- 1State Key Laboratory of Crop Gene Exploration and Utilization in Southwest China, Rice Research Institute, Sichuan Agricultural University, Chengdu, China
- 2Department of Research and Development, Luzhou Taifeng Seed Industry Co., Ltd., Luzhou, Sichuan, China
- 3Department of Research and Development, Zoeve Seed Co., Ltd., Chengdu, Sichuan, China
Crop resistance to herbicides is crucial for agricultural productivity and sustainability amidst escalating challenges of weed resistance. Uncovering herbicide resistant genes is particularly important for rice production. In this study, we tested the resistance to three commonly used herbicides: glufosinate, glyphosate and mesotrione of 421 diverse rice cultivars and employed genome-wide association studies (GWAS) to unravel the genetic underpinnings of resistance to these three herbicides in rice. We discovered that cultivated rice exhibited rich variation in resistance to the three herbicides, and the differences among subpopulations were significant. Six identified associations harboring candidate genes for resistance to these herbicides were significant. Among them, RGlu6 and RGly8 were the major QTL for resistance to glufosinate and glyphosate, respectively. The favorable alleles of RGlu6 and RGly8 were primarily present in japonica cultivars that originated from Europe, highlighting the geographic and genetic diversity of herbicide resistance and emphasizing the localized selection pressures in European rice varieties. Moreover, our findings might suggest that traditional target genes may not contain tolerant alleles in nature, and alternative mechanisms with novel loci associated with resistance may work. By mapping the genes for herbicide resistance, our results may help develop new strategies to combat the dual challenges on effective weed management and herbicide sustainability.
Introduction
Rice is a crucial staple food for more than half of the global population, playing an essential role in agricultural production, especially in Asian countries (FAO, http://www.fao.org). Weeds pose a horrible threat to rice by competing for nutrients, water, sunlight, and space, and by increasing the incidence of diseases and insect pests (Akbar et al., 2011). This competition can lead to substantial reductions in rice yield and grain quality, up to over 40% due to weed damage (Burgos et al., 2014). The shift from transplanting to direct seeding, driven by urbanization and labor shortages has exacerbated weed problems (Chaudhary et al., 2023). Traditional methods for weed control, such as tillage, irrigation, hand-weeding etc., are labor-intensive and resource-demanding. Using herbicides is the most effective way to control weeds, but many herbicides also damage or kill rice plants (Jin et al., 2022). The development and use of herbicide-resistant cultivars for an effective control of weeds without harming the plant have therefore become critical in modern agricultural practices (Kawahara et al., 2013).
Glufosinate, glyphosate and mesotrione are three widely used herbicides and significant progress has been made in understanding and developing resistant plants to these herbicides. As a major competitive inhibit target of glufosinate, glutamine synthase (GS) plays a pivotal role in plants’ resistance to glufosinate and mutations in GS genes could enhance plants’ resistance to glufosinate (Tian et al., 2015). Glyphosate is a broad-spectrum post-emergent herbicide and mainly targets 5-enolpyruvylshikimate-3-phosphate synthase (EPSPS), a key enzyme in the biosynthesis of aromatic amino acids, and phenolics, and gain of function of EPSPS genes could endow plants with resistance to glyphosate (Sammons and Gaines, 2014). It has been reported that acetyltransferases (ACEs) synthesizing genes can be induced by mesotrione, indicating a role for ACEs in resistance to mesotrione of crops (Chen et al., 2022). In addition, other genes like OsACC1 which encodes acetyl-CoA carboxylase and OsALS1 which encodes acetolactate synthase were reported to be responsible for resistance to herbicides like aryloxyphenoxypropionates (APPs) and pyrimidinyl carboxy (PC) herbicides, respectively (Okuzaki et al., 2007; Xu et al., 2021). Researchers have successfully created herbicide-resistant rice varieties through artificial mutagenesis, genome editing, introducing and overexpression of these genes (Li et al., 2016; Maeda et al., 2019; Chen et al., 2021; Ren et al., 2023; Zhang et al., 2023). Despite these advancements, the genetic resources for herbicide resistance are still limited in rice. The evolution of herbicide-resistant weeds due to the extensive use of chemical control strategies has further complicated this issue. For instance, over 255 weed species worldwide have developed resistance to at least one herbicide, including 42 species resistant to glyphosate (Perotti et al., 2019). This situation underscores the need for continuous research and development of new herbicide resistance mechanisms and the identification of novel resistance genes to ensure sustainable rice production.
Genome-wide association studies (GWAS) have emerged as a powerful tool for dissecting the genetic basis of complex quantitative traits in plants (Zhou et al., 2017, 2021a, 2021b). This method enables the identification of genetic variants associated with specific traits by analyzing the entire genome (Xia et al., 2022). In this study, we employed GWAS to investigate the genetic basis of resistance to three different herbicides in a diverse rice germplasm population. This approach allowed us to identify key genetic loci associated with herbicide resistance, providing valuable insights into the development of new herbicide-tolerant rice varieties. By leveraging the genetic diversity present in natural populations, GWAS offers a robust framework for enhancing our understanding of herbicide resistance and for breeding rice varieties with improved resistance profiles.
Materials and methods
Plant materials and phenotyping
The 421 cultivated rice varieties used in this study were selected from diverse germplasms collected domestically and internationally, and the names, geographical origin, and subpopulation information of which have been previously reported (Zhao et al., 2015). In 2022, these rice varieties were planted at the South Breeding Base of Sichuan Agricultural University in Lingshui County, Hainan Province, China. The seeds of each were individually harvested in April 2023, uniformly air-dried and placed in the seed storage facility in the Rice Research Institute of Sichuan Agricultural University in Chengdu, Sichuan Province.
Sixty grains of each accession were sown in a flowerpot (15 cm diameter and 16 cm height) in July 2023 with three replications for herbicide evaluation in the Chengdu Campus of Sichuan Agricultural University. Half-lethal dose of glyphosate, glufosinate and mesotrione solutions was sprayed, respectively at the 3-4 leaf stage as 2.25 L/hm2 of 41% glyphosate (Fuhua Chemical), 3.00 L/hm2 of 20% glufosinate (BASF) and 1.20 L/hm2 of 10% mesotrione (Hubei Jiahui Xingcheng Biotechnology Co., Ltd.). The response of each pot to herbicide injury was scored with 0-4 scale five days after the application of glyphosate and glufosinate, but seven days after the application of mesotrione, respectively, where 0 was for the plants with no apparent injury, level 1 for slight effect on seedlings, level 2 for moderate effect, level 3 for severe effect and 4 with the most severe injury.
