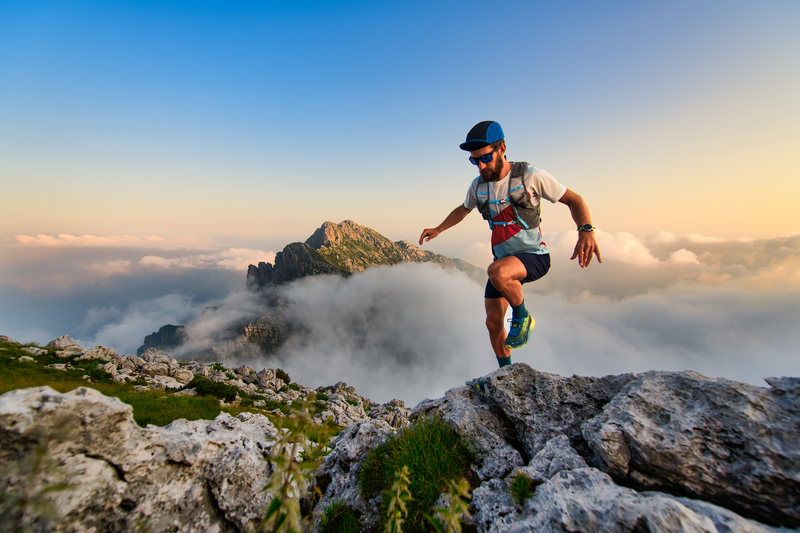
95% of researchers rate our articles as excellent or good
Learn more about the work of our research integrity team to safeguard the quality of each article we publish.
Find out more
ORIGINAL RESEARCH article
Front. Plant Sci. , 03 October 2024
Sec. Plant Breeding
Volume 15 - 2024 | https://doi.org/10.3389/fpls.2024.1448961
Northern corn leaf blight (NCLB), caused by Setosphaeria turcica, is a major fungal disease affecting maize production in sub-Saharan Africa. Utilizing host plant resistance to mitigate yield losses associated with NCLB can serve as a cost-effective strategy. In this study, we conducted a high-resolution genome-wide association study (GWAS) in an association mapping panel and linkage mapping with three doubled haploid (DH) and three F3 populations of tropical maize. These populations were phenotyped for NCLB resistance across six hotspot environments in Kenya. Across environments and genotypes, NCLB scores ranged from 2.12 to 5.17 (on a scale of 1–9). NCLB disease severity scores exhibited significant genotypic variance and moderate-to-high heritability. From the six biparental populations, 23 quantitative trait loci (QTLs) were identified, each explaining between 2.7% and 15.8% of the observed phenotypic variance. Collectively, the detected QTLs explained 34.28%, 51.37%, 41.12%, 12.46%, 12.11%, and 14.66% of the total phenotypic variance in DH populations 1, 2, and 3 and F3 populations 4, 5, and 6, respectively. GWAS, using 337,110 high-quality single nucleotide polymorphisms (SNPs), identified 15 marker–trait associations and several putative candidate genes linked to NCLB resistance in maize. Joint linkage association mapping (JLAM) identified 37 QTLs for NCLB resistance. Using linkage mapping, JLAM, and GWAS, several QTLs were identified within the genomic region spanning 4 to 15 Mbp on chromosome 2. This genomic region represents a promising target for enhancing NCLB resistance via marker-assisted breeding. Genome-wide predictions revealed moderate correlations with mean values of 0.45, 0.44, 0.55, and 0.42 for within GWAS panel, DH pop1, DH pop2, and DH pop3, respectively. Prediction by incorporating marker-by-environment interactions did not show much improvement. Overall, our findings indicate that NCLB resistance is quantitative in nature and is controlled by few major-effect and many minor-effect QTLs. We conclude that genomic regions consistently detected across mapping approaches and populations should be prioritized for improving NCLB resistance, while genome-wide prediction results can help incorporate both major- and minor-effect genes. This study contributes to a deeper understanding of the genetic and molecular mechanisms driving maize resistance to NCLB.
As a staple crop in sub-Saharan Africa (SSA), maize underpins food security and livestock production while also providing a vital source of income for smallholder farmers. This crop contributes no less than 30% of the aggregate caloric intake for people in this region (Nuss and Tanumihardjo, 2010). In this context, initiatives focusing on enhancing productivity and sustainability hold the potential to empower maize-dependent smallholder farming communities. Although advances in agricultural research and technology have resulted in a gradual increase in maize production, average maize field productivity in SSA (∼1.7 tons/ha) is far below the global average (∼5 tons/ha) (Prasanna et al., 2020). This can be attributed to various abiotic and biotic stressors, including low soil nitrogen (Das et al., 2019; Ndlovu et al., 2022; Kimutai et al., 2023), inadequate availability and use of farm inputs (e.g., improved seed and fertilizer), insect pest infestations (Kansiime et al., 2023), and disease incidences. Some of these stresses have a negative effect on grain quality or nutrient composition (Sharma and Carena, 2016; Ndlovu et al., 2024a), with far-reaching implications for household nutrition in smallholder communities. Most importantly, diseases such as gray leaf spot (GLS) (Lehmensiek et al., 2001; Benson et al., 2015; Kibe et al., 2020a), maize lethal necrosis (MLN) (De Groote et al., 2016; Beyene et al., 2017; Boddupalli et al., 2020), and northern corn leaf blight (NCLB) (Ding et al., 2015; Chen et al., 2016; Galiano-Carneiro et al., 2021) have been reported to cause considerable losses in maize production systems. The latter has been widely observed to significantly reduce maize grain yield on a global scale, as reported in earlier studies (De Rossi et al., 2022; Raymundo and Hooker, 1981).
NCLB (also known as Turcicum leaf blight) is a foliar disease of maize caused by the ascomycete fungus Setosphaeria turcica (anamorph Exserohilum turcicum) (Li et al., 2018; Jindal et al., 2019; Cao et al., 2020; Ahangar et al., 2022). Setosphaeria turcica is categorized into distinct races distinguished by their virulence levels against Ht (Helminthosporium turcicum) genes in maize (Galiano-Carneiro and Miedaner, 2017). Ht genes are recognized for providing race-specific qualitative resistance that is inherited through single genes (Bentolila et al., 1991; Zaitlin et al., 1993; Yin et al., 2003; Xiao et al., 2007; Chung et al., 2010). However, environmental factors may influence the expression of the Ht genes within maize or the avirulence genes of Setosphaeria turcica, leading to resistance that is unstable and/or less durable (Rashid et al., 2020). NCLB is commonly widespread in mid-altitude tropical and sub-tropical regions with moderate temperatures (17°C–28°C) and cloudy weather coupled with high rainfall and high humidity (Hooda et al., 2017; Bankole et al., 2023). Tropical regions show high pathogen abundance and genetic diversity, resulting in inflated disease severity and a high risk of resistance breakdown (Rashid et al., 2020). These favorable conditions allow the fungus to spread biotrophically during the initial infection process and later switch to a necrotrophic lifestyle. Infections manifest as lesions on leaves and necrosis, leading to a drastic reduction in the photosynthetic potential of the host plant, leading to huge grain yield losses (Ramathani et al., 2011; Ding et al., 2015). The disease affects maize at all growth stages, and infection at an early stage leads to premature death and reduced vigor (Welz and Geiger, 2000; Muiru et al., 2007).
The key to tackle NCLB in tropical maize lies in uncovering the pathogen’s mechanism of infection and the factors that govern resistance. Wisser et al. (2006) alluded that understanding the genetic architecture of host resistance is the foundation of disease management. This is also highly relevant for resistance breeding programs (Yang et al., 2017). However, despite the modern tools and advancements in this field, the genetic basis of resistance to several foliar diseases (like NCLB) in maize is still not well understood. Several studies focusing on the genetics of NCLB resistance documented a combination of both qualitative and quantitative types of inheritance (Welz and Geiger, 2000; Poland et al., 2011; Li et al., 2018). Qualitative resistance stems from allelic variations at only one or two resistance genes, with allele effects large enough to reliably deduce a plant’s resistance genotype from its phenotype (St. Clair, 2010), irrespective of environmental fluctuations (Yang et al., 2017). Quantitative resistance, which is widely used by maize breeders (Yang et al., 2017), refers to the contribution of several genes with small additive effects for the expressed resistance. Resistance to NCLB has been reported to be governed by genes with additive effects coming from a few major genes (such as Ht1, Ht2, Ht3, ht4, Htnl, Html, and NN) (Welz and Geiger, 2000; Kaefer et al., 2017; Li et al., 2018). Earlier breeding schemes have predominantly relied on qualitative resistance governed mainly by major gene effects, conferred by Ht genes, which are vulnerable to break down of resistance and are impeded by the emergence of new races of the pathogen through recombination events or mutation (Wende et al., 2018). In tropical settings, a broad-based quantitative resistance to NCLB is preferred. This can be achieved either through quantitative disease resistance loci (dQTLs) independently or in conjunction with potent Ht genes (Rashid et al., 2020). Carson and Dyke (1994) alluded that quantitative resistance is more durable because it includes both major and minor effect quantitative trait loci (QTL). In this respect, for the development of comprehensive resistance to NCLB in maize, a combination of both additive and non-additive effects is required (Ogliari et al., 2007; Vieira et al., 2009).
Several mapping studies have been conducted to identify QTLs associated with NCLB resistance across diverse maize germplasm and environments. Freymark et al. (1993) and Dingerdissen et al. (1996) identified five QTLs, which together explained 48% of total phenotypic variance (PVE) for NCLB resistance in F2:3 populations. With a high-density genetic map, Chen et al. (2016) identified a major QTL qNCLB5.04 (for NCLB disease severity and lesion size), which explained 20% of the observed phenotypic variation. Another study by Omondi et al. (2023) identified 18 QTLs, which together explained 64.9% of the total PVE in NCLB disease severity. Poland et al. (2011) and Li et al. (2018) used populations derived from multiple parents in nested association mapping (NAM) design and identified 29 and 48 NCLB-associated QTLs, respectively. Depending on the type of population used for QTL mapping, researchers reported the presence of both additive and non-additive effects for NCLB resistance in these studies.
Genome-wide association study (GWAS) is another robust and cost-effective method for dissecting polygenic traits, enhancing mapping resolution, and complementing linkage mapping techniques (Gowda et al., 2021). GWAS has been employed to detect allelic variations for MLN (Gowda et al., 2015), GLS (Kibe et al., 2020a), tar spot complex (Cao et al., 2017), corn rust (Wang et al., 2023), sorghum downy mildew (Rashid et al., 2018), and NCLB (Galiano-Carneiro et al., 2021). Several research studies have documented GWAS results on NCLB resistance in maize, predominantly concentrating on temperate germplasm and environments (Rashid et al., 2020). Using a large association mapping panel with 999 inbred lines, Ding et al. (2015) revealed 81 significantly associated SNPs for NCLB resistance. Another study by Van Inghelandt et al. (2012) identified significant SNPs on chromosomes 2, 5, 6, 7, and 9. Although numerous QTLs that control resistance to NCLB have been identified in maize, the current understanding of the genetic architecture particularly in the germplasm adapted to SSA is low. Indeed, GWAS and linkage analyses have their own merits and demerits when used independently. For example, because of population structure, GWAS generally shows higher false positive rates compared to linkage analysis (Yu et al., 2006; Zhang et al., 2018). In this respect, methods that incorporate linkage mapping and GWAS can bring together the merits of both approaches. Such combined approaches have been successfully applied to reveal the genetic basis of complex quantitative traits like MLN (Sitonik et al., 2019) and tar spot complex (Cao et al., 2017).
