- 1National Forestry and Grassland Administration Engineering Research Centre for Southwest Forest and Grassland Fire Ecological Prevention, College of Forestry, Sichuan Agricultural University, Chengdu, China
- 2National Forestry and Grassland Administration Key Laboratory of Forest Resources Conservation and Ecological Safety on the Upper Reaches of the Yangtze River and Forestry Ecological Engineering in the Upper Reaches of the Yangtze River Key Laboratory of Sichuan Province, Chengdu, China
Pinus yunnanensis Franch. is a native species in southwestern China, characterized by high polymorphism. However, the environmental drivers of intraspecific variation in its functional traits remain poorly understood. In this study, we examined the relationships between five functional traits (bark thickness, tree height, leaf dry matter content, leaf length, and specific leaf area) and habitat conditions across 20 populations, representing three varieties: var. yunnanensis (the original variety), var. pygmaea, and var. tenuifolia. Our experiments aimed to determine whether the functional traits varied among the three varieties under different environmental conditions. As specific leaf area and leaf dry matter content showed no significant correlations with any environmental factors, we focused our analysis on the remaining three traits. Using random forest models, we assessed the significance of each environmental factor and found the following: Temperature seasonality was a key determinant of tree height; soil particle size (clay and sand) had the strongest influence on bark thickness; and for leaf length, precipitation during the driest quarter was the most important factor. These findings offer insights into the variation in functional traits of P. yunnanensis and enhance our understanding of its adaptation to diverse environments.
1 Introduction
Plant functional traits are any measurable attributes that directly or indirectly affect plant adaptability (Violle et al., 2007; Albert et al., 2010, 2012). Intraspecific variation in traits reflects the outcomes of evolutionary processes that individuals have responded to abiotic and biotic environmental constraints (Valladares et al., 2007). The polymorphism of traits within a species can enable individuals to adapt to diverse environmental conditions (Matías and Jump, 2014; Seidel and Menzel, 2016). For instance, Pittosporopsis kerrii (Icacinaceae), one of the most abundant tree species in the Xishuangbanna tropical seasonal rainforest in southwestern China, exhibits increased leaf thickness and decreased specific leaf area with increased elevation (Xu et al., 2020). Nothofagus pumilio (Nothofagaceae), a tree species widely distributed in southern Chile, shows a decreasing trend in leaf mass per area with increasing temperature, and a decreasing trend in wood density with increasing precipitation (Fajardo and Piper, 2011). Investigating the intraspecific variation in functional traits can provide insights into the evolutionary mechanisms of these traits and facilitate understanding of how a given plant species adapt to different environments (Turcotte and Levine, 2016; Silva et al., 2019).
Pinus yunnanensis, an endemic species of southwestern China, grows in diverse geological settings and exhibits considerable intraspecific phenotypic variation (Sun et al., 2020a). Pinus yunnanensis is typically propagated by seeds and has winged seeds, belonging to the category of cross-pollination, with wind as the pollination medium. Due to its wide distribution and complex, diverse survival environments, it has led to the gradual independent evolution of different ecotypes in adaptation to various environments (Su et al., 2015; Xu et al., 2015, 2016a; Sun et al., 2020b). The significant differences in traits among these varieties are believed to be caused by anthropogenic factors and geographic conditions. Within the species, it exhibits significant morphological and genetic variation. Due to its wide distribution and a wide range of habitats, P. yunnanensis exhibits considerable intraspecific variation in many traits, resulting in three varieties: var. yunnanensis (the original variety), var. pygmaea, and var. tenuifolia (Pausas et al., 2021). Var. yunnanensis and var. tenuifolia are trees that generally grow above 15 m, while var. pygmaea is a multi-stemmed shrub that only grows around 1-3 m. In the wildfire-prone Yunnan-Guizhou Plateau region, the three variants gradually also developed different adaptation strategies, such as the arboreal variants of var. tenuifolia and var. yunnanensis which are better adapted to surface fires, and the shrubby variant of var. pygmaea, which is adapted to crown fires (Su et al., 2019) (Figure 1). The leaves of var. yunnanensis are typically between 10 and 30 centimeters in length, whereas var. pygmaea has shorter leaves, ranging from 7 to 13 centimeters; var. tenuifolia possesses the longest leaves, reaching up to 36 centimeters (Wang et al., 2023).
Intraspecific variation is a result of the combined effects of environmental factors, such as temperature, precipitation and soil characteristics, rather than being driven by a single factor (Piao et al., 2014; Seddon et al., 2016; Jung et al., 2017). Although the intraspecific variation in functional traits of Pinus yunnanensis has been widely investigated, most previous studies have been limited to specific environmental factors, which could not accurately assess the mechanism of intraspecific variations in Pinus yunnanensis (Han et al., 2015; Liu et al., 2023). Consequently, it is imperative to explore the impacts of various factors on the intraspecific variation observed in Pinus yunnanensis.
Environmental factors play an essential role in plant life and adaption (Anderson et al., 2020; Xiang et al., 2022; Aqeel et al., 2023; Chen et al., 2024). In this study, In this study, we investigated how habitat conditions(precipitation and temperature data, influence environmental variables such as nitrogen content, organic carbon content, sandy soil content, soil clay content, and coarse debris content (0.5-15.3 cm in size) in the soil) affect intraspecific variation in five functional traits (leaf length, specific leaf area, bark thickness, height, and leaf dry matter content) of Pinus yunnanensis. Since Pinus yunnanensis has evolved different variants in response to different fire behaviors in fire-prone habitats, the relevant plant height, leaf traits, and bark thickness are the traits that can better demonstrate the traits related to fire adaptation, and better help us to understand the fire adaptive responses of plants under the disturbance of fire factors. Aiming to understand how these habitat conditions collectively have shaped these traits.
2 Methods
2.1 Study sites and sample collection
Pinus yunnanensis is one of the dominant species in the Central Yunnan Plateau as well as in the Western Yun-Gui Plateau. In this study, we selected 20 P. yunnanensis populations (7 populations for var. yunnanensis, 7 populations for var. pygmaea, and 6 populations for var. tenuifolia) ranging from 377 to 2555 m (Table 1; Figure 2) Mature natural forests were selected for field survey and recording during sampling, and when selecting samples, we chose mature individuals with good growth within the sample plots to ensure the accuracy and scientific validity of the study. The sampling and trait measurement methods were derived from previously published studies of congeneric species (Urbaniak et al., 2011; Xu et al., 2016a). Six healthy, mature individuals were selected from each population for sampling. Sampled trees were located at least 10 meters apart within each population. Two-year-old needles were randomly sampled from three to five vigorous branches on each sampled individual and immediately placed in labeled bags.
