- 1School of Communication and Media, Kennesaw State University, Kennesaw, GA, United States
- 2School of Government and International Affairs, Kennesaw State University, Kennesaw, GA, United States
- 3College of Communication, Media and Information, University of Colorado, Boulder, CO, United States
How are attitudes formed in the 21st Century, and who sets the agenda for initial COVID-19 coverage in the United States? We explore these questions using a random sample of 6 million tweets from a population of 224 million tweets collected between January 2020 and June 2020. In conjunction with a content analysis of legacy media such as newspapers, we examine the second-level agendamelding process during the onset of the COVID-19 pandemic in the United States. The findings demonstrate that in the early weeks of the pandemic, public opinion on Twitter about the virus was distinctly different than the coverage of the issue in the traditional media. The attributes used to describe it on social media demonstrate users relying on their past experiences and personal beliefs to talk about the virus. In the 1st week of February, public opinion, traditional media, and social media converged, but traditional media soon becomes the main agenda setter of COVID-19 for 13 weeks. However, for the final 5 weeks of our sample, traditional media are taken over by social media. The findings also show that, except for a few weeks at the onset of the outbreak, Twitter users relied on their personal experiences far less than what statistical models predicted and allowed. Instead, traditional media and social media to shape their opinion of the issue.
1. Introduction
How are attitudes formed in the 21st Century, and who set the agenda for initial COVID-19 coverage in the United States? We explore these questions using a random sample of 6 million tweets from a population of 224 million collected between January 2020 and June 2020 and a content analysis of legacy media such as newspapers. We examined the second-level agendamelding process during the onset of the COVID-19 pandemic in the United States.
Severe acute respiratory syndrome coronavirus 2 (SARS-CoV-2), commonly referred to as COVID-19, was first identified in December 2019 and quickly spread across the world to create, arguably, the first pandemic of the 21st Century. As COVID-19 spread worldwide, so did misinformation about the virus, its severity, associated symptoms, safety guidelines, and even the vaccines developed to fight it (Puri et al., 2020). In response to the pandemic, scholars have explored the role of media and social media in this public health crisis from agenda-setting and framing perspectives (Miller et al., 2021; Palm et al., 2021), the magnitude of spread (Kouzy et al., 2020), susceptibility of audiences and the effects of misinformation (Kim et al., 2020; Roozenbeek et al., 2020), the reasons behind acceptance of misinformation (Gollust et al., 2020), as well as analyses of media content about the virus (Muddiman et al., 2020). We add to this literature using a second-level “agendamelding” approach.
Our findings suggest that in the early weeks of the pandemic, public opinion about the virus was distinctly different on Twitter than the coverage of the issue in traditional media. The attributes used to describe it on social media demonstrate users relying on their past experiences and personal beliefs to talk about the virus. In the 1st week of February, public opinion, traditional media, and social media converged, but traditional media soon became the main agenda setter of COVID-19 for 13 weeks. However, for the final five weeks of our sample, social media overtake traditional media. The findings also show that, except for a few weeks at the onset of the outbreak, Twitter users relied on their personal experiences far less than what statistical models predicted and allowed traditional media and social media to shape their opinion of the issue.
2. Agenda setting and agendamelding
Agenda setting is how media organizations identify and promulgate the main issues and events for public consideration and discourse. The idea behind agenda setting can be traced back to political scientist (Cohen, 1963), who discovered that general knowledge of foreign affairs was closely related to foreign news available in newspapers; he stated that while media “may not be successful much of the time in telling people what to think, but it is stunningly successful in telling its readers what to think about” (p. 13).
McCombs and Shaw (1972) found empirical support for this idea by combining media content analysis, an audience survey, and the ranking of agendas; they called it the “agenda-setting function of the press.” Since its introduction, researchers have found support for the agenda-setting effect in more than 600 peer-reviewed studies (e.g., see Griffin et al., 2014; McCombs, 2014; Kim et al., 2017; Wanta, 2019).
New communication technologies such as email, online newspapers, chat rooms, social media, and websites representing every ideological, commercial, and personal niche changed how millions, or perhaps billions, of people from around the world communicate and opened new areas for research to communication scholars (McCombs, 2005). Chaffee and Metzger (2001) argue that the idea that “on the Internet anyone can be an author” has diminished the “mass-ness” of mass media, questioning whether the media continue to set the agenda. They argue that the diversification of sources, made possible by new communication technologies, has resulted in fragmented and competing media agendas that challenge the basic assumption of the agenda-setting theory, which is that people get their information from a uniform media agenda (Chaffee and Metzger, 2001).
Academics, however, have extended their inquiries across these online domains and have found that, similar to traditional media, online media can influence an issue's salience in audiences across the country (Guo and Vargo, 2017).
Historically, the traditional media have been credited for setting the agenda for online discussions (Roberts et al., 2002), but online media have an agenda-setting power. The salience of issues in blogs, tweets, and discussion boards is as likely to precede the traditional media coverage of those issues as to follow Russell Neuman et al. (2014). The independent agenda-setting power of online media have even been observed in comparing the print and online versions of the same media outlet (Althaus and Tewksbury, 2002). Recent studies also support the notion that traditional online media and social networking websites set the agenda for one another (Haim et al., 2018).
The notion that a second-level of agenda setting also happens when the “attributes” used to describe issues in the media transfer to audiences was first tested during the 1976 presidential primaries (Becker and McCombs, 1978). Support for agenda-setting level two (Ghanem, 1997; McCombs et al., 1997, 2000; Lopez-Escobar et al., 1998; Golan and Wanta, 2001) led McCombs and Shaw (1993) to posit that the media not only tell audiences “what to think about, but also how to think about it, and consequently, what to think” (p. 65).
McCombs et al. (2000) identified “substantive” attributes and “affective” attributes that contribute to the understanding of the agenda-setting effect. Affective attributes refer to the valence characteristics of an object (i.e., positive, neutral, or negative) that draw emotional responses from the audience (Kiousis et al., 1999, 2007; McCombs et al., 2000). Substantive attributes, on the other hand, refer to cognitive characteristics that describe an object (e.g., the age of a candidate or a candidate's connection to a former president) in a manner that helps structure the news and differentiate among various topics (Kiousis et al., 1999, 2007; McCombs et al., 2000).
Second-level agenda setting has long been associated with framing to such an extent that some even consider the terms attribute and frame interchangeable (Kiousis et al., 1999). Some communication scholars define framing as the act of “select[ing] some aspects of a perceived reality and mak[ing] them more salient in a communicating text, in such a way as to promote a particular problem definition, causal interpretation, moral evaluation, and/or treatment recommendation for the item described” (Entman, 1993, p. 52). Others have defined it as “the central organizing idea for news content that supplies a context and suggests what the issue is through the use of selection, emphasis, exclusion, and elaboration” (Tankard et al., 1991, p. 3). Scheufele and Tewksbury (2007) argue that the term framing refers to the assumption that how an issue is presented and galvanized by the media impacts how the audience perceives the issue.
Weaver (2007, p. 143) observed that even in a single issue of the Journal of Communication, authors employed a wide range of “definitions of framing, including problem definitions, causal interpretations, moral evaluations, and treatment recommendations, as well as key themes, phrases, and words.” Reese (2007) noted that many studies only have the term “framing” in common. “Authors often give an obligatory nod to the literature before proceeding to do whatever they were going to do in the first place” (Reese, 2007, p. 151). Weaver (2007) cites this chasm as the reason behind the proliferation of framing studies in recent history. Despite the similarities between framing and second-level agenda setting, they are not identical processes (Weaver, 2007). McCombs (1997) believes that conceptualizing frames as attributes and bringing framing under the umbrella of agenda setting “brings some order and parsimony to the vast literature on framing whose popularity led to highly diverse even incompatible—applications and definitions” (p. 6).
Though we focus on second-level agenda-setting and agendamelding, specifically, third-level agenda-setting warrants some discussion because it is closely related to second-level agenda-setting and a predecessor to agendamelding. The main idea behind third-level agenda setting is that each object is usually described in the media by more than one attribute. Moreover, objects are often frequently mentioned with a set of attributes, creating a linked network of objects and attributes. As a result, the salience of the networks of objects and attributes is transferred to audiences in a bundle (Guo et al., 2012).
Third-level agenda setting, also known as Network Agenda Setting (NAS), is based on the foundations of the associative network model of memory (Anderson and Bower, 1980; Anderson, 2016) as well as the cognitive network model (Santanen et al., 2000). NAS posits that the audience's cognitive representation of objects and attributes is akin to a network-like structure in which any given node (e.g., object or attribute) is connected to numerous other nodes (Guo et al., 2012).
In short, the NAS model asserts that issues can be either implicitly or explicitly linked in news coverage resulting in the construction of contextual meanings in the audience's mind (Vargo et al., 2014a). While first- and second-level agenda setting focus on discrete objects and attributes of a bigger picture, third-level agenda setting aims to paint the whole picture of reality constructed by the news media and individuals' cognitive maps using network analysis tools (Guo, 2012). Instead of examining the prominence of issues through frequency counts, the network agenda-setting model turns to the centrality of issues and the location of individual issue nodes in terms of how close they are to the center of a network (Vargo et al., 2014a). As a result, the unit of analysis in third-level agenda setting is a dyad—two issues or attributes mentioned together (Guo et al., 2012). The NAS model “hypothesizes that news media have the capability to construct the connections among agendas, thereby constructing the centrality of certain agenda elements in the public's mind” (Guo et al., 2012, p. 56).
As the evolution of the Agenda Setting theory continued, Shaw et al. (1999) proposed that traditional media are not unitary agenda setters; instead, the public agenda is set through an agendamelding process. Agendamelding is premised on the notion that public agenda—or issues that the public finds salient (McCombs and Shaw, 1972)—is the result of a melding process, whereby audiences mix traditional media agendas with social media agendas (including the agenda of those they interact with in person, or via mediated means) and their own personal agenda, which exists independent of (or despite) the media agenda (Minooie, 2019; Shaw et al., 2019; Bantimaroudis et al., 2020; McWhorter, 2020). By considering an individual's personal agenda—their predispositions toward certain issues, beliefs, and policies—an agendamelding approach allows for the study of “subcultural agendas” that “meld” with one another and create “ideological bonds among community members” (Bantimaroudis et al., 2020, p. 122).
The main proposition in Agendamelding—that different types of media (sometimes called “old” and “new”) interact with one another to form the audience's agenda—is an idea scholars have extensively explored since the 1960s (McLuhan, 1962; Schramm, 1963; Dance and Gerbner, 1967). This notion has received more attention recently with some scholars like Shaw et al. (1999) and Chadwick (2013) arguing that the interaction between media types is not always at the expense of one type of media but rather it is a process that results in a balance between various media types.
Chadwick (2013) argues that we are “in the middle of a chaotic transition period induced by the rise of digital media,” (p. 4) and while the undeniable rise of internet (a “new” medium by consensus) is significant, “just as significant is the fact that television has not declined” (p. 52). This means that audiences are consuming both types of media and melding the information they receive—which is the quintessential argument in agendamelding. Similarly, Gilardi et al. (2022) compared three different streams of agendas from different traditional and social media sources during the Swiss national elections and found that neither leads the other two in terms of setting the agenda more than it is led by them.
Shaw et al. (2019) offer a statistical approach—the “agenda community attraction” (ACA) to calculate the contribution of each source of agenda. The ACA calculates the contribution of the personal agenda to the public agenda, given the traditional media's agenda-setting correlation. The ACA makes it possible for researchers to measure the extent to which traditional sources of information like newspapers and television broadcasts contributed to opinion formation about COVID-19 in the United States and social media's contribution.