Genomic prediction (GP), previously developed for dairy breeding, has also been used in plant breeding for disease resistance. GP utilizes all available phenotyped and genotyped marker data of a training set to build a prediction model while bypassing the need for QTL detection (Meuwissen et al., 2001). GP has successfully been applied in the prediction of resistance to diseases such as Gibberella ear rot (Han et al., 2016), MLN (Gowda et al., 2015, 2018), and Goss’s wilt (Cooper et al., 2019) in maize. However, only a few studies have reported GP in NCLB resistance in maize (Technow et al., 2013; Omondi et al., 2023). GP studies have included genotype-by-environment interactions by performing overall predictions across environments (Heffner et al., 2011), within environments (Burgueño et al., 2012; Heslot et al., 2014), or using marker-by-environment interactions (Jarquín et al., 2014; Lopez-Cruz et al., 2015). In this respect, the objectives of the study were (1) to investigate the phenotypic variation for NCLB resistance and its correlation with other agronomic traits in tropical and sub-tropical maize lines; (2) to use linkage mapping, JLAM, and GWAS to identify genomic regions associated with NCLB resistance; and (3) to assess the potential of utilizing GP in breeding to improve NCLB resistance.
Here, we evaluated one association mapping (IMAS) panel, three biparental doubled haploid (DH) populations, and three biparental F3 populations across diverse environments in Kenya (Table 1). The parental lines used to form DH populations (CML494, CML504, CML511, and CML550) and F3 populations (CML505, CZL074, CZL009, CZL0723, CZL0719, and LapostaSeqC7-F103-1-2-1-1) showed a wide variation for foliar diseases including NCLB, corn rust, and GLS and known donors for low soil nitrogen stress and drought tolerance. More information on these populations has been reported in earlier studies (Ertiro et al., 2020b; Sitonik et al., 2019). The IMAS panel (composed of 390 inbred lines) was evaluated in replicated trials established across six Kenyan locations (i.e., Alupe 2012, Alupe 2013, Alupe 2014, Kibos 2013, Kibos 2014, and Embu 2013). The field trials were established in disease hotspot locations under natural infestation during the long rainy seasons (March to August). All three locations—Kibos [−0.03861°S, 34.81596°E; 1,193 m above mean sea level (masl); 865-mm mean annual rainfall), Alupe (0.503725°N, 34.12148°E; 1,153 masl; 1,400-mm mean annual rainfall), and Embu (0°31′52″ S 37°27′02″ E, 1,406 masl; 1,206-mm mean annual rainfall)—have a bimodal rainfall distribution. All three DH populations [CML494×CML550 (DH pop 1), CML504 × CML550 (DH pop 2), and CML511 × CML550 (DH pop 3)] were evaluated in Kakamega (0°17′3.19″ N 34°45′8.24″ E, 1,535 masl) and Kitale (1.0191°N 35.0023°E, 1900 masl) for 2 years in 2014 and 2015. Three F3 populations (F3 pop 4, CZL074 × LaPostaSeqC7-F103-1-2-1-1; F3 pop 5, CZL009 × CML505; and F3 pop 6, CZL0723 × CZL0719) were evaluated in two locations in Kakamega and Embu in 2013 during long rainy season (Table 1; Supplementary Table S1). All populations were evaluated in one row of 4-m plots, with two replications. For all these trials, two seeds were planted per hill and thinned to a single plant per hill 3 weeks after emergence. This was done to ensure a uniform plant density per entry. Alpha (α)–lattice experimental design was used, and all standard agronomic practices were applied.
Table 1. Trait means, estimates of heritability, and variance components for NCLB disease severity in the IMAS panel, DH, and F3 populations evaluated in multiple environments.
The locations used in this study are all hotspots for NCLB. Disease severity data were visually rated based on an ordinal scale of 1 (highly resistant, without disease symptoms) to 9 (highly susceptible, leading to necrosis) (Ding et al., 2015). For each population, each location-year combinations were treated as an independent environment, which resulted in six environments for the IMAS panel, four environments for DH populations, and two environments for each F3 population. In addition to the NCLB disease severity score, data were collected for other agronomic traits, including days to anthesis (AD; days from planting to 50% pollen shed), days to silking (SD; days from planting to 50% silking), and anthesis to silking interval (ASI; calculated as the difference between SD and AD). Plant height (PH; measured as the length in centimeters from the base of a plant to the insertion of the first tassel branch of the same plant for 10 representative plants per plot) and ear height (EH; measured as the length in centimeters from the base of a plant to the internode of the top ear of the same plant for 10 representative plants per plot). Ear position (EPO) was calculated as the ratio between PH and EH. GLS disease severity data were recorded at the mid-silking stage and scored plot-wise on an ordinal scale of 1 (highly resistant, without disease symptoms) to 9 (highly susceptible, leading to necrosis). Before harvesting the crop, the number of ears in each plot with portion of the ear exposed was recoded and expressed as a percentage of poor husk cover (HC) relative to the total number of ears harvested. Grain yield (GY) was calculated using the field weight of ears per plot, assuming an 80% shelling percentage and adjusting for a moisture content of 12.5%. Grain texture (TEX) was measured on a 1-to-5 scale (where 1 = flint, 2 = semi-flint, 3 = intermediate, 4 = semi-dent, and 5 = dent). NCLB disease severity data were recorded on an ordinal scale of 1 to 9, and the data met all the assumptions of the applied statistical model (i.e., normally distributed, constant variance, and independent) (Rawlings et al., 1998).
Analysis of variance for individuals and across environments was carried out using the ASREML-R (Gilmour et al., 2015; Kibe et al., 2020a, b) for the IMAS panel and DH and F3 populations. The linear mixed model with the restricted maximum likelihood (REML) was used to calculate all variance components. The study treated replication as a fixed effect and all other treatment effects as random. As defined by Knapp et al. (1985), the components of variance were estimated using the complete random effects model, whereas broad-sense heritability was calculated as the ratio of genotypic to PVE. META-R software (Alvarado et al., 2015) was used to generate best linear unbiased predictions (BLUPs) and best linear unbiased estimators (BLUEs), which were used in downstream processes.
The IMAS panel and DH populations used in this study have also been used in earlier studies for different traits (Sitonik et al., 2019; Ertiro et al., 2020a; Kibe et al., 2020a). The populations have been routinely used because the lines are representative of tropical and sub-tropical regions, developed over time. For all the inbred lines from the IMAS panel and biparental populations, DNA was extracted, purified, and genotyped with high-density makers using genotyping-by-sequencing (GBS) at the Institute of Genomic Diversity, Cornell University, USA, as described in earlier studies (Elshire et al., 2011; Gowda et al., 2015, 2021; Ndlovu et al., 2022). GBS data for all biparental populations were filtered by using TASSEL version 5.2 (Bradbury et al., 2007) with a criterion of excluding markers with heterozygosity of >5%, minor allele frequency (MAF) of < 0.05, and a minimum count of 90%. Only polymorphic SNPs between the parents and marker loci homozygous for both parents were retained in each biparental population. Finally, SNPs were further filtered with the criteria of minimum distance between adjacent SNPs as ≥200 Kilo base pairs to ensure uniform distribution of markers throughout the genome.
The linkage map for each biparental population was constructed by using QTL IciMapping version 4.1 (Meng et al., 2015). Finally, we used 2,105, 2,699 1,962, 1,160, 1139, and 1,160 high-quality SNPs in DH pop 1, DH pop 2, DH pop 3, F3 pop 4, F3 pop 5, and F3 pop 6, respectively. The linkage map was constructed by using these SNPs and by selecting the most significant markers using stepwise regression. A likelihood ratio test was used to calculate the logarithm of odds (LOD) for each marker at a score of >3 with a 30-cM maximum distance between two loci. The Kosambi mapping function (Kosambi, 1944) was used to transform the recombination frequencies between two linked loci. BLUPs across environments were used to detect QTLs based on inclusive interval mapping for each population. Phenotypic variation explained by individual QTLs and total variation explained by all QTLs together was estimated. QTL naming was done with the letter “q” indicating QTL, followed by an abbreviation of the trait name, the chromosome, and the marker position, respectively. For comparisons of QTL positions detected in different biparental populations, QTL sharing the same flanking markers and within the same chromosome interval were defined as the same QTL.
All three DH populations were genotyped with GBS markers and then combined for filtering. As a first step in quality control, we excluded markers with a heterozygosity of >5%, MAF of < 0.05, and a minimum count of 90%. For joint linkage association mapping (JLAM), markers were further filtered to retain SNPs with <1% missing values. As a result, a set of 7,490 SNPs that are uniformly distributed across the genome were retained for JLAM analyses. BLUPs across locations were used for the analyses. A linear model comprising co-factors and population effect (Würschum, 2012) was used to implement JLAM as it performs well for association analysis in segregating populations. This model was explained in detail by Liu et al. (2011) and Würschum (2012). In brief, with this model, in a first step, stepwise multiple linear regression was used in addition to population effect to select the cofactors based on the Schwarz Bayesian criterion (Schwarz, 1978), and, in the second step, P-values for the association of each marker with phenotypic value were calculated for the F-test by comparing a full model (including SNP effect) against a reduced model (without SNP effect) [for details see Reif et al. (2010)]. R software version 4.2.1 (R_Core_Team, 2023) was used to carry out genome-wide scans for QTLs, and cofactors were selected using PROC GLM SELECT from SAS 9.4 (SAS Institute, 2015).
The raw GBS data set was subjected to a filter at 90% minimum count, with a MAF > 0.05 and heterozygosity < 5% to retain high-density markers. After these quality checks, 337,110 high-quality SNPs were retained for GWAS analyses. BLUPs across locations were used as phenotypes in association mapping scans. Population structure and linkage disequilibrium plots were already reported in earlier studies (Kibe et al., 2020b), so we used the same information in this study. Principal components (PC) were calculated using TASSEL version 5.2 (Bradbury et al., 2007). The R package “FarmCPU-Fixed and random model Circulating Probability Unification” with GAPIT (Genome Association and Prediction Integrated Tool) was used for GWAS analysis (Tang et al., 2016). The first three PCs were used in the model. A false discovery rate (FDR; p < 0.05) was used to correct for multiple testing while determining the significance threshold. To summarize GWAS results per chromosome, Manhattan scatter plots were generated. The −log10 P-values for all the analyzed SNPs for NCLB disease severity data were used to construct the Manhattan plots. Quantile-quantile (Q-Q) plots were plotted from the estimated −log10 (P) from the association panel for the NCLB disease severity trait.
SNPs detected in the association panel or from JLAM results were examined as polymorphisms in linkage disequilibrium with putative candidate genes from the “B73” RefGen_v2. Candidate genes were identified through BLAST searches against the “B73” RefGen_v2. (https://www.maizegdb.org/gbrowse/maize_v2). Putative candidate genes were selected by delving into the information gene ontology, Kyoto Encyclopedia of Genes and Genomes, and protein families (Pfam) (Ashburner et al., 2000; Bateman et al., 2004; Kanehisa and Goto, 2000). The presence of the protein-coding genes was searched within the range of 10 kb (5 kb upstream and downstream) in the vicinity of the detected SNPs.
