- 1Soybean Breeding and Genetics Lab, Centre de recherche sur les grains (CÉROM) Inc., St-Mathieu-de-Beloeil, QC, Canada
- 2Department of Plant Science, McGill University, Montréal, QC, Canada
Deciphering the gene regulatory networks of critical quantitative trait loci associated with early maturity provides information for breeders to unlock soybean’s (Glycine max (L.) Merr.) northern potential and expand its cultivation range. The E8-r3 locus is a genomic region regulating the number of days to maturity under constant short-day photoperiodic conditions in two early-maturing soybean populations (QS15524F2:F3 and QS15544RIL) belonging to maturity groups MG00 and MG000. In this study, we developed a combinatorial expression quantitative trait loci mapping approach using three algorithms (ICIM, IM, and GCIM) to identify the regions that regulate three candidate genes of the E8-r3 locus (Glyma.04G167900/GmLHCA4a, Glyma.04G166300/GmPRR1a, and Glyma.04G159300/GmMDE04). Using this approach, a total of 2,218 trans (2,061 genes)/7 cis (7 genes) and 4,073 trans (2,842 genes)/3,083 cis (2,418 genes) interactions were mapped in the QS15524F2:F3 and QS15544RIL populations, respectively. From these interactions, we successfully identified two hotspots (F2_GM15:49,385,092-49,442,237 and F2_GM18:1,434,182-1,935,386) and three minor regions (RIL_GM04:17,227,512-20,251,662, RIL_GM04:31,408,946-31,525,671 and RIL_GM13:37,289,785-38,620,690) regulating the candidate genes of E8-r3 and several of their homologs. Based on co-expression network and single nucleotide variant analyses, we identified ALTERED PHLOEM DEVELOPMENT (Glyma.15G263700) and DOMAIN-CONTAINING PROTEIN 21 (Glyma.18G025600) as the best candidates for the F2_GM15:49,385,092-49,442,237 and F2_GM18:1,434,182-1,935,386 hotspots. These findings demonstrate that a few key regions are involved in the regulation of the E8-r3 candidates GmLHCA4a, GmPRR1a, and GmMDE04.
Introduction
Soybean (Glycine max (L.) Merr.) is the most important leguminous oilseed crop and significantly contributes to maintaining food security on a global scale. This crop is mainly cultivated in countries located in warm temperate, subtropical, and/or tropical areas, and the contribution of northern countries such as Canada (2%) to the global soybean output remains modest (The American Soybean Association, 2023). Current projections suggest limited growth in soybean production for countries located in tropical and subtropical countries (Ali et al., 2022); however, due to the projected rise in world population and anticipated growth in the international need for soybean-related food and industrial goods, global production will need to increase to supply the growing demand (Unc et al., 2021). One approach to partly solve this problem is to improve soybean adaptability to northern regions beyond its actual limits (~54°N) and fine-tune its reproductive phenology by identifying the critical transcription factors regulating the extra-early flowering and maturity phenotypes.
Transcription factors (TFs) are critical proteins that regulate the transcription of one or multiple downstream targets by binding to cis-regulatory elements (CRE) with their DNA-binding domains (DBD) (Bylino et al., 2020). In soybean, 6,150 TFs (3,747 loci) belonging to 57 families have been predicted (Jin et al., 2017), with several having reported flowering regulatory functions such as E1 (Xia et al., 2012), E1-like-a (Liu et al., 2022), E1-like-b (Zhu et al., 2019), and LHY1a/1b/2a/2b (Glyma.16G017400, Glyma.07G048500, Glyma.19G260900, and Glyma.03G261800, respectively) (Bian et al., 2017). In Arabidopsis, core regulators of the circadian clock are all TFs and include CIRCADIAN CLOCK-ASSOCIATED1 (AtCCA1), LATE ELONGATED HYPOCOTYL (AtLHY), and the evening-expressed gene, TIMING OF CAB EXPRESSION1 (AtTOC1) (Wang and Ma, 2013). As the main biological timekeeper, the circadian clock gates the global molecular response to the environmental cues, the zeitgebers, in a timely fashion. From an agronomical standpoint, these multiple interlocked transcription-translation feedback loops comprised within the circadian clock regulate essential metabolic functions (e.g. photosynthesis and reproductive phenology) with potential effects on critical traits such as maturity, yield, and disease resistance (Hotta, 2021). In particular, the cryptochrome and phytochrome photoreceptors regulate many key aspects of the circadian clock and act as a molecular bridge between photosynthesis, development, and reproduction (Venkat and Muneer, 2022). As a consequence, photosynthesis and reproduction are intertwined at the molecular level due to specific genes (e.g. PHYTOCHROME A2/A3) acting to control photoperiodic flowering (Lin et al., 2022).
Generating a compendium of interactions for one specific TF is challenging due to the transient nature of the regulatory mechanisms and the intricate density of the underlying regulatory networks. One approach to solve this issue is to perform expression quantitative trait loci (eQTL) mapping on a genome-wide scale to identify proximal/cis (within a 1-Mbp window of the transcription start site) and distal/trans single nucleotide polymorphisms influencing the level of messenger RNA (mRNA) expression (Gilad et al., 2008; Westra and Franke, 2014). Expression quantitative trait loci hotspots are genetic variations, most often located in genes coding for TFs, that regulate the expression level of numerous genes, often in the hundreds to thousands (Choi et al., 2020). Obtaining sufficient statistical power is often challenging in eQTL mapping due to the prohibitive financial cost associated with sufficient reading depth and the computational burden of transcriptome-wide measurements on hundreds of lines. To overcome this challenge, numerous mapping algorithms, such as Genome-wide composite interval mapping (GCIM) (Zhang et al., 2020) and Inclusive Composite Interval mapping (Li et al., 2007), have been developed to improve the identification of small-effect eQTLs, which are most often located in trans (Westra and Franke, 2014). We believe that used in conjunction, these methods have an increased ability to identify regions of interest for given phenotypes and can also be used to map eQTL interactions and associated regulatory hotspots with increased precision.
In a previous study, we identified a QTL region named E8-r3 located between the GM04:41,808,599 and GM04:42,376,237 flanking markers that regulates the number of days to maturity under a constant short-day photoperiod in two early-maturing soybean populations (QS15524F2:F3 and QS15544RIL) (Gélinas Bélanger et al., 2024a). The same region was not identified when these populations were grown under fluctuating long-day conditions under Canadian field conditions, suggesting that this region is specifically involved in photoperiodic responses under short days. In this previous study, we also identified that this region regulates the expression of several genes, including E6 (Glyma.04G050200), an ortholog of Arabidopsis thaliana EARLY FLOWERING 3 that has been demonstrated to have an effect on the flowering of soybean using a combinatorial eQTL mapping approach (Fang et al., 2021; Gélinas Bélanger et al., 2024a). The associated QTL region identified for the short-day phenotypic response encompasses 29 genes and is implicated in the ‘Photosynthesis - antenna proteins’ KEGG pathway. In total, we have proposed three candidate genes (Glyma.04G168300, Glyma.04G167900, and Glyma.04G166300) for this region based on a candidate single nucleotide polymorphisms (SNPs) analysis. Two of these genes, Glyma.04G166300 (PSEUDO–RESPONSE REGULATOR 1a; GmPRR1a) (Liu et al., 2009; Dietz et al., 2022) and Glyma.04G168300 (CYCLING DOF FACTOR 3; GmCDF3) (Corrales et al., 2017), encode TFs involved in the circadian clock, developmental processes and regulation of maturity, thus suggesting that TFs might be involved in the regulation of the E8-r3 locus. The other gene, Glyma.04G167900 (LIGHT-HARVESTING CHLOROPHYLL-PROTEIN COMPLEX I SUBUNIT A4a; LHCA4a), is involved in photosynthetic activities and possibly regulated by TFs located in cis or in trans. Recently, a gene coding for a MADS-box transcription factor, Glyma.04G159300 (MADS-BOX DOWNREGULATED BY E1 04; GmMDE04), was found to be statistically associated with the GM04:39,294,836 marker for the flowering time (i.e. R1 stage), maturity (i.e. R8 stage), and reproductive length (i.e. the difference between R8 and R1) traits (Escamilla et al., 2024). Although this gene is located outside of E8-r3 flanking markers, our lab is currently reconsidering its potential role as a regulator for this locus based on the results found by Escamilla et al. (2024). The objective of the present study is to identify novel eQTLs using an approach combining multiple mapping techniques in two early-maturing soybean populations. Overall, this study aims at (i) validating an eQTL mapping pipeline based on a combinatorial mapping strategy; (ii) identifying eQTL signals and hotspots regulating the genes involved in flowering, maturity, and photosynthesis; (iii) locating the eQTL signals interacting with the E8-r3 region; and (iv) identifying candidate TFs and characterizing their co-expression networks.
Materials and methods
Plant materials, growing conditions and phenotyping
The populations and phenotyping procedures were generated and performed as detailed in Gélinas Bélanger et al. (2024a). Briefly, the QS15524F2:F3 population was generated from a biparental cross between ‘Maple Arrow’ (MG00; later-maturing accession) × ‘OAC Vision’ (PI 567787) (MG000; earlier-maturing accession), now herein respectively referred as MA and OV. The QS15544RIL population was generated from the biparental cross between ‘AAC Mandor’ (MG00; later-maturing accession) × ‘9004’ (MG000; earlier-maturing accession), the former now being herein referred to as MD. The parental lines in each population were fixed for their E1 (Glyma.06G207800) (Xia et al., 2012), E2 (Glyma.10G221500) (Watanabe et al., 2011), E3 (Glyma.19G224200) (Watanabe et al., 2009), and E4 (Glyma.20G090000) (Liu et al., 2008) alleles. As such, the genotypes for the QS15524F2:F3 parental lines were e1-nl/e2-ns/E3Ha/e4-SORE-1 and e1-as/e2-ns/e3-tr/e4p.T832QfsX21 for the QS15544RIL parental lines. The e4p.T832QfsX21 allele is a rare premature stop codon mutation previously identified in Tardivel et al. (2019).
The QS15524F2:F3 population was grown and phenotyped in a greenhouse during the winter of 2017-2018 at the Centre de recherche sur les grains inc. (CÉROM) in St-Mathieu-de-Beloeil (QC, Canada), GPS coordinates 45°34’57.9”N 73°14’11.4”W. In the case of QS15544RIL, the population (F5:F6 generation) was grown and phenotyped in a greenhouse during the winter of 2019-2020. Plants for the offspring and parental lines were sown on December 14th 2017 and October 25th 2019 for the QS15524F2:F3 and QS15544RIL populations, respectively. During the experiments, natural photoperiod was below 12h but maintained artificially at 12h using sodium halogen lights at all time before flowering since flowering for all plants happened before the March Equinox. Both populations were grown following a custom Modified Augmented Design (Lin & Poushinsky, 1983, 1985) with 19 individuals per table and one parent per table. For each population, the plants were sown in one-gallon pots containing a ProMix-garden soil (1:1 v:v) (Premier Tech Horticulture, Rivière-du-Loup, QC, Canada) potting mix. For the QS15524F2:F3 offspring, one seed was planted per pot, whereas three seeds were sown per pot for the QS15544RIL offspring. As reported by Gélinas Bélanger et al. (2024a), the OV and MA parents of the QS15524F2:F3 population respectively matured in 85 and 96 days. For the QS15544RIL population, it was observed that the MD and ‘9004’ lines matured in 87.5 and 87.2 days, respectively.
Sampling, nucleic acid extraction and sequencing
The sampling and sequencing procedures were performed as detailed in Gélinas Bélanger et al. (2024a). Briefly, leaf tissue for RNA extraction was harvested by making six 4 mm plugs in the uppermost expanding middle leaflet of the trifoliate leaf 4 hours after sunrise at the V4 leaf stage (25 days post-seeding), frozen immediately in liquid nitrogen and stored at -80°C until further use (Figure 1A). The time points were chosen based on previously published data indicating highest expression of flowering genes four hours after sunrise (Kong et al., 2010; Sun et al., 2011). Furthermore, the V4 stage was determined based on preliminary qRT-PCR analyses of the expression of the flowering genes Glyma.16G150700 (FLOWERING LOCUS T 2A; GmFT2a) and Glyma.16G044100 (FLOWERING LOCUS T 5A; GmFT5a) in the parental lines (data not shown). To do so, we compared the expression for the V1 to V5 stages and chose the stage which exhibited the highest expression for both of these genes as the FT florigens promote the transition to reproductive development and flowering. The extraction and purification of total DNA from leaf tissue was performed using the Omega Bio-Tek Mag-bind Plant Kit and Mag-Bind Total Pure NGS kit (Omega Biotek, Georgia state, USA). Construction of the whole genome sequencing (WGS) libraries for the QS15524F2:F3 parental lines was performed by pooling the leaf tissue from the five pots of each parent. Extraction of total DNA and library preparation was performed at the Génome Québec Innovation Centre (Montréal, Canada) using the NxSeq® AmpFREE Library Preparation kit (Lucigen, Wisconsin, U.S.A.). The two parental libraries were combined and sequenced at a 15X depth on the Illumina HiSeq X platform with 150 bp paired-end reads.
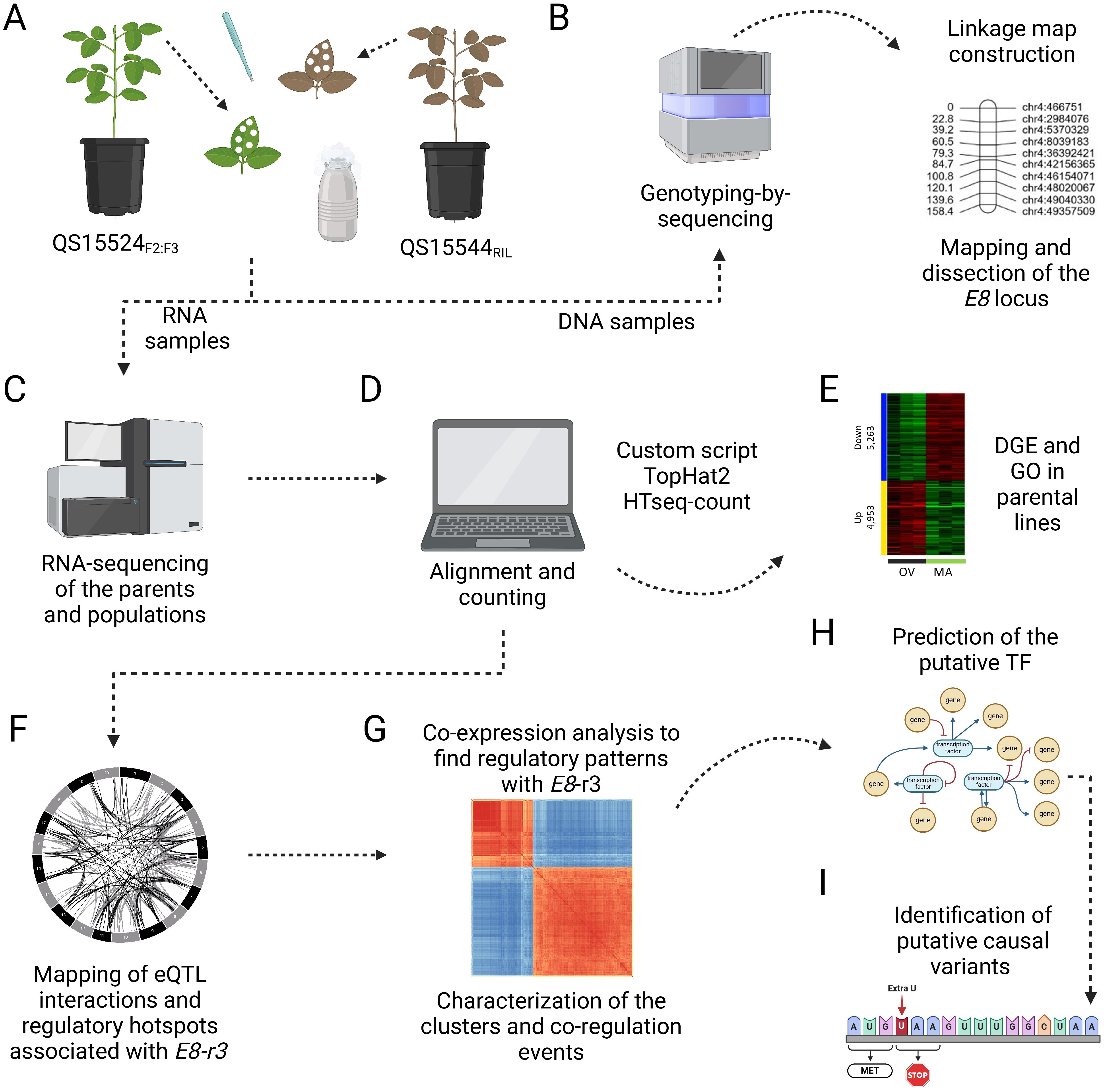
Figure 1. Experimental pipeline to identify candidate TFs involved in the regulation of E8-r3 genes. (A) Leaf tissue collection of the middle leaflet at the V4 stage followed by DNA and RNA extraction. (B) Generation of the linkage map with the genotyping-by-sequencing datasets and mapping of the E8-r3 candidate genes with ICIM and GCIM as detailed in Gélinas Bélanger et al. (2024a). (C) Isolation of mRNA and sequencing on the Illumina NovaSeq6000 platform. (D) Alignment and counting of the RNA-seq datasets using various bioinformatic scripts. (E) Identification of differentially expressed genes in the parental lines to validate the experimental conditions. (F) Mapping of the overlapping eQTL interactions using three algorithms (ICIM, IM, and GCIM) and identification of the trans interactions associated with GmLHCA4a, GmPRR1a, and GmMDE04 candidate genes found in the E8-r3 region. (G) Building of CENs between the genes to identify homologous genes and closely associated candidate TFs. (H) Deeper investigations of the candidate TFs using TWCENs, gene expression, and FRSPD_GO annotations. (I) Identification of putative causal variants in the candidate TFs. Created with BioRender.com.
