- Maize Research Institute/State Key Laboratory of Crop Gene Exploration and Utilization in Southwest China, Sichuan Agricultural University, Chengdu, Sichuan, China
When genomic prediction is implemented in breeding maize (Zea mays L.), it can accelerate the breeding process and reduce cost to a large extent. In this study, 11 yield-related traits of maize were used to evaluate four genomic prediction methods including rrBLUP, HEBLP|A, RF, and LightGBM. In all the 11 traits, rrBLUP had similar predictive accuracy to HEBLP|A, and so did RF to LightGBM, but rrBLUP and HEBLP|A outperformed RF and LightGBM in 8 traits. Furthermore, genomic prediction-based heterotic pattern of yield was established based on 64620 crosses of maize in Southwest China, and the result showed that one of the parent lines of the top 5% crosses came from temp-tropic or tropic germplasm, which is highly consistent with the actual situation in breeding, and that heterotic pattern (Reid+ × Suwan+) will be a major heterotic pattern of Southwest China in the future.
Introduction
Maize (Zea mays L.), one of the most important cereal crops throughout the world, has been widely used as food, biofuel, feed and raw materials of many industrial products (Yang et al., 2011a). In order to increase maize production, molecular marker techniques such as RFLP, SSR, and SNP have been developed to improve agronomic traits of economic importance (Stevens, 2008; Prasanna et al., 2010; Inghelandt et al., 2010). However, conventional marker-assisted selection (MAS) can only utilize the markers tightly linked to large or moderate-effect QTL and fails to deal with quantitative traits controlled by minor-polygene (Heffner et al., 2009; Jannink et al., 2010; Chai et al., 2012; Hao et al., 2014).
Meuwissen et al. (2001) pioneered the technique of genomic prediction to solve this problem. First, training population with genotypic and phenotypic information is utilized to compute genetic effect of each marker at the level of genome-wide markers, and then the genomic estimated breeding values (GEBV) of each individual in candidate population with only genotypic information are obtained. The fact that all marker information is included in genomic prediction contributes to higher predictive accuracy and genetic gain. Applied successfully in the breeding programs of animals and plants such as dairy cattle, beef cattle, pigs, sheep, maize, wheat, and rice (Hayes et al., 2009; Heffner et al., 2011; Meuwissen et al., 2013; Fristche-Neto et al., 2018; Cui et al., 2020; Alemu et al., 2024), many genomic prediction methods have been developed and can be categorized as (1) parametric methods such as Genomic Best Linear Unbiased Prediction (GBLUP), ridge regression Best Linear Unbiased Prediction (rrBLUP), Bayes, Haseman-Elston regression Best Linear Prediction (HEBLP), and RHPP (Meuwissen et al., 2001; VanRaden, 2008; Endelman, 2011; Habier et al., 2011; Xu et al., 2014; Liu and Chen, 2017; Liu and Yu, 2023), and (2) non-parametric methods such as Random Forest (RF), Support Vector Machine (SVM), Light Gradient Boosting Machine (LightGBM) and deep learning (DL) (Ogutu et al., 2011; Montesinos-López et al., 2018; Bellot et al., 2018; Yan et al., 2021).
Maize is the first crop to apply genomic prediction (Bernardo and Yu, 2007). Riedelsheimer et al. (2012) used 56110 SNPs to predict combining abilities of 7 biomass- and bioenergy-related traits of maize and found that prediction accuracies ranged from 0.72 to 0.81. Zhao et al. (2012) analyzed European maize elite breeding population and found that prediction accuracies of grain moisture and grain yield were respectively 0.90 and 0.58. Navarro et al. (2017) used 30000 markers to predict flower time traits including days to anthesis (DA) and days to silking (DS) for 4471 maize landraces via rrBLUP and found that the average prediction accuracy was 0.45. Li et al. (2020) utilized 8 genomic prediction methods to predict 10 traits of maize and found that the prediction accuracy ranged from 0.382 to 0.795. Xiao et al. (2021) utilized 8652 F1 hybrids from maize CUBIC population to predict days to tasseling (DTT), plant height (PH) and ear weight (EW) and found that the prediction accuracies of DTT, PH, and EW were 0.76, 0.81, and 0.66 respectively. Studies above demonstrated that genomic prediction was a highly effective technique to improve maize.
Southwest China is one of the three main production areas of maize in the country. In order to increase yield and adapt to complex ecological condition in this region, the breeders introduced tropical or subtropical maize germplasm such as ETO, Suwan, Dentado Amarillo, Tuson and Tuxpeno into the local maize germplasm to broaden the narrow genetic base (Zhang et al., 2016; Leng et al., 2019). Furthermore, a number of inbred lines containing tropical or subtropical maize germplasm were used to obtain excellent hybrid. In this study, we generated 2077 hybrids derived from random crosses of 360 inbred lines to perform genomic prediction analysis of 11 yield-related traits and furthermore established genomic prediction-based heterotic pattern of yield.
Materials and methods
Phenotypic data collection of maize
In this study, 360 maize inbred lines of wide genetic background and origin were collected, including the most representative inbred lines in Southwest China, inbred lines exchanged from the other breeding units of Sichuan, temperate inbred lines introduced from North China, tropic inbred lines introduced from the other breeding units, and inbred lines cultivated by our institute (Supplementary Table S1). From 64,620 crosses obtained through pairing of the 360 inbred lines, 2077 were randomly selected to perform field evaluation. All hybrids were planted in Field data of 11 traits including row number per ear, ear length, ear diameter, grain number per row, grain length, grain width, grain thickness, hundred grain weight, weight per unit volume, and yield (kg/mu) for 2077 crosses were collected at Gasa town, Jinghong city (21°95’N, 100°75’E, 520 meters above sea level), Yunnan province, China, in 2019. All hybrids were planted in one-row plot using a complete block design. Ten plants were planted in each row. The row length was 2.5 m and row spacing was 0.8 m. The plant density was about 3300 plants/mu. Field management followed standard procedures. For quality control, five plants in the middle of each row were selected to obtain the phenotypes. No further phenotypic corrections were performed on the basis of the other hybrid.
Genotypic data collection of maize inbred lines
Genomic DNA of the 360 inbred lines were extracted via CTAB method, and their qualities were evaluated with a Nanodrop 2000 Spectrophotometer (Thermo Fisher Scientific). High-quality DNA was genotyped via 48K liquid phase gene chip and sequenced on an Illumina Nova 150-bp paired-end sequencing platform in China Golden Marker (Beijing, China). Quality control of raw reads was performed via Trimmomatic-0.36 (Lohse et al., 2012) with default parameter, and then the filtered data were mapped to B73_RefGen_v4 genome with BWA software (Li and Durbin, 2009). The SNPs were called with GATK (McKenna et al., 2010) and further filtered with VCFtools (Danecek et al., 2011). The parameters used in SNP filters ration were: –minDP 4, –minGQ 10, –max-missing 0.8, –maf 0.05.
Construction of phylogenetic tree
45425 SNPs were used to generate distance matrix using VCF2Dis (https://github.com/BGI-shenzhen/VCF2Dis) and then phylogenetic tree was constructed based on distance matrix via FastME2.1.6.4 (Lefort et al., 2015).
Genotyping of maize hybrids
The genotypes of 2077 hybrid were obtained based on those of the inbred lines. 33009 of 45425 SNPs were kept according to the following criteria: (1) there are at least two kinds of genotype at each locus; (2) the percentage of “NA” at each locus is not more than 0.3; (3) the percentage of rare alleles at each locus is not less than 0.1.
