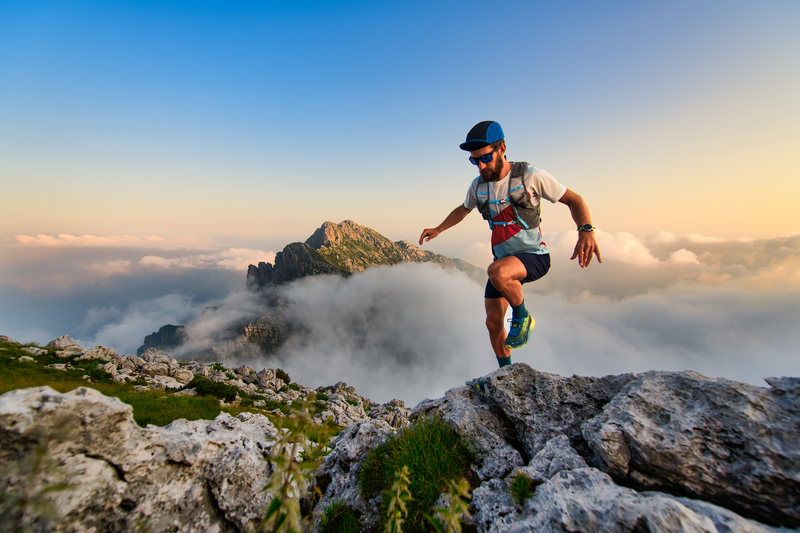
94% of researchers rate our articles as excellent or good
Learn more about the work of our research integrity team to safeguard the quality of each article we publish.
Find out more
ORIGINAL RESEARCH article
Front. Plant Sci. , 02 December 2024
Sec. Functional Plant Ecology
Volume 15 - 2024 | https://doi.org/10.3389/fpls.2024.1476576
Global warming has significantly altered plant phenology by advancing the timing of leaf emergence, impacting vegetation productivity and adaptability. Winter and spring temperatures have commonly been used to explain spring phenology shifts, but we still lack a solid understanding of the effects of interactions between conditions in different seasons. This study utilizes normalized difference vegetation index (NDVI) and meteorological data to examine the effects of changes in winter and spring temperatures and precipitation on the start of the vegetation growing season (SOS) at high latitudes in China from 1982 to 2015. We found that SOS in Northeast China, as a whole, showed a weak advancing trend (moving earlier in the year), but with obvious regional differences. Even within the same vegetation type, changes in SOS were faster in the cold north (1.9 days/decade) and the cold and dry northwest (1.6 days/decade) than the regional averages for deciduous needleleaf forests (DNF; 1.2 days/decade) and grasslands (0.6 days/decade). Increases in spring temperatures dominate forest SOS advancement, while grassland SOS is mainly influenced by winter and spring precipitation. Decreases in winter minimum temperature (Tmin) enhance the spring temperature sensitivity of SOS. The way that winter precipitation regulates the spring temperature sensitivity of SOS differs among vegetation types: increasing sensitivity in grasslands but suppressing it in DNF. The moderating effects of winter conditions account for the greatest part of the regional differences in the magnitude of change in SOS. Our findings highlight that, although rising spring temperatures significantly affect SOS, winter Tmin and precipitation are crucial for understanding spatial SOS differences, particularly in cold, arid high-latitude regions. Winter conditions play an essential role in regulating the response of vegetation SOS to spring climate at high latitudes. These results suggest that considering the moderating effect of winter climate can facilitate more accurate predictions of temperature-driven phenological changes under future climate change.
● Spring phenology at high latitudes exhibits varying spatial trends.
● Lower winter minimum temperatures enhance the efficiency of vegetation leaf-out in response to spring warming.
● The regulation effect of winter snow differs between grasslands and needleleaf forests.
Vegetation phenology is a natural phenomenon that occurs in an annual cycle where plants are affected by seasonal climate patterns (White et al., 2009). Changes in phenology are among the most direct indicators of vegetation evolution, strongly controlled by climate change (Shen et al., 2018; Vogel, 2022). The start of the vegetation growing season (start of season or SOS) is an important phenology indicator for terrestrial ecosystems in response to changing climates (Caparros-Santiago et al., 2021; Piao et al., 2019). Changes in SOS can influence the terrestrial carbon and water cycles, with a potential impact on the productivity and stabilization of terrestrial ecosystems (Ji et al., 2021; Xu et al., 2020).
Numerous studies have confirmed that global warming has significantly altered vegetation phenology, with SOS gradually advancing in recent years (Gao and Zhao, 2022; Liu et al., 2022). However, although spring leaf phenology as a whole has shown a clear trend of advancement with warming, there are notable differences among species and across regions. The most pronounced changes in vegetation phenology are mainly at high latitudes, and the trend is greater for woody plants than for herbaceous plants (Cheng et al., 2021; Chu et al., 2021; Zhao et al., 2015). In general, spring leaf emergence is co-regulated by a series of factors that vary from environment to environment (Chuine et al., 2016).
In temperate zones, precipitation and, especially, temperature are considered to be the most important environmental factors controlling the phenology of plants (Du et al., 2019a; Zhang et al., 2022a). Warmer temperatures in early spring have been shown to promote earlier vegetation germination (Garzón et al., 2024; Montgomery et al., 2020; Park et al., 2020; Piao et al., 2015), with the highest correlations between spring temperatures and SOS found in the Northern Hemisphere (Chen et al., 2023a; Li et al., 2021). Studies modeling phenological changes based on average daily temperatures, though, ignore the potentially different contributions of daytime and nighttime warming to plant development (Peng et al., 2013; Zhong et al., 2023). Diurnal warming patterns may play different or even opposing roles in spring phenological changes (Meng et al., 2020).
Precipitation also has a very important effect on SOS. Precipitation plays a crucial role in regulating vegetation growth and community structure in many regions (Chen et al., 2023b, 2024; Shen et al., 2015a), especially arid and semi-arid regions (Hu et al., 2022; Korell et al., 2021; Small et al., 2018). Moreover, Shen et al. found that even in swamp ecosystems with favorable moisture conditions, vegetation growth can be limited by changes in precipitation (Shen et al., 2023).
In general, there is still no consensus regarding the linearity of the SOS response to climate change, especially given the fact that shifts in SOS vary spatially and temporally. The sensitivity of phenology to temperature appears to have weakened in recent decades, especially in Europe (Peng et al., 2018; Wang et al., 2019). The mediating effect of winter temperatures on SOS has been proposed as a possible factor (Chamberlain and Wolkovich, 2021; Fu et al., 2015a), but how it does so remains controversial. The existence of complex abiotic–biotic relationships suggests that the ecological memory of winter climate may alter the response of SOS to spring warming (Ogle et al., 2015). Some studies have posited that the accumulated amount of winter cold temperatures affects the amount of forcing needed to trigger germination bursts and thus affects SOS (Ettinger et al., 2020). Germination of plant leaves in temperate and boreal regions usually requires a certain cold temperature accumulation to break bud endodormancy, followed by the arrival of warmer conditions (known as forcing temperatures) to trigger cell growth and leaf development (Man et al., 2017). However, other researchers have proposed that it is the number of cold winter days, rather than the accumulated cold temperatures, that is the main factor affecting SOS (Beil et al., 2021). Winter precipitation also affects spring phenology at mid- to high latitudes in the Northern Hemisphere in a complex way (Jin et al., 2019; Yun et al., 2018). The SOS may be advanced or delayed by a few days or even weeks depending on changes in precipitation during the pre-season period (Li et al., 2023; Wang et al., 2022a).
Characterizing how winter climate mediates the response of vegetation SOS to warming spring temperatures is critical to bridging this conceptual disconnect and better understanding how SOS is likely to change as the climate warms. However, studies addressing this issue to date have focused on average winter temperatures and have been mainly conducted on single species through controlled experiments and in situ observational studies rather than (Man et al., 2017; Zhang et al., 2022b, 2018) comparing different vegetation types at large spatial scales. In addition, little consideration has been given to potential differences in the effects of daytime and nighttime temperatures or to the possible effects of changes in winter precipitation. These gaps limit our ability to predict regional and global changes in SOS in a warming climate and the resulting changes in ecosystem structure and function.
The methods of phenological observation mainly include ground observation and remote sensing (Berra and Gaulton, 2021; Gao and Zhang, 2021). Ground observations are traditionally carried out by directly monitoring plants and climate variables, which may limit the study to a small area and a few species. Remote sensing monitoring compensates for the shortcomings of ground-based observation, providing a data source for community-scale and regional-scale vegetation phenology studies and becoming a widely used tool for phenology research (Smith and Ramsay, 2020; Wang et al., 2022b), although it too is limited to the temporal, spatial, and spectral resolution of the remote sensor data (Gong et al., 2024; Piao et al., 2019).