The genotyping-by-sequencing (GBS) libraries of the QS15524F2:F3 and QS15544RIL mapping populations were prepared using the PstI/MspI enzymes as described in Abed et al. (2019) at the Institute of Integrative Biology and Systems (Laval University, Québec, Canada). Sequencing of the QS15524F2:F3 GBS libraries was performed by randomly combining a total of 91 barcoded samples per library and by sequencing with four Ion PI V3 chips per library (Figure 1B). For the QS15544RIL population, the samples were randomly divided into two sets of 91 samples and sequenced with two Ion PI V3 chips per library. Sequencing for all libraries was performed on the Ion Proton Sequencer and HiQ chemistry at the Institute of Integrative Biology and Systems (Laval University, Québec, Canada).
Total RNA was extracted from samples using a standard Trizol RNA extraction procedure with two extra ethanol rinses to improve purity. Messenger RNA (mRNA) was isolated using the NEBNext mRNA stranded library preparation kit (New England Biolabs, Ontario, Canada) at the Génome Québec Innovation Centre (Montréal, Canada). Two libraries containing 96 pooled samples were prepared per population. Each library was then sequenced on two Illumina NovaSeq6000 S2 (QS15524F2:F3) or S4 (QS15544RIL) lanes at the Génome Québec Innovation Centre (Montréal, Canada), with four sequencing lanes per population and a total of 8000 M and 9600 M paired-end reads per population, respectively (Figure 1C).
Bioinformatics
The bioinformatic analyses were performed as detailed in Gélinas Bélanger et al. (2024a). Briefly, alignment of all the sequences was performed using version 2 of the Glycine max reference genome (Gmax_275_v2.0) (https://phytozome-next.jgi.doe.gov/) (Accessed 8 December 2017; https://data.jgi.doe.gov/). Processing of the WGS sequencing datasets of the QS15524F2:F3 parental lines was performed using the fast-WGS pipeline with the default settings (Torkamaneh et al., 2017). The processing of GBS datasets was performed using the fast-GBS pipeline (Torkamaneh et al., 2017) (Figure 1B). Variant calling was performed with Platypus version 0.8.1 (Rimmer et al., 2014) with the following commands: –minReads=2, –minMapQual=20 and –minBaseQual=20. Subsequently, a filtering step using vcftools version 0.1.16 (Danecek et al., 2011) was performed with the following parameters: (i) remove non-biallelic sites; (ii) remove InDels; (iii) remove scaffolds; and (iv) filter alleles using the –maxmissing 0.2, –maf 0.3 and –mac 4 commands. Self-imputation was then performed on the missing data for the QS15524F2:F3 and QS15544RIL populations using Beagle version 4.1.0 (Browning and Browning, 2007) with twelve iterations. Phasing was then performed with Convert2Map (https://bitbucket.org/jerlar73/convert-genotypes-to-mapping-files/src/master/) using the fast-WGS resequencing data for the QS15524F2:F3 parental lines and the GmHapMap dataset for the QS15544 parental lines. A last round of filtering was performed in the QS15544RIL dataset by removing all SNPs with > 10% heterozygous calls before binning with QTL IciMapping (Meng et al., 2015). For QS15524F2:F3, the binning step was performed with Genotype Corrector.
Processing of the RNA datasets was performed using multiple publicly available software tools with an in-house script (Figure 1D). Briefly, adapters were removed using Trimmomatic version 0.33 (Bolger et al., 2014) with the following options: ILLUMINACLIPTruSeq3-SE.fa:2:30:15, LEADING:3 and TRAILING:3, SLIDINGWINDOW:3:20, and MINLEN:32. Filtered reads were then aligned to the soybean reference transcriptome using TopHat2 version 2.1.1 (Kim et al., 2013). Aligned reads were then counted using HTSeq-count version 0.6.1 (Anders et al., 2015) and were filtered to be considered expressed only if they met the following criteria: (i) min raw counts of at least two to be considered active in a given line; and (ii) transcription recorded in a minimum of 25% of the population. This filtering step resulted in gene sets comprising 38,692 and 40,218 genes for the QS15524F2:F3 and QS15544RIL populations, respectively.
Linkage map construction
The linkage maps were built as described in Gélinas Bélanger et al. (2024a) (Figure 1B). Briefly, the maps were generated using QTL IciMapping version 4.2 (Meng et al., 2015) with the Kosambi mapping function. For both maps, the markers were anchored to their physical positions when ordering and the resulting linkage groups (LGs) were split when gaps exceeded 30 cM. The robustness of both linkage maps was previously demonstrated in two previous studies that aimed at mapping reproductive (Gélinas Bélanger et al., 2024a) and seed quality (Gélinas Bélanger et al., 2024b) traits by plotting the (i) genetic distance versus the physical position and (ii) the pairwise recombination fraction and LOD score (Figure 1B). In addition, the high-quality of the linkage maps was assessed by confirming the synteny between the physical and genetic positions of the markers (data not shown).
Measurement of differential gene expression
Measurement of differential gene expression was performed in the QS15524F2:F3 (OV vs MA; Supplementary Table S1A) and QS15544RIL (MD vs ‘9004’; Supplementary Table S1B) parental lines (Figure 1E). Each analysis was performed using the early-maturing parent (QS15524F2:F3, OV; QS15544RIL, MD) as the reference line. Due to low-quality data in the RNA-seq datasets in the QS15524F2:F3 parental lines, two replicates were removed each from the OV and MA samples, thus resulting in a total of three replicates per parent. Similarly, one replicate was removed from the MD and ‘9004’ samples in the QS15544RIL parental lines, resulting in a total of four replicates per parent. The expressed gene sets comprised 38,692 genes for the QS15524F2:F3 parents and 40,218 genes for the QS15544RIL parents which were filtered using the aforementioned parameters. Differentially expressed gene analysis was performed in iDEP.96 (Ge et al., 2018) using the DESeq2 function with a false discovery rate (FDR) adjusted p-value threshold fixed at 0.05 and a minimum fold change of 2.0. The normalization of the transcripts for the GO analysis and the eQTL mapping (see below) was performed using the DESeq2 R package (Love et al., 2014). Volcano plots and heatmaps were respectively generated using the online version of VolcaNoseR (Goedhart and Luijsterburg, 2020) and iDEP.96.
Gene ontology enrichment
Gene ontology (GO) enrichment was performed on the parental downregulated and upregulated gene sets using the Soybase_GOtool (https://www.soybase.org/goslimgraphic_v2/dashboard.php) as detailed in Morales et al. (2013) (Figure 1E). The Fisher test p-values obtained with the Soybase_GOtool were adjusted using the Bonferroni correction with a threshold for these corrected p-values fixed at 0.01. In Soybase, the obtained p-value is automatically multiplied by the number of scanned genes (e.g. p-value 0.003 X 4000 genes = Bonferroni corrected p-value of 12), leading to p-values that can be above 1. From this list of results, the GO terms associated with molecular functions and cellular components were manually removed using https://biodbnet-abcc.ncifcrf.gov/db/db2db.php, and only the GO terms associated with biological processes were retained. Following this step, we manually curated and retained GO terms associated with the following biological functions from Soybase (Grant et al., 2009): (i) flowering; (ii) reproduction; (iii) senescence; (iv) photosynthesis; and (v) development. This list of GO terms included a total of 162 annotations (Supplementary Table S1C) and is herein referred to as FRSPD_GO (Flowering/Reproduction/Senescence/Photosynthesis/Development). In this paper, this list was used to annotate the enriched FRSPD terms in the parental differentially expressed gene (DEG) datasets, mapped eQTL interactions, and genes found in co-expression networks (CEN).
Expression quantitative trait loci analysis
Transcriptome-wide eQTL analysis was performed on normalized transcript abundances for the 176 lines of the QS15524F2:F3 population (38,692 genes) and the 162 lines of the QS15544RIL (40,218 genes) population (Figure 1F). The mapping of eQTL was performed using a combinatorial approach which includes the use of three different algorithms: (i) Inclusive composite interval mapping (ICIM) approach implemented in QTL IciMapping version 4.2 (Meng et al., 2015); (ii) Interval mapping (IM) from QTL IciMapping version 4.2 (Meng et al., 2015); and (iii) Genome-wide compositive interval mapping (GCIM) method in the QTL.gCIMapping.GUI.v2.0.GUI package (Zhang et al., 2020). The LOD thresholds for ICIM and IM were calculated in QTL IciMapping with 1000 permutations using an α of 0.05 and a walking step of 1 cM for genome-wide scanning. To limit the computational burden (i.e. at least 1,000 permutations for 38,692 and 40,218 genes), we performed permutations on 100 randomly sampled gene transcripts (i.e. 1,000 permutations X transcripts for 100 randomly selected genes = 100,000 permutations) as performed in West et al. (2007); Wang et al. (2010, 2014), and Huang et al. (2020). Subsequently, the global permutation threshold was calculated as the 95th percentile of the representative null distribution and equaled to (i) 4.01 for ICIM in QS15544RIL; (ii) 3.99 for IM in QS15544RIL; (iii) 4.13 for ICIM in QS15524F2:F3; and (iv) 4.12 for IM in QS15524F2:F3. For GCIM, the fixed model component was chosen for the QS15544RIL population and the fixed-restricted maximum likelihood (REML) component was chosen for the QS15524F2:F3 population, both with a walking speed of 1 cM. In the QTL.gCIMapping.GUI.v2.0.GUI package, the likelihood function is only available for F2 populations and was chosen based on prior testing. For GCIM, the default LOD threshold suggested in the literature is 2.5 for QTL studies; however, the thresholds were increased to 7.5 for the QS15524F2:F3 and 4.0 for the QS15544RIL populations to reduce the noise and remove minor eQTL interactions. The contrasting LOD thresholds for the QS15524F2:F3 and QS15544RIL populations were chosen based on preliminary tests performed using the different functions implemented in the QTL.gCIMapping.GUI.v2.0.GUI package. Following the mapping of interactions with the three algorithms, all of the significant interactions were classified either as cis-acting or trans-acting. Interactions were classified as cis-acting if within 1,000,000 bp region from the transcription start site (TSS) of the studied gene, whereas interactions were considered trans-acting if identified outside this 1,000,000 bp region or on another chromosome.
To increase our confidence in the eQTL regions identified by the three methods, only signals identified by at least two methods and within 1 Mbp of each other were retained. To do so, the interactions were split between cis-acting and trans-acting, and the size of each of the mapped eQTL regions (i.e. all of the interactions identified with the three aforementioned algorithms) was manually adjusted by adding 500,000 bp both upstream and downstream of the loci. The overlapping regions were subsequently identified using the genomic peak Venn function implemented in https://www.bioinformatics.com.cn/en, a free online platform for data analysis and visualization. To compute the interactions using this software, each interaction was codified as the following: cis/trans_genename_interactingchromosome startregioninteraction endregioninteraction. For example, trans_Glyma.01G123600_GM05 40000 200000 would represent the region 40,000 – 200,000 on chromosome GM05 interacting in trans with Glyma.01G123600. The overlaps were identified using a pairwise comparison using the ICIM interactions as the reference signals in the ICIM vs. IM and ICIM vs. GCIM analyses. In addition, the IM signals were used as references in the IM vs. GCIM analysis. Trans interactions overlapping cis regions were de facto considered as cis.
Regulatory hotspot mapping
To uncover regions associated with the regulation of the expression of multiple genes, we decided to identify the hotspots involved in the modulation of a high number of trans interactions (Figure 1F). To do so, marker pairs delineating trans hotspots were qualified based on their respective (i) number of trans interactions and (ii) trans interaction density, and only those meeting both of these criteria were considered as markers flanking a hotspot region. The number of interactions was identified by summing the number of trans interactions associated with a specific pair of markers and only the pairs of markers that were above the 95th (minor hotspot) or the 99th (major hotspot) percentiles threshold of all marker pairs from that population were retained. The trans interaction density was quantified by identifying the average number of trans interactions per kbp associated with the distance between the flanking of markers. A specific marker pair was deemed significant if its density was above the 80th percentile of all of the calculated trans-interaction densities. To facilitate the reading and understanding of the paper, each of the loci presented in this article is distinguished using either F2 (QS15524F2:F3) or RIL (QS15544RIL) in front of the region’s name (e.g. F2_GM18:1,911,667-1,935,386). All of the Circos plots present in this paper were drawn using Circa V1 https://omgenomics.gumroad.com/l/circa.
Co-expression network analysis and identification of homologous genes using protein homology
Co-expression networks were built for the target genes and candidate TFs to understand their global expression pattern within the transcriptome (Figure 1G). To understand the general expression pattern of the interactions associated with a specific hotspot, we generated the pairwise Pearson correlation coefficients (PCC) for the queried genes and clustered them using the pheatmap package (https://github.com/raivokolde/pheatmap) implemented in R. Transcriptome-wide CENs (TWCENs) were also generated using the QS15524F2:F3 (38,692 genes) and QS15544RIL (40,218 genes) expression datasets. To do so, PCCs were generated using ≥ 0.85 (positive TWCEN, herein named POSTWCEN) or ≤ -0.85 (negative TWCEN, herein named NEGTWCEN) as thresholds for the expression datasets for the 176 and 162 lines for QS15524F2:F3 and QS15544RIL, respectively. The genes deemed significant based on these thresholds were then annotated using the Soybase_GOtool to identify FRSPD_GO functions. Identification of homologous genes was performed using their peptide sequence with the Blast function in Phytozome V13 (Goodstein et al., 2012), and peptide sequences exhibiting an E ≤ 1e-5 were considered homologous.
