). Limited information goodness-of-fit testing in multidimensional contingency tables. Psychometrika. 71, 713–732. doi: 10.1007/s11336-005-1295-9
McWhae, L. E., Paradies, Y., and Pedersen, A. (2015). Bystander antiracism on behalf of Muslim Australians: The role of ethnocentrism and conformity. Aust. Commun. Psychol. 27, 6–20.
Meade, A. W. (2010). A taxonomy of effect size measuRes. for the differential functioning of items and scales. J. Appl. Psychol. 95, 728–743. doi: 10.1037/a0018966
Monaghan, C., and Bizumic, B. (2023). Dimensional models of personality disorders: Challenges and opportunities. Front. Psychiatry. 14, 1098452. doi: 10.3389/fpsyt.2023.1098452
Monaghan, C., Bizumic, B., Williams, T., and Sellbom, M. (2020). Two-dimensional Machiavellianism: Conceptualization, theory, and measurement of the views and tactics dimensions. Psychol. Assess. 32, 277–293. doi: 10.1037/pas0000784
Montgomery, J. M., and Cutler, J. (2013). Computerized adaptive testing for public opinion surveys. Polit. Anal. 21, 172–192. doi: 10.1093/pan/mps060
Morizot, J., Ainsworth, A. T., and Reise, S. P. (2009). “Towards modern psychometrics,” in Handbook of Research Methods in Personality Psychology, eds. R. W., Robins, R. C., Fraley, R. F., Krueger (New York, NY: The Guilford Press).
Muraki, E. (1992). A generalized partial credit model: Application of an EM algorithm. ETS Res. Rep. Ser. 16, 159–176. doi: 10.1177/014662169201600206
Mutz, D. C. (2021). Winners and losers: The psychology of foreign trade. Princeton University Press. doi: 10.1515/9780691203041
Mutz, D. C., and Lee, A. H.-Y.. (2020). How much is one American worth? How competition affects trade preferences. Am. Polit. Sci. Rev. 114, 1179–1194. doi: 10.1017/S0003055420000623
Neuliep, J. W., and McCroskey, J. C. (1997). The development of a US and generalized ethnocentrism scale. Commun. Res. Rep. 14, 385–398. doi: 10.1080/08824099709388682
Orey, B. D., and Park, H. (2012). Nature, nurture, and ethnocentrism in the Minnesota Twin Study. Twin Res. Hum. Genet. 15, 71–73. doi: 10.1375/twin.15.1.71
Orlando, M., and Thissen, D. (2000). Likelihood-based item-fit indices for dichotomous item response theory models. Appl. Psychol. Meas. 24, 50–64. doi: 10.1177/01466216000241003
Orlando, M., and Thissen, D. (2003). Further investigation of the performance of S-X2: An item fit index for use with dichotomous item response theory models. Appl. Psychol. Meas. 27, 289–298. doi: 10.1177/0146621603027004004
Pérez, E. O. (2015). Xenophobic rhetoric and its political effects on immigrants and their co-ethnics. Am. J. Polit. Sci. 59, 549–564. doi: 10.1111/ajps.12131
Piquero, A. R., Macintosh, R., and Hickman, M. (2002). The validity of a self-reported delinquency scale comparisons across gender, age, race, and place of residence. Sociol. Methods Res. 30, 492–529. doi: 10.1177/0049124102030004002
Raîche, G., Walls, T. A., Magis, D., Riopel, M., and Blais, J. G. (2013). Non-graphical solutions for Cattell's scree test. Methodol. Eur. J. Res. Methods Behav. Soc. Sci. 9, 23. doi: 10.1027/1614-2241/a000051
Rauthmann, J. F. (2013). Investigating the MACH–IV with item response theory and proposing the trimmed MACH*. J. Pers. Assess. 95, 388–397. doi: 10.1080/00223891.2012.742905
Reckase, M. D. (2009). Multidimensional Item Response Theory. New York: Springer. 79–112. doi: 10.1007/978-0-387-89976-3
Reise, S. P., and Revicki, D. A. (2014). Handbook of Item Response Theory Modeling: Applications to Typical Performance Assessment. Oxford, UK: Routledge. doi: 10.4324/9781315736013
Reise, S. P., Rodriguez, A., Spritzer, K. L., and Hays, R. D. (2018). Alternative approaches to addressing non-normal distributions in the application of IRT models to personality measures. J. Pers. Assess. 100, 363–374. doi: 10.1080/00223891.2017.1381969
Reise, S. P., and Yu, J. (1990). Parameter recovery in the graded response model using MULTILOG. J. Educ. Meas. 27, 133–144. doi: 10.1111/j.1745-3984.1990.tb00738.x
Reiss, S., Klackl, J., Proulx, T., and Jonas, E. (2019). Strength of socio-political attitudes moderates electrophysiological responses to perceptual anomalies. PLoS ONE. 14, e0220732. doi: 10.1371/journal.pone.0220732
Revelle, W. (2014). Psych: ProceduRes. for psychological, psychometric, and personality research. Northwest University Evanst Ill. 165.
