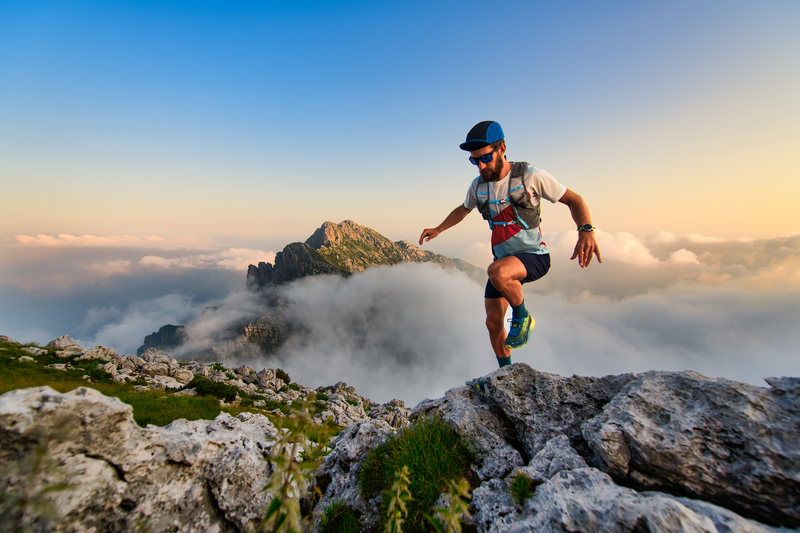
94% of researchers rate our articles as excellent or good
Learn more about the work of our research integrity team to safeguard the quality of each article we publish.
Find out more
ORIGINAL RESEARCH article
Front. Plant Sci. , 14 August 2024
Sec. Crop and Product Physiology
Volume 15 - 2024 | https://doi.org/10.3389/fpls.2024.1440859
This article is part of the Research Topic Meeting the Triple-H Challenge: Advanced Crop-Soil-Fertilizer Management Strategies to Maximize Crop Yield, Quality, and Nutrient Efficiency View all 15 articles
Introduction: The depth of fertilizer application significantly influences soil nitrate concentration (SNC), sunflower root length density (RLD), sunflower nitrogen uptake (SNU), and yield. However, current studies cannot precisely capture subtle nutrient variations between soil layers and their complex relationships with root growth. They also struggle to assess the impact of different fertilizer application depths on sunflower root development and distribution as well as their response to the spatial and temporal distribution of nutrients.
Methods: The Agricultural Production Systems sIMulator (APSIM) model was employed to explore the spatial and temporal patterns of nitrogen distribution in the soil at three controlled-release fertilizer (CRF) placement depths: 5, 15, and 25 cm. This study investigated the characteristics of the root system regarding nitrogen absorption and utilization and analyzed their correlation with sunflower yield formation. Furthermore, this study introduced the modified Jaccard index (considering the compatibility between soil nitrate and root length density) to analyze soil-root interactions, providing a deeper insight into how changes in CRF placement depth affect crop growth and nitrogen uptake efficiency.
Results: The results indicated that a fertilization depth of 15 cm improved the modified Jaccard index by 6.60% and 7.34% compared to 5 cm and 25 cm depths, respectively, maximizing sunflower yield (an increase of 9.44%) and nitrogen absorption rate (an increase of 5.40%). This depth promoted a greater Root Length Density (RLD), with an increases of 11.95% and 16.42% compared those at 5 cm and 25 cm, respectively, enhancing deeper root growth and improving nitrogen uptake. In contrast, shallow fertilization led to higher nitrate concentrations in the topsoil, whereas deeper fertilization increased the nitrate concentrations in the deeper soil layers.
Discussion: These results provide valuable insights for precision agriculture and sustainable soil management, highlighting the importance of optimizing root nitrogen absorption through tailored fertilization strategies to enhance crop production efficiency and minimize environmental impact.
Nitrogen is crucial for optimal crop yields and is a key nutrient for plant growth (Tabuchi et al., 2007; Heuer et al., 2017). The global utilization rate of nitrogen fertilizers is generally low, often around 40%–53% (Chen et al., 2021b; Wang et al., 2023a), leading to inefficiencies in agricultural practices, where farmers frequently apply excessive nitrogen fertilizer, surpassing the crops’ actual growth requirements (Nie et al., 2022; Wang et al., 2022a). Over-application of nitrogen fertilizers increases agricultural costs and causes environmental issues, as reported globally (Chen and Graedel, 2016; Ma et al., 2019; Chen et al., 2021a). Additionally, the excessive application of nitrogen fertilizers can contribute to increased greenhouse gas emissions, such as nitrous oxide (N2O), which has high global warming potential (Kong et al., 2021; Ren et al., 2021; Wu et al., 2023). Therefore, improving the efficiency of nitrogen fertilizer use is vital for global food security, sustainable economic development, and mitigating the ecological impact of agriculture. Studies have demonstrated that adopting more precise fertilization methods and improved agronomic techniques can significantly enhance nitrogen utilization efficiency (NUE) (Radočaj et al., 2022; Ren et al., 2022; Luo et al., 2023). For example, adjusting fertilization strategies, such as altering the depth of fertilization, can increase nitrogen use efficiency by 38.37%, yield by 13.83%, and reduce nitrogen loss by 70.23% compared with traditional methods (Wu et al., 2021). Changing the timing of fertilization can reduce total nitrogen input by 15% without yield loss (Xie et al., 2007). Controlled-release fertilizers (CRF), a novel type of fertilizer (Haydar et al., 2024), regulate the nitrogen release rate through coating technologies (Sim et al., 2021) and matching crop nitrogen demand (Slafer and Savin, 2018; Vejan et al., 2021). This enhances the nitrogen uptake (Li et al., 2020), lowers soil inorganic nitrogen concentration, and reduces the nitrogen loss (Azeem et al., 2014; Momesso et al., 2022; Zhang et al., 2024). Moreover, strategies to enhance crop NUE include selecting crop varieties that utilize soil nitrogen effectively and reduce nitrogen loss (Ciampitti et al., 2022). This is crucial to sustainable agricultural development. Therefore, adopting a comprehensive approach to nitrogen fertilization management and crop selection is important for improving NUE (Kant et al., 2011; Guo et al., 2020; Geng et al., 2021). Integrating these methods can effectively enhance agricultural production efficiency and reduce environmental impacts, contributing to global food security and sustainable development.
Modern agricultural research has emphasized the importance of matching plant root systems with available soil resources for efficient nutrient absorption (Jin et al., 2017; Gebre and Earl, 2021; Chen et al., 2022c). Root-foraging processes exhibit significant spatiotemporal heterogeneity owing to varying soil resource availability across different layers (Mou et al., 2012; Postma et al., 2014; Hui et al., 2022). Adjusting fertilization depth is a critical agronomic practice that can significantly affect root growth and distribution and optimize nutrient absorption efficiency, especially for nitrogen (Su et al., 2015; Chen et al., 2022a, 2024). Studies have demonstrated that spatially adjusting soil nitrate content under different rainfall conditions enhances deep soil root characteristics, indirectly improving the nitrogen nutrient status of wheat plants (Wang et al., 2022b). In trials with spring maize, increasing fertilization depth compared to conventional methods resulted in significant improvements in root length density: 18% for vertical roots, 14% for inter-row roots, and 24% for intra-row roots at soil depths of 0–1.0m. Similarly, the root surface area density increased by 39%, 17%, and 22%, respectively (Wu et al., 2022b). The application of fertilizer at depths of 15 and 25 cm, rather than 5 cm, increased maize nitrogen absorption by 8.07% and 17.41%, NUE by 17.79% and 38.37%, and maize yield by 5.68% and 13.83%, respectively (Wu et al., 2021). Optimizing the fertilization depth is crucial for improving NUE and regulating crop growth and yield (Jia et al., 2023; Wang et al., 2023b). These practices enable the agricultural production to mitigate environmental impacts while ensuring sufficient crop nutrition and promoting sustainability. This approach is particularly relevant for deep-rooting crops such as sunflowers, maize, and wheat, which can access nutrients from deeper soil layers (Connor and Hall, 1997; Wasson et al., 2012; Lynch, 2013; Zhang et al., 2023).