Prediction of transcription factors and identification of candidate single nucleotide polymorphisms
Following the mapping of eQTL interactions and regulatory hotspots, we predicted putative transcription factors that could be regulators for the four candidate genes (GmPRR1a, GmMDE04, GmLHCA4a, and GmCDF3) of the E8-r3 locus, a region previously identified between the GM04:41,808,599 and GM04:42,376,23 flanking markers (Figure 1H; Supplementary Table S1D) (Gélinas Bélanger et al., 2024a). To do so, we generated a list of 4,611 putative TFs (herein named TF_list4,611) (Supplementary Table S1E). The TF_list4,611 was generated by merging the genes with annotated TF functions from the PlantTFDB (Jin et al., 2017) and Soybase (Grant et al., 2009) databases. This corresponded to a total of 658 and 864 unique genes from the PlantTFDB and Soybase, respectively. In addition, another common 3,089 genes were identified in both databases. To identify the best candidate TFs present in the identified loci, we subsequently annotated each of them using the (i) differential transcript expression datasets from the parents; (ii) positive and negative co-expression network datasets; and (iii) Soybase gene ontology annotations.
In addition, we used a custom variant analysis pipeline similar to the one detailed in Gélinas Bélanger et al. (2024a) to identify putative causal mutations in the predicted TFs (Figure 1I). Prediction of deleterious effects of the SNPs within these TFs was performed using Ensembl Variant Effect Predictor (VEP) with Glycine_max_v2.1 (McLaren et al., 2016). Mutations predicted as having moderate or high consequences on the protein structure or located in the 3’UTR/5’UTR were retained, whereas the others were removed from the dataset. The putative effects of the identified missense mutations were then predicted using Sorting Intolerant From Tolerant 4G (SIFT4g) (Ng and Henikoff, 2003; Kumar et al., 2009). To predict the effects of these mutations, we generated a database using the annotations of G. max Wm82.a2.v1 from EnsemblPlants and the SIFT4G_Create_Genomic_DB guidelines https://github.com/pauline-ng/SIFT4G_Create_Genomic_DB. Single nucleotide polymorphisms with SIFT scores ≥ 0.05 were considered as tolerated and those < 0.05 were considered as deleterious. Following the identification of the candidate SNPs, we verified the genotypes associated with them using the GmHapMap dataset and retained only the SNPs that were present in a single parental line.
Results
Linkage map construction and differential gene expression the parental lines
In our previous QTL study (Gélinas Bélanger et al., 2024a), 541,106,451 (QS15524F2:F3) and 286,844,986 (QS15544RIL) unique single-end reads were generated during the sequencing step of the full mapping populations. After filtering, two linkage maps were generated from 1,613 (QS15524F2:F3; Supplementary Tables S1F, G) and 2,746 (QS15544RIL; Supplementary Tables S1F, H) high-quality GBS-derived SNP markers. To validate our choice of experimental conditions for both of our populations (i.e. RNA collected from the middle leaflet of the trifoliate leaf 4 hours after sunrise at the V4 leaf stage), we performed a differential gene expression analysis in both pairs of parental lines. Based on this analysis, we identified 10,216 DEGs (4,953 up-regulated genes and 5,263 down-regulated genes in OV) in the QS15524F2:F3 parents (Supplementary Figures S1A, B; Supplementary Table S1I) and 1,430 DEGs (438 upregulated genes and 992 down-regulated genes in MD) in the QS15544RIL parents (Supplementary Figures S1C, D; Supplementary Table S1J). To find an explanation to the large difference of DEGs between each population, we inspected the pedigrees of QS15524F2:F3 and QS15544RIL but did not find any obvious factors (e.g., a cross using a very exotic line) that would have caused this discrepancy (data not shown). Overall, we found that two of our candidate genes, GmCDF3 and GmMDE04, were upregulated in the OV parental line of the QS15524F2:F3 population. In addition, we found that many FRSPD_GO terms were significantly enriched for both populations (e.g. ‘Regulation of Flower Development’ in the QS15524F2:F3 parents, Bonferroni corrected p-value of 3.05E-76), thus indicating a large difference in the abundance of transcripts of FRSPD genes in the parental lines of both populations (Supplementary Table S1K).
Mapping of eQTL interactions
Subsequently, the linkage maps were used to perform genome-wide mapping of eQTL interactions for the QS15524F2:F3 (38,693 genes) and QS15544RIL (40,223 genes) populations using a combinatorial approach based on the IM, ICIM, and GCIM algorithms populations using acombinatorial approach based on the IM, ICIM, and GCIMalgorithms (Supplementary Table S1L; S1m). In the QS15524F2:F3 population, the ICIM (4,735 trans/17 cis), IM (1,714 trans/10 cis), and GCIM (10,906 trans/32 cis) methods identified a varying number of interactions (Table 1). The same analysis was performed with the QS15544RIL population, with IM (17,375 trans/5,337 cis) having the highest number of interactions followed by ICIM (7,941 trans/2,862 cis) and then GCIM (4,418 trans/2,375 cis) (Table 1). To reduce the number of regions for further analyses, we decided to retain only interactions that were identified by at least two algorithms and which were overlapping or within a 1,000,000 bp distance from each other (Table 1; Supplementary Table S1N, S1o, S1p). This merging step reduced the number of interactions to 2,218 trans (2,061 genes)/7 cis (7 genes) (Figure 2A) and 4,073 trans (2,842 genes)/3,083 cis (2,418 genes) (Figure 3A) for the QS15524F2:F3 and QS15544RIL populations, respectively (Supplementary Table S1Q). Using our combinatorial approach, we identified that the trans interactions were regulated by a total of 280 regions covering a total of ≈ 212.19 Mbp in the QS15524F2:F3 population and 1,213 regions covering a total of ≈ 588.03 Mbp in the QS15544RIL population. The number of interactions per region was between 1 and 507 with a density between 3.67E-5 and 1,481 interactions/kbp for the QS15524F2:F3 population. For the QS15544RIL population, the number of interactions per region was between 1 and 450 with a density between 3.09E-7 and 100 interactions/kbp.
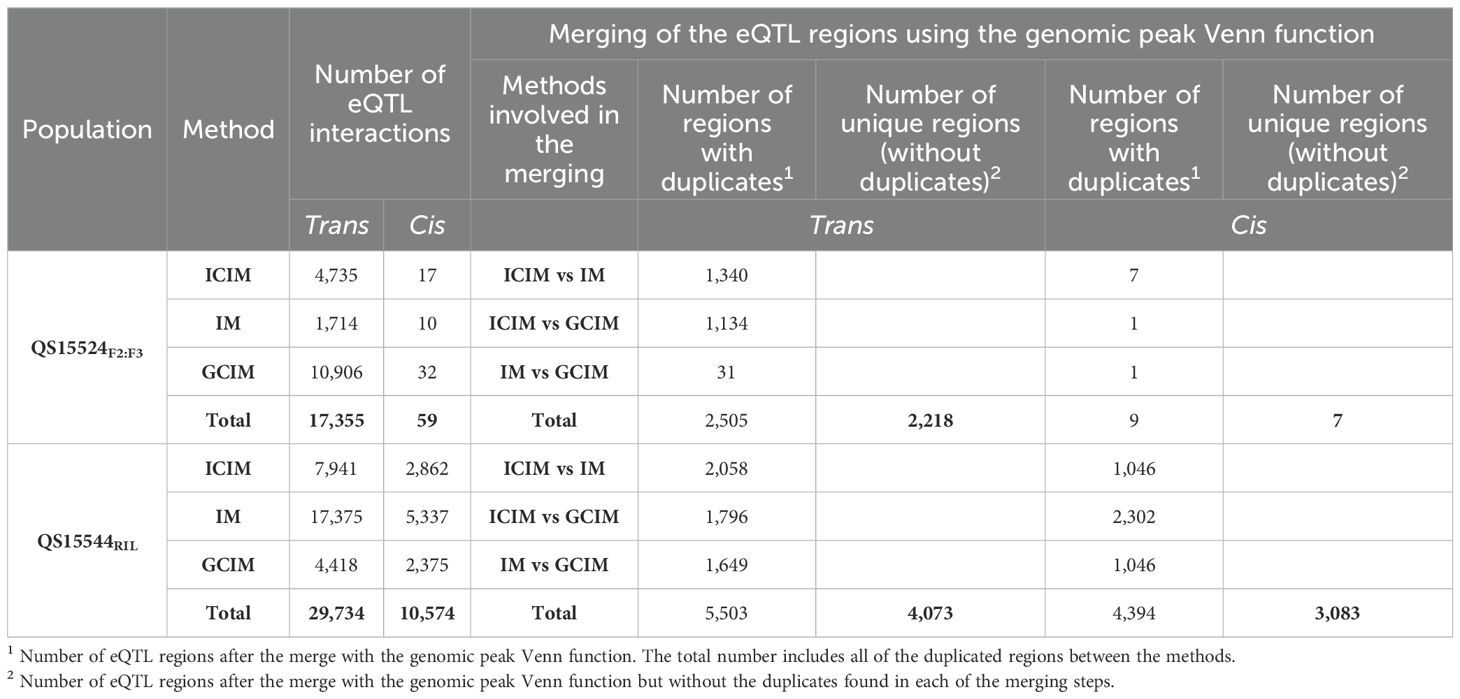
Table 1. Number of eQTL interactions and eQTL regions before and after the merge using the genomic peak Venn function.
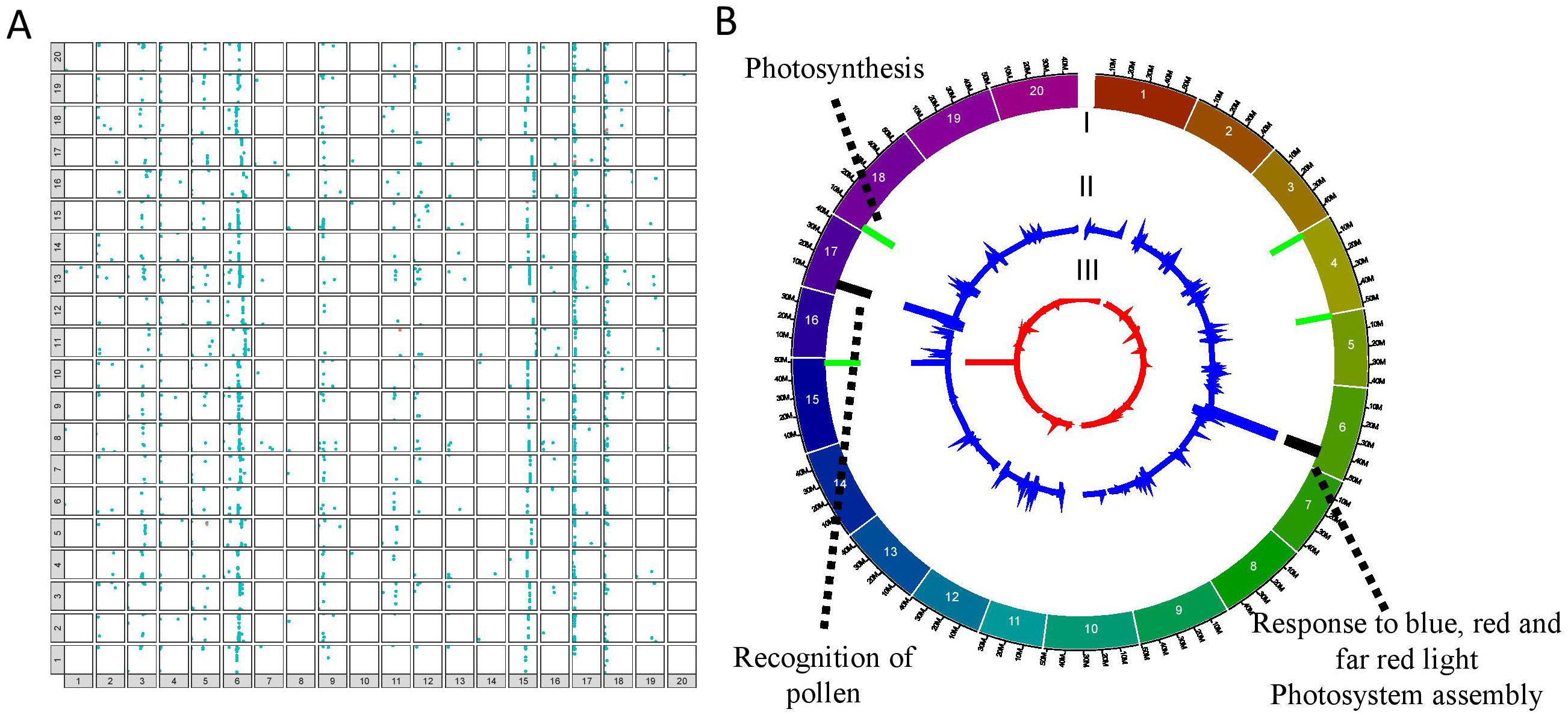
Figure 2. Mapping of the eQTL interactions and regulatory major hotspots using the combinatorial approach in the QS15524F2:F3 population. (A) Identification of the eQTL interactions found with at least two mapping algorithms in the QS15524F2:F3 population. The X-axis represents regulating regions, whereas the Y-axis represents the locations of the target genes. Cis interactions and trans interactions are respectively illustrated as the orange and light blue dots. (B) Mapping of the regulatory hotspots. Level I, locations of the hotspots. Major and minor hotspots are respectively indicated using black and green rectangles. Level II, number of eQTL interactions per marker. Level III, eQTL density per marker. The dotted lines indicate the FRSPD_GO functions significantly associated with the hotspots (Bonferroni p-value, 0.01).
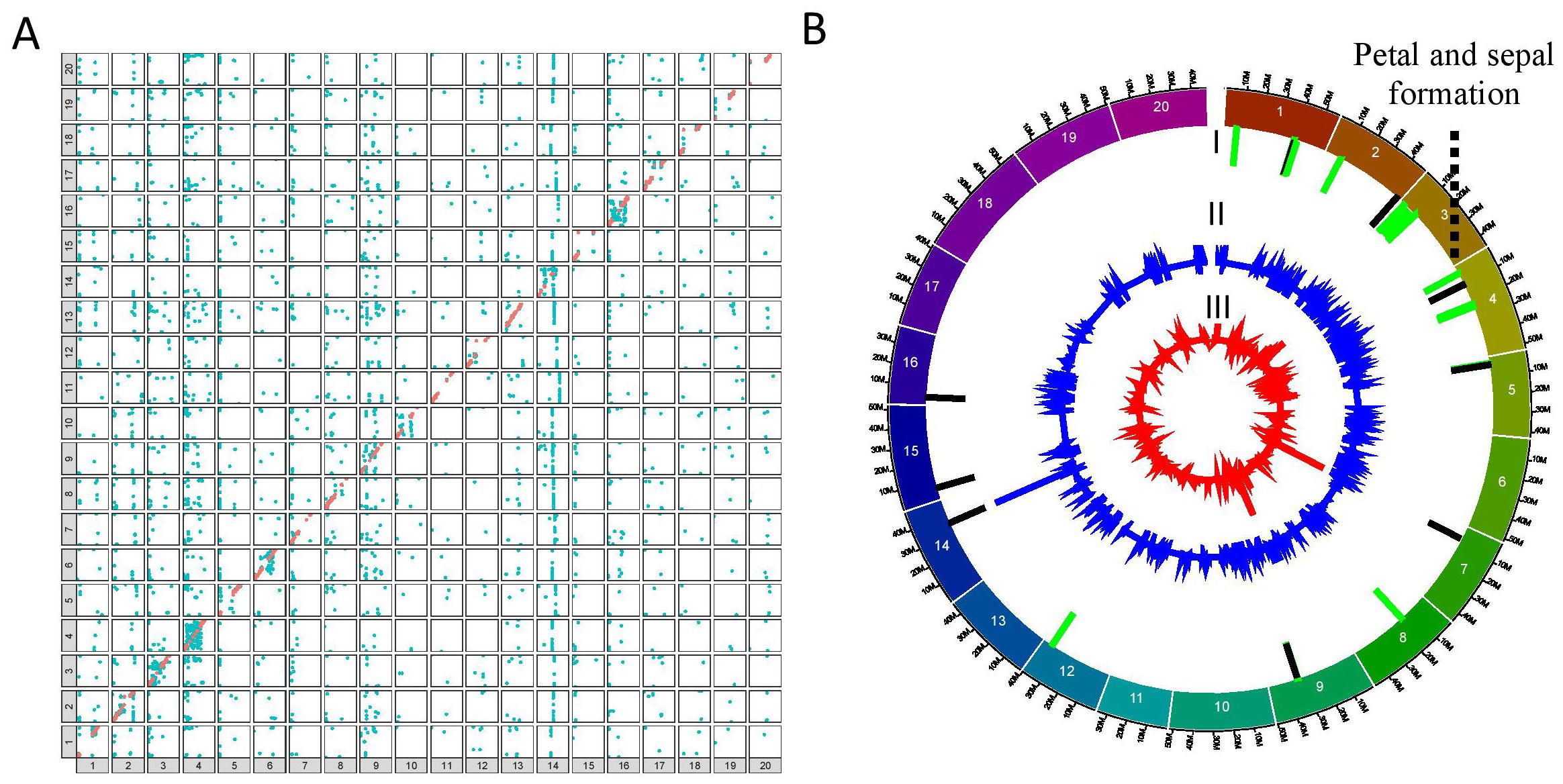
Figure 3. Mapping of the eQTL interactions and regulatory major hotspots using the combinatorial approach in the QS15544RIL population. (A) Identification of the eQTL interactions found with at least two mapping algorithms in the QS15544RIL population. The X-axis represents regulating regions, whereas the Y-axis represents the locations of the target genes. Cis interactions and trans interactions are respectively illustrated as the orange and light blue dots. (B) Mapping of the regulatory hotspots. Level I, locations of the hotspots. Major and minor hotspots are respectively indicated using black and green rectangles. Level II, number of eQTL interactions per marker. Level III, eQTL density per marker. The dotted lines indicate the FRSPD_GO functions significantly associated with the hotspots (Bonferroni p-value, 0.01).