The Agenda Community Attraction formula (Shaw et al., 2019) is formally written as:
Where AS1 represents the agenda-setting correlation between traditional media agenda and the public agenda. 1–AS1 represents an estimation of the agenda-setting correlation between horizontal media agenda and the public agenda—which, when available, can be replaced by the actual observed correlation between horizontal media agenda and the public agenda (Shaw et al., 2019).
The formula includes the power of the correlation between traditional (or vertical) media (AS1) and the audience. The formula also recognizes that in most cases, social (or horizontal) media play a role in setting the agenda—which is the difference between AS1 and a perfect agenda-setting effect (or 1.00). Weaver et al. (2010) posited that the effect not accounted for by traditional (vertical) media— (AS1)2–or social (horizontal) media—[1–(AS1)2]—is the result of the individual's personal preferences—e.g., their judgments, voting history, and beliefs, which has been empirically tested in several studies (Minooie, 2021).
Thus, the outcome of the ACA formula is an estimate of the contribution of the personal agenda—or audiences' personal preferences, beliefs, and experiences—to the public agenda. Traditional (vertical) media are gate-kept sources of information that disseminate information to the public (e.g., newspapers, books, television newscasts, and social media posts by traditional media accounts). Social (horizontal) media are sources of information that may or may not be gate-kept but disseminate information to a particular target audience (or niche audience) rather than the public at large (although the information may be accessible by the public at large, it is intended for interested parties). This would include, for example, specialized or trade magazines, word-of-mouth, blogs, or social media posts by users who are not media professionals.
For example, if in a given context the traditional agenda-setting correlation is a relatively strong 0.85, then ACA = 1 – [(0.85)2 + (1–0.85) 2], or ACA = 0.255. If an independent measure of the social (horizonal) media's agenda setting correlation is available, it would take the place of (1–0.85). Figure 1 displays the relevant components of the ACA formula and how each contributes to the overall concept.
From a practical perspective, citizens' main concern while trying to get information and form their own opinions about any crisis—including COVID-19—is whose information can be trusted and how that information confirms or revises prior attitudes. This project helps fill this gap by adopting an agendamelding approach by studying social media content about the virus in conjunction with a content analysis of traditional media in the United States over 6 months starting from January 2020, shortly after the virus was first identified, through June 2020, when many states lifted their original stay-at-home orders.
Implementing this approach has several implications for the scientific community, public health communicators, media gatekeepers, and the public. In this study, we aim to identify the efficacy of the vertical (top-down) dissemination of information from authorities and public health experts through traditional media and compare that with the efficacy of the horizontal spread of information (i.e., the peer-to-peer dissemination of information on social media) at the beginning of a public health crisis with political implications. The findings can help guide communication and media professionals to allocate their resources better and place more emphasis on the dissemination means that are likely to have the most effect on the formation of the public agenda in future crises—which in turn, could result in a more cohesive response and better management of the crises.
2.1. Empirical expectations and questions
Based on the preceding literature review, we generate several testable hypotheses and empirical questions we need to explore to answer our primary research question. Given that agendamelding is born out of the agenda-setting line of research, our first hypothesis seeks to establish a second-level agenda-setting effect, or the notion that the attributes used by mass media to describe an issue prompt the public to describe those issues using the same attributes (Ghanem, 1997; McCombs et al., 1997; Lopez-Escobar et al., 1998). Therefore, instead of “the agenda,” we will use “the attribute agenda” to perform our analyses.
H1: There is a positive correlation between the attributes used by the mass media (e.g., traditional media) to describe COVID-19 and the attributes used by the public (e.g., tweets) to discuss the same issue.
The agenda community attraction formula requires a second correlation (in addition to the one hypothesized above) between the social media agenda and the public agenda. Therefore, we hypothesize:
H2: There is a positive correlation between the attributes used on social media to describe COVID-19 and the attributes used by the public to describe the same issue.
To take a holistic approach and evaluate the contribution of various sources of information to the public attribute agenda on COVID-19, we will address the following empirical questions:
EQ1: To what extent do traditional media, social media, and the personal preferences of individuals explain the variations in the public attribute agenda on COVID-19?
EQ2: How do public sentiments about COVID-19 change over time?
In other words, how do the three sources of information about COVID-19 impact each other and the public agenda over time?
And finally:
EQ3: Which source of information best explains the changes in public sentiment toward COVID-19?
3. Data and method
3.1. Traditional media sample
To get a sense of the agenda during the early days of the pandemic, we created constructed week samples of major newspapers and television network news programming between January 11, 2020, and June 28, 2020. Constructed week samples, which follow Riffe et al. (1996, 2014), control for sources of “systematic variation” in the issue coverage. Specifically, we sampled newspaper articles about the virus appearing in The New York Times, the Wall Street Journal, The Los Angeles Times, and the Washington Post. We chose these papers for our sample because they are in the top portion of newspapers in circulation in the United States and cover national and regionally specific audiences (e.g., L.A. Times for the west coast). For broadcast and television programming, we sampled transcripts of ABC World News Tonight, CBS Evening News, NBC Nightly News, and MSNBC's Rachel Maddow Show, Fox's Hannity, and CNN's Anderson Cooper 360. Like the newspaper sample, these selected shows give us the broadest set of media agendas transmitted via traditional means and cover both broadcast and cable news outlets. To generate our dataset, we constructed 1 week of each of the four papers and the five broadcast programs for each month in the study period (January 2020–June 2020).1
We employed three human coders to code for “attributes” or frames. Using a codebook,2 the coders trained to code the sample for the attributes used to describe the issue of COVID-19. To develop the universe of attributes coded in our analysis we follow Ogbodo et al. (2020), who use content analysis to identify a standard set of frames used in world news outlets during the onset of the pandemic. We use their attributes to observe the extent to which these frames are present in United States-based news outlets and to link these attributes to horizontal (i.e., social media posts by average users) information dissemination. The unit of analysis for newspaper articles is individual headlines and ledes. For the broadcast artifacts, we use propositional units (i.e., each paragraph of text for a segment on COVID-19) for coding, whereby each proposition in the program is considered entirely regardless of its length. After coding was complete, we removed the Maddow, Hannity, and Cooper items from our traditional media sample and included them in our social media sample as “heavily partisan talk shows that focus on the vertical media agendas but offer a different spin” represent horizontal media (Shaw et al., 2019, p. 63). The process resulted in a sample of close to eight thousand (n = 7,970) headlines, ledes, and propositions about COVID-19 in the 6 months of the study.
The human coders were trained to identify the presence of as many as 14 attributes selected through a review of the literature on the media coverage of COVID-19 in the sample (e.g., see Ogbodo et al., 2020). To ensure intercoder reliability, a subsample of 158 units3 was double-coded by all three coders. A Krippendorff's Alpha inter-coder reliability test indicated that the content was coded reliably (α = 0.782). See Appendix B for a list of intercoder reliability for each attribute.
3.2. Social media sample
To assess the extent to which and how people in the United States discussed the COVID-19 pandemic, we use a dataset of tweets from Twitter.com. Twitter, one of the world's most popular social media sites, is a valid tool for this kind of analysis because it allows individuals to engage with each other in real time without any traditional gatekeeping on information or credentials. Also worth noting, Twitter can be a valuable macro barometer for American public opinion and public sentiment (Vargo et al., 2014b; Karami et al., 2018) even if the user base for Twitter is not generally representative of the American public as a whole (Wojcik and Hughes, 2019). Crucially, we are trying to explain and assess how traditional and horizontal media communicate to and reflect each other; we want to evaluate and measure how these media engage with each other. Twitter is an ideal forum for data to capture this phenomenon.
Given the discrepancy in the naming of the virus in the early days of the pandemic, we created a list of 27 keywords through a preliminary content analysis of tweets and media coverage in the early days of the pandemic. The keywords ranged from the now-conventional “COVID,” “COVID19,” and “COVID-19,” to names like “Corona Virus,” “CoronaVirus,” “nCov,” and even xenophobic names like “China Virus,” and “Wuhan Virus,” that were used by some people, including the then-president of the United States, Donald J. Trump (Rahman, 2021). We developed the list of keywords in the early days of the pandemic. Gallagher et al. (2021) conducted a thorough search for the keywords to refer to the pandemic based on a complete dataset of COVID-19 tweets and identified a total of 570 keywords that capture the body of global tweets about the pandemic. The 27 keywords we used in our study are among the 570 used by Gallagher et al. (2021), but some keywords about the pandemic outside the United States (e.g., coronavirusindia, coronavirusitalia, etc.), keywords not in English, and keywords about developments that happened after we launched our study (e.g., “drive-through testing,” “phase 1 trial,” etc.) are missing from our list of keywords.
Using a Python script, we collected 224 million unique tweets containing at least one of the keywords occurring between January 2020 and June 2020. To ensure that only users' sentiments from the United States were captured, we extracted only tweets with a United States geotag to form the sampling population (N = 54 million), of which 6 million tweets (n = 6,161,735) were randomly sampled for analysis. We then separated the tweets posted by or mentioned traditional media accounts (n = 53,311) as they represent the information disseminated by vertical media, albeit on a social media platform. Retweets and tweets that mentioned other (but non-traditional) media accounts were also separated (n = 1,249,401) to represent the social (horizontal) media. The remaining tweets that did not mention another account (n = 4,859,023) represent the personal views and beliefs of the tweeter.
We built and trained several supervised machine learning models using the coded traditional media sample to content-analyze the sample of tweets. These include models trained using Apple's Core ML Transfer Learning model, TensorFlow Keras models, Scikit-learn models, and Google's AutoML models. After comparing the accuracy of the models, we used one of Google's AutoML models with a precision of 74.38% (the highest among the models we trained) to code the Twitter sample.
AutoML is part of Google's Natural Language Processing (NLP) API, among the most popular and accurate machine learning tools for text classification and sentiment analysis among scholars (Hopkins and King, 2010; O'Connor et al., 2010; Franch, 2013). To ensure inter-coder reliability, we hand-coded a sub-sample (n = 100) of the tweets coded with our AutoML model. A Krippendorff's Alpha test indicates that the machine learning model coded the tweets reliably (α = 0.726).
In addition to content analyzing the tweets, the tweet ID, the tweeter's handle, the number of retweets the tweet received, the location from which the tweet originated, and the URLs mentioned in the tweet were also extracted. Additionally, we determined whether the tweet was authored by the user or a retweet (in which case, the handle of the original author was also extracted.). We used this information to determine whether the tweet represented the opinions of the tweeter (and should be included in the public attribute agenda sample), or the propagation of the opinions expressed in other tweets or media outlets (social media attribute agenda sample). Retweets and tweets including a link to an external source were classified as traditional/vertical if the original author of the tweet (in case of retweets) or the sources mentioned in the tweet met the traditional/vertical criteria as laid out by Shaw et al. (2019); otherwise, they were coded as social/horizontal.
4. Results
To test our hypotheses, the media sample and the Twitter sample datasets were organized by week (N = 22). Then, the attribute agenda of each dataset is determined by ranking the salience of each attribute in each week for both datasets based on the frequency of the attributes used.