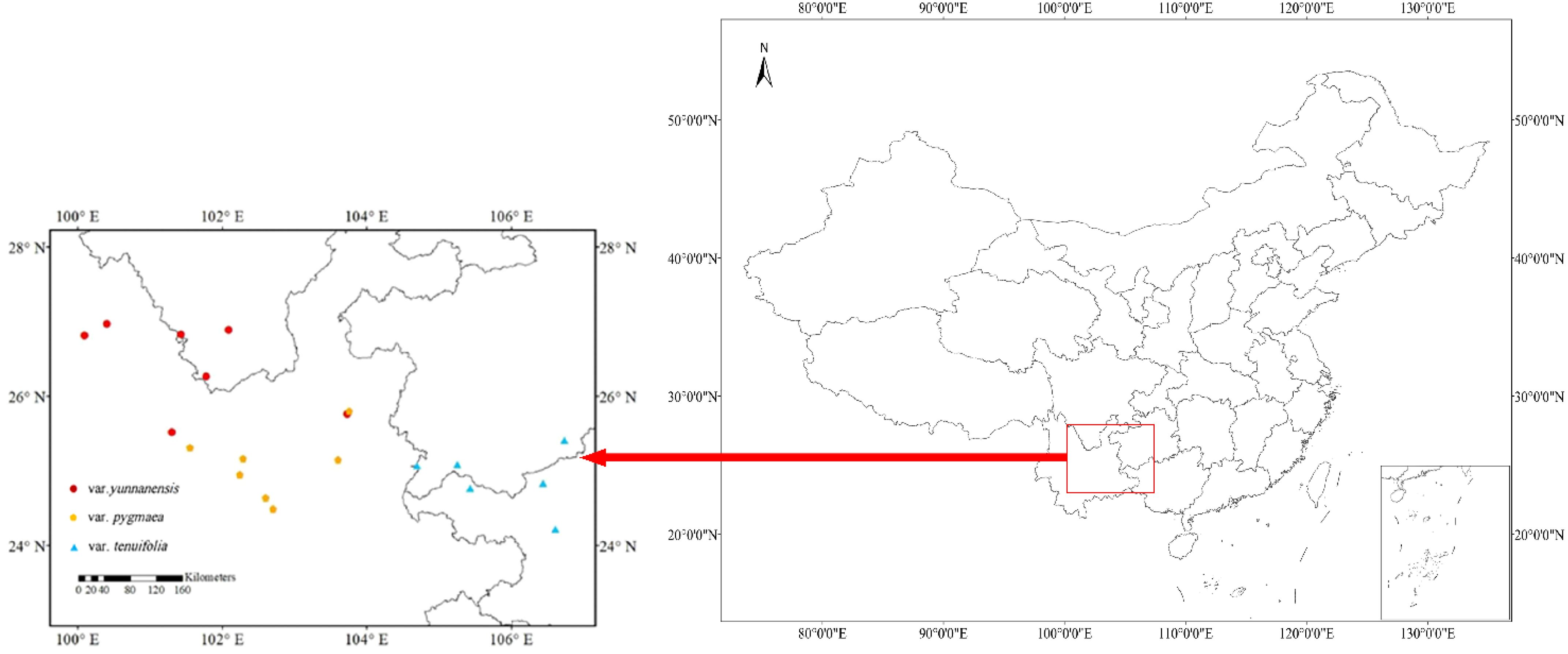
Figure 2. Distribution of P. yunnanensis populations sampled around Yunnan-Guizhou Plateau in this study shown on a map of China.
2.2 Functional traits measurement
Tree height: Six mature individuals were selected to measure tree height at each sampling site. The measurements were carried out by using a combination of a laser rangefinder, a leveling instrument, and a leveling rod. Place the laser rangefinder at a certain distance from the tree. First, use a level and a leveling rod to determine a horizontal line and ensure that the laser rangefinder is positioned on the horizontal line; and don’t easily move the rangefinder. Aim the laser beam emitted by the rangefinder at the tree’s root position to obtain a distance parameter L1 and record the angle between the beam and the horizontal line, denoted as ; the distance parameter from the tree tip position to the laser rangefinder is L2, with the beam and the horizontal line making an angle ; and record the vertical distance from the rangefinder to the tree trunk, which is L0. By inputting these parameters into the following mathematical function calculation formula, Tree height (m) can be calculated as (Cornelissen et al., 2003):
Bark thickness: The bark thickness was obtained by blades and saws to cut and remove, followed by using a vernier caliper for bark thickness measurement. The bark thickness of var. yunnanensis and var. tenuifolia were selected at a height of around 1.3 m above the ground. As for var. pygmea, due to its smaller stature, measurements were taken near ground level.
Leaf dry matter content: Fresh leaf and stem samples are washed and immersed in distilled water to ensure full saturation for 24 hours. After saturation, excess surface water was gently removed using absorbent paper, and the samples were weighed to determine their saturated mass (m1). The samples were then placed in an electronic drying oven at 65°C until a constant mass was achieved and reweighed (m2). Subsequently, leaf dry matter content (g/g) was calculated as (Polley et al., 2022):
Leaf length and specific leaf area: Number the leaves in groups of six leaves each. Flatten them and place them on the scanner bed (HP Laser MFP 136w), scanning each leaf at a resolution of 300 DPI. Save the scanned images in JPEG format. Use ImageJ 1.53a (NIH, Bethesda, MD, USA) software to measure the length, width, and leaf area of each leaf in the scanned images (Katabuchi, 2015), with units in centimeters (cm) and square centimeters (cm²) respectively. The specific leaf area is the ratio of leaf area to leaf dry matter content, expressed in units of cm²/g.
2.3 Environmental data collection
Nineteen bioclimatic variables and latitude data were obtained from the World Climate Database (WorldClim: https://www.worldclim.org/data/index.html). We used the high spatial resolution (30 arc-s, ~1 km at the Equator) from it (Hijmans et al., 2005) The 30 arc-second resolution means that the data cover the globe in a grid of about 1 kilometer, with each grid point providing an average value of a climate variable. Soil data, including nitrogen content, organic carbon content, sandy soil content, soil clay content, and soil coarse debris content of 0.5-15.0 cm of soil at 1 km spatial resolution (Hengl et al., 2017), were obtained from SoilGrids (https://soilgrids.org/) (Chen et al., 2022). Finally, we gathered data for 24 environmental variables.
2.4 Data analysis
The variation of functional traits across P. yunnanensis intraspecific differences was analyzed by the One-way ANOVA method, and we set the significance level equal to 0.05. We used the Pearson correlation coefficient to analyze correlations between functional traits and environmental factors. The relative importance of different environmental factors for functional traits was evaluated by a random forest model in randomForest package (4.7-1.1). Random forest model has the highest prediction accuracy, and the random forest model of environmental factor impact traits developed accordingly has better accuracy and prediction ability than the stepwise regression model. The relative importance of each factor to functional traits is further analyzed by using the random forest regression model (GFBI consortium et al., 2019; Mantas et al., 2019; Wang et al., 2022) (Figure 5). All statistical analysis and plotting were performed using R 4.1.1 (R Foundation for Statistical Computing, Vienna, Austria) and Origin 2021 (Origin Lab Corporation, Northampton, MA, USA). Creating distribution maps was done using ArcGIS 10.2 (Esri, Redlands, CA, USA).
