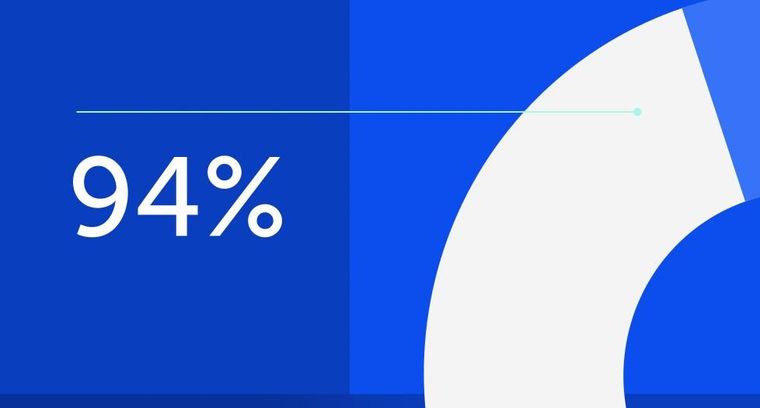
94% of researchers rate our articles as excellent or good
Learn more about the work of our research integrity team to safeguard the quality of each article we publish.
Find out more
ORIGINAL RESEARCH article
Front. Polit. Sci., 25 March 2022
Sec. Politics of Technology
Volume 4 - 2022 | https://doi.org/10.3389/fpos.2022.849763
This article is part of the Research TopicThe Politics of Digital MediaView all 6 articles
Several scholars have demonstrated a positive link between political polarization and the resistance to COVID-19 prevention measures. At the same time, political polarization has also been associated with the spread of misinformation. This study investigates the theoretical linkages between polarization and misinformation and measures the flow of misinformation about COVID-19 in the comment sections of four popular YouTube channels for over 16 months using big data sources and methods. For the analysis, we downloaded about 3.5M English language YouTube comments posted in response to videos about the pandemic. We then classified the comments into one of the two following categories by applying a supervised Natural Language Processing classifier: (1) fake: comments that contain claims and speculation which are verifiably not true; and (2) legitimate: comments that do not fall into the fake category. The results show that the level of misinformation in YouTube comment sections has increased during the pandemic, that fake comments attract statistically more likes, and that the ratio of fake comments increased by 0.4% per month. These findings suggest that once introduced into an online discussion, misinformation potentially leads to an escalating spiral of misinformation comments, which undermines public policy. Overall, the results signal alarming pandemic-related misinformation and, potentially, rising levels of affective polarization. We place these results in context and point out the limitations of our approach.
The COVID-19 pandemic has taken a tragic toll on human wellbeing and gave rise to a severe global economic contraction (Baker et al., 2020), renewed scientific controversies (Fleerackers et al., 2021), and a worldwide upsurge of negative emotions such as anger, fear, and trauma (Han et al., 2020; Shanahan et al., 2020; Trnka and Lorencova, 2020). While the pandemic wrought havoc on the economy and the health of individuals, it has also affected ongoing tendencies in politics and media consumption. For instance, there is evidence of an association between party affiliation and the willingness to be vaccinated against COVID-19 (Fridman et al., 2021). Partisanship also influences the implementation of and compliance with public health measures (Grossman et al., 2020; Adolph et al., 2021). In addition, media scholarship indicates that social media platforms, as a primary source of news about the pandemic (Cinelli et al., 2020), contribute to the dissemination of inaccurate information about the virus and the pandemic (Allington et al., 2021). In a similar vein, Bridgman et al. (2020) found evidence of a positive association between social media exposure and non-compliance with social distancing rules mediated by misperceptions about the pandemic.
Given these findings, social media platforms play an essential part in political factionalism and the expression of negative emotions, such as anger, that are associated with the spread of dubious information, COVID-19 misperceptions, and non-compliance with preventive measures (Garrett et al., 2019; Bridgman et al., 2020; Milosh et al., 2021). “To the degree that informational uses of social media promotes political participation, this increased participation can lead to the spread of misinformation” regarding governmental affairs, science, and natural disasters (Valenzuela et al., 2019). Therefore, it is indispensable to understand the state and dynamics of misinformation dissemination on social media platforms in critical times like these (Milosh et al., 2021).
For that reason, this paper examines the flow of COVID-19 related misinformation and its potentially polarization-induced nature. The study is structured as follows. First, we provide an overview of previous theory and empirical evidence about the causal role of anger and the mediating role of affective polarization on the spread of misinformation in conversations on social media platforms. Based on this overview, we formulate the research questions. After that, we present a unique data set containing 2.5M comments and 900k replies, downloaded via the official YouTube API from the comment section of four YouTube channels, including CNN, The Epoch Times, the World Health Organization, and Fox News. We then apply a deep neural network-based supervised Natural Language Processing (NLP) classifier to this data to classify user comments as legitimate or fake. Finally, we answer the research questions, contextualize the findings in the discussion, and point out the limitations of our approach.
The term fake news gained traction during the US presidential election in 2016 (Lazer et al., 2018; Van Duyn and Collier, 2019). However, the term predates this moment and has been used to describe various phenomena such as news satire, parody, fabrication, manipulation, advertising, and propaganda (Coe et al., 2014; Wardle and Derakhshan, 2017). Moreover, the use of the term by former US president Trump added a polemic meaning that is often used to discredit legacy news media (Quandt et al., 2019). The idea of fake news has also been employed to draw attention to the extensive spread of deceptive information in the form of misinformation, disinformation, hyperpartisan news, or conspiracy theories about the pandemic on social media platforms like Facebook, Twitter, YouTube, and Tiktok (Pennycook and Rand, 2021; Shahrezaye et al., 2021). In this study, when we use the term “fake news,” we refer to social media posts that make claims and put forward verifiably untrue speculations according to our analysis using a pre-trained BERT classifier. Additionally, a legitimate social media post is one that cannot be classified as fake (Patwa et al., 2021).