Our first hypothesis posits there is a correlation between the traditional media agenda and the public agenda. Spearman's rho correlations between the attribute agenda of the traditional media sample and the attribute agenda of the tweets in our sample that were not originated by traditional media accounts were statistically significant in 16 of the 22 weeks in our study timeframe, partially supporting the first hypothesis (H1). The second hypothesis predicts a correlation between the social (horizontal) media attribute and public attribute agendas. Spearman's rho correlations between the attribute agenda of the traditional media sample and the attribute agenda of the tweets in our sample that did not mention any traditional media revealed statistically significant correlations between the two in 14 of the 22 weeks in our study timeframe, again, demonstrating partial support for our hypothesis (H2). Table 1 displays the week-by-week correlations along with the major COVID-related events. Figure 2 displays the major events that took place each week alongside the traditional (vertical) media and social (horizontal) media attribute agenda correlations for the week.
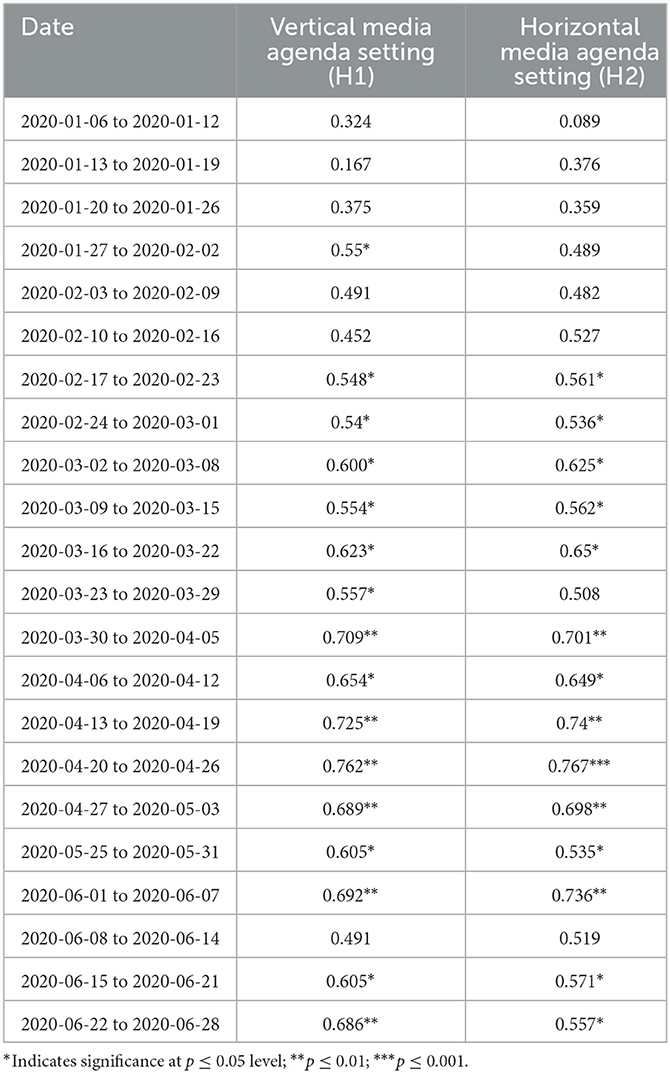
Table 1. Weekly Spearman's rho correlations between traditional (vertical) media attribute agenda and the public agenda (H1) and Social (horizontal) media attribute agenda and the public agenda (H2).
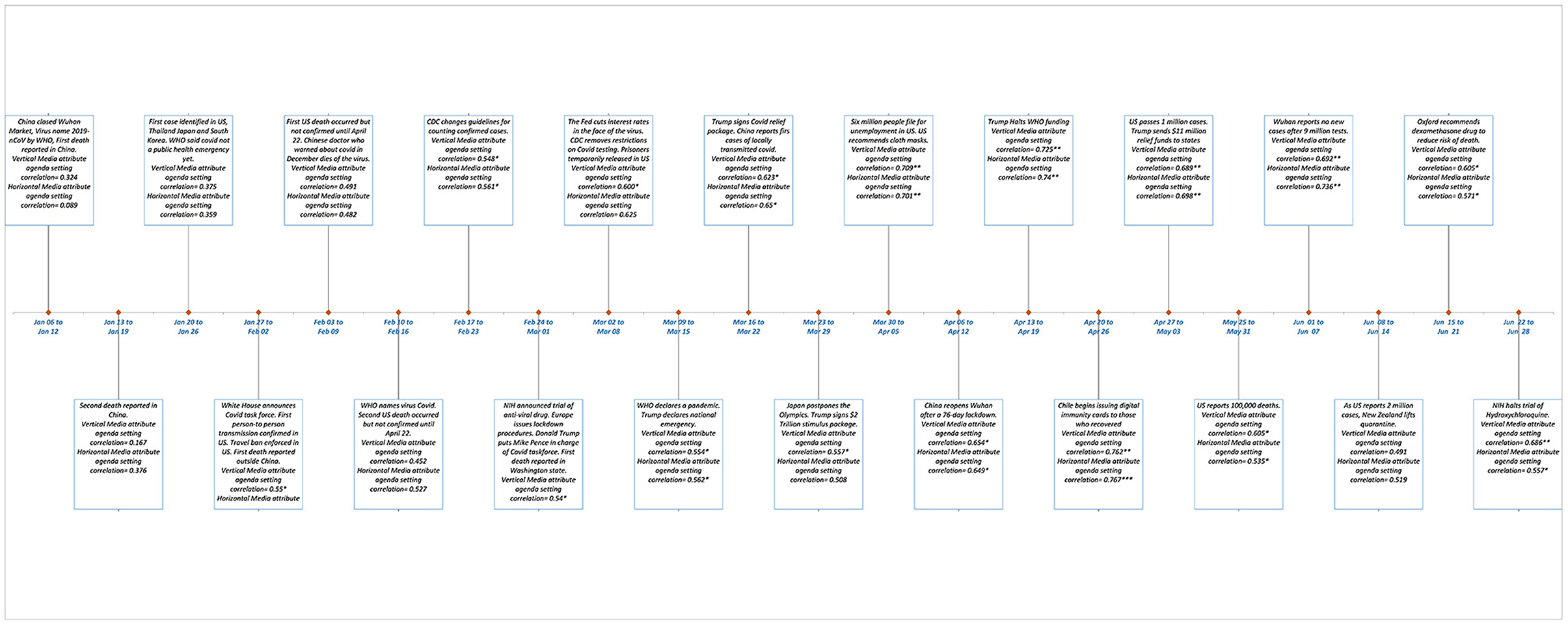
Figure 2. Weekly milestone graph of COVID-19 events and the traditional (vertical) media and social (horizontal) media attribute agenda correlations.
These correlations show the extent to which traditional media and Twitter users used the same language to discuss the COVID-19 pandemic.4 It is essential to note that the non-significant correlations occurred in the early weeks of the pandemic when there was not even a consensus on the name of the virus and can be explained by the equivocal coverage of the pandemic. Thus, these results provide strong justification to support the first two hypotheses.
To address the empirical questions, we use the Agenda Community Attraction (ACA) formula (Shaw et al., 2019) to calculate the contribution of the personal attribute agenda of Twitter users to the public attribute agenda.
Our first empirical question asks: To what extent do traditional media, social media, and the personal preferences of individuals explain the variations in the public attribute agenda on COVID-19? Given that we have, through H1 and H2, identified both the traditional (vertical) media attribute agenda-setting correlations and the social (horizontal) attribute agenda-setting, the predicted and actual contribution of personal attribute agenda to the public agenda can be calculated. We applied the ACA formula but used attribute agenda setting values in lieu of agenda setting values to estimate the personal attribute agenda. Table 2, which is modeled after McCombs et al. (2014) representation of personal agenda, compares the theoretical and actual contribution of personal attribute agenda to the public agenda (p. 798).
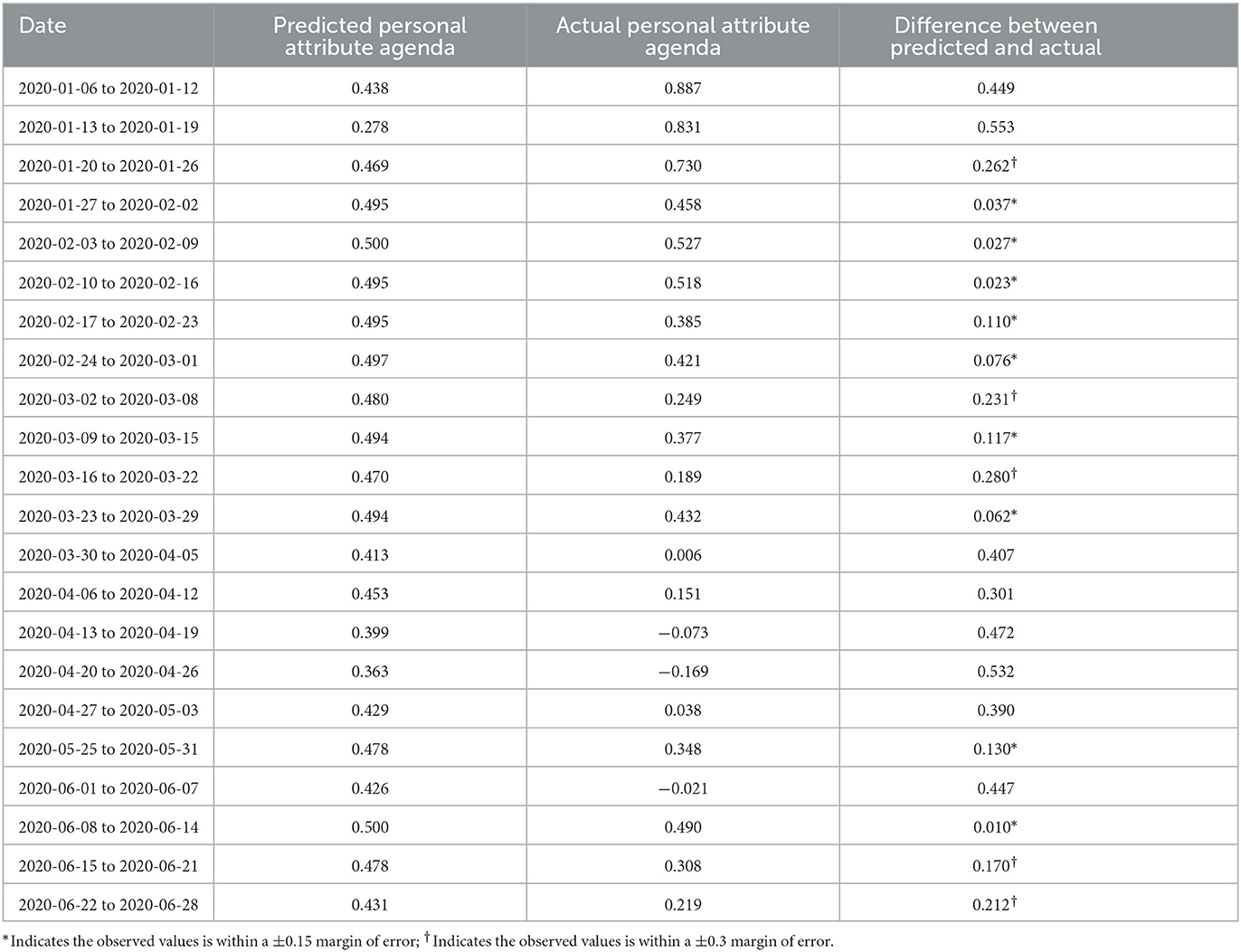
Table 2. Weekly contribution of personal attribute agenda (predicted and actual) effect size to the public attribute agenda.