In this paper, we used GIMMS 3g normalized difference vegetation index (NDVI) and meteorological data from 1982 to 2015 to investigate the spatial and temporal characteristics of SOS of different vegetation types and their responses to winter and spring climate change. Our study focuses on Northeast China, which is located in the mid- to high latitudes of the Northern Hemisphere and is highly sensitive to climate change, mainly focusing on the moderating role of winter climatic conditions on the efficiency of vegetation SOS in response to spring warming. We make the following assumptions: 1) winter precipitation’s role has a certain connection with vegetation type, and 2) there is a diurnal difference in winter temperatures’ regulation effect. Our aim was to enhance the ability to understand and predict changes in vegetation phenology under warming conditions. Our results may provide valuable insights for predicting future changes in SOS, as well as in vegetation productivity and adaptability, on which to base forest management decisions to cope with global warming.
The study area belongs to the middle and high latitudes of the Northern Hemisphere and is located in Northeast China (Figure 1) (38°72′–53°55′N, 115°52′–135°09′E), which is known for high sensitivity to global climate change. It administratively comprises the provinces of Heilongjiang, Jilin, and Liaoning, as well as three cities and one league (Tongliao, Chifeng, Xing’anmeng, and Hulunbei’er) of eastern Inner Mongolia (Zhang et al., 2023). The study area encompasses a total of approximately 1.27 million km2. This region, most of which belongs to the temperate continental monsoon climate zone, is one of the largest forest carbon pools in China (Qiu et al., 2020; Sun and Liu, 2019).
The study area encompasses a wide range of spatial variability in climate conditions, characterized by a longitudinal gradient in precipitation (higher in the east and lower in the west) and a latitudinal gradient in temperature (higher in the south and lower in the north), with an east–west difference of 833 mm in annual average precipitation (Figure 2B) and a north–south difference of 16.83°C in annual average temperature (Figure 2C). The region’s diverse vegetation types (Figure 2A) (Luo et al., 2020) allow for a comparative study of the effects of a warming climate on vegetation phenology.
Figure 2. (A) Distribution of the three vegetation types. (B) Spatial distribution of average annual precipitation in Northeast China from 1982 to 2015. (C) Spatial distribution of average temperature in Northeast China from 1982 to 2015.
Satellite-derived NDVI data covering the 1982–2015 period were obtained from the GIMMS NDVI 3g dataset, which has a spatial resolution of 8 km × 8 km and a temporal resolution of 15d. This global vegetation index change dataset was produced by the U.S. National Aeronautics and Space Administration (NASA) (http://ecocast.arc.nasa.gov/data/pub/gimms/3g.v1) and is appropriate for determining long-term trends in global vegetation activity.
Land use data were accessed from the European Space Agency (ESA) Climate Change Initiative (CCI) annual land cover dataset (https://maps.elie.ucl.ac.be/CCI/viewer/download.php), which has a spatial resolution of 300 m and an estimated classification accuracy of more than 70%. Areas with no change in vegetation cover from 1982 to 2015 were selected to categorize the land cover types in the study area, including deciduous needleleaf forests (DNF), deciduous broadleaf forest (DBF), and grassland (Figure 2A).
We accessed meteorological data from the CRUTS v4.07 dataset produced by the UK National Centre for Atmospheric Science (NCAS) (https://crudata.uea.ac.uk/cru/data/hrg), which has a spatial resolution of 0.5° and spans the time period 1901–2022. We extracted the 1982–2015 period monthly maximum temperature (Tmax), minimum temperature (Tmin), and precipitation values and calculated the seasonal averages for each year, i.e., winter (December from the previous year to February of the current year) and spring (“March to May” of the current year). We resampled the land use data and meteorological data to be consistent with the 8-km spatial resolution NDVI data (Deng et al., 2019; Zhang et al., 2022c).
In this study, the Polyfit-Maximum method was used to determine SOS. This method is based on the principle that the first period of significant increase in the vegetation index (NDVI) each year marks the SOS (Piao et al., 2006). Compared to other methods for extracting candidate SOS dates, this method reduces the effects of aerosols, clouds, and other factors (Jeong et al., 2011). Numerous studies have been conducted using this method to calculate the SOS (Ma et al., 2022; Shen et al., 2019; Yuan et al., 2020). Following this method, the multi-year average of NDVI was first calculated to derive the NDVI time series with an interval of 15 days. Then, the temporal rate of change of NDVI was calculated according to Equation 1:
where the NDVIratio(t) is the rate of change of NDVI at time t, t is a certain moment (calculated from January 1), and (t + 1) indicates the next moment, with an interval of 15 days.
NDVI as computed from satellite remote sensing imagery often has some outliers due to non-vegetation effects such as clouds, atmospheric interference, and the solar radiation angle. In order to minimize the influence of the outliers on the phenological assessments, the polynomial maximum method was used, and the sixth-degree polynomial was found to better fit the NDVI time series (Equation 2):
where NDVI(t) is the threshold value corresponding to day t calculated in Equation 1 and a1, a2, a3, …, a6 are the fitting parameters, which are calculated by least squares regression.
We used the one-dimensional linear regression method (Shen et al., 2019) to analyze the trend of SOS and climate indicators at the regional scale and the image element scale. The trend was calculated as shown in Equation 3:
where Slope indicates the slope of the regression equation for variable P, Pi is the value of the variable in year i, and n is the number of years in the study period. When the slope is positive, it indicates that a delay in SOS has occurred; conversely, a negative slope signals that SOS is advancing (occurring earlier in the year).
where Rsos indicates the average SOS trend for each of the four special study regions, Tsos indicates the average SOS trend for the vegetation type corresponding to each region, and Esos indicates the rate of increase or decrease in Rsos compared to Tsos.
To exclude the interference of other variables and explore the relationships between individual climate indicators and SOS separately, we used a partial correlation analysis to analyze the relationship between seasonal climate indicators and SOS for each period. The formula is shown in Equation 5 (Su et al., 2022):
where rxy.z is the partial correlation coefficient between x and y after the variable z is kept fixed, rxy is the relationship between x and y, ryz is the correlation coefficient between y and z, and rxz is the difference between the x and z.
The significance of the partial correlation coefficients in this paper was examined using t-tests. To further analyze the effect of climate change on SOS, this paper also compared the trends of SOS and the partial correlation coefficients of SOS with Tmax and Tmin under different temperature and precipitation trends in winter (Shen et al., 2023).
The spatial distribution of the vegetation SOS in Northeast China from 1982 to 2015 is shown in Figure 3A. The average SOS ranged from 107.5 to 128.2 the day of the year (DOY), from mid-April to early May, with an average SOS of 115.7 DOY for the entire study area. SOS dates differ among the different vegetation types and different distribution areas of the same vegetation type. The average SOS of grassland was the earliest (113.8 DOY), followed by DNF (115.6 DOY) and then DBF (117.7 DOY). By location, DBF SOS appeared earlier in the southern and lower elevation portions of the study area and later in the more northern, higher elevation areas of the Changbai Mountains, Lesser Khingan Mountains, and eastern Greater Khingan Mountains.
Figure 3. (A) Spatial distribution of multi-year average of the start of the vegetation growing season (SOS) by day of the year (DOY) in Northeast China, 1982–2015. (B) Box plot of multi-year average SOS values for grasslands, deciduous needleleaf forests (DNF), and deciduous broadleaf forests (DBF). Lighter to darker colors correspond to panel A representing earlier to later SOS, on average. The box represents the middle 50% of the SOS data, the line inside the box represents the median, the vertical lines extend to the minimum and maximum values of the dataset, and the data points that lie outside the maximum and minimum values are the extreme values. (C) Spatial trends in SOS from 1982 to 2015. Pixel points with dots indicate significant trends (p < 0.05). Red boxes mark the subregions focused on in this study. (D) Trends in regional average SOS (days per decade) from 1982 to 2015.
From 1982 to 2015, on average, DNF advanced by 1.2 days per decade, DBF by 0.1, and grassland by 0.6 (Figure 3D). However, the trend of SOS showed obvious spatial differences, with some pixels showing significant changes. Spatially, the pixels showing an advancing trend in SOS of DNF accounted for 81.9% (12.2%, p < 0.05), mainly focused on the northern region of the Greater Khingan Mountains (Figure 3C). The advancing trend in SOS was especially pronounced along the northern boundary of the study area (Figure 3C, Region 1), with a significant trend averaging 1.9 days per decade. This represents a 58% faster rate of advancement compared with the regional average trend for DNF SOS.
The proportion of grassland pixels showing an advancing trend in SOS was 62.9% (31.4%, p < 0.05), with the area with significant advancement trends concentrated in the northwestern and central portions of the study area (Figure 3C, Region 2). On average, Region 2 grassland SOS advanced by 1.6 days/decade, which is 167% faster than the regional average trend for grasslands (Figure 3D). However, the southwestern part of the study area, which is mainly grassland, saw a significant delaying trend of 2.6 days/decade (Figure 3C, Region 3). The majority of the DBF pixels located in the eastern part of the study area saw a delay in SOS (60.3%, including 6.1%, p < 0.05) (Figure 3C, Region 4), with an average delay of 1.2 days/decade, while the proportion of pixels showing an advancing trend was only 38.8% (7.6%, p < 0.05), mainly focused on the southern part of the Changbai Mountains.