BLUEs across environments for the IMAS panel, each of the DH populations, and across DH populations were used for the analysis. Because all DH populations and IMAS panel were genotyped with GBS SNPs, we filtered in single file by excluding SNPs with MAF < 5%, heterozygosity > 5%, and no missing values. For all populations, the same set of high-quality uniformly distributed 4,000 SNPs was used. Among different GS models, ridge regression BLUP (RR-BLUP; Meuwissen et al., 2001; Endelman, 2011) appears well suited for regular plant breeding trials (Albrecht et al., 2011). Moreover, RR-BLUP has the advantage of being computationally less intensive, which is important in cross-validation studies. Therefore, we used RR-BLUP in the present study. GP analyses were conducted by using the package rrBLUP in R program (Endelman, 2011; Zhao et al., 2012; Crossa et al., 2017; R_Core_Team, 2023). We applied five-fold cross-validations in three different scenarios: the “within population” approach where both training and estimation set are derived from within each DH population and IMAS panel called as within-within approach. The second scenario is across-within populations, where all DH populations together form training set and individual DH population will be an estimation set. A third scenario is a combined population prediction approach where either all DH populations were combined and/or all DH populations together with IMAS panel were used to form both training and estimation sets. For each scenario, 100 iterations were performed for the sampling of the training and estimation sets. The predictive ability within each scenario (within-within, across-within, and combined all) was estimated by the Pearson correlation coefficient between the corrected phenotypic values (BLUEs) for each population and their predicted Genomic estimated breeding values (GEBVs).
The genotype-by-environment interaction plays a key role in genome selection and prediction in multi-environmental trials, across environments or at each environment usually is improved modeling the genotype-by-environment interaction (Burgueño et al., 2008). We evaluated three different models using the IMAS panel to evaluate prediction accuracy by doing prediction by environment or considering the genotype-by-environment interaction. The first model is a simple model in which each environment is analyzed individually. The second model includes an environmental effect in it, but marker effects are the same across environments. Finally, we fit a model in which marker effects vary by environment, it is a marker by environment interaction component. The model includes an overall marker effect across environments plus a specific environmental effect of each marker (Lopez-Cruz et al., 2015). For the analysis, we used a modified version of the scripts presented in github.com/MarcooLopez/Genomic-Selection/blob/master/multi_environment.mdfollowed the scripts. Here, we mimic two scenarios of prediction: one in which 82 genotypes (30%) were not evaluated in any environment (CV1) and the second in which 82 (30%) genotypes were not tested in two of six environments (CV2). Prediction accuracy was measured as the Pearson correlation between observed and predicted values in each environment and across environments.
One association panel, three DH, and three F3 populations were evaluated in NCLB disease hotspots in Kenya. Assessments of NCLB disease severity for each population at each location revealed significant genotypic variances (Supplementary Table S1), indicating sufficient disease pressure and differential response among the tested genotypes. In addition to genotypic variations, there were significant positive correlations between locations for each population (Supplementary Table S2). The locations selected for assessing each population differed in terms of NCLB disease severity (significant environmental variations). These variations offered valuable insights into the reaction of individual lines to NCLB at different locations.
Analyses of variance within populations and across environments revealed significant variations in disease severity (Table 1). On a scale of 1.0–9.0, NCLB disease severity was high in DH populations, with mean scores of 5.17 (CML494 × CML550 DH pop 1) and 4.69 (CML511 × CML550 DH pop 3). Across the studied populations, CZL0723 × CZL0719 F3 pop 6 (mean = 3.06) and CZL0009 × CML505 F3 pop 5 (mean = 2.21) had the lowest disease severity scores (Table 1). The distribution frequency of mean NCLB disease severity scores resembled a normal pattern (Figure 1). Across environments, analysis of variance revealed significant genotypic and G × E variations (P < 0.05) in all populations except for CZL0723 × CZL0719 F3 pop 6 (Table 1). Broad-sense heritability was moderate to high. In the correlation analysis, NCLB disease severity was positively and significantly correlated to AD, PH, and GLS (Figure 2). However, NCLB was negatively and significantly correlated to GY, ER, EPO, and EA. GY was positively and significantly correlated with PH and EPO but negatively correlated with ER, GLS, EA, and PA. ER was negatively and significantly correlated with NCLB, GLS, PH, AD, and GY (Figure 2). PH was positively correlated with AD and GLS.
Figure 1. Phenotypic distribution of NCLB disease severity scores (on a 1-to-9 scale) in IMAS association panel, three DH populations, and combined DH populations, and three F3 populations evaluated across locations.
Figure 2. Pairwise phenotypic correlations between northern corn leaf blight scores and other traits in combined three DH populations evaluated in three environments. Correlation values > 0.10 and >0.15 were interpreted as significant at 0.05 and 0.01 levels, respectively. GYG, grain yield; AD, days to anthesis; PH, plant height; EH, ear height; EPO, ear position; HC, husk cover; GLS, gray leaf spot; NCLB, Northern corn leaf blight; ER, ear rot; EA, ear aspect; PA, plant aspect.
The maize populations used in this study for QTL mapping were also evaluated in earlier studies (Ertiro et al., 2020a; Kibe et al., 2020a; Sitonik et al., 2019), which provided detailed genetic map information. Table 2 shows details of the QTLs associated with NCLB in the studied tropical maize populations. Three QTLs associated with NCLB were detected in DH pop 1, which explained a total phenotypic variation of 34.3% (Table 2). The detected QTLs were found on chromosomes 2 and 7. For DH pop 2, six QTLs were detected on chromosomes 1, 2, 6, 9, and 10. These QTLs together explained 51.4% of total phenotypic variation. In DH pop 3, four QTLs were detected; among them, two QTLs were located on chromosome 3 and the others on chromosomes 1 and 10. Together, they explained 41.1% of the total phenotypic variation. In F3 pop 4, two QTLs were identified which together explained 12.5% of total PVE. In F3 pop 5, four QTLs were detected for resistance to NCLB, which together explained 12.7% of the total PVE. In F3 pop 6, there were four QTLs detected which together explained 14.7% of the total phenotypic variations. The proportion of PVE explained by each QTL in each population varied from 10.2% to 15.8% for DH pop 1, 2.8% to 15.8% for DH pop 2, 9.9% to 13.1% for DH pop 3, 5.9% and 9.6% for F3 pop 4, 6.2% to 9% for F3 pop 5, and 4.1% to 10.9% for F3 pop 6 (Table 2).
Table 2. Analysis of QTLs associated with NCLB resistance in DH and F3 biparental populations evaluated in multiple environments.
Through JLAM, we identified 37 QTLs associated with resistance to NCLB. These QTLs were distributed in all 10 chromosomes and together explained 49.4% of the total PVE (Table 3). Unlike linkage mapping where phenotypic variation explained by each QTL showed minor to major effects (2.8%–15.8%), JLAM-detected QTLs showed minor effects, ranging from 0.5% to 2.1%. Most of the QTLs identified through JLAM were located on chromosome 2 (eight QTLs) followed by chromosome 5 (seven QTLs). Large variation was observed in SNP allele substitution effects which varied between −0.48 and 0.34 (Table 3). The putative candidate genes associated with these identified QTLs and their predicted function are also reported in Table 3, which are either directly or indirectly involved in plant defense (Table 3).
Table 3. Analysis of NCLB-associated markers, allele substitution (α) effects, and the explained proportion of phenotypic variance (R2) of the joint linkage association mapping in multiple segregating DH populations.
The QTLs or SNPs detected through GWAS, JLAM, and linkage mapping were compared on a physical map, which revealed several QTLs overlapped at same genomic regions (Figure 3). The SNP detected through JLAM at 36 Mbp (qNCLB1_36) is located within the QTL region detected in F3 pop4 (qNCLB-01-41). QTL detected in DH pop3 (qNCLB1_238) is adjacently located with SNP detected through JLAM (qNCLB1_248). Among the 37 QTLs identified through JLAM, 10 were co-located within the QTLs identified through individual population-based QTL mapping (Tables 2, 3). On chromosome 1, SNP S1_36801883 was co-located within the QTL qNCLB1-40 identified in F3 pop 6 (Table 2). On chromosome 2, SNP S2_15475173 and S2_197936812 were co-located within the QTL qNCLB2-14 and qNCLB2-197, respectively (identified in DH pop 1). SNP S4_218847954 was co-located within the QTL (qNCLB4-220) from F3 pop 5. SNPs S5_26100208 and S7_127888863 were co-located within the QTLs detected in DH pop 2, qNCLB5-24 and qNCLB7-126 on chromosomes 5 and 7, respectively. Three SNPs from chromosome 8—S8_21847291, S8_35814899, and S8_146017787—were co-located within the QTLs qNCLB8-20 from pop 5, qNCLB8-30 from pop 4, and qNCLB8-146 from pop 6, respectively (Tables 2, 3).
Figure 3. Mapping of NCLB resistance-associated QTLs detected based on individual biparental-based linkage mapping, joint linkage association mapping (JLAM), and IMAS association mapping panel (AMP). Red, green, and blue colors represent QTL detected under biparental-based linkage mapping (pop 1, pop 2, pop 3, pop 4, pop 5, and pop 6), JLAM, and AMP approaches, respectively.
The IMAS panel was used to identify and validate genomic regions for resistance to NCLB through GWAS. Fifteen marker–trait associations were identified by using 337,110 high-quality SNPs filtered from the raw GBS data set (Table 4; Figure 4). Because both fixed and random effect models are iteratively used in FarmCPU, which helps in avoiding overfitting of the model by stepwise regression (Liu et al., 2016), we used the FarmCPU model in the association mapping. The detected markers were distributed across all chromosomes except chromosomes 9 and 10, with P-values ranging from 5.58 × 10−14 to 8.29 × 10−06. The Manhattan plot revealed the highest peak on chromosome 5 (S5_24157791). Our analysis identified SNPs whose physical coordinates co-localized with chromosome bins where QTLs associated with NCLB resistance had been previously reported. For instance, SNP S6_165023408 was co-located with QTL qNCLB6-165 [detected in JLAM (Tables 3, 4)]. Using B73 maize genome V2.0, predicted gene annotations were studied to identify putative candidate genes associated with NCLB resistance. Numerous SNP associations identified in this study were situated within genes featuring functional domains related to metabolism, stress tolerance, and plant development. For example, SNP S2_213818302 was associated with peroxidase activity and oxidase stress responses. SNP S6_100083188, on the other hand, was associated with the gene responsible for phosphoglycerate kinase (PGK) activity that is involved in plant defense response (Table 4).
Table 4. Highly significant SNPs identified in GWAS analysis of DH and IMAS panel that were evaluated for NCLB resistance.
Figure 4. Manhattan and quantile-quantile (Q-Q) plots generated using a mixed linear model for NCLB scores across environments. The x-axis indicates the SNP location along the 10 chromosomes, with chromosomes separated by different colors. The significance level was set at P = 2 × 10–5 at 0.05 false discovery rate (FDR) and is represented on the plot by the dashed horizontal line. The position of SNPs along the 10 maize chromosomes is shown on the x-axis, with each color indicating distinct maize chromosomes. The −log10(P observed) is shown on the y-axis.