3 Results
3.1 Intraspecific variation in five functional traits within P. yunnanensis
Bark thickness of var. yunnanensis (1.83 ± 0.54 cm) and var. tenuifolia (1.69 ± 0.38 cm) is significantly higher than that of var. pygmea (0.55 ± 0.13 cm) (p ≤ 0.05). Tree height of var. tenuifolia is 19.61 ± 3.11 m, followed by var. yunnanensis (1.83 ± 0.54 cm), and tree height of var. pygmea is only 2.28 ± 0.52 m. Leaf length of var. pygmea (18.06 ± 1.13 cm) is significantly shorter than that of var. yunnanensis (23.35 ± 2.57 cm) and var. tenuifolia (23.48 ± 0.30 cm) (p ≤ 0.001), while no significant differences are observed between var. yunnanensis (23.35 ± 2.57 cm) and var. tenuifolia (23.48 ± 0.30 cm) (p ≤ 0.05). Both specific leaf area and leaf dry matter content show no significant differences among the three varieties (Figure 3).
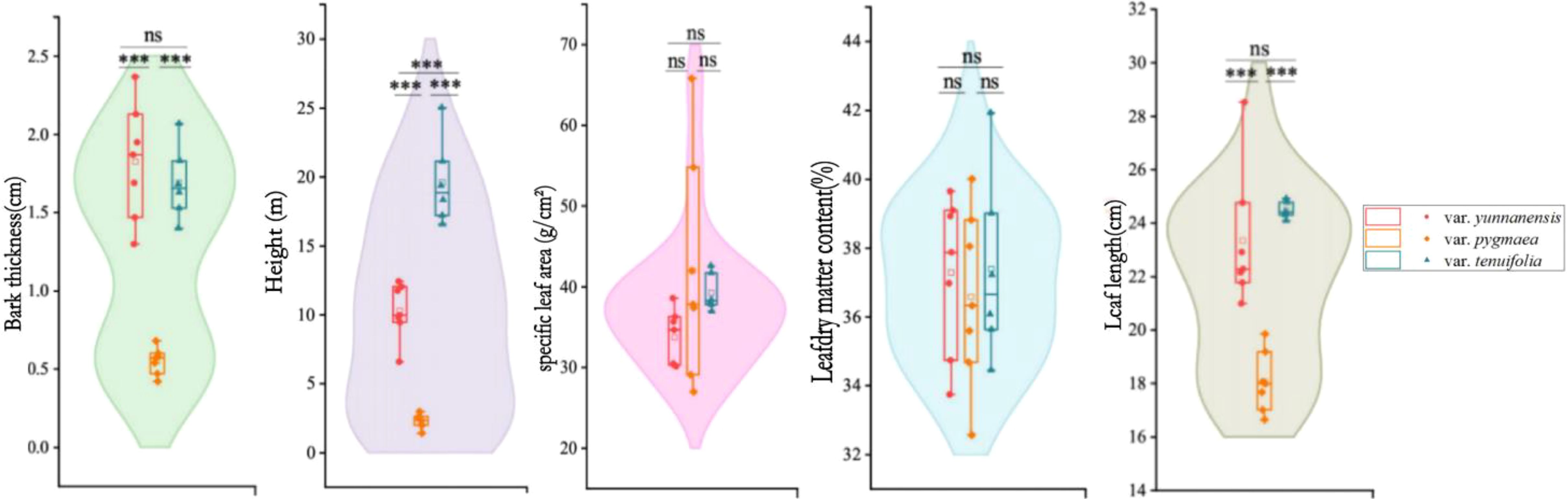
Figure 3. Variations in functional traits of P. yunnanensis. The value in this figure is expressed as mean ± SD (the same below), and the asterisk and letter indicate the significance of the difference between the traits of the three variations (∗∗∗, p ≤ 0.001; ns, p > 0.05.).
3.2 Relationships among these five studied functional traits
There was a significant positive correlation among bark thickness, tree height and leaf length (p ≤ 0.01). Specific leaf area and leaf dry matter content showed no significant relationships with other functional traits (p > 0.05, Figure 4).
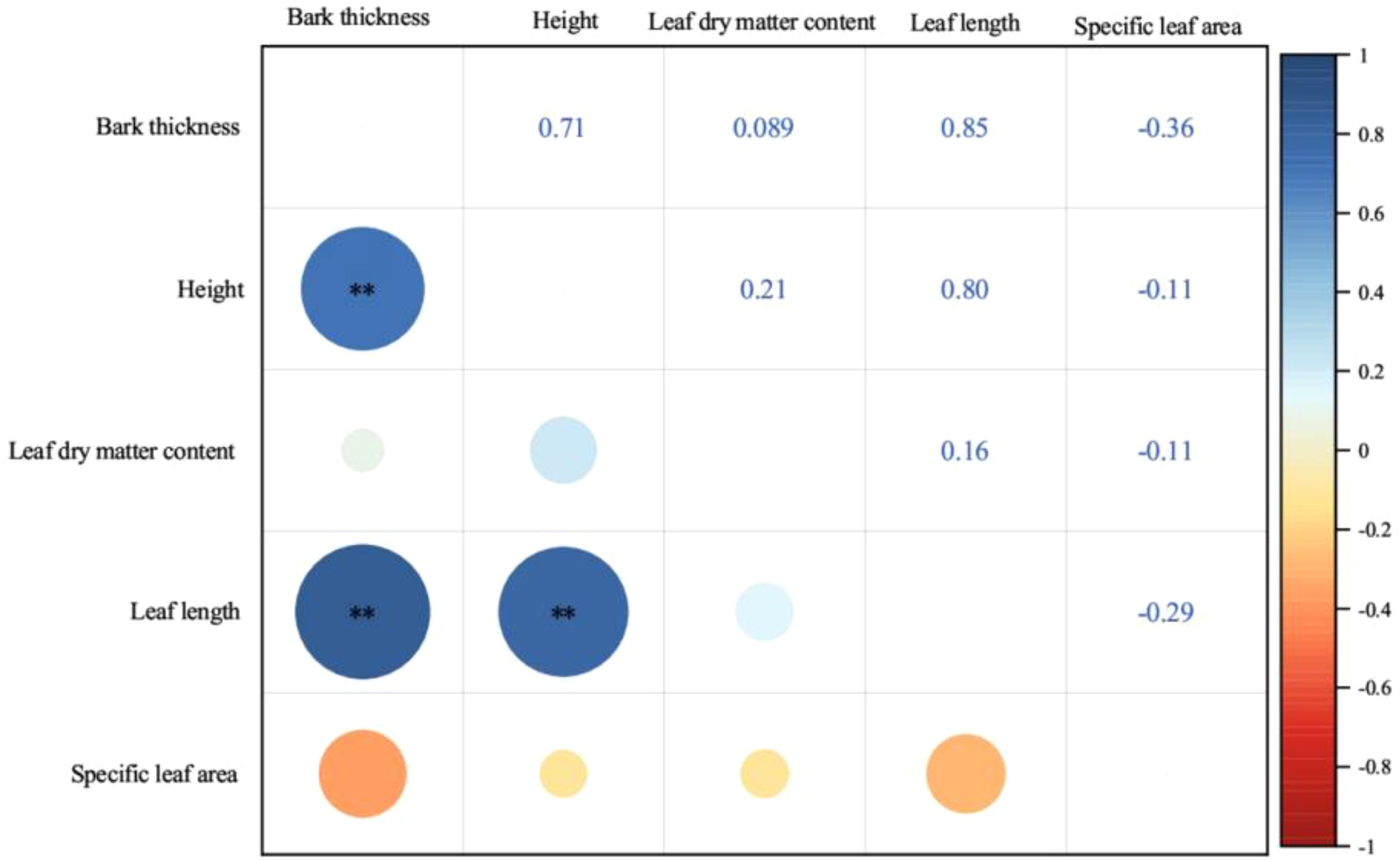
Figure 4. Pearson’s correlation between functional traits of P. yunnanensis. Blue color refers to positive correlation and red color refers negative correlation. The size of the circle marks the size of the correlation coefficient. (∗∗, p ≤ 0.01; unmarked, p > 0.05.).
