- 1Department of Soil and Crop Sciences, Texas A&M University, College Station, TX, United States
- 2Texas A&M AgriLife Research Center, Beaumont, TX, United States
A low-input-based farming system can reduce the adverse effects of modern agriculture through proper utilization of natural resources. Modern varieties often need to improve in low-input settings since they are not adapted to these systems. In addition, rice is one of the most widely cultivated crops worldwide. Enhancing rice performance under a low input system will significantly reduce the environmental concerns related to rice cultivation. Traits that help rice to maintain yield performance under minimum inputs like seedling vigor, appropriate root architecture for nutrient use efficiency should be incorporated into varieties for low input systems through integrated breeding approaches. Genes or QTLs controlling nutrient uptake, nutrient assimilation, nutrient remobilization, and root morphology need to be properly incorporated into the rice breeding pipeline. Also, genes/QTLs controlling suitable rice cultivars for sustainable farming. Since several variables influence performance under low input conditions, conventional breeding techniques make it challenging to work on many traits. However, recent advances in omics technologies have created enormous opportunities for rapidly improving multiple characteristics. This review highlights current research on features pertinent to low-input agriculture and provides an overview of alternative genomics-based breeding strategies for enhancing genetic gain in rice suitable for low-input farming practices.
1 Introduction
Agriculture is one of the survival factors for humans on this planet. However, our intensive agriculture practices severely endangered our climate. Agriculture and related activities emitted 9.3 billion tons of CO2 eq. in 2018 (FAO, 2020). Additionally, the agriculture sector contributed around 9% of the US greenhouse gas emission and was the largest supplier of the US N2O emission in 2021 (EPA, 2023). Notably, fertilizer application aiming for higher nitrogen availability was the reason for higher N2O emission, and the contribution of urea fertilization in CO2 emission was 5.2 MMT CO2 eq. (EPA, 2023). The extensive use of chemical fertilizers and pesticides also harms the ecosystem by contaminating groundwater and other natural resources. Although these practices ensure higher yields, they are destroying our environment.
Thus, we need a transition to a sustainable as well as low-input (LI) agriculture system to avoid further environmental problems in the future.
We can plan a sustainable agriculture system by ensuring economic profitability, a healthy environment, and social development while safeguarding our natural resources (Horrigan et al., 2002). One way to build a sustainable agriculture system is to modify our modern farming systems into low-input systems. For instance, researchers described that low-input systems could lead to sustainable agriculture by achieving efficiency in conventional practices (Hill and MacRae, 1996). A profitable yield can be achieved with lower fertilizer input with appropriate nutrient management (Good and Beatty, 2011; Chen et al., 2014). Popular high-yielding varieties require a constant supply of synthetic fertilizers and pesticide. For this reason, the success of these LI systems depends on the development of high yielding cultivars adapted to low-input conditions. Further, Evans (1996) stated that the success of any cropping system depends on the synergistic interaction between all inputs, such as fertilizers, irrigation, weed control, etc. Therefore, agronomic practices that ensure positive interaction between all these inputs will be crucial for LI systems.
Rice is one of the most extensively consumed cereals globally. It is the number one staple food among developing countries and also the most vulnerable crop to climate change (Wassmann et al., 2010). Since a large portion of world population consume rice, rice production needs to be increased to ensure food security across the globe. Further, the global population is forecasted to cross 9 billion by 2050 and more than 10 billion by 2080 (UN, 2022). For this reason, maintaining rice acreage across the globe is very important. However, extensive use of chemical fertilizers and pesticides significantly contributes to agricultural pollution. In this context, growing rice cultivars suited for LI systems is vital in practicing sustainable agriculture.
However, the most challenging part of developing crop cultivars for the LI system is incorporating tolerance to various biotic and abiotic factors, nutrient use efficiency, and higher yields. Therefore, rice breeders should target multiple traits to develop extremely resource-efficient cultivars. From the era of the green revolution, several genotypes were characterized with specific traits through conventional breeding approaches. The genomics era has opened many windows for rice breeders to understand the function of different pathways with traits of interest, which directly support rice improvement. It is challenging for rice breeders to combine all the traits into single cultivars, thus integrated breeding approaches help to develop multiple-traits cultivars (Singh et al., 2024). Fortunately, modern genomics tools increased the efficacy of multi-trait breeding and reduced the time required for integrating numerous traits. Besides, the low-cost genotyping techniques have enabled plant breeders to decipher multiple characteristics of a population in a cheaper and faster way. Speed breeding can be incorporated with various modern breeding tools in numerous steps of a breeding pipeline to reduce the breeding cycle and enhance selection accuracy and efficiency (Gudi et al., 2022). However, more effort must be made to include all the modern genomic tools to develop varieties suitable for LI agriculture. As far as we know, none of the suitable articles are available discussing the LI system for sustainable agriculture regarding the breeding perspectives. Keeping this in mind, our goal is to prepare a precise review on rice breeding for the LI system. Hence, we reviewed the requisite traits and genomics-based strategies for breeding rice for a low-input agriculture system.
2 Environmental impact and need for LI in rice production system
Following the “green revolution,” chemical fertilizer and pesticide use in modern agriculture has increased tremendously worldwide (Figure 1). Although high-input systems ensure rapid fiscal growth, these systems damage the environment. High-input rice cultivation is practiced around the globe to feed the massive population. Rice has higher greenhouse gas emission potential than other major cereals. For example, rice has a 467% higher GWP (Global Warming Potential) than wheat and 169% higher GWP than maize (Linquist et al., 2012). Rice fields are significant contributors to CH4 and N20. Previous data suggested that rice fields are responsible for 30% and 11% of world agricultural CH4 and N2O emissions, respectively (IPCC, 2007). For this extensive rice cultivation, carbon dioxide and other greenhouse gases are increasing quickly, endangering all living beings on Earth.
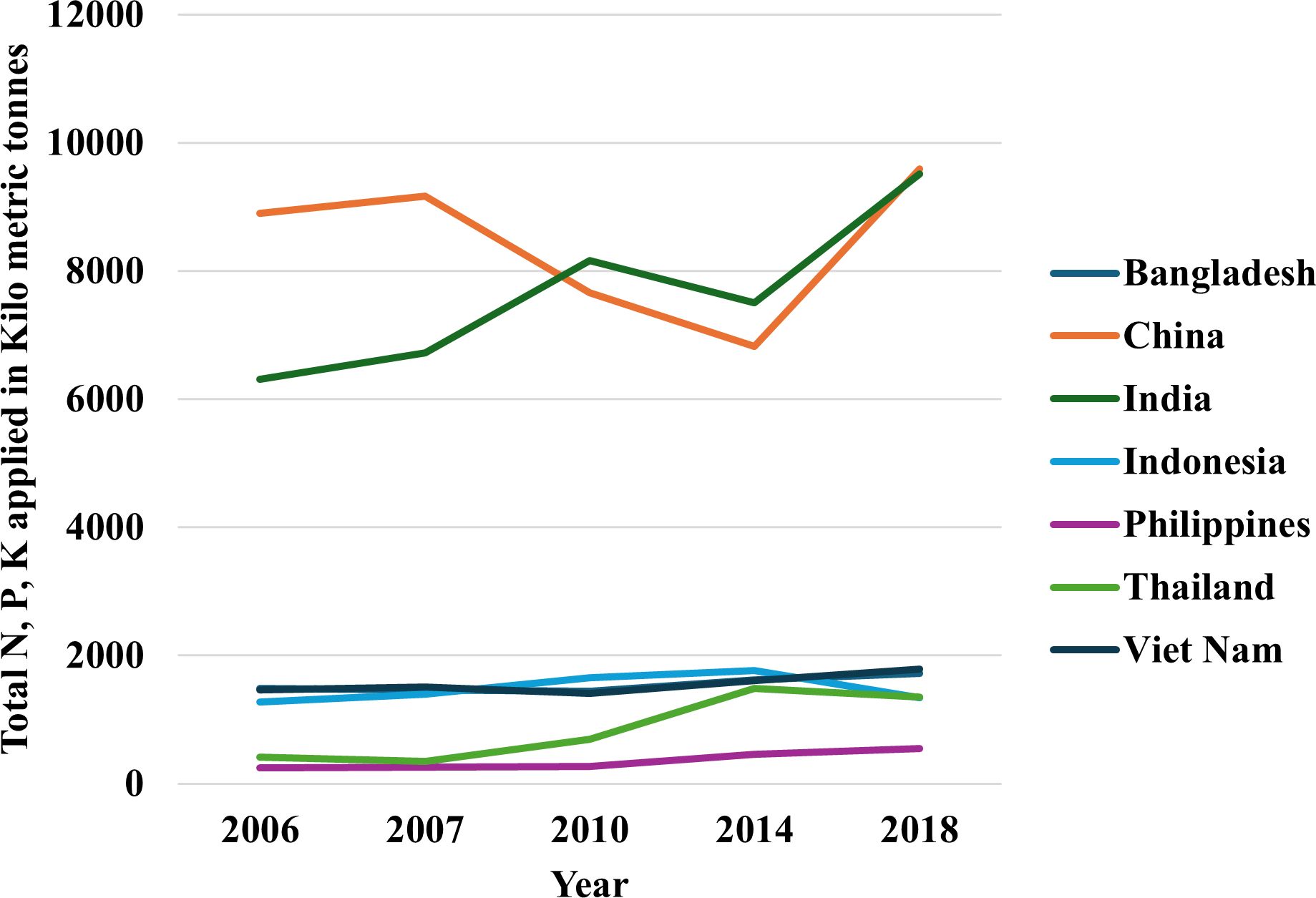
Figure 1 Total N, P2O5, K2O fertilizer used in rice per year in top rice producing countries over the last two dacades (Ludemann et al. 2022a). The dataset used for this figure can be found in Ludemann et al. (2022b).
Furthermore, the heavy dosage of pesticides and fertilizers exert residual effects on foods, creating carcinogenic impact on the consumer’s body. Besides, pesticides contaminate natural resources like water and alter the harmony between many biological processes. Excessive input is closely related to the environmental damage caused by rice cultivation (Ahmad et al., 2023). Therefore, an alternative low-input system is needed to safeguard our nature while sustaining agricultural growth.
Efficient use of fertilizer and exploring plant’s inherent resource use efficiency will reduce greenhouse gas emissions and remove the risk of contamination from chemical pesticides. A previous study reported that low input farming will help in better management of soil fertility in the long run. Since LI farming increases the amount of organic C and stored nutrients in the soil, LI farming will gradually increase soil health (Clark et al., 1998). Management practices like crop rotation will establish proper nutrient cycling and preserve soil productivity. The LI system will also indirectly positively affect biodiversity by minimizing water and air pollution. Moreover, the LI system is the most feasible remedy for the negative impact of conventional agriculture systems.
3 Low-input agriculture
The ongoing modern high-input agriculture system needs an alternate solution due to the fast-changing climate and various agricultural pollutions. Despite having wide cultivation areas across the globe, rice production can be intensified sustainably (Yuan et al., 2021). Though improved varieties are being developed worldwide, significant yield gaps exist in many rice cropping regions. Inefficient fertilizer use and lack of proper management are the prime causes of this discrepancy (Yuan et al., 2021). Moreover, it is essential to simultaneously increase both yield and resource use efficiency for a sustainable farming system. Breeding for better nutrient use efficiency will help to reduce the yield gap present in certain rice growing regions.
Some rice cropping systems have lower yields despite having higher N input (Yuan et al., 2021). In those systems, N (Nitrogen) input can be reduced while having higher yield by increasing crop resource use efficiency (Yuan et al., 2021). Therefore, the development of resource-use-efficient rice cultivars that can be cultivated in LI systems is recommended. Researchers began to emphasize the value of LI systems around the turn of the twenty-first century. These systems use the least production input while management procedures are upheld to guarantee a successful crop output (Parr et al., 2020). The primary focus of low-input systems is minimizing off-farm resources such as pesticides and fertilizer to reduce environmental pollution and improve soil health.
Additionally, LI system relies on on-farm resources like management practices to generate maximum yield output. Notably, LI systems offer a plethora of environmental benefits over conventional methods. For instance, the LI system ensures lower rates of N leaching and mineralization, which helps maintain future sustainability (Poudel et al., 2002). A study conducted for eight years with crop rotation with different crops, including rice, showed that low-input and organic farming improved soil chemical properties (Clark et al., 1998). Although organic farming has long been touted as a sustainable agriculture practice, it produces lower yield per acre than conventional farming (Seufert et al., 2012).
In contrast, the LI system can reduce the trade-offs between environmentally benign farming methods and financial success. Crops in LI systems must rely on effective resource utilization and innate defensive mechanisms since they get minimal external input. Consequently, LI systems yield less than traditional systems. Two significant issues that restrict profitability under low input systems are the lack of N supply and weed competition (Clark et al., 1999). Cultivars suited for low input circumstances should be designed considering multiple traits and a range of selection environments to address different yield-limiting issues. In summary, LI agriculture needs inclusions of two major factors for sustainable rice farming: selection of resource efficient crops and adaptation of proper management practices to minimize the wastage of natural resources. Initially, a goal might be set up for 20% resources reduction during rice crop management i.e. nitrogen and/or and water use, while maintaining similar or enhanced yield.
4 Breeding for low-input agriculture
Plant characteristics are the decisive factor for adaptation in a particular environment. Similarly, the ability of the plant to survive in a pesticide-free and nutrient-scarce system is essential for LI system. Therefore, traits that improve nutrient-usage-efficiency, weed competency, and multiple stress tolerance are critical for LI systems. Breeding for ideal rice plant ensures the incorporation of important traits into a single genotype to develop cultivars for low-input rice. Genes that control seedling establishment, vigor and multiple stress tolerance must be utilized to enhance seedling establishment to the pre-booting stage (Figure 2). For better yield under LI systems, genes controlling grain yield and nutrient use efficiency should be explored and utilized (Figure 2).