Identification and characterization of the hotspots and regions associated with E8-r3
To identify important regulatory regions controlled by or controlling the E8-r3 region, we began by classifying our trans eQTL regions into minor regions or hotspots (either minor or major hotspots) to identify the most promising regions (Supplementary Table S1R). To do so, we retrieved all of the regions above the 95th (minor hotspot) or 99th (major hotspot) percentiles for the number of interactions and the 80th percentile for the eQTL interaction density to identify either minor or major hotspots (Table 2). For the QS15524F2:F3 population, the thresholds corresponded to 29 to 259 interactions for minor hotspots or ≥ 260 interactions for major hotspots with densities ≥ 0.09 interactions/kbp. For the QS15544RIL population, the thresholds corresponded to 9 to 16 interactions for minor hotspots or ≥ 17 interactions for major hotspots with densities ≥ 0.17 interactions/kbp. Using these thresholds, 8 hotspots (2 major and 6 minor) were identified in the QS15524F2:F3 population (i.e. 2.85% of the total number of identified regions) (Figure 2B; Table 2). Similarly, 34 hotspots (9 major and 25 minor) were identified in the QS15544RIL population (i.e. 1.23% of the total number of identified regions), with a large number of hotspots located near each other on chromosomes GM01, GM03, GM04, GM05 and GM09 (Figure 3B; Table 2). Following the identification of the hotspots, we noticed that several had markers in common, suggesting that these regions might be regulated by one or several common loci. This included the (i) F2_GM18:1,434,182-1,911,667 and F2_GM18:1,911,667-1,935,386 and the (ii) F2_GM15:49,385,092-49,442,075 and F2_GM15:49,442,075-49,442,237 regions of the QS15524F2:F3 population. Due to their close location, we merged the neighboring loci into two merged regions named F2_GM18:1,434,182-1,935,386 and F2_GM15:49,385,092-49,442,237 for the subsequent analyses. To characterize the hotspots, we subsequently performed a GO enrichment analysis on each of them and observed that four (i.e. three in QS15524F2:F3 and one in QS15544RIL) were significantly enriched with terms associated with FRSPD functions (Figures 2B, 3B; Supplementary Table S1S).
As we were interested in understanding the role of the E8-r3 region and its interactions, we investigated whether the identified eQTL minor regions and eQTL hotspots interacted in trans with our four candidate genes (GmCDF3, GmPPR1a, GmLHCA4a, and GmMDE04) (Table 3). On the whole, we identified that the F2_GM18:1,434,182-1,935,386 hotspot was involved in the regulation of GmLHCA4a. We also detected that GmPPR1a was regulated by the F2_GM15:49,385,092-49,442,237 hotspot as well as the RIL_GM04:17,227,512-20,251,662, RIL_GM04:31,408,946-31,525,671 and RIL_GM13:37,289,785-38,620,690 minor regions. For GmMDE04, we identified one interaction with the F2_GM15:49,385,092-49,442,237 hotspot and one interaction with the RIL_GM04:17,227,512-20,251,662 minor region. No interactions were observed for GmCDF3, and as such, this gene was not investigated further. In addition to the interactions with the E8-r3 candidate genes, we also identified several trans regulatory events with five additional genes (Glyma.04G168100, Glyma.04G168000, Glyma.04G169300, Glyma.04G168200, and Glyma.04G169100) found in the E8-r3 locus, including several loci found on GM04 (Supplementary Table S1Q).
The F2_GM18:1,434,182-1,935,386 hotspot regulates GmLHCA4a and several homologous genes
To further understand the role of the F2_GM18:1,434,182-1,935,386 hotspot, we investigated to understand the specific FRSPD_GO functions of the 91 interactions (90 genes1). In addition, we used the TF_list4,611 to identify candidate transcription factors and found three (Glyma.18G020900, Glyma.18G025600, and Glyma.18G025800) that were located within or close to this hotspot (Figure 4A). To understand the co-expression patterns between the 90 target genes and three candidate transcription factors, we generated a CEN using pairwise PCCs between these 93 genes (Figure 4B).
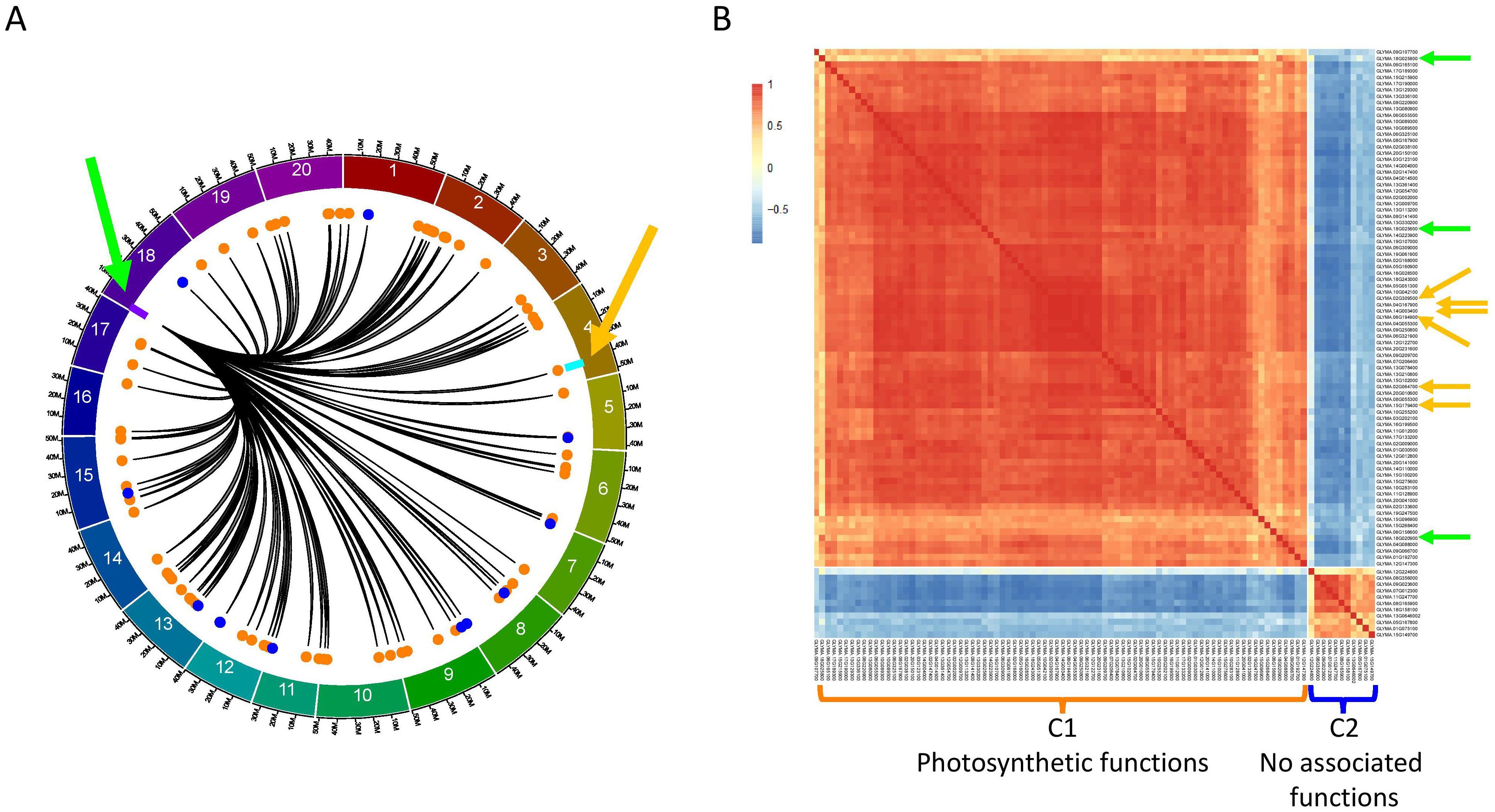
Figure 4. Characterization of the F2_GM18:1,434,182-1,935,386 hotspot and its interaction with GmLHCA4a in the QS15524F2:F3 population. (A) Identification of the 91 trans interactions (90 genes) associated with the F2_GM18:1,434,182-1,935,386 hotspot. Colored dots represent the C1 (orange color; 79 genes) and C2 (royal blue color; 11 genes) clusters depicted in panel (B) Orange arrow, location of the candidate gene GmLHCA4a and the E8-r3 locus (light blue rectangle). Green arrow, location of the three candidate TFs (Glyma.18G020900, Glyma.18G025600, and Glyma.18G025800) and the F2_GM18:1,434,182-1,935,386 hotspot (purple rectangle). (B) Co-expression network with the 90 target genes and three candidate TFs. Orange arrows, location of the six LHCA genes (GmLHCA4a, Glyma.02G064700/GmLHCA1, Glyma.02G309500/GmLHCA3a, Glyma.06G194900/GmLHCA4b, Glyma.14G003400/GmLHCA3b, and Glyma.15G179400/GmLHCA6). Green arrows, location of the three candidate TFs. The C1 cluster is significantly associated with photosynthetic functions, whereas C2 is not enriched with any FRSPD_GO terms.
By doing so, we observed that 79 of the target genes, including GmLHCA4a, exhibited a similar co-expression pattern and were grouped as such into the F2_GM18:1,434,182-1,935,386_C1 cluster, a group specifically enriched with terms related to photosynthesis and response to light stimulus. Another group comprising 11 target genes was grouped in the F2_GM18:1,434,182-1,935,386_C2 cluster, a group without significantly enriched functions. We found that the three candidate TFs that were identified for the F2_GM18:1,434,182-1,935,386 hotspot all clustered in the C1 group, with Glyma.18G025600 exhibiting the highest co-expression values with GmLHCA4a. Interestingly, we also discovered that the C1 cluster comprised a total of six LHCA homologs annotated with photosynthesis, response to light, photosystem I, and chlorophyll-binding functions in Soybase: (i) Glyma.04G167900/GmLHCA4a (our candidate gene); (ii) Glyma.02G064700/GmLHCA1; (iii) Glyma.02G309500/GmLHCA3a; (iv) Glyma.06G194900/GmLHCA4b; (v) Glyma.14G003400/GmLHCA3b; and (vi) Glyma.15G179400/GmLHCA6.
Functional investigation and variant analysis of the candidate transcription factors regulating LHCA homologs
After identifying the three candidate TFs, we found that these genes were not annotated with FRSPD functions in Soybase. To gain further insights about them, we investigated the TWCENs between these genes and the 38,692 genes dataset from the QS15524F2:F3 population (Figure 5A; Supplementary Table S1T). Using a PCC threshold of ≥ 0.85 (POSTWCEN), we found that the POSTWCEN of Glyma.18G020900, Glyma.18G025600 and Glyma.18G025800 respectively comprised 2,230, 527 and 136 genes. For these three candidate TFs, we also constructed the NEGTWCEN using a PCC threshold of ≤ -0.85 and discovered that the NEGTWCEN of Glyma.18G020900, Glyma.18G025600 and Glyma.18G025800 respectively comprised 444, 1,849 and 0 genes. The genes found in POSTWCEN and NEGTWCEN of Glyma.18G025600 were significantly enriched with functions associated with flower development, photosynthesis, and chlorophyll-binding, whereas the POSTWCEN and NEGTWCEN of Glyma.18G020900 were less strongly associated with these functions (Figure 5B; Supplementary Table S1U). For Glyma.18G025800, we found that its POSTWCEN and NEGTWCEN were not significantly enriched with any GO terms.