RR-BLUP has the advantage of being computationally less intensive, which is important in cross-validation studies and suits well for routine application in plant breeding trials (Albrecht et al., 2011). Therefore, we used the RR-BLUP model (Endelman, 2011) to estimate the performance of tropical maize genotypes under NCLB disease pressure (Figure 5). The prediction accuracy was highest for combined all three DH populations (r = 0.88) followed by prediction across DH populations and GWAS panel (r = 0.79). Overall, the prediction accuracies across genotypes were moderate to high (0.32 to 0.88). Average prediction accuracies within DH populations were relatively higher: 0.44, 0.55, and 0.42 for DH pop 1, DH pop 2, and DH pop 3, respectively. The IMAS association panel had a prediction accuracy of 0.45. For the across-within prediction scenario, the accuracy values were 0.32, 0.49, and 0.42 when estimation set is DH pop 1, DH pop 2, and DH pop 3, respectively.
Figure 5. Genome-wide prediction correlations for NCLB resistance in biparental and IMAS association panel based on three different scenarios. WW (within-within) scenario, estimation and prediction sets are derived within populations; AW (across-within) scenario, combined populations serve as a training set and an estimation set is from single biparental population; and combined scenario, where combine all populations and randomly derive both training and testing set and evaluate with five-fold cross-validation.
Table 5 shows the results of genome-wide prediction correlations using and not using marker by environment effect or, similarly, genotype-by-environment effect. The major difference between by environment and across environment prediction is because, for by environment prediction, we used un-tested genotypes to calculate Pearson correlation, whereas, for across environment prediction, we used all genotypes, because all genotypes were missing in at least two environments. As usual, under CV2 cross-validation, the prediction accuracy is high because there are not completely untested genotypes. The comparison between models shows that the across model is better in average to predict by environments in four and five environments in CV1 and CV2, respectively. The single model without marker by environment interaction was only better than the other models in CV1 to predict at location Embu12. The model with marker by environment interaction presented the largest prediction accuracy in one environment in each, CV1 (Alupe14) and CV2 (Embu12), and to do prediction across environments.
Table 5. Average Pearson correlation and standard deviation (n = 100), between observed and predicted NCLB disease severity values by environment and across environment in two cross-validation scenarios.
Assessing the genetic architecture of complex disease-resistance traits in plants requires extensive and accurate phenotyping. In environments where pathogen genetic diversity and disease pressure are high, broad-based quantitative resistance is essential because it is less broken by pathogen evolution (Galiano-Carneiro and Miedaner, 2017). Quantitative resistance to NCLB is governed by many genes (polygenic), with most QTL having minor effects and few having major phenotypic effects (Poland et al., 2011; Van Inghelandt et al., 2012). In this study, the NCLB scores for the IMAS association panel and each biparental population showed that resistance to NCLB in tropical maize is quantitative (Figure 1). This was well supported by earlier studies on association panels (Poland et al., 2011; Van Inghelandt et al., 2012) and biparental maize populations (Ranganatha et al., 2021; Omondi et al., 2023). We also observed significant genotypic and G × E interaction variances (Table 1). Estimates of broad sense heritability on a mean line basis (within individual populations) ranged from moderate to high. This suggests that the estimated variance within each population is reliable and indicates the potential for significant progress in selecting for NCLB resistance in this tropical maize germplasm.
Understanding the correlation and interactions between different traits is crucial in the development of marker-assisted-based multi-trait strategies (Xu and Crouch, 2008). Our results showed that NCLB has a negative and a significant relationship with grain yield. Similar findings of the negative relationship between NCLB and GY have been reported in earlier studies (Pataky et al., 1998; Weems, 2016; Razzaq et al., 2019). The negative correlation between plant height and NCLB in this study corroborated with Welz and Geiger (2000) who found a correlation coefficient of −0.27 (P = 0.01) between these two traits. The significant negative correlation indicates taller plants may have better resistance to NCLB. On the other hand, this will enhance lodging problems and having taller plants resistant to NCLB may not hold in different germplasm, so it warrants more studies before using in breeding selection.
The SNP-based linkage maps are being used to map NCLB resistance via linkage mapping, helping researchers understand the function of the chromosomal region or loci at the gene level. In this study, we used three DH populations and three F3 populations and identified genomic locations on all chromosomes except on chromosomes 1 and 9 (Table 2). Six major effect QTLs (>10% PVE) were identified; among them, three were in DH pop1, located on chromosomes 2 and 7. The moderate effect QTLs on chromosome 2 (qNCLB2_14 and qNCLB2_197) were detected within the QTL region reported in an earlier study that used DH populations (Omondi et al., 2023). The major effect QTL qNCLB7_14 detected in DH pop 1 (bin 7.02) was also co-located by Wang et al. (2018) with 13.16% of phenotypic variance explained. Bin 4.09 is another important region that harbors NCLB resistance QTLs. Chromosomal bins 1.03–06, 4.04–06, 5.04–07, 8.02–03, 8.05–06, and 9.02–04 have been consistently identified across multiple QTL mapping studies (Rashid et al., 2020). Welz et al. (1999) also found five QTLs located on different chromosome bins 1.06/1.07, 3.07, 4.03, 5.04, and 6.05/6.06 in a temperate F3 population. Most of the QTLs detected in this study overlapped with earlier studies (Miedaner et al., 2020; Galiano-Carneiro et al., 2021; Ranganatha et al., 2021; Omondi et al., 2023), indicating the presence of consistent QTLs across regions. This supports the potential for enhancing NCLB resistance across different genetic backgrounds through either marker-assisted recurrent selection or genome-wide selection.
For JLAM, even though the total PVE explained by the 37 QTLs was 49.4%, individual phenotypic variation for each QTL was less than 2.5% (Table 3), indicating that NCLB resistance being a polygenic trait. As expected, JLAM improved the resolution within the QTL intervals, finding new QTLs that are hard to find in individual population-based linkage mapping (Figure 3). Six of the QTLs identified by JLAM lay within or just outside of the confidence intervals identified earlier (Tables 2, 3; Figure 3). Many previous studies on resistance to NCLB have implicated the use of qualitative resistance in temperate and tropical germplasm (Welz and Geiger, 2000; Galiano-Carneiro and Miedaner, 2017). These are mostly Ht genes including Ht1, Ht2, Ht3, Ht4, Htn1, and Html, which could be partially or fully dominant (Carson, 1995; Welz and Geiger, 2000; Ogliari et al., 2005; Pataky and Ledencan, 2006). HtP, a dominant gene, and rt, a recessive gene conferring resistance to E. turcicum have been mapped on bins 2.08 and 3.06, respectively (Ogliari et al., 2007). These two regions were in proximity to SNPs S2_197936812 (qNCLB2-197) and S3_212501774 (qNCLB3-212) identified through JLAM (Table 3). Marker S2_197936812 lies at the exact location of the upper confidence interval of QTL qNCLB-197 identified through linkage mapping within DH pop 1, which coincidentally explained the highest phenotypic variation within that population. However, S3_212501774 found on chromosome 3 fell outside the confidence interval of QTL qNCLN3-213 in DH pop 3 (Tables 2, 3). The QTL qNCLB5-26 was co-located within the QTL qNCLB5-24 identified in DH pop 2 at bin 5.03 (Tables 2, 3). This is also a region identified for NCLB resistance through NAM in earlier studies (Poland et al., 2011). Interestingly, QTLs were identified in five genomic regions across chromosome 2 (10-15 Mbp), chromosome 4 (220-239 Mbp), chromosome 5 (22-26 Mbp), chromosome 6 (161-166 Mbp), and chromosome 8 (19-35 Mbp) using all three mapping approaches (Figure 3). These genomic regions where QTLs detected via linkage mapping, JLAM, and GWAS not only demonstrated stability but also contributed to narrowing down the confidence interval of the QTLs. These are the regions need to prioritize for future marker assisted breeding for NCLB resistance.
The identified significantly associated 15 SNPs were distributed on all maize chromosomes except chr 9 and 10. The total phenotypic variance explained by each SNP was <9%, indicating that resistance to NCLB is a polygenic in nature. Loci conferring resistance to NCLB has been detected through association mapping in previous studies (Wisser et al., 2006; Jamann et al., 2014). SNP S8_174614781 identified in our GWAS lay within the upper confidence interval of QTL identified in linkage mapping within F3 pop 5. Likewise, S6_165014618 on chromosome 6 identified via JLAM was closely associated with SNP S6_165023408 identified via GWAS. Because we did not observe deviation of SNP toward the expected P-value of Q-Q plots, putative candidate genes associated with significant SNP gave greater confidence in response to NCLB disease severity (Table 4). SNPs S1_17844737 on chromosome 1 and S3_122496339 on chromosome 8 were associated with genes GRMZM2G059020 and GRMZM2G340251, which code for ATP-dependent helicase activity and binding (Table 4). Using SNP-based bulk segregant analysis, Zhai et al. (2022) reported ATP-dependent helicase with disease resistance QTLs associated with NCLB in maize.
The candidate gene GRMZM2G031981 associated with S2_13777598 on chromosome 2 codes for heat shock protein (HSP) binding and folding. HSP proteins play a pivotal role within the intricate cellular system of molecular chaperones and catalysts for protein folding (Mayer and Bukau, 2005; Diogo-Jr et al., 2023). These HSPs function as chaperones by either enhancing the stability of newly synthesized proteins to facilitate proper folding or by aiding in the re-folding process of proteins that have been compromised due to cellular stress (Abou-Deif et al., 2019). HSPs are crucial for maintaining the integrity of PRRs on the cell membrane and R proteins inside the cell, ensuring that they are ready to counter potential threats (Park and Seo, 2015; Berka et al., 2022).
GRMZM2G450717 on chromosome 2 encodes peroxidase activity. Peroxidase activity contributes to lignification in plant cell walls, facilitating the production of phenolic compounds and ultimately reinforcing cell walls to prevent pathogen intrusion during infection (Almagro et al., 2008; Yanti, 2015). Earlier studies have also documented the role that peroxidases in plant growth and development (Li, 2023). The gene was also associated with heme binding and oxidative response. Under stress, earlier studies have reported on the role of heme-mediated homeostasis in plants (Singh and Bhatla, 2022; Wu et al., 2022). Another SNP identified in this study, S3_150390803, was linked to GRMZM2G176968, which codes for quercetin sulphate biosynthesis. Under osmotic stress, quercetin has been shown to enhance seed germination and vigor (Yang et al., 2021). GRMZM2G150337 gene that codes for G protein–coupled receptor signaling pathway was significantly associated with SNP S4_239705109. The G protein–coupled receptor genes have been shown in earlier studies to enhance chilling tolerance in maize (Zhou et al., 2023). Similarly, GRMZM2G107444 that codes for response to freezing was associated with SNP S5_24157791 on chromosome 5.
The candidate gene GRMZM5G811022 associated with S6_100083188 on chromosome 6 codes for PGK activity. In activating plant defense mechanisms, protein kinases have been shown to be important in signaling during pathogen recognition. In a GWAS for NCLB resistance study, Rashid et al. (2020) identified significant SNPs on chromosome 7 that were associated with a gene coding for the protein kinase superfamily.
S3_150390803 and S8_174614781 were linked to GRMZM2G366795 and GRMZM2G118770, which codes for WRKY transcription factor 51, and oxidoreductase and malic enzyme activity, respectively. WRKY transcription factors, specific to plants, are significant regulators of the expression of genes involved in defense responses against pathogen attack (Huo et al., 2021). They also have been associated with regulating maize antioxidant defense under cadmium (Hong et al., 2017) and salt stress tolerance (Hu et al., 2021). On the other hand, malic enzymes (malate oxidoreductases), crucial in the photosynthetic C4 pathway (Ludwig, 2016; Bovdilova et al., 2019), facilitate the oxidative decarboxylation of malate into pyruvate, a reaction pivotal in several metabolic pathways. This study showed the usefulness of GWAS in revealing genomic regions associated with NCLB resistance. The identified genomic regions and candidate genes are crucial, and their validation would aid in comprehending the genetic and molecular mechanisms underlying NCLB host resistance.