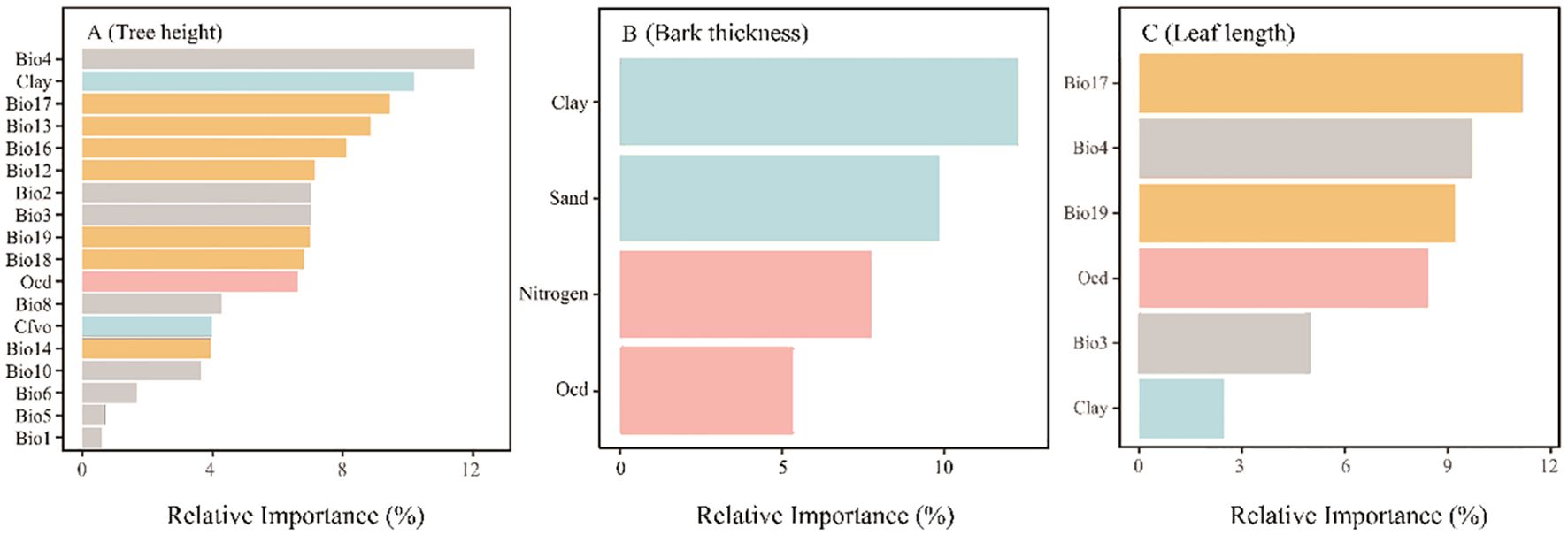
Figure 5. Relative Importance of different environmental factors to the Tree height (A), Bark thickness (B) and Leaf length (C) in P. yunnanensis. Every concrete meaning of environmental variables refer to Table 2.
3.3 Environmental determinants of intraspecific variation in functional traits
Specific leaf area and leaf dry matter content were not related to any environmental factor.
Tree height had a significant relationship with most environmental factors, including significant positive correlations with annual mean temperature, temperature seasonality, max temperature of warmest month, min temperature of coldest month, mean temperature of wettest quarter, mean temperature of warmest quarter, annual precipitation, precipitation of wettest month, precipitation of driest month, precipitation of wettest quarter, precipitation of driest quarter, precipitation of warmest quarter, precipitation of coldest quarter, and organic carbon density, while significant negative correlations with mean diurnal range, isothermality, Vol. fraction of coarse fragments and proportion of clay particles.
Bark thickness had a significant positive relationship (p ≤ 0.05) with total nitrogen, organic carbon density, and proportion of sand particles, while a negative relationship with proportion of clay particles. We also found that bark thickness had no significant relationship with all climatic factors.
Leaf length had a significant positive correlation with temperature seasonality, precipitation of driest quarter, precipitation of coldest quarter and organic carbon density (p ≤ 0.05). However, it displayed a significant negative correlation with isothermality and proportion of clay particles (p ≤ 0.05) (Table 3).
3.4 Relative importance of different environmental factors to tree height, bark thickness and leaf length
Temperature seasonality had the most relative importance to tree height, explaining 12.06%, while the relative importance of annual mean temperature was the smallest, accounting for only 0.58%.
The relative importance of soil particle size (clay and sand) was higher in bark thickness, contributed 12.28% and 9.83%, respectively. The relative importance of total nitrogen to bark thickness is second (7.75%), and the relative importance of organic carbon density is the smallest (5.32%).
In terms of needle leaf length, precipitation of driest quarter had the highest relative importance in leaf length (11.16%), followed by temperature seasonality (9.71%), precipitation of coldest quarter (9.21%), organic carbon density (8.42%), and isothermality (4.98%). The relative importance of clay was the least (2.48%).
4 Discussion
We found that some functional traits of P. yunnanensis exhibited high variability among different populations and varieties. For instance, our research showed substantial differences in the thickness and width of P. yunnanensis var. tenuifolia needles among seven populations with distinct geographical origins, noting that the needles of this variety were smaller compared to those of P. yunnanensis. Similarly, Huang et al. (Huang et al., 2016) observed considerable variation in the leaf length of P. yunnanensis within seven different populations, with a coefficient of variation (CV) of 11.1%, which is comparable to our result of 15.1%. In addition to five functional traits, some other traits of P. yunnanensis also showed significant intraspecific differences, for example, the length, width, and weight of the cones (Xu et al., 2016b); Seed length and width (Cai et al., 2016); and the needle bundle also changed (Wang et al., 2004). The multiple functional traits of P. yunnanensis exhibit significant intraspecific variation, making it become an ideal material for studying the species’ evolution. Intraspecific variation represents the foundation of biological evolution and constitutes a crucial adaptive strategy for species to cope with environmental changes (Shen et al., 2020; Huang et al., 2022). By investigating the phenomenon of intraspecific variation in functional traits, we can gain insight into the adaptive strategies that operate within species and the diversity of genetic traits.