Sunflower, known for its strong root system adaptability, significantly enhances nitrogen absorption rate, utilization, and crop yield through deep root growth (Kiniry et al., 1992; Ma et al., 2021). Its root system can extend into deeper soil layers, effectively utilizing nutrients beyond the surface layer (Thorup-Kristensen et al., 2020). However, current agricultural research lacks sufficient analysis of the impact of moderately deep nitrogen fertilization on root distribution and spatiotemporal matching of soil nutrients in sunflower cultivation. In agricultural studies, an accurate understanding of the correlation between soil nitrate content and root length density is crucial to elucidate the interactions between crop roots and soil nutrients (Li et al., 2018; Lopez et al., 2022). This analysis deepens our understanding of how roots adapt to nutrient distribution, thereby affecting growth and yield. Despite their significance, current analytical methods often fail to precisely capture subtle differences between soil layers and their complex relationship with root growth. Therefore, the aim of this study was to develop a refined and comprehensive analytical method to accurately assess the correlation between soil nitrate distribution and root growth. We introduced an improved weighted Jaccard index (Real and Vargas, 1996; Bag et al., 2019), which considers not only the direct match between soil nitrate content and root length density but also the interrelationships among different soil layers, providing a more comprehensive analytical perspective. This method allowed us to reveal the complex interactions between crop roots and soil nutrients more precisely, providing theoretical support for optimizing fertilization strategies and improving crop production efficiency (Duan et al., 2019; Velasco-Muñoz et al., 2021).
The main objectives of this study were to (1) analyze the impact of deep CRF on the growth distribution of the sunflower root system and its role in yield and nutrient use efficiency, (2) investigate the compatibility between deep CRF and root system growth, and (3) determine the ideal CRF depth to promote an adaptive root system structure in sunflowers through more effective fertilization methods, thereby achieving higher yields. Moreover, this experiment can also contribute to refining existing soil and crop models to simulate root growth, crop yield, and nutrient use efficiency.
The experiment was conducted in the Ganzhaomiao Town experimental field, Linhe District, Bayannaoer City, Inner Mongolia, to investigate the effects of different fertilizer depths over two consecutive growing seasons in 2020 and 2021. The site coordinates are 107°16′42″E and 40°47′54″N, with predominantly silty loam soil (USDA classification). The 0–100 cm soil layer had an average bulk density of 1.41 g/cm3, an average organic matter mass ratio of 6.19 g/kg, an average hydrolysable nitrogen mass ratio of 34.43 mg/kg, an average available phosphorus mass ratio of 1.84 mg/kg, and an average available potassium mass ratio of 113.04 mg/kg. The soil salt content prior to planting averaged 3.2 g/kg, with an average pH of 8.4. The experimental site experiences an annual average temperature of 6.8 °C, precipitation of 138.8 mm, and total annual sunshine hours of 3229.9 h.
The test field comprised 12 plots, each 144 m2 (14 m×6 m), arranged in triplicate for the four treatments. This study used CRF with a nitrogen application rate of 225 kg/ha. Three depths of fertilizer application were tested: 5 ± 0.5 cm (low), 15 ± 0.5 cm (medium), and 25 ± 0.5 cm (high). Additionally, treatments with TNF at 225 kg/ha and a depth of 5 ± 0.5 cm were included. Plastic films were used between the plots to isolate them and to prevent water, salt, and nitrogen interactions. The CRF was the sixth generation from Luyang, with an N:P:K ratio of 28:12:10. The CRF was applied to the farmland at the corresponding depth through manual trenching before mulching. For the TNF, diammonium phosphate (containing 18% N and 46% P2O5) was used as the base fertilizer and was applied at the same depth through manual trenching before mulching, similar to the CRF treatment; topdressing consisted of urea, which was manually spread before irrigation at the bud stage. Diammonium phosphate (1/3 N) was applied before sowing, and urea (2/3 N) was manually applied before irrigation at the budding stage. Sunflowers were irrigated to a depth of 120 mm during their growth period in both 2020 and 2021 using furrow irrigation on July 14th. All the other field management practices were consistent. Sunflower (Xinjiang Sanrui, SH361) was planted using a mechanical film covering and manual planting. The planting pattern involved one film for every two rows, with sunflower plant and row spacing set at 0.4 m and 0.9 m, respectively, resulting in a planting density of approximately 27,777 plants per hectare. Sowing occurred on May 22, 2020, and May 30, 2021, with harvest dates set for September 24 and September 29 of the respective years, resulting in growth periods of 126 and 123 d.
Meteorological data (Figure 1), including solar radiation, air temperature, relative humidity, precipitation, and wind speed, were collected at one-hour intervals using an automated meteorological station (Onset Computer Inc., U30, Hobo, USA) located in the experimental field.
Figure 1. Precipitation (Rain), maximum and minimum temperatures (Tmax and Tmin), and solar radiation (Srad) during the crop fertility period in 2020 and 2021.
Soil water and NO3-N contents were measured using a soil auger (Beijing New Landmark Soil Equipment Co., Ltd., 0301, XDB, CHN) in covered and exposed areas at vertical depths of 0–10 cm, 10–20 cm, 20–30 cm, 30–40 cm, 40–50 cm, 50–60 cm, 60–80 cm, and 80–100 cm. Samples were collected every 10–15 d, and each sample was replicated three times. The soil samples were divided into two parts: one for measuring soil moisture content and the other air-dried, crushed, and sieved through a 1 mm mesh. Subsequently, 5 g of the sample was soaked in 25 mL of 2 mol/L potassium chloride solution. After stirring and filtering, the NO3-N concentration was determined using an ultraviolet spectrophotometer (Beijing General Instruments Co., Ltd., TU-1901, Beijing, China).
Total nitrogen concentration in sunflowers was determined using the micro-Kjeldahl method. Every 15–20 d, five sunflower plants were randomly selected from the diagonal and central areas of each plot using a five-point method. The plant samples were ground, sieved, and digested with a H2SO4-H2O2 solution. Total nitrogen concentration was measured using a Kjeldahl apparatus (China Ocean Energy Future Technology Group, K9860, China).
During the seedling stage (June 26, 2020 and July 2, 2021, respectively), bud stage (July 20, 2020 and July 26, 2021, respectively), flowering stage (August 9, 2020 and August 12, 2021, respectively), and post-flowering stage (September 10, 2020 and September 18, 2021, respectively), root samples from well-grown sunflowers were collected from the soil cross-section at depths of 0–10 cm, 10–20 cm, 20–30 cm, 30–40 cm, 40–50 cm, 50–60 cm, 60–70 cm, 70–80 cm, and 80–100 cm. The collected root samples were washed, sun-dried, and scanned using the Epson Perfection V700 PHOTO scanner. The root parameters were determined using WinRHIZO software.
From each plot, ten sunflowers were consistently selected for measuring mature sunflower yield, and this process was repeated three times.
The Agricultural Production Systems sIMulator (APSIM) is a versatile process-based modeling platform designed specifically for agricultural systems. It integrates multiple modules that cover various crop types, soil dynamics, and management strategies. APSIM can simulate crop growth, soil moisture, and nitrogen dynamics on a daily scale and is applicable to diverse management practices, farming systems, and environmental conditions. This model has been widely used in numerous studies to simulate agricultural production systems worldwide. The inputs to the model included daily meteorological data (such as solar radiation, maximum/minimum temperatures, and precipitation) and soil hydraulic parameters (such as bulk density, saturated water content, field capacity, and wilting point).
This study adopted the APSIM simulation, integrating the modules for fertilizers, soil moisture, soil nitrogen, and sunflowers. APSIM modules that control water and soil nitrogen are particularly crucial. The APSIM SoilWat module employs a cascade water balance model to estimate water and solute movement between soil layers, surface runoff and evaporation, and drainage from the system. This module balances the water content in each soil layer based on inputs and outputs. Soil water movement occurred through saturated, unsaturated, and oversaturated flows, each of which had specific model equations and parameters. The SoilN module manages the plant-available nitrogen supply, nitrate leaching, and nitrogen loss through denitrification. This module tracks the nutrient flow in the nitrogen cycle through mineralization, immobilization, nitrification, denitrification, and urea hydrolysis processes. Simulations involving CRF were conducted by adjusting the urea hydrolysis rate to approximate the CRF conditions. The APSIM model accommodates various fertilizer types, including urea and CRF, by introducing different release periods into the inorganic fertilizer module. The APSIM sunflower module simulated the daily root system growth from germination to grain-filling onset. The increase in root depth was calculated based on the daily growth rate and multiple factors. Daily root biomass growth was proportional to the shoot yield. Each growth stage had a specified root-to-shoot ratio that changed continuously from emergence to flowering. APSIM simulated the daily aging of root mass and length at a specific ratio, with aged roots becoming new organic matter incorporated into the soil nitrogen module.