Genome wide association study
GWAS analyses for herbicide resistance traits were performed for the entire panel and for indica and japonica group separately, using mixed linear models to take population structure and relative kinship into consideration for statistical association. SNPs with a minor allele frequency (MAF) greater than 5% and a missing rate less than 15% were selected for the association analysis, and 6.3 million variants, including 5.5 million SNPs and 800,000 InDels, were used for GWAS, as well as estimating population structure and kinship coefficients. The thresholds of genome-wide significance were determined using a modified Bonferroni correction as described by Li et al. (2012), where the total number of SNPs (M) used for threshold calculation was replaced by the effective number of SNPs (Me), in mixed linear models provided by EMMAX program (Kang et al., 2010). The physical locations of the SNPs and InDels were identified based on the Rice Annotation version 7.0 of the variety Nipponbare (Kawahara et al., 2013) from the website http://rice.uga.edu/.
The Bayesian clustering program fast Structure (Raj et al., 2014) was used to calculate different levels of K (K = 2-5), and the command choose_K.py was employed to identify the model complexity for maximizing the marginal likelihood. LD heatmaps and gene haplotypes were constructed using SNPs and InDels (MAF >0.03) from the 1 Mb regions around each peak SNP using R package ‘LD heatmap’ (Shin et al., 2006).
Variant-function-based association analyses
We annotated the functional variations within a 1 Mb region surrounding the associated loci using snpEff software (Cingolani et al., 2012), and classified all polymorphisms in the candidate region into four groups, as for 1) those predicted to induce amino acid exchanges or to change splicing junctions (GT or AG at the beginning or end of an intron, respectively), 2) those located at the 5′ flanking sequences of genes (e.g., within 2kb of the first ATG, typically the promoter region), 3) those located within a gene but did not meet the criteria for Group I or II (for example, located in a coding region but not predicted to change an amino acid, an intron, or a 3′ noncoding sequence), and 4) those located outside of coding regions. By identifying the most significant variant in Group I, potential candidate genes were determined.
Gene haplotype and geographic distribution analyses
The haplotype of each candidate gene was analyzed by downloading the genotypes of variant sites located 2kb upstream of the transcription start site and within the coding sequence of the gene from the RiceVarMap 2.0 (ricevarmap.ncpgr.cn) (Zhao et al., 2021). To improve the efficiency of the haplotype analysis, those variant sites with a minor allele frequency less than 0.05 and a missing rate greater than 10% were filtered out. The genotypes of remaining high-quality variants were used for haplotype classification. To better display the distribution pattern of haplotypes, only those haplotypes containing more than 10 varieties were shown. The information on geographical distribution of the 421 accessions was also downloaded from the RiceVarMap website. The world map was plotted using the ‘maps’ package in R (Becker and Wilks, 1995), and the characteristics of different haplotypes on geographical distribution were displayed in pie chart form using the ‘ape’ package in R (Paradis and Schliep, 2019).
Statistical analyses
Violin plots, correlations, and multiple comparisons were constructed using phenotypic means from three replicates for each accession. The P values for Pearson’s correlation coefficients were calculated by two-sided t-tests using the cor.test() function in R (Ihaka and Gentleman, 1996). Multiple comparisons of phenotypic data among different subgroups or haplotypes were conducted using the duncan.test() command in the ‘agricolae’ package in R, with a significant probability at 0.01. The ‘vioplot’ package in R was used to create violin plots for the multiple comparisons (https://github.com/TomKellyGenetics/vioplot).
Result
The distribution of three herbicide resistance in rice germplasm
By grading the herbicide resistance, we observed significant differences among the 421 accessions responding to the three herbicides, indicating the feasibility of the grading standards (Figures 1A; Supplementary Figure S1). The cultivars generally exhibited low resistance to all three herbicides, with average resistance ratings around level 3. Less than 5% cultivars exhibited relatively high resistance (resistance ratings smaller than level 2 to the three herbicides, and a Temperate Japonica cultivar HB-6-2 was the relatively highest glufosinate and glyphosate resistant cultivar in the tested materials (Supplementary Figure S1; Supplementary Tables S4, S5). There is a significant positive correlation between the resistance to the three herbicides in cultivated rice (r2 = 0.26,0.13,0.14), although the correlation coefficients are relatively low (Figure 1B). Among them, the positive correlation between glufosinate resistance and glyphosate resistance is the most significant, with a correlation coefficient of 0.26 (p= 7.5 × 10-5).
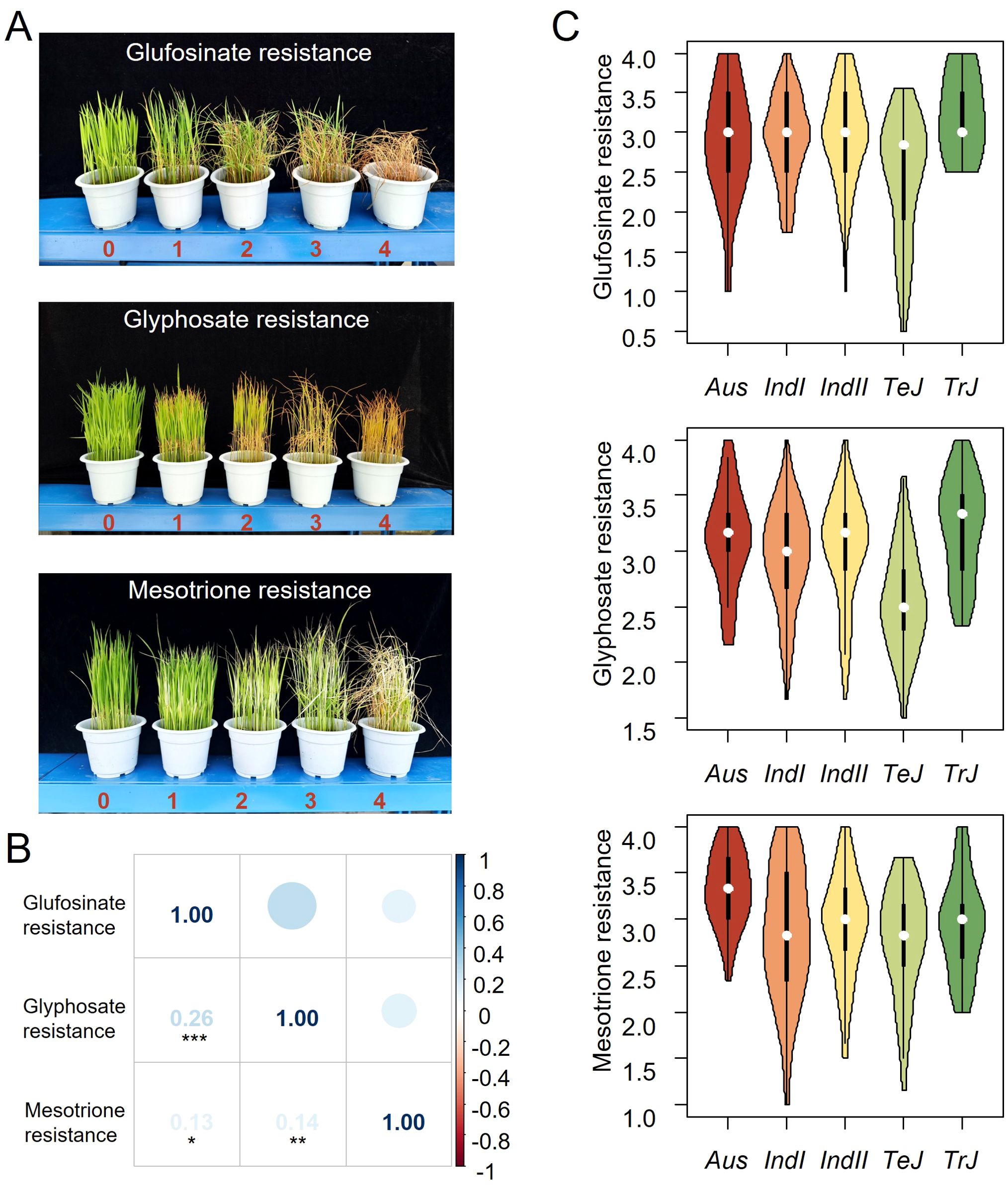
Figure 1. Identification, phenotypic distribution, and correlation of resistance to three herbicides in cultivated rice. (A) Grading criteria for tolerance to three herbicides. (B) Correlation among three herbicide tolerances in cultivated rice. (C) Phenotypic distribution of tolerance to three herbicides across different subgroups in cultivated rice.