Estimation of SNP heritability
The genetic model of a quantitative trait can be written as
where y is an vector for the phenotypic values; X is a incidence matrix for fixed effects; b is a vector of fixed effects; p is the number of fixed effects; Z is genotype matrix; m is the number of markers; u is a vector of additive effects following ; e is a vector of residual error following . The SNP heritability was calculated as:
We estimated and via the program GCTA v1.94.1, which consists of two steps: (1) computing the genetic relationship matrix (GRM) of all individuals based on all SNPs; (2) estimating additive genetic variance of a trait based on above GRM via the restricted maximum likelihood (REML) (Yang et al., 2011b).
Evaluation of genomic prediction of 11 yield-related traits
Two parametric genomic prediction methods including rrBLUP (Endelman, 2011) and HEBLP|A (Liu and Chen, 2017) and two non-parametric genomic prediction methods including RF and LightGBM were used in this study.
rrBLUP is one of the first methods for genomic prediction and has been taken as a baseline model (Meuwissen et al., 2001). The formula of rrBLUP is written as the following:
in which y is the vector of the phenotype; μ is the vector of mean value; Z is the genotype matrix; u is the vector of additive effects of the causal loci following where is the additive genetic variance; e is the vector of residual error following . The BLUP solution for u is written as where . The ridge parameter λ is computed on the tenfold cross-validated partial likelihood that minimized the residuals between predicted and observed phenotypes within the training population.
HEBLP|A is highly efficient in computation due to its estimation of heritability via Haseman-Elston regression (Liu and Chen, 2017). HEBLP|A and rrBLUP have similar model, but are different in the strategy of computing λ. HEBLP|A uses IBS-based Haseman-Elston (HE) to estimate and then .
RF is one of the most popular and powerful machine learning algorithms and has already been applied to a wide variety of genomic problems (Ogutu et al., 2011). RF uses bootstrap sample of the training population to build B decision trees and then averages the output of the ensemble of B trees to predict candidate individuals. The formula is as follows:
in which is the ith RF trees.
LightGBM, a very popular machine learning algorithm, was developed by Microsoft in 2017 and has been widely used in dealing with extremely large data with ultra-high efficiency (Ke et al., 2017). LightGBM uses a gradient-based one-side sampling (GOSS) and exclusive feature bundling (EFB) to reduce computational time. The basic process of the LightGBM is described as follows (Mienye and Sun, 2022):
1. Merge mutually exclusive features xi based on training population using the EFB.
2. Initialize where is the loss function.
3. For
i. Compute the absolute values of gradients:
ii. Resample the training population using the GOSS:
where A is samples with larger gradients and B is samples with small gradients.
iii. Calculate information gain values.
iv. Obtain a new decision tree on D.
v. Update .
4. Output: .
For RF and LightGBM methods, we utilized “randomForest” and “LightGBM” function in R package with the default parameters. The correlation coefficient between the phenotypes and the predicted genotypic values was considered as the prediction accuracy. 10 replications were used to evaluate the prediction accuracy of genomic prediction methods.
Genomic prediction of yield of all crossing combinations
The 360 inbred lines generated 64620 crossing combinations in total. We utilized 2077 hybrids with the phenotypic and genotypic data to predict the yield performance of 64620 crossing combinations via HEBLP|A under additive model.
Results
Phylogenetic tree analysis of the 360 maize inbred lines
As was demonstrated in the phylogenetic tree, the 360 maize inbred lines fell into three groups including temperate germplasm (90 inbred lines), tropical germplasm (106 inbred lines), and temp-tropic germplasm (164 inbred lines) (Figure 1). The temperate group contains temperate inbred lines from North China such as Zheng58, Chang7-2, Mo17, Dan340, PH6WC, PH4CV, and Jing724 and those from Southwest China such as Y9614 and 4011. Most of the inbred lines in the tropical group have mixed genetic background of Suwan, Tuxpeno, and CIMMTY, with the parent lines of main hybrids in Southwest China QR723, WG646, ZNC442, Xian21A, Rekangbai67, XZ50612, and 7031 being examples. As for temp-tropic group, most inbred lines such as SCML0849, CL11, R18, GH35, F19, SH1070, Q1 come from PB inbred lines and improved Reid inbred lines.
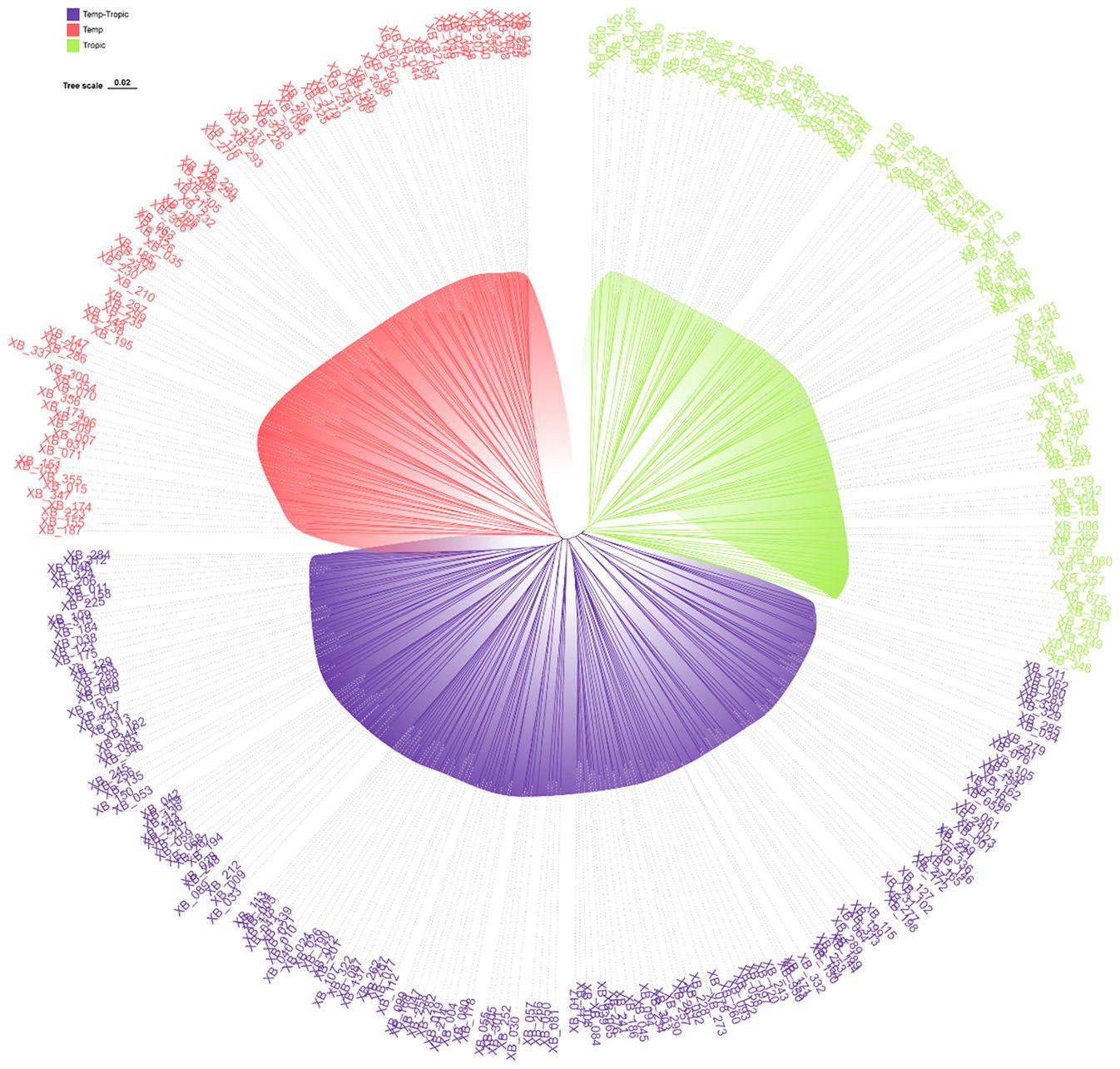
Figure 1. Phylogenetic tree for 360 maize inbred lines using 45425 SNP markers. Temperate germplasm (red), Temp-Tropic germplasm (purple), and Tropic germplasm (green).