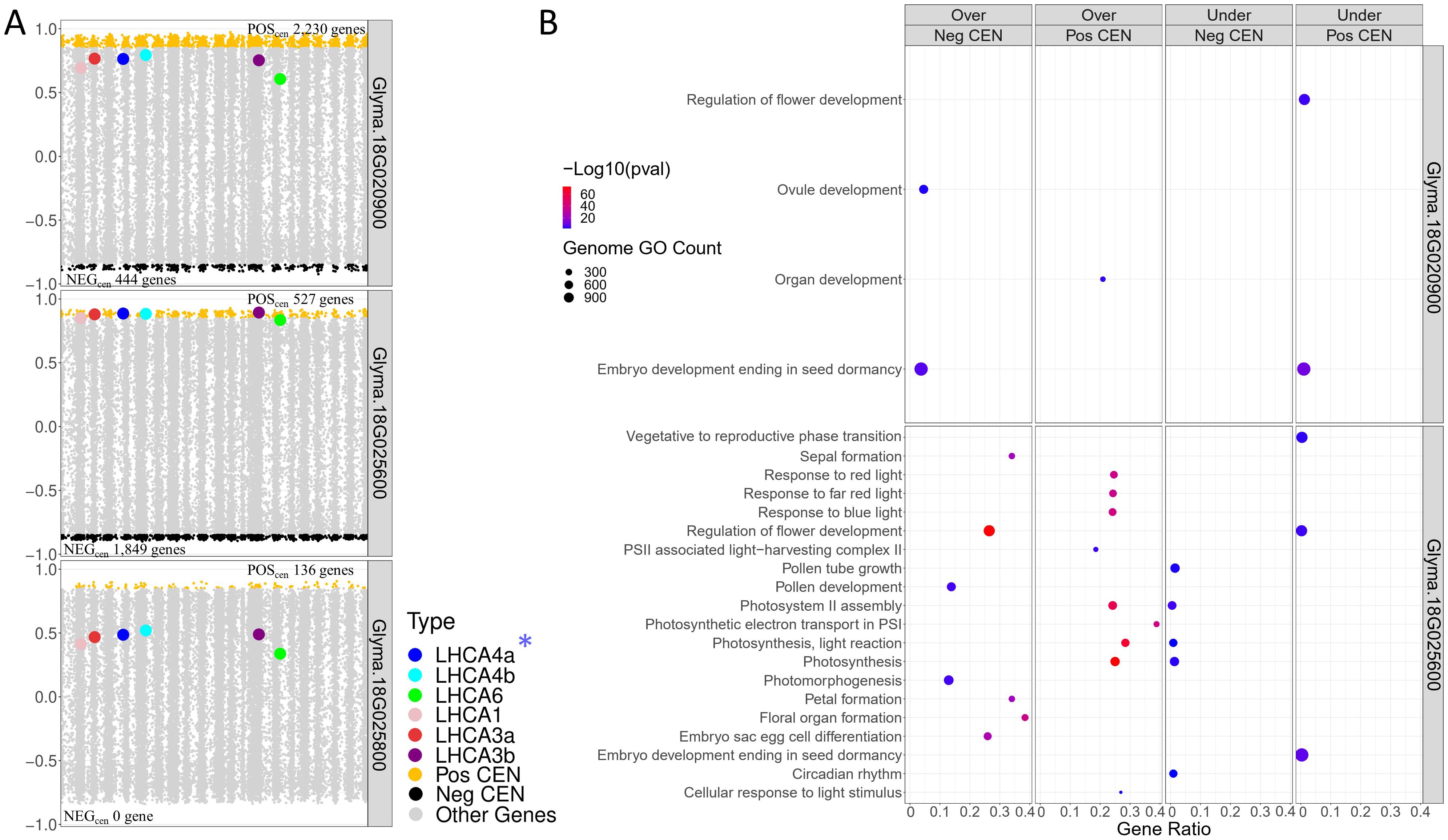
Figure 5. Transcriptome-wide co-expression network for the three candidate TFs of the F2_GM18_1,434,182-1,935,386 hotspot. (A) Positive and negative TWCENs for the three candidate TFs using PCC thresholds of ≥ 0.85 (POSTWCEN) and ≤ -0.85 (NEGTWCEN). As shown in the panel, Glyma.18G020900 exhibits the largest POSTWCEN (2,230 genes) followed by Glyma.18G025600 (527 genes), and Glyma.18G025800 (136 genes). For the NEGTWCEN, Glyma.18G025600 (1,849 genes) displays the largest network, whereas the network of Glyma.18G020900 is smaller (444 genes). No gene was found for the NEGTWCEN of Glyma.18G025800. The highest level of co-expression between the LHCA homologs and a candidate TF was achieved with Glyma.18G025600 with a mean PCC of 0.87 for the six homologs. In comparison, the mean PCC of Glyma.18G020900 and Glyma.18G025800 for these six homologs were 0.73 and 0.45, respectively. GmLHCA4a, the candidate target gene for the E8-r3 locus, is highlighted with an asterisk. (B) Functional annotation of the POSTWCEN and NEGTWCEN of each candidate TF. The POSTWCEN and NEGTWCEN of the Glyma.18G025600 gene were significantly enriched with a large number of FRSPD genes associated with photosynthetic properties such as light response. Only gene annotations that are either over-represented (i.e., “Over” facet) or under-represented (i.e., “Under” facet) are displayed in the figure. Non-FRSPD annotations were not displayed for visualization purposes, but are available in Supplementary Table S1U.
To further understand the putative roles of the three candidate TFs located in the F2_GM18:1,434,182-1,935,386_C1 cluster, we investigated their expression profiles, as well as the presence of mutations, in the parental lines. Based on our observations, Glyma.18G020900 (Fold change, 3.66; FDR adjusted p-value, 1.93E-08) and Glyma.18G025800 (Fold change, 2.17; FDR adjusted p-value, 0.047), were found to be significantly upregulated in ‘OAC Vision’, whereas Glyma.18G025600 was not differentially expressed (Fold change, 1.45; FDR adjusted p-value, 0.07) (Supplementary Figure S2; Supplementary Table S1I). Deeper investigations using our candidate SNP identification pipeline led to the identification of two SNPs in Glyma.18G025600, but none of the other candidate TFs (Table 4). Overall, the presence of variants in the 3’UTR of Glyma.18G025600 but not in the other candidates, the high co-expression values between this candidate and all of the LHCA homologs, and the FRSPD functions associated with its POSTWCEN and NEGTWCEN suggest that Glyma.18G025600 is the most likely candidate for the F2_GM18:1,434,182-1,935,386 hotspot.
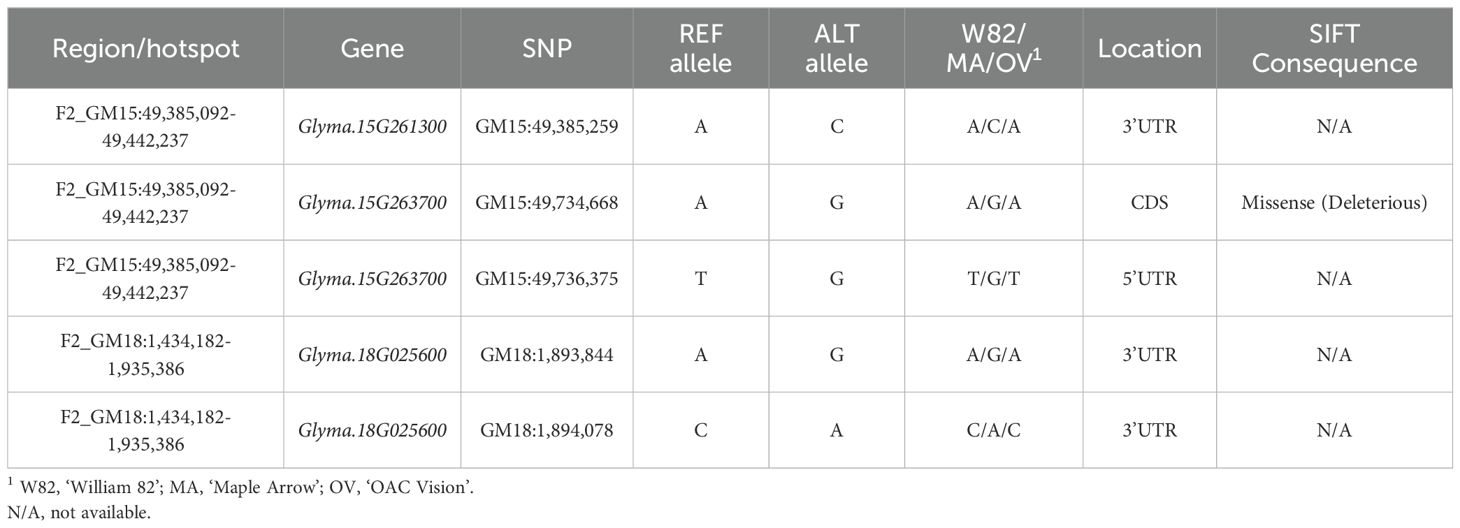
Table 4. Single nucleotide polymorphisms for the candidate transcription factors of the QS15524F2:F3 population.
The F2_GM15:49,385,092-49,442,237 hotspot regulates GmPRR1 and GmMDE homologs
In addition to the regulation of GmLHCA4a, we also identified several regions regulating two E8-r3 candidate genes, GmPRR1a, previously identified in Gélinas Bélanger et al. (2024a), and GmMDE04, proposed by Escamilla et al. (2024). As previously mentioned, both genes were found to be regulated by the F2_GM15:49,385,092-49,442,237 hotspot (Figure 6A). To further investigate the networks interacting with this hotspot, we generated a CEN comprising 285 genes based on 293 trans interactions (53 from F2_GM15:49,385,092-49,442,075 and 240 from F2_GM15:49,442,075-49,442,2372). Along, we used the TF_list4,611 to identify candidate TFs and found two, Glyma.15G261300 and Glyma.15G263700, that were located within or close to the region. Although the Glyma.15G263700 TF was not found within the F2_GM15:49,385,092-49,442,237 hotspot, it was still included in the analysis due to its close proximity with this region (< 300 kbp). Subsequently, we generated a CEN with the 285 target genes along with the 2 candidate TFs (Figure 6B) and observed the formation of two separate co-expression clusters: F2_GM15:49,385,092-49,442,237_C1 (184 target genes and one candidate TF, Glyma.15G261300) and F2_GM15:49,385,092-49,442,237_C2 (101 target genes and one candidate TF, Glyma.15G263700). Although no specific function was found to be significantly associated with the 184 target genes of the F2_GM15:49,385,092-49,442,237_C1 cluster, this cluster contained GmPRR1a and two of its homologs (Glyma.17G102200/PRR1d and Glyma.07G171200/RESPONSE REGULATOR 2/ARR2). In addition, we identified GmMDE04 and two homologs, Glyma.13G052100 (GmMDE13) and Glyma.19G034600 (GmMDE19), in the F2_GM15:49,385,092-49,442,237_C2 cluster, a group enriched with an oxidative photosynthetic carbon pathway function. Interestingly, one additional MDE homolog, GmMDE17 (Glyma.17G081200), was found to be regulated by the F2_GM15:49,385,092-49,442,237 hotspot with ICIM (LOD, 4.75; PVE, 8.56%), but none of the two other software (Supplementary Table S1L).
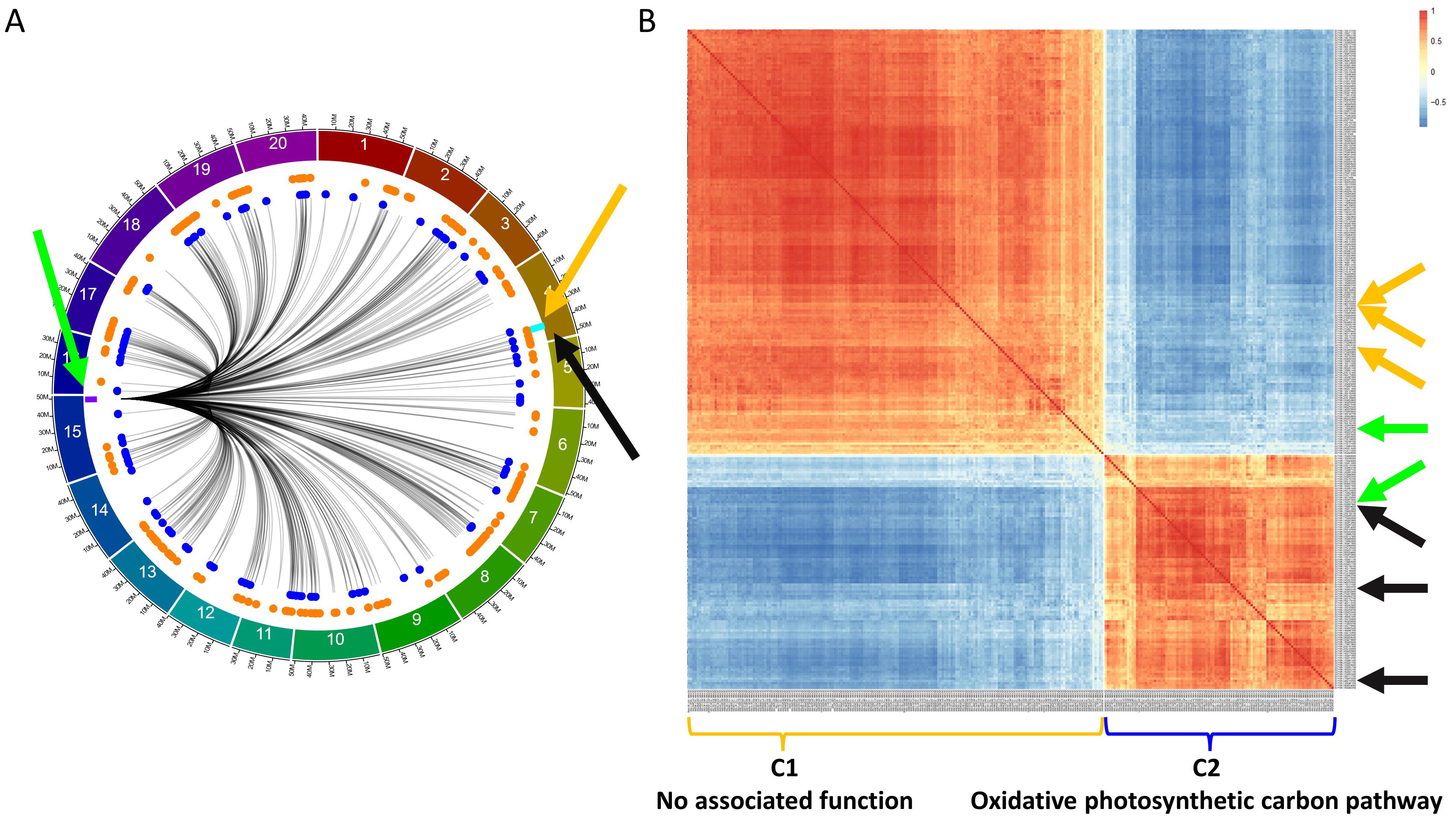
Figure 6. Characterization of the F2_GM15:49,385,092-49,442,237 hotspot and its interaction with GmPRR1a, GmMDE04, and their homologs in the QS15524F2:F3 population. (A) Identification of 293 trans interactions (285 genes) with the F2_GM15:49,385,092-49,442,237 hotspot. Green arrow, location of the two candidate TFs (Glyma.15G261300 and Glyma.15G263700) and F2_GM15:49,385,092-49,442,237 hotspot (purple rectangle). The locations of GmPRR1a, GmMDE04, and the E8-r3 locus are respectively indicated by the orange arrow, black arrow, and light blue rectangle. Orange and royal blue dots respectively represent the genes located in the C1 and C2 clusters. (B) Co-expression network with the 285 genes and the two candidate TFs. Orange arrows, location of GmPRR1a, and two PRR homologs (GmPRR1d and GmARR2). Black arrows, location of GmMDE04, and two MDE homologs (GmMDE13 and GmMDE19). Green arrows, location of the candidate TFs. The target genes and candidate TF found in the C1 cluster are indicated with the orange bracket, whereas those found in the C2 cluster are indicated with the royal blue bracket. Based on a functional enrichment analysis, the C2 cluster is associated with the term ‘oxidative photosynthetic carbon pathway’, whereas C1 is not associated with any terms.
Functional investigation and variant analysis of the candidate transcription factors regulating PRR and MDE homologs
Following the building of the CEN, we generated TWCENs for both candidate TFs of the F2_GM15:49,385,092-49,442,075 hotspot (Supplementary Table S1T). Using a PCC threshold of ≤ -0.85, we found that the NEGTWCEN of Glyma.15G263700 was large and comprised 1,284 genes, whereas Glyma.15G261300 had none (Figure 7A). Nothing conclusive was found for the POSTWCEN of both candidates as the POSTWCEN of Glyma.15G263700 comprised only 21 genes and Glyma.15G261300 had none. Subsequently, we performed a GO enrichment analysis on the NEGTWCEN of Glyma.15G263700 and discovered that it was significantly enriched with various FRSPD terms associated with flowering, floral organ formation, and photomorphogenesis (Figure 7B; Supplementary Table S1U). This result is coherent with the fact that the Glyma.15G263700 candidate TF is annotated with terms related to flower development in Soybase, whereas Glyma.15G261300 is not annotated with FRSPD functions. To gain insights regarding the putative roles of these two candidate TFs, we investigated their expression profiles, as well as the presence of mutations, in the parental lines. In the QS15524F2:F3 parents, Glyma.15G263700 (Fold change, 2.60; FDR adjusted p-value, 5.15E-04) was differentially expressed, but not Glyma.15G261300 (Fold change, 1.90; FDR adjusted p-value, 0.03) (Supplementary Figure S2; Supplementary Table S1I). Based on our variant analysis pipeline, we discovered mutations in both genes, with Glyma.15G263700 displaying a missense mutation predicted to be deleterious by the SIFT algorithm and Glyma.15G261300 having a 3’UTR variant at position GM15:49,385,259 (Table 4).