The spread of fake news via comments and videos (Li et al., 2020) on YouTube has been addressed in general as well as in the particular case of COVID-19. For instance, Davidson et al. (2017) used a combination of linguistic and parser features to identify misinformation comments on YouTube. Chen et al. (2012) reported the widespread occurrence of offensive comments on YouTube. Moreover, Serrano et al. (2020) showed that misinformation comments help to detect fake and conspiratorial videos about COVID-19 on YouTube. However, to the best of our knowledge, no study examined the pandemic's misinformation dynamics over an extended time period and on a large scale on the YouTube platform.
In the following paragraphs, we present three primary contributing factors that explain why people fall for misinformation and how online misinformation and polarization relate to each other.
Individuals don't necessarily update their perception about matters by assigning equal weight to various signals such as experts' opinions, scientific facts, or online news pieces. Based on identity protective cognition theory, the consistency of signals with existing values and cultural outlooks affects the weight or importance individuals attribute to signals when they update their perceptions (Pogarsky et al., 2017). In other words, people pursue evidence and signals that reinforce their group predisposition and culture. Identity protective cognition can partly explain the ideological conflicts and polarization over scientifically well-established matters like climate change, gun violence, and vaccination (Kahan, 2012). In addition, Van Bavel and Pereira (2018) used identity protective cognition to establish that individuals fail to discern misinformation because they place their political identity and predispositions above the inherent value of the evidence they are exposed to. Put differently, on average, people have a propensity to believe in news pieces congruent with their predispositions (Druckman and Bolsen, 2011).
Relying on partisan affection as a certain type of predisposition, Garrett et al. (2019) argued that selective media consumption leads to increased compliance with inter-group social norms and greater willingness to endorse negative stereotyping of the out-group, which provokes the spread of misperceptions. They claim that “affective polarization is likely to encourage both these behaviors: the more unfavorable the attitude toward the outgroup, the more the individual will want to promote the ingroup, and to reinforce his or her position within it.” Therefore, we argue that affective polarization is an important mediator linking partisan media exposure and the spread of misinformation.
However, two counterarguments challenge the scope and validity of this theorem. First, new empirical evidence suggests that “people are somewhat better at discerning truth from falsehood when [being asked to carefully judge] politically concordant news compared with politically discordant news” (Pennycook and Rand, 2021). This indicates that the effect of partisanship might not be as strong as previously thought. Second, a pattern of association between political identity and belief in specific ideas cannot be interpreted as a causal relationship because these variables are confounded with other latent variables and beliefs (Tappin et al., 2020).
People rely on various heuristic shortcuts when dealing with complex and emotional matters. One latent feature of news pieces that increases people's propensity to fall for misinformation is the source heuristic. Individuals are more likely to believe in the information provided by elites and politicians who hold congruent beliefs (Gallagher et al., 2021). For instance, attributing a false claim to former President Trump decreased Democrats' belief in that claim while increasing that of Republicans (Swire et al., 2017).
Additionally, positive social feedback measured by the number of likes and shares increases the probability of believing misinformation content regardless of who posted the content (Avram et al., 2020). Similarly, repetition or prior exposure to fake news affects individuals' belief in misinformation. “A single prior exposure to a fake news headline increases later belief in the headline. Remarkably, this is evident even if the headline is extremely implausible and inconsistent with one's political partisanship” (Pennycook and Rand, 2021).
Finally, there is what is called the emotionally evocative misinformation heuristic. Online moral outrage, online users shaming, and punishing supposed wrongdoers are an inevitable reality of online communication. Users “can express outrage online with just a few keystrokes, from the comfort of their bedrooms, either directly to the wrongdoer or to a broader audience. With even less effort, people can repost or react to others' angry comments. Because the tools for easily and quickly expressing outrage online are literally at our fingertips, a person's threshold for expressing outrage is probably lower online than offline” (Crockett, 2017). This may explain why misinformation content is often provocative, shocking, and intended to intensify anger (Gervais, 2015). Martel et al. (2020) found causal evidence that exposure to such morally and emotionally charged information increases belief in misinformation. Additionally, Weeks (2015) provided causal evidence that anger and resentment heighten the effect of partisan reasoning, leading to partisan processing of misinformation. Furthermore, he speculated that “political attitudes alone are not enough to drive partisan processing of misinformation, but rather attitudes that are tied to anger or resentment.” In other words, the existence of anger in combination with partisan affections may exacerbate the propensity of forming misconceptions and spreading misinformation.
Scholars have established that the levels of polarization about issues related to COVID-19 have increased in many countries. For instance, Sides et al. (2020) investigated more than 400,000 interviews covering all US states for a period longer than a year and concluded that the “partisan divide open[ed] up as Republican concern and support [for COVID-19 containment measures] drop[ped] more quickly.” In a study of self-identified Democrats and Republicans, Fridman et al. (2021) observed an asymmetric polarization in the USA between March and August 2020 concerning vaccination hesitancy. Similarly, prior studies have shown that in various countries, the level of negative emotions, such as fear and anxiety, has increased as the pandemic, the preventive measures, and negative economic effects prolonged (Benke et al., 2020; Shanahan et al., 2020; Smith et al., 2021; Manchia et al., 2022).