NCLB is known to carry both major effect (qualitative) and minor effect (quantitative) genes, which makes it difficult to improve the resistance only based on selection of few QTLs or marker-assisted selection (Omondi et al., 2023). However, the observed wide range of heritability estimates from low to high in multiple populations (Table 1) coupled with identification of consistent QTLs across populations suggests that NCLB resistance is predominantly controlled by additive effects. Several studies on genetic analyses of NCLB resistance reported the predominance of additive effects over non-additive effects (Vivek et al., 2010; Sibiya et al., 2013; Badu-Apraku et al., 2021). These results favor improving resistance through recurrent selection. Traditional recurrent selection is time-consuming and resource-intensive, and, on the other hand, genomic selection that captures all variations from small to large effects is well for such kind of traits to improve effectively. In tropical maize, genomic selection has been used for various economically important traits (Gowda et al., 2015, 2021; Ndlovu et al., 2022; Kimutai et al., 2023; Ndlovu et al., 2024b). Predictions within a population for NCLB resistance are moderate to high (0.42 to 0.55), which is comparable to earlier reported prediction accuracies by Omondi et al. (2023). Having an independent training population like historical data or related population data and being able to predict the breeding populations is more desirable for routine application of genomic selection in breeding. Here, we tried to predict individual DH populations by combining all DH populations as a training set. The prediction accuracies are moderate (r = 0.32 to 0.49) but comparable to the square root of the heritability estimates, which is equivalent to phenotypic selection efficiency (Lorenzana and Bernardo, 2009; Kibe et al., 2020a; Omondi et al., 2023). In the tropics, the opportunity to complete three cycles per year for genomic selection enables breeders to achieve high selection gain per year. Even if we are interested in predicting genotype performance in specific environments, it is important to include additional information from other environments, which help to improve the prediction accuracy. The marker by environment effect did not show much improvement in genome prediction compared to average prediction correlations and their standard deviations obtained in both CV1 and CV2 scenario (Table 5). This kind of model performs better in conditions in which the genotype-by-environment effect is large and marker effects are different in different environments. Overall, our results suggest that it is useful to have a common training population to predict NCLB resistance in multiple linked but distinct maize populations. This also helps the breeder to improve NCLB resistance and use their resources optimally in developing multiple stress-tolerant lines and hybrids.
To dissect the genetic basis of NCLB resistance, we used linkage mapping, JLAM, GWAS, and genomic selection on the IMAS panel and biparental-based DH and F3 populations evaluated in multiple locations in Kenya. Linkage mapping in six biparental populations identified several minor- and major-effect QTLs with few overlapping across populations. JLAM identified 37 QTLs associated with NCLB resistance and many of them are co-located within the QTL detected in individual populations. Using 337,110 high-quality SNPs, GWAS identified 15 marker–trait associations. The putative candidate genes identified in the study are directly or indirectly involved in plant defense responses. However, their proposed functions require further validation to confirm the involvement of these genes in NCLB resistance. Several genomic regions were identified, which were found to be overlapping across different mapping approaches and with earlier studies. These genomic regions can serve as a potential target to improve CLB resistance. Genomic selection is a powerful methodology in plant breeding; however, its implementation is challenging because predictive model behavior depends on the specific conditions in which genome selection is used. Our results demonstrated that phenotypic selection to improve NCLB resistance under high disease pressure can be successfully supported by incorporating genomic selection in the ongoing breeding programs. Furthermore, significant variations in NCLB resistance observed across several populations imply that combining various sources of resistant alleles can be instrumental in increasing the levels of NCLB resistance in tropical maize.
The datasets presented in this study can be found in online repositories. The names of the repository/repositories and accession number(s) can be found in the article/Supplementary Material. The markers used in this study were also used in our earlier study Kibe et al., 2020a; data used in this study can be found at https://data.cimmyt.org/dataset.xhtml?persistentId=hdl%3A11529%2F10548467.
NN: Data curation, Formal analysis, Software, Validation, Writing – original draft, Writing – review & editing. MG: Conceptualization, Investigation, Methodology, Resources, Supervision, Writing – original draft, Writing – review & editing. YB: Conceptualization, Validation, Visualization, Writing – review & editing. BD: Investigation, Validation, Visualization, Writing – review & editing. SL: Methodology, Validation, Writing – review & editing. DM: Investigation, Methodology, Validation, Writing – review & editing. VO: Data curation, Formal analysis, Writing – review & editing. JB: Formal analysis, Methodology, Software, Writing – review & editing. JC: Conceptualization, Methodology, Visualization, Writing – review & editing. CS: Investigation, Resources, Validation, Writing – review & editing. PM: Methodology, Visualization, Writing – review & editing. GB: Validation, Visualization, Writing – review & editing. BP: Funding acquisition, Project administration, Resources, Supervision, Writing – review & editing.
The author(s) declare financial support was received for the research, authorship, and/or publication of this article. The success of this paper is highly attributed to the mother projects which include DTMA, WEMA, IMAS, and AG2MW (Accelerating Genetic Gains in Maize and Wheat for Improved Livelihoods, B&MGF Investment ID INV-003439) projects funded by the Bill & Melinda Gates Foundation, Foundation for Food and Agriculture Research (FFAR), and United States Agency for International Development (USAID). NN, PM, and CS acknowledge funding from the European Commission DESIRA funded LEG4DEV Project [FOOD/2020/418-901] and the Science Foundation Ireland Principal Investigator Grant [13/IA/1820].
The authors thank the CIMMYT field technicians for their support in the management of phenotypic evaluation trials and Laboratory technicians for preparing genotyping samples. We also thank Dr. Edward S Buckler and the Institute of Genomic Diversity, Cornell University, for the high-density genotyping (GBS) and imputation service.
The authors declare that the research was conducted in the absence of any commercial or financial relationships that could be construed as a potential conflict of interest.
All claims expressed in this article are solely those of the authors and do not necessarily represent those of their affiliated organizations, or those of the publisher, the editors and the reviewers. Any product that may be evaluated in this article, or claim that may be made by its manufacturer, is not guaranteed or endorsed by the publisher.
The Supplementary Material for this article can be found online at: https://www.frontiersin.org/articles/10.3389/fpls.2024.1448961/full#supplementary-material
Supplementary Table 1 | *, ** significant at P< 0.05 and 0.01, respectively; LSD, least significant difference at 5%, CV, coefficient of variation; KKM, Kakamega; KIT, Kitale.
Supplementary Table 2 | Genotypic correlations among locations for NCLB resistance in IMAS AM panel and six biparental populations. *, ** significant at P< 0.05 and 0.01, respectively; KKM, Kakamega; KIT, Kitale.
Abou-Deif, M. H., Rashed, M. A.-S., Khalil, K. M., Mahmoud, F. E.-S. (2019). Proteomic analysis of heat shock proteins in maize (Zea mays L.). Bull. Natl. Res. Centre 43, 199. doi: 10.1186/s42269-019-0251-2
Ahangar, M. A., Wani, S. H., Dar, Z. A., Roohi, J., Mohiddin, F., Bansal, M., et al. (2022). Distribution, etiology, molecular genetics and management perspectives of northern corn leaf blight of maize (Zea mays L.). Phyton (0031-9457) 91, 2111–2133. doi: 10.32604/phyton.2022.020721
Albrecht, T., Wimmer, V., Auinger, H.-J., Erbe, M., Knaak, C., Ouzunova, M., et al. (2011). Genome-based prediction of testcross values in maize. Theor. Appl. Genet. 123, 339–350. doi: 10.1007/s00122-011-1587-7
Almagro, L., Gómez Ros, L. V., Belchi-Navarro, S., Bru, R., Ros Barceló, A., Pedreño, M. A. (2008). Class III peroxidases in plant defence reactions. J. Exp. Bot. 60, 377–390. doi: 10.1093/jxb/ern277
Alvarado, G., López, M., Vargas, M., Pacheco, Á., Rodríguez, F., Burgueño, J., et al. (2015). META-R (Multi Environment Trail Analysis with R for Windows) Version 6.04. In: International, M., Wheat Improvement, C. Eds. (Mexico: CIMMYT Research Data & Software Repository Network).