Figure 3 graphs the values reported in Tables 1, 2 similar to how Shaw et al. (2019) illustrated the changes in the contribution of various sources to the public agenda (p. 94, 95, and 114). The figure displays the changes in the contribution of the personal attribute agenda to public opinion on COVID-19 compared to the traditional (vertical) media attribute agenda and social (horizontal) attribute agenda over the span of the first 6 months of the pandemic.
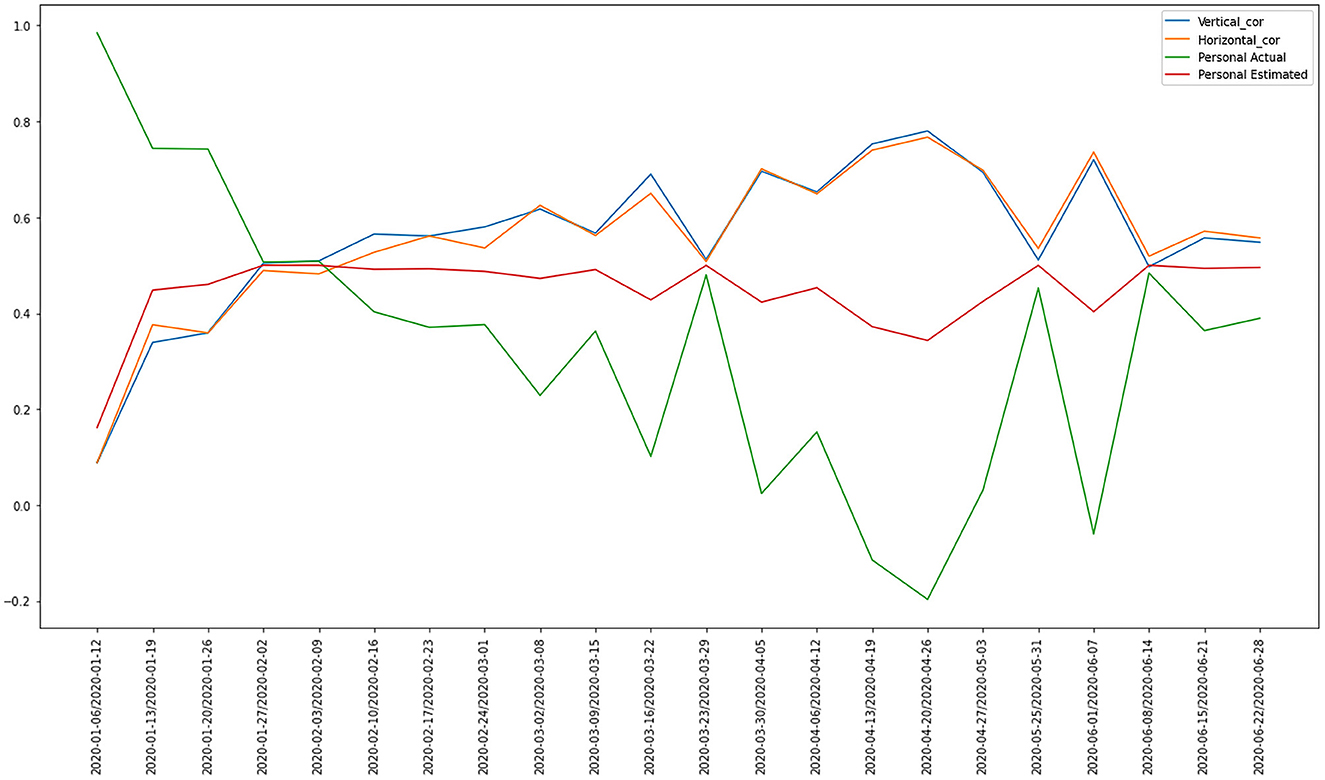
Figure 3. Agendamelding graph of the contribution of traditional media attribute agenda, social media attribute agenda, and actual and expected personal attribute agenda to the public attribute agenda of the issue of COVID-19.
As Figure 3 demonstrates, at the onset of the pandemic in January 2020, people almost exclusively relied on their own attitudes. Still, by the 1st week of February, their attitudes converged with the traditional media, in that their reliance on their preexisting attitudes drastically reduced (to 0.4 from 0.8 2 weeks earlier) and their reliance on traditional media increased (to 0.5 from 0.1 2 weeks earlier) and their reliance on horizontal media increased (to 0.4 from 0.1 2 weeks earlier). From the 2nd week of February 2020, traditional media and social media managed to take over and set the attribute agenda of the conversation around COVID-19. In May 2020, social media and other non-traditional media (which include heavily partisan media) managed to become the dominant attribute agenda setters on the issue of COVID-19.
We employed a series of autoregressive integrated moving-average (ARIMA) time-series modeling analyses to address the second question. ARIMA models are often used with time-series first- and second-level agenda-setting analysis and are recognized as an effective way to predict dependent variables (Kim et al., 2016). ARIMA was first proposed for journalism research in 1981 (Maisel and Wunsch, 1981). ARIMA models can model stationary and autocorrelation components (Gonzenbach, 1996), which has resulted in an “overwhelming majority of agenda-setting research” relying on ARIMA modeling for time series analyses (Vargo, 2011).
One of the requirements of time-series analysis is a minimum of 30 to 40 time points (Sayre et al., 2010). To meet this requirement, we used daily data (as opposed to weekly data used in hypothesis testing). Given this transition, there were days in which neither traditional media content nor Twitter met the sampling criteria and had to be removed from the sample resulting in 94 data points. Therefore, the minimum data point requirement is met.
Augmented Dickey-Fuller (ADF) tests for each of the three variables (i.e., traditional/vertical media, social/horizontal media, and personal preferences) revealed that the time series is stationary. Stationary time series have linear tendencies characterized by short-term variations but long-term stability and, therefore, do not have seasonality or trends. Table 3 displays the results of the ADF test.

Table 3. Stationarity of traditional media attribute agenda, social media attribute agenda, and personal attribute agenda.
ARIMA (1,0,1)—i.e., 1 day lag added to the model, zero difference to produce stationary data, and 1 day lag added to the error term—models find all three variables are significant predictors of the public attribute agenda on the issue of COVID, while zero lag ARIMAs (0,0,0) finds significance for personal preferences and social media, but not traditional media. The first model using traditional media correlations explained more than 96% of the variance in the public attribute agenda (R2 = 0.963) in the period of the study.
Overall, Traditional media (β = 0.778, p < 0.001) had the strongest effect on how the public perceived the Coronavirus, followed by social media (β = 0.431, p < 0.001) and the audiences' personal agenda (β = 0.311, p < 0.001). Figure 4 demonstrates how our ARIMA (1,0,1) model's predictions using the vertical media correlation values compare with the observed public attribute agenda values.
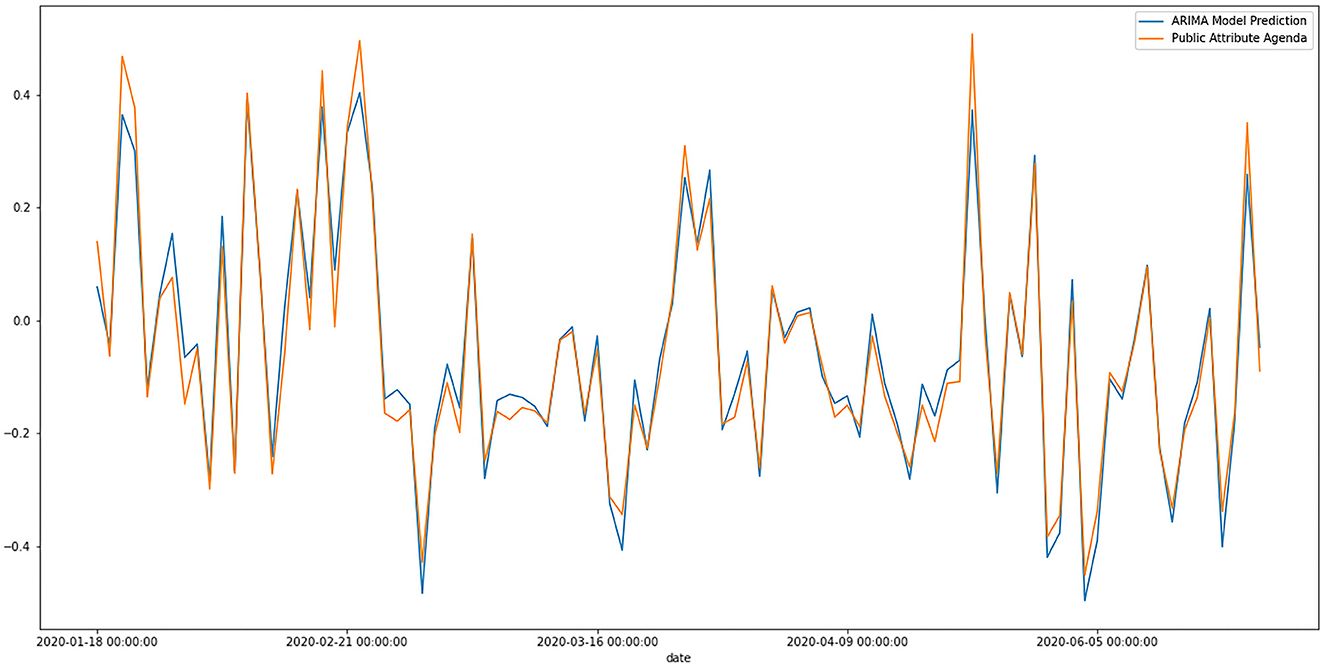
Figure 4. ARIMA (1, 0, 1) model's predictions compared with the public attribute agenda from January 18, 2020 to June 28, 2020.
In sum, the results demonstrate significant relationships between the attributes used by traditional media to describe the issue of COVID-19 and attribute agendas of social media and the personal attribute agenda of audiences.
5. Findings and discussion
5.1. General implications
By incorporating an attribute agendamelding approach and the ARIMA time-series analysis, this study looks at the relationships between the different attributes used in traditional media to describe COVID-19 and attributes used by social media and audiences to describe the virus. Our findings support the notion that audiences meld attribute agendas from different sources of information to form their opinions about an issue—in this case, COVID-19. Specifically, the attributes of traditional media, social media, and the audience's personal attributes were all positively correlated with the public attribute agenda.
5.2. Explaining differences in ACA predictions and empirical findings
In several weeks of our analysis timeframe, we observed a significantly higher contribution of personal preferences than the ACA predicted. This means that during those weeks, Twitter users relied more on their own understanding and language to discuss the COVID-19 pandemic. While in some weeks, the difference between the expected and actual values was within the established 0.15 ACA margin of error (n = 9), in most weeks, the differences were within ±0.3.
This discrepancy between the ACA prediction and the actual values may stem from the disjointed way information was disseminated in the media during this early period of the pandemic. For instance, the first confirmed case of COVID-19 was detected on December 1, 2019, in Wuhan, China (Wu et al., 2020). However, it was not reported to the WHO until December 31, and the organization did not give it the 2019-nCoV name until January 7, 2020. The Wuhan Municipal Health Commission reported the first death on January 11th. The first confirmed case on US soil (in Washington State) was not identified until January 21 (CNN Editorial Research, 2021). As late as January 23, 2020, the WHO maintained that it was “too early to consider that this event is a Public Health Emergency of International Concern” (WHO, 2020, p. 2). It was not until January 29 that the White House announced that it was forming a taskforce to help monitor and contain the spread of the virus. This announcement marked an uptick in media reports about COVID-19. In fact, in our media sample, we barely had double-digit media reports about the virus until the week of January 20 (see Figure 5), which is to be expected as we are exclusively focusing on U.S. media and attitudes.