In order to explain the spatial variability of SOS trends in the study area across different regions and vegetation types, we analyzed the changes in winter and spring precipitation and temperature during the period of 1982–2015 (Figure 4). We found that winter precipitation (Figure 4A) and spring Tmin (Figure 4D) generally showed an increasing trend across the study area. In contrast, changes in spring precipitation (Figure 4B), spring Tmax (Figure 4F), and winter temperature (Tmax and Tmin) (Figures 4C, E) exhibited significant spatial variations. In particular, winter temperature (Tmin and Tmax) showed a decreasing trend north of the Greater Khingan Mountains and in the northwestern and central parts of the study area, especially in Region 1 and Region 2, where the SOS significantly advanced. Spatial variability in winter temperatures may account for some of the spatial differences in vegetation SOS change, but the spatial differences in spring Tmax and precipitation change may also contribute. Therefore, to further determine the reasons for the spatial variation in SOS change, we analyzed the relationship between SOS and seasonal climate change.
Figure 4. Trends of regional climate indicators in Northeast China from 1982 to 2015. (A) Winter precipitation. (B) Spring precipitation. (C) Winter Tmin. (D) Spring Tmin. (E) Winter Tmax. (F) Spring Tmax. Pixel points with dots indicate significant trends (p < 0.05).
We first analyzed the partial correlation between average SOS and climate indicators for different vegetation types (Figure 5) and found that the SOS of forests (especially DBF) was strongly influenced by temperature. Forest SOS showed significant negative correlations with both winter and spring temperatures (Tmin and Tmax) and a weaker negative correlation with precipitation. Grassland SOS was co-regulated by Tmin, Tmax, and precipitation, showing significant negative correlations with winter temperatures (Tmin and Tmax) as well as winter and spring precipitation while showing weaker correlations with spring temperatures.
Figure 5. Partial correlations between regional average start of the vegetation growing season (SOS) and climate indicators.
On a pixel-by-pixel basis (Supplementary Tables S1, S2), DNF SOS and DBF SOS were most affected by spring Tmax, followed by spring Tmin; the proportions of pixels with negative correlations between SOS and spring Tmax were 100% (85.26%, p < 0.05) for DNF and 99.85% (86.9%, p < 0.05) for DBF (Figure 6F); for spring Tmin, they were 99.42% (52.99%, p < 0.05) and 99.13% (65.24%, p < 0.05), respectively (Figure 6D). This is the same as the partial correlation between average SOS and climate factors (Figure 5).
Figure 6. Partial correlation analysis of the start of the vegetation growing season (SOS) with climate indicators from 1982 to 2015. Pixel points with dots indicate significant trends (p < 0.05). (A) Winter precipitation. (B) Spring precipitation. (C) Winter Tmin. (D) Spring Tmin. (E) Winter Tmax. (F) Spring Tmax.
While the effect of precipitation on grassland SOS was similar, that relationship showed obvious spatial differences across seasons: the pixels with significant negative correlations between grassland SOS and winter precipitation (27.68%, p < 0.05) were mainly concentrated in the northwestern and central (Region 2) parts of the study area (Figure 6A), while for spring precipitation (35.84%, p < 0.05), they were mainly concentrated in the southwest (Region 3) (Figure 6B). In terms of the effect of precipitation on forest SOS, unlike the weak correlation with regional average SOS (Figure 5), there were regions of significant negative correlations between winter or spring precipitation and SOS for both DNF (26.74% and 3.6%, p < 0.05) and DBF (18.55% and 39.78%, p < 0.05) (Figures 6A, B).
Although winter temperatures were negatively correlated with the trends of grassland and DNF SOS overall (Figure 5), we found a positive partial correlation in portions of the study area, particularly in the northwestern and central parts (Region 2) and the northern boundary (Region 1) (Figures 6C, E). Winter Tmin, in particular, showed a positive correlation for approximately half of the study area pixels (54.88% for DNF and 47.03% for grassland). This was true for smaller portions of the grassland area: spring Tmin (15.8%, p < 0.05) and Tmax (6.55%, p < 0.05) (Figures 6D, F). The partial correlations between SOS and climate indicators indicate that the climate response of SOS differs among regions of Northeast China, even for the same vegetation type (Figure 6).
Spatial variability in the partial correlations between SOS and these climate indicators may explain the differences in SOS changes (Figure 3C). We found that the regions where winter temperatures showed positive effects on SOS overlapped with the regions where SOS significantly advanced (Regions 1 and 2). In light of winter temperature trends (Figures 4C, E), we noted that the decrease in Tmin and Tmax in winter promotes the advancement of SOS. This phenomenon is more pronounced in Regions 1 and 2, which are located in cold and arid regions (Figure 7). Moreover, by comparing the positive correlations between SOS and winter Tmin or Tmax (Figures 6C, E) in the context of the SOS trends (Figure 3C), we found that winter Tmin is the most important driver of changes in vegetation SOS.
Figure 7. Influence of multi-year average temperature and precipitation conditions on winter Tmin and Tmax trends in the study area: (A) average temperature and (B) average total precipitation. Purple indicates Tmax, and green indicates Tmin. The lines indicate the linear fit for the winter temperature trends, and the shading represents the 95% confidence band.
Subsequently, we examined the effects of winter temperature decreases on the SOS trend (Supplementary Figures S1A, S2A), as well as the partial correlation of SOS with spring temperatures in Region 1 (primarily DNF) and Region 2 (primarily grassland) (Figures 8, 9) so as to clarify the moderating role of winter temperatures on SOS and its response to spring temperatures. The results showed that the advancing trend of SOS (Supplementary Figures S1 and S2) and the negative partial correlation between spring Tmin and SOS increased gradually with the magnitude of the winter Tmin decline (Figures 8A, 9A). However, the effect of winter Tmin on the partial correlation between SOS and spring Tmax differed between Regions 1 and 2. Greater declines in winter Tmin increased the partial correlation between SOS and spring Tmax (Figure 8C) in the grasslands of Region 2 but had no effect on the DNF in Region 1 (Figure 9C). This suggests that a decrease in winter Tmin can increase the rate of the SOS response to spring temperatures, but the regulatory effect differed between the vegetation types. For DNF, this moderating effect was focused on the response of SOS to spring Tmin, whereas for grasslands, the response of SOS to both spring Tmin and Tmax was modulated by winter Tmin.
Figure 8. Moderating role of winter climate indicators in Region 1 [deciduous needleleaf forests (DNF)] on the spring temperature sensitivity of the start of the vegetation growing season (SOS). (A) The effect of winter Tmin trends on the partial correlation between SOS and Tmin. (B) The effect of winter precipitation trends on the partial correlation between SOS and Tmin. (C) The effect of winter Tmin trends on the partial correlation between SOS and Tmax. (D) The effect of winter precipitation trends on the partial correlation between SOS and Tmax. The lines indicate the linear fit for the partial correlation coefficient, and the shading represents the 95% confidence band.
Figure 9. Moderating role of winter climate indicators in Region 2 (grassland) on the spring temperature sensitivity of the start of the vegetation growing season (SOS). (A) The effect of winter Tmin trends on the partial correlation between SOS and Tmin. (B) The effect of winter precipitation trends on the partial correlation between SOS and Tmin. (C) The effect of winter Tmin trends on the partial correlation between SOS and Tmax. (D) The effect of winter precipitation trends on the partial correlation between SOS and Tmax. The lines indicate the linear fit for the partial correlation coefficient, and the shading represents the 95% confidence band.
Because SOS in both Region 1 DNF and Region 2 grassland are also affected by winter precipitation (Figure 6), we also analyzed the effect of increases in winter precipitation on the trend of SOS (Supplementary Figures S1B, S2B) and the partial correlation of SOS with spring temperatures (Figures 8B, D, 9B, D). We found that changes in winter precipitation trends had completely opposite effects on SOS in Region 1 and Region 2. For DNF in Region 1, we found that increased winter precipitation is associated with slower advancement of SOS (Supplementary Figure S1B) and a reduction in the partial correlation between SOS and spring Tmin (Figure 8B), but not spring Tmax (Figure 8D). By contrast, for grasslands, winter precipitation has the same effect as winter Tmin: both are associated with a faster-advancing trend in SOS and greater partial correlations of SOS with spring Tmin and Tmax. Thus, the changes in thermal and moisture conditions in the wintertime, not only spring warming, are of primary importance for vegetation SOS. The effects of winter and spring conditions on SOS are likely to be different, with winter climate change influencing the speed of the effect of spring warming.