Evaluation of genomic prediction for the 11 yield-related traits in maize hybrids
The SNP heritability of the 11 yield-related traits of 2077 hybrids was estimated via GCTA analysis under the additive model and ranged from 0.058 for grain thickness to 0.883 for row number per ear (Figure 2). The result indicated that most traits were mainly controlled by additive effects.
Evaluation of genomic prediction for the 11 yield-related traits was performed via rrBLUP, HEBLP|A, RF, and LightGBM (Table 1) with the size of training population and candidate population being 1800 and 277 respectively. The prediction accuracies ranged from 0.232 to 0.833 across traits and models. The row number per ear showed the highest prediction accuracy (average value across all methods being 0.796) and grain thickness had the lowest prediction accuracy (average value cross all methods being 0.302). The Pearson’s correlation coefficient between heritability and average prediction accuracy was significant ( and ), indicating that the heritability influenced prediction accuracy to a great extent, and the result was consistent with previous researches (Daetwyler et al., 2008; Liu and Chen, 2018). Among the four methods, rrBLUP and HEBLP|A have similar prediction accuracy in all the 11 traits (for example, and in row number per ear), and so do RF and LightGBM (for example, and in row number per ear). The parametric methods (rrBLUP and HEBLP|A) outperformed non-parametric methods (RF and LightGBM) in 8 traits (Row number per ear, Ear length, Ear diameter, Grain number per row, Grain length, Grain width, Hundred grain weight, and Weight per unit volume), and the latter outperformed the former only in grain thickness, which may be mainly controlled by non-additive effects such as dominance, epistasis and genotype-by-environment interaction. Both two categories of methods had similar predictive accuracy in 2 traits (Cob diameter and Yield).
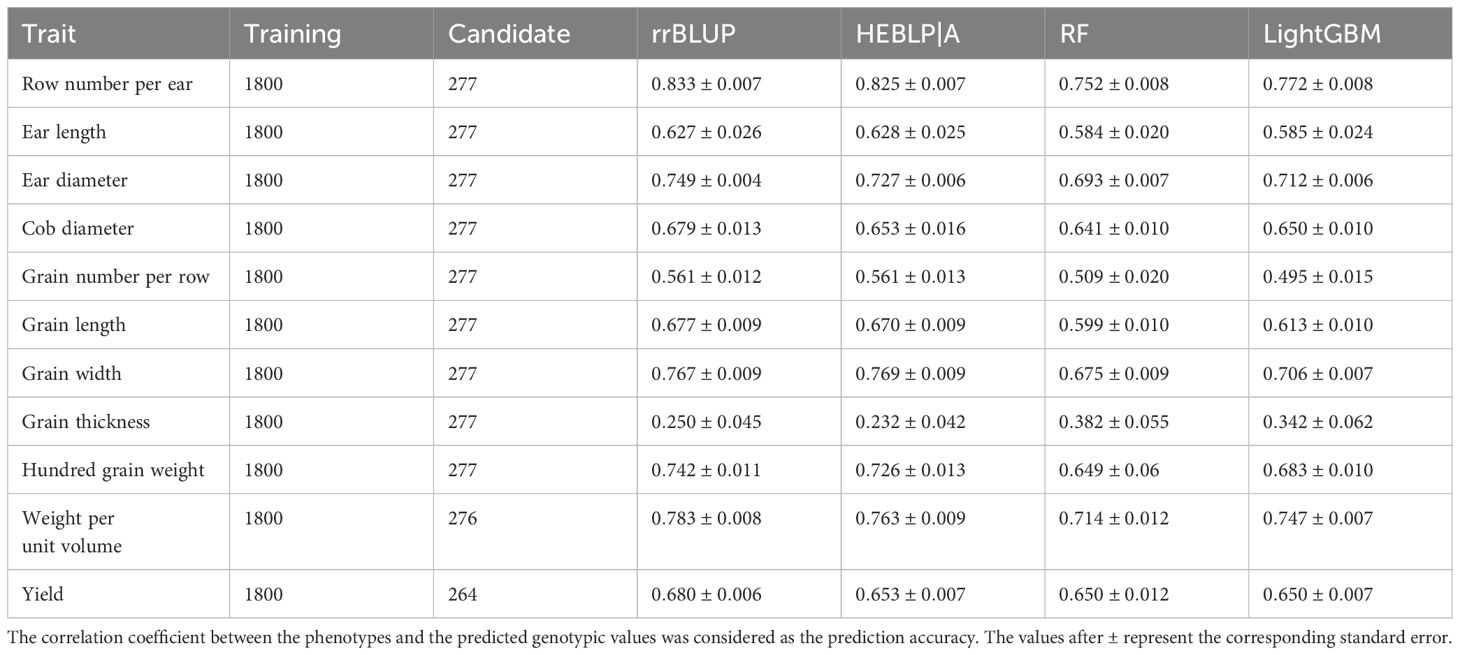
Table 1. Comparison of genomic predictability among rrBLUP, HEBLP|A, RF, and LightGBM for 11 yield related traits of maize based on 10 simulations.
Prediction of GEBVs of yield for all crossing combinations
GEBVs for yield of the 64620 crosses generated by the 360 inbred lines were obtained via 2077 hybrids with phenotypic and genotypic values under additive model via HEBLP|A and were sorted in descending order. The average GEBV of all crosses was 341.50 for yield, and that of the top 200 crosses, the top 5% (3231 ones) and the top 10% (6462 ones) was 558.35, 491.78 and 469.20 respectively. If breeders use the crosses of the best predictive accuracy in cultivation (the top 10% for example), a significant genetic gain can be expected.
To evaluate the general combining ability (GCA) of an inbred line, its mean GEBV of the 359 crosses was obtained by crossing the remaining 359 ones with it, and consequently there were 44 inbred lines with mean GEBVs over 400kg, among which some most popular inbred lines in Southwest China were found, such as ZNC442, QR273, YA8201, Xian21A, Rekangbai67, XZ50612, and F19. There are 29 tropic inbred lines (proportion is 65.9%) and 11 temp-tropic ones (proportion is 25%) among the 44 inbred lines, indicating that inbred lines with tropic and temp-tropic germplasm play an important role in the maize hybrid breeding of Southwest China. In contrast, the mean GEBVs of some pure temperate inbred lines introduced from North China such as 478, Zheng58, Chang7-2, Dan340, 91227, M54, and NH60 were quite low, but their improved version demonstrated high GCA when tropic germplasm was added. For example, comparing the inbred line 91227 with LX2715, its improved version via introducing tropic germplasm, we found a significant increase of the mean GEBV from 297.2kg to 349.3kg. Therefore, tropic or temp-tropic germplasms can help pure temperate inbred lines from North China to adapt to ecological environment, reducing their defects in Southwest China such as low resistance to diseases and pests when they are used in maize breeding. Generally speaking, most of the local temperate inbred lines from Southwest China contain a certain proportion of tropic germplasm through long-term improvement. In addition, the result of some representative inbred lines in Southwest China including PH6WC (temperate), GH35, SCML0849, and NG5 (temp-tropic), and WG646, ZNC442, XZ50612, QR273, and XL8242 (tropic) has showed that tropic inbred lines have better performance than temperate and temp-tropic ones (Figure 3). In particular, the mean GEBV of the 359 crosses corresponding to XL8242 (a newest tropic inbred line cultivated by our unit) ranked the first among the 360 inbred lines and has been used to breed some hybrids successfully (Table 2).