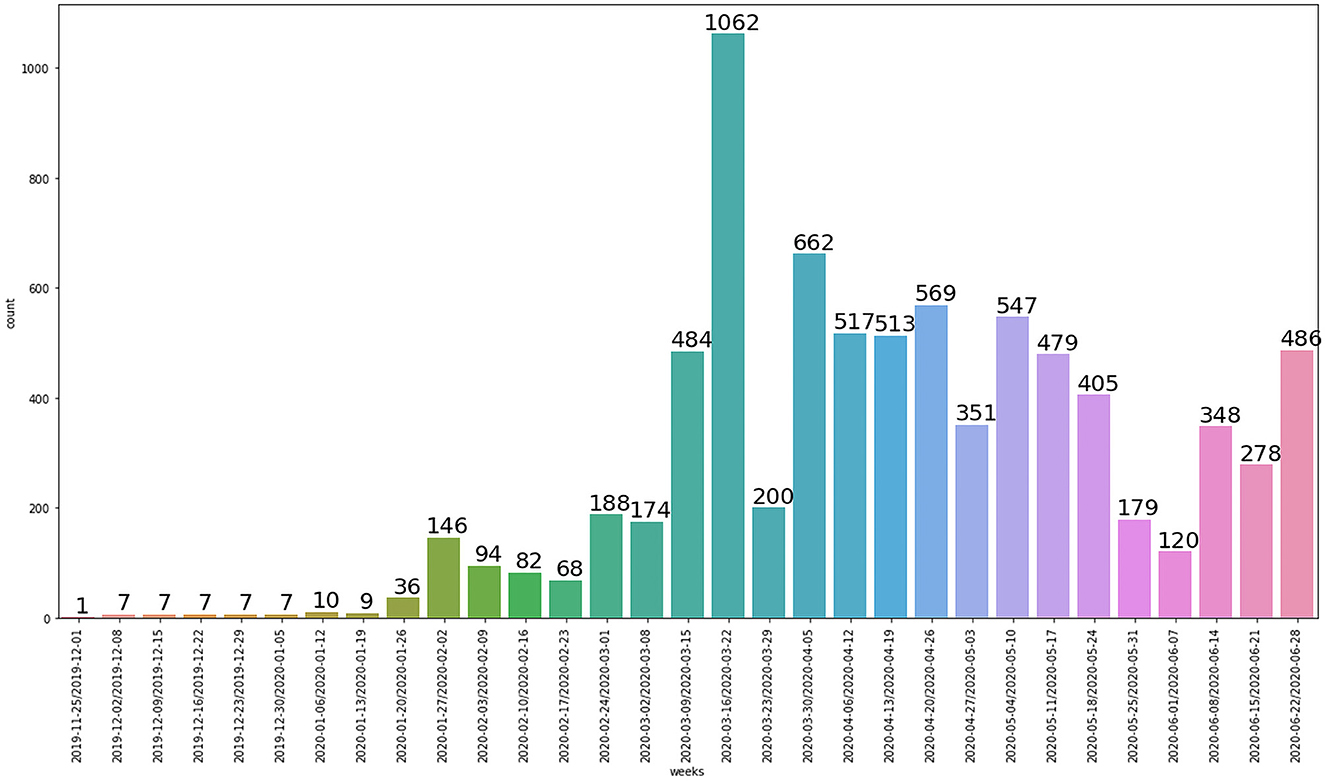
Figure 5. Frequency of news items in the sample about COVID-19 from November 11, 2019 to June 28, 2020.
Given the scarcity of news reports about the virus and the absence of agreement among news organizations (and even health organizations) on how to describe the virus—in other words, the absence of a media attribute agenda about the virus—it stands to reason that there was no salience to be transferred from the media to the public. This explains the non-significant correlations in the early weeks of our study. To better understand how the agendas of the media and the public differed during this period, it is instructive to look at examples.
5.2.1. Illustrative examples
Figures 6, 7 display the changes in attribute ranking for February 3–9 and February 10–16, respectively. In the 1st week, “misinformation” was the number one attribute coded in the social media sample. However, it was the second least common attribute in the traditional media sample. For the traditional media, the most common attribute used to discuss COVID-19 is “economic consequences,” but that attribute is in the middle of the pack for social media users. Moving on to the 2nd week, we see again that social media users most commonly use misinformation when discussing COVID-19 between February 10–16, while—again—this is the second least used attribute among traditional media in our sample. For this week, in the traditional media sample, “fear and panic” is the most common attribute, which is the second most common attribute for Twitter users in our sample.
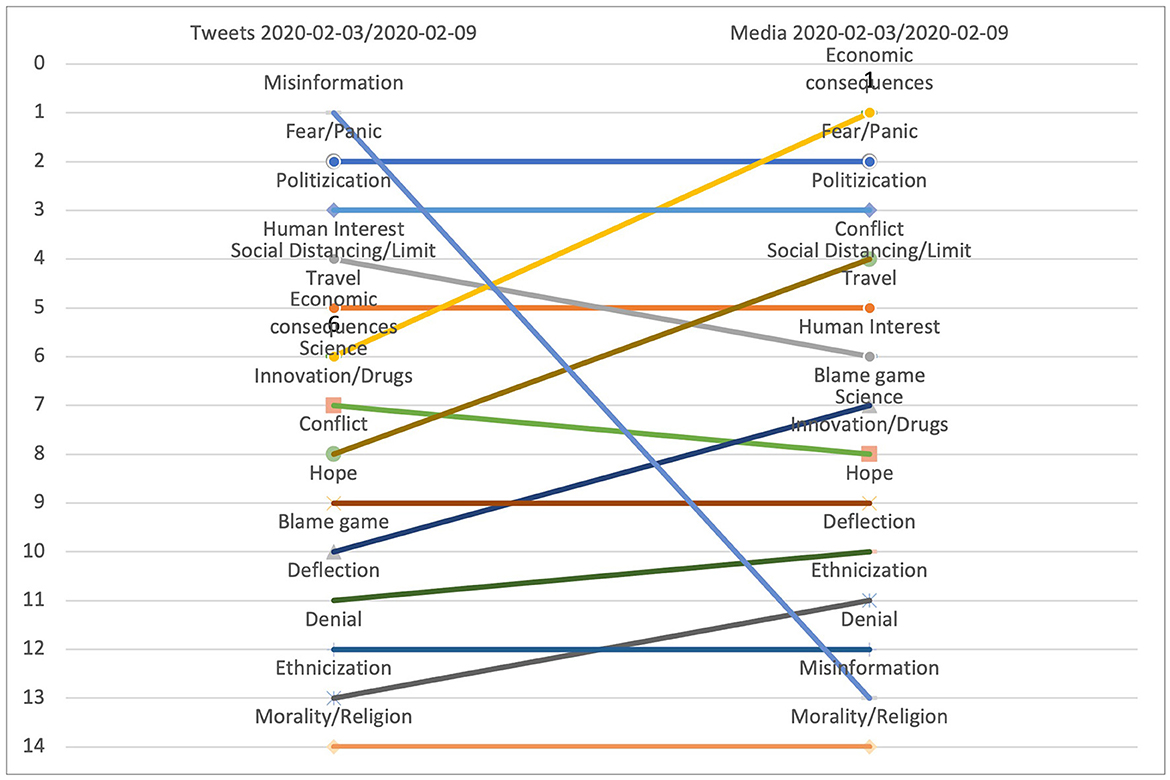
Figure 6. The rank of attributes used to describe COVID-19 on our Twitter (left) and traditional media (right) sample between February 2, 2020, and February 9, 2020. Lower ranks indicate higher frequency.
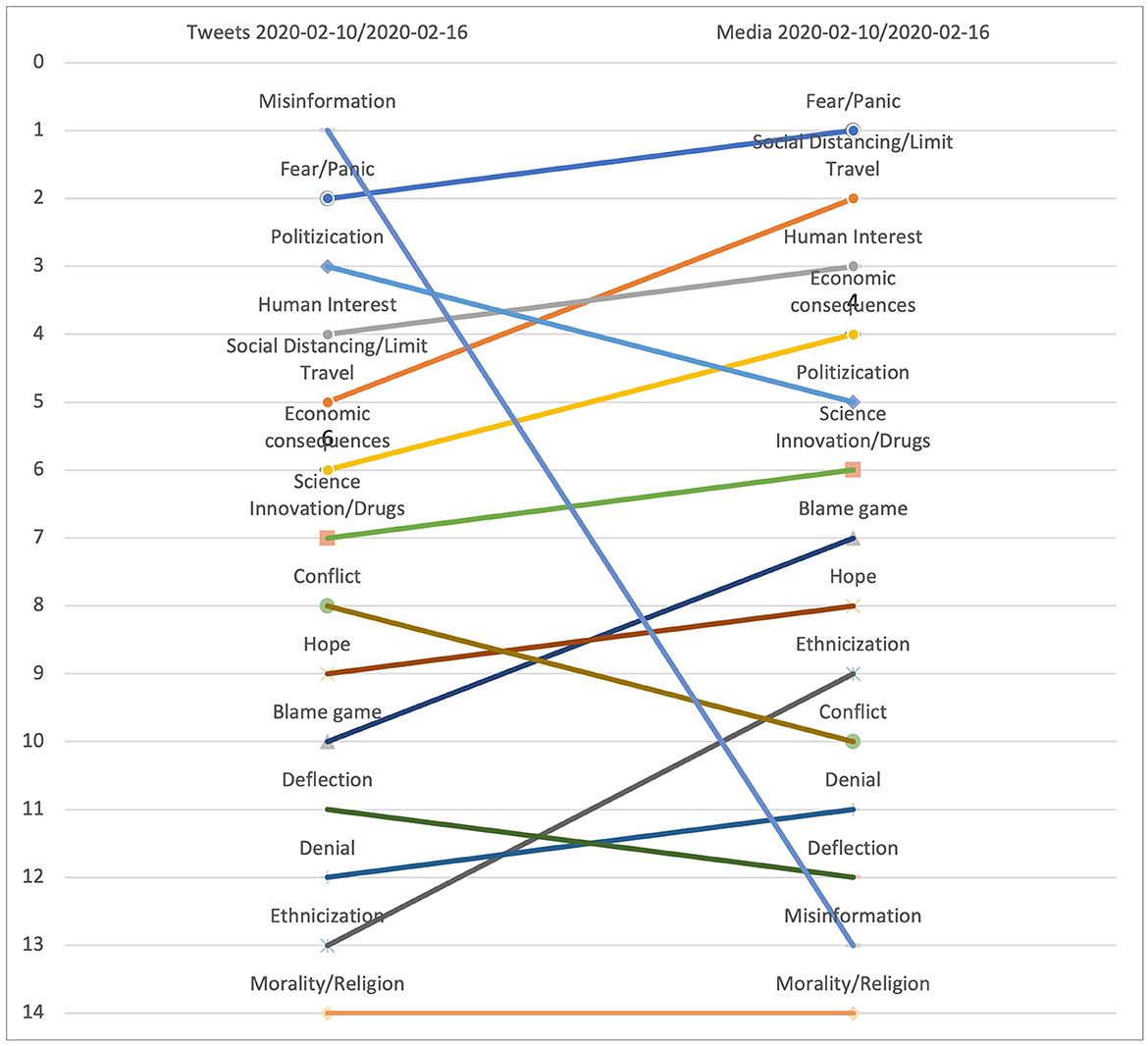
Figure 7. The rank of attributes used to describe COVID-19 on our Twitter (left) and traditional media (right) sample between February 10, 2020, and February 16, 2020. Lower ranks indicate higher frequency.