In Region 3 (primarily grasslands) and Region 4 (primarily DBF), the effect of changes in winter Tmin and precipitation was weaker than that in Regions 1 and 2. For Region 3, neither changes in winter Tmin nor precipitation had an obvious effect on grassland SOS (Supplementary Figure S3). For Region 4, the negative correlation of SOS with spring Tmin and Tmax increases slightly with an increase in the magnitude of winter Tmin and precipitation trend (Supplementary Figures S4C, E, F). This contrasts with our finding in Region 1 that DNF SOS sensitivity is regulated by winter Tmin (Supplementary Figure S1C).
From 1982 to 2015, the overall advancement in average SOS in the study area was consistent with the trend of early greening of the vegetation in the Northern Hemisphere at middle and high latitudes (Jeong et al., 2011; Jiang et al., 2023; Schwartz et al., 2006). The average SOS ranged from 107.5 to 128.2 DOY with an average SOS of 115.7 DOY for the entire study area. This is similar to previous studies showing SOS dates of 100–130 DOY in Northeast China (Qiao et al., 2019; Zhang et al., 2022c). The average SOS of grassland was the earliest (113.8 DOY), followed by that of DNF (115.6 DOY) and then DBF (117.7 DOY), which can be attributed to the fact that the woody plants are less sensitive to the climate than herbaceous plants (Post and Stenseth, 1999). For woody plants, the different water transport structures of conifers and broadleaf trees affect the water supply in the face of low late-winter temperatures, while conifers are virtually unaffected, which may contribute to the fact that the DNF SOS precedes the DBF SOS (Cochard and Tyree, 1990; Wang et al., 1992).
Since the SOS in the study area was focused on mid-April to early May, changes in winter and spring climates were taken into account in analyzing the effect of climate on SOS. There were differences in the response of regional average SOS to climatic change among the different vegetation types, with forest SOS being mainly influenced by spring Tmin and Tmax and weakly influenced by precipitation, whereas grassland SOS is more affected by winter and spring precipitation. Our results are consistent with the results of previous studies (Hernández Ayala et al., 2021; Wang et al., 2022c; Zhao et al., 2024). Previous studies have confirmed the important role of precipitation and temperature on vegetation growth (Chen et al., 2018; Jiao et al., 2021; Zhang et al., 2022a).
However, unlike the opposite response of vegetation growth to diurnal warming reported by Meng et al. (2020), we found that forest SOS was significantly and negatively correlated with both spring Tmin and Tmax, although the correlation is higher with spring Tmax. This is because the growth of vegetation is controlled by the balance of photosynthesis (in the daytime) and respiration (at night) (Peng et al., 2013; Sun et al., 2023). SOS, though, is not simply a function of ordinary vegetation growth: our study focuses on the process of new leaves appearing in spring. The process of growing new leaves relies upon the preceding year’s stored organic matter, and when plants break out of winter dormancy to grow new leaves, they depend mainly on spring temperature and moisture conditions (Jiao et al., 2021; Zhang et al., 2022a). The increase in spring Tmin and Tmax may accelerate heat accumulation that favors early SOS (Zhang et al., 2023).
In response to winter Tmin and Tmax, the regional average SOS of all three vegetation types showed consistent and significant negative correlations, with the most significant correlation in DBF. This can be explained by spatial variation in the role of winter temperatures, with SOS responding positively to winter Tmin in some areas of DNF and grassland, weakening the overall negative correlation between regional average SOS and winter Tmin. Our results show that an increase in spring Tmax and spring Tmin can meet the heat accumulation threshold required for vegetation growth and significantly promote the advancement of SOS in forests, similar to previous findings on temperate woody plants (Fu et al., 2015b; Zhang et al., 2023). Grasslands, in contrast, are more sensitive to precipitation because they are located in drier regions. In addition, since herbaceous plants have shallower root systems and are more susceptible to soil moisture fluctuations than forests (Chen et al., 2015), changes in spring and winter precipitation significantly affect the SOS of grassland.
This study examined in detail the characteristics of SOS changes in four regions of Northeast China, finding significant trends in Regions 1–3. When we attributed the SOS changes to climate indicators, we found that this study area has experienced a trend of lower winter temperatures (Tmin and Tmax)—unlike the general trend of global warming (Li et al., 2015a; Luo et al., 2024; Richardson, 2022; Scafetta, 2024) and winter temperatures in most of the world (McCabe and Wolock, 2010). This is mainly due to the fact that our study period of 1982–2015 includes the so-called “warming hiatus” of 1998–2012, during which winter cooling was experienced in some terrestrial biomes, temporarily obscuring the continued rise in global temperatures (Li et al., 2015b, 2015; Trenberth et al., 2014). Other studies have also noted the occurrence of decreasing winter Tmin and Tmax in high-latitude boreal forests around this time (Cohen et al., 2012; Deng et al., 2019).
The study area overall showed increases in spring Tmin and Tmax but a decrease in winter Tmin, resulting in a significant advancing trend of DNF SOS. In most of Region 1, the northernmost and coldest region, dominated by DNF, the SOS showed a significant advancing trend that overlapped with the area where winter Tmin was positively correlated with SOS. The partial correlation of DNF SOS with winter Tmin here was positive, and the partial correlations with spring Tmin and winter precipitation were significantly negative.
As previously noted, SOS in most cold-temperate plants is the result of the interaction between winter cold and spring heat (Linkosalo et al., 2008; Luedeling et al., 2009; Park et al., 2018). Temperate vegetation overwinters in a dormant state to protect sensitive growing tissues from frost damage; coming out of dormancy and resuming growth in the spring involve meeting a required threshold of winter cold and then accumulating spring heat (Arlo Richardson et al., 1974; Luedeling et al., 2009). Previous studies on the effects of winter and spring temperature changes on SOS have focused on the effects of average temperatures on vegetation (Yu et al., 2010; Zhang et al., 2022b), which may have led to an underestimation of the effects of cold winter nighttime temperatures on SOS.
Our study found that the role of winter Tmin was stronger than that of winter Tmax in the regulation of the spring temperature response of grassland and DNF SOS in cold, arid regions. This is due to seasonal differences in the effects of diurnal temperature on vegetation activity, with SOS mainly affected by Tmax in spring and by Tmin in winter (Deng et al., 2019). Nighttime cooling in winter increases the efficiency of spring Tmin warming on leaf emergence due to a higher accumulation of chilling units; this induces a positive correlation between winter Tmin and forest SOS (Liu et al., 2024; Meng et al., 2020; Zhang et al., 2022c). While the significant effect of spring Tmax on SOS makes it unregulated by winter Tmin, this supports our conclusion: it indicates that the effects of winter temperatures on the spring temperature sensitivity of SOS are mainly driven by changes in winter Tmin and primarily regulate the effect of spring Tmin on DNF SOS.
In this study, precipitation showed a countervailing effect on DNF SOS: the increasing trend of winter precipitation weakened the advancing trend of SOS in DNF. This is similar to the inhibitory effect of precipitation on the growth of temperate DNF (Fang et al., 2005). Lower winter Tmin would seem to have met the cold demand threshold for vegetation to break out of dormancy with the arrival of spring warming. However, higher soil temperatures due to increased winter snowpack (Maurer and Bowling, 2014; Sanmiguel-Vallelado et al., 2021; Wilson et al., 2020) instead delayed the fulfillment of the cold demand of vegetation, leading to a weaker trend in the advancement of SOS. Thus, an acceleration in the decreasing trend of winter Tmin favors an increase in the partial correlation between spring Tmin and DNF SOS, whereas an uptick in the increasing trend of winter precipitation has an inhibitory effect on the increase in the partial correlation between spring Tmin and DNF SOS.
In the cold, arid grasslands of Region 2, located in the northwestern and central portions of the study area, the response of grassland SOS to winter Tmin and spring Tmin was the same as that of DNF SOS in Region 1. An increase in the downward trend in winter Tmin in this region had a similar effect, increasing the negative partial correlation of SOS with spring Tmin. In addition, an increase in the magnitude of the decreasing trend of winter Tmin can also increase the response of SOS to warming spring Tmax. This is because the mechanism by which winter temperature modulates the efficiency of the spring temperature response of grassland SOS is different from DNF SOS. The combination of decreased winter Tmin and increased winter precipitation favors snow accumulation (Barrere et al., 2018; Zhang et al., 2019). It can provide water available for the following year’s plant growth as spring temperatures rise, reducing the demand for plants for pre-season precipitation (Cong et al., 2021). Water availability is important for herbaceous plant growth in arid and semi-arid regions, where water availability is often insufficient due to high soil salinity and low water content (Wang et al., 2022d) and where vegetation tends to become more sensitive to moisture conditions (Shen et al., 2015a, 2015, 2018). In addition, the presence of snow can serve to regulate the soil temperature at a considerably higher level than the air temperature (Freppaz et al., 2008; Groffman et al., 2001): the higher soil temperature as well as the sufficient water availability results in the advancement of the grassland SOS. As a result, the trend of advancement of grassland SOS gradually increased with the increasing trend of rising precipitation in winter while contributing to the increasing negative partial correlation between Tmin and Tmax and SOS in spring.