This study calibrated the APSIM model using 2020 data and validated it with 2021 data, with the aim of minimizing discrepancies between observed and measured values. APSIM version 7.10 was employed in this study.
In the APSIM model, the calibrated soil parameters are summarized as follows. The soil hydraulic parameters included saturated hydraulic conductivities (Ks1, Ks2, Ks3, and Ks4) with values of 53.1, 32.6, 26.5, and 32.2 mm/mm, respectively, for layers 0–20 cm, 20–40 cm, 40–60 cm and 60–100 cm, respectively. The saturated water contents (θs1, θs2, θs3, and θs4) of these layers were calibrated to 0.270, 0.281, 0.442, and 0.462 mm/mm, respectively. The field capacities (Fc1, Fc2, Fc3, and Fc4) were set to 0.243, 0.256, 0.421, and 0.452 mm/mm, respectively, and the wilting point water contents (θwp1, θwp2, θwp3, and θwp4) were calibrated to 0.09, 0.09, 0.10, and 0.11 mm/mm, respectively. Nitrogen turnover parameters included a soil nitrification potential of 40 μg NH4-/g soil, NH4- concentration at half potential of 90 ppm, denitrification rate coefficient of 0.0006 kg soil/mg C per day, and a power term (water factor for denitrification) set at 1.
The calibrated genetic coefficient values of the APSIM model for sunflowers are detailed as follows. The effective accumulated temperature from the end of the seedling stage to flower bud differentiation (tt_endjuv_to_init) was calibrated to 450°C/d. The temperature required for flower bud differentiation into flag leaves (tt_fi_to_flag) was set at 430°C/d. The effective accumulated temperature from flowering to the start of grain (tt_flower_to_start_grain) was 150°C/d, and from flowering to maturity (tt_flower_to_maturity) it is calibrated at 1100°C/d. The total leaf area coefficient (tpla_prod_coef) was set at 0.017, and the leaf emergence rate (rel_leaf_init_rate) at 0.5 leaf/d. The leaf senescence coefficient (spla_prod_coef) and the intercept (spla_intercept) were calibrated to 0.0035 and 0.01, respectively. The radiation use efficiency (RUE) is set at 1.15 g/MJ, and the daily increase in the harvest index (hi_incr) was 0.011.
(1) Principle and Improvement of the Jaccard Index
In bioinformatics and statistics, the Jaccard index can be widely applied to quantify similarity or overlap between two sample sets (Equation 1) (Real and Vargas, 1996). It is calculated as the ratio of the size of the intersection to the union of two sets, A and B:
where A∩B is the number of elements in the intersection of sets A and B, and A∪B is the number of elements in their union.
To apply this index to analyze nitrate-nitrogen content and root length density, these metrics should first be categorized. The nitrate nitrogen content was divided into ten gradations within the 0–48 mg/kg range, with each gradation spanning 4.8 mg/kg. Similarly, the root length density was divided into ten gradations within the 0–2.8 cm/cm3 range, with each gradation spanning 0.28 cm/cm³. This categorization assigns each sample point to a specific gradation, thereby simplifying the matching analysis.
This study introduced a weighted mechanism to consider the correlation between adjacent gradations. Even if the samples were not in the same gradation, a certain degree of match was assigned if they were in adjacent gradations, based on their distance. The weight for a perfect match (same gradation) was 1; for adjacent gradations, it was 0.8; and it decreased by 0.2 for more distant gradations, until it reached 0. This weighting reflects the actual differences between the samples, thus enhancing the analysis. The improved formula for the weight calculation is:
The weight calculation formula can be represented as
where W(g) is the weight at grade g; G is the specified grade (e.g., grade of soil nitrate concentration or root length density); g represents the current grade under consideration, ranging from 1 to 10; and α is the weight interval, which is a fixed constant that controls the rate of change in weight with distance. In this study, α was set to 0.2.
The weighted intersection and union are calculated as follows:
where N∩R is the weighted intersection of soil nitrate nitrogen and root length density, and N∪R is the weighted union, with WN(g) and WR(g) being the weights for soil nitrate nitrogen and root length density at gradation g, respectively.
The Weighted Root Jaccard Index is calculated as:
where RJIndex is the Weighted Root Jaccard Index, and n is the number of days per growth stage.
This improved method involves the computation of weights, weighted intersection and union, and Weighted RJIndex. This approach facilitated a more precise assessment of the match between soil nitrate-nitrogen content and root length density, particularly concerning subtle differences between the soil layers. This advancement provided a new tool for agricultural and ecological research, improving our understanding of the interactions between crop root systems and soil nutrients.
(2) NUE is a key agricultural indicator that measures the efficiency of crop nitrogen fertilizer utilization. It is calculated as the ratio of the crop yield to the amount of nitrogen absorbed by the crop.
where Y (kg/ha) is the yield of each treatment, and SNU (kg/ha) is the sunflower nitrogen uptake.
In this study, the performance of the model in simulating soil NO3-N concentration (SNC), sunflower root length density (RLD), crop nitrogen uptake, and yield was assessed using several statistical methods. These included the Coefficient of Determination (R2), where a higher value indicated a better fit of the model to the data; Root Mean Square Error (RMSE), where lower values indicated a better fit; and Mean Absolute Error (MAE), where a lower value indicated more accurate model predictions.
Where, Mi represents the observed value, Si represents the simulated value, M is the average of the observed value, and n is the sample size.
In this study, data processing was conducted using Microsoft Excel 2019, and graphical representations were generated using Origin 2021 software, Python programming was used to calculate the correlation between soil nitrate nitrogen and sunflower root length density.
This APSIM model was employed to simulate soil NO3-N concentration (SNC), sunflower root length density (RLD), crop nitrogen uptake, and yield throughout the entire growth cycle. The model was calibrated using experimental data from 2020 and validated using data from 2021. Performance evaluation included key indicators such as the coefficient of determination (R2) (Equation 7), root mean square error (RMSE) (Equation 8), and mean absolute error (MAE) (Equation 9) (Figures 2, 3 and Table 1).
Figure 2. Statistical results for APSIM calibration (2020) and validation (2021) for soil NO3-N concentrations (SNC), controlled-release fertilizer (CRF) at three depths of N-fertilizer application (5, 15, and 25 cm) and traditional nitrogen fertilizer (TNF) applied at a 5 cm depth. R2, RMSE, and MAE are the determination coefficient, root mean square error, and mean absolute error, respectively.
Figure 3. Statistical results for APSIM calibration (2020) and validation (2021) for root length density (RLD), controlled-release fertilizer (CRF) at three depths of N-fertilizer application (5, 15, and 25 cm) and traditional nitrogen fertilizer (TNF) applied at a 5 cm depth. R2, RMSE, and MAE are the determination coefficient, root mean square error, and mean absolute error, respectively.
Table 1. Statistical results for APSIM calibration (2020) and validation (2021) for nitrogen uptake and yield, controlled-release fertilizer (CRF) at three depths of N-fertilizer application (5, 15, and 25 cm) and traditional nitrogen fertilizer (TNF) applied at a 5 cm depth.
During 2020–2021, the RMSE values were 0.421–1.801 mg/kg for SNC. 0.062–0.093 cm/cm3 for sunflower RLD, 5.07–8.88 kg/ha for sunflower nitrogen uptake, and 168.37–178.52 kg/ha for yield. The average R2 values were 0.895 (SNC), 0.894 (RLD), 0.764 (NU), and 0.893 (yield), with corresponding average MAE values of 0.989 mg/kg, 0.071 cm/cm3, 6.37 kg/ha, and 151.45 kg/ha. These findings indicate the high precision and strong predictive capability of the model for simulating these variables from 2020 to 2021.