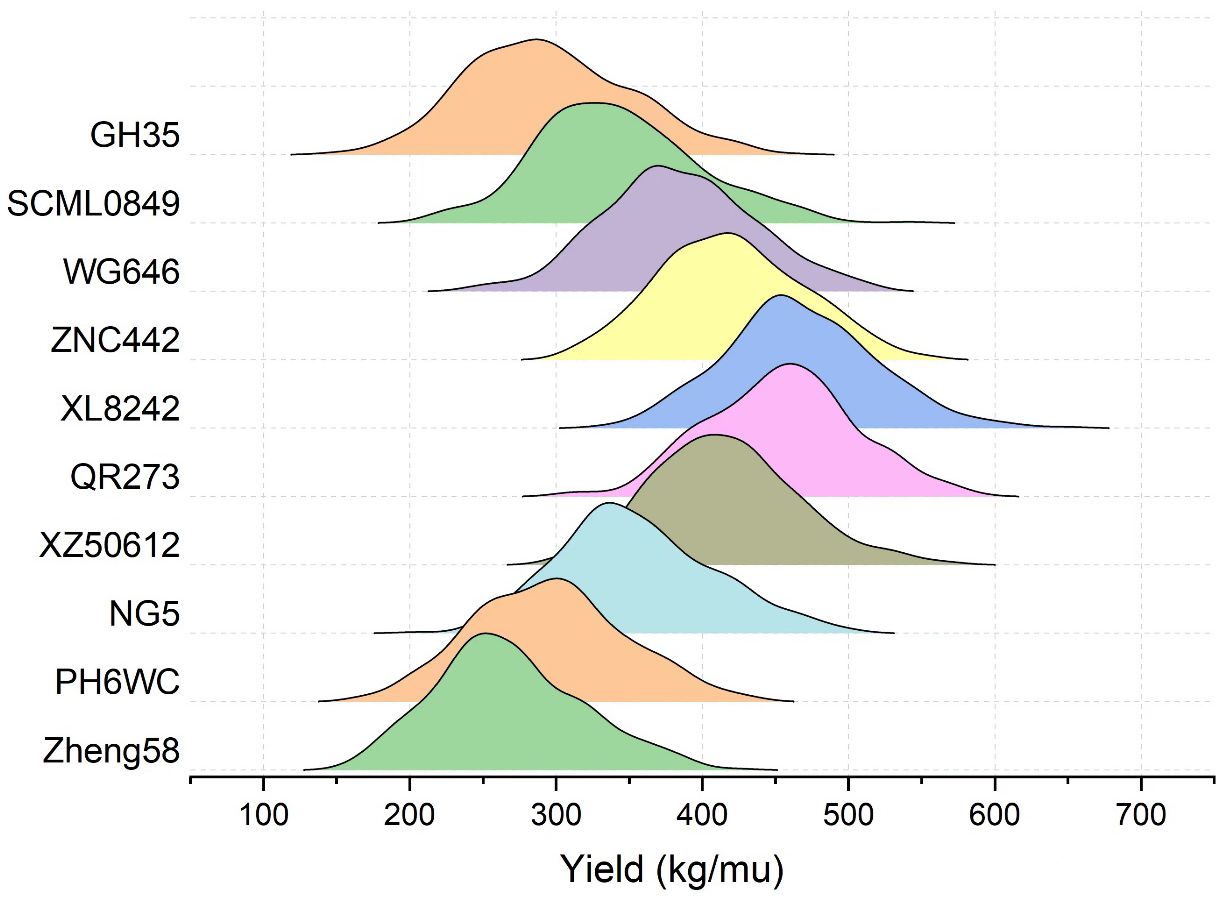
Figure 3. The GEBV distribution of the 359 crosses corresponding the representative inbred lines in Southwest China. Zheng58 (temperate line from North China) was taken as control inbred line. Temperate inbred line in Southwest China (PH6WC), temp-tropic inbred lines in Southwest China (GH35, SCML0849, and NG5), and tropic inbred lines in Southwest China (WG646, ZNC442, XZ50612, QR273, and XL8242).
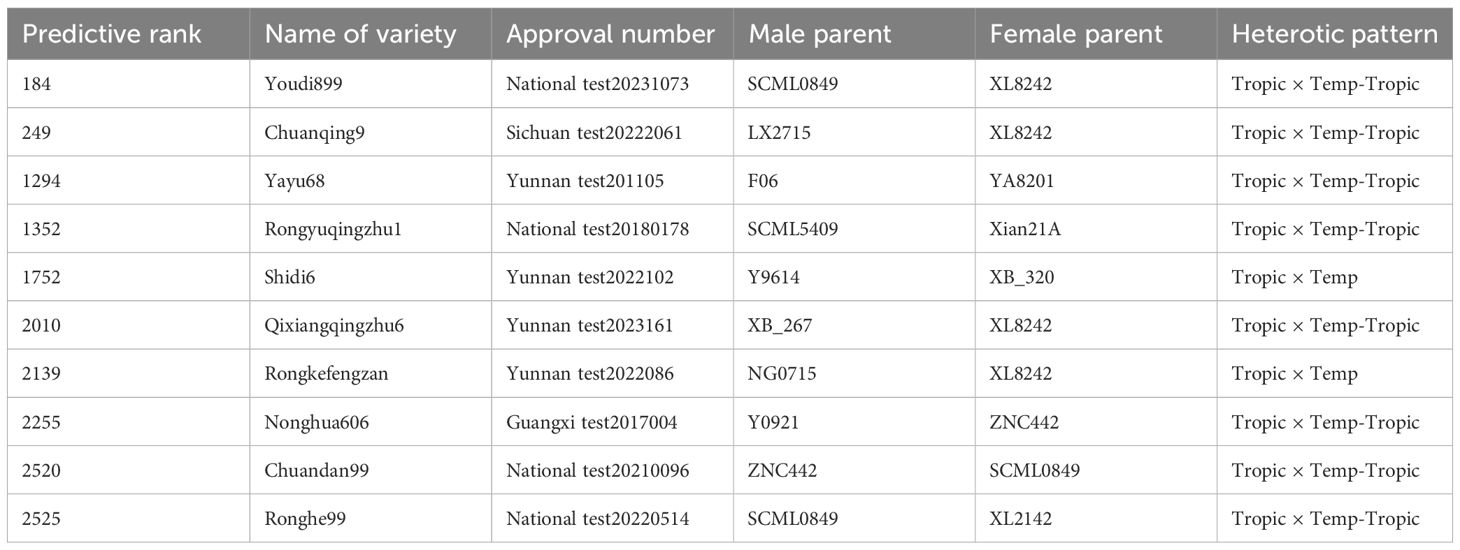
Table 2. Basic information of the maize varieties from 5% top crosses based on genomic prediction of yield.