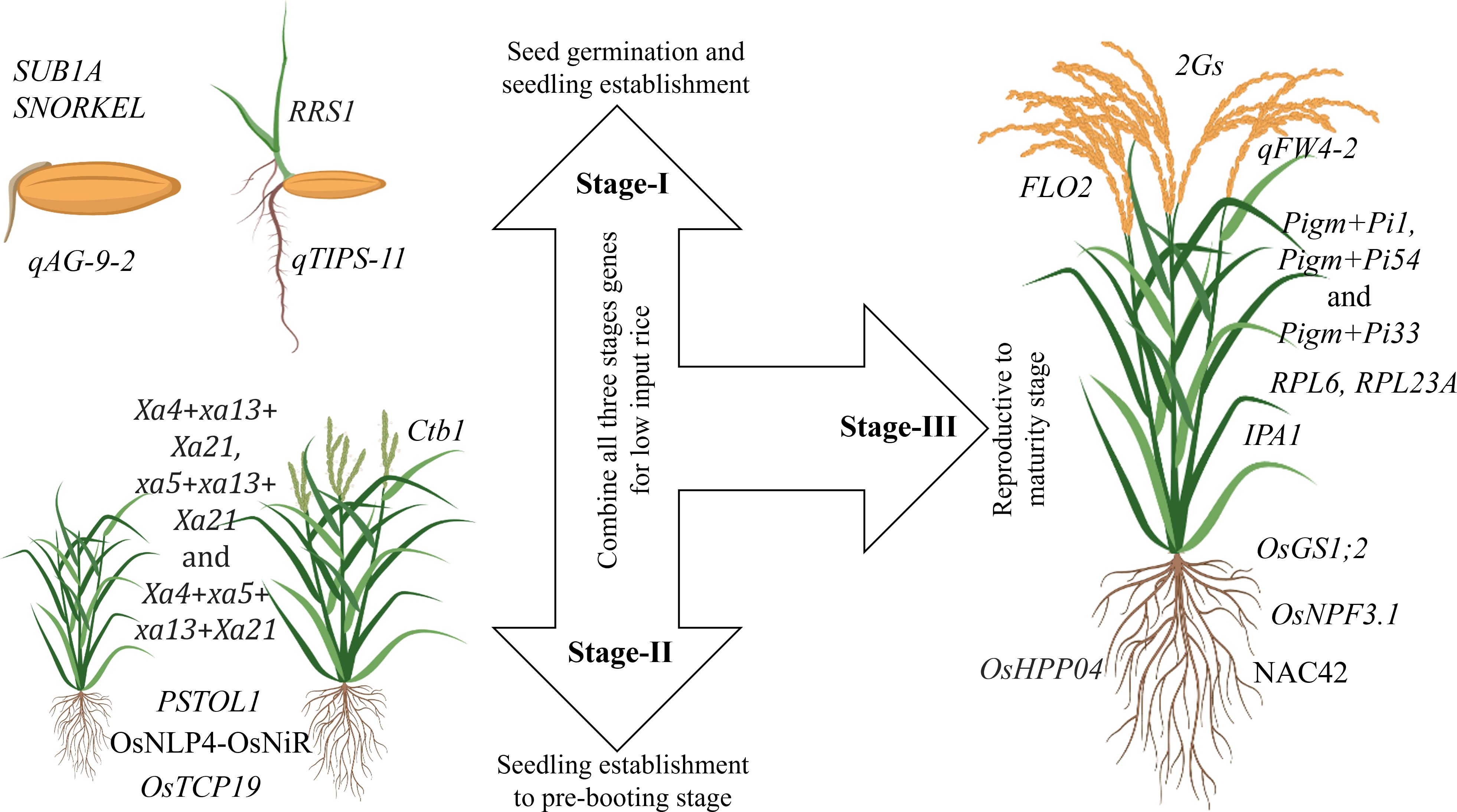
Figure 2 Important genes and QTLs necessary for developing ideal rice cultivar for LI systems. Stage-I: Germination and seedling vigor under various stress conditions; SUB1A gene confers tolerance to submergence, SNORKEL gene controls plant elongation to escape deepwater (Miro and Ismail, 2013), qAG-9–2 is associated with tolerance to flooding during germination (Angaji et al., 2010), qTIPS‐11 is associated with increased lateral root number(Wang et al., 2018a), and RRS1 is a negative regulator of root development. Knockout of RRS1 in plants enhances root growth, including longer root length, lateral root length, and higher lateral root density, drought resistance by promoting water absorption and improving water use efficiency (Gao et al., 2023). Stage-II: Vegetative to pre-booting stage; Effective gene combinations like Xa4+xa13+Xa21, xa5+xa13+Xa21 and Xa4+xa5+ xa13+Xa21 are widely utilized for bacterial blight resistance (Pradhan et al., 2020), OsNLP4-OsNiR is associated with increased tiller number and yield through enhancing nitrogen assimilation and nitrogen-use-efficiency (Yu et al., 2021), OsTCP19 allele is closely associated with high tillering response to soil nitrogen (Liu et al., 2021), PSTOL1 enhances grain yield in phosphorus-deficient soil and also acts as an enhancer of early root growth, thereby enabling the plants to acquire more phosphorus and other nutrients (Gamuyao et al., 2012), Ctb1 is associated with cold tolerance at the booting stage (Saito et al., 2010). Stage-III: Reproductive to maturity; IPA1 controls the ideal plant architecture in rice and is associated with drought tolerance (Chen et al., 2023a); 2Gs genes influence grain weight and grain number i.e., GW7 enhances grain weight) and DEP2 grain number. Both are co-localized on chromosome 7. Thus, there is a possibility of simultaneous introgression for both grain number and weight improvement (Singh et al., 2024), qFW4-2 is associated with flag leaf size and photosynthetic capacity. FLO2 plays a pivotal regulatory role in grain size and starch quality by affecting storage substance accumulation in the endosperm (She et al., 2010), OsHPP04 is associated with resistance to root-knot nematode without any adverse effects on plant growth (Huang et al., 2023), Among the cloned genes, Pigm+Pi1, Pigm+Pi54 and Pigm+Pi33 are the most effective gene combination patterns to achieve the stable broad-spectrum resistance to both leaf blast and panicle blast under various conditions, these resistance gene combination patterns have potential in gene pyramiding breeding (Ning et al., 2020), OsNAC42 activates a haplotype of nitrate transporter OsNPF6.1HapB that confers high nitrogen use efficiency by increasing yield under low nitrogen supply (Tang et al., 2019), OsGS1;2 and OsNPF3.1 increase NUE (Hang et al., 2024), RPL6 and RPL23A increase water use efficiency (Moin et al., 2016, 2017).
4.1 NUE and PUE
Rice fields are heavily fertilized almost all over the world. An estimation of fertilizer use in rice across top rice producing countries have been depicted in Figure 1. For low-input systems, nitrogen (N) and phosphorus (P) are comparatively more crucial than other nutrients (Wolfe et al., 2008). Remobilizing leaf-stored nitrogen during grain filling is essential in increasing nitrogen use efficiency (Mickelson et al., 2003; Fan et al., 2007). In cereals, N remobilization accounts for a significant portion of grain N content (Kichey et al., 2007; Fan et al., 2020). Similarly, remobilizing stored P contributes to a substantial portion of phosphorus loading in grain (Julia et al., 2016). Previous studies suggested that pectin helps remobilize P (Zhu et al., 2015). However, the presence of nitrate interferes with the pectin synthesis and hinders P remobilization (Zhu et al., 2018). For these reasons, breeding for both nitrogen and phosphorus use efficiency is a challenging task.
4.2 Root traits
Researchers also emphasized the importance of root morphology for optimal nutrient acquisition, as roots are a vital participant in nutrient uptake. However, the N and P uptake requires opposing root types for maximum efficacy. In general, less lateral development and a deeper root structure with fewer axial roots are recommended for higher nitrogen usage efficiency. On the contrary, more axial root and lateral growth are needed for improved P and K efficiency (Lynch, 2022).During the past few years, numerous studies have been conducted on features linked to nitrogen use efficiency. Plants uptake nitrogen as a nitrate ion (Lynch, 2019). In addition, the “steep, cheap, and deep-rooted” ideotype has improved corn’s ability to collect nutrients in ideotype breeding (Lynch, 2013). In rice, two classes of lateral roots named L-types (long and thick) and S-type (short and small) are found (Yamauchi et al., 1987; Ajmera et al., 2022). Henceforth, increasing the fraction of nodal roots with smaller diameters, shallower nodal root angles, and large densities of L-type roots may boost yield potential in low nitrogen conditions (Ajmera et al., 2022). Conversely, P efficiency may be attained by increasing axial root production, shallower axial root development, root hair length, and root hair biomass (Lynch, 2019). Therefore, combining diverse ideotypes with root phenotypes can be a helpful strategy for breeding nutrient usage efficiency (Lynch, 2019). Nonetheless, the phenotyping of roots is tedious and requires much effort and time. For this reason, high-throughput phenotyping systems can be very advantageous in various breeding programs. Therefore, root phenotyping for better nutrient use efficiency can be easily achieved. For root phenotyping, image processing systems like GT-RootS (Borianne et al., 2018), DeepLabv3 (Shen et al., 2020), and ChronoRoot (Gaggion et al., 2021) were claimed to be helpful in high throughput phenotyping.
4.3 WUE
Rice cultivation heavily depends on the availability of water. Minimizing the water requirement in rice fields is beneficial from both the environmental and economic viewpoint. In general, WUE is measured by studying the leaf structure and gas exchange dynamics in leaves. Most researchers used the Carbon isotope discrimination (Δ13C) method to study WUE in rice. Lower Δ13C is an indication of higher WUE, and researchers located multiple QTLs governing WUE across the rice genome through this method ( (Xu et al., 2009; This et al., 2010; Roja et al., 2016).
Multiple genes related to WUE in rice have been identified. For example, RPL6 and RPL23A are documented as target genes for increasing rice WUE (Moin et al., 2016, 2017). Another study highlighted the role of the OsαCA1 gene in increasing rice WUE (He et al., 2023). Transgenic rice expressing AtTOR genes also showed higher WUE (Bakshi et al., 2017). In addition, the BLANKET LEAF (BKL) gene of Oryza nivara is also suggested as a potential target for increasing water use efficiency (Hamaoka et al., 2017). Interestingly, a study reported that aquaporin expression profiles in rice roots are also a significant determinant of WUE (Nada and Abogadallah, 2014).
Since there is no rapid and cost-effective method of measuring WUE, breeding for WUE is arduous and time-consuming. Meanwhile, management practices in rice field can be manipulated to ensure maximum WUE. For example, previous studies reported that alternate wetting drying could increase WUE in rice without hampering yield (de Avila et al., 2015; Wang et al., 2016, 2020a). Therefore, a modified crop management system with proper fertilization can be an excellent way to increase water use efficiency in rice (Xue et al., 2013).
4.4 Early vigor
Early vigor is another essential feature of the LI system. Early vigorous plants will have the requisite strength to compete against weeds. In rice, many studies identified traits that regulate early vigor in various conditions (Namuco et al., 2009). Traits such as specific leaf area, leaf area index, early tillering ability, root length, root density, time to maturity, and growth duration affect the weed competitiveness of rice (Dingkuhn et al., 1999; Fofana and Rauber, 2000; de Vida et al., 2006; Rao et al., 2007) also demonstrated that early vigor and light interception traits are essential for weed competitiveness in rice.
4.5 Others
Besides weeds, plants must fight invading pathogens from multiple sources. Since the LI input system will use no or low amount of pesticides, plants should have inherent defense capacity against various diseases. Luckily, the magnitude of soil-borne disease is much lower in the LI system due to better soil quality (van Bruggen et al., 2016). A recent study reported that rice-pulse rotation can improve the microbiome diversity and decrease the pathogen population in aerobic rice field ( (Panneerselvam et al., 2023). Yet, the crop must achieve resistance against other pathogens prevalent in LI systems. The stay-green trait is also essential for abiotic stress tolerance. For delayed senescence, the stay-green trait can provide prolonged photosynthesis, but reducing remobilization can hamper nitrogen use efficiency (Plett et al., 2017). UAV-based techniques can contribute to plant breeding through quick and efficient phenotyping capacity (Xie and Yang, 2020). Recently, sensor-based phenotyping has been practiced in many crops. For example, multiple sensor-based UAV systems were described for measuring crop canopy-related traits and environmental data in soybean and wheat (Bai et al., 2016). In rice, UAV-based phenotyping is also being used for assessing nutrient content and disease resistance (Lu et al., 2021a; Bai et al., 2023; Shaodan et al., 2023).
Furthermore, phenotyping only in the LI system will not provide sufficient material for an ideal LI system. A sustainable commercial breeding program for LI systems should combine performance data from both high-input and LI systems (Muellner et al., 2014). To identify candidate alleles for LI agriculture, we should compare the performance of specific alleles from each input level and select the superior lines (Atlin and Frey, 1989). Combining phenotyping and genotyping data can produce unique inferences on features, and these conclusions may serve as the basis for applying contemporary breeding tactics to modify plants targeting LI systems.
4.6 Genomics-based breeding for LI system
The spate of inexpensive sequencing techniques emerged as a blessing for plant breeding. Likewise, genotyping has become a routine task in breeding programs, and combining genotype and phenotyping data can provide critical information about specific traits of interest. As a result, characterizing and discovering new markers, genes, and QTLs for the desired phenotype has become more convenient nowadays. In addition, creating a sustainable agricultural system will require both the survival capacity of wild cultivars and superior agronomic traits from elite cultivars. Consequently, combining genes from wild and elite cultivars can be a way to develop sustainable cultivars (Dawson et al., 2008). The introgression of genes has been practiced in the pre-omic era through marker-assisted backcross breeding. Marker-assisted breeding for single genes from cultivated cultivars or marker-assisted backcross breeding for single genes from landraces has been practiced in different crops (Prasanna et al., 2020). Over the past few years, QTLs affecting characteristics essential for LI systems have also been explored (Table 1). Multiple regions of rice chromosomes have important QTLs related to resource use efficiency and seedling establishment (Figure 3). For example, QTLs related to nitrogen use efficiency, phosphorus uptake, phosphorus translocation, and root number have been reported between 30.1 to 44 cM region on chromosome 1 in rice (Wei et al., 2011; Wang et al., 2014). Phosphorus and nitrogen use efficiency related QTLs were also mapped between 75.7–84.5 cM region on chromosome 2 and 55–73.8 cM region on chromosome 7. On chromosome 11, QTLs controlling root traits and phosphorus use efficiency are located between the 2 to 5.2 cM region. However, most of the QTLs are polygenic in nature, thus it is challenging to enhance genetic gain in breeding improvement. This issue was resolved with the advent of genome-wide association studies (GWAS) that can detect numerous QTLs for more accurate documentation of allelic controls of the traits.
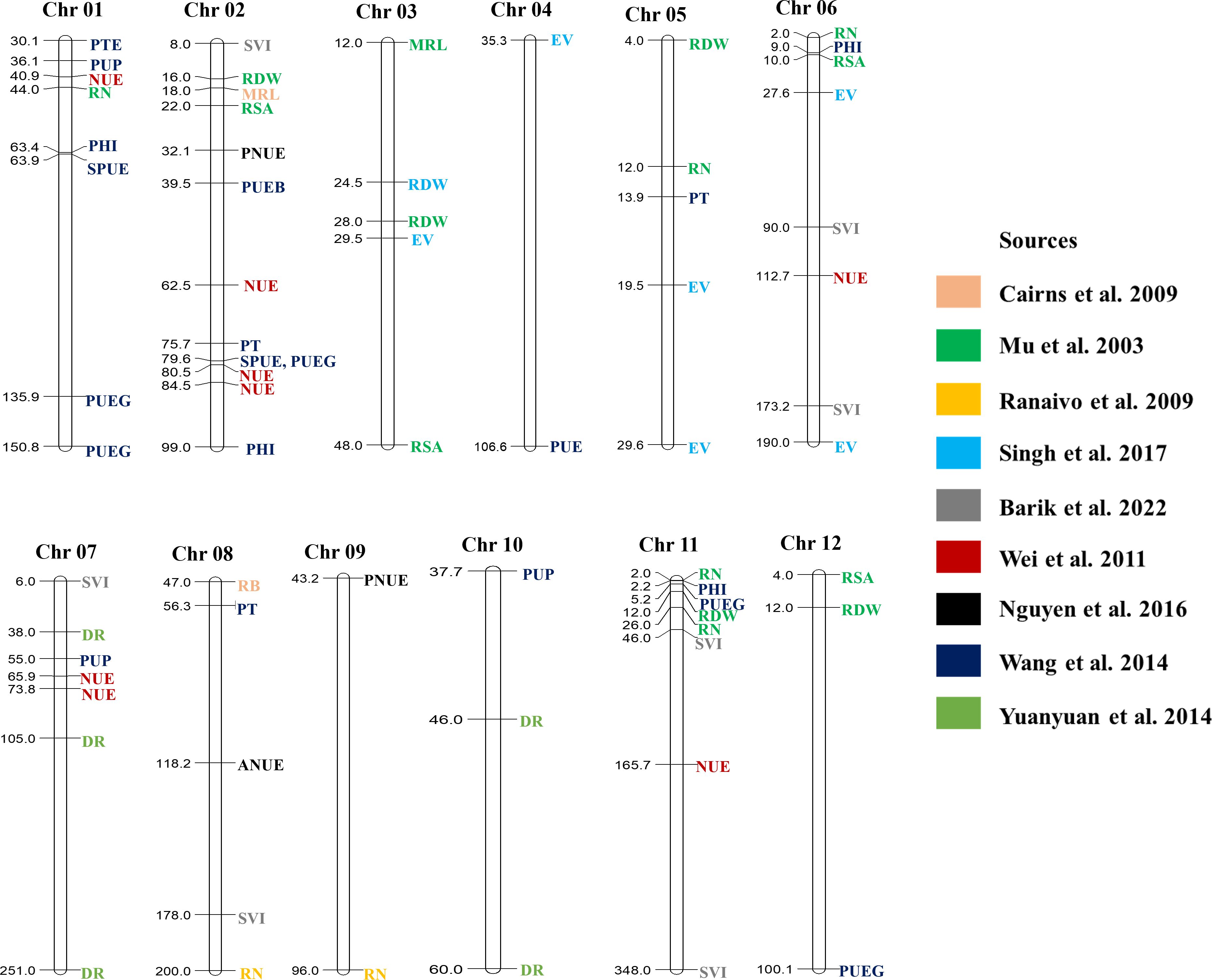
Figure 3 Reported QTLs in rice controlling traits related to LI systems. MRL, Maximum root length; RB, Root Biomass; DR, The number of deep roots; RSA, Root surface area; RN, The number of roots; RDW, Root dry weight; EV, Early vigor; NUE, Nitrogen use efficiency; PNUE, Physiological nitrogen use efficiency; ANUE, Agronomic nitrogen use efficiency; PTE, Phosphorus translocation efficiency; PUP, Phosphorus uptake at maturity; PHI, Phosphorus harvest index; SPUE, Straw phosphorus use efficiency; PUEG, Phosphorus use efficiency for grain yield; PUE, Phosphorus use efficiency; PUEB, Phosphorus use efficiency for biomass; PTE, Phosphorus translocation; SVI, Seedling vigor index; All genetic distance are in cM unit.