In contrast, in the warm, arid southwestern portion of Region 3 (mainly grasslands), the warm climatic conditions and the weak decreases in Tmin during the winter do not serve to conserve the snowpack, and this, combined with the region’s reduced spring precipitation and poor moisture levels, and the lack of available water resulted in a delay in the emergence of grassland SOS in the region (Ren et al., 2017). In Region 4, encompassing the warm and humid Lesser Khingan Mountains and eastern Changbai Mountains, the decline in spring Tmax made it difficult to meet the heat demand required for plant leaf unfolding, resulting in a delayed trend in DBF SOS; the severe heat deficit facilitated the spring temperature response of SOS when the decreasing trend in winter Tmin in the region was reduced (Du et al., 2019b; Liu et al., 2024). In contrast, the response of SOS to spring temperatures in this region was promoted when the increase in soil temperatures due to winter snowpack led to an increase in the rising trend of winter precipitation. In addition, the southern part of Changbai Mountain is close to the ocean and has a warm and humid climate, and the increase in winter snow melts quickly in late winter, coupled with the increase in spring temperatures, which are good thermal and hydrological conditions that lead to the advancement of SOS in the region. Overall, the modulation of the efficiency of the spring temperature response of SOS by winter climate change and its modalities differed significantly between regions and vegetation types.
This study only considers the effects of temperature and precipitation as drivers of changes in vegetation phenology. Of course, geographic factors such as elevation can affect vegetation phenology (Shahzad et al., 2024). However, each of the main vegetation distribution areas in the study area falls within a limited elevation range, and any effects of elevation change are largely captured by changes in temperature (Ohmura, 2012). We also noted that socio-economic conditions and policy regulation should not be ignored (Marambe and Wijesundara, 2021), but given the relatively coarse (8 km × 8 km) pixel size used in our analysis, we cannot detect local variations that may be attributable to human activity (Gong et al., 2024; Piao et al., 2019). In future research, attempts should be made to combine remote sensing data with ground observations (Piao et al., 2020) and to integrate imagery from different types of sensors to explore the effects of geographic factors, as well as more climatic factors, on vegetation SOS.
This study demonstrates the relationships between climate indicators and changes in the start of the vegetation growing season in the high latitudes of Northeast China over recent decades.
First, we found that the SOS in high latitudes in China showed an overall trend of advancement, but the trend of SOS varied significantly in space and by vegetation types. The SOS in the cold north and the cold, arid northwestern–central parts of the study area advanced significantly with trends of 1.9 days/decade and 1.6 days/decade, respectively. These trends represent an increase in SOS advancement efficiency of 58% and 167% compared to the regional average SOS for DNF and grasslands, respectively. The SOS in the warm, arid southwest and warm, humid Lesser Khingan Mountains and Changbai Mountain regions in the eastern part of the study area were delayed with trends of 2.6 days/decade and 1.2 days/decade, respectively.
Second, warming had opposing effects on SOS depending on when it occurred. Decreasing spring temperatures constrain the initiation of vegetation SOS, contributing to the delay in DBF SOS in the Changbai Mountains. In contrast, decreases in winter temperature, mainly nighttime temperature, enhance the process of recovering from the dormancy phase, contributing to the higher efficiency of SOS response to spring temperatures and the asymmetric modulation of SOS spring temperature response efficiency by winter Tmin and Tmax.
Third, the moderating effect of winter precipitation on the efficiency of the SOS spring temperature response differs with vegetation type. An increase in winter precipitation delayed the satisfaction of DNF cold demand in the cold northern region, which was detrimental to the breaking of vegetation dormancy, thus inhibiting the efficiency of the spring temperature response of the DNF SOS. For the cold, arid northwestern and central regions, an increase in snow cover improves the moisture conditions required for vegetation emergence and enhances the efficiency of the grassland SOS response to spring temperatures.
In summary, this paper demonstrates the moderating role of changes in winter climate in affecting the SOS responsiveness to spring temperature, and it emphasizes that the moderating role of winter climate should be considered when exploring vegetation phenology–spring climate connections. This knowledge can help to more precisely and reasonably predict temperature-driven phenological changes under future climate change.
The original contributions presented in the study are included in the article/Supplementary Material. Further inquiries can be directed to the corresponding author.
MC: Writing – review & editing, Writing – original draft, Visualization, Software, Project administration, Methodology, Formal analysis, Data curation, Conceptualization. MH: Writing – review & editing, Visualization. BL: Writing – review & editing, Project administration, Funding acquisition. WZ: Writing – review & editing, Software, Data curation. RM: Writing – review & editing. WH: Writing – review & editing, Visualization, Software. ZD: Writing – review & editing, Software, Data curation.
The author(s) declare financial support was received for the research, authorship, and/or publication of this article. This work was funded by the National Natural Science Foundation of China (Grant No. 41877416).
The authors thank Yuanhang Su and Yu Zhang for their assistance with the methods.
The authors declare that the research was conducted in the absence of any commercial or financial relationships that could be construed as a potential conflict of interest.
All claims expressed in this article are solely those of the authors and do not necessarily represent those of their affiliated organizations, or those of the publisher, the editors and the reviewers. Any product that may be evaluated in this article, or claim that may be made by its manufacturer, is not guaranteed or endorsed by the publisher.
The Supplementary Material for this article can be found online at: https://www.frontiersin.org/articles/10.3389/fpls.2024.1476576/full#supplementary-material
Arlo Richardson, E., Seeley, S. D., Walker, D. R. (1974). A model for estimating the completion of rest for ‘Redhaven’ and ‘Elberta’ peach trees1. HortScience 9, 331–332. doi: 10.21273/HORTSCI.9.4.331
Barrere, M., Domine, F., Belke-Brea, M., Sarrazin, D. (2018). Snowmelt events in autumn can reduce or cancel the soil warming effect of snow–vegetation interactions in the arctic. J. Climate 31, 9507–9518. doi: 10.1175/JCLI-D-18-0135.1
Beil, I., Kreyling, J., Meyer, C., Lemcke, N., Malyshev, A. V. (2021). Late to bed, late to rise—Warmer autumn temperatures delay spring phenology by delaying dormancy. Global Change Biol. 27, 5806–5817. doi: 10.1111/gcb.15858
Berra, E. F., Gaulton, R. (2021). Remote sensing of temperate and boreal forest phenology: A review of progress, challenges and opportunities in the intercomparison of in-situ and satellite phenological metrics. For. Ecol. Manage. 480, 118663. doi: 10.1016/j.foreco.2020.118663
Caparros-Santiago, J. A., Rodriguez-Galiano, V., Dash, J. (2021). Land surface phenology as indicator of global terrestrial ecosystem dynamics: A systematic review. ISPRS J. Photogramm. Remote Sens. 171, 330–347. doi: 10.1016/j.isprsjprs.2020.11.019
Chamberlain, C. J., Wolkovich, E. M. (2021). Late spring freezes coupled with warming winters alter temperate tree phenology and growth. New Phytol. 231, 987–995. doi: 10.1111/nph.17416