Different subpopulations varied in resistance to the three herbicides
A total of 6.3 million high-quality variants, including 5.5 million SNPs and 0.8 million InDels, queried from RiceVarMap (http://ricearmap.ncpgr.cn), were used to estimate population structure and Kinship coefficients, as well as GWAS. When K equaled 2, the 421 accessions in the whole panel were divided into 300 Indica, 106 Japonica and 15 Intermediate ones. And When K equaled 5, the 300 Indica accessions were further divided into 38 Aus, 143 Indica I (IndI), 119 Indica II (Ind II), while the 106 Japonica accessions were divided into 60 Temperate Japonica (TeJ) and 46 Tropical Japonica (TrJ). Based on this population structure, we noticed a varying resistance to different herbicides of different subgroups (Figure 1C). For glufosinate and glyphosate, the TeJ subgroup exhibited significantly higher resistance than other subgroups (Figure 1C); for halosulfuron-methyl, the Aus subgroup showed significantly lower resistance than other subgroups. Thus, the cultivars with different genetic backgrounds demonstrated diverse herbicide resistance.
Loci associated with herbicide resistance
The strict inbreeding mating habits of rice have led to significant subpopulation structure and considerable linkage disequilibrium (LD), which reduce the mapping resolution of association studies and increase the occurrence of type I errors (Zhou et al., 2017). To address these issues, we used a mixed model to correct the interferences from subpopulation structure by incorporating the first three principal components (PCs) as covariates in the model, and conducted genome-wide association studies (GWAS) in the Indica group (303 accessions), Japonica group (181 accessions), and the entire group (all varieties) (see Methods). The calculated genome-wide significance thresholds, based on a nominal level of 0.05, were P = 6.6 × 10-8, 8.7 × 10-8, and 2.0 × 10-7 for the whole panel, Indica and Japonica, respectively (Supplementary Table S1).
Five significant loci associated with resistance to glufosinate and glyphosate, but none to mesotrione were identified in the whole panel (Figure 2; Supplementary Figures S2, S3). Detailed association results for each herbicide in each subpopulation are presented in Table 1. Three loci associated with glufosinate resistance in the whole panel totally explained 25.76% of the phenotypic variance, with effect of each varying from 15.95% to 19.20% (Supplementary Table S2). For glyphosate resistance, two loci accounted for 26.69% of the phenotypic variance in total, and 5.15% and 20.06% alone, indicating a significant role of the additive effects on the variation of glyphosate resistance (Supplementary Table S3).
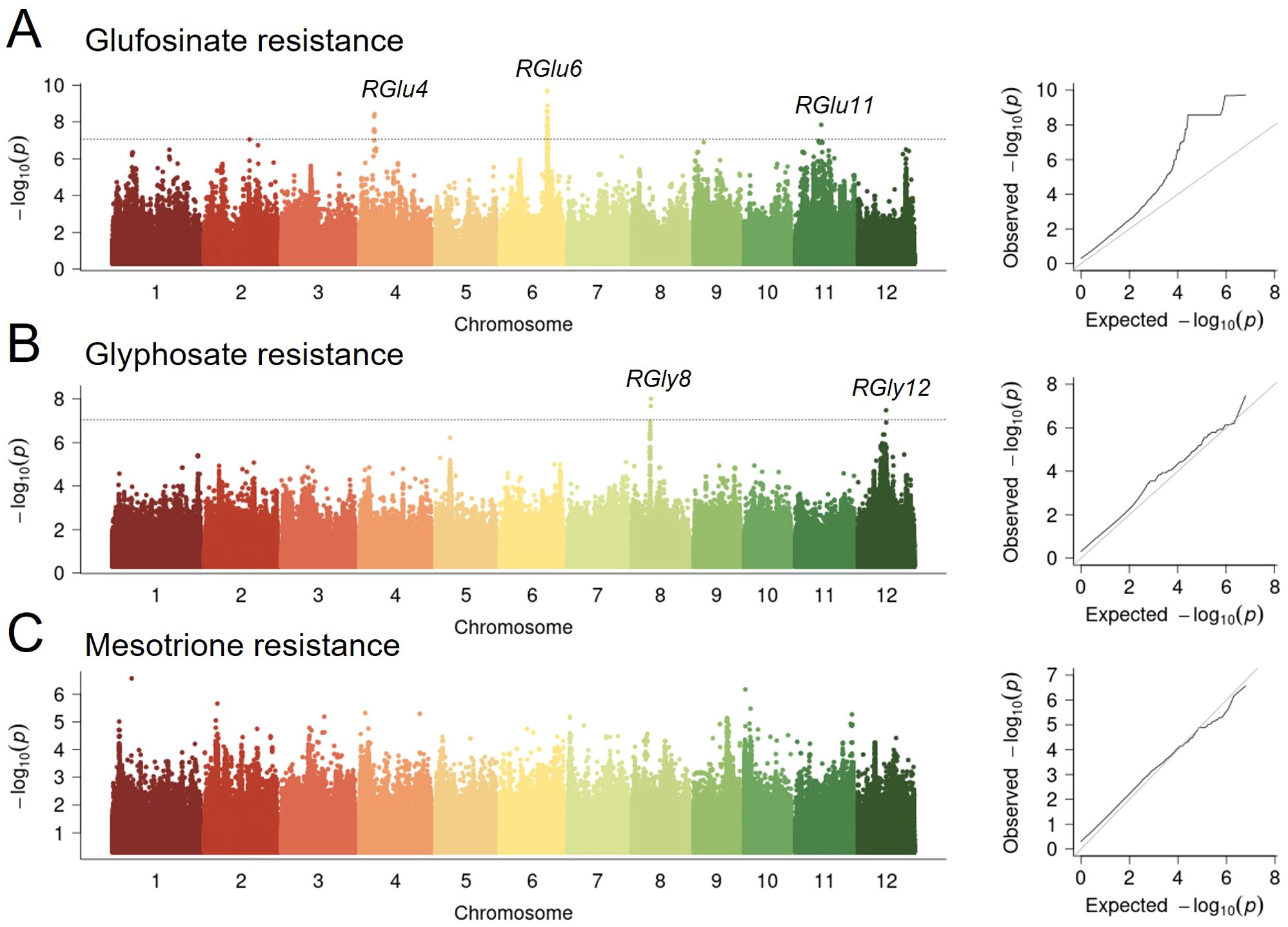
Figure 2. Genome-wide association results for resistance to three herbicides in 421 cultivated rice. Manhattan plots and quantile–quantile plots depicting GWAS results using a mixed model for tolerance to glufosinate (A), glyphosate (B) and mesotrione (C). Associations identified in all accessions (lower), indica subspecies (middle), and japonica subspecies (upper). The x axis depicts the physical location of SNPs across the 12 chromosomes of rice and the y axis depicts the -log10 (P value).