Therefore, based on the articulated association between polarization, affect, and misinformation, we expect the levels of pandemic-related misinformation to follow an upward trend. However, the literature shows contradictory results. For instance, employing a survey of 1050 Americans, Romer and Jamieson (2020) showed that belief in misinformation about the pandemic and in conspiracy theories remained stable between March and July 2020. But belief in pandemic-related misinformation and conspiracy theories grew in November 2020 and afterward, which might have occurred due to the emergence of mass vaccination drives as a salient issue in media reports at that time (Hornsey et al., 2021; Islam et al., 2021). Thus, existing evidence about the prevalence of misinformation is inconclusive mainly because the findings of prior studies are limited by a small number of observations and a short time frame of analysis.
In contrast to prior studies, we will rely on a large-scale dataset of millions of wild non-experimental social postings collected over a time span longer than a year to test if the prevalence of misinformation has increased. Due to a rise in polarization and because of the negative emotions incited by the pandemic, we expect an increase in misinformation. As part of the analysis, we will also measure what portion of the information shared in the comment sections contains misinformation.
RQ1: What percentage of YouTube comments constitute fake content?
RQ2: Did the level of misinformation increase as the pandemic has progressed?
In addition to these questions, we will examine the extent of engagement misinformation creates. Because misinformation tends to be controversial or contain emotionally charged messages, we assume that comments which contain misinformation attract more engagement in terms of likes and responses. An additional research question follows from this:
RQ3: Do misinformation comments create more engagement than legitimate comments?
Video messages as a medium of communication have been extensively used throughout the pandemic (Covolo et al., 2017; Li et al., 2020; Serrano et al., 2020). Because new media are often characterized by their interactive possibilities, researchers have increasingly focused on commenting features and their impact on user attitudes and behaviors (Su et al., 2018). Such features enable users of social media platforms to express their opinions, find out about the viewpoints of others and participate in the production and interpretation of news (Stroud et al., 2016). At the same time, YouTube has been implicated in the spread of misinformation and polarization (Ribeiro et al., 2020). These circumstances make YouTube a particularly suitable case to analyze.
YouTube API offers a search functionality to look for videos with specific keywords in their title or description. However, this function returns only the most recent videos and is not practical when searching for older videos. Therefore, we relied on the Crowdtangle API (Silverman, 2019) and looked for the Facebook posts with YouTube videos with one of the following COVID-19 pandemic related keywords in their Facebook text: “pandemic,” “hygiene,” “health,” “lockdown,” “school,” “Fauci,” “covid,” “corona,” “vaccine,” “virus,” “AstraZeneca,” “Pfizer,” “BioNTech,” “Johnson,” “J&J,” and “Wuhan.”
Then, we filtered out YouTube videos posted after the 11th of March 2020, the day the World Health Organization declared the spread of the virus a pandemic, by the following YouTube channels: “CNN,” “The Epoch Times,” “World Health Organization (WHO),” “Fox News.” We chose these channels because their pandemic-related videos reached a wide audience on other social media platforms, such as Facebook, and they represent different degrees of partisanship across the political spectrum (Morris, 2005). Furthermore, the comment sections of these channels have been open for comments by the public during the pandemic.
We selected videos containing at least one of the keywords mentioned above in their YouTube title or YouTube description. The final list includes 1,565 videos posted by one of the four YouTube channels between the 11th of March 2020 and the 29th of July 2021. As a next step, we used the YouTube Data API1 to download the comments posted in the comment section belonging to the videos. Table 1 shows some simple statistics on the data.
The scale of this study and the size of its data set prohibit a manual approach to data analysis and make probabilistic deep learning methods the most viable option available. Supervised language-based classification methods are implemented widely in similar fake news and misinformation detection studies (Singhania et al., 2017; Kaliyar et al., 2020; Chen et al., 2021). In the context of pandemic-related misinformation, Serrano et al. (2020) used supervised language-based classification methods to classify YouTube comments into fake or legitimate categories. Furthermore, they used this information to group COVID-19 YouTube videos into misinformation or factual classes with an accuracy of 89.4%. Das et al. (2021) relied on an ensemble of supervised language-based classifiers and achieved 98% accuracy in detecting pandemic-related misinformation in social media posts.
In line with Serrano et al. (2020) and Das et al. (2021), this study relies on a baseline neural language modeling method to train a supervised text classifier. Specifically, we use Bidirectional Encoder Representations from Transformers (BERT) (Devlin et al., 2018) to train a fake COVID-19 comment classifier. BERT classifiers generally do better than most other methods for classifying text data (Reveilhac and Morselli, 2022). Additionally, text classification based on the BERT model requires no text cleaning as this model can process stop words. For the details about this model, we refer interested readers to Gupta et al. (2020) and Serrano et al. (2020). We used 10,700 manually labeled COVID-19 fake news social media posts as the training data set (Patwa et al., 2021). Additionally, we enriched this data set by combining it with Serrano et al.'s (2020) conspiratorial YouTube comments. The final dataset included 11,771 social media posts related to misinformation about COVID-19, 4,798 of which were labeled as misinformation.
It is essential to get a good grasp of what information our classifier interprets as fake and legitimate. Patwa et al. (2021) collected social posts from sources like Facebook, Instagram, news articles, and other public sources that fact-checking websites, such as PolitiFact, Snopes, and Boomlive, have tagged as fake or “verified to be not true.” We excerpt the following table from Patwa et al. (2021) (Table 2) to provide an illustrative overview.
We trained the BERT-based classifier on 90% of the manually labeled data and tested its performance on the other 10% out-of-bag sample to examine the accuracy of the classifier. We could achieve 91% accuracy of prediction using a baseline BERT classifier (Table 3). Table 4 shows 10 of the YouTube comments classified as fake using our BERT classifier. The highlighted ones are those that the classifier has classified mistakenly.