Chen, X., An, S., Inouye, D. W., Schwartz, M. D. (2015). Temperature and snowfall trigger alpine vegetation green-up on the world’s roof. Global Change Biol. 21, 3635–3646. doi: 10.1111/gcb.12954
Chen, C., He, B., Guo, L., Zhang, Y., Xie, X., Chen, Z. (2018). Identifying critical climate periods for vegetation growth in the northern hemisphere. J. Geophys. Res.: Biogeosci. 123, 2541–2552. doi: 10.1029/2018JG004443
Chen, F., Man, W., Wang, S., Esper, J., Meko, D., Büntgen, U., et al. (2023b). Southeast Asian ecological dependency on Tibetan Plateau streamflow over the last millennium. Nat. Geosci. 16, 1151–1158. doi: 10.1038/s41561-023-01320-1
Chen, F., Wang, T., Zhao, X., Esper, J., Ljungqvist, F. C., Büntgen, U., et al. (2024). Coupled Pacific Rim megadroughts contributed to the fall of the Ming Dynasty’s capital in 1644 CE. Sci. Bull. 69, 3106–3114. doi: 10.1016/j.scib.2024.04.029
Chen, H., Zhao, J., Zhang, H., Zhang, Z., Guo, X., Wang, M. (2023a). Detection and attribution of the start of the growing season changes in the Northern Hemisphere. Sci. Total Environ. 903, 166607. doi: 10.1016/j.scitotenv.2023.166607
Cheng, W. Y., Li, Z., Yan, L. M. (2021). Uniforming spring phenology under non-uniform climate warming across latitude in China. Sci. Total Environ. 762, Artikkel 143177. doi: 10.1016/j.scitotenv.2020.143177
Chu, X., Man, R., Zhang, H., Yuan, W., Tao, J., Dang, Q. L. (2021). Does climate warming favour early season species? Front. Plant Sci. 12, 765351. doi: 10.3389/fpls.2021.765351
Chuine, I., Bonhomme, M., Legave, J.-M., García de Cortázar-Atauri, I., Charrier, G., Lacointe, A., et al. (2016). Can phenological models predict tree phenology accurately in the future? The unrevealed hurdle of endodormancy break. Global Change Biol. 22, 3444–3460. doi: 10.1111/gcb.13383
Cochard, H., Tyree, M. T. (1990). Xylem dysfunction in Quercus: vessel sizes, tyloses, cavitation and seasonal changes in embolism. Tree Physiol. 6, 393–407. doi: 10.1093/treephys/6.4.393
Cohen, J. L., Furtado, J. C., Barlow, M. A., Alexeev, V. A., Cherry, J. E. (2012). Arctic warming, increasing snow cover and widespread boreal winter cooling. Environ. Res. Lett. 7, 14007. doi: 10.1088/1748-9326/7/1/014007
Cong, N., Huang, K., Zhang, Y. (2021). Unsynchronized driving mechanisms of spring and autumn phenology over northern hemisphere grasslands. Front. For. Glob. Change 3, 610162. doi: 10.3389/ffgc.2020.610162
Deng, G., Zhang, H., Guo, X., Shan, Y., Ying, H., Rihan, W., et al. (2019). Asymmetric effects of daytime and nighttime warming on boreal forest spring phenology. Remote Sens. 11, 1651. doi: 10.3390/rs11141651
Du, J., He, Z., Piatek, K. B., Chen, L., Lin, P., Zhu, X. (2019b). Interacting effects of temperature and precipitation on climatic sensitivity of spring vegetation green-up in arid mountains of China. Agric. For. Meteorol. 269-270, 71–77. doi: 10.1016/j.agrformet.2019.02.008
Du, J., Li, K., He, Z., Chen, L., Lin, P., Zhu, X. (2019a). Daily minimum temperature and precipitation control on spring phenology in arid-mountain ecosystems in China. Int. J. Climatol. 40, 2568–2579. doi: 10.1002/joc.6351
Ettinger, A. K., Chamberlain, C. J., Morales-Castilla, I., Buonaiuto, D. M., Flynn, D. F. B., Savas, T., et al. (2020). Winter temperatures predominate in spring phenological responses to warming. Nat. Climate Change 10, 1137–1142. doi: 10.1038/s41558-020-00917-3
Fang, J., Piao, S., Zhou, L., He, J., Wei, F., Myneni, R. B., et al. (2005). Precipitation patterns alter growth of temperate vegetation. Geophys. Res. Lett. 32, L21411. doi: 10.1029/2005GL024231
Freppaz, M., Celi, L., Marchelli, M., Zanini, E. (2008). Snow removal and its influence on temperature and N dynamics in alpine soils (Vallée d’Aoste, northwest Italy). J. Plant Nutr. Soil Sci. 171, 672–680. doi: 10.1002/jpln.200700278
Fu, Y. H., Piao, S., Vitasse, Y., Zhao, H., De Boeck, H. J., Liu, Q., et al. (2015b). Increased heat requirement for leaf flushing in temperate woody species over 1980–2012: effects of chilling, precipitation and insolation. Global Change Biol. 21, 2687–2697. doi: 10.1111/gcb.12863
Fu, Y. H., Zhao, H., Piao, S., Peaucelle, M., Peng, S., Zhou, G., et al. (2015a). Declining global warming effects on the phenology of spring leaf unfolding. Nature 526, 104–107. doi: 10.1038/nature15402
Gao, F., Zhang, X. (2021). Mapping crop phenology in near real-time using satellite remote sensing: challenges and opportunities. J. Remote Sens. 2021, 1–14. doi: 10.34133/2021/8379391
Gao, X., Zhao, D. (2022). Impacts of climate change on vegetation phenology over the Great Lakes Region of Central Asia from 1982 to 2014. Sci. Total Environ. 845, 157227. doi: 10.1016/j.scitotenv.2022.157227
Garzón, M. B., Baillou, F., Silva, F. C. E., Faria, C., Marchi, M., Vendramin, G. G., et al. (2024). Warmer springs favour early germination of range-wide Quercus suber L. populations. Eur. J. For. Res. 143, 157–168. doi: 10.1007/s10342-023-01614-4
Gong, Z., Ge, W., Guo, J., Liu, J. (2024). Satellite remote sensing of vegetation phenology: Progress, challenges, and opportunities. ISPRS J. Photogramm. Remote Sens. 217, 149–164. doi: 10.1016/j.isprsjprs.2024.08.011
Groffman, P. M., Driscoll, C. T., Fahey, T. J., Hardy, J. P., Fitzhugh, R. D., Tierney, G. L. (2001). Colder soils in a warmer world: A snow manipulation study in a northern hardwood forest ecosystem. Biogeochemistry 56, 135–150. doi: 10.1023/A:1013039830323
Hernández Ayala, J. J., Mann, J., Grosvenor, E. (2021). Antecedent rainfall, excessive vegetation growth and its relation to wildfire burned areas in California. Earth Space Sci. 8, e2020EA001624. doi: 10.1029/2020EA001624
Hu, Y., Li, X. Y., Guo, A. X., Yue, P., Guo, X. X., Lv, P., et al. (2022). Species diversity is a strong predictor of ecosystem multifunctionality under altered precipitation in desert steppes. Ecol. Indic. 137, Artikkel 108762. doi: 10.1016/j.ecolind.2022.108762
Jeong, S.-J., Ho, C.-H., Gim, H.-J., Brown, M. E. (2011). Phenology shifts at start vs. end of growing season in temperate vegetation over the Northern Hemisphere for the period 1982–2008. Global Change Biol. 17, 2385–2399. doi: 10.1111/j.1365-2486.2011.02397.x
Ji, S., Ren, S., Li, Y., Dong, J., Wang, L., Quan, Q., et al. (2021). Diverse responses of spring phenology to preseason drought and warming under different biomes in the North China Plain. Sci. Total Environ. 766, 144437. doi: 10.1016/j.scitotenv.2020.144437
Jiang, N., Shen, M., Chen, J., Yang, W., Zhu, X., Wang, X., et al. (2023). Continuous advance in the onset of vegetation green-up in the Northern Hemisphere, during hiatuses in spring warming. NPJ Climate Atmos. Sci. 6, 7. doi: 10.1038/s41612-023-00343-0
Jiao, W., Wang, L., Smith, W. K., Chang, Q., Wang, H., D’Odorico, P. (2021). Observed increasing water constraint on vegetation growth over the last three decades. Nat. Commun. 12, 3777. doi: 10.1038/s41467-021-24016-9
Jin, H., Jönsson, A. M., Olsson, C., Lindström, J., Jönsson, P., Eklundh, L. (2019). New satellite-based estimates show significant trends in spring phenology and complex sensitivities to temperature and precipitation at northern European latitudes. Int. J. Biometeorol. 63, 763–775. doi: 10.1007/s00484-019-01690-5
Korell, L., Auge, H., Chase, J. M., Harpole, W. S., Knight, T. M. (2021). Responses of plant diversity to precipitation change are strongest at local spatial scales and in drylands. Nat. Commun. 12, 2489. doi: 10.1038/s41467-021-22766-0
Li, J., Guan, J., Han, W., Tian, R., Lu, B., Yu, D., et al. (2023). Important role of precipitation in controlling a more uniform spring phenology in the Qinba Mountains, China. Front. Plant Sci. 14, 1074405. doi: 10.3389/fpls.2023.1074405
Li, C., Stevens, B., Marotzke, J. (2015b). Eurasian winter cooling in the warming hiatus of 1998–2012. Geophys. Res. Lett. 42, 8131–8139. doi: 10.1002/2015GL065327