Functional and haplotype analyses of RGlu6 for rice glufosinate resistance
Since RGlu6 explained the highest phenotypic variation (R2 = 19.20%, Supplementary Table S2), we chose it as a major QTL for rice glufosinate resistance and conducted variant-function-based association analysis of the associated loci regions on RGlu6 (see Methods). By analyzing the function of variants within 500 kb upstream and downstream of the peak SNP and the linkage disequilibrium (LD) blocks in that segment (Figures 3A, B), we analyzed the candidate genes and functional variants of RGlu6. LD decay around RGlu6 was rapid with no obvious LD blocks, where the most significant functional variant (vg0623197432) was only 6 kb away from the peak SNP (Table 1). A significant glufosinate resistance difference between type A and type G of vg0623197432 variant was observed (p= 1.8×10-10, Figure 3C). Since variant vg0623197432 was located on the exon of LOC_Os06g39070, which encodes a UDP-glucosyl transferase (UGT), we consider it as a candidate gene for RGlu6.
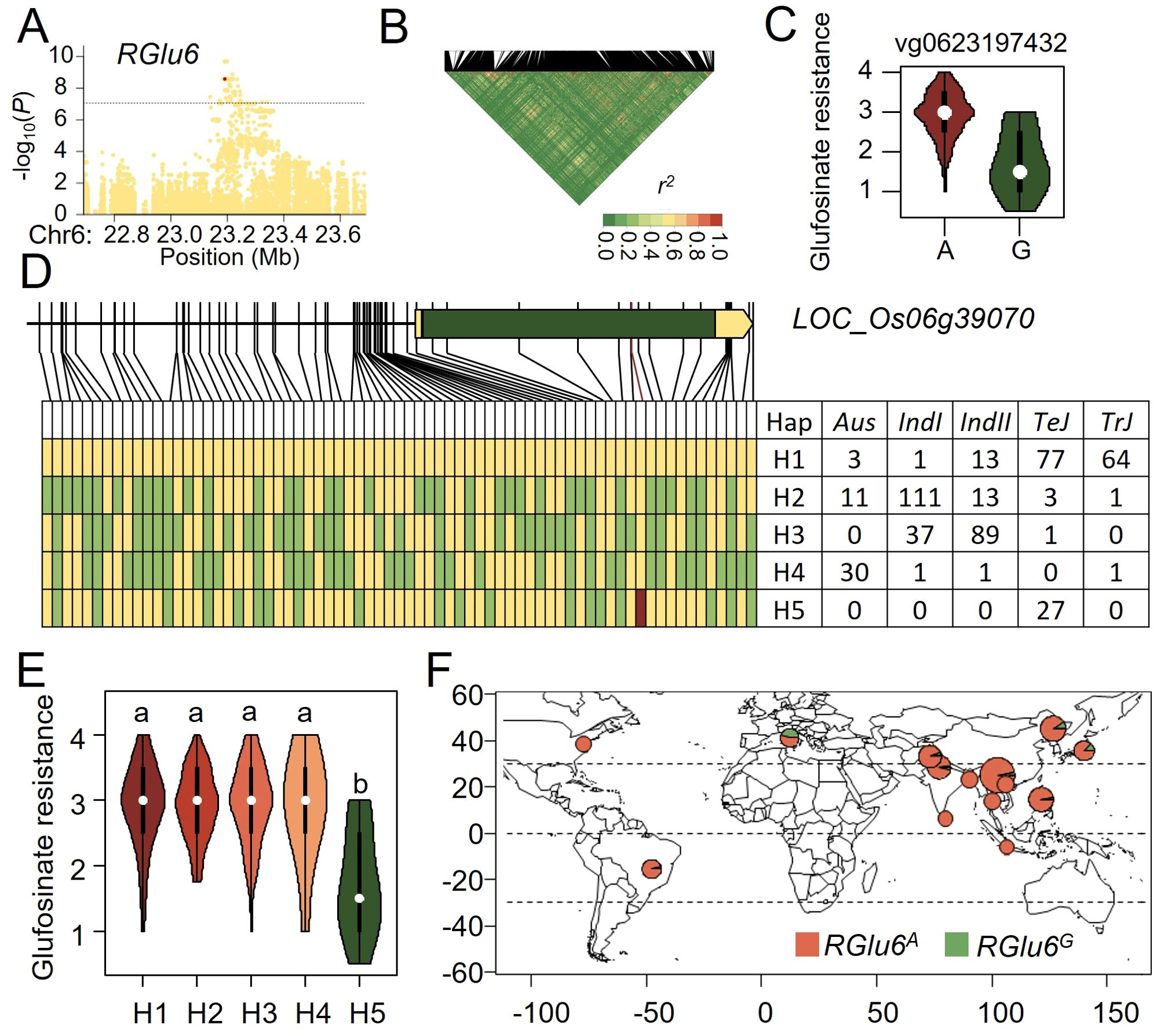
Figure 3. Natural variation of RGlu6 affect glufosinate resistance in rice. (A) Manhattan plot for the 1 Mb region around the glufosinate resistance-associated locus RGlu6. (B) LD heatmap for the region around RGlu6. (C) Potential functional variants at RGlu6 and their effects on glufosinate tolerance. (D) Haplotype analysis of the candidate gene at RGlu6. Yellow represents the reference genome genotype, green represents alternate genotypes, and red represents functional variants. (E) Phenotypic distribution of the five haplotypes at RGlu6. (F) Geographic distribution of accessions with different RGlu6 allele in the GWAS panel.