The study demonstrated that there were significant correlations and close coordination among different traits within the species of P. yunnanensis. This correlation between traits has been previously identified in other studies (Messier et al., 2010; Anderegg et al., 2018). Our findings indicate that bark thickness tends to increase in taller trees, this trait imparts mechanical strength and drought protection to the trunk (Paine et al., 2010; Brando et al., 2012). Furthermore, Leaf length is positively correlated with both tree height and bark thickness, reflecting the greater light availability in taller trees, which in turn affects leaf characteristics (Niinemets and Kull, 1995). These findings reflect the common changes in tree height, bark thickness, and needle length. Our results strongly support the existence and rationality of a system of associations between plant resource allocation and growth strategies (Reich, 2014; Zhao et al., 2016).
The results of our study indicate a significant correlation between functional traits and environmental factors. This correlation encompasses both long-term selection of genotypes by environmental factors (genetic differentiation) and individual plasticity responses to environmental factors (Lebedeva and Gerasimova, 2009; Stamp and Hadfield, 2020). Previous studies have also got similar conclusions (Wright et al., 2005; Markesteijn et al., 2010; Vasey et al., 2022). The functional traits of P. yunnanensis were found to be positively correlated with precipitation, temperature, temperature seasonality, organic carbon content, and organic carbon density in soil, but negatively correlated with proportion of clay particles and Vol. fraction of coarse fragments. These findings are consistent with those of other ecological inquiries. For example, the wood density of Pinus sylvestris was found to be positively correlated with precipitation (Laforest-Lapointe et al., 2014), while the leaf porosity of the holly was proportional to precipitation (Niinemets, 2015). The environmental factors that had the greatest influence on the intraspecific variation of functional traits of P. yunnanensis were elucidated in the random forest importance ranking. Our study confirm that temperature and moisture levels in the environment are the most important factors affecting its variability. Other studies have had similar results (Zhao et al., 2008; Jankowski et al., 2019; Popović et al., 2022). Specific leaf area and leaf dry matter content are weakly correlated with environmental factors. We know that specific leaf area can be calculated as leaf area/leaf dry weight, so one possible reason for the weak correlation of specific leaf area with environmental factors is that leaf area and leaf dry weight are almost directly covariant, a possibility that also applies to leaf dry matter content. In addition, the interaction of climatic and soil factors on intraspecific variation in plant traits (Ordoñez et al., 2009) may counteract each other’s effect on specific leaf area and leaf dry matter content. The convergence of these findings emphasizes the complex interactions between functional traits and environmental conditions, highlighting the critical role of phenotypic plasticity in the adaptive strategies and ecological distribution of species (Nicotra et al., 2010; Valladares et al., 2014; Henn et al., 2018). In the context of global climate change, phenotypic plasticity may be an important mechanism for organisms to adapt to new environmental conditions (Alberto et al., 2013; Donelson et al., 2018) and thus merits further investigation. Although this study focused on the effects of climatic and soil factors on differences in plant traits, the potential effects of environmental factors such as light and altitude on plant growth and acclimatization have not been considered. Light directly affects the rate of photosynthesis in plants, which in turn acts on leaf area and morphology (Tan et al., 2022), while changes in altitude may indirectly affect plant physiological traits through changes in temperature, barometric pressure, and radiation (Daunicht and Brinkjans, 1996; Rahman et al., 2020). Future studies could incorporate these factors into the analysis to gain a more comprehensive understanding of the mechanisms of plant trait adaptation.
This reveals the existence of more complex and coordinated phenotypes than those found in single-spectrum or one-trait cluster approaches. This coordinated covariation is the result of natural selection, and other combinations of traits may occur under different environmental conditions (Negret et al., 2015). Only 5 traits were selected for comparative study in this research, and other traits may also be correlated (Cai et al., 2016), so this study has certain limitations. In light of the potential for sustained alterations in environmental conditions within the distribution range of the Pinus yunnanensis, the coordinated covariation of functional traits may serve to enhance the species’ capacity for adaptation to environmental change (Laforest-Lapointe et al., 2014; Carvalho et al., 2020; Jiang et al., 2021). Consequently, an examination of the intraspecific variations in traits and an investigation of the relationship between trait variation and environmental factors will provide insights into the evolutionary process of the species, thereby facilitating an understanding of how the species evolved to adapt to diverse environments (Jung et al., 2010; Zuleta et al., 2022).
5 Conclusion
Plant functional traits are key attributes indicative of plant growth conditions, and these traits are individually or jointly adapted to the external environment and change in response to environmental changes. Incorporating intraspecific variation in plant functional traits into ecological studies can better reveal species interactions, population responses to spatial and temporal environmental gradients. In this study, our results suggest that different environmental conditions induce intraspecific variation in P. yunnanensis, five functional traits differed significantly among the three varieties, providing evidence for coordinated differences in traits and their covariates under external conditions. The correlations among the tree functional traits reveal that intraspecific variation in plant functional traits is also not the result of a single environmental factor, but is subject to a combination of several different environmental factors. Variation in plant functional traits across environmental gradients is driven by biotic and abiotic factors. The molecular mechanisms underlying intraspecific variation and other trait variations in P. yunnanensis are still unclear. To better understand the intraspecific variatis of P. yunnanensis, these aspects need further research.
Data availability statement
The original contributions presented in the study are included in the article/supplementary material. Further inquiries can be directed to the corresponding author.
Author contributions
XS: Formal analysis, Writing – original draft. YL: Software, Supervision, Writing – original draft, Writing – review & editing. DY: Methodology, Software, Supervision, Writing – review & editing. SL: Investigation, Resources, Writing – review & editing. WG: Methodology, Software, Writing – review & editing. HZ: Resources, Writing – review & editing. DC: Resources, Writing – review & editing. SZ: Resources, Writing – review & editing. XC: Funding acquisition, Methodology, Resources, Supervision, Writing – review & editing.
Funding
The author(s) declare financial support was received for the research, authorship, and/or publication of this article. This work was supported by National Natural Science Foundation of China (grant number 32101532, 32422060) and the Natural Science Foundation of Sichuan Province (grant number 2023NSFSC1278).
Acknowledgments
We would like to thank Mingrui Xian from Southwest Survey and Planning Institute of National Forestry and Grassland Administration, Kunming, China providing us with sample polts location of Pinus yunnanensis during the fieldwork.
Conflict of interest
The authors declare that the research was conducted in the absence of any commercial or financial relationships that could be construed as a potential conflict of interest.
Generative AI statement
The author(s) declare that no Generative AI was used in the creation of this manuscript.
Publisher’s note
All claims expressed in this article are solely those of the authors and do not necessarily represent those of their affiliated organizations, or those of the publisher, the editors and the reviewers. Any product that may be evaluated in this article, or claim that may be made by its manufacturer, is not guaranteed or endorsed by the publisher.