Table 4. Sample fake YouTube comments classified using our BERT classifier (highlighted = wrong classification).
RQ1: To answer the first research question, we applied the pre-trained BERT classifier to the retrieved YouTube comments and to the replies to these comments. Overall, the classifier categorized about 21% of the comments and 15% of the replies as fake. Among the four channels studied, the World Health Organization received the highest ratio of fake comments followed by CNN and Fox News. The Epoch Times received the fewest fake comments. This pattern replicates for the replies, although the ratio of misinformation in replies is lower than that in comments for all four channels. Figure 1 below illustrates these findings.
Figure 1. The ratio of misinformation in (A) comments and (B) replies (legitimate = class 0, fake = class 1).
RQ2: This research question addresses the level of misinformation and its development during the time of observation. We computed the monthly average proportion of fake comments for all users. This statistic is defined in two steps. First, we calculated the monthly number of fake comments divided by the number of all comments for each user. We then averaged these values over all the users. This statistic is based on the comments only and ignores the replies because responses are a function of the original comment. Including responses would thus bias the results. Figure 2A depicts the development of the mean users' ratio of fake comments during the measurement period. The regression results show a statistically significant coefficient of 0.004, implying that the portion of misinformation increases by a rate of 0.4% per month (see Table 5).
Figure 2. Misinformation timelines: (A) average proportion of fake comments per user and month (averaged over all users) and (B) ratio of the number of users posting only fake comments to those posting only legitimate comments. The gray areas represent the 95% confidence interval.
Furthermore, we computed the monthly ratio of the number of users who only posted misinformation to those who only post legitimate information. Figure 2B visualizes the timeline of this ratio. Based on the regression results, the ratio of the number of users posting only fake comments to those posting only legitimate comments increased, on average, by 0.5% per month (Table 6).
Table 6. Report of regressing proportion of the number of users posting only fake comments against time.
RQ3: To address research question 3, we used a t-test and compared the average likes of fake comments to the likes received by legitimate comments. Fake comments collect 5.753 likes on average compared to legitimate comments that receive 5.626 likes. Based on the one-sided t-test results, fake comments receive statistically more likes than legitimate comments. In addition, we tested the assumption that misinformed comments are more likely to attract replies that contain misinformation than comments that contain no misinformation. Figure 3 provides visual evidence that supports the plausibility of this assumption. That is, silhouette (B) in Figure 3 is fatter than silhouette (A). We used a one-sided t-test to verify that the average ratio of fake replies to fake comments (0.21%) is statistically notably larger than the average ratio of fake replies to legitimate comments (0.13%).
Finally, we regressed the total number of comments against the number of fake comments for each video to test if the proportion of fake comments increases as the discussions get more involved. Figure 4 shows the results in the logarithmic scale. Table 7 summarizes the coefficients of the log-log regression models (we excluded the intercepts).
As Figure 4, Table 7 depict, we can observe that the absolute number of fake comments increases as the total number of comments increases. In more detail, Table 7 demonstrates that an increase of 1% in the number of comments on videos of the Epoch Times YouTube channel, on average, leads to a 1.05% increase in the number of fake comments. WHO, CNN, and Fox News show an opposite trend and are in the second to fourth order considering this statistic. These results are partly in contrast to what Coe et al. (2014) underlined. Analyzing newspaper website comments, they suggested that “the frequency of incivility [is] not associated with the number of comments made during a given discussion.” However, The Epoch Times represents a significantly positive association.
Public discourse has always had its share of misinformation and misperception. Still, the digitization of communication and the proliferation of social media platforms in the 21st century have increased the speed and expanded the dissemination boundaries (McIntyre, 2018), exacerbating the potential social and political damage of misinformation. Many scholars have portrayed the deleterious nature of this phenomenon. For instance, Anderson et al. (2014), in their pioneering work, introduced the concept of the “nasty effect,” which demonstrates that “exposure to uncivil blog comments can polarize risk perceptions of [unfamiliar issues] along the lines of religiosity and issue support.” Furthermore, uncivil comments can also lead to the impression of more social polarization and segregation (Hwang et al., 2014). Additionally, higher narrow-mindedness and less satisfaction with online discussions are among the other harmful consequences of the spread of uncivil comments on social media platforms (Gervais, 2015, 2017).
In extreme situations, such as the COVID-19 pandemic, distorting information and the promotion of misinformation and polarizing narratives on social media platforms endanger the public's collective health and cause considerable uncertainty and anger (Han et al., 2020; Milosh et al., 2021). Bridgman et al. (2020) established that “misperceptions regarding the virus are in turn associated with less compliance with social distancing measures, even when controlling for a broad range of other attitudes and characteristics. [...] Association between social media exposure and social distancing non-compliance is eliminated when accounting for the effect of misperceptions, providing evidence that social media is associated with non-compliance through increasing misperceptions about the virus.”
To shed light on the spread of pandemic-related misinformation on the YouTube platform, this study examined the spread of misinformation about COVID-19 in the comment section of four YouTube channels with a data set of 2.5M comments and 900k replies. To phrase the research questions, we called on prior literature about the relationship between misinformation and polarization. We then applied a supervised NLP classifier to the data and found the following. Depending on the YouTube channel, we observed that between 16% and 25% of comments contained misinformation and that between 13 and 19% of replies included misinformation. Most notably, we provide evidence for a steep increase of misinformation in discussions about COVID-19 on YouTube, illustrated by two main findings. First, the portion of misinformation increased by a rate of 0.4% per month. Second, the number of users that post misinformation only has increased by 0.5% each month. Consistent with our expectations, we also find that fake comments receive more attention and attract more fake replies than factual comments.