Further, breeders are empowered to genotype more correctly due to the improvement in sequencing quality and decline in cost (Varshney et al., 2021). Rice has a smaller genome among cereals, so genomic data may be quickly processed and interpreted using various computational tools. Numerous GWAS studies have recently discovered QTLs and candidate genes for traits required for the LI system (Table 2). Unfortunately, these studies have yet to employ phenotyping under the LI method. Hence, phenotyping data for existing LI systems should be included better to predict the marker-trait association (MTA) vital for LI systems. Apart from this, breeders can quickly uncover novel MTA using genotyping data, and the findings may be applied to upcoming research projects. Molecular markers and genomic selection (GS) can be employed in rice breeding to select variations linked with desirable traits discovered by GWAS (Chen et al., 2019). Since GWAS may indicate prospective regions or alleles for alteration, GWAS data can also be employed in crop improvement using CRISPR-Cas9-based gene editing (Tsakirpaloglou et al., 2023). However, GWAS approaches present considerable statistical challenges due to low power in detecting rare variants. Researchers use numerous techniques to address this problem, including enormous sample sizes or multiple biparental cross populations (Huang and Han, 2014).
Additionally, for a better understanding of gene function and a lower error rate, the post-GWAS study should incorporate multiple in-silico analyses leveraging bioinformatic tools. For instance, further research should be performed to exclude misleading genes other than causal genes inside an LD block containing significant SNPs (Tibbs Cortes et al., 2021). Additionally, SNP-based GWAS may yield spurious conclusions. For this reason, many researchers choose GWAS based on haplotypes. Haplotypes, for instance, can circumvent issues with SNP-based GWAS studies, such as linkage drag, biallelic nature, and the absence of uncommon alleles (Bhat et al., 2021). A haplotype is a group of alleles inherited with a low chance of recent recombination for several polymorphisms on the same chromosome (Stram, 2017). SNP markers are less effective and potent than haplotype markers, and recent research has revealed improved haplotype-based GWAS and GS in plants. Considering these advantages, it is possible to find valuable markers, genes, and QTLs for the rice LI system using haplotype- or SNP-based GWAS.
4.7 Genomic selection for LI systems
Genomic selection (GS) significantly shortens breeding cycles and improves selection efficiency in a less expensive way. While association mapping can identify many QTLs and candidate genes, it cannot identify genomic regions contributing to small effects. Therefore, GS has a greater potential to capture all the effects found in the genomic information than association mapping. Additionally, the power and linkage drag issues of the GWAS technique are resolved by the GS method. Similarly, GS is more effective than conventional marker-assisted selection (MAS) (Lorenz et al., 2011). The first consideration to starting genomic selection is the appropriate size for the training population and the testing population. Plant breeders, as opposed to animal breeders, can use a small training population to implement genomic selection in cereals (Lorenz et al., 2011). Due to this, GS in rice has vast potential for crop improvement for LI systems. In addition, the genetic relationship between the training and testing population also plays a vital role in prediction accuracy. For instance, more genetically related lines in the training population set might enhance prediction accuracy (Liu et al., 2018b). Also, the distance between the training population and the testing population can lower prediction ability across the breeding cycle selection (Robertsen et al., 2019). Therefore, the training and testing population should be maintained carefully, covering all these issues.
The LI system should also cover many criteria along with stability in a diverse environment. Crops under the LI system must endure variable environmental conditions through their genetic ability to survive. So, studying GxE interaction will be advantageous for developing LI system-adapted cultivars. Moreover, GS has the capacity to add GxE interaction into the prediction model (Crossa et al., 2017), which is particularly helpful in predicting the impact of the environment on genotype stability. Furthermore, the incorporation of GxE interaction with the prediction model increased prediction accuracy in many kinds of cereal. For instance, the genomic prediction model addressing GxE interaction increased selection accuracy in bread wheat, maize, and legumes (Burgueño et al., 2012; Cuevas et al., 2016; Crossa et al., 2017; Jarquín et al., 2017). Another crucial point for the LI system is the selection of multiple traits. Since the desired response of cultivar in a LI system relies on several traits, a multi-traits-based selection approach will be perfect for LI systems. Researchers reported increased efficiency in genomic prediction through a multi-trait random regression model for daily water usage and shoot biomass in rice (Baba et al., 2020). Multiple traits-based GS performs better amid the unavailability of phenotypic data for every individual or trait (Jia and Jannink, 2012). Ben Hassen et al. (2018) showed that the multi-environment model enhances the prediction ability of heading dates, panicle weight, and nitrogen balance index under different water management conditions in rice. Furthermore, traits that show low heritability can be more effectively bred through a multi-trait-based genomic selection model (Xu et al., 2021). Since superior performance depends on several traits, it is advantageous to predict the performance of certain traits based on other traits. In this context, multi-trait GS can forecast the performance of traits that are incredibly challenging to phenotype from other related traits (Xu et al., 2021). Through the advancement of high-throughput techniques, components can be related to predicting the performance of certain traits. For example (Sun et al., 2017), studied the prediction accuracy of wheat grain yield from secondary traits such as canopy temperature and normalized difference vegetative index. Again, the inclusion of the selection index in the prediction model can also increase the efficacy in the prediction for suitable genotypes. For example, Wang et al. (2019) included a selection index in the GS model to better predict grain yield through auxiliary traits in rice.
4.8 Gene editing for LI systems
The recent advances in gene editing techniques have made gene editing a fast, safe, and reliable option for crop improvement. Since manipulation can be done within the target genome, the breeders save some time and effort. Besides, GWAS can identify novel genomic regions that can be modified through genome editing. In this context, CRISPR-Cas9-based techniques can advance the trait improvement process by targeting certain traits in an advanced line. Several genes/alleles or miRNAs have been identified as important for LI agriculture’s trait improvement (Table 3). Genome editing techniques can be applied to improve some of the traits by modifying these alleles. For increasing nitrogen use efficiency (NUE), transporter(s) related to each nutrient and genes regulating the desired ideotype should be targeted. Fortunately, some recent studies successfully integrated CRISPR-based genome editing for NUE in different crops. For instance, Karunarathne et al. (2022) demonstrated the scope of increasing NUE in barley by targeting the ARE1 gene. Lu and Zhu (2017) showed the efficiency of base editing with the NRT1.1B-indica allele that contributes to the higher nitrate uptake in indica rice (Hu et al., 2015). Similarly, past studies documented that genetic manipulation could modify root development. For instance, OsACS mutants showed reduced lateral root growth under phosphorus deficiency, suggesting a scope of enhancing lateral root growth by overexpressing ethylene synthesis-related genes in rice (Lee et al., 2019). Kitomi et al. (2020) modified a homolog of DRO1 (Deep rooting-1) to modify the root angle for increasing rice yield under saline conditions. Additionally, multiplex genome editing created a new opportunity to target several plant genes simultaneously. Since many disease-resistant traits are controlled by more than one gene, this multi-gene-based editing will be beneficial in developing rice cultivars resistant to multiple diseases. Although many genes have been pyramided for disease resistance in plants, the multiplex genome editing approach will be a quicker and more pragmatic solution. For example, researchers documented rice blast and bacterial blight-resistant sterile lines through multiplex genome editing by adding specific mutations into TMS5, Pi21, and Xa13 genes (Li et al., 2019a). Moreover, knocking out the genes related to susceptibility, enhancing the expression of resistance genes, and changing the interaction between the effector and target can be potential areas of modification through genome editing (Bisht et al., 2019). One study showed that a single nucleotide polymorphism site in eIF4G is responsible for resistance against Rice Tungro Spherical Virus (Lee et al., 2010). Such sites can be easily edited by CRISPR-based genome editing techniques. Additionally, gene-edited products can remove the dissatisfaction associated with GM (genetically modified) crops since Cas9 can be removed from plants through natural segregation.
5 Prospects of organic rice production
The safe food movement made organic farming (OF) a practical alternative to crop production, as people became increasingly cautious about their food choices. As a corollary, growers across the world are becoming more interested in organic rice. Like the low-input systems, varieties for organic systems are also being tested in the conventional systems. However, a combination of evolutionary and participatory breeding will be helpful to develop superior varieties for both organic and low-input systems. Many farmers choose to prefer the standard high-input methods over the organic systems since the yield of OF is significantly lower than that of conventional systems. However, the low yield of organic rice is offset by a better benefit-cost ratio, which compensates for the reduced yield. For instance, research done in Thailand showed that despite the poor yield, OF had higher economic benefits due to the cheap production cost and higher market value (Arunrat et al., 2022). Furthermore, organic rice may be a financially feasible alternative for farmers in developing nations that have limited resources since organic amendments combined with management practices can establish a profitable organic farm (Mishra et al., 2018). In addition to the health advantages, organic rice farming has a positive impact on the environment. Soil organic carbon and soil carbon sequestration, for instance, will be less affected by organic farming in the context of climate change (Arunrat et al., 2021). Further, sustainable crop production methods should safeguard the complex biological interactions between many organisms within a given environment. Fortunately, rice fields’ biodiversity may be preserved and increased via the use of organic agriculture practices (Katayama et al., 2019). Nevertheless, due to the current interconnectedness of other stakeholders, only OF practices cannot maintain an entirely sustainable system. Accordingly, a combination of OF and other agricultural techniques is required for sustainability (Reganold and Wachter, 2016). For instance, an efficient organic fertilization system combined with crop rotation is the most effective method for variable climatic conditions (Arnés et al., 2013).
6 Hunt for microbiome-friendly release
Nature has endowed wild plants with a unique association capability with microbes, and this association benefits crops in many ways. However, the domestication of wild plants adversely affected the beneficial plant-microbe interaction of wild species (Pérez-Jaramillo et al., 2016). Modern plant breeding should emphasize utilizing maximum symbiosis from crop cultivars and select materials hospitable to soil microbial composition. In this context, using beneficial microbes from wild plants and seed endophytic bacteria can be a positive way of achieving better sustainability (L’Hoir and Duponnois, 2021).
Non-leguminous crops like rice can be improved through the exploitation of arbuscular mycorrhizal associations. Microbes help plants in many ways, including adverse effects on disease-forming pathogens, abiotic stress tolerance, and nutrient uptake efficiency (Bulgarelli et al., 2013). In particular, Arbuscular mycorrhizal fungi (AMF) helps plants to maintain proper growth under P deficiency (Harrison et al., 2002; Smith et al., 2003). Arbuscular mycorrhizal symbiosis can also be a potential way of improving rice yield and stress tolerance (Mbodj et al., 2018). Previous studies have reported the transfer of nutrients such as N, P, S, and Fe from mycorrhiza-like fungi to plants. A study showed that Piriformospora indica transfers Fe to rice (Verma et al., 2022). Very few efforts have been made to identify genetic factors controlling the root microbiome of rice under stress conditions. A recent study identified 10 SNPs and ten candidate genes related to the root microbiome of rice under drought stress. They identified more major fungal clusters. One of the fungal clusters, Pleosporales, is a crucial member of the rice seed microbiome (Eyre et al., 2019) and has previously been shown to improve plant growth and nitrogen content (Vergara et al., 2019). Another study identified 23 putative QTLs related to root colonization in rice (Davidson et al., 2019). In addition, substituting OsCERK1DY allele from wild rice to an indica variety ZH11 improved the phosphorus uptake by increasing AMF colonization (Huang et al., 2020). One study also found four QTLs related to associative N2 fixation in rice (Wu et al., 1995; Kaeppler et al., 2000).
Though soil is the primary determinant of microbial composition, plant species also play a crucial role in determining microbiome. Researchers have documented the effect of various genotypes in maintaining microbiome composition (Bergelson et al., 2019; Schmid et al., 2019; Jacoby et al., 2020). For example, Lundberg et al. (2012) documented that different Arabidopsis accessions have distinct microbiome compositions. In rice, researchers also found genotypic variation in microbiome composition. For example, root endophytes like Azoarcus spp. and Acremonium preferentially prefer wild species for colonization (Engelhard et al., 2000). Researchers found differences in nitrogen derived from air (ndfa) by comparing diverse rice lines (Shrestha and Ladha, 1996). Traditional varieties with high ndfa value showed high associative fixation and sustained 12 months on N-free medium (Rolfe et al., 1997).
For this reason, screening genotypes for microbiome friendliness should be considered. Two things need to be appropriately considered for comparing the genotypes’ feasibility in plant-microbiome interactions: root morphology and root exudates.
Root morphology can influence microbiome composition by affecting nutrient availability. For example, longer roots reduce microbial biomass by decreasing nitrogen availability (Pérez-Jaramillo et al., 2017; Wan et al., 2021). Root traits such as root length, root hair, and root branching patterns are essential in determining microbial diversity (Eisenhauer et al., 2017; Robertson-Albertyn et al., 2017; Wang et al., 2017). Previous studies reported that roots having less root diameter attract more microbes (Szoboszlay et al., 2015; Wang et al., 2020b). Bacteria also can modify root traits by exerting certain phytohormones (Grover et al., 2021). AMF preferred larger lateral roots in rice for colonization (Gutjahr et al., 2009).
Further, root exudates are one of the prime factors for studying the rhizosphere. Metabolomics is the perfect means of characterizing rhizosphere based on metabolites. Metabolites can facilitate communication between beneficial microbes for adapting to adverse conditions or protecting plants against pathogens (Jacoby et al., 2020). Root exudates create a favorable environment for microbes by providing a proper nutritional source (Sasse et al., 2018). In addition, utilizing ‘holo-omic’ and ‘exometabolomics’ in the breeding pipeline can decipher useful plant-microbe interactions (Sasse et al., 2018; Nerva et al., 2022).
LI systems can be an effective solution for fostering and maintaining healthy associations between microorganisms. In low-input systems, management techniques and landscape variation impact the soil microbiota and enzyme activity (Wickings et al., 2016). However, the judicious application of different management approaches will determine the extent of the association.