References
Albert, C. H., De Bello, F., Boulangeat, I., Pellet, G., Lavorel, S., Thuiller, W. (2012). On the importance of intraspecific variability for the quantification of functional diversity. Oikos 121, 116–126. doi: 10.1111/j.1600-0706.2011.19672.x
Albert, C. H., Thuiller, W., Yoccoz, N. G., Soudant, A., Boucher, F., Saccone, P., et al. (2010). Intraspecific functional variability: extent, structure and sources of variation. J. Ecol. 98, 604–613. doi: 10.1111/j.1365-2745.2010.01651.x
Alberto, F. J., Aitken, S. N., Alía, R., González-Martínez, S. C., Hänninen, H., Kremer, A., et al. (2013). Potential for evolutionary responses to climate change - evidence from tree populations. Glob. Change Biol. 19, 1645–1661. doi: 10.1111/gcb.12181
Anderegg, L. D. L., Berner, L. T., Badgley, G., Sethi, M. L., Law, B. E., HilleRisLambers, J. (2018). Within-species patterns challenge our understanding of the leaf economics spectrum. Ecol. Lett. 21, 734–744. doi: 10.1111/ele.12945
Anderson, R., Bayer, P. E., Edwards, D. (2020). Climate change and the need for agricultural adaptation. Curr. Opin. Plant Biol. 56, 197–202. doi: 10.1016/j.pbi.2019.12.006
Aqeel, M., Ran, J., Hu, W., Irshad, M. K., Dong, L., Akram, M. A., et al. (2023). Plant-soil-microbe interactions in maintaining ecosystem stability and coordinated turnover under changing environmental conditions. Chemosphere 318, 137924. doi: 10.1016/j.chemosphere.2023.137924
Brando, P. M., Nepstad, D. C., Balch, J. K., Bolker, B., Christman, M. C., Coe, M., et al. (2012). Fire-induced tree mortality in a neotropical forest: the roles of bark traits, tree size, wood density and fire behavior. Glob. Change Biol. 18, 630–641. doi: 10.1111/j.1365-2486.2011.02533.x
Cai, N., Xu, Y., Chen, S., He, B., Li, G., Li, Y., et al. (2016). Variation in seed and seedling traits and their relations to geo-climatic factors among populations in Yunnan Pine (Pinus yunnanensis). J. For. Res. 27, 1009–1017. doi: 10.1007/s11676-016-0228-z
Carvalho, B., Bastias, C. C., Escudero, A., Valladares, F., Benavides, R. (2020). Intraspecific perspective of phenotypic coordination of functional traits in Scots pine. PloS One 15, e0228539. doi: 10.1371/journal.pone.0228539
Chen, G., Qin, Y., Wang, J., Li, S., Zeng, F., Deng, F., et al. (2024). Stomatal evolution and plant adaptation to future climate. Plant Cell Environ. 47, 3299–3315. doi: 10.1111/pce.14953
Chen, S., Arrouays, D., Leatitia Mulder, V., Poggio, L., Minasny, B., Roudier, P., et al. (2022). Digital mapping of GlobalSoilMap soil properties at a broad scale: A review. Geoderma 409, 115567. doi: 10.1016/j.geoderma.2021.115567
Cornelissen, J. H. C., Lavorel, S., Garnier, E., Díaz, S., Buchmann, N., Gurvich, D. E., et al. (2003). A handbook of protocols for standardised and easy measurement of plant functional traits worldwide. Aust. J. Bot. 51, 335. doi: 10.1071/BT02124
Daunicht, H. J., Brinkjans, H. J. (1996). Plant responses to reduced air pressure: advanced techniques and results. Adv. space research: Off. J. Committee Space Res. (COSPAR) 18, 273–281. doi: 10.1016/0273-1177(95)00889-m
Donelson, J. M., Salinas, S., Munday, P. L., Shama, L. N. S. (2018). Transgenerational plasticity and climate change experiments: Where do we go from here. Glob. Change Biol. 24, 13–34. doi: 10.1111/gcb.13903
Fajardo, A., Piper, F. I. (2011). Intraspecific trait variation and covariation in a widespread tree species (Nothofagus pumilio) in southern Chile. New Phytol. 189, 259–271. doi: 10.1111/j.1469-8137.2010.03468.x
GFBI consortium, Steidinger, B. S., Crowther, T. W., Liang, J., Van Nuland, M. E., Werner, G. D. A., et al. (2019). Climatic controls of decomposition drive the global biogeography of forest-tree symbioses. Nature 569, 404–408. doi: 10.1038/s41586-019-1128-0
Han, J., Shen, Z., Ying, L., Li, G., Chen, A. (2015). Early post-fire regeneration of a fire-prone subtropical mixed Yunnan pine forest in Southwest China: Effects of pre-fire vegetation, fire severity and topographic factors. For. Ecol. Manage. 356, 31–40. doi: 10.1016/j.foreco.2015.06.016
Hengl, T., de Jesus, J. M., Heuvelink, G. B. M., Gonzalez, M. R., Kilibarda, M., Blagotić, A., et al. (2017). SoilGrids250m: Global gridded soil information based on machine learning. PloS One 12, e0169748. doi: 10.1371/journal.pone.0169748
Henn, J. J., Buzzard, V., Enquist, B. J., Halbritter, A. H., Klanderud, K., Maitner, B. S., et al. (2018). Intraspecific trait variation and phenotypic plasticity mediate alpine plant species response to climate change. Front. Plant Sci. 9. doi: 10.3389/fpls.2018.01548
Hijmans, R. J., Cameron, S. E., Parra, J. L., Jones, P. G., Jarvis, A. (2005). Very high resolution interpolated climate surfaces for global land areas. Int. J. Climatology 25, 1965–1978. doi: 10.1002/joc.1276
Huang, Y., Mao, J., Chen, Z., Meng, J., Xu, Y., Duan, A., et al. (2016). Genetic structure of needle morphological and anatomical traits of Pinus yunnanensis. J. For. Res. 27, 13–25. doi: 10.1007/s11676-015-0133-x
Huang, B., Mao, J., Zhao, Y., Sun, Y., Cao, Y., Xiong, Z. (2022). Similar Pattern of Potential Distribution of Pinus yunnanensis Franch and Tomicusyunnanensis Kirkendall under Climate Change in China. Forests 13, 1379. doi: 10.3390/f13091379
Jankowski, A., Wyka, T. P., Żytkowiak, R., Danusevičius, D., Oleksyn, J. (2019). Does climate-related in situ variability of Scots pine (Pinus sylvestris L.) needles have a genetic basis? Evidence from common garden experiments. Tree Physiol. 39, 573–589. doi: 10.1093/treephys/tpy145
Jiang, F., Cadotte, M. W., Jin, G. (2021). Individual-level leaf trait variation and correlation across biological and spatial scales. Ecol. Evol. 11, 5344–5354. doi: 10.1002/ece3.7425
Jung, M., Reichstein, M., Schwalm, C. R., Huntingford, C., Sitch, S., Ahlström, A., et al. (2017). Compensatory water effects link yearly global land CO2 sink changes to temperature. Nature 541, 516–520. doi: 10.1038/nature20780