We constructed six heterotic patterns with three germplasms (Figure 4) and the results showed that the mean GEBV of Tropic × Tropic, Tropic × Temp-Tropic, Tropic × Temp, Temp-Tropic × Temp-Tropic, Temp-Tropic × Temp and Temp × Temp is , , , , , and respectively (Figure 5). Among the 5% top crosses, 21% are Tropic × Tropic mode and 66.4% are Temperate (or Temp-Tropic) × Tropic mode, indicating that Temperate (or Temp-Tropic) × Tropic mode is a major heterotic pattern. There are some approved commercial varieties among the 5% top crosses including Youdi899, Chuanqing9, Yayu68, Rongyuqingzhu1, Shidi6, Qixiangqingzhu6, Rongkefengzan, Nonghua606, Chuandan99, and Ronghe99 (Table 2). Take Youdi899 for example, deriving from SCML0849 and XL8242, it ranked 184 in all crosses and had an excellent performance in Southwest China (Figure 6). In 2020-2022 years’ national spring maize cultivar regional trials (middle and high altitude region) in Southwest China, its mean yield of two years is 814.3kg and the production increased by 14.8% compared with the control variety. In 2021-2022 years’ autonomous production test, its mean yield was 783.1kg and the production increased by 11.6% compared with the control variety. After the identification of field inoculation diseases, this variety was highly resistant to northern maize leaf blight and southern maize leaf blight, moderately resistant to ear rot, maize stalk rot, grey leaf spot, southern maize rust, and susceptible to northern maize leaf blight. Tropic × Tropic mode account for relative high proportion because Jinghong City of Yunnan Province, the place where the training population of maize was planted, belongs to tropic-subtropic region, and the result conforms well to practical breeding. In the future, we will further investigate heterotic mode of maize in other regions of Southwest China (including middle and low altitude region and middle and high altitude region) via genomic prediction.
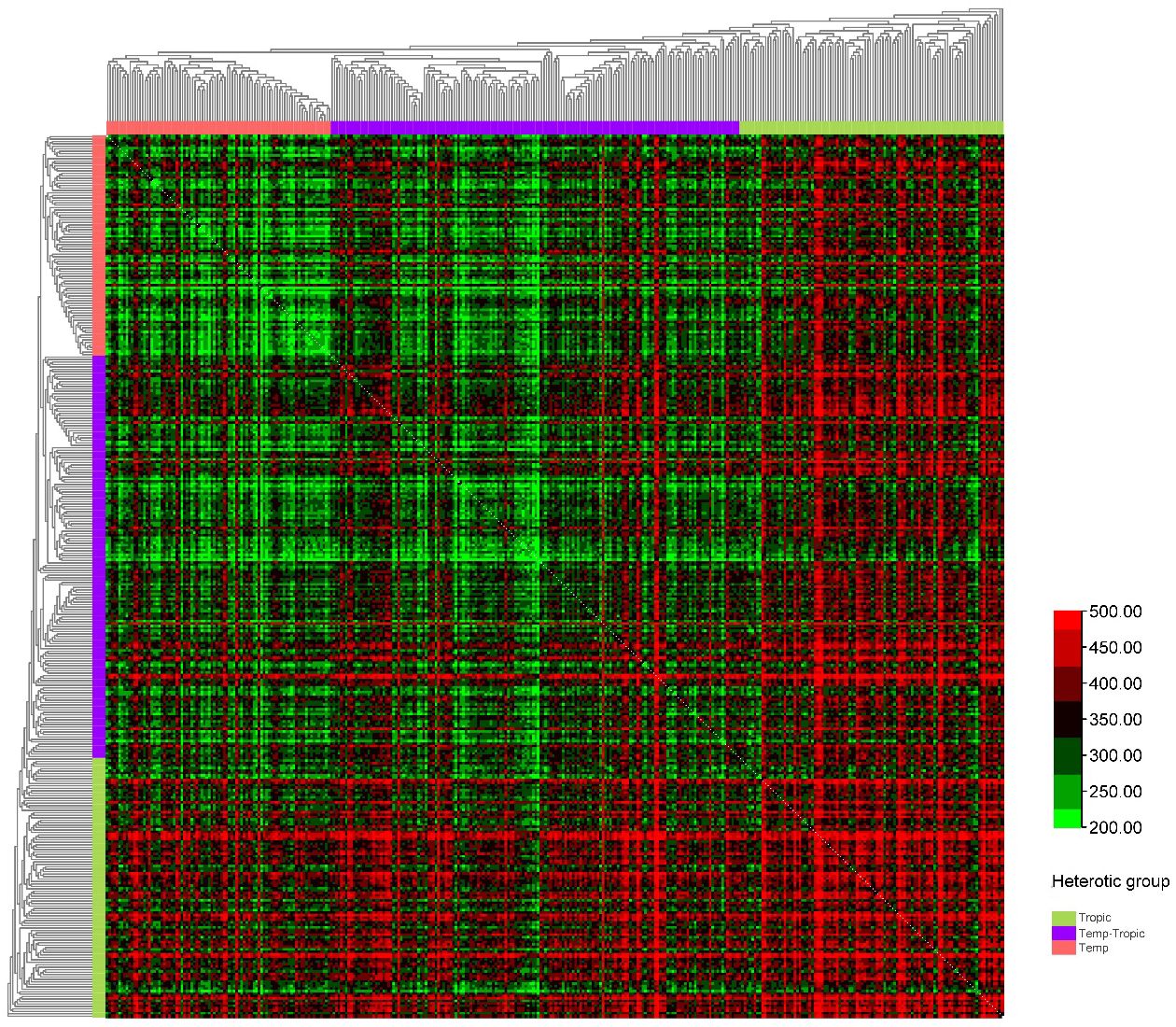
Figure 4. Heterotic pattern in maize hybrid breeding of Southwest China based on genomic prediction of yield (kg/mu).