7 Integrated breeding approaches for LI
A shift to LI agriculture would reduce pollution, reward farmers financially, and protect the environment. The current practice of developing high-yielding varieties fails to consider low-input cultivars (Vanloqueren and Baret, 2008). So, an integrative breeding approach should be designed to cover every facet of sustainable agriculture (Figure 4). Transforming modern agriculture to a low-input sustainable system requires new varieties that can thrive under extreme conditions. Varieties for the LI system should be equipped with multiple traits, making it a cumbersome task. Fortunately, the advent of modern technologies can be incorporated in the breeding program to reduce the timeframe required for developing a variety of LI systems. The first step will be exploring germplasms for enhanced resource use efficiency and multiple stress resistance. Since most of our materials are adapted to high input conditions, we must screen many materials for starting any breeding program for LI systems. Recent phenomics developments will help breeders rapidly screen several germplasms to identify the perfect candidate that can be included in the crossing program. The flexibility of machine learning algorithms can infer multiple parameters just by analyzing field images. Important traits such as early vigor and nutrient use efficiency can be tested within a shorter time with adjustment in image processing pipeline.
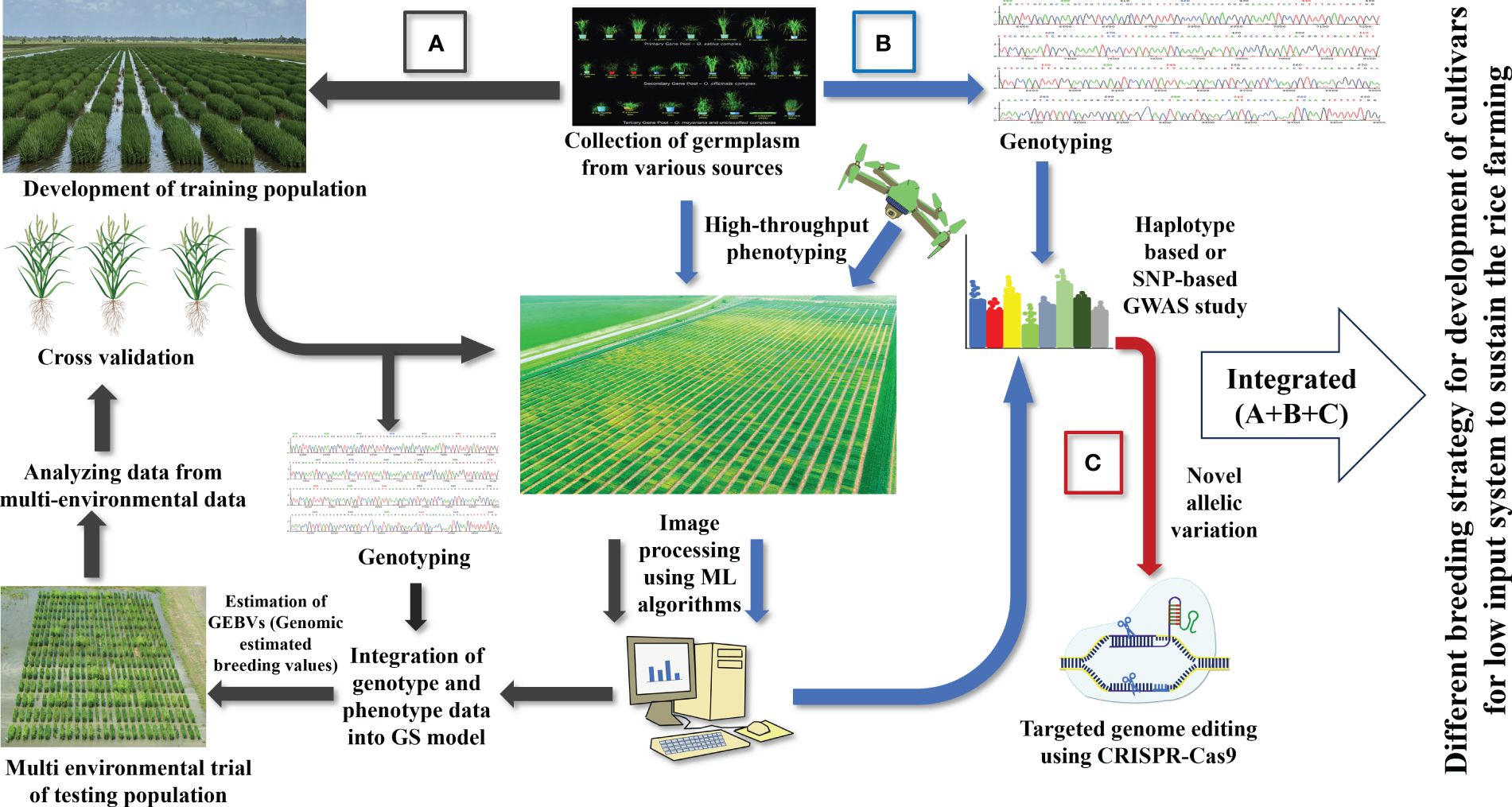
Figure 4 Various breeding strategies for developing rice cultivars for low input system: (A) Genomic selection approach to rapidly develop rice cultivars for LI systems, (B) genome wide association study for identifying QTLs, genes, and allelic variation important for LI systems, (C) CRISPR-based gene editing tool to create desired modifications.
Further, genetic factors behind traits like early vigor, nutrient use efficiency, and stress resistance have already been explored. For instance, genes controlling nitrogen assimilation and utilizations like OsNPF3.1, OsNPF7.1, OsNRT2.1 can be targeted for better nitrogen use efficiency. Rice diseases like blast, and bacterial leaf blight are vastly studied, and multiple genes are identified, for instance, OsCPK4 for blast resistance, Xa23 for bacterial leaf blight resistance, OsOSN1 for sheath blight resistance. Pyramiding multiple genes will be an excellent way to develop advanced lines for low-input agriculture. Another critical aspect of sustainable agriculture is root traits. The modern phenomics pipeline has lessened the difficulty in phenotyping root traits. Traits such as root length, diameter, and hair density would have been complex to study without phenomics platforms. Incorporation of root phenomics in the screening program will efficiently identify good lines for resource use efficiency. Various metabolomics studies can be done simultaneously to identify microbe-friendly genotypes since the microbiome is crucial for maintaining sustainability. Also, we must explore new genes, QTLs, and markers under low-input conditions by utilizing cheaper genotyping data. A genome-wide association study should be conducted for all the essential traits for identifying new markers and genomic regions associated with desired traits. Searching for these genes or alleles to introgress into existing lines will be beneficial for developing an ideal variety for the LI system. In addition, identified genes can be validated easily by incorporating genome editing tools. This validation step will increase the success rate before starting to pyramid multiple genes. Since genotyping has become cheaper than phenotyping, genomic selection will be a handy tool in any breeding program. While training the genomic selection model, we should incorporate all the relevant traits for the LI system. Finally, genomic selection will be helpful in rapidly testing advanced lines.
8 Conclusion and future perspectives
We must modify our conventional agricultural methods into resource-efficient systems to attain sustainability in agricultural practices. In this context, modern development in genomic research can facilitate rice breeding for low-input systems. A successful rice breeding program for a low-input system will require fast identification of traits that promote resource use efficiency, better protection against pathogens, and incorporating these traits into one cultivar. Utilizing all modern tools throughout the process will increase selection efficiency and reduce the number of breeding cycles required. Since rice is cultivated across a range of environments, breeding practices should consider precise estimation of genotype-environment interaction. Recent developments in environmental modeling have created new opportunities to predict the performance of certain genotypes by including multiple environmental covariates in the prediction model. Besides, developing countries may face adverse environmental conditions due to climate change. Testing the performance of LI rice in stressed environments will provide better insights into its suitability in developing countries.
Initially, we must identify early vigorous rice genotypes that can compete with weeds. Tolerance to multiple stress factors will also play a vital role. Moreover, phenotyping for root traits will be the most effective way to search for nutrient use efficiency. Genes related to nitrogen transportation and assimilation, like OsNLP4, OsNPF7.7, and gs3 allele, can be advantageous. In general, nutrient use efficiency traits are polygenic in nature. QTLs associated with nitrogen, phosphorus, and potassium use efficiency have already been mapped in rice. For early vigor OsIPMS1, OsHIPL1 genes can be utilized. Root traits such as root lengths, diameter, and surface area are essential for nutrient uptake. Fortunately, many high-throughput platforms have been developed to facilitate these phenotyping processes. Although people are using many of these platforms regularly, we should be careful to limit the misinterpretation of the data. In addition, breeders should cross-validate their results for better accuracy. Besides, researchers should continuously improve machine learning and deep learning algorithms for better accuracy in high-throughput phenotyping. Also, cross-validation should be a routine task associated with all these methods.
Further, cheaper NGS data can be used in genome-wide association mapping for identifying genes, QTLs, and markers for multiple traits. Proper selection of genotyping platforms, marker system, and accurate phenotyping will determine the efficiency of this method. Besides, the genomic selection model can help evaluate many lines for multiple traits in a shorter time. Also, the ability to use a multi-trait model or incorporation of phenomics data made GS a powerful tool in breeding for low-input systems. In predictive breeding, minimizing the error rate is a vital step. For this reason, data analysts should carefully manage the statistical packages to reduce the error rate. Breeders should also focus on newer statistical models that can generate more accurate predictions of their dataset.
Furthermore, genome editing can also help modify target genes for nutrient use efficiency or stress tolerance. However, we must be careful with gene editing tools and transgenic approaches since these techniques have acceptability issues. Gene editing can reduce the time for introducing the desired variation, but transforming plants for editing often becomes difficult. Fortunately, people are working on DNA and tissue culture-free methods for better efficiency in genome editing (Altpeter et al., 2016). Sometimes, getting a homozygous mutation becomes complex with the increased number of target genes in multiplex editing. More work on multiplex editing can render this a helpful approach for targeting multiple genes for low-input systems.
For developing a sustainable farming system, the soil microbiome must be taken care of. Microbes not only improve soil health but also improve nutrient availability to plants. For this reason, all lines should be checked thoroughly for microbiome compatibility. Metabolomic and phenomics studies can be used to study the genotypic effect on soil microbiome. Attaining sustainability relies on covering every facet of environmental safety. Hence, breeding rice for the LI system should be carefully crafted to ensure maximum protection of all natural resources.
Author contributions
SJ: Conceptualization, Data curation, Formal analysis, Methodology, Software, Visualization, Writing – original draft, Writing – review & editing. GS: Methodology, Writing – review & editing, Visualization. AP: Visualization, Writing – review & editing. LT: Writing – review & editing. ES: Writing – review & editing. ST: Conceptualization, Funding acquisition, Methodology, Supervision, Writing – review & editing, Resources.
Funding
The author(s) declare that no financial support was received for the research, authorship, and/or publication of this article.
Acknowledgments
We acknowledge Texas A&M AgriLife Research and Texas Rice Research Foundation (TRRF) for providing the funding support.
Conflict of interest
The authors declare that the research was conducted in the absence of any commercial or financial relationships that could be construed as a potential conflict of interest.
Publisher’s note
All claims expressed in this article are solely those of the authors and do not necessarily represent those of their affiliated organizations, or those of the publisher, the editors and the reviewers. Any product that may be evaluated in this article, or claim that may be made by its manufacturer, is not guaranteed or endorsed by the publisher.