Jung, V., Violle, C., Mondy, C., Hoffmann, L., Muller, S. (2010). Intraspecific variability and trait-based community assembly. J. Ecol. 98, 1134–1140. doi: 10.1111/j.1365-2745.2010.01687.x
Katabuchi, M. (2015). LeafArea: an R package for rapid digital image analysis of leaf area. Ecol. Res. 30, 1073–1077. doi: 10.1007/s11284-015-1307-x
Laforest-Lapointe, I., Martínez-Vilalta, J., Retana, J. (2014). Intraspecific variability in functional traits matters: Case study of Scots pine. Oecologia 175, 1337–1348. doi: 10.1007/s00442-014-2967-x
Lebedeva, I. I., Gerasimova, M. I. (2009). Factors of soil formation in soil classification systems. Eurasian Soil Sci. 42, 1412–1418. doi: 10.1134/S1064229309120138
Liu, Y., Wu, J., Wu, D., Li, S., Wang, L. (2023). Seasonal variation in δ13C of Pinus. yunnanensis and Pinus. armandii at different stand ages. Sci. Rep. 13, 7938. doi: 10.1038/s41598-023-34920-3
Mantas, C. J., Castellano, J. G., Moral-García, S., Abellán, J. (2019). A comparison of random forest based algorithms: random credal random forest versus oblique random forest. Soft Comput. 23, 10739–10754. doi: 10.1007/s00500-018-3628-5
Markesteijn, L., Iraipi, J., Bongers, F., Poorter, L. (2010). Seasonal variation in soil and plant water potentials in a Bolivian tropical moist and dry forest. J. Trop. Ecol. 26, 497–508. doi: 10.1017/S0266467410000271
Matías, L., Jump, A. S. (2014). Impacts of predicted climate change on recruitment at the geographical limits of Scots pine. J. Exp. Bot. 65, 299–310. doi: 10.1093/jxb/ert376
Messier, J., McGill, B. J., Lechowicz, M. J. (2010). How do traits vary across ecological scales? A case for trait-based ecology. Ecol. Lett. 13, 838–848. doi: 10.1111/j.1461-0248.2010.01476.x
Negret, B., Canessa, R., Valladares, F., Armesto, J. J., Pérez, F. (2015). Functional traits variation explains the distribution of Aextoxicon punctatum (Aextoxicaceae) in pronounced moisture gradients within fog-dependent forest fragments. Front. Plant Sci. 6. doi: 10.3389/fpls.2015.00511
Nicotra, A. B., Atkin, O. K., Bonser, S. P., Davidson, A. M., Finnegan, E. J., Mathesius, U., et al. (2010). Plant phenotypic plasticity in a changing climate. Trends Plant Sci. 15, 684–692. doi: 10.1016/j.tplants.2010.09.008
Niinemets, Ü. (2015). Is there a species spectrum within the world-wide leaf economics spectrum? Major variations in leaf functional traits in the Mediterranean sclerophyll Quercus ilex. New Phytol. 205, 79–96. doi: 10.1111/nph.13001
Niinemets, U., Kull, O. (1995). Effects of light availability and tree size on the architecture of assimilative surface in the canopy of Picea abies: variation in needle morphology. Tree Physiol. 15, 307–315. doi: 10.1093/treephys/15.5.307
Ordoñez, J. C., Van Bodegom, P. M., Witte, J. M., Wright, I. J., Reich, P. B., Aerts, R. (2009). A global study of relationships between leaf traits, climate and soil measures of nutrient fertility. Glob. Ecol. Biogeogr. 18, 137–149. doi: 10.1111/j.1466-8238.2008.00441.x
Paine, C. E. T., Stahl, C., Courtois, E. A., Patiño, S., Sarmiento, C., Baraloto, C. (2010). Functional explanations for variation in bark thickness in tropical rain forest trees. Funct. Ecol. 24, 1202–1210. doi: 10.1111/j.1365-2435.2010.01736.x
Pausas, J. G., Su, W., Luo, C., Shen, Z. (2021). A shrubby resprouting pine with serotinous cones endemic to southwest China. Ecology 102, e03282. doi: 10.1002/ecy.3282
Piao, S., Nan, H., Huntingford, C., Ciais, P., Friedlingstein, P., Sitch, S., et al. (2014). Evidence for a weakening relationship between interannual temperature variability and northern vegetation activity. Nat. Commun. 5, 5018. doi: 10.1038/ncomms6018
Polley, H. W., Collins, H. P., Fay, P. A. (2022). Community leaf dry matter content predicts plant production in simple and diverse grassland. Ecosphere 13, e4076. doi: 10.1002/ecs2.4076
Popović, V., Nikolić, B., Lučić, A., Rakonjac, L., Šešlija Jovanović, D., Miljković, D. (2022). Morpho-anatomical trait variability of the Norway spruce (Picea abies (L.) Karst.) needles in natural populations along elevational diversity gradient. Trees 36, 1131–1147. doi: 10.1007/s00468-022-02277-1
Rahman, I. U., Afzal, A., Iqbal, Z., Hart, R., Abd_Allah, E. F., Alqarawi, A. A., et al. (2020). Response of plant physiological attributes to altitudinal gradient: Plant adaptation to temperature variation in the Himalayan region. Sci. Total Environ. 706, 135714. doi: 10.1016/j.scitotenv.2019.135714
Reich, P. B. (2014). The world-wide ‘fast–slow’ plant economics spectrum: a traits manifesto. J. Ecol. 102, 275. doi: 10.1111/jec.2014.102.issue-2
Seddon, A. W. R., Macias-Fauria, M., Long, P. R., Benz, D., Willis, K. J. (2016). Sensitivity of global terrestrial ecosystems to climate variability. Nature 531, 229–232. doi: 10.1038/nature16986
Seidel, H., Menzel, A. (2016). Above-ground dimensions and acclimation explain variation in drought mortality of scots pine seedlings from various provenances. Front. Plant Sci. 7. doi: 10.3389/fpls.2016.01014
Shen, J., Li, Z., Gao, C., Li, S., Huang, X., Lang, X., et al. (2020). Radial growth response of Pinus yunnanensis to rising temperature and drought stress on the Yunnan Plateau, southwestern China. For. Ecol. Manage. 474, 118357. doi: 10.1016/j.foreco.2020.118357
Silva, M. C., Teodoro, G. S., Bragion, E. F. A., Van Den Berg, E. (2019). The role of intraspecific trait variation in the occupation of sharp forest-savanna ecotones. Flora 253, 35–42. doi: 10.1016/j.flora.2019.03.003
Stamp, M. A., Hadfield, J. D. (2020). The relative importance of plasticity versus genetic differentiation in explaining between population differences; a meta-analysis. Ecol. Lett. 23, 1432–1441. doi: 10.1111/ele.13565