Among other things, these results are noteworthy because they imply that users flock to politically moderate outlets to spread misinformation. The YouTube channel run by the WHO and CNN attracted the most misinformation. In contrast, Fox News and The Epoch Times, two staunchly partisan outlets, attract considerably less misinformation. This effect may come about because partisan social media users produce more misinformation content (Grinberg et al., 2019) and tend to post it to express dissatisfaction (Chipidza, 2021). Even though the four channels under consideration do not allow for widely generalizable conclusions, these results are noteworthy because they highlight the role that misinformation plays in challenging the legitimacy of news outlets, especially of politically moderate outlets like the WHO or CNN.
As discussed earlier, the ratio of the number of users posting sheer misinformation comments to those who post exclusively legitimate comments has increased by 0.5% each month. Three potential reasons may explain this development. First, a rich body of literature shows a positive association between misinformation content on social media and polarization (Sobieraj and Berry, 2011; Hwang et al., 2014; Jiang et al., 2020; Shahrezaye et al., 2021). The emergence of new conspiracy theories, such as those about vaccines (Hornsey et al., 2021; Islam et al., 2021), might have increased the belief in misinformation about the pandemic and, accordingly, affective polarization. Second, new evidence shows that the YouTube search algorithm and other social media platforms amplify political extremism (Ribeiro et al., 2020; Huszár et al., 2021; Kolomeets and Chechulin, 2021). This kind of algorithmic bias may steer users toward higher levels of polarization as time passes. Third, individuals have different threshold levels for mobilization. While relatively few users with low thresholds mobilize against a particular topic at the earliest stages, large parts of users with higher thresholds slowly activate as the viability signals of mobilization increase (Margetts et al., 2015). We speculate that, with the passage of time, prolonged exposure to discussions on social media platforms that include misinformation serves as strong cues to incrementally mobilize against public health measures.
Our study relies heavily on the BERT-based supervised classifier that achieved an accuracy of 91% on the test sample. Further research could rely on ensemble methods (Das et al., 2021) or dictionary-based approaches (Reveilhac and Morselli, 2022) to improve the accuracy of the results. Additionally, our classifier uses a combination of two data sets (Serrano et al., 2020; Patwa et al., 2021) to train the fake comment classifier. Both of these data sets were compiled in the early days of the pandemic and, therefore, do not include any training data on recent COVID-19 misinformation and conspiracy theories. Additionally, we did not distinguish between bots or automated accounts and human users (Kolomeets and Chechulin, 2021). Future research might use more current training data and may distinguish between comments posted by humans and by bots to ameliorate any inaccuracies resulting from this. Furthermore, our results apply to English-speaking YouTube audiences only. Future research could compare other social media platforms and online discussions in different languages. Such an approach might shed light on the effect of platform affordances and of cultural contingencies on misinformation and may provide more empirical evidence to improve our understanding of misinformation and polarization about the pandemic. Finally, because this is a single-platform study based on data from one social network and deliberately examines the divisive issue of COVID-19, we need to be cautious about generalizing to whole populations. Discussions on other social media platforms and about other less divisive topics might be subject to different dynamics.
The raw data supporting the conclusions of this article will be made available by the authors, without undue reservation.
MS: conceptualization, methodology, investigation, data curation, formal analysis, visualization, and writing—original draft. VS: conceptualization, investigation, project administration, formal analysis, and writing—original draft. MM: conceptualization, supervision, and writing—reviewing and editing. All authors contributed to the article and approved the submitted version.
The authors declare that the research was conducted in the absence of any commercial or financial relationships that could be construed as a potential conflict of interest.
All claims expressed in this article are solely those of the authors and do not necessarily represent those of their affiliated organizations, or those of the publisher, the editors and the reviewers. Any product that may be evaluated in this article, or claim that may be made by its manufacturer, is not guaranteed or endorsed by the publisher.
Adolph, C., Amano, K., Bang-Jensen, B., Fullman, N., and Wilkerson, J. (2021). Pandemic politics: Timing state-level social distancing responses to COVID-19. J. Health Polit. Policy Law 46, 211–233. doi: 10.1215/03616878-8802162
Allington, D., Duffy, B., Wessely, S., Dhavan, N., and Rubin, J. (2021). Health-protective behavior, social media usage and conspiracy belief during the COVID-19 public health emergency. Psychol. Med. 51, 1763–1769. doi: 10.1017/S003329172000224X