Ashburner, M., Ball, C. A., Blake, J. A., Botstein, D., Butler, H., Cherry, J. M., et al. (2000). Gene ontology: tool for the unification of biology. The Gene Ontology Consortium. Nat. Genet. 25, 25–29. doi: 10.1038/75556
Badu-Apraku, B., Bankole, F. A., Fakorede, M. A. B., Ayinde, O., Ortega-Beltran, A. (2021). Genetic analysis of grain yield and resistance of extra-early maturing maize inbreds to northern corn leaf blight. Crop Sci. 61, 1864–1880. doi: 10.1002/csc2.20479
Bankole, F. A., Badu-Apraku, B., Salami, A. O., Falade, T. D., Bandyopadhyay, R., Ortega-Beltran, A. (2023). Variation in the morphology and effector profiles of Exserohilum turcicum isolates associated with the Northern Corn Leaf Blight of maize in Nigeria. BMC Plant Biol. 23, 386. doi: 10.1186/s12870-023-04385-7
Bateman, A., Coin, L., Durbin, R., Finn, R. D., Hollich, V., Griffiths-Jones, S., et al. (2004). The Pfam protein families database. Nucleic Acids Res. 32, D138–D141. doi: 10.1093/nar/gkh121
Benson, J. M., Poland, J. A., Benson, B. M., Stromberg, E. L., Nelson, R. J. (2015). Resistance to gray leaf spot of maize: genetic architecture and mechanisms elucidated through nested association mapping and near-isogenic line analysis. PloS Genet. 11, e1005045. doi: 10.1371/journal.pgen.1005045
Bentolila, S., Guitton, C., Bouvet, N., Sailland, A., Nykaza, S., Freyssinet, G. (1991). Identification of an RFLP marker tightly linked to the Ht1 gene in maize. Theor. Appl. Genet. 82, 393–398. doi: 10.1007/BF00588588
Berka, M., Kopecká, R., Berková, V., Brzobohatý, B., Černý, M. (2022). Regulation of heat shock proteins 70 and their role in plant immunity. J. Exp. Bot. 73, 1894–1909. doi: 10.1093/jxb/erab549
Beyene, Y., Gowda, M., Suresh, L. M., Mugo, S., Olsen, M., Oikeh, S. O., et al. (2017). Genetic analysis of tropical maize inbred lines for resistance to maize lethal necrosis disease. Euphytica 213, 224. doi: 10.1007/s10681-017-2012-3
Boddupalli, P., Suresh, L., Mwatuni, F., Beyene, Y., Makumbi, D., Gowda, M., et al. (2020). Maize lethal necrosis (MLN): Efforts toward containing the spread and impact of a devastating transboundary disease in sub-Saharan Africa. Virus Res. 282, 197943. doi: 10.1016/j.virusres.2020.197943
Bovdilova, A., Alexandre, B. M., Höppner, A., Luis, I. M., Alvarez, C. E., Bickel, D., et al. (2019). Posttranslational modification of the NADP-malic enzyme involved in C4 photosynthesis modulates the enzymatic activity during the day. Plant Cell 31, 2525–2539. doi: 10.1105/tpc.19.00406
Bradbury, P. J., Zhang, Z., Kroon, D. E., Casstevens, T. M., Ramdoss, Y., Buckler, E. S. (2007). TASSEL: software for association mapping of complex traits in diverse samples. Bioinformatics 23, 2633–2635. doi: 10.1093/bioinformatics/btm308
Burgueño, J., Crossa, J., Cornelius, P. L., Yang, R. C. (2008). Using factor analytic models for joining environments and genotypes without crossover genotype × environment interaction. Crop Science. 48, 1–15. doi: 10.2135/cropsci2007.11.0632
Burgueño, J., de los Campos, G., Weigel, K., Crossa, J. (2012). Genomic prediction of breeding values when modeling genotype × environment interaction using pedigree and dense molecular markers. Crop Sci. 52, 707–719. doi: 10.2135/cropsci2011.06.0299
Cao, S., Loladze, A., Yuan, Y., Wu, Y., Zhang, A., Chen, J., et al. (2017). Genome-wide analysis of tar spot complex resistance in maize using genotyping-by-sequencing SNPs and whole-genome prediction. Plant Genome 10, 1–14. doi: 10.3835/plantgenome2016.10.0099
Cao, Z., Zhang, K., Guo, X., Turgeon, B. G., Dong, J. (2020). A genome resource of setosphaeria turcica, causal agent of northern leaf blight of maize. Phytopathology 110, 2014–2016. doi: 10.1094/PHYTO-06-20-0225-A
Carson, M. (1995). A new gene in maize conferring the” chlorotic halo” reaction to infection by Exserohilum turcicum. Plant Dis. 79 (7), 717–720. doi: 10.1094/PD-79-0717
Carson, M. L., Dyke, C. G. (1994). Effect of light and temperature on expression of partial resistance of maize to Exserohilum turcicum. Plant Dis. 78 (5), 519–522. doi: 10.1094/PD-78-0519
Chen, G., Wang, X., Long, S., Jaqueth, J., Li, B., Yan, J., et al. (2016). Mapping of QTL conferring resistance to northern corn leaf blight using high-density SNPs in maize. Mol. Breed. 36, 1–9. doi: 10.1007/s11032-015-0421-3
Chung, C.-L., Jamann, T., Longfellow, J., Nelson, R. (2010). Characterization and fine-mapping of a resistance locus for northern leaf blight in maize bin 8.06. Theor. Appl. Genet. 121, 205–227. doi: 10.1007/s00122-010-1303-z
Cooper, J. S., Rice, B. R., Shenstone, E. M., Lipka, A. E., Jamann, T. M. (2019). Genome-wide analysis and prediction of resistance to goss’s wilt in maize. Plant Genome 12, 180045. doi: 10.3835/plantgenome2018.06.0045
Crossa, J., Pérez-Rodríguez, P., Cuevas, J., Montesinos-López, O., Jarquín, D., De Los Campos, G., et al. (2017). Genomic selection in plant breeding: methods, models, and perspectives. Trends Plant Sci. 22, 961–975. doi: 10.1016/j.tplants.2017.08.011
Das, B., Atlin, G. N., Olsen, M., Burgueño, J., Tarekegne, A., Babu, R., et al. (2019). Identification of donors for low-nitrogen stress with maize lethal necrosis (MLN) tolerance for maize breeding in sub-Saharan Africa. Euphytica 215, 1–15. doi: 10.1007/s10681-019-2406-5
De Groote, H., Oloo, F., Tongruksawattana, S., Das, B. (2016). Community-survey based assessment of the geographic distribution and impact of maize lethal necrosis (MLN) disease in Kenya. Crop Prot. 82, 30–35. doi: 10.1016/j.cropro.2015.12.003
De Rossi, R. L., Guerra, F. A., Plazas, M. C., Vuletic, E. E., Brücher, E., Guerra, G. D., et al. (2022). Crop damage, economic losses, and the economic damage threshold for northern corn leaf blight. Crop Prot. 154, 105901. doi: 10.1016/j.cropro.2021.105901
Ding, J., Ali, F., Chen, G., Li, H., Mahuku, G., Yang, N., et al. (2015). Genome-wide association mapping reveals novel sources of resistance to northern corn leaf blight in maize. BMC Plant Biol. 15, 1–11. doi: 10.1186/s12870-015-0589-z
Dingerdissen, A., Geiger, H., Lee, M., Schechert, A., Welz, H. (1996). Interval mapping of genes for quantitative resistance of maize to Setosphaeria turcica, cause of northern leaf blight, in a tropical environment. Mol. Breed. 2, 143–156. doi: 10.1007/BF00441429
Diogo-Jr, R., de Resende Von Pinho, E. V., Pinto, R. T., Zhang, L., Condori-Apfata, J. A., Pereira, P. A., et al. (2023). Maize heat shock proteins—prospection, validation, categorization and in silico analysis of the different ZmHSP families. Stress Biol. 3, 37. doi: 10.1007%2Fs44154-023-00104-2
Elshire, R. J., Glaubitz, J. C., Sun, Q., Poland, J. A., Kawamoto, K., Buckler, E. S., et al. (2011). A robust, simple genotyping-by-sequencing (GBS) approach for high diversity species. PloS One 6, e19379. doi: 10.1371/journal.pone.0019379
Endelman, J. B. (2011). Ridge regression and other kernels for genomic selection with R package rrBLUP. Plant Genome 43, 250–255. doi: 10.3835/plantgenome2011.08.0024
Ertiro, B. T., Labuschagne, M., Olsen, M., Das, B., Prasanna, B. M., Gowda, M. (2020a). Genetic dissection of nitrogen use efficiency in tropical maize through genome-wide association and genomic prediction. Front. Plant Sci. 11, 474. doi: 10.3389/fpls.2020.00474
Ertiro, B. T., Olsen, M., Das, B., Gowda, M., Labuschagne, M. (2020b). Efficiency of indirect selection for grain yield in maize (Zea mays L.) under low nitrogen conditions through secondary traits under low nitrogen and grain yield under optimum conditions. Euphytica 216, 1–12. doi: 10.1007/s10681-020-02668-w
Freymark, P., Lee, M., Woodman, W., Martinson, C. (1993). Quantitative and qualitative trait loci affecting host-plant response to Exserohilum turcicum in maize (Zea mays L.). Theor. Appl. Genet. 87, 537–544. doi: 10.1007/BF00221876
Galiano-Carneiro, A. L., Kessel, B., Presterl, T., Miedaner, T. (2021). Intercontinental trials reveal stable QTL for Northern corn leaf blight resistance in Europe and in Brazil. Theor. Appl. Genet. 134, 63–79. doi: 10.1007/s00122-020-03682-1
Galiano-Carneiro, A. L., Miedaner, T. (2017). Genetics of resistance and pathogenicity in the maize/Setosphaeria turcica pathosystem and implications for breeding. Front. Plant Sci. 8, 1490. doi: 10.3389/fpls.2017.01490
Gilmour, A., Gogel, B., Cullis, B., Welham, S., Thompson, R. (2015). ASReml User Guide Release 4.1 Structural Specification, VSN International Ltd, Hemel Hempstead, HP1 1ES, UK. Available at: www.vsni.co.uk.
Gowda, M., Beyene, Y., Makumbi, D., Semagn, K., Olsen, M. S., Bright, J. M., et al. (2018). Discovery and validation of genomic regions associated with resistance to maize lethal necrosis in four biparental populations. Mol. Breed. 38, 1–16. doi: 10.1007/s11032-018-0829-7
Gowda, M., Das, B., Makumbi, D., Babu, R., Semagn, K., Mahuku, G., et al. (2015). Genome-wide association and genomic prediction of resistance to maize lethal necrosis disease in tropical maize germplasm. Theor. Appl. Genet. 128, 1957–1968. doi: 10.1007/s00122-015-2559-0
Gowda, M., Makumbi, D., Das, B., Nyaga, C., Kosgei, T., Crossa, J., et al. (2021). Genetic dissection of Striga hermonthica (Del.) Benth. resistance via genome-wide association and genomic prediction in tropical maize germplasm. Theor. Appl. Genet. 134, 941–958. doi: 10.1007/s00122-020-03744-4
Han, S., Utz, H. F., Liu, W., Schrag, T. A., Stange, M., Würschum, T., et al. (2016). Choice of models for QTL mapping with multiple families and design of the training set for prediction of Fusarium resistance traits in maize. Theor. Appl. Genet. 129, 431–444. doi: 10.1007/s00122-015-2637-3
Heffner, E. L., Jannink, J., Sorrells, M. E. (2011). Genomic selection accuracy using multifamily prediction models in a wheat breeding program. Plant Genome. 4, 65–75. doi: 10.3835/plantgenome.2010.12.0029
Heslot, N., Akdemir, D., Sorrells, M. E., Jannink, J. L. (2014). Integrating environmental covariates and crop modeling into the genomic selection framework to predict genotype by environment interactions. Theor. Appl. Genet. 127, 463–480. doi: 10.1007/s00122-013-2231-5
Hong, C., Cheng, D., Zhang, G., Zhu, D., Chen, Y., Tan, M. (2017). The role of ZmWRKY4 in regulating maize antioxidant defense under cadmium stress. Biochem. Biophys. Res. Commun. 482, 1504–1510. doi: 10.1016/j.bbrc.2016.12.064
Hooda, K., Khokhar, M., Shekhar, M., Karjagi, C. G., Kumar, B., Mallikarjuna, N., et al. (2017). Turcicum leaf blight—sustainable management of a re-emerging maize disease. J. Plant Dis. Prot. 124, 101–113. doi: 10.1007/s41348-016-0054-8
Hu, W., Ren, Q., Chen, Y., Xu, G., Qian, Y. (2021). Genome-wide identification and analysis of WRKY gene family in maize provide insights into regulatory network in response to abiotic stresses. BMC Plant Biol. 21, 427. doi: 10.1186/s12870-021-03206-z