Using the genotypes of the 2 kb promoter and coding region variations of LOC_Os06g39070 with 61 SNPs and 13 InDels, we categorized RGlu6 of these 421 cultivars into 5 haplotypes, namely H1 - H5 (Figure 3D). Haplotype H1 was predominantly present in TeJ and TrJ, H2 and H3 mainly in IndI and IndII, H4 in Aus, and H5 only in TeJ, respectively. Multiple comparison demonstrated no significant differences were among haplotypes H1-H4, while haplotype H5 exhibited significantly higher resistance to glufosinate than others, indicating beneficial allelic variations of H5 haplotype (Figure 3E). Thus, we classified RGlu6 into two allelic genotypes with merging the indistinct H1-H4 into RGluA genotype, and considering H5 as RGluG genotype. By analyzing the geographic distribution of these allelic genotypes (Supplementary Table S4), we observed that the accessions with the RGlu6G genotype mainly originated from Europe (Figure 3F), which indicates that RGlu6G may have arisen from a mutation and was retained locally through natural selection.
Functional and haplotype analyses of RGly8 for rice glyphosate resistance
Analyzing candidate genes at the RGly8 locus for rice glyphosate resistance in a rapid LD decay around RGly8, we found that vg0808951557 was the most significant functional variant located only 7 kb from the peak SNP (Figures 4A, B). The T to C mutation of vg0808951557 which was in the fourth exon of LOC_Os08g14850, led to an amino acid changed from serine to proline (Figure 4C). Since LOC_Os08g14850 encodes a resistance protein and was reported to be involved biotic and abiotic stresses, we considered it as a candidate gene for RGly8.
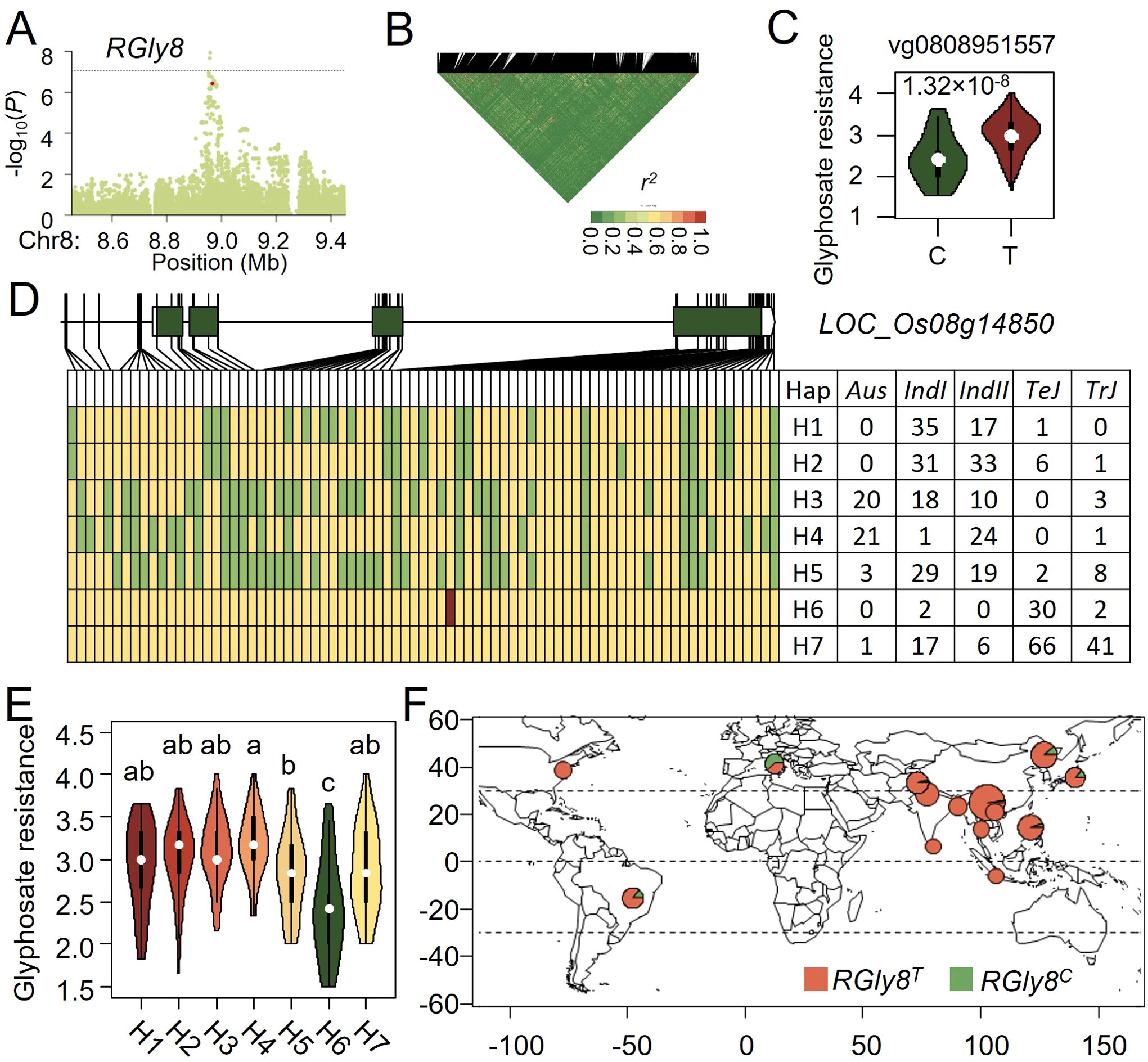
Figure 4. Natural variation of RGly8 affect glyphosate resistance in rice. (A) Manhattan plot for the 1 Mb region around the glyphosate resistance-associated locus RGly8. (B) LD heatmap for the region around RGly8. (C) Potential functional variants at RGlu6 and their effects on glyphosate tolerance. (D) Haplotype analysis of the candidate gene at RGly8. Yellow represents the reference genome genotype, green represents alternate genotypes, and red represents functional variants. (E) Phenotypic distribution of the five haplotypes at RGly8. (F) Geographic distribution of accessions with different RGly8 allele in the GWAS panel.
Seventy four SNPs and five InDels were used to analyze the haplotypes of RGly8 and seven haplotypes, namely H1 - H7 were categorized based on the glyphosate resistance and genotypes of the 421 accessions (Figure 4D). Haplotypes H1-H5 were predominantly present in Indica, while H6 and H7 were mainly present in Japonica. The superior C genotype of vg0808951557 was exclusively found in the H6 haplotype. Multiple comparisons revealed significant differences for glyphosate resistance among these seven haplotypes, with H6 haplotype exhibiting a significantly higher glyphosate resistance than other haplotypes (Figure 4E). Therefore, we designated the H6 haplotype as the allelic genotype RGly8C, and the other haplotypes as RGly8T. Analysis of the geographic distribution of these alleles showed that the superior allele RGly8C primarily originated from Europe, the same as that of RGlu6G (Figure 4F; Supplementary Table S4).