Li, K., Wang, C., Sun, Q., Rong, G., Tong, Z., Liu, X., et al. (2021). Spring phenological sensitivity to climate change in the northern hemisphere: comprehensive evaluation and driving force analysis. Remote Sens. 13, 1972. doi: 10.3390/rs13101972
Li, Q., Yang, S., Xu, W., Wang, X. L., Jones, P., Parker, D., et al. (2015c). China experiencing the recent warming hiatus. Geophys. Res. Lett. 42, 889–898. doi: 10.1002/2014GL062773
Li, Y., Zhao, M., Motesharrei, S., Mu, Q., Kalnay, E., Li, S. (2015a). Local cooling and warming effects of forests based on satellite observations. Nat. Commun. 6, 6603. doi: 10.1038/ncomms7603
Linkosalo, T., Lappalainen, H. K., Hari, P. (2008). A comparison of phenological models of leaf bud burst and flowering of boreal trees using independent observations. Tree Physiol. 28, 1873–1882. doi: 10.1093/treephys/28.12.1873
Liu, Z., Fu, Y. H., Shi, X., Lock, T. R., Kallenbach, R. L., Yuan, Z. (2022). Soil moisture determines the effects of climate warming on spring phenology in grasslands. Agric. For. Meteorol. 323, 109039. doi: 10.1016/j.agrformet.2022.109039
Liu, Y., Liu, X., Fu, Z., Zhang, D., Liu, L. (2024). Soil temperature dominates forest spring phenology in China. Agric. For. Meteorol. 355, 110141. doi: 10.1016/j.agrformet.2024.110141
Luedeling, E., Zhang, M., McGranahan, G., Leslie, C. (2009). Validation of winter chill models using historic records of walnut phenology. Agric. For. Meteorol. 149, 1854–1864. doi: 10.1016/j.agrformet.2009.06.013
Luo, S., Gu, X., Lv, N., Guan, Y., Kong, D., Liu, J., et al. (2024). Elevated increases in human-perceived winter temperature in urban areas over China: Acceleration by urbanization. Urban Climate 55, 101979. doi: 10.1016/j.uclim.2024.101979
Luo, X., LI, Y., Yin, H., Sui, M. (2020). Response of NDVI to SPEI at different temporal scales in Northeast China. Chin. J. Ecol. 39, 412–421. doi: 10.13292/j.1000-4890.202002.025
Ma, R., Shen, X., Zhang, J., Xia, C., Liu, Y., Wu, L., et al. (2022). Variation of vegetation autumn phenology and its climatic drivers in temperate grasslands of China. International. J. Appl. Earth Observ. Geoinform. 114, 103064. doi: 10.1016/j.jag.2022.103064
Man, R., Lu, P., Dang, Q. L. (2017). Insufficient chilling effects vary among boreal tree species and chilling duration. Front. Plant Sci. 8, 1354. doi: 10.3389/fpls.2017.01354
Marambe, B., Wijesundara, S. (2021). Effects of climate change on weeds and invasive alien plants in Sri Lankan agro-ecosystems: policy and management implications. Front. Agron. 3, 641006. doi: 10.3389/fagro.2021.641006
Maurer, G. E., Bowling, D. R. (2014). Seasonal snowpack characteristics influence soil temperature and water content at multiple scales in interior western U.S. mountain ecosystems. Water Resour. Res. 50, 5216–5234. doi: 10.1002/2013WR014452
McCabe, G. J., Wolock, D. M. (2010). Long-term variability in Northern Hemisphere snow cover and associations with warmer winters. Clim. Change 99, 141–153. doi: 10.1007/s10584-009-9675-2
Meng, L., Zhou, Y., Li, X., Asrar, G. R., Mao, J., Wanamaker, A. D., et al. (2020). Divergent responses of spring phenology to daytime and nighttime warming. Agric. For. Meteorol. 281, 107832. doi: 10.1016/j.agrformet.2019.107832
Montgomery, R. A., Rice, K. E., Stefanski, A., Rich, R. L., Reich, P. B. (2020). Phenological responses of temperate and boreal trees to warming depend on ambient spring temperatures, leaf habit, and geographic range. Proc. Natl. Acad. Sci. 117, 10397–10405. doi: 10.1073/pnas.1917508117
Ogle, K., Barber, J. J., Barron-Gafford, G. A., Bentley, L. P., Young, J. M., Huxman, T. E., et al. (2015). Quantifying ecological memory in plant and ecosystem processes. Ecol. Lett. 18, 221–235. doi: 10.1111/ele.12399
Ohmura, A. (2012). Enhanced temperature variability in high-altitude climate change. Theor. Appl. Climatol. 110, 499–508. doi: 10.1007/s00704-012-0687-x
Park, H., Jeong, S.-J., Ho, C.-H., Park, C.-E., Kim, J. (2018). Slowdown of spring green-up advancements in boreal forests. Remote Sens. Environ. 217, 191–202. doi: 10.1016/j.rse.2018.08.012
Park, H., Jeong, S., Peñuelas, J. (2020). Accelerated rate of vegetation green-up related to warming at northern high latitudes. Global Change Biol. 26, 6190–6202. doi: 10.1111/gcb.15322
Peng, S., Piao, S., Ciais, P., Myneni, R. B., Chen, A., Chevallier, F., et al. (2013). Asymmetric effects of daytime and night-time warming on Northern Hemisphere vegetation. Nature 501, 88–92. doi: 10.1038/nature12434
Peng, Y., Yang, J.-X., Zhou, X.-H., Peng, P.-H., Li, J.-J., He, W.-M. (2018). Warming delays the phenological sequences of an autumn-flowering invader. Ecol. Evol. 8, 6299–6307. doi: 10.1002/ece3.4177
Piao, S., Fang, J., Zhou, L., Ciais, P., Zhu, B. (2006). Variations in satellite-derived phenology in China’s temperate vegetation. Global Change Biol. 12, 672–685. doi: 10.1111/j.1365-2486.2006.01123.x
Piao, S., Liu, Q., Chen, A., Janssens, I. A., Fu, Y., Dai, J., et al. (2019). Plant phenology and global climate change: Current progresses and challenges. Global Change Biol. 25, 1922–1940. doi: 10.1111/gcb.14619
Piao, S., Tan, J., Chen, A., Fu, Y. H., Ciais, P., Liu, Q., et al. (2015). Leaf onset in the northern hemisphere triggered by daytime temperature. Nat. Commun. 6, 6911. doi: 10.1038/ncomms7911
Piao, S., Wang, X., Park, T., Chen, C., Lian, X., He, Y., et al. (2020). Characteristics, drivers and feedbacks of global greening. Nat. Rev. Earth Environ. 1, 14–27. doi: 10.1038/s43017-019-0001-x
Post, E., Stenseth, N. C. (1999). Climatic variability, plant phenology, and northern ungulates. Ecology 80, 1322–1339. doi: 10.1890/0012-9658(1999)080[1322:CVPPAN]2.0.CO;2
Qiao, D., Zhou, J., Liang, S., Fu, X. (2019). Combined effects of precipitation and temperature on the responses of forest spring phenology to winter snow cover dynamics in northeast China. IEEE Access PP, 1–1. doi: 10.1109/ACCESS.2019.2943202
Qiu, Z., Feng, Z., Song, Y., Li, M., Zhang, P. (2020). Carbon sequestration potential of forest vegetation in China from 2003 to 2050: Predicting forest vegetation growth based on climate and the environment. J. Clean. Prod. 252, 119715. doi: 10.1016/j.jclepro.2019.119715
Ren, S., Yi, S., Peichl, M., Wang, X. (2017). Diverse responses of vegetation phenology to climate change in different grasslands in inner Mongolia during 2000–2016. Remote Sens. 10, 17. doi: 10.3390/rs10010017
Richardson, M. T. (2022). Prospects for detecting accelerated global warming. Geophys. Res. Lett. 49, e2021GL095782. doi: 10.1029/2021GL095782
Sanmiguel-Vallelado, A., Camarero, J. J., Morán-Tejeda, E., Gazol, A., Colangelo, M., Alonso-González, E., et al. (2021). Snow dynamics influence tree growth by controlling soil temperature in mountain pine forests. Agric. For. Meteorol. 296, 108205. doi: 10.1016/j.agrformet.2020.108205
Scafetta, N. (2024). Impacts and risks of “realistic” global warming projections for the 21st century. Geosci. Front. 15, 101774. doi: 10.1016/j.gsf.2023.101774
Schwartz, M. D., Ahas, R., Aasa, A. (2006). Onset of spring starting earlier across the Northern Hemisphere. Global Change Biol. 12, 343–351. doi: 10.1111/j.1365-2486.2005.01097.x
Shahzad, K., Alatalo, J. M., Zhu, M., Cao, L., Hao, Y., Dai, J. (2024). Geographic conditions impact the relationship between plant phenology and phylogeny. Sci. Total Environ. 945, 174083. doi: 10.1016/j.scitotenv.2024.174083
Shen, X., Liu, B., Henderson, M., Wang, L., Wu, Z., Wu, H., et al. (2018). Asymmetric effects of daytime and nighttime warming on spring phenology in the temperate grasslands of China. Agric. For. Meteorol. 259, 240–249. doi: 10.1016/j.agrformet.2018.05.006
Shen, X., Liu, B., Xue, Z., Jiang, M., Lu, X., Zhang, Q. (2019). Spatiotemporal variation in vegetation spring phenology and its response to climate change in freshwater marshes of Northeast China. Sci. Total Environ. 666, 1169–1177. doi: 10.1016/j.scitotenv.2019.02.265