This study analyzed the 2020 and 2021 experimental data to investigate the relationship between fertilizer application depth and the distribution of SNC (Figure 4). The results indicated that at a depth of 5 cm, the SNC in the soil surface was significantly higher than that in deeper layers. In contrast, depths of 15 and 25 cm resulted in higher SNC in the deeper soil layers. Throughout the growth period, SNC under TNF treatment was lower than that under CRF treatment with the same amount of nitrogen. Specifically, during the 2020 and 2021 experimental periods, in the 0–10 cm soil layer during the sunflower seedling stage, the SNC of CRF-5 was 86.83% and 695.73% higher than that of the CRF-15 and CRF-25 treatments, respectively. At the budding and flowering stages, it was higher by 35.73% and 206.27%, respectively. In the 10–20 cm soil layer, at the seedling stage, the SNC of the CRF-15 treatment was 93.63% and 144.94% higher than that of the CRF-5 and CRF-25 treatments, respectively. At the budding and flowering stages, they were 11.08% and 33.28% higher, respectively. In the deep soil layer (20–100 cm), from the seedling stage to the end of the flowering stage, the SNC of the CRF-25 treatment was 19.91% and 13.44% higher than that of the CRF-5 and CRF-15 treatments, respectively. At the maturity stage, within the 0-40 cm soil layer, the SNC of the CRF-5 treatment increased by 5.00% and 18.81% compared with CRF-15 and CRF-25, respectively. In the 40–60 cm soil layer, CRF-25 increased by 11.84% and 8.13% compared with CRF-5 and CRF-15, respectively.
Figure 4. Soil NO3-N concentrations (SNC) in 0–100 cm soil under controlled-release fertilizer (CRF) at three depths of N-fertilizer application (5, 15, and 25 cm) and traditional nitrogen fertilizer (TNF) applied at a 5 cm depth from 2020 to 2021.
Throughout the growth period, the SNC of the CRF-5 treatment in the 0–100 cm soil layer was 114.01% higher than that of the TNF-5 treatment at the same nitrogen application rate. These findings are important for understanding the effects of deep CRF application on SNC distribution and its potential effects on soil fertility and plant growth.
The 2020–2021 data demonstrated that within each treatment group, sunflower RLD initially increased and then decreased throughout the growth period. Overall, RLD decreased gradually with increasing soil depth across the entire soil profile (Figure 5). Under the CRF-5 treatment, RLD was mainly concentrated in the surface layer, whereas under the CRF-15 and CRF-25 treatments, it increased at greater depths. Specifically, during the seedling stage, the RLD of the CRF-15 treatment in the 0–100 cm soil profile was 11.15% and 14.02% higher than that of the CRF-5 and CRF-25 treatments, respectively. At the budding, flowering, and maturity stages, these differences changed to 9.38% and 15.21%, 12.05% and 14.37%, and 17.63% and 19.67%, respectively, indicating that the RLD under CRF-15 treatment reached its maximum value at all growth stages. These findings suggest that fertilizer depth can influence the downward growth and distribution of the root system and that an appropriate depth can enhance sunflower RLD at different growth stages.
Figure 5. Effects of controlled-release fertilizer (CRF) at three depths of N-fertilizer application (5, 15, and 25 cm) and traditional nitrogen fertilizer (TNF) applied at a depth of 5 cm on the vertical distribution and distribution proportion of different soil layers (bar charts embedded in each figure) of root length density (RLD) of sunflower at seedling, budding, flowering, and maturity growth stages from 2020 to 2021.
During the seedling, budding, flowering, and maturity stages of sunflower growth, the RLD of the CRF-5 treatment was 26.01%, 22.17%, 27.23%, and 19.32% higher than that of the TNF-5 treatment with the same amount of nitrogen, respectively. This suggests that CRF may enhance the growth of the crop root system by adjusting SNC. The bar charts in each figure illustrate that, under CRF treatments, as the depth of fertilizer application increased, the proportion of RLD in the 0–20 cm soil layer gradually decreased, whereas RLD in the 20–100 cm soil layer showed an increasing trend. These findings highlight the potential of different CRF fertilizer placement positions to optimize crop root distribution and promote downward root growth.
Using the root system Jaccard index (Equations 2–5) to quantify the match between crop RLD and SNC revealed the effect of CRF treatment depth on their compatibility (Figure 6). During the seedling stages of 2020 and 2021, the average Jaccard index of the root system in the 0–20 cm soil layer for the CRF-25 treatment was 48.48% and 34.44% higher than that for the CRF-5 and CRF-15 treatments, respectively, indicating better compatibility between RLD and SNC in the soil surface layer. However, during the budding, flowering, and maturity stages, the CRF-15 treatment had the highest average Jaccard index of root system. Specifically, during the budding and flowering stages, the CRF-15 treatment was 81.28% and 7.27% higher than that of the CRF-5 and CRF-25 treatments, respectively, whereas at the maturity stage, it increased by 11.18% and 17.42%, respectively. In the 20–60 cm soil layer, the Jaccard index ranking of the average root system during the seedling stage was CRF-5>CRF-25>CRF-15. During the budding and flowering stages, it was CRF-15>CRF-5>CRF-25, with the CRF-15 treatment being 15.76% and 10.37% higher than that of the CRF-5 and CRF-25 treatments, respectively. By the maturity stage, it changed to CRF-25>CRF-15>CRF-5, with the CRF-25 treatment being 38.58% and 15.83% higher than the CRF-5 and CRF-15 treatments, respectively. In the deeper 60–100 cm soil layer, the Jaccard index ranking of the root system during the seedling and budding plus flowering stages was CRF-5>CRF-15>CRF-25. At the maturity stage, there was essentially no difference in the average Jaccard index of the root system among the different fertilizer depths.
Figure 6. Root Jaccard Index of soil NO3-N concentrations (SNC) and root length density (RLD) in the 0–100cm soil profile during the growing season under controlled-release fertilizer (CRF) at three depths of N-fertilizer applications (5, 15, and 25 cm) and traditional nitrogen fertilizer (TNF) applied at a 5 cm depth, from 2020 to 2021.
Throughout the 0–100 cm soil profile, the average Jaccard index of the root system was consistently better for the CRF-15 treatment than for CRF-25 and CRF-5, increasing by 6.60% and 7.34%, respectively, during the seedling, budding plus flowering, and maturity stages. This suggests that CRF application at a depth of 15 cm can significantly improve the match between RLD and SNC throughout the growth period. Additionally, the root system Jaccard index for the CRF-5 treatment was 20.45% higher than that for the TNF-5 treatment with the same nitrogen application rate, indicating that CRF treatments can better optimize the match between RLD and SNC than TNF treatments.
This study investigated the effects of different CRF fertilizer application depths (5, 15, and 25 cm) on sunflower yield, nitrogen uptake (NU), and NUE in field experiments (Table 2). Synthesizing the data from 2020 and 2021 revealed a significant impact of fertilizer application depth on these indicators. In terms of yield, the CRF-15 treatment outperformed the other treatments in both years, with yields of 8.62% and 10.25% higher than the CRF-5 and CRF-25, respectively, indicating that medium-depth fertilization promoted crop growth and yield. Compared with TNF-5, the CRF-5 increased the yield by 30.62% under the same nitrogen application rate. CRF-15 exhibited the highest NU capacity, which was 4.82% and 5.97% higher than that of CRF-5 and CRF-25, respectively, highlighting the role of medium-depth fertilization in promoting effective NU. Regarding NUE (Equation 6), CRF-15 also exhibited higher efficiency, with NUE 3.63% and 4.04% higher than those of CRF-5 and CRF-25, respectively. This suggests that at a depth of 15 cm, the NUE was optimized, enhancing the nitrogen absorption and utilization efficiency of the crop. Compared to TNF-5, under the same nitrogen application rate, the NU and NUE under CRF-5 increased by 14.95% and 13.45%, respectively, indicating that CRF treatment significantly improved yield, NU, and NUE compared to TNF.
Table 2. Biennial comparative analysis of yield, nitrogen uptake (NU), and nitrogen use efficiency (NUE) under controlled-release fertilizer (CRF) at three depths of N-fertilizer application (5, 15, and 25 cm) and traditional nitrogen fertilizer (TNF) applied at a 5 cm depth from 2020 to 2021.