References
Ahmad, A., Zoli, M., Latella, C., Bacenetti, J. (2023). Rice cultivation and processing: Highlights from a life cycle thinking perspective. Sci. Total Environ. 871, 1–12. doi: 10.1016/j.scitotenv.2023.162079
Ajmera, I., Henry, A., Radanielson, A. M., Klein, S. P., Ianevski, A., Bennett, M. J., et al. (2022). Integrated root phenotypes for improved rice performance under low nitrogen availability. Plant Cell Environ. 45, 805–822. doi: 10.1111/pce.14284
Alfatih, A., Wu, J., Zhang, Z. S., Xia, J. Q., Jan, S. U., Yu, L. H., et al. (2020). Rice NIN-LIKE PROTEIN 1 rapidly responds to nitrogen deficiency and improves yield and nitrogen use efficiency. J. Exp. Bot. 71, 6032–6042. doi: 10.1093/jxb/eraa292
Altpeter, F., Springer, N. M., Bartley, L. E., Blechl, A. E., Brutnell, T. P., Citovsky, V., et al. (2016). Advancing crop transformation in the era of genome editing. Plant Cell 28, 1510–1520. doi: 10.1105/tpc.16.00196
Angaji, S. A., Septiningsih, E. M., Mackill, D. J., Ismail, A. M. (2010). QTLs associated with tolerance of flooding during germination in rice (O ryza sativa L.). Euphytica 172, 159–168. doi: 10.1007/s10681-009-0014-5
Arnés, E., Antonio, J., Del Val, E., Astier, M. (2013). Sustainability and climate variability in low-input peasant maize systems in the central Mexican highlands. Agric. Ecosyst. Environ. 181, 195–205. doi: 10.1016/j.agee.2013.09.022
Arunrat, N., Sereenonchai, S., Chaowiwat, W., Wang, C., Hatano, R. (2022). Carbon, nitrogen and water footprints of organic rice and conventional rice production over 4 years of cultivation: A case study in the Lower North of Thailand. Agronomy 12, 380. doi: 10.3390/agronomy12020380
Arunrat, N., Sereenonchai, S., Wang, C. (2021). Carbon footprint and predicting the impact of climate change on carbon sequestration ecosystem services of organic rice farming and conventional rice farming: A case study in Phichit province, Thailand. J. Environ. Manag. 289, 112458. doi: 10.1016/j.jenvman.2021.112458
Atlin, G. N., Frey, K. J. (1989). Breeding crop varieties for low-input agriculture. Am. J. Altern. Agric. 4, 53–58. doi: 10.1017/S0889189300002721
Baba, T., Momen, M., Campbell, M. T., Walia, H., Morota, G. (2020). Multi-trait random regression models increase genomic prediction accuracy for a temporal physiological trait derived from high-throughput phenotyping. PloS One 15, 1–17. doi: 10.1371/journal.pone.0228118
Bai, G., Ge, Y., Hussain, W., Baenziger, P. S., Graef, G. (2016). A multi-sensor system for high throughput field phenotyping in soybean and wheat breeding. Comput. Electron Agric. 128, 181–192. doi: 10.1016/j.compag.2016.08.021
Bai, X., Fang, H., He, Y., Zhang, J., Tao, M., Wu, Q., et al. (2023). Dynamic UAV phenotyping for rice disease resistance analysis based on multisource data. Plant Phenomics 5, 0019. doi: 10.34133/plantphenomics.0019
Bakshi, A., Moin, M., Kumar, M. U., Reddy, A. B. M., Ren, M., Datla, R., et al. (2017). Ectopic expression of Arabidopsis Target of Rapamycin (AtTOR) improves water-use efficiency and yield potential in rice. Sci. Rep. 7, 1–16. doi: 10.1038/srep42835
Banerjee, J., Maiti, M. K. (2010). Functional role of rice germin-like protein1 in regulation of plant height and disease resistance. Biochem. Biophys. Res. Commun. 394, 178–183. doi: 10.1016/j.bbrc.2010.02.142
Barik, S. R., Pandit, E., Sanghamitra, P., Mohanty, S. P., Behera, A., Mishra, J., et al. (2022). Unraveling the genomic regions controlling the seed vigour index, root growth parameters and germination per cent in rice. PloS One 17, 1–24. doi: 10.1371/journal.pone.0267303
Barnaby, J. Y., McClung, A. M., Edwards, J. D., Pinson, S. R. M. (2022). Identification of quantitative trait loci for tillering, root, and shoot biomass at the maximum tillering stage in rice. Sci. Rep. 12, 1–13. doi: 10.1038/s41598–022-17109-y
Ben Hassen, M., Monaco, F., Facchi, A., Romani, M., Valè, G., Sali, G. (2018). Economic performance of traditional and modern rice varieties under different water management systems. Sustainability 9 (3), 347. doi: 10.3390/su9030347
Bergelson, J., Mittelstrass, J., Horton, M. W. (2019). Characterizing both bacteria and fungi improves understanding of the Arabidopsis root microbiome. Sci. Rep. 9, 24. doi: 10.1038/s41598-018-37208-z
Bhat, J. A., Yu, D., Bohra, A., Ganie, S. A., Varshney, R. K. (2021). Features and applications of haplotypes in crop breeding. Commun. Biol. 4, 1–12. doi: 10.1038/s42003-021-02782-y
Bi, Y. M., Kant, S., Clark, J., Gidda, S., Ming, F., Xu, J., et al. (2009). Increased nitrogen-use efficiency in transgenic rice plants over-expressing a nitrogen-responsive early nodulin gene identified from rice expression profiling. Plant Cell Environ. 32, 1749–1760. doi: 10.1111/j.1365-3040.2009.02032.x
Bisht, D. S., Bhatia, V., Bhattacharya, R. (2019). Improving plant-resistance to insect-pests and pathogens: The new opportunities through targeted genome editing. Semin. Cell Dev. Biol. 96, 65–76. doi: 10.1016/j.semcdb.2019.04.008
Borianne, P., Subsol, G., Fallavier, F., Dardou, A., Audebert, A. (2018). GT-RootS: An integrated software for automated root system measurement from high-throughput phenotyping platform images. Comput. Electron Agric. 150, 328–342. doi: 10.1016/j.compag.2018.05.003
Bulgarelli, D., Schlaeppi, K., Spaepen, S., Van Themaat, E. V. L., Schulze-Lefert, P. (2013). Structure and functions of the bacterial microbiota of plants. Annu. Rev. Plant Biol. 64, 807–838. doi: 10.1146/annurev-arplant-050312-120106
Bundó, M., Coca, M. (2016). Enhancing blast disease resistance by overexpression of the calcium-dependent protein kinase OsCPK4 in rice. Plant Biotechnol. J. 14, 1357–1367. doi: 10.1111/pbi.12500
Burgueño, J., de los Campos, G., Weigel, K., Crossa, J. (2012). Genomic prediction of breeding values when modeling genotype× environment interaction using pedigree and dense molecular markers. Crop Sci. 52, 707–719. doi: 10.2135/cropsci2011.06.0299
Cairns, J. E., Namuco, O. S., Torres, R., Simborio, F. A., Courtois, B., Aquino, G. A., et al. (2009). Investigating early vigour in upland rice (Oryza sativa L.): Part II. Identification of QTLs controlling early vigour under greenhouse and field conditions. Field Crops Res. 113, 207–217. doi: 10.1016/j.fcr.2009.05.007
Chandran, V., Wang, H., Gao, F., Cao, X. L., Chen, Y. P., Li, G. B., et al. (2019). miR396-osgrfs module balances growth and rice blast disease-resistance. Front. Plant Sci. 9. doi: 10.3389/fpls.2018.01999
Chen, X., Cui, Z., Fan, M., Vitousek, P., Zhao, M., Ma, W., et al. (2014). Producingmore grain with lower environmental costs. Nature 514, 486–489. doi: 10.1038/nature13609
Chen, G., Feng, H., Hu, Q., Qu, H., Chen, A., Yu, L., et al. (2015). Improving rice tolerance to potassium deficiency by enhancing OsHAK16p: WOX11-controlled root development. Plant Biotechnol. J. 13, 833–848. doi: 10.1111/pbi.12320
Chen, Z., Feng, Z., Kang, H., Zhao, J., Chen, T., Li, Q., et al. (2019). Identification of new resistance loci against sheath blight disease in rice through genome-wide association study. Rice Sci. 26, 21–31. doi: 10.1016/j.rsci.2018.12.002
Chen, T., Yang, S., Wei, T., Li, Y., Wang, S., Su, Y. (2023b). Overexpression of OsGS1;2 for improved nitrogen use efficiency and grain yield of rice: A field test. Field Crops Res. 303, 1–10. doi: 10.1016/j.fcr.2023.109146
Chen, F., Zhang, H., Li, H., Lian, L., Wei, Y., Lin, Y., et al. (2023a). IPA1 improves drought tolerance by activating SNAC1 in rice. BMC Plant Biol. 23, 1–12. doi: 10.1186/s12870-023-04062-9
Chen, J., Zhang, Y., Tan, Y., Zhang, M., Zhu, L., Xu, G., et al. (2016). Agronomic nitrogen-use efficiency of rice can be increased by driving OsNRT2.1 expression with the OsNAR2.1 promoter. Plant Biotechnol. J. 14, 1705–1715. doi: 10.1111/pbi.12531
Chujo, T., Miyamoto, K., Ogawa, S., Masuda, Y., Shimizu, T., Kishi-Kaboshi, M., et al. (2014). Overexpression of phosphomimic mutated OsWRKY53 leads to enhanced blast resistance in rice. PloS One 9, 1–14. doi: 10.1371/journal.pone.0098737
Clark, M. S., Horwath, W. R., Shennan, C., Scow, K. M. (1998). Changes in soil chemical properties resulting from organic and low-input farming practices. Agron. J. 90, 662–671. doi: 10.2134/agronj1998.00021962009000050016x
Clark, S., Klonsky, K., Livingston, P., Temple, S. (1999). Crop-yield and economic comparisons of organic, low-input, and conventional farming systems in California’s Sacramento Valley. Am. J. Altern. Agric. 14, 109–121. doi: 10.1017/S0889189300008225
Crossa, J., Pérez-Rodríguez, P., Cuevas, J., Montesinos-López, O., Jarquín, D., de los Campos, G., et al. (2017). Genomic selection in plant breeding: methods, models, and perspectives. Trends Plant Sci. 22, 961–975. doi: 10.1016/j.tplants.2017.08.011
Cuevas, J., Crossa, J., Soberanis, V., Pérez-Elizalde, S., Pérez-Rodríguez, P., Campos, G. D. L., et al. (2016). Genomic prediction of genotype × environment interaction kernel regression models. Plant Genome 9 (3), 1–20. doi: 10.3835/plantgenome2016.03.0024
Davidson, H., Shrestha, R., Cornulier, T., Douglas, A., Travis, T., Johnson, D., et al. (2019). Spatial effects and GWA mapping of root colonization assessed in the interaction between the rice diversity panel 1 and an arbuscular mycorrhizal fungus. Front. Plant Sci. 10. doi: 10.3389/fpls.2019.00633
Dawson, J. C., Huggins, D. R., Jones, S. S. (2008). Characterizing nitrogen use efficiency in natural and agricultural ecosystems to improve the performance of cereal crops in low-input and organic agricultural systems. Field Crops Res. 107, 89–101. doi: 10.1016/j.fcr.2008.01.001
de Avila, L. A., Martini, L. F. D., Mezzomo, R. F., Refatti, J. P., Campos, R., Cezimbra, D. M., et al. (2015). Rice water use efficiency and yield under continuous and intermittent irrigation. Agron. J. 107, 442–448. doi: 10.2134/agronj14.0080
de Vida, F. B. P., Laca, E. A., Mackill, D. J., Fernández, G. M., Fischer, A. J. (2006). Relating rice traits to weed competitiveness and yield: a path analysis. Weed Sci. 54, 1122–1131. doi: 10.1614/WS-06-042R.1
Dimkpa, S. O. N., Lahari, Z., Shrestha, R., Douglas, A., Gheysen, G., Price, A. H. (2016). A genome-wide association study of a global rice panel reveals resistance in Oryza sativa to root-knot nematodes. J. Exp. Bot. 67, 1191–1200. doi: 10.1093/jxb/erv470
Dingkuhn, M., Johnson, D. E., Sow, A., Audebert, A. Y. (1999). Relationships between upland rice canopy characteristics and weed competitiveness. Field Crops Res. 61, 79–95. doi: 10.1016/S0378-4290(98)00152-X
Dinh, L. T., Ueda, Y., Gonzalez, D., Tanaka, J. P., Takanashi, H., Wissuwa, M. (2023). Novel QTL for lateral root density and length improve phosphorus uptake in rice (Oryza sativa L.). Rice 16, 1–15. doi: 10.1186/s12284-023-00654-z
Dong, Z., Li, W., Liu, J., Li, L., Pan, S., Liu, S., et al. (2019). The rice phosphate transporter protein osPT8 regulates disease resistance and plant growth. Sci. Rep. 9, 1–10. doi: 10.1038/s41598–019-41718–9
Eisenhauer, N., Lanoue, A., Strecker, T., Scheu, S., Steinauer, K., Thakur, M. P., et al. (2017). Root biomass and exudates link plant diversity with soil bacterial and fungal biomass. Sci. Rep. 7, 44641. doi: 10.1038/srep44641
Engelhard, M., Hurek, T., Reinhold-Hurek, B. (2000). Preferential occurrence of diazotrophic endophytes, Azoarcus spp., in wild rice species and land races of Oryza sativa in comparison with modern races. Environ. Microbiol. 2, 131–141. doi: 10.1046/j.1462-2920.2000.00078.x
EPA. (2023). Inventory of U.S. greenhouse gas emissions and sinks: 1990-2021. (U.S. Environmental Protection Agency, EPA 430-R-23-002). Available at: https://www.epa.gov/ghgemissions/inventory-us-greenhouse-gas-emissions-and-sinks-1990-202.
Evans, L. T. (1996). Crop evolution, adaptation and yield (Cambridge, England: Cambridge University Press).
Eyre, A. W., Wang, M., Oh, Y., Dean, R. A. (2019). Identification and characterization of the core rice seed microbiome. Phytobiomes J. 3, 148–157. doi: 10.1094/PBIOMES-01-19-0009-R
Fan, X., Jia, L., Li, Y., Smith, S. J., Miller, A. J., Shen, Q. (2007). Comparing nitrate storage and remobilization in two rice cultivars that differ in their nitrogen use efficiency. J. Exp. Bot. 58, 1729–1740. doi: 10.1093/jxb/erm033
Fan, X., Xie, D., Chen, J., Lu, H., Xu, Y., Ma, C., et al. (2014). Over-expression of OsPTR6 in rice increased plant growth at different nitrogen supplies but decreased nitrogen use efficiency at high ammonium supply. Plant Sci. 227, 1–11. doi: 10.1016/j.plantsci.2014.05.013
Fan, T., Yang, W., Zeng, X., Xu, X., Xu, Y., Fan, X., et al. (2020). A rice autophagy gene osATG8b is involved in nitrogen remobilization and control of grain quality. Front. Plant Sci. 11. doi: 10.3389/fpls.2020.00588
Fang, Z., Xia, K., Yang, X., Grotemeyer, M. S., Meier, S., Rentsch, D., et al. (2013). Altered expression of the PTR/NRT1 homologue OsPTR9 affects nitrogen utilization efficiency, growth and grain yield in rice. Plant Biotechnol. J. 11, 446–458. doi: 10.1111/pbi.12031
FAO (2020). Emissions due to agriculture. global, regional and country trends 2000–2018 (Rome: FAOSTAT Analytical Brief Series No 18).
Feng, Z., Kang, H., Li, M., Zou, L., Wang, X., Zhao, J., et al. (2019). Identification of new rice cultivars and resistance loci against rice black-streaked dwarf virus disease through genome-wide association study. Rice 12, 1–13. doi: 10.1186/s12284-019-0310-1
Filipe, O., De Vleesschauwer, D., Haeck, A., Demeestere, K., Höfte, M. (2018). The energy sensor OsSnRK1a confers broad-spectrum disease resistance in rice. Sci. Rep. 8, 1–12. doi: 10.1038/s41598–018-22101–6
Fofana, Rauber (2000). Weed suppression ability of upland rice under low-input conditions in West Africa. Weed Res. 40, 271–280. doi: 10.1046/j.1365-3180.2000.00185.x
Gaggion, N., Ariel, F., Daric, V., Lambert, É., Legendre, S., Roulé, T., et al. (2021). ChronoRoot: High-throughput phenotyping by deep segmentation networks reveals novel temporal parameters of plant root system architecture. Gigascience 10, 1–15. doi: 10.1093/gigascience/giab052
Gamuyao, R., Chin, J. H., Pariasca-Tanaka, J., Pesaresi, P., Catausan, S., Dalid, C., et al. (2012). The protein kinase Pstol1 from traditional rice confers tolerance of phosphorus deficiency. Nature 488, 535–539. doi: 10.1038/nature11346
Gao, Z., Wang, Y., Chen, G., Zhang, A., Yang, S., Shang, L., et al. (2019). The indica nitrate reductase gene OsNR2 allele enhances rice yield potential and nitrogen use efficiency. Nat. Commun. 10, 1–10. doi: 10.1038/s41467-019-13110-8
Gao, Y., Xu, Z., Zhang, L., Li, S., Wang, S., Yang, H., et al. (2020). MYB61 is regulated by GRF4 and promotes nitrogen utilization and biomass production in rice. Nat. Commun. 11, 1–10. doi: 10.1038/s41467-020-19019-x
Gao, J., Zhao, Y., Zhao, Z., Liu, W., Jiang, C., Li, J., et al. (2023). RRS1 shapes robust root system to enhance drought resistance in rice. New Phytol. 238, 1146–1162. doi: 10.1111/nph.18775
Good, A. G., Beatty, P. H. (2011). Fertilizing nature: A tragedy of excess in the commons. PloS Biol. 9, 1–9. doi: 10.1371/journal.pbio.1001124
Goto, S., Sasakura-Shimoda, F., Suetsugu, M., Selvaraj, M. G., Hayashi, N., Yamazaki, M., et al. (2015). Development of disease-resistant rice by optimized expression of WRKY45. Plant Biotechnol. J. 13, 753–765. doi: 10.1111/pbi.12303
Grover, M., Bodhankar, S., Sharma, A., Sharma, P., Singh, J., Nain, L. (2021). PGPR mediated alterations in root traits: way toward sustainable crop production. Front. Sustain Food Syst. 4. doi: 10.3389/fsufs.2020.618230
Gudi, S., Kumar, P., Singh, S., Tanin, M. J., Sharma, A. (2022). Strategies for accelerating genetic gains in crop plants: Special focus on speed breeding. Physiol. Mol. Biol. Plants 28, 1921–1938. doi: 10.1007/s12298-022-01247-8
Gutjahr, C., Casieri, L., Paszkowski, U. (2009). Glomus intraradices induces changes in root system architecture of rice independently of common symbiosis signaling. New Phytol. 182, 829–837. doi: 10.1111/j.1469-8137.2009.02839.x
Hamaoka, N., Yasui, H., Yamagata, Y., Inoue, Y., Furuya, N., Araki, T., et al. (2017). A hairy-leaf gene, BLANKET LEAF, of wild Oryza nivara increases photosynthetic water use efficiency in rice. Rice 10, 1–11. doi: 10.1186/s12284-017-0158-1
Hang, J., Wu, B., Qiu, D., Yang, G., Fang, Z., Zhang, M. (2024). OsNPF3.1, a nitrate, abscisic acid and gibberellin transporter gene, is essential for rice tillering and nitrogen utilization efficiency. J. Integr. Agric. 23, 1087–1104. doi: 10.1016/j.jia.2023.04.024
Harrison, M. J., Dewbre, G. R., Liu, J. (2002). A phosphate transporter from Medicago truncatula involved in the acquisition of phosphate released by arbuscular mycorrhizal fungi. Plant Cell 14, 2413–2429. doi: 10.1105/tpc.004861
Hartley, T. N., Thomas, A. S., Maathuis, F. J. M. (2020). A role for the OsHKT 2;1 sodium transporter in potassium use efficiency in rice. J. Exp. Bot. 71, 699–706. doi: 10.1093/jxb/erz113
He, Y., Chen, S., Liu, K., Chen, Y., Cheng, Y., Zeng, P., et al. (2022). OsHIPL1, a hedgehog-interacting protein-like 1 protein, increases seed vigour in rice. Plant Biotechnol. J. 20, 1346–1362. doi: 10.1111/pbi.13812
He, Y., Cheng, J., He, Y., Yang, B., Cheng, Y., Yang, C., et al. (2019). Influence of isopropylmalate synthase OsIPMS1 on seed vigour associated with amino acid and energy metabolism in rice. Plant Biotechnol. J. 17, 322–337. doi: 10.1111/pbi.12979
He, Y., Duan, W., Xue, B., Cong, X., Sun, P., Hou, X., et al. (2023). OsαCA1 affects photosynthesis, yield potential, and water use efficiency in rice. Int. J. Mol. Sci. 24, 1–15. doi: 10.3390/ijms24065560
Hill, S. B., MacRae, R. J. (1996). Conceptual framework for the transition from conventional to sustainable agriculture. J. Sustain. Agric. 7, 81–87. doi: 10.1300/J064v07n01_07
Hong, Y., Liu, Q., Cao, Y., Zhang, Y., Chen, D., Lou, X., et al. (2019). The OsMPK15 negatively regulates Magnaporthe oryza and Xoo disease resistance via SA and JA signaling pathway in rice. Front. Plant Sci. 10. doi: 10.3389/fpls.2019.00752
Horrigan, L., Lawrence, R. S., Walker, P. (2002). How sustainable agriculture can address the environmental and human health harms of industrial agriculture. Available online at: http://ehpnet1.niehs.nih.gov/docs/2002/110p445–456horrigan/abstract.html. doi: 10.1289/ehp.02110445
Hu, B., Wang, W., Ou, S., Tang, J., Li, H., Che, R., et al. (2015). Variation in NRT1. 1B contributes to nitrate-use divergence between rice subspecies. Nat. Genet. 47, 834–838. doi: 10.1038/ng.3337
Hu, B., Zhou, Y., Zhou, Z., Sun, B., Zhou, F., Yin, C., et al. (2021). Repressed OsMESL expression triggers reactive oxygen species-mediated broad-spectrum disease resistance in rice. Plant Biotechnol. J. 19, 1511–1522. doi: 10.1111/pbi.13566
Huang, W., Bai, G., Wang, J., Zhu, W., Zeng, Q., Lu, K., et al. (2018). Two splicing variants of OsNPF7.7 regulate shoot branching and nitrogen utilization efficiency in rice. Front. Plant Sci. 9. doi: 10.3389/fpls.2018.00300
Huang, X., Han, B. (2014). Natural variations and genome-wide association studies in crop plants. Annu. Rev. Plant Biol. 65, 531–551. doi: 10.1146/annurev-arplant-050213-035715
Huang, R., Li, Z., Mao, C., Zhang, H., Sun, Z., Li, H., et al. (2020). Natural variation at Os CERK 1 regulates arbuscular mycorrhizal symbiosis in rice. New Phytol. 225, 1762–1776. doi: 10.1111/nph.16158
Huang, L.y., Li, X.x., Zhang, Y., Fahad, S., Wang, F. (2022). dep1 improves rice grain yield and nitrogen use efficiency simultaneously by enhancing nitrogen and dry matter translocation. J. Integr. Agric. 21, 3185–3198. doi: 10.1016/j.jia.2022.07.057
Huang, Q., Lin, B., Cao, Y., Zhang, Y., Song, H., Huang, C., et al. (2023). CRISPR/Cas9-mediated mutagenesis of the susceptibility gene OsHPP04 in rice confers enhanced resistance to rice root-knot nematode. Front. Plant Sci. 14, 1134653. doi: 10.3389/fpls.2023.1134653
Huang, L. F., Lin, K. H., He, S. L., Chen, J. L., Jiang, J. Z., Chen, B. H., et al. (2016). Multiple patterns of regulation and overexpression of a ribonuclease-like pathogenesis-related protein gene, OsPR10a, conferring disease resistance in rice and Arabidopsis. PloS One 11, 1–27. doi: 10.1371/journal.pone.0156414
IPCC (2007). “Climate change 2007: Impacts, adaptation and vulnerability,” in Contribution of working group II to the fourth assessment report of the intergovernmental panel on climate change. Eds. Parry, M. L., Canziani, O. F., Palutikof, J. P., van der Linden, P. J., Hanson, C. E. (Cambridge, UK: Cambridge University Press), 976.