Anderson, A. A., Brossard, D., Scheufele, D. A., Xenos, M. A., and Ladwig, P. (2014). The “nasty effect:” online incivility and risk perceptions of emerging technologies. J. Computer-Med. Commun. 19, 373–387. doi: 10.1111/jcc4.12009
Avram, M., Micallef, N., Patil, S., and Menczer, F. (2020). Exposure to social engagement metrics increases vulnerability to misinformation. arXiv[Preprint]. arXiv:2005.04682. doi: 10.37016/mr-2020-033
Baker, S. R., Bloom, N., Davis, S. J., and Terry, S. J. (2020). Covid-Induced Economic Uncertainty (No. w26983). Cambridge, MA: National Bureau of Economic Research. doi: 10.3386/w26983
Benke, C., Autenrieth, L. K., Asselmann, E., and Pané-Farré, C. A. (2020). Lockdown, quarantine measures, and social distancing: associations with depression, anxiety and distress at the beginning of the COVID-19 pandemic among adults from Germany. Psychiatry Res. 293, 113462. doi: 10.1016/j.psychres.2020.113462
Bridgman, A., Merkley, E., Loewen, P. J., Owen, T., Ruths, D., Teichmann, L., et al. (2020). The causes and consequences of COVID-19 misperceptions: understanding the role of news and social media. Harvard Kennedy Schl. Misinform. Rev. 1. doi: 10.37016/mr-2020-028
Chen, B., Chen, B., Gao, D., Chen, Q., Huo, C., Meng, X., et al. (2021). “Transformer-based language model fine-tuning methods for covid-19 fake news detection,” in International Workshop on Combating Online Hostile Posts in Regional Languages during Emergency Situation (Cham: Springer), 83–92. doi: 10.1007/978-3-030-73696-5_9
Chen, Y., Zhou, Y., Zhu, S., and Xu, H. (2012). “Detecting offensive language in social media to protect adolescent online safety,” in 2012 International Conference on Privacy, Security, Risk and Trust and 2012 International Conference on Social Computing (Amsterdam: IEEE), 71–80. doi: 10.1109/SocialCom-PASSAT.2012.55
Chipidza, W. (2021). The effect of toxicity on COVID-19 news network formation in political subcommunities on Reddit: an affiliation network approach. Int. J. Inf. Manage. 61, 102397. doi: 10.1016/j.ijinfomgt.2021.102397
Cinelli, M., Quattrociocchi, W., Galeazzi, A., Valensise, C. M., Brugnoli, E., Schmidt, A. L., et al. (2020). The COVID-19 social media infodemic. Sci. Rep. 10, 16598. doi: 10.1038/s41598-020-73510-5
Coe, K., Kenski, K., and Rains, S. A. (2014). Online and uncivil? Patterns and determinants of incivility in newspaper website comments. J. Commun. 64, 658–679. doi: 10.1111/jcom.12104
Covolo, L., Ceretti, E., Passeri, C., Boletti, M., and Gelatti, U. (2017). What arguments on vaccinations run through YouTube videos in Italy? A content analysis. Human Vaccines Immunotherap. 13, 1693–1699. doi: 10.1080/21645515.2017.1306159
Crockett, M. J. (2017). Moral outrage in the digital age. Nat. Human Behav. 1, 769–771. doi: 10.1038/s41562-017-0213-3
Das, S. D., Basak, A., and Dutta, S. (2021). A heuristic-driven ensemble framework for covid-19 fake news detection. arXiv[Preprint]. arXiv:2101.03545. doi: 10.1007/978-3-030-73696-5_16
Davidson, T., Warmsley, D., Macy, M., and Weber, I. (2017). “Automated hate speech detection and the problem of offensive language,” in Proceedings of the International AAAI Conference on Web and Social Media, Vol. 11 (Montréal, QC).
Devlin, J., Chang, M. W., Lee, K., and Toutanova, K. (2018). Bert: pre-training of deep bidirectional transformers for language understanding. arXiv[Preprint]. arXiv:1810.04805. doi: 10.48550/arXiv.1810.04805
Druckman, J. N., and Bolsen, T. (2011). Framing, motivated reasoning, and opinions about emergent technologies. J. Commun. 61, 659–688. doi: 10.1111/j.1460-2466.2011.01562.x
Fleerackers, A., Riedlinger, M., Moorhead, L., Ahmed, R., and Alperin, J. P. (2021). Communicating scientific uncertainty in an age of COVID-19: an investigation into the use of preprints by digital media outlets. Health Commun. 1–13. doi: 10.1080/10410236.2020.1864892
Fridman, A., Gershon, R., and Gneezy, A. (2021). COVID-19 and vaccine hesitancy: a longitudinal study. PLoS ONE 16, e0250123. doi: 10.1371/journal.pone.0250123
Gallagher, R. J., Doroshenko, L., Shugars, S., Lazer, D., and Foucault Welles, B. (2021). Sustained online amplification of COVID-19 elites in the United States. Soc. Media+ Soc. 7, 20563051211024957. doi: 10.1177/20563051211024957
Garrett, R. K., Long, J. A., and Jeong, M. S. (2019). From partisan media to misperception: affective polarization as mediator. J. Commun. 69, 490–512. doi: 10.1093/joc/jqz028
Gervais, B. T. (2015). Incivility online: affective and behavioral reactions to uncivil political posts in a web-based experiment. J. Inform. Technol. Politics 12, 167–185. doi: 10.1080/19331681.2014.997416
Gervais, B. T. (2017). More than mimicry? The role of anger in uncivil reactions to elite political incivility. Int. J. Public Opinion Res. 29, 384–405. doi: 10.1093/ijpor/edw010
Grinberg, N., Joseph, K., Friedland, L., Swire-Thompson, B., and Lazer, D. (2019). Fake news on Twitter during the 2016 US presidential election. Science 363, 374–378. doi: 10.1126/science.aau2706
Grossman, G., Kim, S., Rexer, J. M., and Thirumurthy, H. (2020). Political partisanship influences behavioral responses to governors' recommendations for COVID-19 prevention in the United States. Proc. Nat. Acad. Sci. U.S.A. 117, 24144–24153. doi: 10.1073/pnas.2007835117
Gupta, S., Bolden, S., Kachhadia, J., Korsunska, A., and Stromer-Galley, J. (2020). “PoliBERT: classifying political social media messages with BERT,” in Social, Cultural and Behavioral Modeling (SBP-BRIMS 2020) Conference (Washington, DC).