Huo, T., Wang, C.-T., Yu, T.-F., Wang, D.-M., Li, M., Zhao, D., et al. (2021). Overexpression of ZmWRKY65 transcription factor from maize confers stress resistances in transgenic Arabidopsis. Sci. Rep. 11, 4024. doi: 10.1038/s41598-021-83440-5
Jamann, T. M., Poland, J. A., Kolkman, J. M., Smith, L. G., Nelson, R. J. (2014). Unraveling genomic complexity at a quantitative disease resistance locus in maize. Genetics 198, 333–344. doi: 10.1534/genetics.114.167486
Jarquín, D., Crossa, J., Lacaze, X., Du, C. P., Daucourt, J., Lorgeou, J., et al. (2014). A reaction norm model for genomic selection using high-dimensional genomic and environmental data. Theor. Appl. Genet. 127, 595–607. doi: 10.1007/s00122-013-2243-1
Jindal, K. K., Tenuta, A. U., Woldemariam, T., Zhu, X., Hooker, D. C., Reid, L. M. (2019). Occurrence and distribution of physiological races of Exserohilum turcicum in Ontario, Canada. Plant Dis. 103, 1450–1457. doi: 10.1094/PDIS-06-18-0951-SR
Kaefer, K. A. C., Schuelter, A. R., Schuster, I., Marcolin, J., Vendruscolo, E. C. G. (2017). Association mapping and genetic control for northern leaf blight (‘Exserohilum turcicum’) resistance in maize lines. Aust. J. Crop Sci. 11, 1346–1353. doi: 10.21475/ajcs.17.11.10.pne678
Kanehisa, M., Goto, S. (2000). KEGG: kyoto encyclopedia of genes and genomes. Nucleic Acids Res. 28, 27–30. doi: 10.1093/nar/28.1.27
Kansiime, M. K., Rwomushana, I., Mugambi, I. (2023). Fall armyworm invasion in Sub-Saharan Africa and impacts on community sustainability in the wake of Coronavirus Disease 2019: reviewing the evidence. Curr. Opin. Environ. Sustainability 62, 101279. doi: 10.1016/j.cosust.2023.101279
Kibe, M., Nair, S. K., Das, B., Bright, J. M., Makumbi, D., Kinyua, J., et al. (2020a). Genetic dissection of resistance to gray leaf spot by combining genome-wide association, linkage mapping, and genomic prediction in tropical maize germplasm. Front. Plant Sci. 11. doi: 10.3389/fpls.2020.572027
Kibe, M., Nyaga, C., Nair, S. K., Beyene, Y., Das, B., Bright, J. M., et al. (2020b). Combination of linkage mapping, GWAS, and GP to dissect the genetic basis of common rust resistance in tropical maize germplasm. Int. J. Mol. Sci. 21, 6518. doi: 10.3390/ijms21186518
Kimutai, C., Ndlovu, N., Chaikam, V., Ertiro, B. T., Das, B., Beyene, Y., et al. (2023). Discovery of genomic regions associated with grain yield and agronomic traits in Bi-parental populations of maize (Zea mays. L) Under optimum and low nitrogen conditions. Front. Genet. 14. doi: 10.3389/fgene.2023.1266402
Knapp, S., Stroup, W., Ross, W. (1985). Exact confidence intervals for heritability on a progeny mean basis 1. Crop Sci. 25, 192–194. doi: 10.2135/cropsci1985.0011183X002500010046x
Kosambi, D. D. (1944). The estimation of map distance from recombination values. Ann Eugen. 12, 172–175. doi: 10.1111/j.1469-1809.1943.tb02321.x
Lehmensiek, A., Esterhuizen, A., Van Staden, D., Nelson, S., Retief, A. (2001). Genetic mapping of gray leaf spot (GLS) resistance genes in maize. Theor. Appl. Genet. 103, 797–803. doi: 10.1007/s001220100599
Li, S. (2023). Novel insight into functions of ascorbate peroxidase in higher plants: More than a simple antioxidant enzyme. Redox Biol. 64, 102789. doi: 10.1016/j.redox.2023.102789
Li, Y.-x., Chen, L., Li, C., Bradbury, P. J., Shi, Y.-S., Song, Y., et al. (2018). Increased experimental conditions and marker densities identified more genetic loci associated with southern and northern leaf blight resistance in maize. Sci. Rep. 8, 6848. doi: 10.1038/s41598-018-25304-z
Liu, W., Gowda, M., Steinhoff, J., Maurer, H. P., Würschum, T., Longin, C. F. H., et al. (2011). Association mapping in an elite maize breeding population. Theor. Appl. Genet. 123, 847–858. doi: 10.1007/s00122-011-1631-7
Liu, X., Huang, M., Fan, B., Buckler, E. S., Zhang, Z. (2016). Iterative usage of fixed and random effect models for powerful and efficient genome-wide association studies. PloS Genet. 12, e1005767. doi: 10.1371/journal.pgen.1005767
Lopez-Cruz, M., Crossa, J., Bonnett, D., Dreisigacker, S., Poland, J., Jannink, J.-L., et al. (2015). Increased prediction accuracy in wheat breeding trials using a marker × environment interaction genomic selection model. G3: Genes Genomes Genet. 5, 569–582. doi: 10.1534/g3.114.016097
Lorenzana, R. E., Bernardo, R. (2009). Accuracy of genotypic value predictions for marker-based selection in biparental plant populations. Theor. Appl. Genet. 120, 151–161. doi: 10.1007/s00122-009-1166-3
Ludwig, M. (2016). The roles of organic acids in C4 photosynthesis. Front. Plant Sci. 7, 647. doi: 10.3389/fpls.2016.00647
Mayer, M., Bukau, B. (2005). Hsp70 chaperones: cellular functions and molecular mechanism. Cell. Mol. Life Sci. 62, 670–684. doi: 10.1007/s00018-004-4464-6
Meng, L., Li, H., Zhang, L., Wang, J. (2015). QTL IciMapping: integrated software for genetic linkage map construction and quantitative trait locus mapping in biparental populations. Crop J. 3, 269–283. doi: 10.1016/j.cj.2015.01.001
Meuwissen, T. H., Hayes, B. J., Goddard, M. (2001). Prediction of total genetic value using genome-wide dense marker maps. genetics 157, 1819–1829. doi: 10.1093/genetics/157.4.1819
Miedaner, T., Boeven, A. L. G.-C., Gaikpa, D. S., Kistner, M. B., Grote, C. P. (2020). Genomics-assisted breeding for quantitative disease resistances in small-grain cereals and maize. Int. J. Mol. Sci. 21, 9717. doi: 10.3390/ijms21249717
Muiru, W., Mutitu, E., Kimenju, J. (2007). Reaction of some Kenyan maize genotypes to Turcicum leaf blight under greenhouse and field conditions. Asian J. Plant Sci. 6, 1190–1196. doi: 10.3923/ajps.2007.1190.1196
Ndlovu, N., Gowda, M. S., Beyene, Y., Chaikam, V., Nzuve, F., Makumbi, D., et al. (2024a). Genomic loci associated with grain yield under well-watered and waterstressed conditions in multiple bi-parental maize populations. Front. Sustain. Food Syst. 8, 1391989. doi: 10.3389/fsufs.2024.1391989
Ndlovu, N., Kachapur, R. M., Beyene, Y., Das, B., Makumbi, D., Spillane, C., et al. (2024b). Linkage mapping and genomic prediction of grain quality traits in tropical maize (Zea mays L.). Front. Genet. 15, 1353289. doi: 10.3389/fgene.2024.1353289
Ndlovu, N., Spillane, C., McKeown, P. C., Cairns, J. E., Das, B., Gowda, M. (2022). Genome-wide association studies of grain yield and quality traits under optimum and low-nitrogen stress in tropical maize (Zea mays L.). Theor. Appl. Genet. 135, 4351–4370. doi: 10.1007/s00122-022-04224-7
Nuss, E. T., Tanumihardjo, S. A. (2010). Maize: a paramount staple crop in the context of global nutrition. Compr. Rev. Food Sci. Food Saf. 9, 417–436. doi: 10.1111/j.1541-4337.2010.00117.x
Ogliari, J. B., Guimarães, M. A., Camargo, L. E. A. (2007). Chromosomal locations of the maize (Zea mays L.) HtP and rt genes that confer resistance to Exserohilum turcicum. Genet. Mol. Biol. 30, 630–634. doi: 10.1590/S1415-47572007000400021
Ogliari, J. B., Guimarães, M. A., Geraldi, I. O., Camargo, L. E. A. (2005). New resistance genes in the Zea mays: Exserohilum turcicum pathosystem. Genet. Mol. Biol. 28, 435–439. doi: 10.1590/S1415-47572005000300017
Omondi, D. O., Dida, M. M., Berger, D. K., Beyene, Y., Nsibo, D. L., Juma, C., et al. (2023). Combination of linkage and association mapping with genomic prediction to infer QTL regions associated with gray leaf spot and northern corn leaf blight resistance in tropical maize. Front. Genet. 14, 1282673. doi: 10.3389/fgene.2023.1282673
Park, C.-J., Seo, Y.-S. (2015). Heat shock proteins: a review of the molecular chaperones for plant immunity. Plant Pathol. J. 31, 323. doi: 10.5423/PPJ.RW.08.2015.0150
Pataky, J., Ledencan, T. (2006). Resistance conferred by the Ht1 gene in sweet corn infected by mixtures of virulent and avirulent Exserohilum turcicum. Plant Dis. 90, 771–776. doi: 10.1094/PD-90-0771
Pataky, J., Raid, R., Du Toit, L., Schueneman, T. (1998). Disease severity and yield of sweet corn hybrids with resistance to northern leaf blight. Plant Dis. 82, 57–63. doi: 10.1094/PDIS.1998.82.1.57
Poland, J. A., Bradbury, P. J., Buckler, E. S., Nelson, R. J. (2011). Genome-wide nested association mapping of quantitative resistance to northern leaf blight in maize. Proc. Natl. Acad. Sci. 108, 6893–6898. doi: 10.1073/pnas.1010894108
Prasanna, B., Nair, S. K., Babu, R., Gowda, M., Zhang, X., Xu, Y., et al. (2020). “Increasing genetic gains in maize in stress-prone environments of the tropics,” in Kole, C. Eds. Genomic Designing of Climate-Smart Cereal Crops (Cham: Springer), 97–132. doi: 10.1007/978-3-319-93381-8_3
R_Core_Team (2023). R: A Language and Environment for Statistical Computing (Vienna, Austria: R Foundation for Statistical Computing). Available at: https://www.R-project.org/.
Ramathani, I., Biruma, M., Martin, T., Dixelius, C., Okori, P. (2011). Disease severity, incidence and races of Setosphaeria turcica on sorghum in Uganda. Eur. J. Plant Pathol. 131, 383–392. doi: 10.1007/s10658-011-9815-1
Ranganatha, H. M., Lohithaswa, H. C., Pandravada, A. (2021). Mapping and validation of major quantitative trait loci for resistance to northern corn leaf blight along with the determination of the relationship between resistances to multiple foliar pathogens of maize (Zea mays L.). Front. Genet. 11, 548407. doi: 10.3389/fgene.2020.548407
Rashid, Z., Singh, P. K., Vemuri, H., Zaidi, P. H., Prasanna, B. M., Nair, S. K. (2018). Genome-wide association study in Asia-adapted tropical maize reveals novel and explored genomic regions for sorghum downy mildew resistance. Sci. Rep. 8, 366. doi: 10.1038/s41598-017-18690-3
Rashid, Z., Sofi, M., Harlapur, S. I., Kachapur, R. M., Dar, Z. A., Singh, P. K., et al. (2020). Genome-wide association studies in tropical maize germplasm reveal novel and known genomic regions for resistance to Northern corn leaf blight. Sci. Rep. 10, 21949. doi: 10.1038/s41598-020-78928-5
Rawlings, J. O., Pantula, S. G., Dickey, D. A. (1998). Applied regression analysis: a research tool (New York: Springer).
Raymundo, A., Hooker, A. (1981). Measuring the relationship between northern corn leaf blight and yield losses. Plant Dis. 65, 325–327. doi: 10.1094/PD-65-325
Razzaq, T., Khan, M. F., Awan, S. I. (2019). Study of Northern Corn Leaf Blight (NCLB) on Maize (Zea mays L.) Genotypes and its Effect on Yield. Sarhad J. Agric. 35, 1164–1174. doi: 10.17582/journal.sja/2019/35.4.1166.1174
Reif, J. C., Liu, W., Gowda, M., Maurer, H. P., Mohring, J., Fischer, S., et al. (2010). Genetic basis of agronomically important traits in sugar beet (Beta vulgaris L.) investigated with joint linkage association mapping. Theor. Appl. Genet. 121, 1489–1499. doi: 10.1007/s00122-010-1405-7
SAS Institute (2015) SAS statistical software ver. 9.4, cary, North Carolina. Available at: https://www.sas.com/en_ie/software/viya.html.