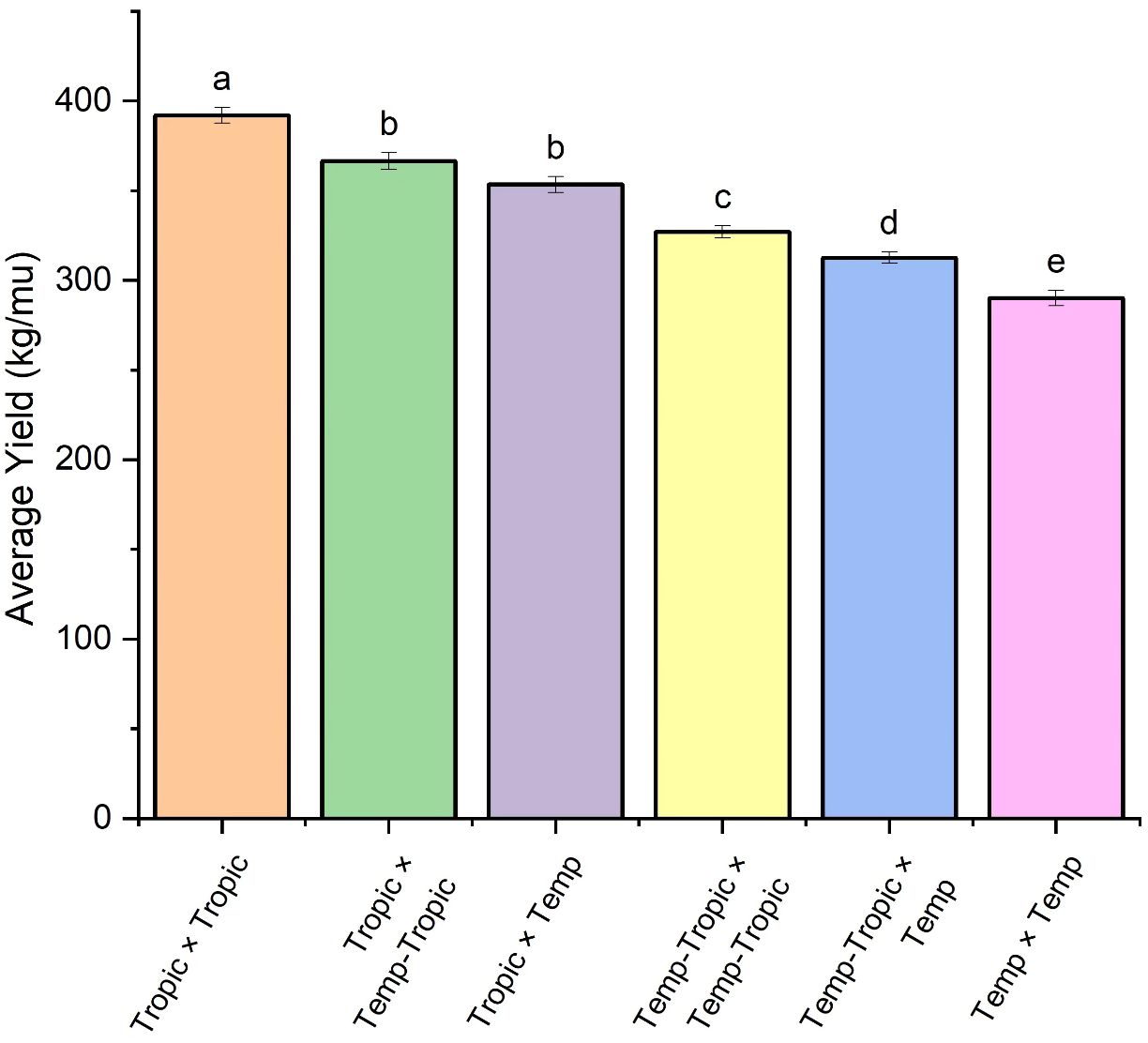
Figure 5. Average yield of different heterotic groups based on genomic prediction. The lowercase letters (a, b, c, d, and e) have significant difference.
Discussion
It is critical for modern plant breeding to predict superior individuals with high accuracy (Heffner et al., 2009; Voss-Fels et al., 2019). When there is a large number of inbred lines in the process of maize breeding, it is difficult to identify superior hybrid crosses from crosses (N represents number of inbred lines) via conventional breeding methods because it is time consuming and too expensive. Being a useful technique to select superior hybrid crosses, genomic prediction has been successfully applied to maize breeding currently (Albrecht et al., 2011; Zhao et al., 2012; Riedelsheimer et al., 2012; Technow et al., 2014; Li et al., 2020; Wang et al., 2020; Luo et al., 2023).
To our knowledge, this is the first study about a large-scale application of genomic prediction in Southwest China’s maize hybrid breeding. We evaluated the genomic prediction accuracies of 11 yield-related traits in an F1 population with 2077 F1 maize hybrids and found that the predictive accuracies of most traits except grain thickness were higher than 55%. It is indicated that genomic prediction is a promising tool in maize breeding programs. Comparison of four methods demonstrated that parametric ones (rrBLUP and HEBLP|A) outperformed non-parametric ones (RF and LightGBM) in 8 out of 11 traits, which is consistent with previous studies that parametric methods outperformed machine-learning methods when the traits were mainly controlled by additive effects (Abdollahi-Arpanahi et al., 2020; Alves et al., 2020; Yu et al., 2023).
Increasing yield is the most important objective in hybrid maize production (Li et al., 2011; Peng et al., 2011; Tian et al., 2024), but it is difficult to improve yield trait via phenotypic selection or conventional MAS because it is a complex trait controlled by a large number of quantitative trait loci (QTL) with small effects (Ndlovu et al., 2022). In this study, the predictive accuracy of yield was about 65% when four genomic prediction methods were evaluated with 2077 maize crosses. According to the prediction of the yield performance of all 64620 crosses, one parent of the top 5% crosses has at least a tropic or temp-tropic inbred lines, and 86.4% of those top 5% have at least a tropic inbred line, which is highly consistent with practical breeding. It is indicated that tropic inbred lines play an important role in selecting superior hybrids in Southwest China.
With serious increase of diseases and pests of maize at present, it is more and more difficult for Reid × Suwan, the conventional heterotic pattern of Southwest China (Ni et al., 1996; Pan et al., 2020) to tackle the problem. Therefore, we proposed a new heterotic pattern (Reid+ × Suwan+) in which Reid+ represents the introgression from tropical germplasm or other temperate germplasm to Reid inbred lines, and Suwan+ represents the introgression from other tropical germplasm to Suwan inbred lines. It will become a major heterotic pattern of Southwest China in the future.
Data availability statement
The original contributions presented in the study are included in the article. Further inquiries can be directed to the corresponding authors.
Author contributions
HLL: Conceptualization, Data curation, Formal analysis, Funding acquisition, Investigation, Methodology, Project administration, Resources, Software, Supervision, Validation, Visualization, Writing – original draft, Writing – review & editing. YX: Investigation, Writing – review & editing. CX: Data curation, Formal analysis, Funding acquisition, Investigation, Writing – review & editing. LL: Data curation, Resources, Writing – review & editing. RW: Resources, Writing – review & editing. TR: Writing – review & editing. HL: Conceptualization, Funding acquisition, Resources, Supervision, Writing – review & editing.
Funding
The author(s) declare financial support was received for the research, authorship, and/or publication of this article. This study was supported by National Key Research and Development Project of China (Grant No. 2023YFD1201101), Major Science and Technology Project of Sichuan Province (Grant No. 2022ZDZX0013), the National Natural Science Foundation of China (Grant No. 32271984 and 32101673), the Natural Science Foundation of Sichuan Province (Grant No. 2023NSFSC0222 and 2022NSFSC1774), and Science and Technology Program of Liangshan Prefecture (Grant No. 24YYYJ0183 and 24YYYJ0184).
Conflict of interest
The authors declare that the research was conducted in the absence of any commercial or financial relationships that could be construed as a potential conflict of interest.
Publisher’s note
All claims expressed in this article are solely those of the authors and do not necessarily represent those of their affiliated organizations, or those of the publisher, the editors and the reviewers. Any product that may be evaluated in this article, or claim that may be made by its manufacturer, is not guaranteed or endorsed by the publisher.