Overall, these findings suggested that CRF application at a depth of 15 cm significantly enhances sunflower yield, NU capability, and NUE. This depth likely benefits from being closer to the main root zone of the crop, facilitating effective nitrogen absorption and utilization by the root system.
This study indicated a significant impact of CRF application depth on SNC distribution, which is crucial for enhancing NUE and reducing environmental pollution (Xia et al., 2022). Shallow CRF application (5 cm) resulted in notably higher NO3-N concentrations in the surface soil than in the deeper layers, whereas deeper application (15 and 25 cm) increased NO3-N concentration in the lower soil layers (Figure 4). This is consistent with previous findings (Wu et al., 2022a; Chen et al., 2022c). The impact of CRF application depth on SNC distribution was multifaceted. Shallow fertilization leads to surface soil NO3-N accumulation owing to higher evaporation rates and limited water penetration, whereas deep fertilization promotes the downward NO3-N percolation with soil moisture, reaching deeper layers (Kanwar et al., 1985). Moreover, fertilization depth may influence root system efficiency in NO3-N absorption. The shallow fertilization can be more readily absorbed, affecting NO3-N distribution, whereas deep fertilization may stimulate downward root growth for NO3-N absorption from the deeper soil layers (Liu et al., 2018).
In summary, the effect of fertilization depth on SNC can be influenced by various factors, such as moisture dynamics, microbial activity, and root absorption. Understanding these mechanisms is crucial for guiding fertilization strategies in modern agriculture with the aim of enhancing nitrogen fertilizer efficiency and mitigating environmental pollution.
RLD is a crucial indicator of root distribution in the soil, directly affecting the ability of a crop to absorb NO3-N (Dusserre et al., 2009). This study demonstrated a strong correlation between RLD and SNC, highlighting the close link between soil nitrogen availability and crop root system structure. Across different fertilizer depth conditions, changes in root length density reflect the adaptation of the root system to nitrogen availability (Liu et al., 2018; Chen et al., 2022a). Shallow fertilization (5 cm) resulted in a higher RLD in the surface soil, indicating root growth in areas with higher SNC (Jia et al., 2020). In contrast, deep fertilization (15 and 25 cm) led to relatively higher RLD in deeper soil layers, suggesting downward root growth to access nitrogen in deeper layers (Figure 5) (van der Bom et al., 2020).
Root development and distribution are vital mechanisms for crops to adapt to the soil environment, particularly regarding soil nutrient distribution (Giehl and von Wiren, 2014; Zhao et al., 2020; Kang et al., 2022). The RLD distribution indicated that crop roots could adjust their growth based on the NO3-N distribution, thereby optimizing nutrient absorption (Chen et al., 2020). To effectively absorb the nitrogen, roots should maintain an appropriate growth density in high-N areas (Fageria and Moreira, 2011). Therefore, adjusting fertilizer depth to optimize root growth and the distribution is a potential strategy for influencing soil nitrogen distribution. For instance, medium-depth CRF fertilization (15 cm) may better match the fertilizer with the main root system distribution area, improving nitrate-nitrogen absorption efficiency (Figure 6).
In summary, the correlation between root length density and SNC demonstrated how crop roots responded and adapted to soil nitrogen distribution. This understanding is vital for developing effective fertilization strategies, particularly for enhancing NUE and crop growth. Adjusting fertilizer depth can optimize SNC distribution and influence root structure and function, thereby improving nitrogen absorption and utilization efficiency (Morris et al., 2017).
In this study, different CRF fertilization depths (5, 15, and 25 cm) exhibited significant effects on the sunflower yield, NU, and NUE (Table 2). These effects could result from two main factors. First, fertilization depth directly affected NO3-N distribution in the soil. Second, it indirectly affected root system distribution and functionality. NO3-N distribution is critical for crop growth and yield, as higher concentrations in areas with denser root systems lead to increased absorption efficiency, which is conducive to higher yields (Barber and Mackay, 1986). Therefore, alignment between fertilization depth and root system distribution is crucial for maximizing yield.
In a spring maize experiment (Wu et al., 2021), trials were conducted at fertilizer depths of 5, 15, 25, and 35 cm. The results indicated that at 15 cm and 25 cm depths, maize yield increased by 5.68% and 13.83%, respectively, compared to the 5 cm depth, with NUE improving by 17.79% and 38.37%, respectively. The highest yield and NUE were observed at a depth of 25 cm. However, the 15 cm depth treatment yielded 3.90% and 4.32% higher NUE than the 5 cm and 25 cm treatments, respectively, with average NUE increases of 0.67% and 1.56%, respectively. The highest yield and NUE were obtained at the depth of 15 cm (Table 2). These variations in results may be attributed to meteorological conditions such as precipitation, soil texture, and initial nutrient concentration in the soil. Overall, the study suggested that appropriately increasing CRF application depth can enhance the crop yield and NUE in agricultural production. Medium-depth fertilization may be preferred because it avoids the excessive SNC in the surface layer and reduces the NO3-N distribution in deeper soil layers, ensuring more even nitrogen distribution across soil layers where crop roots are concentrated. This depth also minimizes N volatilization and leaching losses (Min et al., 2021; Wu et al., 2021), enhancing the availability of NO3-N for crop absorption and use. In medium-depth soils, N had a longer contact time with the root systems, improving NUE. Furthermore, the soil moisture and temperature conditions at this depth may promote root growth and enhance microbial activity, which facilitates N transformation through mineralization, making it more readily absorbable by crops (Niu et al., 2021). Therefore, this study demonstrated that the medium-depth application of CRF (such as at 15 cm) performed best, likely due to the creation of favorable conditions for crop nitrogen absorption, resulting in improved yield and NUE (Chen et al., 2022b). These findings are significant for guiding agricultural practices, particularly the application of precision fertilization techniques, to enhance NUE and crop productivity.
The findings of this study hold practical value in agriculture. Optimizing CRF application depth can improve NUE, reduce costs (Sainju et al., 2006), and increase yields (Liu et al., 2022; Wu et al., 2022b), thus benefiting small-scale farmers with limited resources. This optimization also mitigates the environmental impact of nitrogen fertilizers, which could be crucial for addressing climate change and protecting ecosystems (Wu et al., 2021). The application of the weighted Jaccard index proposed a refined method for analyzing soil-root system interactions. This index considered not only the direct match between the SNC and RLD but also the match between adjacent gradations. While showing advantages, the practical application of the weighted Jaccard index faced challenges such as selecting the appropriate gradation and weight distribution, which significantly affected the analysis results. Future research should focus on effectively determining these parameters effectively to ensure the accuracy and reliability of the analysis. Additionally, further exploration is needed regarding the performance of the index in handling extreme values. Future studies could also investigate its application in broader agricultural and ecological research, such as assessing the responses of different crop varieties to soil nutrients or evaluating the impact of climate change on soil-root system interactions (Cangioli et al., 2022; Lamichhane et al., 2024).
Future research should be conducted under a broader range of geographical and climatic conditions to verify the general applicability of the findings of this study, considering the influence of different soil types and environmental conditions. It is also crucial to investigate how different crop varieties respond to fertilization depth, and how this interacts with crop genetic and physiological traits. Additionally, long-term experimental studies are needed to understand the lasting impact of fertilization depth on soil health and ecosystem services, such as carbon storage and biodiversity.
The APSIM model was used to analyze the effects of CRF application depth on SNC, sunflower RLD, nitrogen uptake, and yield. The results showed that CRF depth significantly influenced SNC distribution, affecting root growth and nitrogen absorption. Shallow fertilization increased the NO3-N concentration in the soil surface layer, whereas deep fertilization moved NO3-N to deeper soil layers. Medium-depth fertilization at 15 cm indicated the best performance in enhancing sunflower yield and nitrogen absorption, highlighting the importance of optimizing CRF application depth to improve NUE and promote crop growth. The application of an improved Jaccard index provided a new method for quantifying soil-root system interactions and enhancing understanding. However, the applicability of this study was limited to specific environmental and crop conditions. Future studies should validate these findings across a broader range of environmental and crop varieties. Further exploration of the long-term impacts of adjusting the fertilization depth on soil health and ecosystem services is necessary.
The original contributions presented in the study are included in the article/supplementary material. Further inquiries can be directed to the corresponding author.