The point here is that during this early stage of the pandemic, we can ascribe the absence of significant correlations in those 2 weeks to the organic rise of misinformation on social media before traditional media had a chance to disambiguate misconceptions about the virus.
The only week after February 2020 where no correlations are found is June 8, 2020, to June 14, 2020. Again, “misinformation” ranked first in our Twitter sample but second to last in our media sample. In this week, “fear and panic” stories about COVID-19 dominated our media sample, followed by “social distancing” (ranked fifth in the Twitter sample) and “economic consequences” (ranked sixth in the Twitter sample). Figure 8 shows the relative ranking, by sample, for this week. Because it ranked so highly for the early pandemic period on social media, it may be instructive to look at some “misinformation” examples to understand how they are coded. To reiterate, we are building from previously published studies on relevant attributes, but our coders (human and machine) are doing original work.
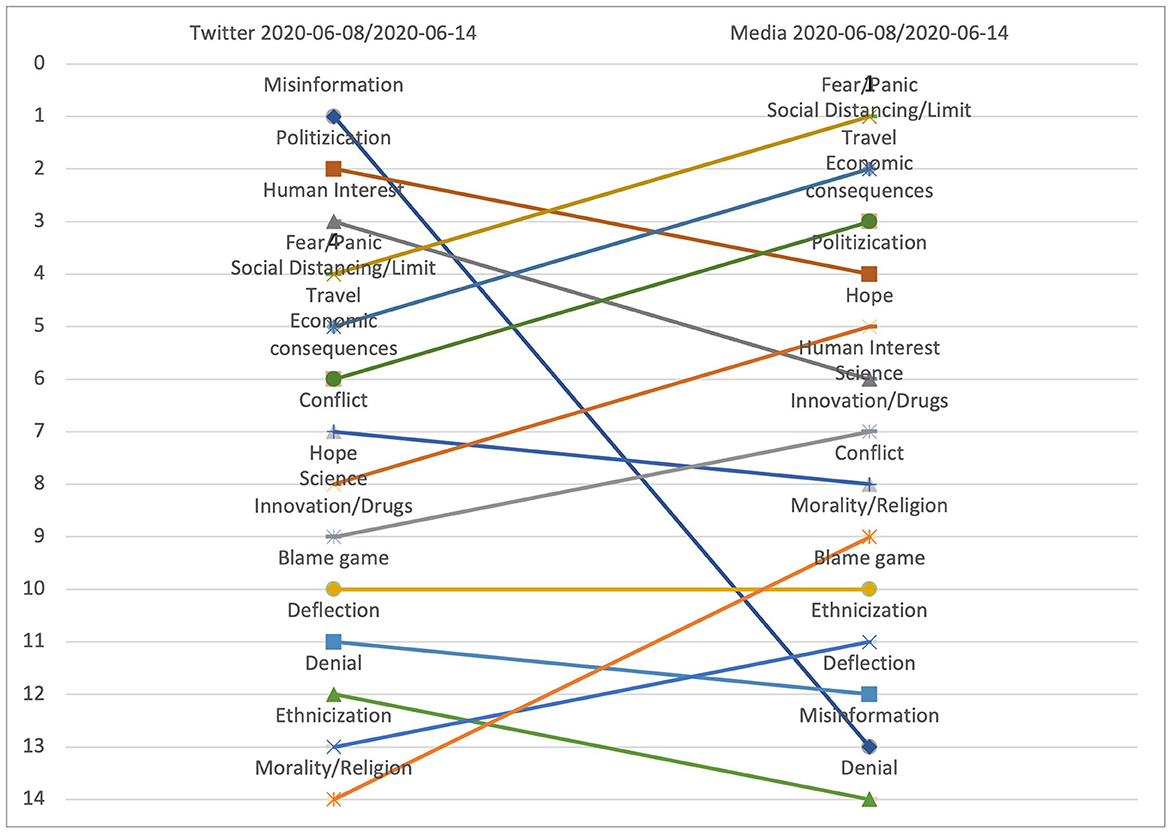
Figure 8. The rank of attributes used to describe COVID-19 on our Twitter (left) and traditional media (right) sample between June 08, 2020, and June 14, 2020. Lower ranks indicate higher frequency.
As a first example, some of the tweets categorized as “misinformation” by our model during this period were the usual conspiracy theories that question the information provided by official sources, such as this one by conservative political commentator Steven Crowder (@scrowder), whose now-deleted tweet, “Was anything we were told about the coronavirus true?,” was retweeted by more than two thousand people.
Other misinformation examples include this tweet by Senator Rand Paul, “Good News! People who catch coronavirus but have no symptoms rarely spread the disease. Translation: sending kids back to school does not require millions of test kits. Asymptomatic spread of coronavirus is ‘very rare,' WHO says.” The tweet has since been retweeted more than 6,000 times and is considered “misinformation” for Sen. Paul's editorialization of the actual WHO report.
However, a possible explanation for the discrepancy in the misinformation ranking between the media and Twitter is the rise of tweets containing misinformation in the aftermath of the Minneapolis city council's decision to disband the police department in the wake of the Black Lives Matter protests. These posts made it to the sample because they either contained a COVID-19 hashtag or addressed aspects of the pandemic in addition to the BLM movement. Because people using social media may say nearly anything they would like when highly salient events are happening simultaneously, it is natural that social media users would discuss them in the same posts.
Many of the tweets our model labeled as “misinformation” in this period are from accounts that have since been suspended and include posts like this, from @Tombx7M, saying that “in the police free future…Remember this in November. Protect your family by voting the radical out #MorningJoe #protest2020 #covid19.” Our model classified this tweet as “misinformation” for inclusion of the phrase “police free future” as a matter of fact, with no qualifier. Another example of such tweets is this one from the now-suspended account of @LehneSue, which said, “@realDonaldTrump COVID19 ACROSS THE COUNTRY, DEM MAYOR & GOVERNOR OK WITH PROTESTORS, OK WITH RIOTERS, VIOLENCE https://t.co/APk6SyIcxT.” Again, despite being only marginally related to the pandemic, this tweet was included in our sample and was labeled as “misinformation” by our model—as Democratic mayors and governors openly condemned riots and violence.
Although some of these tweets may seem only marginally related to the disease and the virus, they were a big part of the civil discourse at the height of the COVID-19 pandemic. The pandemic was politicized and used as a political tool, and much of the COVID-19 fact-checks were about claims made by, or about, politicians (Luengo and García-Marín, 2020). Leaving these tweets out of the analysis would ignore a large part of the audience's traditional media and social media diet.
5.3. Traditional media can affect social media attributes
Our time series analysis demonstrated that attribute agendas of traditional media influence the attribute agendas on social media. In the literature, an intermedia agenda-setting relationship has been found between traditional media and various online platforms such as political blogs, YouTube videos, and Twitter (Meraz, 2009; Sayre et al., 2010; Vargo, 2011; Kim et al., 2016; Guo and Vargo, 2020). Since journalists in mainstream media use Twitter as a source for news gathering and interacting with their users, the effect of traditional media on social media has been questioned. Some scholars argue that social media have become the leading agenda setters because of their ability to influence the attention devoted to a particular issue (Ceron et al., 2016); others have proposed that social media users source and distribute their own information independent of traditional media (Newmann et al., 2012). Given that our zero-lag model did not find a significant effect of traditional media on the public attribute agenda, but our one-day-lag model did, we can attribute at least some of the variation in social media attribute agenda to mainstream media.
Lending further support to this argument is the absence of any traditional media or social media effect on public agenda in the early weeks of the pandemic when there was no cohesive media attribute agenda regarding COVID-19. This increased uncertainty among audiences and highlighted their need for orientation (NFO), which is defined as the driving force behind an individual's desire to get information from the media and has been used to explain why individuals are affected differently by agenda-setting effects (Camaj and Weaver, 2013; Camaj, 2014). When the need for orientation is high (e.g., during a global pandemic with a novel virus), audiences look to the media for clues to make sense of the world around them (Weaver, 1980). The equivocal response of traditional media in the early days of the pandemic prompted the public to rely more on their personal preference when melding agendas. The heightened ambiguity laid the groundwork for a robust agenda-setting effect when the media finally converged and arrived at a unified attribute agenda. Our results are in line with Lee et al. (2022) finding that individuals with moderate or high NFO tended “to get information about COVID-19 from all the media—vertical media, conservative and liberal horizontal media, and social media” as opposed to just traditional (vertical) media or just social (horizontal) media (p. 19).
By separating tweets from traditional media accounts and those citing traditional media sources from organic tweets that do not rely on traditional media for information (at least explicitly), we attempted to unblur the line between traditional media on Twitter and the Twitterverse. Our findings show that an attribute agendamelding process is at play between conventional media, social media, and the personal preferences of audiences during the early days of the COVID-19 pandemic in the United States.
6. Conclusions
We started this project by asking: How are attitudes formed in the 21st Century, and who set the attribute agenda for the initial COVID-19 coverage in the United States? The findings show that in the early weeks of the pandemic, public opinion on Twitter about the virus was distinctly different from the coverage of the issue in traditional media. The attributes used to describe it on social media demonstrate users relying on their past experiences and personal beliefs to talk about the virus. In the 1st week of February, public opinion, traditional media, and social media converged, but traditional media soon became the leading agenda setter of COVID-19 for 13 weeks. However, for the final 5 weeks of our sample, traditional media are taken over by social media. The findings also show that aside from a few weeks at the onset of the outbreak, Twitter users relied on their personal experiences far less than what statistical models predict and allowed traditional media and social media to shape their opinion of the issue. In sum, the findings support that traditional media can play a crucial role in driving the agenda during a pandemic.
The practical implications of our project are helpful for public health practitioners and crisis communicators. Our findings suggest that audiences likely rely on traditional media for information in the face of an unknown phenomenon. When traditional media disseminate unequivocal information and there is agreement between various traditional sources of information. However, if traditional media present equivocal information with little to no agreement, audiences rely on their own personal experience to make sense of the uncertainty, which results in a cacophony of unverified, mis-, or even disinformation. Public health practitioners and crisis communicators can prevent this by presenting a unified front and disseminating good information at the onset of the crisis through traditional media.
Despite this study's theoretical and practical contributions, there are caveats with any research. First, the sample size of our traditional media will prevent wide generalization of our findings. We use only four papers in the United States and six television programs. While these outlets indeed represent mainstream, traditional media, they are not the universe of American newspaper and television outlets. Furthermore, although we collected data using systematic methods thought to be as exhaustive as possible, the sample is not a census. Similarly, we are focused only on the first 6 months of the COVID-19 pandemic. We specifically focus on this period because the attributes used to discuss and frame media coverage are negotiated early during novel crisis events, but attributes change over time. Larger data sets that include more extensive time periods and more keywords could be used to analyze the attribute agendamelding process.
The coding categories of the attributes could also pose a limitation. To compensate for this drawback, the coding categories were created based on existing studies of COVID and conducted intercoder reliability tests after training coders. Despite several studies using content analysis on COVID-19-related media (e.g., Ogbodo et al., 2020; Gallagher et al., 2021) there needs to be consistent operationalization and categorization of issue and attribute agendas in the literature. We add to this conversation, but future scholars should attempt to develop comprehensive operational definitions of these concepts.