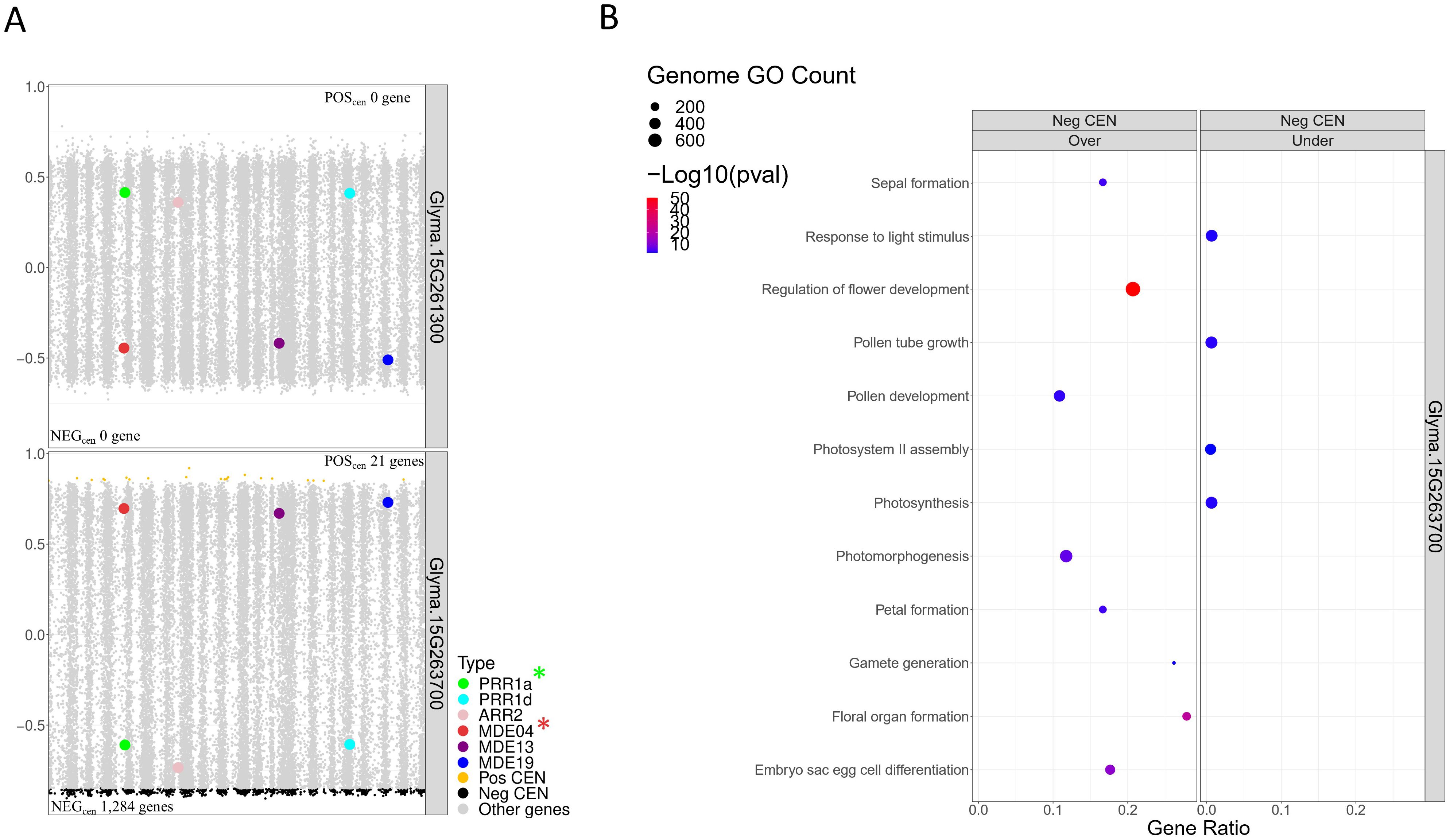
Figure 7. Transcriptome-wide co-expression network for the candidate TFs of the F2_GM15:49,385,092-49,442,237 hotspot. (A) Positive and negative TWCENs for the two candidate TFs using PCC thresholds of ≥ 0.85 (POSTWCEN) and ≤ -0.85 (NEGTWCEN). As shown in the panel, only Glyma.15G263700 displayed a large NEGTWCEN (1,284 genes). The NEGTWCEN of Glyma.15G261300 (0 genes) and the POSTWCEN of both candidates (Glyma.15G263700, 21 genes; Glyma.15G261300, 0 genes) were small. The candidate target genes GmPRR1a and GmMDE04 are highlighted with asterisks. (B) Functional annotation of the NEGTWCEN of Glyma.15G263700. This NEGTWCEN is strongly enriched with terms associated with flowering and response to light functions. Only gene annotations that are either over-represented (i.e., “Over” facet) or under-represented (i.e., “Under” facet) are displayed in the figure. Non-FRSPD annotations were not displayed for visualization purposes, but are available in Supplementary Table S1U.
GmPRR1 and GmMDE homologs are regulated by the same minor regions
Following these discoveries from the F2_GM15:49,385,092-49,442,237 hotspot (Figure 8A), we investigated further to determine whether similar co-regulation events could be observed for minor regions interacting in trans with GmPRR and GmMDE homologs. On the whole, we identified three different minor regions in the QS15544RIL population that were interacting with three GmPRR (GmPRR1a, GmPPR1d, and Glyma.05G025000/GmPRR4), and two GmMDE (GmMDE04, and Glyma.06G205800/GmMDE06) homologous genes: (i) RIL_GM04:17,227,512-20,251,662 (Figure 8B); (ii) RIL_GM04:31,408,946-31,525,671 (Figure 8C); and (iv) RIL_GM13:37,289,785-38,620,690 (Figure 8D). Interestingly, GmPPR1d was found to be regulated by a region located between markers GM04:22,010,259 and GM04:26,441,718 which is adjacent to the minor region RIL_GM04:17,227,512-20,251,662 regulating GmPRR1a and GmMDE04 (Supplementary Table S1Q). For each of the regions, the number of candidate TFs ranged between 1 (for RIL_GM04:31,408,946-31,525,671) to 6 (for RIL_GM13:37,289,785-38,620,690) (Supplementary Table S1V). We performed the same analyses (i.e. expression analysis, TWCEN, and variant analysis pipeline) for these minor regions than for the F2_GM18:1,434,182-1,935,386 and F2_GM15:49,385,092-49,442,237 hotspots (Supplementary Table S1V). Overall, two (out of 11) candidates were annotated with FRSPD terms and none of them were found to be differentially expressed in the parental lines due to FDR-adjusted p-values that were above the threshold. We constructed TWCENs for all of the candidates but only Glyma.04G135400 was found to have POSTWCEN (446 genes) and NEGTWCEN (445 genes) that were significantly enriched with GO terms including the ‘Phytochrome binding’ term (Supplementary Table S1U). Using our custom variant analysis pipeline, we found a total of five mutations in two different candidates (Glyma.04G135400 and Glyma.13G285400) (Table 5). Based on these observations, we think that Glyma.04G135400 is the best candidate for RIL_GM04:17,227,512-20,251,662. Still, we think that more research on neighboring candidate TFs outside of the RIL_GM04:31,408,946-31,525,671 and RIL_GM13:37,289,785-38,620,690 needs to be performed to identify better candidates.
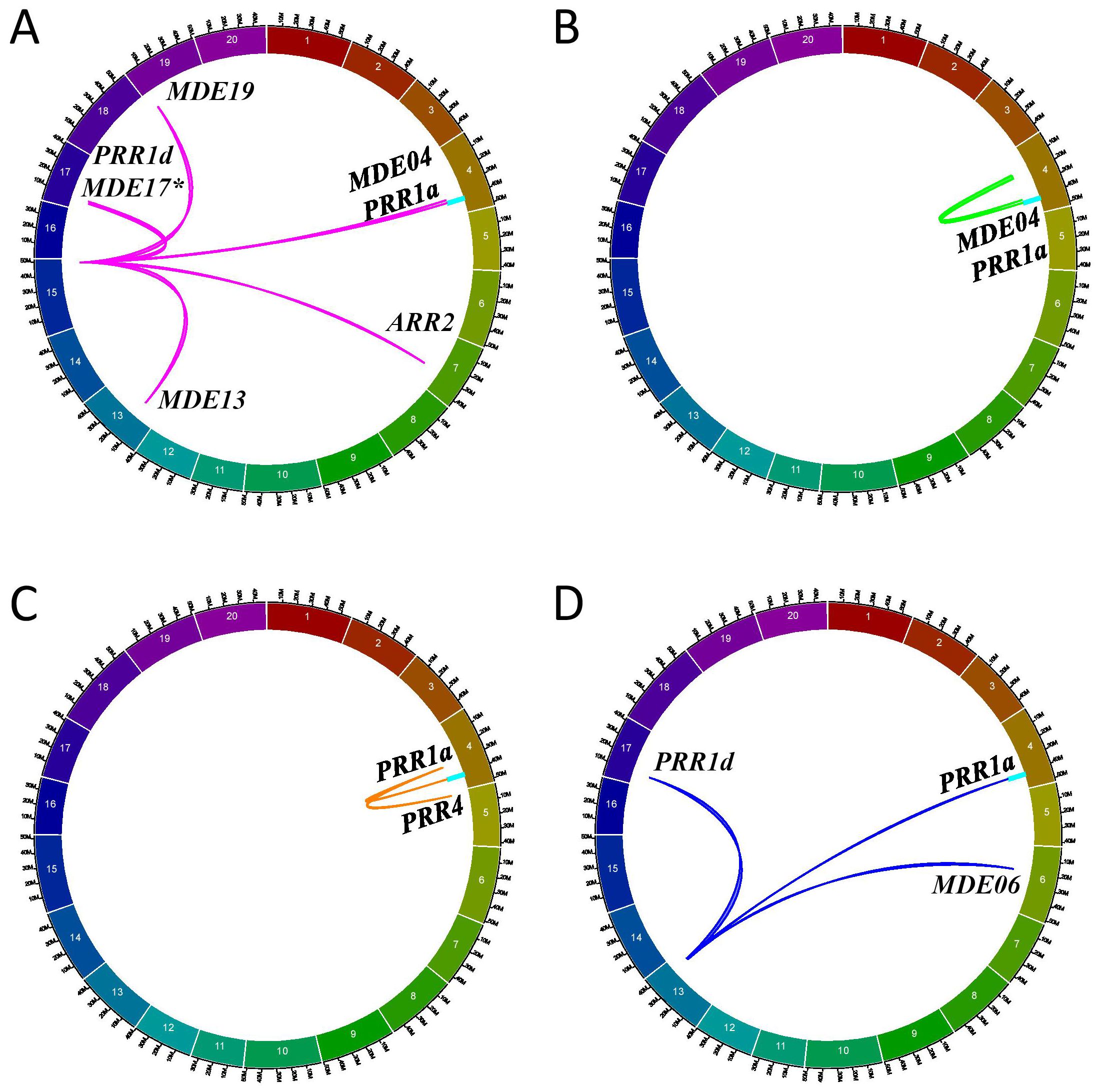
Figure 8. Minor regions regulating the PRR and MDE homologs in the QS1544RIL population. Circos plots illustrating the interactions between the F2_GM15:49,385,092-49,442,237 (A), RIL_GM04:17,227,512-20,251,662 (B), RIL_GM04:31,389,583-31,525,671 (C), and RIL_GM13:37,289,785-38,620,690 (D) regions and the different PRR and MDE homologs, including the candidate target genes GmPRR1a and GmMDE04 that are located in the E8-r3 locus (light blue rectangle). The F2_GM15:49,385,092-49,442,237 is regulating an additional MDE homolog, GmMDE17. The asterisk denotes that this additional homolog has been mapped with only one algorithm (ICIM) instead of two like all the other interactions.
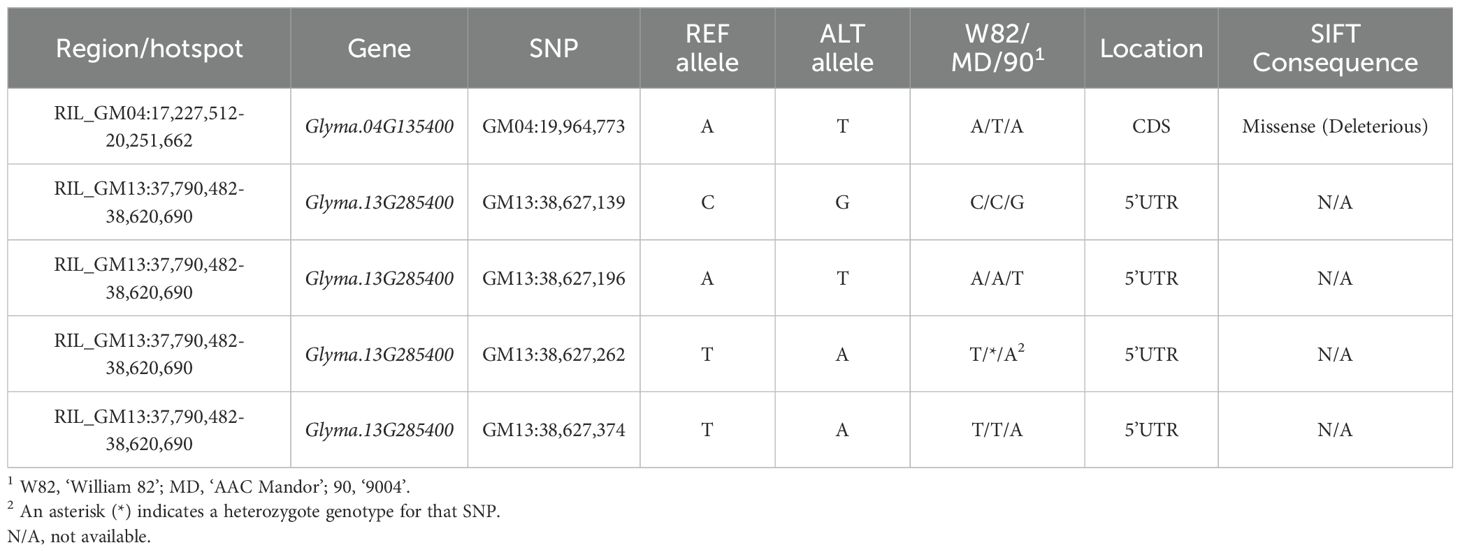
Table 5. Single nucleotide polymorphisms for the candidate transcription factors of the QS15544RIL population.
Discussion
The F2_GM18:1,434,182-1,935,386 hotspot is a hub for the coordinated regulation of the light response and photosynthetic mechanisms
Photosystem I (PSI) is located in the thylakoid membrane and is a multiprotein complex that plays a crucial role in oxygenic photosynthesis by oxidizing plastocyanin and reducing ferredoxin (Sláma et al., 2023). PSI is divided into the core complex and the outer antenna complexes (also known as Light-Harvesting Complex I; LHCI). The role of the LHCI is to harvest light and transfer the excitation energy of the electrons to the reaction center. The antenna of the Light-Harvesting Complex I comprises four subunits which are the products of Lhca1-4 genes in Arabidopsis. We previously demonstrated that the E8-r3 region (GM04:41,808,599-42,376,237) regulates the number of days to maturity under a constant short-day photoperiod in both QS15524F2:F3 and QS15544RIL. Based on these observations, we proposed the Glyma.04G167900 (GmLHCA4a) gene as a potential candidate for this region using a candidate SNP analysis (Gélinas Bélanger et al., 2024a). In previous studies, GmLHCA4a has been identified as a candidate for the q4–2 locus regulating leaf-related traits (i.e. leaf size and shape) and chlorophyll content (Yu et al., 2020). Furthermore, GmLHCA4a has been suggested to be involved in the number of days to flowering as Liu et al. (2021) observed a 1.8-day difference between two GmLHCA4a haplotypes under short-day growth conditions. In the present study, we demonstrated that the F2_GM18:1,434,182-1,935,386 hotspot regulates the C1 cluster, a group of 79 genes regulating photosynthesis and light response mechanism in the QS15524F2:F3 population. The F2_GM18:1,434,182-1,935,386_C1 cluster includes six Light-Harvesting Complex homologs and several genes associated with PSI (e.g. Glyma.10G042100/PHOTOSYSTEM I SUBUNIT E-2) and PSII (e.g. Glyma.10G089300, Glyma.10G089500 and Glyma.15G275600; all PHOTOSYSTEM II 5 KDA proteins). In soybean, 62 proteins, including 34 LHC A/B proteins, have been predicted to be involved in the regulation of the Light-Harvesting Complex (Lan et al., 2022). Consequently, the genes that were identified for the light response subcluster represent only a fraction of the LHC genes within the soybean genome.