These results suggested that resistance to both glufosinate and glyphosate may have been locally selected in Europe, leading to an increase in the frequency of superior resistance alleles in rice accessions originated from that region.
Discussion
The intense application of herbicides has effectively assisted in weeds control but meanwhile accelerated the evolution of resistance to herbicides of both crops and weeds (Peterson et al., 2018). Discovering and developing herbicide-resistant crop is one of the most promising ways for weed control and crop production. In this study, we tested the resistance to three frequently herbicides, namely glufosinate, glyphosate and mesotrione, of 421 diverse rice accessions. Varying degrees of resistance to these herbicides were discovered (Supplementary Figure S1), indicating the possibility of natural herbicide-resistant rice varieties. Besides, a relatively higher resistance in average to these three herbicides in TeJ compared to other subpopulations were observed (Figure 1C). Since TeJ mainly originated from Europe, a higher herbicides selection pressure in Europe might be the cause (Peterson et al., 2018).
GS and EPSPS are the major competitively inhibited targets of glufosinate and glyphosate, respectively, and rice varieties endowed with resistance to these two herbicides were mostly genetically modified on genes encoding GS and EPSPS (Achary et al., 2020). OsGS1;1 and OsGS2, encoding glutamine synthetase, were reported to be involved in rice growth and resistance to abiotic stresses, but the resistance to glufosinate has not been reported (Cai et al., 2010; James et al., 2018). Our study aimed to identify natural variant loci associated with resistance to either of glufosinate and glyphosate in rice through GWAS, but none was detected in their target gene regions (Figures 2A, B, Supplementary Figures S2, S3). This result suggested that either these target genes might not possess alleles to confer natural herbicide resistance, or such natural resistance alleles may be either too weak or too rare to be detected by GWAS.
Since the 421 geographically and genetically diverse rice cultivars displayed various resistance to the three herbicides (Figure 1C, Supplementary Figure S1) and QTL which were reported to be associated with resistance to these herbicides did not overlap with neither EPSPS genes nor GS genes (Bao et al., 2022; Xu et al., 2023), alternative mechanisms of plant herbicide resistance beyond just reducing the direct interaction between the target gene protein and the herbicide might exist. In the association analysis for glufosinate resistance, we defined LOC_Os06g39070 which encodes a UDP-glucosyl transferase (UGT) as a candidate gene. UGT is a class of enzymes widely found in plants, whose primary function is to transfer glucose units from uridine diphosphate glucose (UDP-glucose) to a variety of acceptor molecules, including hormones, toxins, and secondary metabolites (Lairson et al., 2008; Tiwari et al., 2016). In rice, UGT genes participates in response to abiotic and biotic stresses, and gain of function of OsUGTs could improve rice resistance to various stresses through elevating scavenging reactive oxygen species (ROS) and plant hormones (Wang et al., 2021, 2023; He et al., 2023). Thus, LOC_Os06g39070 might reduce the toxicity of glufosinate through hormones or ROS scavenging processes. In the association analysis for glyphosate resistance, LOC_Os08g14850 which encodes resistance protein CC-NBS-LRR was detected (Figures 2B, 4). Previous studies have shown that resistance proteins play a role not only in plant responses to biotic stress but also in responses to abiotic stress (Atkinson and Urwin, 2012). LOC_Os08g14850/SCR8 has been reported to be involved in rice’s resistance to bacterial blight and mutant scr8 displayed a defected root growth phenotype (Hu et al., 2021). Taking these together, LOC_Os08g14850 might be a potential target for enhancing rice resistance to glyphosate.
Haplotype analysis of RGlu6 and RGly8 revealed that superior alleles of RGlu6 and RGly8 are predominantly present in TeJ (Figures 3D, E, 4D, E). Geographic analysis of RGlu6G and RGlyC showed that either of them were originated from Europe (Figures 3F, 4F). These results indicated a possible severe herbicide pressure in Europe (Devos et al., 2006).
In summary, this study reveals the genetic basis of rice resistance to three herbicides through genome-wide association analysis, offering new insights for deciphering new mechanisms of herbicide resistance and breeding herbicide-resistant rice varieties.
Conclusion
Enhancing rice resistance to herbicides is the most effective way to maintain rice production against weeds. In this study, we tested the resistance to three major herbicides, namely glufosinate, glyphosate and mesotrione of 421 diverse rice cultivars and identified 5 significant associations for rice resistance to glufosinate and glyphosate. By candidate gene-based GWAS, we predicted two genes, LOC_Os06g39070 and LOC_Os08g14850 for rice resistance to glufosinate and glyphosate, and found superior alleles for these two genes against glufosinate and glyphosate, respectively. Our results will shed light on the genetic diversity of rice resistance to herbicides and provide promising targets for enhancing rice resistance to herbicides.
Data availability statement
The datasets presented in this study can be found in online repositories. The names of the repository/repositories and accession number(s) can be found in the article/Supplementary Material.
Author contributions
PX: Conceptualization, Methodology, Supervision, Writing – original draft. YQ: Funding acquisition, Supervision, Writing – review & editing. MM: Data curation, Investigation, Writing – original draft. TL: Formal analysis, Investigation, Writing – review & editing. FR: Investigation, Writing – review & editing. LX: Investigation, Writing – review & editing. JC: Investigation, Writing – original draft. GX: Investigation, Writing – original draft. YC: Investigation, Software, Writing – original draft. HF: Investigation, Writing – original draft. GZ: Investigation, Writing – original draft. YX: Funding acquisition, Supervision, Writing – review & editing. DX: Data curation, Funding acquisition, Investigation, Resources, Writing – review & editing, Conceptualization, Writing – original draft.
Funding
The author(s) declare financial support was received for the research, authorship, and/or publication of this article. This work was supported by the Science and Technology Department of Sichuan Province, China (Project No. 2023NSFSC1933, 2023NSFSC0215, and 2024NSFSC0333), the Sichuan National Science Foundation Innovation Research Group (Project No 2023NSFSC 1996), and the Bureau of Science and Technology of Chengdu City, Sichuan China (Project No 2022-YF09-00036-SN and 2023-YF08-00005-SN).
Conflict of interest
Author YQ was employed by Luzhou Taifeng Seed Industry Co., Ltd. Author YX was employed by Zoeve Seed Co., Ltd.
The remaining authors declare that the research was conducted in the absence of any commercial or financial relationships that could be construed as a potential conflict of interest.
Publisher’s note
All claims expressed in this article are solely those of the authors and do not necessarily represent those of their affiliated organizations, or those of the publisher, the editors and the reviewers. Any product that may be evaluated in this article, or claim that may be made by its manufacturer, is not guaranteed or endorsed by the publisher.