There are also concerns about using machine learning models to code tweets. Our model has a relatively high accuracy rate and an intercoder reliability test conducted on a subsample of tweets. These should allay some of the concerns associated with computer-assisted coding. However, asymmetrical data (in terms of the differences in the length of units in the training data compared to the length of units in the final data) always poses a challenge. Furthermore, we do not assess or identify partisanship or assign an ideological valence to tweets in our sample. There is no doubt partisanship played a significant role in responses to the pandemic as time moved forward, but that is not something we account for in this study.
Finally, the time frame of the data is limited because we collected it during the first 6 months of the COVID-19 pandemic. It remains to be seen if data collected over a more extended period will support the findings of our work presented here.
Data availability statement
The raw data supporting the conclusions of this article will be made available by the authors, without undue reservation.
Ethics statement
The studies involving human participants were reviewed and approved by Kennesaw State University Institutional Review Board. Written informed consent for participation was not required for this study in accordance with the national legislation and the institutional requirements.
Author contributions
MM wrote the first draft of the paper, did data analysis, and wrote code for Google sentiment analysis. JT assisted with the first draft, coordinated research assistants, and generated traditional news source content data. MM and JT contributed equally to the final draft and final edits. CV gathered and dehydrated Twitter data used for analysis. All authors contributed to the article and approved the submitted version.
Funding
MM and JT were supported by a 2020 Kennesaw State University, Norman J. Radow College of Humanities and Social Sciences, Scholarship Support Grant, and Google Cloud COVID-19 research credits program.
Acknowledgments
Previous versions of this paper were presented at the Annual Meeting of the Midwest Political Science Association (2021), Annual Meeting of the American Political Science Association (2021), and the Annual Meeting of the Association for Education in Journalism and Mass Media Southeast Colloquium (2022). We thank the conference discussants and fellow panelists for their helpful suggestions for improving this manuscript. We would like to acknowledge the work of our undergraduate research assistants—Mia Gonzales, Zaria Richey, and Carina Worm—in gathering and coding the raw content data. We would also like to acknowledge Kennesaw State University's High-Performance Computing (HPC) cluster that made analyzing our data possible (Boyle and Aygun, 2021).
Conflict of interest
The authors declare that the research was conducted in the absence of any commercial or financial relationships that could be construed as a potential conflict of interest.
Publisher's note
All claims expressed in this article are solely those of the authors and do not necessarily represent those of their affiliated organizations, or those of the publisher, the editors and the reviewers. Any product that may be evaluated in this article, or claim that may be made by its manufacturer, is not guaranteed or endorsed by the publisher.
Supplementary material
The Supplementary Material for this article can be found online at: https://www.frontiersin.org/articles/10.3389/fpos.2023.1021855/full#supplementary-material
Footnotes
1. ^Initially, we attempted to include data from December 2019 to ensure we captured both horizontal and vertical media activity from the absolute start of the pandemic. There was, however, no substantial attention to COVID-19 in the United States until January 2020, which resulted in no data in December 2019.
2. ^See the Appendix A for the codebook.
3. ^The size of the subsample was determined by Riffe et al. (2014) method.
4. ^For weekly Spearman's rho correlations between traditional (vertical) media attribute agenda and Social (horizontal) media attribute agenda (see Appendix C).
References
Althaus, S. L., and Tewksbury, D. (2002). Agenda setting and the “new” news: Patterns of issue importance among readers of the paper and online versions of the New York Times. Commun. Res. 29, 180–207. doi: 10.1177/0093650202029002004
Anderson, J. R., and Bower, G. H. (1980). Human Associative Memory: A Brief Edition. Hillsdale, NJ: L. Erlbaum Associates.
Annual Meeting of the American Political Science Association (2021). Seattle, WA, September, 30–October, 3.
Annual Meeting of the Association for Education in Journalism and Mass Media Southeast Colloquium (2022). Memphis, TN, March, 17–19.
Bantimaroudis, P., Sideri, M., Ballas, D., Panagiotidis, T., and Ziogas, T. (2020). Conspiracism on social media: An agenda melding of group-mediated deceptions. Int. J. Media Cult. Polit. 16, 115–138. doi: 10.1386/macp_00020_1
Becker, L. B., and McCombs, M. E. (1978). The role of the press in determining voter reactions to presidential primaries. Human Commun. Res. 4, 301–307. doi: 10.1111/j.1468-2958.1978.tb00716.x
Boyle, T., and Aygun, R. (2021). Kennesaw State University HPC Facilities and Resources. Digital Commons Training Materials. Available online at: https://digitalcommons.kennesaw.edu/training/10
Camaj, L. (2014). Need for orientation, selective exposure, and attribute agenda-setting effects. Mass Commun. Soc. 17, 689–712. doi: 10.1080/15205436.2013.835424
Camaj, L., and Weaver, D. H. (2013). Need for orientation and attribute agenda-setting during a U.S. election campaign. Int. J. Commun. 7, 1442–1463. Available online at: https://ijoc.org/index.php/ijoc/article/view/1921/937
Ceron, A., Curini, L., and Iacus, S. M. (2016). First- and second-level agenda setting in the Twittersphere: An application to the Italian political debate. J. Inf. Technol. Polit. 13, 159–174. doi: 10.1080/19331681.2016.1160266
Chadwick, A. (2013). The Hybrid Media System: Politics and Power. Oxford ; New York: Oxford University Press. doi: 10.1093/acprof:oso/9780199759477.001.0001
Chaffee, S. H., and Metzger, M. J. (2001). The end of mass communication? Mass Commun. Soc. 4, 365–379. doi: 10.1207/S15327825MCS0404_3
CNN Editorial Research (2021). COVID-19 Pandemic Timeline Fast Facts. CNN. Available online at: https://www.cnn.com/2021/08/09/health/covid-19-pandemic-timeline-fast-facts/index.html (accessed January 04, 2021).
Dance, F. E. X., and Gerbner, G. (1967). “Mass media and human communication theory,” in Human Communication Theory: Original Essays (New York: Harper and Holt, Rinehart and Winston) 40–60.
Entman, R. M. (1993). Framing: Toward clarification of a fractured paradigm. J. Commun. 43, 51–58. doi: 10.1111/j.1460-2466.1993.tb01304.x
Franch, F. (2013). (Wisdom of the Crowds) 2 : 2010 UK Election Prediction with Social Media. J. Inf. Technol. Polit. 10, 57–71. doi: 10.1080/19331681.2012.705080
Gallagher, R. J., Doroshenko, L., Shugars, S., Lazer, D., and Foucault Welles, B. (2021). Sustained online amplification of COVID-19 Elites in the United States. Soc. Media Soc. 7, 20563051211024956. doi: 10.1177/20563051211024957
Ghanem, S. (1997). “Filling in the tapestry: The second level of agenda setting,” in Communication and Democracy: Exploring the Intellectual Frontiers in Agenda-Setting Theory, eds. M. E. McCombs, D. L. Shaw, and D. H. Weaver (Mahwah, NJ: Lawrence Erlbaum Associates) 3–14.
Gilardi, F., Gessler, T., Kubli, M., and Müller, S. (2022). Social media and political agenda setting. Polit. Commun. 39, 39–60. doi: 10.1080/10584609.2021.1910390
Golan, G., and Wanta, W. (2001). Second-level agenda setting in the New Hampshire primary: A comparison of coverage in three newspapers and public perceptions of candidates. Journal. Mass Commun. Quart. 78, 247–259. doi: 10.1177/107769900107800203
Gollust, S. E., Nagler, R. H., and Fowler, E. F. (2020). The emergence of COVID-19 in the US: A public health and political communication crisis. J. Health Polit. Policy Law 45, 967–981. doi: 10.1215/03616878-8641506
Gonzenbach, W. J. (1996). The Media, the President, and Public Opinion: A Longitudinal Analysis of the Drug Issue, 1984-1991. Mahwah, N.J: Erlbaum.
Griffin, E. A., Ledbetter, A., and Sparks, G. G. (2014). A First Look at Communication Theory. Ninth Edition. New York: McGraw-Hill Humanities/Social Sciences/Languages.
Guo, L. (2012). The application of social network analysis in agenda setting research: A methodological exploration. J. Broadc. Electr. Media 56, 616–631. doi: 10.1080/08838151.2012.732148
Guo, L., and Vargo, C. (2020). “Fake News” and Emerging Online Media Ecosystem: An Integrated Intermedia Agenda-Setting Analysis of the 2016 U.S. Presidential Election. Commun. Res. 47, 178–200. doi: 10.1177/0093650218777177
Guo, L., and Vargo, C. J. (2017). Who determines the global news agenda? A big data analysis of international news flow on the internet. Paper presented at ICA 2017, Mass Communication and Society Division, San Diego, CA.
Guo, L., Vu, H. T., and McCombs, M. E. (2012). An expanded perspective on agendasetting effects. exploring the third level of agenda setting. Rev. de Comunic. 11, 51–68. Available online at: https://revistadecomunicacion.com/article/view/2755
Haim, M., Weimann, G., and Brosius, H.-B. (2018). Who sets the cyber agenda? Intermedia agenda-setting online: the case of Edward Snowden's NSA revelations. J. Comput. Soc. Sci. 1, 277–294. doi: 10.1007/s42001-018-0016-y
Hopkins, D. J., and King, G. (2010). A method of automated nonparametric content analysis for social science. Am. J. Political Sci. 54, 229–247. doi: 10.1111/j.1540-5907.2009.00428.x
Karami, A., Bennett, L. S., and He, X. (2018). Mining public opinion about economic issues: Twitter and the U.S. Presidential Election. IJSDS 9, 18–28. doi: 10.4018/IJSDS.2018010102
Kim, H. K., Ahn, J., Atkinson, L., and Kahlor, L. A. (2020). Effects of COVID-19 misinformation on information seeking, avoidance, and processing: a multicountry comparative study. Sci. Commun. 42, 586–615. doi: 10.1177/1075547020959670
Kim, Y., Gonzenbach, W. J., Vargo, C. J., and Kim, Y. (2016). First and Second Levels of Intermedia Agenda Setting: Political Advertising, Newspapers, and Twitter During the 2012 U.S. Presidential Election. Int. J. Commun. 10, 4550–4569. Available online at: https://ijoc.org/index.php/ijoc/article/view/5555
Kim, Y., Kim, Y., and Zhou, S. (2017). Theoretical and methodological trends of agenda-setting theory: A thematic analysis of the last four decades of research. Agenda Setting J. 1, 5–22. doi: 10.1075/asj.1.1.03kim
Kiousis, S., Bantimaroudis, P., and Ban, H. (1999). Candidate image attributes: Experiments on the substantive dimension of second level agenda setting. Commun. Res. 26, 414–428. doi: 10.1177/009365099026004003
Kiousis, S., Popescu, C., and Mitrook, M. (2007). Understanding influence on corporate reputation: An examination of public relations efforts, media coverage, public opinion, and financial performance from an agenda-building and agenda-setting perspective. J. Public Relat. Res. 19, 147–165. doi: 10.1080/10627260701290661
Kouzy, R., Abi Jaoude, J., Kraitem, A., El Alam, M. B., Karam, B., Adib, E., et al. (2020). Coronavirus goes viral: quantifying the COVID-19 misinformation epidemic on Twitter. Cureus. 12, e7255. doi: 10.7759/cureus.7255
Lee, T., Johnson, T. J., and Weaver, D. H. (2022). Navigating the Coronavirus Infodemic: Exploring the Impact of Need for Orientation, Epistemic Beliefs and Type of Media Use on Knowledge and Misperception about COVID-19. Mass Commun. Soc. 2022, 1–26. doi: 10.1080/15205436.2022.2046103
Lopez-Escobar, E., Llamas, J. P., and McCombs, M. E. (1998). Agenda setting and community consensus: First and second level effects. Int. J. Public Opinion Res. 10, 335–348. doi: 10.1093/ijpor/10.4.335
Luengo, M., and García-Marín, D. (2020). The performance of truth: politicians, fact-checking journalism, and the struggle to tackle COVID-19 misinformation. Am. J. Cult. Sociol. 8, 405–427. doi: 10.1057/s41290-020-00115-w
Maisel, R., and Wunsch, R. (1981). ARIMA time series analysis and forecasting public opinion. Public Opin. Quart. 45, 422–427. doi: 10.1093/poq/45.3.422
McCombs, M. E. (1997). New frontiers in agenda setting: Agendas of attributes and frames. Mass Commun. Rev. 24, 32–52.