Schwarz, G. (1978). Estimating the dimension of a model. Ann. Stat. 6 (2), 461–464. doi: 10.1214/aos/1176344136
Sharma, S., Carena, M. (2016). Grain quality in Maize (Zea mays L.): Breeding implications for short-season drought environments. Euphytica 212, 247–260. doi: 10.1007/s10681-016-1764-5
Sibiya, J., Tongoona, P., Derera, J., Makanda, I. (2013). Smallholder farmers’ perceptions of maize diseases, pests, and other production constraints, their implications for maize breeding and evaluation of local maize cultivars in KwaZulu-Natal, South Africa. Afr. J. Agric. Res. 8, 1790–1798. doi: 10.5897/AJAR12.1906
Singh, N., Bhatla, S. C. (2022). Heme oxygenase-nitric oxide crosstalk-mediated iron homeostasis in plants under oxidative stress. Free Radical Biol. Med. 182, 192–205. doi: 10.1016/j.freeradbiomed.2022.02.034
Sitonik, C., Suresh, L., Beyene, Y., Olsen, M. S., Makumbi, D., Oliver, K., et al. (2019). Genetic architecture of maize chlorotic mottle virus and maize lethal necrosis through GWAS, linkage analysis and genomic prediction in tropical maize germplasm. Theor. Appl. Genet. 132, 2381–2399. doi: 10.1007/s00122-019-03360-x
St. Clair, D. A. (2010). Quantitative disease resistance and quantitative resistance loci in breeding. Annu. Rev. Phytopathol. 48, 247–268. doi: 10.1146/annurev-phyto-080508-081904
Tang, Y., Liu, X., Wang, J., Li, M., Wang, Q., Tian, F., et al. (2016). GAPIT version 2: An enhanced integrated tool for genomic association and prediction. Plant Genome 9 (2). doi: 10.3835/plantgenome2015.11.0120
Technow, F., Bürger, A., Melchinger, A. E. (2013). Genomic prediction of northern corn leaf blight resistance in maize with combined or separated training sets for heterotic groups. G3: Genes Genomes Genet. 3, 197–203. doi: 10.1534/g3.112.004630
Van Inghelandt, D., Melchinger, A. E., Martinant, J.-P., Stich, B. (2012). Genome-wide association mapping of flowering time and northern corn leaf blight (Setosphaeria turcica) resistance in a vast commercial maize germplasm set. BMC Plant Biol. 12, 1–15. doi: 10.1186/1471-2229-12-56
Vieira, R. A., Tessmann, D. J., Scapim, C. A., Hata, F. T., de Araújo Rodovalho, M., Barreto, R. R. (2009). Genetic resistance of new popcorn hybrids to foliar diseases. Crop Breed. Appl. Biotechnol. 9 (2), 140–146. doi: 10.12702/1984-7033.v09n02a06
Vivek, B. S., Odongo, O., Njuguna, J., Imanywoha, J., Bigirwa, G., Diallo, A., et al. (2010). Diallel analysis of grain yield and resistance to seven diseases of 12 African maize (Zea mays L.) inbred lines. Euphytica 172, 329–340. doi: 10.1007/s10681-009-9993-5
Wang, Y., Mu, C.-h., Li, X.-d., Duan, C.-x., Wang, J.-j., Lu, X., et al. (2023). Genome-wide association and transcriptome reveal genetic basis for Southern Corn Rust in maize. J. Integr. Agric. doi: 10.1016/j.jia.2023.10.039
Wang, J., Xu, Z., Yang, J., et al. (2018). qNCLB7.02, a novel qtl for resistance to northern corn leaf blight in maize. Mol. Breed. doi: 10.1007/s11032-017-0770-1
Weems, J. D. (2016). Evaluation of race population distribution, fungicide sensitivity, and fungicide control of Exserohilum turcicum, the causal agent of northern leaf blight of corn (Urbana, Illinois: University of Illinois).
Welz, H., Geiger, H. (2000). Genes for resistance to northern corn leaf blight in diverse maize populations. Plant Breed. 119, 1–14. doi: 10.1046/j.1439-0523.2000.00462.x
Welz, H., Schechert, A., Geiger, H. (1999). Dynamic gene action at QTLs for resistance to Setosphaeria turcica in maize. Theor. Appl. Genet. 98, 1036–1045. doi: 10.1007/s001220051165
Wende, A., Shimelis, H., Gwata, E. T. (2018). Genetic variability for resistance to leaf blight and diversity among selected maize inbred lines. In: El-Esawi, M. A. Eds. Maize germplasm-characterization and genetic approaches for crop improvement. London, UK: IntechOpen Limited, p. 39–56. doi: 10.5772/intechopen.70553
Wisser, R. J., Balint-Kurti, P. J., Nelson, R. J. (2006). The genetic architecture of disease resistance in maize: a synthesis of published studies. Phytopathology 96, 120–129. doi: 10.1094/PHYTO-96-0120
Wu, Y., Li, J., Wang, J., Dawuda, M. M., Liao, W., Meng, X., et al. (2022). Heme is involved in the exogenous ALA-promoted growth and antioxidant defense system of cucumber seedlings under salt stress. BMC Plant Biol. 22, 329. doi: 10.1186/s12870-022-03717-3
Würschum, T. (2012). Mapping QTL for agronomic traits in breeding populations. Theor. Appl. Genet. 125, 201–210. doi: 10.1007/s00122-012-1887-6
Xiao, W., Zhao, J., Fan, S., Li, L., Dai, J., Xu, M. (2007). Mapping of genome-wide resistance gene analogs (RGAs) in maize (Zea mays L.). Theor. Appl. Genet. 115, 501–508. doi: 10.1007/s00122-007-0583-4
Xu, Y., Crouch, J. H. (2008). Marker-assisted selection in plant breeding: From publications to practice. Crop Sci. 48, 391–407. doi: 10.2135/cropsci2007.04.0191
Yang, Q., Balint-Kurti, P., Xu, M. (2017). Quantitative disease resistance: dissection and adoption in maize. Mol. Plant 10, 402–413. doi: 10.1016/j.molp.2017.02.004
Yang, J., Zhang, L., Jiang, L., Zhan, Y. G., Fan, G. Z. (2021). Quercetin alleviates seed germination and growth inhibition in Apocynum venetum and Apocynum pictum under mannitol-induced osmotic stress. Plant Physiol. Biochem. 159, 268–276. doi: 10.1016/j.plaphy.2020.12.025
Yanti, Y. (2015). Peroxidase enzyme activity of rhizobacteria-introduced shallots bulbs to induce resistance of shallot towards bacterial leaf blight (Xanthomonas axonopodis pv allii). Proc. Chem. 14, 501–507. doi: 10.1016/j.proche.2015.03.067
Yin, X., Wang, Q., Yang, J., Jin, D., Wang, F., Wang, B., et al. (2003). Fine mapping of the Ht2 (Helminthosporium turcicum resistance 2) gene in maize. Chin. Sci. Bull. 48, 165–169. doi: 10.1360/03tb9034
Yu, J., Pressoir, G., Briggs, W. H., Vroh Bi, I., Yamasaki, M., Doebley, J. F., et al. (2006). A unified mixed-model method for association mapping that accounts for multiple levels of relatedness. Nat. Genet. 38, 203–208. doi: 10.1038/ng1702
Zaitlin, D., DeMars, S., Ma, Y. (1993). Linkage of rhm, a recessive gene for resistance to southern corn leaf blight, to RFLP marker loci in maize (Zea mays) seedlings. Genome 36, 555–564. doi: 10.1139/g93-076
Zhai, R., Huang, A., Mo, R., Zou, C., Wei, X., Yang, M., et al. (2022). SNP-based bulk segregant analysis revealed disease resistance QTLs associated with northern corn leaf blight in maize. Front. Genet. 13, 1038948. doi: 10.3389/fgene.2022.1038948
Zhang, Y., Liu, P., Zhang, X., Zheng, Q., Chen, M., Ge, F., et al. (2018). Multi-locus genome-wide association study reveals the genetic architecture of stalk lodging resistance-related traits in maize. Front. Plant Sci. 9, 611. doi: 10.3389/fpls.2018.00611
Zhao, Y., Gowda, M., Liu, W., Würschum, T., Maurer, H. P., Longin, F. H., et al. (2012). Accuracy of genomic selection in european maize elite breeding populations. Theor. Appl. Genet. 124, 769–776. doi: 10.1007/s00122-011-1745-y
Keywords: northern corn leaf blight (NCLB), genome-wide association study (GWAS), quantitative trait locus (QTL) mapping, genomic selection (GS), tropical maize, sub-Saharan Africa (SSA)
Citation: Ndlovu N, Gowda M, Beyene Y, Das B, Mahabaleswara SL, Makumbi D, Ogugo V, Burgueno J, Crossa J, Spillane C, McKeown PC, Brychkova G and Prasanna BM (2024) A combination of joint linkage and genome-wide association study reveals putative candidate genes associated with resistance to northern corn leaf blight in tropical maize. Front. Plant Sci. 15:1448961. doi: 10.3389/fpls.2024.1448961
Received: 14 June 2024; Accepted: 05 September 2024;
Published: 03 October 2024.
Edited by:
Leonardo Velasco, Spanish National Research Council (CSIC), SpainReviewed by:
Arfang Badji, National Crops Resources Research Institute (NaCRRI), UgandaCopyright © 2024 Ndlovu, Gowda, Beyene, Das, Mahabaleswara, Makumbi, Ogugo, Burgueno, Crossa, Spillane, McKeown, Brychkova and Prasanna. This is an open-access article distributed under the terms of the Creative Commons Attribution License (CC BY). The use, distribution or reproduction in other forums is permitted, provided the original author(s) and the copyright owner(s) are credited and that the original publication in this journal is cited, in accordance with accepted academic practice. No use, distribution or reproduction is permitted which does not comply with these terms.
*Correspondence: Manje Gowda, bS5nb3dkYUBjZ2lhci5vcmc=
†ORCID: Noel Ndlovu, orcid.org/0000-0002-1832-0251
Manje Gowda, orcid.org/0000-0003-4434-6364
Yoseph Beyene, orcid.org/0000-0001-7221-2617
Biswanath Das, orcid.org/0000-0003-2928-5030
Suresh L.M., orcid.org/0000-0001-6438-6502
Dan Makumbi, orcid.org/0000-0002-1801-5986
Veronica Ogugo, orcid.org/0000-0003-1067-9283
Juan Burgueno, orcid.org/0000-0002-1468-4867
Jose Crossa, orcid.org/0000-0001-9429-5855
Charles Spillane, orcid.org/0000-0003-3318-323X
Peter C. McKeown, orcid.org/0000-0002-7255-6062
Galina Brychkova, orcid.org/0000-0003-2452-7635
Boddupalli M. Prasanna, orcid.org/0000-0002-5761-2273
Disclaimer: All claims expressed in this article are solely those of the authors and do not necessarily represent those of their affiliated organizations, or those of the publisher, the editors and the reviewers. Any product that may be evaluated in this article or claim that may be made by its manufacturer is not guaranteed or endorsed by the publisher.
Research integrity at Frontiers
Learn more about the work of our research integrity team to safeguard the quality of each article we publish.