Han, J., Cha, M., and Lee, W. (2020). Anger contributes to the spread of COVID-19 misinformation. Harvard Kennedy Schl. Misinform. Rev. 1. doi: 10.37016/mr-2020-39
Hornsey, M. J., Chapman, C. M., Alvarez, B., Bentley, S., Salvador Casara, B. G., Crimston, C. R., et al. (2021). To what extent are conspiracy theorists concerned for self versus others? A COVID-19 test case. Europ. J. Soc. Psychol. 51, 285–293. doi: 10.1002/ejsp.2737
Huszár, F., Ktena, S. I., O'Brien, C., Belli, L., Schlaikjer, A., and Hardt, M. (2021). Algorithmic amplification of politics on Twitter. arXiv[Preprint]. arXiv:2110.11010. doi: 10.1073/pnas.2025334119
Hwang, H., Kim, Y., and Huh, C. U. (2014). Seeing is believing: effects of uncivil online debate on political polarization and expectations of deliberation. J. Broadcast. Electron. Media 58, 621–633. doi: 10.1080/08838151.2014.966365
Islam, M. S., Kamal, A. H. M., Kabir, A., Southern, D. L., Khan, S. H., Hasan, S. M., et al. (2021). COVID-19 vaccine rumors and conspiracy theories: the need for cognitive inoculation against misinformation to improve vaccine adherence. PLoS ONE 16, e0251605. doi: 10.1371/journal.pone.0251605
Jiang, J., Chen, E., Yan, S., Lerman, K., and Ferrara, E. (2020). Political polarization drives online conversations about COVID-19 in the United States. Human Behav. Emerg. Technol. 2, 200–211. doi: 10.1002/hbe2.202
Kahan, D. M. (2012). Ideology, motivated reasoning, and cognitive reflection: an experimental study. Judgm. Decis. Mak. 8, 407–424. doi: 10.2139/ssrn.2182588
Kaliyar, R. K., Goswami, A., Narang, P., and Sinha, S. (2020). FNDNet-a deep convolutional neural network for fake news detection. Cogn. Syst. Res. 61, 32–44. doi: 10.1016/j.cogsys.2019.12.005
Kolomeets, M., and Chechulin, A. (2021). “Analysis of the malicious bots market,” in 2021 29th Conference of Open Innovations Association (FRUCT) (Tampere: IEEE), 199–205. doi: 10.23919/FRUCT52173.2021.9435421
Lazer, D. M., Baum, M. A., Benkler, Y., Berinsky, A. J., Greenhill, K. M., Menczer, F., et al. (2018). The science of fake news. Science 359, 1094–1096. doi: 10.1126/science.aao2998
Li, H. O. Y., Bailey, A., Huynh, D., and Chan, J. (2020). YouTube as a source of information on COVID-19: a pandemic of misinformation?. BMJ Global Health 5, e002604. doi: 10.1136/bmjgh-2020-002604
Manchia, M., Gathier, A. W., Yapici-Eser, H., Schmidt, M. V., de Quervain, D., van Amelsvoort, T., et al. (2022). The impact of the prolonged COVID-19 pandemic on stress resilience and mental health: a critical review across waves. Europ. Neuropsychopharmacol. 55, 22–83. doi: 10.1016/j.euroneuro.2021.10.864
Margetts, H., John, P., Hale, S., and Yasseri, T. (2015). Political Turbulence: How Social Media Shape Collective Action. Princeton, NJ: Princeton University Press. doi: 10.2307/j.ctvc773c7
Martel, C., Pennycook, G., and Rand, D. G. (2020). Reliance on emotion promotes belief in fake news. Cogn. Res. 5, 1–20. doi: 10.1186/s41235-020-00252-3
Milosh, M., Painter, M., Sonin, K., Van Dijcke, D., and Wright, A. L. (2021). Unmasking partisanship: Polarization undermines public response to collective risk. J. Public Econ. 204:104538. doi: 10.1016/j.jpubeco.2021.104538
Morris, J. S. (2005). The Fox news factor. Harvard Int. J. Press/Politics 10, 56–79. doi: 10.1177/1081180X05279264
Patwa, P., Sharma, S., Pykl, S., Guptha, V., Kumari, G., Akhtar, M. S., et al. (2021). “Fighting an infodemic: Covid-19 fake news dataset,” in International Workshop on Combating Online Hostile Posts in Regional Languages During Emergency Situation (Cham: Springer), 21–29. doi: 10.1007/978-3-030-73696-5_3
Pennycook, G., and Rand, D. G. (2021). The psychology of fake news. Trends Cogn. Sci. 25, 388–402. doi: 10.1016/j.tics.2021.02.007
Pogarsky, G., Roche, S. P., and Pickett, J. T. (2017). Heuristics and biases, rational choice, and sanction perceptions. Criminology 55, 85–111. doi: 10.1111/1745-9125.12129
Quandt, T., Frischlich, L., Boberg, S., and Schatto-Eckrodt, T. (2019). “Fake News,” in The International Encyclopedia of Journalism Studies, eds T. P. Vos, F. Hanusch, D. Dimitrakopoulou, M. Geertsema-Sligh, and A. Sehl, 1st ed. (Wiley), 1–6. doi: 10.1002/9781118841570.iejs0128
Reveilhac, M., and Morselli, D. (2022). Dictionary-based and machine learning classification approaches: a comparison for tonality and frame detection on Twitter data. Political Res. Exchange 4, 2029217. doi: 10.1080/2474736X.2022.2029217
Ribeiro, M. H., Ottoni, R., West, R., Almeida, V. A., and Meira, W. Jr. (2020). “Auditing radicalization pathways on YouTube,” in Proceedings of the 2020 Conference on Fairness, Accountability, and Transparency (Barcelona), 131–141. doi: 10.1145/3351095.3372879
Romer, D., and Jamieson, K. H. (2020). Conspiracy theories as barriers to controlling the spread of COVID-19 in the US. Soc. Sci. Med. 263, 113356. doi: 10.1016/j.socscimed.2020.113356
Serrano, J. C. M., Papakyriakopoulos, O., and Hegelich, S. (2020). “NLP-based feature extraction for the detection of COVID-19 misinformation videos on YouTube,” in Proceedings of the 1st Workshop on NLP for COVID-19 at ACL 2020 (Online).