McCombs, M. E. (2005). A Look at Agenda-setting: past, present and future. Journal. Stud. 6, 543–557. doi: 10.1080/14616700500250438
McCombs, M. E. (2014). Setting the Agenda: The Mass Media and Public Opinion. 2nd ed. Malden, MA: Polity.
McCombs, M. E., Llamas, J. P., Lopez-Escobar, E., and Rey, F. (1997). Candidate images in Spanish elections: Second-level agenda-setting effects. Journal. Mass Commun. Quart. 74, 703–717. doi: 10.1177/107769909707400404
McCombs, M. E., Lopez-Escobar, E., and Llamas, J. (2000). Setting the agenda of attributes in the 1996 Spanish general election. J. Commun. 50, 77–92. doi: 10.1111/j.1460-2466.2000.tb02842.x
McCombs, M. E., and Shaw, D. L. (1972). The agenda-setting function of mass media. Public Opinion Quart. 36, 176. doi: 10.1086/267990
McCombs, M. E., and Shaw, D. L. (1993). The evolution of agenda-setting research: Twenty-five years in the marketplace of ideas. J. Commun. 43, 58–67. doi: 10.1111/j.1460-2466.1993.tb01262.x
McCombs, M. E., Shaw, D. L., and Weaver, D. H. (2014). New directions in agenda-setting theory and research. Mass Commun. Soc. 17, 781–802. doi: 10.1080/15205436.2014.964871
McLuhan, M. (1962). Understanding Media: the Extensions of Man. 1st MIT Press ed. Cambridge, Mass: MIT Press.
McWhorter, C. (2020). The role of agenda melding in measuring news media literacy. JMLE 12, 145–158. doi: 10.23860/JMLE-2020-12-1-11
Meraz, S. (2009). Is There an Elite Hold? Traditional Media to Social Media Agenda Setting Influence in Blog Networks. J. Comput. Med. Commun. 14, 682–707. doi: 10.1111/j.1083-6101.2009.01458.x
Miller, E. A., Simpson, E., Nadash, P., and Gusmano, M. (2021). Thrust into the spotlight: COVID-19 focuses media attention on nursing homes. J. Gerontol. Series B 76, e213–e218. doi: 10.1093/geronb/gbaa103
Minooie, M. (2019). Agendamelding: How audiences meld agendas in Iran. ASJ. 3, 139–164. doi: 10.1075/asj.18010.min
Minooie, M. (2021). Agendamelding: How Americans Meld Agendas. ASJ. 5, 177–204. doi: 10.1075/asj.21002.min
Muddiman, A., Budak, C., Romas, B., Kim, Y., Murray, C., Burniston, M. M., et al. (2020). Cable and Nightly Network News Coverage of Coronavirus. Center for Media Engagement.
Newmann, N., Dutton, W. H., and Blank, G. (2012). Social media in the changing ecology of news: The fourth and fifth estates in Britain. Int. J. Internet Sci. 7, 6–22. Available online at: https://www.ijis.net/ijis7_1/ijis7_1_newman_et_al_pre.html
O'Connor, B., Balasubramanyan, R., and Routledge, B. (2010). “From tweets to polls: linking text sentiment to public opinion time series,” in The Fourth International AAAI Conference on Weblogs and Social Media (Washington, DC: AAAI). doi: 10.1609/icwsm.v4i1.14031
Ogbodo, J. N., Onwe, E. C., Chukwu, J., Nwasum, C. J., Nwakpu, E. S., Nwankwo, S. U., et al. (2020). Communicating health crisis: a content analysis of global media framing of COVID-19. Health Promot. Perspect. 10, 257–269. doi: 10.34172/hpp.2020.40
Palm, R., Bolsen, T., and Kingsland, J. T. (2021). The effect of frames on COVID-19 vaccine hesitancy. Front. Polit. Sci. 3, 661257. doi: 10.3389/fpos.2021.661257
Puri, N., Coomes, E. A., Haghbayan, H., and Gunaratne, K. (2020). Social media and vaccine hesitancy: new updates for the era of COVID-19 and globalized infectious diseases. Human Vacc. Immunother. 16, 2586–2593. doi: 10.1080/21645515.2020.1780846
Rahman, K. (2021). Donald Trump Repeats “China Virus” Slur on Fox News on Same Night As Atlanta Shootings. Newsweek. Available online at: https://www.newsweek.com/donald-trump-said-china-virus-just-before-atlanta-shootings-1576756 (accessed January 05, 2021).
Reese, S. D. (2007). The framing project: A bridging model for media research revisited. J. Commun. 57, 148–154. doi: 10.1111/j.1460-2466.2006.00334.x
Riffe, D., Lacy, S., and Drager, M. W. (1996). Sample Size in Content Analysis of Weekly News Magazines. J. Mass Commun. Quart. 73, 635–644. doi: 10.1177/107769909607300310
Riffe, D., Lacy, S., and Fico, F. (2014). Analyzing Media Messages: Using Quantitative Content Analysis in Research. Third edition. New York: Routledge/Taylor and Francis Group. doi: 10.4324/9780203551691
Roberts, M., Wanta, W., and Dzwo, T.-H. (2002). Agenda setting and issue salience online. Commun. Res. 29, 452–465. doi: 10.1177/0093650202029004004
Roozenbeek, J., Schneider, C. R., Dryhurst, S., Kerr, J., Freeman, A. L. J., Recchia, G., et al. (2020). Susceptibility to misinformation about COVID-19 around the world. R. Soc. Open Sci. 7, 201199. doi: 10.1098/rsos.201199
Russell Neuman, W., Guggenheim, L., Mo Jang, S., and Bae, S. Y. (2014). The dynamics of public attention: agenda-setting theory meets big data: dynamics of public attention. J. Commun. 64, 193–214. doi: 10.1111/jcom.12088
Santanen, E. L., Briggs, R. O., and de Vreede, G.-J. (2000). “The cognitive network model of creativity: a new causal model of creativity and a new brainstorming technique,” in Proceedings of the 33rd Annual Hawaii International Conference on System Sciences (IEEE) 10.
Sayre, B., Bode, L., Shah, D., Wilcox, D., and Shah, C. (2010). Agenda setting in a digital age: Tracking attention to California Proposition 8 in social media, online news and conventional news. Policy Internet 2, 7–32. doi: 10.2202/1944-2866.1040
Scheufele, D. A., and Tewksbury, D. (2007). Framing, Agenda Setting, and Priming: The Evolution of Three Media Effects Models. J. Commun. 57, 9–20. doi: 10.1111/j.0021-9916.2007.00326.x
Schramm, W. (1963). The science of human communication: New directions and new findings in communication research. Basic Books.
Shaw, D. L., McCombs, M. E., Weaver, D. H., and Hamm, B. J. (1999). Individuals, groups, and agenda melding: A theory of social dissonance. Int. J. Public Opin. Res. 11, 2–24. doi: 10.1093/ijpor/11.1.2
Shaw, D. L., Minooie, M., Aikat, D., and Vargo, C. J. (2019). Agendamelding: News, Social Media, Audiences, and Civic Community. New York: Peter Lang. doi: 10.3726/b15023
Tankard, J., Hendrickson, L., Silberman, J., Bliss, K., and Ghanem, S. (1991). “Media frames: Approaches to conceptualization and measurement,” in Association for Education in Journalism and Mass Communication (Boston, MA).
Vargo, C. J. (2011). “Twitter as public salience: An agenda-setting analysis,” in AEJMC Annual Conference (St. Louis, MO: Association for Education in Journalism and Mass Communication).
Vargo, C. J., Guo, L., McCombs, M., and Shaw, D. L. (2014b). Network Issue Agendas on Twitter During the 2012 U.S. Presidential Election. J. Commun. 64, 296–316.
Vargo, C. J., Guo, L., McCombs, M. E., and Shaw, D. L. (2014a). Network issue agendas on Twitter during the 2012 U.S. Presidential Election: Network issue agendas on Twitter. J. Commun. 64, 296–316. doi: 10.1111/jcom.12089
Wanta, W. (2019). “Media in?uence on the public's perceptions of countries: Agenda-setting and international news,” in Bridging Disciplinary Perspectives of Country Image, Reputation, Brand, and Identity, eds. D. Ingenhoff, C. White, A. Buhmann, and S. Kiousis (New York, NY: Routledge) 252–263.
Weaver, D. H. (1980). Audience need for orientation and media effects. Commun. Res. 7, 361–373. doi: 10.1177/009365028000700305
Weaver, D. H. (2007). Thoughts on agenda setting, framing, and priming. J. Commun. 57, 142–147. doi: 10.1111/j.1460-2466.2006.00333.x
Weaver, D. H., Wojdynski, B., McKeever, R., and Shaw, D. L. (2010). “Vertical and or versus? Horizontal communities: Need for orientation, media use and agenda melding,” in Proceedings of the Annual Convention of the World Association for Public Opinion Research (Chicago, IL).
WHO (2020). International Health Regulations Emergency Committee on novel coronavirus in China. Available online at: https://www.who.int/docs/default-source/coronaviruse/transcripts/ihr-emergency-committee-for-pneumonia-due-to-the-novel-coronavirus-2019-ncov-press-briefing-transcript-23012020.pdf?sfvrsn=c1fd337e_2 (accessed January 04, 2021).
Wojcik, S., and Hughes, A. (2019). How Twitter Users Compare to the General Public. Pew Research Center: Internet, Science and Tech. Available online at: https://www.pewresearch.org/internet/2019/04/24/sizing-up-twitter-users/ (accessed February 25, 2022).
Keywords: agendamelding, agenda setting, COVID-19, pandemic, public opinion, social media, Twitter
Citation: Minooie M, Taylor JB and Vargo CJ (2023) Agendamelding and COVID-19: the dance of horizontal and vertical media in a pandemic. Front. Polit. Sci. 5:1021855. doi: 10.3389/fpos.2023.1021855
Received: 17 August 2022; Accepted: 12 May 2023;
Published: 31 May 2023.
Edited by:
Zoe Lefkofridi, University of Salzburg, AustriaReviewed by:
Sharon Meraz, International Union of Railways, FranceJunxiang Chen, University of Pittsburgh, United States
Tom Johnson, The University of Texas at Austin, United States
Copyright © 2023 Minooie, Taylor and Vargo. This is an open-access article distributed under the terms of the Creative Commons Attribution License (CC BY). The use, distribution or reproduction in other forums is permitted, provided the original author(s) and the copyright owner(s) are credited and that the original publication in this journal is cited, in accordance with accepted academic practice. No use, distribution or reproduction is permitted which does not comply with these terms.
*Correspondence: Milad Minooie, bW1pbm9vaWVAa2VubmVzYXcuZWR1