Jacoby, R. P., Chen, L., Schwier, M., Koprivova, A., Kopriva, S. (2020). Recent advances in the role of plant metabolites in shaping the root microbiome. F1000Res 9, 1–9. doi: 10.12688/f1000research
Jarquín, D., Lemes da Silva, C., Gaynor, R. C., Poland, J., Fritz, A., Howard, R., et al. (2017). Increasing genomic-enabled prediction accuracy by modeling genotype× environment interactions in Kansas wheat. Plant Genome 10, plantgenome2016–12. doi: 10.3835/plantgenome2016.12.0130
Jia, Y., Jannink, J. L. (2012). Multiple-trait genomic selection methods increase genetic value prediction accuracy. Genetics 192, 1513–1522. doi: 10.1534/genetics.112.144246
Julia, C., Wissuwa, M., Kretzschmar, T., Jeong, K., Rose, T. (2016). Phosphorus uptake, partitioning and redistribution during grain filling in rice. Ann. Bot. 118, 1151–1162. doi: 10.1093/aob/mcw164
Kaeppler, S. M., Parke, J. L., Mueller, S. M., Senior, L., Stuber, C., Tracy, W. F. (2000). Variation among maize inbred lines and detection of quantitative trait loci for growth at low phosphorus and responsiveness to arbuscular mycorrhizal fungi. Crop Sci. 40, 358–364. doi: 10.2135/cropsci2000.402358x
Karunarathne, S. D., Han, Y., Zhang, X. Q., Li, C. (2022). CRISPR/Cas9 gene editing and natural variation analysis demonstrate the potential for HvARE1 in improvement of nitrogen use efficiency in barley. J. Integr. Plant Biol. 64, 756–770. doi: 10.1111/jipb.13214
Katayama, N., Osada, Y., Mashiko, M., Baba, Y. G., Tanaka, K., Kusumoto, Y., et al. (2019). Organic farming and associated management practices benefit multiple wildlife taxa: a large-scale field study in rice paddy landscapes. J. Appl. Ecol. 56, 1970–1981. doi: 10.1111/1365-2664.13446
Khan, M. U., Li, P., Amjad, H., Khan, A. Q., Arafat, Y., Waqas, M., et al. (2019). Exploring the potential of overexpressed osCIPK2 rice as a nitrogen utilization efficient crop and analysis of its associated rhizo-compartmental microbial communities. Int. J. Mol. Sci. 20, 1–21. doi: 10.3390/ijms20153636
Khong, G. N., Pati, P. K., Richaud, F., Parizot, B., Bidzinski, P., Mai, C. D., et al. (2015). OsMADS26 negatively regulates resistance to pathogens and drought tolerance in rice. Plant Physiol. 169, 2935–2949. doi: 10.1104/pp.15.01192
Kichey, T., Hirel, B., Heumez, E., Dubois, F., Le Gouis, J. (2007). In winter wheat (Triticum aestivum L.), post-anthesis nitrogen uptake and remobilisation to the grain correlates with agronomic traits and nitrogen physiological markers. Field Crops Res. 102, 22–32. doi: 10.1016/j.fcr.2007.01.002
Kim, J. A., Cho, K., Singh, R., Jung, Y. H., Jeong, S. H., Kim, S. H., et al. (2009). Rice OsACDR1 (Oryza sativa accelerated cell death and resistance 1) is a potential positive regulator of fungal disease resistance. Mol. Cells 28, 431–439. doi: 10.1007/s10059-009-0161-5
Kitomi, Y., Hanzawa, E., Kuya, N., Inoue, H., Hara, N., Kawai, S., et al. (2020). Root angle modifications by the DRO1 homolog improve rice yields in saline paddy fields. Proc. Natl. Acad. Sci. 117, 21242–21250. doi: 10.1073/pnas.2005911117
Lee, H. Y., Chen, Z., Zhang, C., Yoon, G. M. (2019). Editing of the OsACS locus alters phosphate deficiency-induced adaptive responses in rice seedlings. J. Exp. Bot. 70, 1927–1940. doi: 10.1093/jxb/erz074
Lee, S. B., Lee, J. Y., Kang, J. W., Mang, H., Kabange, N. R., Seong, G. U., et al. (2022). A novel locus for bakanae disease resistance, qBK4T, identified in rice. Agronomy 12, 1–11. doi: 10.3390/agronomy12102567
Lee, S., Marmagne, A., Park, J., Fabien, C., Yim, Y., Kim, S., et al. (2020). Concurrent activation of OsAMT1; 2 and OsGOGAT1 in rice leads to enhanced nitrogen use efficiency under nitrogen limitation. Plant J. 103, 7–20. doi: 10.1111/tpj.14794
Lee, J. H., Muhsin, M., Atienza, G. A., Kwak, D. Y., Kim, S. M., De Leon, T. B., et al. (2010). Single nucleotide polymorphisms in a gene for translation initiation factor (eIF4G) of rice (Oryza sativa) associated with resistance to rice tungro spherical virus. Mol. Plant-Microbe Interact. 23 (1), 29–38. doi: 10.1094/MPMI-23-1-0029
L’Hoir, M., Duponnois, R. (2021). Combining the seed endophytic bacteria and the back to the future approaches for plant holonbiont breeding. Front. Agron. 3, 724450. doi: 10.3389/fagro.2021.724450
Li, Y., Cao, X. L., Zhu, Y., Yang, X. M., Zhang, K. N., Xiao, Z. Y., et al. (2019b). Osa-miR398b boosts H 2 O 2 production and rice blast disease-resistance via multiple superoxide dismutases. New Phytol. 222, 1507–1522. doi: 10.1111/nph.15678
Li, Q., Lu, X., Wang, C., Shen, L., Dai, L., He, J., et al. (2022). Genome-wide association study and transcriptome analysis reveal new QTL and candidate genes for nitrogen-deficiency tolerance in rice. Crop J. 10, 942–951. doi: 10.1016/j.cj.2021.12.006
Li, S., Shen, L., Hu, P., Liu, Q., Zhu, X., Qian, Q., et al. (2019a). Developing disease-resistant thermosensitive male sterile rice by multiplex gene editing. J. Integr. Plant Biol. 61, 1201–1205. doi: 10.1111/jipb.12774
Linquist, B., Van Groenigen, K. J., Adviento-Borbe, M. A., Pittelkow, C., Van Kessel, C. (2012). An agronomic assessment of greenhouse gas emissions from major cereal crops. Glob Chang Biol. 18, 194–209. doi: 10.1111/j.1365-2486.2011.02502.x
Liu, M. H., Kang, H., Xu, Y., Peng, Y., Wang, D., Gao, L., et al. (2020). Genome-wide association study identifies an NLR gene that confers partial resistance to Magnaporthe oryzae in rice. Plant Biotechnol. J. 18, 1376–1383. doi: 10.1111/pbi.13300
Liu, Z., Li, X., Sun, F., Zhou, T., Zhou, Y. (2017). Overexpression of OsCIPK30 enhances plant tolerance to Rice stripe virus. Front. Microbiol. 8. doi: 10.3389/fmicb.2017.02322
Liu, J., Meng, Q., Xiang, H., Shi, F., Ma, L., Li, Y., et al. (2021). Genome-wide analysis of Dof transcription factors and their response to cold stress in rice (Oryza sativa L.). BMC Genomics 22, 1–12. doi: 10.1186/s12864-021-08104-0
Liu, K., Sakuraba, Y., Ohtsuki, N., Yang, M., Ueda, Y., Yanagisawa, S. (2023). CRISPR/Cas9-mediated elimination of OsHHO3, a transcriptional repressor of three AMMONIUM TRANSPORTER1 genes, improves nitrogen use efficiency in rice. Plant Biotechnol. J. 21, 2169–2172. doi: 10.1111/pbi.14167
Liu, J., Shen, Y., Cao, H., He, K., Chu, Z., Li, N. (2022). OsbHLH057 targets the AATCA cis-element to regulate disease resistance and drought tolerance in rice. Plant Cell Rep. 41, 1285–1299. doi: 10.1007/s00299-022-02859-w
Liu, X., Wang, H., Wang, H., Guo, Z., Xu, X., Liu, J., et al. (2018b). Factors affecting genomic selection revealed by empirical evidence in maize. Crop J. 6, 341–352. doi: 10.1016/j.cj.2018.03.005
Liu, Q., Yan, S., Huang, W., Yang, J., Dong, J., Zhang, S., et al. (2018a). NAC transcription factor ONAC066 positively regulates disease resistance by suppressing the ABA signaling pathway in rice. Plant Mol. Biol. 98, 289–302. doi: 10.1007/s11103-018-0768-z
Lorenz, A. J., Chao, S., Asoro, F. G., Heffner, E. L., Hayashi, T., Iwata, H., et al. (2011). Genomic selection in plant breeding. Knowledge and prospects. Adv. Agron. 110, 77–109. doi: 10.1016/B978-0-12-385531-2.00002-5
Lu, J., Eitel, J. U. H., Engels, M., Zhu, J., Ma, Y., Liao, F., et al. (2021a). Improving Unmanned Aerial Vehicle (UAV) remote sensing of rice plant potassium accumulation by fusing spectral and textural information. Int. J. Appl. Earth Observat. Geoinformation 104, 102592. doi: 10.1016/j.jag.2021.102592
Lu, Q., Wang, C., Niu, X., Zhang, M., Xu, Q., Feng, Y., et al. (2019). Detecting novel loci underlying rice blast resistance by integrating a genome-wide association study and RNA sequencing. Mol. Breed. 39, 1–10. doi: 10.1007/s11032-019-0989-0
Lu, J., Wang, C., Zeng, D., Li, J., Shi, X., Shi, Y., et al. (2021b). Genome-wide association study dissects resistance loci against bacterial blight in a diverse rice panel from the 3000 rice genomes project. Rice 14, 1–13. doi: 10.1186/s12284-021-00462-3
Lu, Y., Zhu, J. K. (2017). Precise editing of a target base in the rice genome using a modified CRISPR/cas9 system. Mol. Plant 10, 523–525. doi: 10.1016/j.molp.2016.11.013
Ludemann, C. I., Gruere, A., Heffer, P., Dobermann, A. (2022a). Global data on fertilizer use by crop and by country. Sci. Data 9, 1–8. doi: 10.1038/s41597-022-01592-z
Ludemann, C. I., Gruere, A., Heffer, P., Dobermann, A. (2022b). Global data on fertilizer use by crop and by country [Dataset] (United Kingdom: Dryad). doi: 10.1038/s41597-022-01592-z
Lundberg, D. S., Lebeis, S. L., Paredes, S. H., Yourstone, S., Gehring, J., Malfatti, S., et al. (2012). Defining the core Arabidopsis thaliana root microbiome. Nature 488, 86–90. doi: 10.1038/nature11237
Lynch, J. P. (2013). Steep, cheap and deep: An ideotype to optimize water and N acquisition by maize root systems. Ann. Bot. 112, 347–357. doi: 10.1093/aob/mcs293
Lynch, J. P. (2019). Root phenotypes for improved nutrient capture: an underexploited opportunity for global agriculture. New Phytol. 223, 548–564. doi: 10.1111/nph.15738
Lynch, J. P. (2022). Harnessing root architecture to address global challenges. Plant J. 109, 415–431. doi: 10.1111/tpj.15560
Mbodj, D., Effa-Effa, B., Kane, A., Manneh, B., Gantet, P., Laplaze, L., et al. (2018). Arbuscular mycorrhizal symbiosis in rice: establishment, environmental control and impact on plant growth and resistance to abiotic stresses. Rhizosphere 8, 12–26. doi: 10.1016/j.rhisph.2018.08.003
Mickelson, S., See, D., Meyer, F. D., Garner, J. P., Foster, C. R., Blake, T. K., et al. (2003). Mapping of QTL associated with nitrogen storage and remobilization in barley (Hordeum vulgare L.) leaves. J. Exp. Bot. 54, 801–812. doi: 10.1093/jxb/erg084
Miro, B., Ismail, A. M. (2013). Tolerance of anaerobic conditions caused by flooding during germination and early growth in rice (Oryza sativa L.). Front. Plant Sci. 4, 269. doi: 10.3389/fpls.2013.00269
Mishra, A. K., Kumar, A., Joshi, P. K., D’Souza, A., Tripathi, G. (2018). How can organic rice be a boon to smallholders? Evidence from contract farming in India. Food Policy 75, 147–157. doi: 10.1016/j.foodpol.2018.01.007
Moin, M., Bakshi, A., Madhav, M. S., Kirti, P. B. (2017). Expression profiling of ribosomal protein gene family in dehydration stress responses and characterization of transgenic rice plants overexpressing RPL23A for water-use efficiency and tolerance to drought and salt stresses. Front. Chem. 5. doi: 10.3389/fchem.2017.00097
Moin, M., Bakshi, A., Saha, A., Udaya Kumar, M., Reddy, A. R., Rao, K. V., et al. (2016). Activation tagging in indica rice identifies ribosomal proteins as potential targets for manipulation of water-use efficiency and abiotic stress tolerance in plants. Plant Cell Environ. 39, 2440–2459. doi: 10.1111/pce.12796
Mu, P., Li, Z., Li, C., Zhang, H., Wu, C., Li, C., et al. (2003). QTL mapping of the root traits and their correlation analysis with drought resistance using DH lines from paddy and upland rice cross. Chin. Sci. Bull. 48, 2718–2724. doi: 10.1007/BF02901763
Muellner, A. E., Mascher, F., Schneider, D., Ittu, G., Toncea, I., Rolland, B., et al. (2014). Refining breeding methods for organic and low-input agriculture: Analysis of an international winter wheat ring test. Euphytica 199, 81–95. doi: 10.1007/s10681-014-1169-2
Nada, R. M., Abogadallah, G. M. (2014). Aquaporins are major determinants of water use efficiency of rice plants in the field. Plant Sci. 227, 165–180. doi: 10.1016/j.plantsci.2014.08.006
Namuco, O. S., Cairns, J. E., Johnson, D. E. (2009). Investigating early vigour in upland rice (Oryza sativa L.): Part I. Seedling growth and grain yield in competition with weeds. Field Crops Res. 113, 197–206. doi: 10.1016/j.fcr.2009.05.008
Nerva, L., Sandrini, M., Moffa, L., Velasco, R., Balestrini, R., Chitarra, W. (2022). Breeding toward improved ecological plant–microbiome interactions. Trends Plant Sci. 27 (11), 1134–1143. doi: 10.1016/j.tplants.2022.06.004
Nguyen, H. T. T., Dang, D. T., Van Pham, C., Bertin, P. (2016). QTL mapping for nitrogen use efficiency and related physiological and agronomical traits during the vegetative phase in rice under hydroponics. Euphytica 212, 473–500. doi: 10.1007/s10681-016-1778-z
Ning, X., Yunyu, W., Aihong, L. (2020). Strategy for use of rice blast resistance genes in rice molecular breeding. Rice Sci. 27, 263–277. doi: 10.1016/j.rsci.2020.05.003
Panneerselvam, P., Senapati, A., Chidambaranathan, P., Prabhukarthikeyan, S. R., Mitra, D., Govindharaj, G. P. P., et al. (2023). Long-term impact of pulses crop rotation on soil fungal diversity in aerobic and wetland rice cultivation. Fungal Biol. 127, 1053–1066. doi: 10.1016/j.funbio.2023.04.005
Parr, J. F., Papendick, R. I., Youngberg, I. G., Meyer, R. E. (2020). “Sustainable agriculture in the United States,” in Sustainable agricultural systems (CRC Press), 50–67.