The Glyma.18G025600 gene is the best candidate regulator for the LHC homologs
From these observations, we identified three candidate TFs (Glyma.18G020900, Glyma.18G025600, and Glyma.18G025800) located within the F2_GM18:1,434,182-1,935,386 hotspot. Based on our co-expression analysis, Glyma.18G020900 and Glyma.18G025600 were strongly co-expressed with the LHC homologs, but only Glyma.18G025600 had POSTWCEN and NEGTWCEN associated with photosynthetic and photosystem I/II regulation functions. Interestingly, Glyma.18G025600 harbored two mutations in its 3’UTR in OV, whereas none were found in Glyma.18G020900. To the best of our knowledge, this is the first time Glyma.18G025600 is proposed as a candidate for the transcriptional regulation of these six LHC homologs and more largely to the targets of the F2_GM18:1,434,182-1,935,386_C1 cluster in soybean. The LOB DOMAIN-CONTAINING PROTEIN 21 gene (Glyma.18G025600; GmLBD21) is the ortholog of AT3G11090 (AtASL12/AtLBD21) in Arabidopsis which belongs to the class 1a of the AS2 protein family (Iwakawa et al., 2002; Shuai et al., 2002). The AtAS2 gene (AT1G65620) encodes a domain that includes a leucine-zipper-like sequence in its amino-terminal half and a cysteine repeat (Matsumura et al., 2009). On a functional level, AtAS2 plays a role in the expansion of flat leaf lamina in Arabidopsis as AtAS2 overexpressing and loss-of-function Arabidopsis mutants respectively exhibited upwardly and downwardly curled leaves (Iwakawa et al., 2002). The gene DOWN IN DARK AND AUXIN1 (AT3G27650; AtDDA1/AtLBD25/AtASL3), a gene closely associated with LATERAL ORGAN BOUNDARIES (AtLOB) and ASYMMETRIC LEAVES2 (AS2), has been suggested to be implicated in photomorphogenesis and auxin response as dda1-1 plants display aberrant hypocotyl elongation and reduced sensitivity to auxin phenotypes (Mangeon et al., 2011). Overall, the current pieces of evidence suggest that Glyma.18G025600 is the best candidate for the F2_GM18:1,434,182-1,935,386 hotspot.
GmPRR1a and GmMDE04 are co-regulated by the same regions
The GmPRR1a and GmMDE04 genes and their homologs are known to have critical impacts on photoperiodic flowering in Arabidopsis and soybean. The soybean PSEUDO RESPONSE REGULATORs 1a and 1d are orthologs of the Arabidopsis DNA-binding transcription factor TIMING OF CAB EXPRESSION 1/PSEUDO-RESPONSE REGULATOR 1 (AtTOC1/AtPRR1) which contains a CCT (CONSTANS, CO-like, TOC1) domain in the C terminus and a pseudo receiver domain in the N terminus (Gendron et al., 2012). In Arabidopsis, this protein is known to be involved in the phytochrome regulation of circadian gene expression and photomorphogenic response (Más et al., 2003) and thus acts as a molecular bridge between environmental cues and clock outputs. In soybean, GmMDE04 (also named GmFULb) is involved in the E1-GmMDEs-GmFT2a/5a-Dt1 signaling pathway and responds to photoperiod at the transcript level (Zhai et al., 2022). Overexpression experiments have demonstrated that the GmMDE06 homolog acts downstream of E1 in the induction of the flowering process, increases the expression of GmFT2a/GmFT5a and promotes the termination of stem growth by repressing Dt1 (Zhai et al., 2022). According to Zhai et al. (2022), GmMDE04 is significantly expressed under short-day conditions versus long-day conditions in the ‘Harosoy-E1’, ‘Zhonghuang 13’ and ‘Gaofeng1’ backgrounds but not in ‘Harosoy-e1’, ‘Kariyutaka’, and ‘Sidou 11’. This gene is the ortholog of the Arabidopsis gene AGAMOUS-LIKE 8/FRUITFUL which induces global proliferative arrest (i.e. the coordinated arrest of all active meristems) by repressing members of the APETALA2 (AtAP2) clade involved in the maintenance of the shoot apical meristem (Martínez-Fernández et al., 2020). As a whole, the suppression in E1 expression has been demonstrated to be tightly associated with the photoperiod-insensitive expression of GmMDEs (Zhai et al., 2022). Structurally, GmMDE04 and GmMDE06 exhibit a higher degree of similitude between each other than for the five other MDE genes (results not shown).
In the present study, we identified two co-regulation events between GmPRR1a and GmMDE04. The first was found in QS15524F2:F3 (F2_GM15:49,385,092-49,442,237 hotspot), whereas the second was discovered in QS15544RIL (RIL_GM04:17,227,512-20,251,662). In addition, we also identified two other co-regulation events between PRR (i.e., GmPRR1d, GmARR2, and GmPRR4) and MDE (GmMDE06, GmMDE13, GmMDE17, and GmMDE19) homologs in the QS15544RIL population. Each of these regulation events were identified by at least two algorithms, except the interaction between GmMDE17 and the F2_GM15:49,385,092-49,442,237 hotspot, thus indicating the robustness and reliability of these interactions. Still, we consider the interaction between GmMDE17 and the F2_GM15:49,385,092-49,442,237 hotspot to be robust as we consider ICIM to be the one of the most reliable algorithms currently available to researchers.Regarding RIL_GM04:17,227,512-20,251,662, we discovered that this locus is located near the E8-r1 locus (RIL_GM04:16,974,874-17,152,230), a locus discovered in the same study as for E8-r3 and in the same population (Gélinas Bélanger et al., 2024a); however, this locus was found for the pod-filling trait under field conditions and was not considered for the present study. Still, the data generated in Gélinas Bélanger et al. (2024a) demonstrate that a critical regulator is found within the same genomic region.
For the co-regulation events associated with the F2_GM15:49,385,092-49,442,237 hotspot, two genes (Glyma.15G261300 and Glyma.15G263700) have been proposed as candidate regulators. At present, several lines of evidence (i.e. functional annotations, TWCEN functions, type of prevailing mutations, and more) suggest that Glyma.15G263700 (ALTERED PHLOEM DEVELOPMENT/GmAPL; also called GmFE) is the best candidate. The GmAPL is the ortholog of AT1G79430 in Arabidopsis, a phloem-specific Myb-related protein involved in the photoperiodic induction of flowering (Abe et al., 2015). Abe et al. (2015) have demonstrated that a missense mutation causing a glycine (G) to glutamic acid (E) substitution causes a late-flowering phenotype in Atfe mutants. Using expression analysis, Abe et al. (2015) have shown that a fully functioning AtFE allele is required for the transcriptional activation of FLOWERING LOCUS T INTERACTING PROTEIN 1 (AtFTIP1), a critical gene involved in the selective trafficking of AtFT protein from phloem companion cells to sieve elements (Liu et al., 2012).
Conclusion
We developed a novel eQTL mapping pipeline that enabled us to identify hundreds of transient cis and trans interactions in the QS15524F2:F3 and QS15544RIL soybean populations. From the trans interactions, we identified four hotspots involved in the regulation of FRSPD functions: (i) F2_GM06:39,892,719-43,437,125, F2_GM17:5,431,473-7,260,313 and F2_GM18:1,434,182-1,935,386 in QS15524F2:F3; and (ii) the RIL_GM04:10,812,813-10,985,437 in QS15544RIL. Deeper investigations identified trans regulatory events between: (i) F2_GM18:1,434,182-1,935,386 and GmLHCA4a; and (ii) several regions identified in QS15524F2:F3 and QS15544RIL and two candidate genes (GmPRR1a and GmMDE04) along with some homologs (GmPRR1d, GmPRR4, and GmMDE06). Using an approach combining the analysis of predicted TFs, TWCEN, annotated functions, and genomic variants, we identified several candidates for these regions of interest, with a focus on GmLBD21 (Glyma.18G025600) and GmAPL (Glyma.15G263700). Overall, the discoveries regarding the loci regulating the three candidate genes for the E8-r3 region (GmLHCA4a, GmPRR1a, and GmMDE04) represent only a small proportion of the trans and cis interactions captured with our combinatorial mapping approach. These findings demonstrate the potential of eQTL interactions and hotspot mapping combined with co-expression analyses to identify a large number of TF-related regulatory events and narrow the number of potential TF candidates.
Data availability statement
The datasets presented in this study can be found in online repositories. The names of the repository/repositories and accession number(s) can be found below: https://www.ncbi.nlm.nih.gov/, BioProject PRJNA1035514.
Author contributions
JGB: Conceptualization, Data curation, Formal analysis, Investigation, Methodology, Software, Visualization, Writing – original draft. TC: Conceptualization, Data curation, Investigation, Methodology, Software, Supervision, Validation, Writing – review & editing. VH-V: Supervision, Writing – review & editing. LO’D: Conceptualization, Funding acquisition, Investigation, Project administration, Resources, Supervision, Writing – review & editing.
Funding
The author(s) declare financial support was received for the research, authorship, and/or publication of this article. The work presented here was supported by Génome Québec and Génome Canada with funds awarded to the SoyaGen Project by the Canadian Field Crop Research Alliance and Agriculture and Agri-Food Canada under the Agri-Innovation Program. JGB was supported by les Fonds de recherche du Québec volet Nature et Technologie, Centre SÈVE, Mitacs, the Natural Sciences and Engineering Research Council of Canada, and Seed World Group.
Acknowledgments
We would like to acknowledge the work of Éric Fortier for the extraction of RNA and phenotypic data collection. In addition, we would like to thank Joannie Berthon for her help with the phenotypic data collection.
Conflict of interest
Authors JGB, TC, and LO’D were employed by the company Centre de recherche sur les grains (CÉROM) Inc.
The remaining author declares that the research was conducted in the absence of any commercial or financial relationships that could be construed as a potential conflict of interest.
The author(s) declared that they were an editorial board member of Frontiers, at the time of submission. This had no impact on the peer review process and the final decision.
Publisher’s note
All claims expressed in this article are solely those of the authors and do not necessarily represent those of their affiliated organizations, or those of the publisher, the editors and the reviewers. Any product that may be evaluated in this article, or claim that may be made by its manufacturer, is not guaranteed or endorsed by the publisher.
Supplementary material
The Supplementary Material for this article can be found online at: https://www.frontiersin.org/articles/10.3389/fpls.2024.1463300/full#supplementary-material
Supplementary Figure 1 | Differentially expressed candidate genes for the E8-r3 locus in the QS15524F2:F3 and QS15544RIL parental lines. Heatmaps showing the number of DEGs in the QS15524F2:F3 (A) and QS15544RIL (C) parental lines. Volcano plots showing the differentially expressed candidate genes in the QS15524F2:F3 (B) and QS15544RIL (D) parental lines. Two candidate E8-r3 genes (GmCDF3 and GmMDE04) have been found to be upregulated in the QS15524F2:F3 parents, whereas none of the four candidates were found to be differentially expressed in the QS15544RIL parents.
Supplementary Figure 2 | Differentially expressed candidate transcription factors in the QS15524F2:F3 parents. Three candidate TFs (Glyma.15G263700, Glyma.18G020900, and Glyma.18G025800), have been found to be differentially expressed in the QS15524F2:F3 parental lines.
Footnotes
- ^ One gene was found to be regulated by both regions.
- ^ Four genes were found to be regulated by both regions.