Supplementary material
The Supplementary Material for this article can be found online at: https://www.frontiersin.org/articles/10.3389/fpls.2024.1441555/full#supplementary-material
References
Abdollahi-Arpanahi, R., Gianola, D., Peńagaricano, F. (2020). Deep learning versus parametric and ensemble methods for genomic prediction of complex phenotypes. Genet. Selection Evol. 52, 12. doi: 10.1186/s12711-020-00531-z
Albrecht, T., Wimmer, V., Auinger, H. J., Erbe, M., Knaak, C., Ouzunova, M., et al. (2011). Genome-based prediction of testcross values in maize. Theor. Appl. Genet. 123, 339–350. doi: 10.1007/s00122-011-1587-7
Alemu, A., Åstrand, J., Montesinos-López, O. A., y Sánchez, J. I., Fernández-Gónzalez, J., Tadesse, W., et al. (2024). Genomic selection in plant breeding: Key factors shaping two decades of progress. Mol. Plant 17, 552–578. doi: 10.1016/j.molp.2024.03.007
Alves, A. A. C., da Costa, R. M., Bresolin, T., Júnior, G. A. F., Espigolan, R., Ribeiro, A. M. F., et al. (2020). Genome-wide prediction for complex traits under the presence of dominance effects in simulated populations using GBLUP and machine learning methods. J. Anim. Sci. 98, skaa179. doi: 10.1093/jas/skaa179
Bellot, P., de los Campos, G., Pérez-Enciso, M. (2018). Can deep learning improve genomic prediction of complex human traits. Genetics 210, 809–819. doi: 10.1534/genetics.118.301298
Bernardo, R., Yu, J. (2007). Prospects for genomewide selection for quantitative traits in maize. Crop Sci. 47, 1082–1090. doi: 10.2135/cropsci2006.11.0690
Chai, Y., Hao, X., Yang, X., Allen, W. B., Li, J., Yan, J., et al. (2012). Validation of DGAT1-2 polymorphisms associated with oil content and development of functional markers for molecular breeding of high-oil maize. Mol. Breed. 29, 939–949. doi: 10.1007/s11032-011-9644-0
Cui, Y., Li, R., Li, G., Zhang, F., Zhu, T., Zhang, Q., et al. (2020). ). Hybrid breeding of rice via genomic selection. Plant Biotechnol. J. 18, 57–67. doi: 10.1111/pbi.13170
Daetwyler, H. D., Villanueva, B., Woolliams, J. A. (2008). Accuracy of predicting the genetic risk of disease using a genome-wide approach. PloS One 3, e3395. doi: 10.1371/journal.pone.0003395
Danecek, P., Auton, A., Abecasis, G., Albers, C. A., Banks, E., DePristo, M. A., et al. (2011). The variant call format and VCFtools. Bioinformatics 27, 2156–2158. doi: 10.1093/bioinformatics/btr330
Endelman, J. B. (2011). Ridge regression and other kernels for genomic selection with R package rrBLUP. Plant Genome 4, 250–255. doi: 10.3835/plantgenome2011.08.0024
Fristche-Neto, R., Akdemir, D., Jannink, J. L. (2018). Accuracy of genomic selection to predict maize single-crosses obtained through different mating designs. Theor. Appl. Genet. 131, 1153–1162. doi: 10.1007/s00122-018-3068-8
Habier, D., Fernando, R. L., Kizilkaya, K., Garrick, D. J. (2011). Extension of the bayesian alphabet for genomic selection. BMC Bioinf. 12, 186. doi: 10.1186/1471-2105-12-186
Hao, X., Li, X., Yang, X., Li, J. (2014). Transferring a major QTL for oil content using marker-assisted backcrossing into an elite hybrid to increase the oil content in maize. Mol. Breed. 34, 739–748. doi: 10.1007/s11032-014-0071-x
Hayes, B. J., Bowman, P. J., Chamberlain, A. J., Goddard, M. E. (2009). Invited review: Genomic selection in dairy cattle: Progress and challenges. J. Dairy Sci. 92, 433–443. doi: 10.3168/jds.2008-1646
Heffner, E. L., Jannink, J. L., Sorrells, M. E. (2011). Genomic selection accuracy using multifamily prediction models in a wheat breeding program. Plant Genome 4, 65–75. doi: 10.3835/plantgenome2010.12.0029
Heffner, E. L., Sorrells, M. E., Jannink, J. L. (2009). Genomic selection for crop improvement. Crop Sci. 49, 1–12. doi: 10.2135/cropsci2008.08.0512
Inghelandt, D. V., Melchinger, A. E., Lebreton, C., Stich, B. (2010). Population structure and genetic diversity in a commercial maize breeding program assessed with SSR and SNP markers. Theor. Appl. Genet. 120, 1289–1299. doi: 10.1007/s00122-009-1256-2
Jannink, J. L., Lorenz, A. J., Iwata, H. (2010). Genomic selection in plant breeding: from theory to practice. Briefings Funct. Genomics 9, 166–177. doi: 10.1093/bfgp/elq001
Ke, G., Meng, Q., Finley, T., Wang, T., Chen, W., Ma, W., et al. (2017). LightGBM: a highly efficient gradient boosting decision tree. Adv. Neural Inf. Process. Syst. 30, 3149–3157.
Lefort, V., Desper, R., Gascuel, O. (2015). FastME 2.0: A comprehensive, accurate, and fast distance-based phylogeny inference program. Mol. Biol. Evol. 32, 2798–2800. doi: 10.1093/molbev/msv150
Leng, Y., Lv, C., Li, L., Xiang, Y., Xia, C., Wei, R., et al. (2019). Heterotic grouping based on genetic variation and population structure of maize inbred lines from current breeding program in Sichuan province, Southwest China using genotyping by sequencing (GBS). Mol. Breed. 39, 38. doi: 10.1007/s11032-019-0946-y
Li, G., Dong, Y., Zhao, Y., Tian, X., Würschum, T., Xue, J., et al. (2020). Genome-wide prediction in a hybrid maize population adapted to Northwest China. Crop J. 8, 830–842. doi: 10.1016/j.cj.2020.04.006
Li, H., Durbin, R. (2009). Fast and accurate short read alignment with Burrows-Wheeler transform. Bioinformatics 25, 1754–1760. doi: 10.1093/bioinformatics/btp324
Li, J. Z., Zhang, Z. W., Li, Y. L., Wang, Q. L., Zhou, Y. G. (2011). QTL consistency and meta-analysis for grain yield components in three generations in maize. Theor. Appl. Genet. 122, 771–782. doi: 10.1007/s00122-010-1485-4
Liu, H., Chen, G. B. (2017). A fast genomic selection approach for large genomic data. Theor. Appl. Genet. 130, 1277–1284. doi: 10.1007/s00122-017-2887-3
Liu, H., Chen, G. B. (2018). A new genomic prediction method with additive-dominance effects in the least-squares framework. Heredity 121, 196–204. doi: 10.1038/s41437-018-0099-5
Liu, H., Yu, S. (2023). A dimensionality-reduction genomic prediction method without direct inverse of the genomic relationship matrix for large genomic data. Plant Cell Rep. 42, 1825–1832. doi: 10.1007/s00299-023-03069-8
Lohse, M., Bolger, A. M., Nagel, A., Fernie, A. R., Lunn, J. E., Stitt, M., et al. (2012). RobiNA: a user-friendly, integrated software solution for RNA-Seq-based transcriptomics. Nucleic Acids Res. 40, W622–W627. doi: 10.1093/nar/gks540
Luo, P., Wang, H., Ni, Z., Yang, R., Wang, F., Yong, H., et al. (2023). Genomic prediction of yield performance among single-cross maize hybrids using a partial diallel cross design. Crop J. 11, 1884–1892. doi: 10.1016/j.cj.2023.09.009
McKenna, A., Hanna, M., Banks, E., Sivachenko, A., Cibulskis, K., Kernytsky, A., et al. (2010). The Genome Analysis Toolkit: A MapReduce framework for analyzing next-generation DNA sequencing data. Genome Res. 20, 1297–1303. doi: 10.1101/gr.107524.110