WR: Writing – review & editing, Data curation, Formal analysis, Methodology, Visualization, Writing – original draft. XL: Funding acquisition, Project administration, Resources, Writing – review & editing. TL: Conceptualization, Funding acquisition, Project administration, Writing – review & editing. NC: Methodology, Validation, Writing – review & editing. MX: Data curation, Supervision, Writing – original draft. BL: Software, Validation, Writing – original draft. QQ: Software, Validation, Writing – original draft. GL: Investigation, Validation, Writing – review & editing.
The author(s) declare financial support was received for the research, authorship, and/or publication of this article. This research was jointly supported by the National Key Research and Development Program of China (2021YFC3201202), Fundamental Research Funds for the Inner Mongolia Universities-Science Foundation for Distinguished Young Scholars of Inner Mongolia Agricultural University (BR220302), Inner Mongolia Science and Technology Program (2022YFHH0039), and National Natural Science Foundation of China (52079064).
We are grateful to MX, BL and QQ for their help with the experiments. We would also like to thank all reviewers for their careful, constructive, and insightful comments regarding this work.
The authors declare that the research was conducted in the absence of any commercial or financial relationships that could be construed as a potential conflict of interest.
All claims expressed in this article are solely those of the authors and do not necessarily represent those of their affiliated organizations, or those of the publisher, the editors and the reviewers. Any product that may be evaluated in this article, or claim that may be made by its manufacturer, is not guaranteed or endorsed by the publisher.
Azeem, B., KuShaari, K., Man, Z. B., Basit, A., Thanh, T. H. (2014). Review on materials & methods to produce controlled release coated urea fertilizer. J. Control Release 181, 11–21. doi: 10.1016/j.jconrel.2014.02.020
Bag, S., Kumar, S. K., Tiwari, M. K. (2019). An efficient recommendation generation using relevant Jaccard similarity. Inf. Sci. 483, 53–64. doi: 10.1016/j.ins.2019.01.023
Barber, S. A., Mackay, A. D. (1986). Root growth and phosphorus and potassium uptake by two corn genotypes in the field. Fertilizer Res. 10, 217–230. doi: 10.1007/bf01049351
Cangioli, L., Mancini, M., Napoli, M., Fagorzi, C., Orlandini, S., Vaccaro, F., et al. (2022). Differential response of wheat rhizosphere bacterial community to plant variety and fertilization. Int. J. Mol. Sci. 23, 3616. doi: 10.3390/ijms23073616
Chen, G., Cai, T., Wang, J., Wang, Y., Ren, L., Wu, P., et al. (2022a). Suitable fertilizer application depth enhances the efficient utilization of key resources and improves crop productivity in rainfed farmland on the loess plateau, China. Front. Plant Sci. 13. doi: 10.3389/fpls.2022.900352
Chen, M., Graedel, T. E. (2016). A half-century of global phosphorus flows, stocks, production, consumption, recycling, and environmental impacts. Global Environ. Change 36, 139–152. doi: 10.1016/j.gloenvcha.2015.12.005
Chen, Y., Hu, S., Guo, Z., Cui, T., Zhang, L., Lu, C., et al. (2021b). Effect of balanced nutrient fertilizer: A case study in Pinggu District, Beijing, China. Sci. Total Environ. 754, 142069. doi: 10.1016/j.scitotenv.2020.142069
Chen, L., Li, K. K., Shi, W. J., Wang, X. L., Wang, E. T., Liu, J. F., et al. (2021a). Negative impacts of excessive nitrogen fertilization on the abundance and diversity of diazotrophs in black soil under maize monocropping. Geoderma 393, 114999. doi: 10.1016/j.geoderma.2021.114999
Chen, J., Liu, L., Wang, Z., Zhang, Y., Sun, H., Song, S., et al. (2020). Nitrogen fertilization increases root growth and coordinates the root-shoot relationship in cotton. Front. Plant Sci. 11. doi: 10.3389/fpls.2020.00880
Chen, X., Liu, P., Zhao, B., Zhang, J., Ren, B., Li, Z., et al. (2022c). Root physiological adaptations that enhance the grain yield and nutrient use efficiency of maize (Zea mays L) and their dependency on phosphorus placement depth. Field Crops Res. 276, 108378. doi: 10.1016/j.fcr.2021.108378
Chen, G., Ren, L., Wang, J., Liu, F., Liu, G., Li, H., et al. (2022b). Optimizing fertilization depth can promote sustainable development of dryland agriculture in the Loess Plateau region of China by improving crop production and reducing gas emissions. Plant Soil 499, 73–89. doi: 10.1007/s11104-022-05795-6
Chen, X., Ren, H., Zhang, J., Zhao, B., Ren, B., Wan, Y., et al. (2024). Deep phosphorus fertilizer placement increases maize productivity by improving root-shoot coordination and photosynthetic performance. Soil Tillage Res. 235, 105915. doi: 10.1016/j.still.2023.105915
Ciampitti, I. A., Briat, J. F., Gastal, F., Lemaire, G. (2022). Redefining crop breeding strategy for effective use of nitrogen in cropping systems. Commun. Biol. 5, 823. doi: 10.1038/s42003-022-03782-2
Connor, D. J., Hall, A. J. (1997). “Sunflower physiology,” in Sunflower technology and production, 35, 113–182. doi: 10.2134/agronmonogr35.c4
Duan, J., Shao, Y., He, L., Li, X., Hou, G., Li, S., et al. (2019). Optimizing nitrogen management to achieve high yield, high nitrogen efficiency and low nitrogen emission in winter wheat. Sci. Total Environ. 697, 134088. doi: 10.1016/j.scitotenv.2019.134088
Dusserre, J., Audebert, A., Radanielson, A., Chopart, J. L. (2009). Towards a simple generic model for upland rice root length density estimation from root intersections on soil profile. Plant Soil 325, 277. doi: 10.1007/s11104-009-9978-0
Fageria, N., Moreira, A. (2011). The role of mineral nutrition on root growth of crop plants. Adv. Agron. 110, 251–331. doi: 10.1016/B978-0-12-385531-2.00004-9
Gebre, M. G., Earl, H. J. (2021). Soil water deficit and fertilizer placement effects on root biomass distribution, soil water extraction, water use, yield, and yield components of soybean [Glycine max (L.) merr.] grown in 1-m rooting columns. Front. Plant Sci. 12. doi: 10.3389/fpls.2021.581127
Geng, Y., Wang, J., Sun, Z., Ji, C., Huang, M., Zhang, Y., et al. (2021). Soil N-oxide emissions decrease from intensive greenhouse vegetable fields by substituting synthetic N fertilizer with organic and bio-organic fertilizers. Geoderma 383, 114730. doi: 10.1016/j.geoderma.2020.114730
Giehl, R. F., von Wiren, N. (2014). Root nutrient foraging. Plant Physiol. 166, 509–517. doi: 10.1104/pp.114.245225
Guo, Y., Chen, Y., Searchinger, T. D., Zhou, M., Pan, D., Yang, J., et al. (2020). Air quality, nitrogen use efficiency and food security in China are improved by cost-effective agricultural nitrogen management. Nat. Food 1, 648–658. doi: 10.1038/s43016-020-00162-z
Haydar, M. S., Ghosh, D., Roy, S. (2024). Slow and controlled release nanofertilizers as an efficient tool for sustainable agriculture: Recent understanding and concerns. Plant Nano Biol. 7, 100058. doi: 10.1016/j.plana.2024.100058
Heuer, S., Gaxiola, R., Schilling, R., Herrera-Estrella, L., Lopez-Arredondo, D., Wissuwa, M., et al. (2017). Improving phosphorus use efficiency: a complex trait with emerging opportunities. Plant J. 90, 868–885. doi: 10.1111/tpj.13423