Shahrezaye, M., Meckel, M., Steinacker, L., and Suter, V. (2021). “COVID-19's (mis) information ecosystem on Twitter: how partisanship boosts the spread of conspiracy narratives on German speaking Twitter,” in Future of Information and Communication Conference (Cham: Springer), 1060–1073. doi: 10.1007/978-3-030-73100-7_73
Shanahan, L., Steinhoff, A., Bechtiger, L., Murray, A. L., Nivette, A., Hepp, U., et al. (2020). Emotional distress in young adults during the COVID-19 pandemic: evidence of risk and resilience from a longitudinal cohort study. Psychol. Med. 1–10. doi: 10.1017/S003329172000241X
Sides, J., Tausanovitch, C., and Vavreck, L. (2020). The politics of covid-19: partisan polarization about the pandemic has increased, but support for health care reform hasn't moved at all. Harv. Data Sci. Rev. doi: 10.1162/99608f92.611350fd
Silverman, B. (2019). CrowdTangle for Academics and Researchers. Available online at: https://www.facebook.com/formedia/blog/crowdtangle-for-academics-and-researchers
Singhania, S., Fernandez, N., and Rao, S. (2017). “3han: A deep neural network for fake news detection,” in International Conference on Neural Information Processing (Cham: Springer), 572–581. doi: 10.1007/978-3-319-70096-0_59
Smith, L. E., Duffy, B., Moxham-Hall, V., Strang, L., Wessely, S., and Rubin, G. J. (2021). Anger and confrontation during the COVID-19 pandemic: a national cross-sectional survey in the UK. J. R. Soc. Med. 114, 77–90. doi: 10.1177/0141076820962068
Sobieraj, S., and Berry, J. M. (2011). From incivility to outrage: political discourse in blogs, talk radio, and cable news. Political Commun. 28, 19–41. doi: 10.1080/10584609.2010.542360
Stroud, N. J., Van Duyn, E., and Peacock, C. (2016). News Commenters and News Comment Readers. Available online at: https://mediaengagement.org/wp-content/uploads/2016/03/ENP-News-Commenters-and-Comment-Readers1.pdf
Su, L. Y.-F., Xenos, M. A., Rose, K. M., Wirz, C., Scheufele, D. A., and Brossard, D. (2018). Uncivil and personal? Comparing patterns of incivility in comments on the Facebook pages of news outlets. New Media Soc. 20, 3678–3699. doi: 10.1177/1461444818757205
Swire, B., Berinsky, A. J., Lewandowsky, S., and Ecker, U. K. (2017). Processing political misinformation: comprehending the Trump phenomenon. R. Soc. Open Sci. 4, 160802. doi: 10.1098/rsos.160802
Tappin, B. M., Pennycook, G., and Rand, D. G. (2020). Thinking clearly about causal inferences of politically motivated reasoning: why paradigmatic study designs often undermine causal inference. Curr. Opin. Behav. Sci. 34, 81–87. doi: 10.1016/j.cobeha.2020.01.003
Trnka, R., and Lorencova, R. (2020). Fear, anger, and media-induced trauma during the outbreak of COVID-19 in the Czech Republic. Psychol. Trauma 12, 546. doi: 10.1037/tra0000675
Valenzuela, S., Halpern, D., Katz, J. E., and Miranda, J. P. (2019). The paradox of participation versus misinformation: social media, political engagement, and the spread of misinformation. Digital Journalism 7, 802–823. doi: 10.1080/21670811.2019.1623701
Van Bavel, J. J., and Pereira, A. (2018). The partisan brain: an identity-based model of political belief. Trends Cogn. Sci. 22, 213–224. doi: 10.1016/j.tics.2018.01.004
Van Duyn, E., and Collier, J. (2019). Priming and fake news: the effects of elite discourse on evaluations of news media. Mass Commun. Soc. 22, 29–48. doi: 10.1080/15205436.2018.1511807
Wardle, C., and Derakhshan, H. (2017). Information disorder: toward an interdisciplinary framework for research and policy making (DGI(2017)09). Council Europe. Available online at: https://coinform.eu/wp-content/uploads/2019/02/Information-Disorder.pdf
Keywords: misinformation, affective polarization, fake news, pandemic, computational social science, social media
Citation: Suter V, Shahrezaye M and Meckel M (2022) COVID-19 Induced Misinformation on YouTube: An Analysis of User Commentary. Front. Polit. Sci. 4:849763. doi: 10.3389/fpos.2022.849763
Received: 06 January 2022; Accepted: 03 March 2022;
Published: 25 March 2022.
Edited by:
Roxana Radu, University of Oxford, United KingdomReviewed by:
Bumsoo Kim, Joongbu University, South KoreaCopyright © 2022 Suter, Shahrezaye and Meckel. This is an open-access article distributed under the terms of the Creative Commons Attribution License (CC BY). The use, distribution or reproduction in other forums is permitted, provided the original author(s) and the copyright owner(s) are credited and that the original publication in this journal is cited, in accordance with accepted academic practice. No use, distribution or reproduction is permitted which does not comply with these terms.
*Correspondence: Viktor Suter, dmlrdG9yLnN1dGVyQHVuaXNnLmNo
Disclaimer: All claims expressed in this article are solely those of the authors and do not necessarily represent those of their affiliated organizations, or those of the publisher, the editors and the reviewers. Any product that may be evaluated in this article or claim that may be made by its manufacturer is not guaranteed or endorsed by the publisher.
Research integrity at Frontiers
Learn more about the work of our research integrity team to safeguard the quality of each article we publish.