References
Abe, M., Kaya, H., Watanabe-Taneda, A., Shibuta, M., Yamaguchi, A., Sakamoto, T., et al. (2015). FE, a phloem-specific Myb-related protein, promotes flowering through transcriptional activation of flowering locus T and flowering locus T interacting protein 1. Plant J. 83, 1059–1068. doi: 10.1111/tpj.12951
Abed, A., Légaré, G., Pomerleau, S., St-Cyr, J., Boyle, B., Belzile, F. J. (2019). “Genotyping-by-Sequencing on the Ion Torrent Platform in Barley. In: Harwood, W. (eds) Barley. Methods in Molecular Biology, vol 1900 (New York, NY: Humana Press). doi: 10.1007/978-1-4939-8944-7_15
Ali, T., Zhou, B., Cleary, D., Xie, W. (2022). The impact of climate change on China and Brazil’s soybean trade. Land 11, 2286. doi: 10.3390/land11122286
Anders, S., Pyl, P. T., Huber, W. (2015). HTSeq—a Python framework to work with high-throughput sequencing data. Bioinformatics 31, 166–169. doi: 10.1093/bioinformatics/btu638
Bian, S., Jin, D., Li, R., Xie, X., Gao, G., Sun, W., et al. (2017). Genome-wide analysis of CCA1-like proteins in soybean and functional characterization of GmMYB138a. Int. J. Mol. Sci. 18, 2040. doi: 10.3390/ijms18102040
Bolger, A. M., Lohse, M., Usadel, B. (2014). Trimmomatic: A flexible trimmer for Illumina sequence data. Bioinformatics 30, 2114–2120. doi: 10.1093/bioinformatics/btu170
Browning, S. R., Browning, B. L. (2007). Rapid and accurate haplotype phasing and missing-data inference for whole-genome association studies by use of localized haplotype clustering. Am. J. Hum. Genet. 81, 1084–1097. doi: 10.1086/521987
Bylino, O. V., Ibragimov, A. N., Shidlovskii, Y. V. (2020). Evolution of regulated transcription. Cells 9, 1–38. doi: 10.3390/cells9071675
Choi, J. H., Kim, T., Jung, J., Joo, J. W. J. (2020). Fully automated web-based tool for identifying regulatory hotspots. BMC Genomics 21, 1–7. doi: 10.1186/s12864-020-07012-z
Corrales, A. R., Carrillo, L., Lasierra, P., Nebauer, S. G., Dominguez-Figueroa, J., Renau-Morata, B., et al. (2017). Multifaceted role of cycling DOF factor 3 (CDF3) in the regulation of flowering time and abiotic stress responses in Arabidopsis. Plant Cell Environ. 40, 748–764. doi: 10.1111/pce.12894
Danecek, P., Auton, A., Abecasis, G., Albers, C. A., Banks, E., DePristo, M. A., et al. (2011). The variant call format and VCFtools. Bioinformatics 27, 2156–2158. doi: 10.1093/bioinformatics/btr330
Dietz, N., Chan, Y. O., Scaboo, A., Graef, G., Hyten, D., Happ, M., et al. (2022). Candidate genes modulating reproductive timing in elite US soybean lines identified in soybean alleles of arabidopsis flowering orthologs with divergent latitude distribution. Front. Plant Sci. 13. doi: 10.3389/fpls.2022.889066
Escamilla, D. M., Dietz, N., Bilyeu, K., Hudson, K., Rainey, K. M. (2024). Genome-wide association study reveals GmFulb as candidate gene for maturity time and reproductive length in soybeans (Glycine max). PLoS One 19, e0294123. doi: 10.1371/journal.pone.0294123
Fang, C., Liu, J., Zhang, T., Su, T., Li, S., Cheng, Q., et al. (2021). A recent retrotransposon insertion of J caused E6 locus facilitating soybean adaptation into low latitude. J. Integr. Plant Biol. 63, 995–1003. doi: 10.1111/jipb.13034
Ge, S. X., Son, E. W., Yao, R. (2018). iDEP: An integrated web application for differential expression and pathway analysis of RNA-Seq data. BMC Bioinf. 19, 1–24. doi: 10.1186/s12859-018-2486-6
Gélinas Bélanger, J., Copley, T. R., Hoyos-Villegas, V., O’Donoughue, L. (2024a). Dissection of the E8 locus in two early maturing Canadian soybean populations. Front. Plant Sci. 15. doi: 10.3389/fpls.2024.1329065
Gélinas Bélanger, J., Copley, T. R., Hoyos-Villegas, V., O’Donoughue, L.. (2024b). Identification of quantitative trait loci associated with seed quality traits in two early-maturing soybean populations. Can. J. Plant Sci. 0, null. doi: 10.1139/cjps-2024-0049
Gendron, J. M., Pruneda-Paz, J. L., Doherty, C. J., Gross, A. M., Kang, S. E., Kay, S. A. (2012). Arabidopsis circadian clock protein, TOC1, is a DNA-binding transcription factor. Proc. Natl. Acad. Sci. 109, 3167–3172. doi: 10.1073/pnas.1200355109
Gilad, Y., Rifkin, S. A., Pritchard, J. K. (2008). Revealing the architecture of gene regulation: the promise of eQTL studies. Trends Genet. 24, 408–415. doi: 10.1016/j.tig.2008.06.001
Goedhart, J., Luijsterburg, M. S. (2020). VolcaNoseR is a web app for creating, exploring, labeling and sharing volcano plots. Sci. Rep. 10, 20560. doi: 10.1038/s41598-020-76603-3
Goodstein, D. M., Shu, S., Howson, R., Neupane, R., Hayes, R. D., Fazo, J., et al. (2012). Phytozome: A comparative platform for green plant genomics. Nucleic Acids Res. 40, 1178–1186. doi: 10.1093/nar/gkr944
Grant, D., Nelson, R. T., Cannon, S. B., Shoemaker, R. C. (2009). SoyBase, the USDA-ARS soybean genetics and genomics database. Nucleic Acids Res. 38, 843–846. doi: 10.1093/nar/gkp798
Hotta, C. T. (2021). From crops to shops: How agriculture can use circadian clocks. J. Exp. Bot. 72, 7668–7679. doi: 10.1093/jxb/erab371
Huang, L., Liu, X., Pandey, M. K., Ren, X., Chen, H., Xue, X., et al. (2020). Genome-wide expression quantitative trait locus analysis in a recombinant inbred line population for trait dissection in peanut. Plant Biotechnol. J. 18, 779–790. doi: 10.1111/pbi.13246
Iwakawa, H., Ueno, Y., Semiarti, E., Onouchi, H., Kojima, S., Tsukaya, H., et al. (2002). The ASYMMETRIC LEAVES2 gene of arabidopsis thaliana, required for formation of a symmetric flat leaf lamina, encodes a member of a novel family of proteins characterized by cysteine repeats and a leucine zipper. Plant Cell Physiol. 43, 467–478. doi: 10.1093/pcp/pcf077
Jin, J., Tian, F., Yang, D. C., Meng, Y. Q., Kong, L., Luo, J., et al. (2017). PlantTFDB 4.0: Toward a central hub for transcription factors and regulatory interactions in plants. Nucleic Acids Res. 45, D1040–D1045. doi: 10.1093/nar/gkw982
Kim, D., Pertea, G., Trapnell, C., Pimentel, H., Kelley, R., Salzberg, S. L. (2013). TopHat2: accurate alignment of transcriptomes in the presence of insertions, deletions and gene fusions. Genome Biol. 14, R36. doi: 10.1186/gb-2013-14-4-r36
Kong, F., Liu, B., Xia, Z., Sato, S., Kim, B. M., Watanabe, S., et al. (2010). Two coordinately regulated homologs of FLOWERING LOCUS T are involved in the control of photoperiodic flowering in Soybean. Plant Physiol. 154, 1220–1231. doi: 10.1104/pp.110.160796
Kumar, P., Henikoff, S., Ng, P. C. (2009). Predicting the effects of coding non-synonymous variants on protein function using the SIFT algorithm. Nat. Protoc. 4, 1073–1082. doi: 10.1038/nprot.2009.86
Lan, Y., Song, Y., Zhao, F., Cao, Y., Luo, D., Qiao, D., et al. (2022). Phylogenetic, structural and functional evolution of the LHC gene family in plant species. Int. J. Mol. Sci. 24, 488. doi: 10.3390/ijms24010488
Li, H., Ye, G., Wang, J. (2007). A modified algorithm for the improvement of composite interval mapping. Genetics 175, 361–374. doi: 10.1534/genetics.106.066811
Li, C. S., Poushinsky, G. (1983). A modified augmented design for an early stage of plant selection involving a large number of test lines without replication. Biometrics, 553–561.
Li, C. S., Poushinsky, G. R. E. G. (1985). A modified augmented design (type 2) for rectangular plots. Canadian J Plant Sci. 65(3), 743–749
Lin, X., Dong, L., Tang, Y., Li, H., Cheng, Q., Li, H., et al. (2022). Novel and multifaceted regulations of photoperiodic flowering by phytochrome A in soybean. Proc. Natl. Acad. Sci. 119, e2208708119. doi: 10.1073/pnas.2208708119
Liu, C., Chen, X., Wang, W., Hu, X., Han, W., He, Q., et al. (2021). Identifying wild versus cultivated gene-alleles conferring seed coat color and days to flowering in Soybean. Int. J. Mol. Sci. 22, 1–22. doi: 10.3390/ijms22041559
Liu, L., Gao, L., Zhang, L., Cai, Y., Song, W., Chen, L., et al. (2022). Co-silencing E1 and its homologs in an extremely late-maturing soybean cultivar confers super-early maturity and adaptation to high-latitude short-season regions. J. Integr. Agric. 21, 326–335. doi: 10.1016/S2095-3119(20)63391-3
Liu, B., Kanazawa, A., Matsumura, H., Takahashi, R., Harada, K., Abe, J. (2008). Genetic redundancy in soybean photoresponses associated with duplication of the phytochrome A gene. Genetics 180, 995–1007. doi: 10.1534/genetics.108.092742
Liu, L., Liu, C., Hou, X., Xi, W., Shen, L., Tao, Z., et al. (2012). FTIP1 is an essential regulator required for florigen transport. PLoS Biol. 10, e1001313. doi: 10.1371/journal.pbio.1001313
Liu, H., Wang, H., Gao, P., Xü, J., Xü, T., Wang, J., et al. (2009). Analysis of clock gene homologs using unifoliolates as target organs in soybean (Glycine max). J. Plant Physiol. 166, 278–289. doi: 10.1016/j.jplph.2008.06.003
Love, M. I., Huber, W., Anders, S. (2014). Moderated estimation of fold change and dispersion for RNA-seq data with DESeq2. Genome Biol. 15, 1–21. doi: 10.1186/s13059-014-0550-8
Mangeon, A., Bell, E. M., Lin, W., Jablonska, B., Springer, P. S. (2011). Misregulation of the LOB domain gene DDA1 suggests possible functions in auxin signalling and photomorphogenesis. J. Exp. Bot. 62, 221–233. doi: 10.1093/jxb/erq259
Martínez-Fernández, I., de Moura, S., Alves-Ferreira, M., Ferrándiz, C., Balanzà, V. (2020). Identification of players controlling meristem arrest downstream of the FRUITFULL-APETALA2 pathway. Plant Physiol. 184, 945–959. doi: 10.1104/pp.20.00800
Más, P., Alabadí, D., Yanovsky, M. J., Oyama, T., Kay, S. A. (2003). Dual role of TOC1 in the control of circadian and photomorphogenic responses in Arabidopsis. Plant Cell 15, 223–236. doi: 10.1105/tpc.006734
Matsumura, Y., Iwakawa, H., Machida, Y., Machida, C. (2009). Characterization of genes in the ASYMMETRIC LEAVES2/LATERAL ORGAN BOUNDARIES (AS2/LOB) family in Arabidopsis thaliana, and functional and molecular comparisons between AS2 and other family members. Plant J. 58, 525–537. doi: 10.1111/j.1365-313X.2009.03797.x
McLaren, W., Gil, L., Hunt, S. E., Riat, H. S., Ritchie, G. R. S., Thormann, A., et al. (2016). The ensembl variant effect predictor. Genome Biol. 17, 1–14. doi: 10.1186/s13059-016-0974-4
Meng, L., Li, H., Zhang, L., Wang, J. (2015). QTL IciMapping: Integrated software for genetic linkage map construction and quantitative trait locus mapping in biparental populations. Crop J. 3, 269–283. doi: 10.1016/j.cj.2015.01.001
Morales, A. M. A. P., O’Rourke, J. A., van de Mortel, M., Scheider, K. T., Bancroft, T. J., Borém, A., et al. (2013). Transcriptome analyses and virus induced gene silencing identify genes in the Rpp4-mediated Asian soybean rust resistance pathway. Funct. Plant Biol. 40, 1029–1047. doi: 10.1071/FP12296
Ng, P. C., Henikoff, S. (2003). SIFT: Predicting amino acid changes that affect protein function. Nucleic Acids Res. 31, 3812–3814. doi: 10.1093/nar/gkg509
Rimmer, A., Phan, H., Mathieson, I., Iqbal, Z., Twigg, S. R. F., Wilkie, A. O. M., et al. (2014). Integrating mapping-, assembly- and haplotype-based approaches for calling variants in clinical sequencing applications. Nat. Genet. 46, 912–918. doi: 10.1038/ng.3036
Shuai, B., Reynaga-Peña, C. G., Springer, P. S. (2002). The Lateral Organ Boundaries gene defines a novel, plant-specific gene family. Plant Physiol. 129, 747–761. doi: 10.1104/pp.010926
Sláma, V., Cupellini, L., Mascoli, V., Liguori, N., Croce, R., Mennucci, B. (2023). Origin of low-lying red states in the lhca4 light-harvesting complex of photosystem I. J. Phys. Chem. Lett. 14, 8345–8352. doi: 10.1021/acs.jpclett.3c02091
Sun, H., Jia, Z., Cao, D., Jiang, B., Wu, C., Hou, W., et al. (2011). GmFT2a, a soybean homolog of flowering locus T, is involved in flowering transition and maintenance. PLoS One 6, 18–20. doi: 10.1371/journal.pone.0029238
Tardivel, A., Torkamaneh, D., Lemay, M.-A., Belzile, F., O’Donoughue, L. S. (2019). A systematic gene-centric approach to define haplotypes and identify alleles on the basis of dense single nucleotide polymorphism datasets. Plant Genome 12, 180061. doi: 10.3835/plantgenome2018.08.0061
The American Soybean Association (2023). SoyStats - International: World Soybean Production, (2021/2022 year) (Am. Soybean Assoc). Available online at: http://soystats.com/ (Accessed December 11, 2023).
Torkamaneh, D., Laroche, J., Bastien, M., Abed, A., Belzile, F. (2017). Fast-GBS: A new pipeline for the efficient and highly accurate calling of SNPs from genotyping-by-sequencing data. BMC Bioinf. 18, 1–7. doi: 10.1186/s12859-016-1431-9
Unc, A., Altdorff, D., Abakumov, E., Adl, S., Baldursson, S., Bechtold, M., et al. (2021). Expansion of agriculture in northern cold-climate regions: A cross-sectoral perspective on opportunities and challenges. Front. Sustain. Food Syst. 5. doi: 10.3389/fsufs.2021.663448
Venkat, A., Muneer, S. (2022). Role of circadian rhythms in major plant metabolic and signaling pathways. Front. Plant Sci. 13. doi: 10.3389/fpls.2022.836244
Wang, X., Ma, L. (2013). Unraveling the circadian clock in Arabidopsis. Plant Signal. Behav. 8, 1–6. doi: 10.4161/psb.23014
Wang, J., Yu, H., Weng, X., Xie, W., Xu, C., Li, X., et al. (2014). An expression quantitative trait loci-guided co-expression analysis for constructing regulatory network using a rice recombinant inbred line population. J. Exp. Bot. 65, 1069–1079. doi: 10.1093/jxb/ert464
Wang, J., Yu, H., Xie, W., Xing, Y., Yu, S., Xu, C., et al. (2010). A global analysis of QTLs for expression variations in rice shoots at the early seedling stage. Plant J. 63, 1063–1074. doi: 10.1111/j.1365-313X.2010.04303.x
Watanabe, S., Hideshima, R., Zhengjun, X., Tsubokura, Y., Sato, S., Nakamoto, Y., et al. (2009). Map-based cloning of the gene associated with the soybean maturity locus E3. Genetics 182, 1251–1262. doi: 10.1534/genetics.108.098772
Watanabe, S., Xia, Z., Hideshima, R., Tsubokura, Y., Sato, S., Yamanaka, N., et al. (2011). A map-based cloning strategy employing a residual heterozygous line reveals that the GIGANTEA gene is involved in soybean maturity and flowering. Genetics 188, 395–407. doi: 10.1534/genetics.110.125062
West, M. A. L., Kim, K., Kliebenstein, D. J., Van Leeuwen, H., Michelmore, R. W., Doerge, R. W., et al. (2007). Global eQTL mapping reveals the complex genetic architecture of transcript-level variation in Arabidopsis. Genetics 175, 1441–1450. doi: 10.1534/genetics.106.064972
Westra, H. J., Franke, L. (2014). From genome to function by studying eQTLs. Biochim. Biophys. Acta - Mol. Basis Dis. 1842, 1896–1902. doi: 10.1016/j.bbadis.2014.04.024
Xia, Z., Watanabe, S., Yamada, T., Tsubokura, Y., Nakashima, H., Zhai, H., et al. (2012). Positional cloning and characterization reveal the molecular basis for soybean maturity locus E1 that regulates photoperiodic flowering. Proc. Natl. Acad. Sci. U. S. A. 109, E2155–E2164. doi: 10.1073/pnas.1117982109
Yu, K., Wang, J., Sun, C., Liu, X., Xu, H., Yang, Y., et al. (2020). High-density QTL mapping of leaf-related traits and chlorophyll content in three soybean RIL populations. BMC Plant Biol. 20, 470. doi: 10.1186/s12870-020-02684-x
Zhai, H., Wan, Z., Jiao, S., Zhou, J., Xu, K., Nan, H., et al. (2022). GmMDE genes bridge the maturity gene E1 and florigens in photoperiodic regulation of flowering in soybean. Plant Physiol. 189, 1021–1036. doi: 10.1093/plphys/kiac092
Zhang, Y., Wen, Y. J., Dunwell, J. M., Zhang, Y. M. (2020). QTL.gCIMapping.GUI v2.0: An R software for detecting small-effect and linked QTLs for quantitative traits in bi-parental segregation populations. Comput. Struct. Biotechnol. J. 18, 59–65. doi: 10.1016/j.csbj.2019.11.005
Keywords: expression quantitative trait loci, regulatory hotspots, early-maturing soybeans, candidate genes, transcription factor, E8-r3 locus, co-expression network analysis
Citation: Gélinas Bélanger J, Copley TR, Hoyos-Villegas V and O’Donoughue L (2024) Integrated eQTL mapping approach reveals genomic regions regulating candidate genes of the E8-r3 locus in soybean. Front. Plant Sci. 15:1463300. doi: 10.3389/fpls.2024.1463300
Received: 11 July 2024; Accepted: 21 October 2024;
Published: 12 November 2024.
Edited by:
Kazuki Matsubara, Institute of Crop Science (NARO), JapanReviewed by:
Ryoma Takeshima, National Agriculture and Food Research Organization (NARO), JapanEri Ogiso-Tanaka, National Museum of Nature and Science, Japan
Copyright © 2024 Gélinas Bélanger, Copley, Hoyos-Villegas and O’Donoughue. This is an open-access article distributed under the terms of the Creative Commons Attribution License (CC BY). The use, distribution or reproduction in other forums is permitted, provided the original author(s) and the copyright owner(s) are credited and that the original publication in this journal is cited, in accordance with accepted academic practice. No use, distribution or reproduction is permitted which does not comply with these terms.
*Correspondence: Louise O’Donoughue, bG91aXNlLm9kb25vdWdodWVAY2Vyb20ucWMuY2E=