Hui, X., Wang, X., Luo, L., Wang, S., Guo, Z., Shi, M., et al. (2022). Wheat grain zinc concentration as affected by soil nitrogen and phosphorus availability and root mycorrhizal colonization. Eur. J. Agron. 134, 126469. doi: 10.1016/j.eja.2022.126469
Jia, X., Wang, Y., Zhang, Q., Lin, S., Zhang, Y., Du, M., et al. (2023). Reasonable deep application of sheep manure fertilizer to alleviate soil acidification to improve tea yield and quality. Front. Plant Sci. 14. doi: 10.3389/fpls.2023.1179960
Jia, Q., Yang, L., An, H., Dong, S., Chang, S., Zhang, C., et al. (2020). Nitrogen fertilization and planting models regulate maize productivity, nitrate and root distributions in semi-arid regions. Soil Tillage Res. 200, 104636. doi: 10.1016/j.still.2020.104636
Jin, K., White, P. J., Whalley, W. R., Shen, J., Shi, L. (2017). Shaping an optimal soil by root-soil interaction. Trends Plant Sci. 22, 823–829. doi: 10.1016/j.tplants.2017.07.008
Kang, J., Peng, Y., Xu, W. (2022). Crop root responses to drought stress: molecular mechanisms, nutrient regulations, and interactions with microorganisms in the rhizosphere. Int. J. Mol. Sci. 23, 9310. doi: 10.3390/ijms23169310
Kant, S., Bi, Y. M., Rothstein, S. J. (2011). Understanding plant response to nitrogen limitation for the improvement of crop nitrogen use efficiency. J. Exp. Bot. 62, 1499–1509. doi: 10.1093/jxb/erq297
Kanwar, R. S., Baker, J. L., Laflen, J. M. (1985). Nitrate movement through the soil profile in relation to tillage system and fertilizer application method. Trans. ASAE 28, 1802–1807. doi: 10.13031/2013.32522
Kiniry, J. R., Blanchet, R., Williams, J. R., Texier, V., Jones, C. A., Cabelguenne, M. (1992). Sunflower simulation using the EPIC and ALMANAC models. Field Crops Res. 30, 403–423. doi: 10.1016/0378-4290(92)90008-w
Kong, D., Jin, Y., Chen, J., Yu, K., Zheng, Y., Wu, S., et al. (2021). Nitrogen use efficiency exhibits a trade-off relationship with soil N2O and NO emissions from wheat-rice rotations receiving manure substitution. Geoderma 403, 115374. doi: 10.1016/j.geoderma.2021.115374
Lamichhane, J. R., Barbetti, M. J., Chilvers, M. I., Pandey, A. K., Steinberg, C. (2024). Exploiting root exudates to manage soil-borne disease complexes in a changing climate. Trends Microbiol. 32, 27–37. doi: 10.1016/j.tim.2023.07.011
Li, J., Xu, X., Lin, G., Wang, Y., Liu, Y., Zhang, M., et al. (2018). Micro-irrigation improves grain yield and resource use efficiency by co-locating the roots and N-fertilizer distribution of winter wheat in the North China Plain. Sci. Total Environ. 643, 367–377. doi: 10.1016/j.scitotenv.2018.06.157
Li, G., Zhao, B., Dong, S., Zhang, J., Liu, P., Lu, W. (2020). Controlled-release urea combining with optimal irrigation improved grain yield, nitrogen uptake, and growth of maize. Agric. Water Manage. 227, 105834. doi: 10.1016/j.agwat.2019.105834
Liu, W., Ma, G., Wang, C., Wang, J., Lu, H., Li, S., et al. (2018). Irrigation and nitrogen regimes promote the use of soil water and nitrate nitrogen from deep soil layers by regulating root growth in wheat. Front. Plant Sci. 9. doi: 10.3389/fpls.2018.00032
Liu, P., Yan, H., Xu, S., Lin, X., Wang, W., Wang, D. (2022). Moderately deep banding of phosphorus enhanced winter wheat yield by improving phosphorus availability, root spatial distribution, and growth. Soil Tillage Res., 220, 105388. doi: 10.1016/j.still.2022.105388
Lopez, G., Ahmadi, S. H., Amelung, W., Athmann, M., Ewert, F., Gaiser, T., et al. (2022). Nutrient deficiency effects on root architecture and root-to-shoot ratio in arable crops. Front. Plant Sci. 13. doi: 10.3389/fpls.2022.1067498
Luo, L., Zhang, X., Zhang, M., Wei, P., Chai, R., Wang, Y., et al. (2023). Improving wheat yield and phosphorus use efficiency through the optimization of phosphorus fertilizer types based on soil P pool characteristics in calcareous and non-calcareous soil. Agronomy 13, 928. doi: 10.3390/agronomy13030928
Lynch, J. P. (2013). Steep, cheap and deep: an ideotype to optimize water and N acquisition by maize root systems. Ann. Bot. 112, 347–357. doi: 10.1093/aob/mcs293
Ma, G., Liu, W., Li, S., Zhang, P., Wang, C., Lu, H., et al. (2019). Determining the optimal N input to improve grain yield and quality in winter wheat with reduced apparent N loss in the North China plain. Front. Plant Sci. 10. doi: 10.3389/fpls.2019.00181
Ma, T., Zeng, W., Lei, G., Wu, J., Huang, J. (2021). Predicting the rooting depth, dynamic root distribution and the yield of sunflower under different soil salinity and nitrogen applications. Ind. Crops Products 170, 113749. doi: 10.1016/j.indcrop.2021.113749
Min, J., Sun, H., Wang, Y., Pan, Y., Kronzucker, H. J., Zhao, D., et al. (2021). Mechanical side-deep fertilization mitigates ammonia volatilization and nitrogen runoff and increases profitability in rice production independent of fertilizer type and split ratio. J. Cleaner Production 316, 128370. doi: 10.1016/j.jclepro.2021.128370
Momesso, L., Crusciol, C. A. C., Cantarella, H., Tanaka, K. S., Kowalchuk, G. A., Kuramae, E. E. (2022). Optimizing cover crop and fertilizer timing for high maize yield and nitrogen cycle control. Geoderma 405, 115423. doi: 10.1016/j.geoderma.2021.115423
Morris, E. C., Griffiths, M., Golebiowska, A., Mairhofer, S., Burr-Hersey, J., Goh, T., et al. (2017). Shaping 3D root system architecture. Curr. Biol. 27, R919–R930. doi: 10.1016/j.cub.2017.06.043
Mou, P., Jones, R. H., Tan, Z., Bao, Z., Chen, H. (2012). Morphological and physiological plasticity of plant roots when nutrients are both spatially and temporally heterogeneous. Plant Soil 364, 373–384. doi: 10.1007/s11104-012-1336-y
Nie, J., Zhou, J., Zhao, J., Wang, X., Liu, K., Wang, P., et al. (2022). Soybean crops penalize subsequent wheat yield during drought in the North China plain. Front. Plant Sci. 13. doi: 10.3389/fpls.2022.947132
Niu, W., Chen, H., Wu, J. (2021). Soil Moisture and Soluble Salt Content Dominate Changes in Foliar delta(13)C and delta(15)N of Desert Communities in the Qaidam Basin, Qinghai-Tibetan Plateau. Front. Plant Sci. 12. doi: 10.3389/fpls.2021.675817
Postma, J. A., Schurr, U., Fiorani, F. (2014). Dynamic root growth and architecture responses to limiting nutrient availability: linking physiological models and experimentation. Biotechnol. Adv. 32, 53–65. doi: 10.1016/j.bioteChadv.2013.08.019
Radočaj, D., Jurišić, M., Gašparović, M. (2022). The role of remote sensing data and methods in a modern approach to fertilization in precision agriculture. Remote Sens. 14, 778. doi: 10.3390/rs14030778
Real, R., Vargas, J. M. (1996). The probabilistic basis of jaccard’s index of similarity. Systematic Biol. 45, 380–385. doi: 10.1093/sysbio/45.3.380
Ren, B., Guo, Y., Liu, P., Zhao, B., Zhang, J. (2021). Effects of urea-ammonium nitrate solution on yield, N(2)O emission, and nitrogen efficiency of summer maize under integration of water and fertilizer. Front. Plant Sci. 12. doi: 10.3389/fpls.2021.700331
Ren, K., Xu, M., Li, R., Zheng, L., Liu, S., Reis, S., et al. (2022). Optimizing nitrogen fertilizer use for more grain and less pollution. J. Cleaner Production 360, 132180. doi: 10.1016/j.jclepro.2022.132180
Sainju, U. M., Whitehead, W. F., Singh, B. P., Wang, S. (2006). Tillage, cover crops, and nitrogen fertilization effects on soil nitrogen and cotton and sorghum yields. Eur. J. Agron. 25, 372–382. doi: 10.1016/j.eja.2006.07.005