Su, W.-H., Shi, Z., Zhou, R., Zhao, Y.-J., Zhang, G.-F. (2015). The role of fire in the Central Yunnan Plateau ecosystem, southwestern China. For. Ecol. Manage. 356, 22–30. doi: 10.1016/j.foreco.2015.05.015
Su, W., Yu, J., Zhang, G., Shi, Z., Wang, L., Zhao, G., et al. (2019). Comparison of the canopy and soil seed banks of Pinus yunnanensis in central Yunnan, China. For. Ecol. Manage. 437, 41–48. doi: 10.1016/j.foreco.2019.01.002
Sun, L., Cai, Y., Zhou, Y., Shi, S., Zhao, Y., Gunnarson, B. E., et al. (2020a). Radial Growth Responses to Climate of Pinus yunnanensis at Low Elevations of the Hengduan Mountains, China. Forests 11, 1066. doi: 10.3390/f11101066
Sun, Y.-Q., Zhao, W., Xu, C.-Q., Xu, Y., El-Kassaby, Y. A., de la Torre, A. R., et al. (2020b). Genetic variation related to high elevation adaptation revealed by common garden experiments in pinus yunnanensis. Front. Genet. 10. doi: 10.3389/fgene.2019.01405
Tan, T., Li, S., Fan, Y., Wang, Z., Ali Raza, M., Shafiq, I., et al. (2022). Far-red light: A regulator of plant morphology and photosynthetic capacity. Crop J. 10, 300–309. doi: 10.1016/j.cj.2021.06.007
Turcotte, M. M., Levine, J. M. (2016). Phenotypic plasticity and species coexistence. Trends Ecol. Evol. 31, 803–813. doi: 10.1016/j.tree.2016.07.013
Urbaniak, L., Karliński, L., Popielarz, R. (2011). Variation of morphological characters of Scots pine (Pinus sylvestris L.) populations in different habitats. Acta Soc Bot. Pol. 72, 37–44. doi: 10.5586/asbp.2003.005
Valladares, F., Gianoli, E., Gómez, J. M. (2007). Ecological limits to plant phenotypic plasticity. New Phytol. 176, 749–763. doi: 10.1111/j.1469-8137.2007.02275.x
Valladares, F., Matesanz, S., Guilhaumon, F., Araújo, M. B., Balaguer, L., Benito-Garzón, M., et al. (2014). The effects of phenotypic plasticity and local adaptation on forecasts of species range shifts under climate change. Ecol. Lett. 17, 1351–1364. doi: 10.1111/ele.12348
Vasey, G. L., Weisberg, P. J., Urza, A. K. (2022). Intraspecific trait variation in a dryland tree species corresponds to regional climate gradients. J. Biogeogr. 49, 2309–2320. doi: 10.1111/jbi.14515
Violle, C., Navas, M., Vile, D., Kazakou, E., Fortunel, C., Hummel, I., et al. (2007). Let the concept of trait be functional! Oikos. Oikos 116, 882–892. doi: 10.1111/j.0030-1299.2007.15559.x
Wang, Y., Chen, X., Gao, M., Dong, J. (2022). The use of random forest to identify climate and human interference on vegetation coverage changes in southwest China. Ecol. Indic. 144, 109463. doi: 10.1016/j.ecolind.2022.109463
Wang, C., Wang, J., Jiang, H. (2004). A Study on Comparative Anatomy of Pinus yunnanensis Needles under Different Habitats. J. Southwest For. Coll. 24, 1–5. doi: 10.11929/j.issn.2095-1914.2004.01.001
Wang, L.-L., Zhou, Q.-Q., Su, W.-H., Xu, Y.-J., Qian, Q.-D., Yang, X., et al. (2023). Responses of fungal community to forest fire are species-specific in Yunnan Plateau, southwest China. J. Plant Ecol. 16, 43. doi: 10.1093/jpe/rtac043
Wright, I. J., Reich, P. B., Cornelissen, J. H. C., Falster, D. S., Groom, P. K., Hikosaka, K., et al. (2005). Modulation of leaf economic traits and trait relationships by climate. Glob. Ecol. Biogeogr. 14, 411–421. doi: 10.1111/j.1466-822x.2005.00172.x
Xiang, Y., Li, Y., Luo, X., Liu, Y., Huang, P., Yao, B., et al. (2022). Mixed plantations enhance more soil organic carbon stocks than monocultures across China: Implication for optimizing afforestation/reforestation strategies. Sci. Total Environ. 821, 153449. doi: 10.1016/j.scitotenv.2022.153449
Xu, Y., Cai, N., Woeste, K., Kang, X., He, C., Li, G., et al. (2016a). Genetic diversity and population structure of pinus yunnanensis by simple sequence repeat markers. For. Sci. 62, 38–47. doi: 10.5849/forsci.15-064
Xu, W., Tomlinson, K. W., Li, J. (2020). Strong intraspecific trait variation in a tropical dominant tree species along an elevational gradient. Plant Divers. 42, 1–6. doi: 10.1016/j.pld.2019.10.004
Xu, Y., Woeste, K., Cai, N., Kang, X., Li, G., Chen, S., et al. (2016b). Variation in needle and cone traits in natural populations of Pinus yunnanensis. J. For. Res. 27, 41–49. doi: 10.1007/s11676-015-0153-6
Zhao, Y.-T., Ali, A., Yan, E.-R. (2016). The plant economics spectrum is structured by leaf habits and growth forms across subtropical species. Tree Physiol. 37, 173–185. doi: 10.1093/treephys/tpw098
Zhao, C., Chen, L., Ma, F., Yao, B., Liu, J. (2008). Altitudinal differences in the leaf fitness of juvenile and mature alpine spruce trees (Picea crassifolia). Tree Physiol. 28, 133–141. doi: 10.1093/treephys/28.1.133
Keywords: Pinus yunnanensis, intraspecific variation, functional traits, phenotypic plasticity, adaptive traits
Citation: Song X, Liu Y, Yu D, Li S, Gao W, Zeng H, Cao D, Zhou S and Cui X (2024) Environmental determinants of intraspecific variation in five functional traits of Pinus yunnanensis Franch. Front. Plant Sci. 15:1501584. doi: 10.3389/fpls.2024.1501584
Received: 25 September 2024; Accepted: 11 November 2024;
Published: 11 December 2024.
Edited by:
Congcong Liu, Minzu University of China, ChinaReviewed by:
Guoyuan Liu, Nantong University, ChinaYuan Li, Natural Resources Institute Finland (Luke), Finland
Copyright © 2024 Song, Liu, Yu, Li, Gao, Zeng, Cao, Zhou and Cui. This is an open-access article distributed under the terms of the Creative Commons Attribution License (CC BY). The use, distribution or reproduction in other forums is permitted, provided the original author(s) and the copyright owner(s) are credited and that the original publication in this journal is cited, in accordance with accepted academic practice. No use, distribution or reproduction is permitted which does not comply with these terms.
*Correspondence: Xinglei Cui, eGluZ2xlaS5jdWlAc2ljYXUuZWR1LmNu
†These authors have contributed equally to this work
‡ORCID: Ying Liu, orcid.org/0009-0004-0644-3184
Wuchao Gao, orcid.org/0000-0002-9832-6273
Xinglei Cui, orcid.org/0000-0002-0853-8986