Shen, M., Piao, S., Cong, N., Zhang, G., Jassens, I. A. (2015a). Precipitation impacts on vegetation spring phenology on the Tibetan Plateau. Global Change Biol. 21, 3647–3656. doi: 10.1111/gcb.12961
Shen, M., Piao, S., Jeong, S.-J., Zhou, L., Zeng, Z., Ciais, P., et al. (2015b). Evaporative cooling over the Tibetan Plateau induced by vegetation growth. Proc. Natl. Acad. Sci. 112, 9299–9304. doi: 10.1073/pnas.1504418112
Shen, X., Shen, M., Wu, C., Penuelas, J., Ciais, P., Zhang, J., et al. (2023). Critical role of water conditions in the responses of autumn phenology of marsh wetlands to climate change on the Tibetan Plateau. Global Change Biol. 30, e17097. doi: 10.1111/gcb.17097
Small, E., Roesler, C., Larson, K. (2018). Vegetation response to the 2012–2014 California drought from GPS and optical measurements. Remote Sens. 10, 630. doi: 10.3390/rs10040630
Smith, A. M., Ramsay, P. M. (2020). A comparison of ground-based methods for obtaining large-scale, high-resolution data on the spring leaf phenology of temperate tree species. Int. J. Biometeorol. 64, 521–531. doi: 10.1007/s00484-019-01839-2
Su, M., Huang, X., Xu, Z., Zhu, W., Lin, Z. (2022). A decrease in the daily maximum temperature during global warming hiatus causes a delay in spring phenology in the China–DPRK–Russia cross-border area. Remote Sens. 14, 1462. doi: 10.3390/rs14061462
Sun, W., Liu, X. (2019). Review on carbon storage estimation of forest ecosystem and applications in China. For. Ecosyst. 7, 4. doi: 10.1186/s40663-019-0210-2
Sun, Y., Zhang, Y., Zheng, Z., Zhao, G., Zhu, Y., Gao, J., et al. (2023). Strong inhibiting effect of daytime warming but weak promoting effect of nighttime warming on carbon use efficiency in Northern Hemisphere. Global Planet. Change 228, 104192. doi: 10.1016/j.gloplacha.2023.104192
Trenberth, K. E., Fasullo, J. T., Branstator, G., Phillips, A. S. (2014). Seasonal aspects of the recent pause in surface warming. Nat. Climate Change 4, 911–916. doi: 10.1038/nclimate2341
Vogel, J. (2022). Drivers of phenological changes in southern Europe. Int. J. Biometeorol. 66, 1903–1914. doi: 10.1007/s00484-022-02331-0
Wang, J., Fang, Y., Zou, X., Chai, G., Wang, Z. (2022a). Diverse responses of vegetation phenology to changes in temperature and precipitation in Northern China. Geocarto Int. 37, 12561–12579. doi: 10.1080/10106049.2022.2068677
Wang, J., Ives, N. E., Lechowicz, M. J. (1992). The relation of foliar phenology to xylem embolism in trees. Funct. Ecol. 6, 469–475. doi: 10.2307/2389285
Wang, Y., Lv, W., Xue, K., Wang, S., Zhang, L., Hu, R., et al. (2022c). Grassland changes and adaptive management on the Qinghai–Tibetan Plateau. Nat. Rev. Earth Environ. 3, 668–683. doi: 10.1038/s43017-022-00330-8
Wang, Z., Ma, Y., Zhang, Y., Shang, J. (2022b). Review of remote sensing applications in grassland monitoring. Remote Sens. 14, 2903. doi: 10.3390/rs14122903
Wang, Y., Shen, X., Jiang, M., Tong, S., Lu, X. (2022d). Daytime and nighttime temperatures exert different effects on vegetation net primary productivity of marshes in the western Songnen Plain. Ecol. Indic. 137, 108789. doi: 10.1016/j.ecolind.2022.108789
Wang, X., Xiao, J., Li, X., Cheng, G., Ma, M., Zhu, G., et al. (2019). No trends in spring and autumn phenology during the global warming hiatus. Nat. Commun. 10, 2389. doi: 10.1038/s41467-019-10235-8
White, M. A., De Beurs, K. M., Didan, K., Inouye, D. W., Richardson, A. D., Jensen, O. P., et al. (2009). Intercomparison, interpretation, and assessment of spring phenology in North America estimated from remote sensing for 1982–2006. Global Change Biol. 15, 2335–2359. doi: 10.1111/j.1365-2486.2009.01910.x
Wilson, G., Green, M., Brown, J., Campbell, J., Groffman, P., Durán, J., et al. (2020). Snowpack affects soil microclimate throughout the year. Clim. Change 163, 705–722. doi: 10.1007/s10584-020-02943-8
Xu, X., Riley, W. J., Koven, C. D., Jia, G., Zhang, X. (2020). Earlier leaf-out warms air in the north. Nat. Climate Change 10, 370–375. doi: 10.1038/s41558-020-0713-4
Yu, H., Luedeling, E., Xu, J. (2010). Winter and spring warming result in delayed spring phenology on the Tibetan Plateau. Proc. Natl. Acad. Sci. 107, 22151–22156. doi: 10.1073/pnas.1012490107
Yuan, M., Zhao, L., Lin, A., Li, Q., She, D., Qu, S. (2020). How do climatic and non-climatic factors contribute to the dynamics of vegetation autumn phenology in the Yellow River Basin, China? Ecol. Indic. 112, 106112. doi: 10.1016/j.ecolind.2020.106112
Yun, J., Jeong, S.-J., Ho, C.-H., Park, C.-E., Park, H., Kim, J. (2018). Influence of winter precipitation on spring phenology in boreal forests. Global Change Biol. 24, 5176–5187. doi: 10.1111/gcb.14414
Zhang, H., Chuine, I., Regnier, P., Ciais, P., Yuan, W. (2022b). Deciphering the multiple effects of climate warming on the temporal shift of leaf unfolding. Nat. Climate Change 12, 193–199. doi: 10.1038/s41558-021-01261-w
Zhang, F., Liu, B., Henderson, M., Shen, X., Su, Y., Zhou, W. (2022c). Changing spring phenology of northeast China forests during rapid warming and short-term slowdown periods. Forests 13, 2173. doi: 10.3390/f13122173
Zhang, H., Liu, S., Regnier, P., Yuan, W. (2018). New insights on plant phenological response to temperature revealed from long-term widespread observations in China. Global Change Biol. 24, 2066–2078. doi: 10.1111/gcb.14002
Zhang, R., Qi, J., Leng, S., Wang, Q. (2022a). Long-term vegetation phenology changes and responses to preseason temperature and precipitation in northern China. Remote Sens. 14, 1396. doi: 10.3390/rs14061396
Zhang, F., Su, Y., Liu, B. (2023). Response characteristics of NDVI to asymmetric diurnal temperature increase and precipitation changes during the forest growing season in Northeast China. J. Beijing Forest. Univ. 45, 34–48. doi: 10.12171/j.1000-1522.20210396
Zhang, R., Sun, C., Zhang, R., Li, W., Zuo, J. (2019). Role of Eurasian snow cover in linking winter-spring Eurasian coldness to the autumn arctic sea ice retreat. J. Geophys. Res.: Atmos. 124, 9205–9221. doi: 10.1029/2019JD030339
Zhao, X., Chen, F., Seim, A., Hu, M., Akkemik, Ü., Kopabayeva, A., et al. (2024). Global warming leads to growth increase in Pinus sylvestris in the Kazakh steppe. For. Ecol. Manage. 553, 121635. doi: 10.1016/j.foreco.2023.121635
Zhao, J., Zhang, H., Zhang, Z., Guo, X., Li, X., Chen, C. (2015). Spatial and temporal changes in vegetation phenology at middle and high latitudes of the northern hemisphere over the past three decades. Remote Sens. 7, 10973–10995. doi: 10.3390/rs70810973
Keywords: vegetation, spring phenology, response efficiency, climate change, minimum temperature, precipitation
Citation: Chen M, Henderson M, Liu B, Zhou W, Ma R, Huang W and Dou Z (2024) Winter climate change mediates the sensitivity of vegetation leaf-out to spring warming in high latitudes in China. Front. Plant Sci. 15:1476576. doi: 10.3389/fpls.2024.1476576
Received: 06 August 2024; Accepted: 12 November 2024;
Published: 02 December 2024.
Edited by:
Manyu Dong, Beijing Normal University, ChinaCopyright © 2024 Chen, Henderson, Liu, Zhou, Ma, Huang and Dou. This is an open-access article distributed under the terms of the Creative Commons Attribution License (CC BY). The use, distribution or reproduction in other forums is permitted, provided the original author(s) and the copyright owner(s) are credited and that the original publication in this journal is cited, in accordance with accepted academic practice. No use, distribution or reproduction is permitted which does not comply with these terms.
*Correspondence: Binhui Liu, YmluaHVpQG5lZnUuZWR1LmNu
Disclaimer: All claims expressed in this article are solely those of the authors and do not necessarily represent those of their affiliated organizations, or those of the publisher, the editors and the reviewers. Any product that may be evaluated in this article or claim that may be made by its manufacturer is not guaranteed or endorsed by the publisher.
Research integrity at Frontiers
Learn more about the work of our research integrity team to safeguard the quality of each article we publish.