Supplementary material
The Supplementary Material for this article can be found online at: https://www.frontiersin.org/articles/10.3389/fpls.2024.1476829/full#supplementary-material
References
Achary, V. M. M., Sheri, V., Manna, M., Panditi, V., Borphukan, B., Ram, B., et al. (2020). Overexpression of improved EPSPS gene results in field level glyphosate tolerance and higher grain yield in rice. Plant Biotechnol. J. 18, 2504–2519. doi: 10.1111/pbi.v18.12
Akbar, N., Jabran, K., Ali, M. A. (2011). Weed management improves yield and quality of direct seeded rice. Aust. J. Crop Sci. 5, 688–694.
Atkinson, N. J., Urwin, P. E. (2012). The interaction of plant biotic and abiotic stresses: from genes to the field. J. Exp. Bot. 63, 3523–3543. doi: 10.1093/jxb/ers100
Bao, J., Gao, Y., Li, Y., Wu, S., Li, J., Dong, Z., et al. (2022). Genetic analysis and fine mapping of zmGHT1 conferring glufosinate herbicide tolerance in maize (Zea mays L.). Int. J. Mol. Sci. 23, 11481. doi: 10.3390/ijms231911481
Becker, R. A., Wilks, A. R. (1995). Constructing a Geographical Database, Statistics Research Report [95.2]. Murray Hill, NJ, USA: AT&T Bell Laboratories.
Burgos, N. R., Singh, V., Tseng, T. M., Black, H., Young, N. D., Huang, Z., et al. (2014). The impact of herbicide-resistant rice technology on phenotypic diversity and population structure of United States weedy rice. Plant Physiol. 166, 1208–1220. doi: 10.1104/pp.114.242719
Cai, H. M., Xiao, J. H., Zhang, Q. F., Lian, X. M. (2010). Co-suppressed glutamine synthetase2 gene modifies nitrogen metabolism and plant growth in rice. Chin. Sci. Bull. 55, 823–833. doi: 10.1007/s11434-010-0075-9
Chaudhary, A., Venkatramanan, V., Kumar Mishra, A., Sharma, S. (2023). Agronomic and environmental determinants of direct seeded rice in south asia. Circul. Econo. Sustain. 3, 253–290. doi: 10.1007/s43615-022-00173-x
Chen, L., Gu, G., Wang, C., Chen, Z., Yan, W., Jin, M., et al. (2021). Trp548Met mutation of acetolactate synthase in rice confers resistance to a broad spectrum of ALS-inhibiting herbicides. Crop J. 9, 750–758. doi: 10.1016/j.cj.2020.11.003
Chen, Z. J., Liu, J., Zhang, N., Yang, H. (2022). Identification, characterization and expression of rice (Oryza sativa) acetyltransferase genes exposed to realistic environmental contamination of mesotrione and fomesafen. Ecotoxicol. Environ. Saf. 233, 113349. doi: 10.1016/j.ecoenv.2022.113349
Cingolani, P., Platts, A., Wang, L. L., Coon, M., Nguyen, T., Wang, L., et al. (2012). A program for annotating and predicting the effects of single nucleotide polymorphisms, SnpEff: SNPs in the genome of Drosophila melanogaster strain w1118; iso-2; iso-3. Fly (Austin). 6, 80–92. doi: 10.4161/fly.19695
Devos, Y., Reheul, D., De Schrijver, A., Cors, F., Moens, W. (2006). Management of herbicide-tolerant oilseed rape in Europe: a case study on minimizing vertical gene flow. Environ. Biosafety Res. 4, 217–222. doi: 10.1051/ebr:2005001
He, Y., Sun, S., Zhao, J., Huang, Z., Peng, L., Huang, C., et al. (2023). UDP-glucosyltransferase OsUGT75A promotes submergence tolerance during rice seed germination. Nat. Commun. 14, 2296. doi: 10.1038/s41467-023-38085-5
Hu, P., Wen, Y., Wang, Y., Wu, H., Wang, J., Wu, K., et al. (2021). Identification and characterization of short crown root 8, a temperature-sensitive mutant associated with crown root development in rice. Int. J. Mol. Sci. 22, 9868. doi: 10.3390/ijms22189868
Ihaka, R., Gentleman, R. (1996). R: a language for data analysis and graphics. J. Comput. Graph. Stat. 5, 299–314. doi: 10.1080/10618600.1996.10474713
James, D., Borphukan, B., Fartyal, D., Ram, B., Singh, J., Manna, M., et al. (2018). Concurrent overexpression of OsGS1;1 and OsGS2 genes in transgenic rice (Oryza sativa L.): Impact on tolerance to abiotic stresses. Front. Plant Sci. 9, 1–19. doi: 10.3389/fpls.2018.00786
Jin, M., Chen, L., Deng, X. W., Tang, X. (2022). Development of herbicide resistance genes and their application in rice. Crop J. 10, 26–35. doi: 10.1016/j.cj.2021.05.007
Kang, H. M., Sul, J. H., Service, S. K., Zaitlen, N. A., Kong, S., Freimer, N. B., et al. (2010). Variance component model to account for sample structure in genome-wide association studies. Nat. Genet. 42, 348. doi: 10.1038/ng.548
Kawahara, Y., de la Bastide, M., Hamilton, J. P., Kanamori, H., McCombie, W. R., Ouyang, S., et al. (2013). Improvement of the Oryza sativa Nipponbare reference genome using next generation sequence and optical map data. Rice 6, 4. doi: 10.1186/1939-8433-6-4
Lairson, L. L., Henrissat, B., Davies, G. J., Withers, S. G. (2008). Glycosyltransferases: structures, functions, and mechanisms. Annu. Rev. Biochem. 77, 521–555. doi: 10.1146/annurev.biochem.76.061005.092322
Li, J., Meng, X., Zong, Y., Chen, K., Zhang, H., Liu, J., et al. (2016). Gene replacements and insertions in rice by intron targeting using CRISPR-Cas9. Nat. Plants 2, 1–6. doi: 10.1038/nplants.2016.139
Li, M.-X., Yeung, J. M. Y., Cherny, S. S., Sham, P. C. (2012). Evaluating the effective numbers of independent tests and significant p-value thresholds in commercial genotyping arrays and public imputation reference datasets. Hum. Genet. 131, 747–756. doi: 10.1007/s00439-011-1118-2
Maeda, H., Murata, K., Sakuma, N., Takei, S., Yamazaki, A., Karim, M. R., et al. (2019). A rice gene that confers broad-spectrum resistance to b-triketone herbicides. Science 365, 393–396. doi: 10.1126/science.aax0379
Okuzaki, A., Shimizu, T., Kaku, K., Kawai, K., Toriyama, K. (2007). A novel mutated acetolactate synthase gene conferring specific resistance to pyrimidinyl carboxy herbicides in rice. Plant Mol. Biol. 64, 219–224. doi: 10.1007/s11103-007-9146-y