Robinson, A. L. (2020). Ethnic diversity, segregation and ethnocentric trust in Africa. Br. J. Polit. Sci. 50, 217–239. doi: 10.1017/S0007123417000540
Rosseel, Y. (2012). LAVAAN: An R package for structural equation modeling. J. Stat. Softw. 48, 1–36. doi: 10.18637/jss.v048.i02
Samejima, F. (1969). Estimation of latent ability using a response pattern of graded scores. Psychom. Monogr. Suppl. 34. 1–97. doi: 10.1007/BF03372160
Samejima, F. (1997). “Graded response model,” in Handbook of Modern Item Response Theory, eds. W. J., Van der Linden, R. K., Hambleton (New York, NY: Springer) 85–100. doi: 10.1007/978-1-4757-2691-6_5
Scherbaum, C. A., Finlinson, S., Barden, K., and Tamanini, K. (2006). Applications of item response theory to measurement issues in leadership research. Leadersh. Q. 17, 366–386. doi: 10.1016/j.leaqua.2006.04.005
Sheppard, H., Bizumic, B., and Calear, A. (2023). Prejudice toward people with borderline personality disorder: Application of the prejudice toward people with mental illness framework. Int. J. Soc. Psychiat. doi: 10.1177/00207640231155056. [Epub ahead of print].
Shou, Y., Sellbom, M., and Xu, J. (2018). Psychometric properties of the Triarchic Psychopathy Measure: An item response theory approach. Personal. Disord. Theory Res. Treat. 9, 217. doi: 10.1037/per0000241
Sibley, C. G., and Houkamau, C. A. (2013). The multi-dimensional model of Māori identity and cultural engagement: Item response theory analysis of scale properties. Cultur. Divers Ethnic. Minor. Psychol. 19, 97. doi: 10.1037/a0031113
Sides, J., and Gross, K. (2013). Stereotypes of muslims and support for the war on terror. J. Polit. 75, 583–598. doi: 10.1017/S0022381613000388
Sirin, C. V., Valentino, N. A., and Villalobos, J. D. (2017). The social causes and political consequences of group empathy. Polit. Psychol. 38, 427–448. doi: 10.1111/pops.12352
Sivanathan, D., Bizumic, B., and Monaghan, C. (2021). The unified narcissism scale: moving towards an integrated measure of narcissism. Personal Sci. 2, 1–24. doi: 10.5964/ps.7417
Smith, L. L., and Reise, S. P. (1998). Gender differences on negative affectivity: an IRT study of differential item functioning on the Multidimensional Personality Questionnaire Stress Reaction Scale. J. Pers. Soc. Psychol. 75, 1350–1362. doi: 10.1037/0022-3514.75.5.1350
Spearman, C. (1907). Demonstration of formulae for true measurement of correlation. Am. J. Psychol. 18, 161–169. doi: 10.2307/1412408
Spearman, C. (1913). Correlations of sums or differences. Br. J. Psychol. 5, 417–426. doi: 10.1111/j.2044-8295.1913.tb00072.x
Tabachnick, B. G., and Fidell, L. S. (2007). Using Multivariate Statistics. Fifth. Boston, USA: Pearson Education Inc.