Meuwissen, T., Hayes, B., Goddard, M. (2013). Accelerating improvement of livestock with genomic selection. Annu. Rev. Anim. Biosci. 1, 221–237. doi: 10.1146/annurev-animal-031412-103705
Meuwissen, T. H. E., Hayes, B. J., Goddard, M. E. (2001). Prediction of total genetic value using genome-wide dense marker maps. Genetics 157, 1819–1829. doi: 10.1093/genetics/157.4.1819
Mienye, I. D., Sun, Y. (2022). A survey of ensemble learning: concepts, algorithms, applications, and prospects. IEEE Access 10, 99129–99149. doi: 10.1109/ACCESS.2022.3207287
Montesinos-López, A., Montesinos-López, O. A., Gianola, D., Crossa, J., Hernández-Suárez, C. M. (2018). Multi-environment genomic prediction of plant traits using deep learners with a dense architecture. G3: Genes Genomes Genet. 8, 3813–3828. doi: 10.1534/g3.118.200740
Navarro, J. A. R., Wilcox, M., Burgueńo, J., Romay, C., Swarts, K., Trachsel, S., et al. (2017). A study of allelic diversity underlying flowering-time adaptation in maize landraces. Nat. Genet. 49, 476–480. doi: 10.1038/ng.3784
Ndlovu, N., Spillane, C., McKeown, P. C., Cairns, J. E., Das, B., Gowda, M. (2022). Genome−wide association studies of grain yield and quality traits under optimum and low−nitrogen stress in tropical maize (Zea mays L.). Theor. Appl. Genet. 135, 4351–4370. doi: 10.1007/s00122-022-04224-7
Ni, X., Liu, L., Lei, B. (1996). Study on the selection of maize inbred line S37 suited to mountain area maize breeding. J. Sichuan Agric. Univ. 14, 366–370. doi: 10.16036/j.issn.1000-2650.1996.03.011
Ogutu, J. O., Piepho, H. P., Schulz-Streeck, T. (2011). A comparison of random forests, boosting and support vector machines for genomic selection. BMC Proc. 5, S11. doi: 10.1186/1753-6561-5-S3-S11
Pan, G. T., Yang, K. C., Li, W. C., Huang, Y. B., Gao, S. B., Lan, H., et al. (2020). A review of the research and application of heterotic groups and patterns of maize breeding in Southwest China. J. Maize Sci. 28, 1–8. doi: 10.13597/j.cnki.maize.science.20200101
Peng, B., Li, Y., Wang, Y., Liu, C., Liu, Z., Tan, W., et al. (2011). QTL analysis for yield components and kernel-related traits in maize across multi-environments. Theor. Appl. Genet. 122, 1305–1320. doi: 10.1007/s00122-011-1532-9
Prasanna, B. M., Pixley, K., Warburton, M. L., Xie, C. X. (2010). Molecular marker-assisted breeding options for maize improvement in Asia. Mol. Breed. 26, 339–356. doi: 10.1007/s11032-009-9387-3
Riedelsheimer, C., Czedik-Eysenberg, A., Grieder, C., Lisec, J., Technow, F., Sulpice, R., et al. (2012). Genomic and metabolic prediction of complex heterotic traits in hybrid maize. Nat. Genet. 44, 217–220. doi: 10.1038/ng.1033
Stevens, R. (2008). Prospects for using marker-assisted breeding to improve maize production in Africa. J. Sci. Food Agric. 88, 745–755. doi: 10.1002/jsfa.3154
Technow, F., Schrag, T. A., Schipprack, W., Bauer, E., Simianer, H., Melchinger, A. E. (2014). Genome properties and prospects of genomic prediction of hybrid performance in a breeding program of maize. Genetics 197, 1343–1355. doi: 10.1534/genetics.114.165860
Tian, J., Wang, C., Chen, F., Qin, W., Yang, H., Zhao, S., et al. (2024). Maize smart-canopy architecture enhances yield at high densities. Nature. 632, 576-584. doi: 10.1038/s41586-024-07669-6
VanRaden, P. M. (2008). Efficient methods to compute genomic predictions. J. Dairy Sci. 91, 4414–4423. doi: 10.3168/jds.2007-0980
Voss-Fels, K. P., Cooper, M., Hayes, B. J. (2019). Accelerating crop genetic gains with genomic selection. Theor. Appl. Genet. 132, 669–686. doi: 10.1007/s00122-018-3270-8
Wang, X., Zhang, Z., Xu, Y., Li, P., Zhang, X., Xu, C. (2020). Using genomic data to improve the estimation of general combining ability based on sparse partial diallel cross designs in maize. Crop J. 8, 819–829. doi: 10.1016/j.cj.2020.04.012
Xiao, Y., Jiang, S., Cheng, Q., Wang, X., Yan, J., Zhang, R., et al. (2021). The genetic mechanism of heterosis utilization in maize improvement. Genome Biol. 22, 148. doi: 10.1186/s13059-021-02370-7
Xu, S., Zhu, D., Zhang, Q. (2014). Predicting hybrid performance in rice using genomic best linear unbiased prediction. PNAS 111, 12456–12461. doi: 10.1073/pnas.1413750111
Yan, J., Xu, Y., Cheng, Q., Jiang, S., Wang, Q., Xiao, Y., et al. (2021). LightGBM: accelerated genomically designed crop breeding through ensemble learning. Genome Biol. 22, 271. doi: 10.1186/s13059-021-02492-y
Yang, X., Gao, S., Xu, S., Zhang, Z., Prasanna, B. M., Li, L., et al. (2011a). Characterization of a global germplasm collection and its potential utilization for analysis of complex quantitative traits in maize. Mol. Breed. 28, 511–526. doi: 10.1007/s11032-010-9500-7
Yang, J., Lee, S. H., Goddard, M. E., Visscher, P. M. (2011b). GCTA: a tool for genome-wide complex trait analysis. Am. J. Hum. Genet. 88, 76–82. doi: 10.1016/j.ajhg.2010.11.011
Yu, G., Cui, Y., Jiao, Y., Zhou, K., Wang, X., Yang, W., et al. (2023). Comparison of sequencing-based and array-based genotyping platforms for genomic prediction of maize hybrid performance. Crop J. 11, 490–498. doi: 10.1016/j.cj.2022.09.004
Zhang, X., Zhang, H., Li, L., Lan, H., Ren, Z., Liu, D., et al. (2016). Characterizing the population structure and genetic diversity of maize breeding germplasm in Southwest China using genome-wide SNP markers. BMC Genomics 17, 697. doi: 10.1186/s12864-016-3041-3
Keywords: genomic prediction, maize, yield-related traits, heterotic pattern of yield, Southwest China
Citation: Xiang Y, Xia C, Li L, Wei R, Rong T, Liu H and Lan H (2024) Genomic prediction of yield-related traits and genome-based establishment of heterotic pattern in maize hybrid breeding of Southwest China. Front. Plant Sci. 15:1441555. doi: 10.3389/fpls.2024.1441555
Received: 31 May 2024; Accepted: 21 August 2024;
Published: 09 September 2024.
Edited by:
Ming Luo, Commonwealth Scientific and Industrial Research Organisation (CSIRO), AustraliaReviewed by:
Zitong Li, Commonwealth Scientific and Industrial Research Organisation (CSIRO), AustraliaChenwu Xu, Yangzhou University, China
Copyright © 2024 Xiang, Xia, Li, Wei, Rong, Liu and Lan. This is an open-access article distributed under the terms of the Creative Commons Attribution License (CC BY). The use, distribution or reproduction in other forums is permitted, provided the original author(s) and the copyright owner(s) are credited and that the original publication in this journal is cited, in accordance with accepted academic practice. No use, distribution or reproduction is permitted which does not comply with these terms.
*Correspondence: Hai Lan, lanhai_maize@163.com; Hailan Liu, lhlzju@hotmail.com
†These authors have contributed equally to this work