Sim, D. H. H., Tan, I. A. W., Lim, L. L. P., Hameed, B. H. (2021). Encapsulated biochar-based sustained release fertilizer for precision agriculture: A review. J. Cleaner Production 303, 127018. doi: 10.1016/j.jclepro.2021.127018
Slafer, G. A., Savin, R. (2018). Can N management affect the magnitude of yield loss due to heat waves in wheat and maize? Curr. Opin. Plant Biol. 45, 276–283. doi: 10.1016/j.pbi.2018.07.009
Su, W., Liu, B., Liu, X., Li, X., Ren, T., Cong, R., et al. (2015). Effect of depth of fertilizer banded-placement on growth, nutrient uptake and yield of oilseed rape (Brassica napus L.). Eur. J. Agron. 62, 38–45. doi: 10.1016/j.eja.2014.09.002
Tabuchi, M., Abiko, T., Yamaya, T. (2007). Assimilation of ammonium ions and reutilization of nitrogen in rice (Oryza sativa L.). J. Exp. Bot. 58, 2319–2327. doi: 10.1093/jxb/erm016
Thorup-Kristensen, K., Halberg, N., Nicolaisen, M., Olesen, J. E., Crews, T. E., Hinsinger, P., et al. (2020). Digging deeper for agricultural resources, the value of deep rooting. Trends Plant Sci. 25, 406–417. doi: 10.1016/j.tplants.2019.12.007
van der Bom, F. J. T., Williams, A., Bell, M. J. (2020). Root architecture for improved resource capture: trade-offs in complex environments. J. Exp. Bot. 71, 5752–5763. doi: 10.1093/jxb/eraa324
Vejan, P., Khadiran, T., Abdullah, R., Ahmad, N. (2021). Controlled release fertilizer: A review on developments, applications and potential in agriculture. J. Control Release 339, 321–334. doi: 10.1016/j.jconrel.2021.10.003
Velasco-Muñoz, J. F., Mendoza, J. M. F., Aznar-Sánchez, J. A., Gallego-Schmid, A. (2021). Circular economy implementation in the agricultural sector: Definition, strategies and indicators. Resources Conserv. Recycling 170, 105618. doi: 10.1016/j.resconrec.2021.105618
Wang, Y., Guo, Q., Xu, Y., Zhang, P., Cai, T., Jia, Z. (2022b). Optimizing urea deep placement to rainfall can maximize crop water-nitrogen productivity and decrease nitrate leaching in winter wheat. Agric. Water Manage. 274, 107971. doi: 10.1016/j.agwat.2022.107971
Wang, Y., Guo, Q., Xu, Y., Zhang, P., Cai, T., Jia, Z. (2023b). Sustainable nitrogen placement depth under different rainfall levels can enhance crop productivity and maintain the nitrogen balance in winter wheat fields. Soil Tillage Res. 233, 105817. doi: 10.1016/j.still.2023.105817
Wang, J., Hussain, S., Sun, X., Zhang, P., Javed, T., Dessoky, E. S., et al. (2022a). Effects of nitrogen application rate under straw incorporation on photosynthesis, productivity and nitrogen use efficiency in winter wheat. Front. Plant Sci. 13. doi: 10.3389/fpls.2022.862088
Wang, X., Wang, M., Chen, L., Shutes, B., Yan, B., Zhang, F., et al. (2023a). Nitrogen migration and transformation in a saline-alkali paddy ecosystem with application of different nitrogen fertilizers. Environ. Sci. pollut. Res. Int. 30, 51665–51678. doi: 10.1007/s11356-023-25984-9
Wasson, A. P., Richards, R. A., Chatrath, R., Misra, S. C., Prasad, S. V., Rebetzke, G. J., et al. (2012). Traits and selection strategies to improve root systems and water uptake in water-limited wheat crops. J. Exp. Bot. 63, 3485–3498. doi: 10.1093/jxb/ers111
Wu, X., Li, J., Xue, X., Wang, R., Liu, W., Yang, B., et al. (2023). Matching fertilization with available soil water storage to tackle the trade-offs between high yield and low N2O emissions in a semi-arid area: Mechanisms and solutions. Agric. Water Manage. 288, 108488. doi: 10.1016/j.agwat.2023.108488
Wu, P., Liu, F., Chen, G., Wang, J., Huang, F., Cai, T., et al. (2022a). Can deep fertilizer application enhance maize productivity by delaying leaf senescence and decreasing nitrate residue levels? Field Crops Res. 277, 108417. doi: 10.1016/j.fcr.2021.108417
Wu, P., Liu, F., Li, H., Cai, T., Zhang, P., Jia, Z. (2021). Suitable fertilizer application depth can increase nitrogen use efficiency and maize yield by reducing gaseous nitrogen losses. Sci. Total Environ. 781, 146787. doi: 10.1016/j.scitotenv.2021.146787
Wu, P., Liu, F., Wang, J., Liu, Y., Gao, Y., Zhang, X., et al. (2022b). Suitable fertilization depth can improve the water productivity and maize yield by regulating development of the root system. Agric. Water Manage. 271, 107784. doi: 10.1016/j.agwat.2022.107784
Xia, H., Riaz, M., Zhang, M., Liu, B., Li, Y., El-Desouki, Z., et al. (2022). Biochar-N fertilizer interaction increases N utilization efficiency by modifying soil C/N component under N fertilizer deep placement modes. Chemosphere 286, 131594. doi: 10.1016/j.chemosphere.2021.131594
Xie, W. X., Wang, G. H., Zhang, Q. C., Guo, H. C. (2007). Effects of nitrogen fertilization strategies on nitrogen use efficiency in physiology, recovery, and agronomy and redistribution of dry matter accumulation and nitrogen accumulation in two typical rice cultivars in Zhejiang, China. J. Zhejiang Univ Sci. B 8, 208–216. doi: 10.1631/jzus.2007.B0208
Zhang, Y., Ren, W., Zhu, K., Fu, J., Wang, W., Wang, Z., et al. (2024). Substituting readily available nitrogen fertilizer with controlled-release nitrogen fertilizer improves crop yield and nitrogen uptake while mitigating environmental risks: A global meta-analysis. Field Crops Res. 306, 109221. doi: 10.1016/j.fcr.2023.109221
Zhang, A., Wang, X.-X., Zhang, D., Dong, Z., Ji, H., Li, H. (2023). Localized nutrient supply promotes maize growth and nutrient acquisition by shaping root morphology and physiology and mycorrhizal symbiosis. Soil Tillage Res. 225, 105550. doi: 10.1016/j.still.2022.105550
Keywords: fertilization depth, root growth, nitrogen fertilizer efficiency, sunflower yield, nutrient matching between root and soil
Citation: Ren W, Li X, Liu T, Chen N, Xin M, Liu B, Qi Q and Li G (2024) Impact of fertilization depth on sunflower yield and nitrogen utilization: a perspective on soil nutrient and root system compatibility. Front. Plant Sci. 15:1440859. doi: 10.3389/fpls.2024.1440859
Received: 30 May 2024; Accepted: 26 July 2024;
Published: 14 August 2024.
Edited by:
Laichao Luo, Anhui Agricultural University, ChinaReviewed by:
Puchang Wang, Guizhou Normal University, ChinaCopyright © 2024 Ren, Li, Liu, Chen, Xin, Liu, Qi and Li. This is an open-access article distributed under the terms of the Creative Commons Attribution License (CC BY). The use, distribution or reproduction in other forums is permitted, provided the original author(s) and the copyright owner(s) are credited and that the original publication in this journal is cited, in accordance with accepted academic practice. No use, distribution or reproduction is permitted which does not comply with these terms.
*Correspondence: Xianyue Li, bGl4aWFueXVlODBAMTI2LmNvbQ==
Disclaimer: All claims expressed in this article are solely those of the authors and do not necessarily represent those of their affiliated organizations, or those of the publisher, the editors and the reviewers. Any product that may be evaluated in this article or claim that may be made by its manufacturer is not guaranteed or endorsed by the publisher.
Research integrity at Frontiers
Learn more about the work of our research integrity team to safeguard the quality of each article we publish.