Peng, X., Hu, Y., Tang, X., Zhou, P., Deng, X., Wang, H., et al. (2012). Constitutive expression of rice WRKY30 gene increases the endogenous jasmonic acid accumulation, PR gene expression and resistance to fungal pathogens in rice. Planta 236, 1485–1498. doi: 10.1007/s00425-012-1698-7
Pérez-Jaramillo, J. E., Carrión, V. J., Bosse, M., Ferrão, L. F. V., De Hollander, M., Garcia, A. A. F., et al. (2017). Linking rhizosphere microbiome composition of wild and domesticated Phaseolus vulgaris to genotypic and root phenotypic traits. ISME J. 11, 2244–2257. doi: 10.1038/ismej.2017.85
Pérez-Jaramillo, J. E., Mendes, R., Raaijmakers, J. M. (2016). Impact of plant domestication on rhizosphere microbiome assembly and functions. Plant Mol. Biol. 90, 635–644. doi: 10.1007/s11103-015-0337-7
Peris-Peris, C., Serra-Cardona, A., Śanchez-Sanuy, F., Campo, S., Ariño, J., Segundo, B. S. (2017). Two NRAMP6 isoforms function as iron and manganese transporters and contribute to disease resistance in rice. Mol. Plant-Microbe Interact. 30, 385–398. doi: 10.1094/MPMI-01-17-0005-R
Phan, N. T. H., Draye, X., Pham, C.V., Bertin, P. (2023). Identification of quantitative trait loci controlling nitrogen use efficiency-related traits in rice at the seedling stage under salt condition by genome-wide association study. Front. Plant Sci. 14. doi: 10.3389/fpls.2023.1197271
Plett, D., Garnett, T., Okamoto, M. (2017). “Molecular genetics to discover and improve nitrogen use efficiency in crop plants,” in Plant macronutrient use efficiency: molecular and genomic perspectives in crop plants (Amsterdam, Netherlands: Elsevier), 93–122. doi: 10.1016/B978–0-12–811308–0.00006–5
Poudel, D. D., Horwath, W. R., Lanini, W. T., Temple, S. R., Van Bruggen, A. H. C. (2002). Comparison of soil N availability and leaching potential, crop yields and weeds in organic, low-input and conventional farming systems in northern California. Agric. Ecosyst. Environ. 90, 125–137. doi: 10.1016/S0167-8809(01)00196-7
Pradhan, S. K., Barik, S. R., Nayak, D. K., Pradhan, A., Pandit, E., Nayak, P., et al. (2020). Genetics, molecular mechanisms and deployment of bacterial blight resistance genes in rice. CRC Crit. Rev. Plant Sci. 39, 360–385. doi: 10.1080/07352689.2020.1801559
Prasanna, B. M., Palacios-Rojas, N., Hossain, F., Muthusamy, V., Menkir, A., Dhliwayo, T., et al. (2020). Molecular breeding for nutritionally enriched maize: status and prospects. Front. Genet. 10, 1392. doi: 10.3389/fgene.2019.01392
Ranaivo, H. N., Lam, D. T., Ueda, Y., Pariasca Tanaka, J., Takanashi, H., Ramanankaja, L., et al. (2022). QTL mapping for early root and shoot vigor of upland rice (Oryza sativa L.) under P deficient field conditions in Japan and Madagascar. Front. Plant Sci. 13. doi: 10.3389/fpls.2022.1017419
Rao, A. N., Johnson, D. E., Sivaprasad, B., Ladha, J. K., Mortimer, A. M. (2007). Weed management in direct-seeded rice. Adv. Agron. 93, 153–255. doi: 10.1016/S0065-2113(06)93004-1
Reganold, J. P., Wachter, J. M. (2016). Organic agriculture in the twenty-first century. Nat. Plants 2, 1–8. doi: 10.1038/nplants.2015.221
Robertsen, C. D., Hjortshøj, R. L., Janss, L. L. (2019). Genomic selection in cereal breeding. Agronomy 9, 1–16. doi: 10.3390/agronomy9020095
Robertson-Albertyn, S., Alegria Terrazas, R., Balbirnie, K., Blank, M., Janiak, A., Szarejko, I., et al. (2017). Root hair mutations displace the barley rhizosphere microbiota. Front. Plant Sci. 8, 1094. doi: 10.3389/fpls.2017.01094
Roja, V., Patil, S., Deborah, D. A., Srividhya, A., Ranjitkumar, N., Kadambari, G., et al. (2016). Finding genomic regions and candidate genes governing water use efficiency in rice. Biol. Plant 60, 757–766. doi: 10.1007/s10535-016-0651-1
Rolfe, B. G., Djordjevic, M. A., Weinman, J. J., Mathesius, U., Pittock, C., Gärtner, E., et al. (1997). Root morphogenesis in legumes and cereals and the effect of bacterial inoculation on root development (Faisalabad, Pakistan: Kluwer Academic Publishers).
Ruan, B., Hua, Z., Zhao, J., Zhang, B., Ren, D., Liu, C., et al. (2019). OsACL-A2 negatively regulates cell death and disease resistance in rice. Plant Biotechnol. J. 17, 1344–1356. doi: 10.1111/pbi.13058
Saito, K., Hayano-Saito, Y., Kuroki, M., Sato, Y. (2010). Map-based cloning of the rice cold tolerance gene Ctb1. Plant Sci. 179, 97–102. doi: 10.1016/j.plantsci.2010.04.004
Salvador-Guirao, R., Baldrich, P., Tomiyama, S., Hsing, Y. I., Okada, K., Segundo, B. S. (2019). Osdcl1a activation impairs phytoalexin biosynthesis and compromises disease resistance in rice. Ann. Bot. 123, 79–93. doi: 10.1093/aob/mcy141
Sasse, J., Martinoia, E., Northen, T. (2018). Feed your friends: do plant exudates shape the root microbiome? Trends Plant Sci. 23, 25–41. doi: 10.1016/j.tplants.2017.09.003
Sattayachiti, W., Wanchana, S., Arikit, S., Nubankoh, P., Patarapuwadol, S., Vanavichit, A., et al. (2020). Genome-wide association analysis identifies resistance loci for bacterial leaf streak resistance in rice (Oryza sativa L.). Plants 9, 1–16. doi: 10.3390/plants9121673
Schmid, M. W., Hahl, T., van Moorsel, S. J., Wagg, C., De Deyn, G. B., Schmid, B. (2019). Feedbacks of plant identity and diversity on the diversity and community composition of rhizosphere microbiomes from a long-term biodiversity experiment. Mol. Ecol. 28, 863–878. doi: 10.1111/mec.14987
Seufert, V., Ramankutty, N., Foley, J. A. (2012). Comparing the yields of organic and conventional agriculture. Nature 485, 229–232. doi: 10.1038/nature11069
Shaodan, L., Yue, Y., Jiayi, L., Xiaobin, L., Jie, M., Haiyong, W., et al. (2023). Application of UAV-based imaging and deep learning in assessment of rice blast resistance. Rice Sci. 30, 652–660. doi: 10.1016/j.rsci.2023.06.005
She, K.-C., Kusano, H., Koizumi, K., Yamakawa, H., Hakata, M., Imamura, T., et al. (2010). A novel factor FLOURY ENDOSPERM2 is involved in regulation of rice grain size and starch quality. Plant Cell 22, 3280–3294. doi: 10.1105/tpc.109.070821
Shen, C., Liu, L., Zhu, L., Kang, J., Wang, N., Shao, L. (2020). High-throughput in situ root image segmentation based on the improved deepLabv3+ Method. Front. Plant Sci. 11. doi: 10.3389/fpls.2020.576791
Shrawat, A. K., Carroll, R. T., DePauw, M., Taylor, G. J., Good, A. G. (2008). Genetic engineering of improved nitrogen use efficiency in rice by the tissue-specific expression of alanine aminotransferase. Plant Biotechnol. J. 6, 722–732. doi: 10.1111/j.1467-7652.2008.00351.x
Shrestha, R. K., Ladha, J. K. (1996). Genotypic variation in promotion of rice dinitrogen fixation as determined by nitrogen-15 dilution. Soil Sci. Soc. America J. 60, 1815–1821. doi: 10.2136/sssaj1996.03615995006000060029x
Shu, X., Wang, A., Jiang, B., Jiang, Y., Xiang, X., Yi, X., et al. (2021). Genome-wide association study and transcriptome analysis discover new genes for bacterial leaf blight resistance in rice (Oryza sativa L.). BMC Plant Biol. 21, 1–13. doi: 10.1186/s12870-021-03041-2
Singh, G., Kaur, N., Khanna, R., Kaur, R., Gudi, S., Kaur, R., et al. (2024). 2Gs and plant architecture: breaking grain yield ceiling through breeding approaches for next wave of revolution in rice (Oryza sativa L.). Crit. Rev. Biotechnol. 44 (1), 139–162. doi: 10.1080/07388551.2022.2112648
Singh, A. P., Pandey, B. K., Mehra, P., Heitz, T., Giri, J. (2020). OsJAZ9 overexpression modulates jasmonic acid biosynthesis and potassium deficiency responses in rice. Plant Mol. Biol. 104, 397–410. doi: 10.1007/s11103-020-01047-2
Singh, U. M., Yadav, S., Dixit, S., Ramayya, P. J., Devi, M. N., Raman, K. A., et al. (2017). QTL hotspots for early vigor and related traits under dry direct-seeded system in rice (Oryza sativa L.). Front. Plant Sci. 8. doi: 10.3389/fpls.2017.00286
Smith, S. E., Smith, F. A., Jakobsen, I. (2003). Mycorrhizal fungi can dominate phosphate supply to plants irrespective of growth responses. Plant Physiol. 133, 16–20. doi: 10.1104/pp.103.024380
Srikanth, B., Subhakara Rao, I., Surekha, K., Subrahmanyam, D., Voleti, S. R., Neeraja, C. N. (2016). Enhanced expression of OsSPL14 gene and its association with yield components in rice (Oryza sativa) under low nitrogen conditions. Gene 576, 441–450. doi: 10.1016/j.gene.2015.10.062
Stram, D. O. (2017). Multi-SNP haplotype analysis methods for association analysis. Stat. Hum. Genet.: Methods Protoc. 1666, 485–504. doi: 10.1007/978-1-4939-7274-6_24
Sun, H., Qian, Q., Wu, K., Luo, J., Wang, S., Zhang, C., et al. (2014). Heterotrimeric G proteins regulate nitrogen-use efficiency in rice. Nat. Genet. 46, 652–656. doi: 10.1038/ng.2958
Sun, J., Rutkoski, J. E., Poland, J. A., Crossa, J., Jannink, J., Sorrells, M. E. (2017). Multitrait, random regression, or simple repeatability model in high-throughput phenotyping data improve genomic prediction for wheat grain yield. Plant Genome 10, 1–12. doi: 10.3835/plantgenome2016.11.0111
Szoboszlay, M., Lambers, J., Chappell, J., Kupper, J. V., Moe, L. A., McNear, J. D.H. (2015). Comparison of root system architecture and rhizosphere microbial communities of Balsas teosinte and domesticated corn cultivars. Soil Biol. Biochem. 80, 34–44. doi: 10.1016/j.soilbio.2014.09.001
Tang, W., Ye, J., Yao, X., Zhao, P., Xuan, W., Tian, Y., et al. (2019). Genome-wide associated study identifies NAC42-activated nitrate transporter conferring high nitrogen use efficiency in rice. Nat. Commun. 10, 5279. doi: 10.1038/s41467-019-13187-1
Tezuka, D., Kawamata, A., Kato, H., Saburi, W., Mori, H., Imai, R. (2019). The rice ethylene response factor OsERF83 positively regulates disease resistance to Magnaporthe oryzae. Plant Physiol. Biochem. 135, 263–271. doi: 10.1016/j.plaphy.2018.12.017
Thapa, R., Septiningsih, E. M. (2021). Genome-wide association study for traits related to seedling vigor in rice. Crop Sci. 61, 3931–3946. doi: 10.1002/csc2.20585
This, D., Comstock, J., Courtois, B., Xu, Y., Ahmadi, N., Vonhof, W. M., et al. (2010). Genetic analysis of water use efficiency in rice (Oryza sativa L.) at the leaf level. Rice 3, 72–86. doi: 10.1007/s12284-010-9036-9
Tibbs Cortes, L., Zhang, Z., Yu, J. (2021). Status and prospects of genome-wide association studies in plants. Plant Genome 14, 1–17. doi: 10.1002/tpg2.20077
To, H. T. M., Le, K. Q., Van Nguyen, H., Duong, L. V., Kieu, H. T., Chu, Q. A. T., et al. (2020). A genome-wide association study reveals the quantitative trait locus and candidate genes that regulate phosphate efficiency in a Vietnamese rice collection. Physiol. Mol. Biol. Plants 26, 2267–2281. doi: 10.1007/s12298-020-00902-2
Tsakirpaloglou, N., Septiningsih, E. M., Thomson, M. J. (2023). Guidelines for performing CRISPR/cas9 genome editing for gene validation and trait improvement in crops. Plants 12, 1–14. doi: 10.3390/plants12203564
UN. (2022). World population prospects 2022: ten key messages (New York, USA: United Nations Department of Global Communications). Available at: https://www.un.org/development/desa/pd/sites/www.un.org.development.desa.pd/files/undesa_pd_2022_wpp_key-messages.pdf.