Tay, L., Meade, A. W., and Cao, M. (2014). An overview and practical guide to IRT measurement equivalence analysis. Organ Res. Methods. 18, 3–46. doi: 10.1177/1094428114553062
Thissen, D., and Steinberg, L. (2009). “Item Response Theory,” in The SAGE Handbook of Quantitative Methods in Psychology (London, UK: SAGE Publications Ltd.) 148–77. doi: 10.4135/9780857020994.n7
Treier, S., and Jackman, S. (2008). Democracy as a latent variable. Am. J. Polit. Sci. 52, 201–217. doi: 10.1111/j.1540-5907.2007.00308.x
Tsutakawa, R. K., and Johnson, J. C. (1990). The effect of uncertainty of item parameter estimation on ability estimates. Psychometrika. 55, 371–390. doi: 10.1007/BF02295293
Uhl, I., Klackl, J., Hansen, N., and Jonas, E. (2018). Undesirable effects of threatening climate change information: A cross-cultural study. Group Process Intergroup Relat. 21, 513–529. doi: 10.1177/1368430217735577
Valentino, N. A., Brader, T., and Jardina, A. E. (2013). Immigration opposition among U.S. whites: general ethnocentrism or media priming of attitudes about latinos? Polit. Psychol. 34, 149–166. doi: 10.1111/j.1467-9221.2012.00928.x
Valentino, N. A., Wayne, C., and Oceno, M. (2018). Mobilizing sexism: The interaction of emotion and gender attitudes in the 2016. US presidential election. Public Opin. Q. 82, 799–821. doi: 10.1093/poq/nfy003
Wall, M. M., Park, J. Y., and Moustaki, I. I. R. T. (2015). modeling in the presence of zero-inflation with application to psychiatric disorder severity. Appl. Psychol. Meas. 39, 583–597. doi: 10.1177/0146621615588184
Wang, W. C., and Yeh, Y. L. (2003). Effects of anchor item methods on differential item functioning detection with the likelihood ratio test. Appl. Psychol. Meas. 27, 479–498. doi: 10.1177/0146621603259902
Warr, P. B., Faust, J., and Harrison, G. J. A. (1967). British ethnocentrism scale. Br. J. Soc. Clin. Psychol. 6, 267–277. doi: 10.1111/j.2044-8260.1967.tb00529.x
Weisberg, Y. J., DeYoung, C. G., and Hirsh, J. B. (2011). Gender differences in personality across the ten aspects of the Big Five. Front. Psychol. 2, 178. doi: 10.3389/fpsyg.2011.00178
Woods, C. M. (2006). Ramsay-curve item response theory (RC-IRT) to detect and correct for nonnormal latent variables. Psychol. Methods. 11, 253–270. doi: 10.1037/1082-989X.11.3.253
Yen, W. M. (1984). Effects of local item dependence on the fit and equating performance of the three-parameter logistic model. Appl. Psychol. Meas. 8, 125–145. doi: 10.1177/014662168400800201
Keywords: ethnocentrism, Ethnocentrism scale, item response theory, confirmatory factor analysis, gender, political ideology
Citation: Monaghan C and Bizumic B (2023) Item response theory approach to ethnocentrism. Front. Polit. Sci. 5:1024729. doi: 10.3389/fpos.2023.1024729
Received: 22 August 2022; Accepted: 05 July 2023;
Published: 01 August 2023.
Edited by:
Nicola Maggini, University of Milan, ItalyReviewed by:
Hugo H. Rabbia, Universidad Nacional de Córdoba, ArgentinaCopyright © 2023 Monaghan and Bizumic. This is an open-access article distributed under the terms of the Creative Commons Attribution License (CC BY). The use, distribution or reproduction in other forums is permitted, provided the original author(s) and the copyright owner(s) are credited and that the original publication in this journal is cited, in accordance with accepted academic practice. No use, distribution or reproduction is permitted which does not comply with these terms.
*Correspondence: Conal Monaghan, Y29uYWwubW9uYWdoYW5AYW51LmVkdS5hdQ==
Disclaimer: All claims expressed in this article are solely those of the authors and do not necessarily represent those of their affiliated organizations, or those of the publisher, the editors and the reviewers. Any product that may be evaluated in this article or claim that may be made by its manufacturer is not guaranteed or endorsed by the publisher.
Research integrity at Frontiers
Learn more about the work of our research integrity team to safeguard the quality of each article we publish.