Paradis, E., Schliep, K. (2019). ape 5.0: an environment for modern phylogenetics and evolutionary analyses in R. Bioinformatics 35, 526–528. doi: 10.1093/bioinformatics/bty633
Perotti, V. E., Larran, A. S., Palmieri, V. E., Martinatto, A. K., Alvarez, C. E., Tuesca, D., et al. (2019). A novel triple amino acid substitution in the EPSPS found in a high-level glyphosate-resistant Amaranthus hybridus population from Argentina. Pest Manage. Sci. 75, 1242–1251. doi: 10.1002/ps.2019.75.issue-5
Peterson, M. A., Collavo, A., Ovejero, R., Shivrain, V., Walsh, M. J. (2018). The challenge of herbicide resistance around the world: a current summary. Pest Manage. Sci. 74, 2246–2259. doi: 10.1002/ps.2018.74.issue-10
Raj, A., Stephens, M., Pritchard, J. K. (2014). fastSTRUCTURE: variational inference of population structure in large SNP data sets. Genetics 197, 573–589. doi: 10.1534/genetics.114.164350
Ren, Y., Liu, B., Jiang, H., Cheng, W., Tao, L., Wu, K., et al. (2023). Precision editing of GLR1 confers glufosinate resistance without yield penalty in rice. Plant Biotechnol. J. 21, 2417–2419. doi: 10.1111/pbi.v21.12
Sammons, R. D., Gaines, T. A. (2014). Glyphosate resistance: State of knowledge. Pest Manage. Sci. 70, 1367–1377. doi: 10.1002/ps.2014.70.issue-9
Shin, J.-H., Blay, S., McNeney, B., Graham, J. (2006). LDheatmap: an R function for graphical display of pairwise linkage disequilibria between single nucleotide polymorphisms. J. Stat. Software Code Snippets 16, 1–9. doi: 10.18637/jss.v016.c03
Tian, Y. S., Xu, J., Zhao, W., Xing, X. J., Fu, X. Y., Peng, R. H., et al. (2015). Identification of a phosphinothricin-resistant mutant of rice glutamine synthetase using DNA shuffling. Sci. Rep. 5, 1–11. doi: 10.1038/srep15495
Tiwari, P., Sangwan, R. S., Sangwan, N. S. (2016). Plant secondary metabolism linked glycosyltransferases: An update on expanding knowledge and scopes. Biotechnol. Adv. 34, 714–739. doi: 10.1016/j.biotechadv.2016.03.006
Wang, T., Li, X., Liu, X., Yang, X., Li, Y.j., Hou, B.k. (2023). Rice glycosyltransferase gene UGT2 functions in salt stress tolerance under the regulation of bZIP23 transcription factor. Plant Cell Rep. 42, 17–28. doi: 10.1007/s00299-022-02933-3
Wang, T., Ma, Y.q., Huang, X.x., Mu, T.j., Li, Y.j., Li, X.k., et al. (2021). Overexpression of OsUGT3 enhances drought and salt tolerance through modulating ABA synthesis and scavenging ROS in rice. Environ. Exp. Bot. 192, 104653. doi: 10.1016/j.envexpbot.2021.104653
Xia, D., Zhou, H., Wang, Y., Ao, Y., Li, Y., Huang, J., et al. (2022). qFC6, a major gene for crude fat content and quality in rice. Theor. Appl. Genet. 135, 2675–2685. doi: 10.1007/s00122-022-04141-9
Xu, S., Fei, Y., Wang, Y., Zhao, W., Hou, L., Cao, Y., et al. (2023). Identification of a seed vigor–related QTL cluster associated with weed competitive ability in direct–seeded rice (Oryza sativa L.). Rice 16, 45. doi: 10.1186/s12284-023-00664-x
Xu, R., Liu, X., Li, J., Qin, R., Wei, P. (2021). Identification of herbicide resistance OsACC1 mutations via in planta prime-editing-library screening in rice. Nat. Plants 7, 888–892. doi: 10.1038/s41477-021-00942-w
Zhang, C., Zhong, X., Li, S., Yan, L., Li, J., He, Y., et al. (2023). Artificial evolution of OsEPSPS through an improved dual cytosine and adenine base editor generated a novel allele conferring rice glyphosate tolerance. J. Integr. Plant Biol. 65, 2194–2203. doi: 10.1111/jipb.13543
Zhao, H., Li, J., Yang, L., Qin, G., Xia, C., Xu, X., et al. (2021). An inferred functional impact map of genetic variants in rice. Mol. Plant 14, 1584–1599. doi: 10.1016/j.molp.2021.06.025
Zhao, H., Yao, W., Ouyang, Y., Yang, W., Wang, G., Lian, X., et al. (2015). RiceVarMap: a comprehensive database of rice genomic variations. Nucleic Acids Res. 43, D1018–D1022. doi: 10.1093/nar/gku894
Zhou, H., Li, P., Xie, W., Hussain, S., Li, Y., Xia, D., et al. (2017). Genome-wide association analyses reveal the genetic basis of stigma exsertion in rice. Mol. Plant 10, 634–644. doi: 10.1016/j.molp.2017.01.001
Zhou, H., Xia, D., Li, P., Ao, Y., Xu, X., Wan, S., et al. (2021a). Genetic architecture and key genes controlling the diversity of oil composition in rice grains. Mol. Plant 14, 456–469. doi: 10.1016/j.molp.2020.12.001
Keywords: rice, herbicide resistance, genome-wide association studies, genetic diversity, glufosinate, glyphosate, mesotrione
Citation: Xu P, Qin Y, Ma M, Liu T, Ruan F, Xue L, Cao J, Xiao G, Chen Y, Fu H, Zhou G, Xie Y and Xia D (2024) Genome-wide association study reveals the genetic basis of rice resistance to three herbicides. Front. Plant Sci. 15:1476829. doi: 10.3389/fpls.2024.1476829
Received: 06 August 2024; Accepted: 12 September 2024;
Published: 01 October 2024.
Edited by:
Yi-Hong Wang, University of Louisiana at Lafayette, United StatesReviewed by:
Bo Peng, Xinyang Normal University, ChinaJie Hu, Fujian Agriculture and Forestry University, China
Copyright © 2024 Xu, Qin, Ma, Liu, Ruan, Xue, Cao, Xiao, Chen, Fu, Zhou, Xie and Xia. This is an open-access article distributed under the terms of the Creative Commons Attribution License (CC BY). The use, distribution or reproduction in other forums is permitted, provided the original author(s) and the copyright owner(s) are credited and that the original publication in this journal is cited, in accordance with accepted academic practice. No use, distribution or reproduction is permitted which does not comply with these terms.
*Correspondence: Yonghua Xie, eGlleWgyNzI4MTFAMTYzLmNvbQ==; Duo Xia, eGlhZHVvMDYxMEBmb3htYWlsLmNvbQ==
†These authors have contributed equally to this work and share first authorship