van Bruggen, A. H. C., Gamliel, A., Finckh, M. R. (2016). Plant disease management in organic farming systems. Pest Manag Sci. 72, 30–44. doi: 10.1002/ps.4145
Vanloqueren, G., Baret, P. V. (2008). Why are ecological, low-input, multi-resistant wheat cultivars slow to develop commercially? a belgian agricultural ‘lock-in’case study. Ecol. Economics 66 (2-3), 436–446. doi: 10.1016/j.ecolecon.2007.10.007
Varshney, R. K., Bohra, A., Yu, J., Graner, A., Zhang, Q., Sorrells, M. E. (2021). Designing future crops: genomics-assisted breeding comes of age. Trends Plant Sci. 26, 631–649. doi: 10.1016/j.tplants.2021.03.010
Vergara, C., Araujo, K. E. C., Sperandio, M. V. L., Santos, L. A., Urquiaga, S., Zilli, J.É. (2019). Dark septate endophytic fungi increase the activity of proton pumps, efficiency of 15 N recovery from ammonium sulphate, N content, and micronutrient levels in rice plants. Braz. J. Microbiol. 50, 825–838. doi: 10.1007/s42770-019-00092-4
Verma, N., Narayan, O. P., Prasad, D., Jogawat, A., Panwar, S. L., Dua, M., et al. (2022). Functional characterization of a high-affinity iron transporter (PiFTR) from the endophytic fungus Piriformospora indica and its role in plant growth and development. Environ. Microbiol. 24, 689–706. doi: 10.1111/1462-2920.15659
Volante, A., Tondelli, A., Aragona, M., Valente, M. T., Biselli, C., Desiderio, F., et al. (2017). Identification of bakanae disease resistance loci in japonica rice through genome wide association study. Rice 10, 1–16. doi: 10.1186/s12284-017-0168-z
Wan, X., Chen, X., Huang, Z., Chen, H. Y. H. (2021). Contribution of root traits to variations in soil microbial biomass and community composition. Plant Soil 460, 483–495. doi: 10.1007/s11104-020-04788-7
Wang, K., Cui, K., Liu, G., Xie, W., Yu, H., Pan, J., et al. (2014). Identification of quantitative trait loci for phosphorus use efficiency traits in rice using a high density SNP map. BMC Genet. 15, 1–15. doi: 10.1186/s12863-014-0155-y
Wang, Q., Li, J., Hu, L., Zhang, T., Zhang, G., Lou, Y. (2013). OsMPK3 positively regulates the JA signaling pathway and plant resistance to a chewing herbivore in rice. Plant Cell Rep. 32, 1075–1084. doi: 10.1007/s00299-013-1389-2
Wang, X. M., Liang, Y. Y., Li, L., Gong, C. W., Wang, H. P., Huang, X. X., et al. (2015). Identification and cloning of tillering-related genes osMAX1 in rice. Rice Sci. 22, 255–263. doi: 10.1016/j.rsci.2015.06.001
Wang, F., Longkumer, T., Catausan, S. C., Calumpang, C. L. F., Tarun, J. A., Cattin-Ortola, J., et al. (2018a). Genome-wide association and gene validation studies for early root vigour to improve direct seeding of rice. Plant Cell Environ. 41, 2731–2743. doi: 10.1111/pce.13400
Wang, Q., Nian, J., Xie, X., Yu, H., Zhang, J., Bai, J., et al. (2018b). Genetic variations in ARE1 mediate grain yield by modulating nitrogen utilization in rice. Nat. Commun. 9, 1–10. doi: 10.1038/s41467-017-02781-w
Wang, L., Pang, X., Li, N., Qi, K., Huang, J., Yin, C. (2020b). Effects of vegetation type, fine and coarse roots on soil microbial communities and enzyme activities in eastern Tibetan plateau. Catena (Amst) 194, 104694. doi: 10.1016/j.catena.2020.104694
Wang, A., Shu, X., Jing, X., Jiao, C., Chen, L., Zhang, J., et al. (2021a). Identification of rice (Oryza sativa L.) genes involved in sheath blight resistance via a genome-wide association study. Plant Biotechnol. J. 19, 1553–1566. doi: 10.1111/pbi.13569
Wang, Q., Su, Q., Nian, J., Zhang, J., Guo, M., Dong, G., et al. (2021b). The Ghd7 transcription factor represses ARE1 expression to enhance nitrogen utilization and grain yield in rice. Mol. Plant 14, 1012–1023. doi: 10.1016/j.molp.2021.04.012
Wang, Q., Wang, N., Wang, Y., Wang, Q., Duan, B. (2017). Differences in root-associated bacterial communities among fine root branching orders of poplar (Populus× euramericana (Dode) Guinier.). Plant Soil 421, 123–135. doi: 10.1007/s11104-017-3449-9
Wang, X., Xu, Y., Li, P., Liu, M., Xu, C., Hu, Z. (2019). Efficiency of linear selection index in predicting rice hybrid performance. Mol. Breed. 39, 1–13. doi: 10.1007/s11032-019-0986-3
Wang, Z., Zhang, W., Beebout, S. S., Zhang, H., Liu, L., Yang, J., et al. (2016). Grain yield, water and nitrogen use efficiencies of rice as influenced by irrigation regimes and their interaction with nitrogen rates. Field Crops Res. 193, 54–69. doi: 10.1016/j.fcr.2016.03.006
Wang, H., Zhang, Y., Zhang, Y., McDaniel, M. D., Sun, L., Su, W., et al. (2020a). Water-saving irrigation is a ‘win-win’ management strategy in rice paddies – With both reduced greenhouse gas emissions and enhanced water use efficiency. Agric. Water Manag 228, 1–7. doi: 10.1016/j.agwat.2019.105889
Waqas, M., Feng, S., Amjad, H., Letuma, P., Zhan, W., Li, Z., et al. (2018). Protein phosphatase (PP2C9) induces protein expression differentially to mediate nitrogen utilization efficiency in rice under nitrogen-deficient condition. Int. J. Mol. Sci. 19, 1–24. doi: 10.3390/ijms19092827
Wassmann, R., Nelson, G. C., Peng, S. B., Sumfleth, K., Jagadish, S. V. K., Hosen, Y., et al. (2010). Rice and global climate change. Rice in the global economy: strategic research and policy issues for food security. (Los Baños Philippines: International Rice Research Institute), 411–432.
Wei, D., Cui, K., Pan, J., Ye, G., Xiang, J., Nie, L., et al. (2011). Genetic dissection of grain nitrogen use efficiency and grain yield and their relationship in rice. Field Crops Res. 124, 340–346. doi: 10.1016/j.fcr.2011.07.003
Wickings, K., Grandy, A. S., Kravchenko, A. N. (2016). Going with the flow: Landscape position drives differences in microbial biomass and activity in conventional, low input, and organic agricultural systems in the Midwestern U.S. Agric. Ecosyst. Environ. 218, 1–10. doi: 10.1016/j.agee.2015.11.005
Wissuwa, M., Kondo, K., Fukuda, T., Mori, A., Rose, M. T., Pariasca-Tanaka, J., et al. (2015). Unmasking novel loci for internal phosphorus utilization efficiency in rice germplasm through genome-wide association analysis. PloS One 10, 1–21. doi: 10.1371/journal.pone.0124215
Wolfe, M. S., Baresel, J. P., Desclaux, D., Goldringer, I., Hoad, S., Kovacs, G., et al. (2008). Developments in breeding cereals for organic agriculture. Euphytica 163, 323–346. doi: 10.1007/s10681-008-9690-9
Wu, D., Li, Y., Cao, Y., Hu, R., Wu, X., Zhang, W., et al. (2021a). Increased glutamine synthetase by overexpression of TaGS1 improves grain yield and nitrogen use efficiency in rice. Plant Physiol. Biochem. 169, 259–268. doi: 10.1016/j.plaphy.2021.11.021
Wu, P., Zhang, G., Ladha, J. K., McCouch, S. R., Huang, N. (1995). Molecular-marker-facilitated investigation on the ability to stimulate N 2 fixation in the rhizosphere by irrigated rice plants. Theor. Appl. Genet. 91, 1177–1183. doi: 10.1007/BF00220926
Wu, J., Zhang, Z. S., Xia, J. Q., Alfatih, A., Song, Y., Huang, Y. J., et al. (2021b). Rice NIN-LIKE PROTEIN 4 plays a pivotal role in nitrogen use efficiency. Plant Biotechnol. J. 19, 448–461. doi: 10.1111/pbi.13475
Xie, C., Yang, C. (2020). A review on plant high-throughput phenotyping traits using UAV-based sensors. Comput. Electron Agric. 178, 1–14. doi: 10.1016/j.compag.2020.105731
Xin, W., Wang, J., Li, J., Zhao, H., Liu, H., Zheng, H., et al. (2021). Candidate gene analysis for nitrogen absorption and utilization in japonica rice at the seedling stage based on a genome-wide association study. Front. Plant Sci. 12. doi: 10.3389/fpls.2021.670861
Xu, Y., Ma, K., Zhao, Y., Wang, X., Zhou, K., Yu, G., et al. (2021). Genomic selection: A breakthrough technology in rice breeding. Crop J. 9, 669–677. doi: 10.1016/j.cj.2021.03.008
Xu, Y., This, D., Pausch, R. C., Vonhof, W. M., Coburn, J. R., Comstock, J. P., et al. (2009). Leaf-level water use efficiency determined by carbon isotope discrimination in rice seedlings: Genetic variation associated with population structure and QTL mapping. Theor. Appl. Genet. 118, 1065–1081. doi: 10.1007/s00122-009-0963-z
Xue, X., Cao, Z. X., Zhang, X. T., Wang, Y., Zhang, Y. F., Chen, Z. X., et al. (2016). Overexpression of OsOSM1 enhances resistance to rice sheath blight. Plant Dis. 100, 1634–1642. doi: 10.1094/PDIS-11-15-1372-RE
Xue, Y., Duan, H., Liu, L., Wang, Z., Yang, J., Zhang, J. (2013). An improved crop management increases grain yield and nitrogen and water use efficiency in rice. Crop Sci. 53, 271–284. doi: 10.2135/cropsci2012.06.0360
Yamaguchi, T., Kuroda, M., Yamakawa, H., Ashizawa, T., Hirayae, K., Kurimoto, L., et al. (2009). Suppression of a phospholipase d gene, ospldb1, activates defense responses and increases disease resistance in rice. Plant Physiol. 150, 308–319. doi: 10.1104/pp.108.131979
Yamauchi, A., Kono, Y., Tatsumi, J. (1987). Quantitative analysis on root system structures of upland rice and maize. Japanese J. Crop Sci. 56, 608–617. doi: 10.1626/jcs.56.608
Yang, J., Guo, Z., Luo, L., Gao, Q., Xiao, W., Wang, J., et al. (2021). Identification of QTL and candidate genes involved in early seedling growth in rice via high-density genetic mapping and RNA-seq. Crop J. 9, 360–371. doi: 10.1016/j.cj.2020.08.010
Yang, X., Nong, B., Chen, C., Wang, J., Xia, X., Zhang, Z., et al. (2023). OsNPF3.1, a member of the NRT1/PTR family, increases nitrogen use efficiency and biomass production in rice. Crop J. 11, 108–118. doi: 10.1016/j.cj.2022.07.001
Yi, K., Wu, Z., Zhou, J., Du, L., Guo, L., Wu, Y., et al. (2005). OsPTF1, a novel transcription factor involved in tolerance to phosphate starvation in rice. Plant Physiol. 138, 2087–2096. doi: 10.1104/pp.105.063115
Yokotani, N., Sato, Y., Tanabe, S., Chujo, T., Shimizu, T., Okada, K., et al. (2013). WRKY76 is a rice transcriptional repressor playing opposite roles in blast disease resistance and cold stress tolerance. J. Exp. Bot. 64, 5085–5097. doi: 10.1093/jxb/ert298
Yoon, D. K., Suganami, M., Ishiyama, K., Kagawa, T., Tanaka, M., Nagao, R., et al. (2022). The gs3 allele from a large-grain rice cultivar, Akita 63, increases yield and improves nitrogen-use efficiency. Plant Direct 6, 1–12. doi: 10.1002/pld3.417
Yu, C., Chen, Y., Cao, Y., Chen, H., Wang, J., Bi, Y. M., et al. (2018). Overexpression of miR169o, an overlapping MicroRNA in response to both nitrogen limitation and bacterial infection, promotes nitrogen use efficiency and susceptibility to bacterial blight in rice. Plant Cell Physiol. 59, 1234–1247. doi: 10.1093/pcp/pcy060
Yu, J., Xuan, W., Tian, Y., Fan, L., Sun, J., Tang, W., et al. (2021). Enhanced OsNLP4-OsNiR cascade confers nitrogen use efficiency by promoting tiller number in rice. Plant Biotechnol. J. 19, 167–176. doi: 10.1111/pbi.13450
Yuan, S., Linquist, B. A., Wilson, L. T., Cassman, K. G., Stuart, A. M., Pede, V., et al. (2021). Sustainable intensification for a larger global rice bowl. Nat. Commun. 12, 1–11. doi: 10.1038/s41467-021-27424-z
Yuan, B., Shen, X., Li, X., Xu, C., Wang, S. (2007). Mitogen-activated protein kinase OsMPK6 negatively regulates rice disease resistance to bacterial pathogens. Planta 226, 953–960. doi: 10.1007/s00425-007-0541-z
Yuanyuan, N., Hui, X., Xiaosong, M., Qiaojun, L., Yi, L., Anling, Z., et al. (2022). Dissecting genetic basis of deep rooting in dongxiang wild rice. Rice Sci. 29, 277–287. doi: 10.1016/j.rsci.2021.11.002
Zeng, M., Yang, J., Wu, K., Wang, H., Sun, K., Chen, Z., et al. (2021). Genome-wide association study reveals early seedling vigour-associated quantitative trait loci in indica rice. Euphytica 217, 1–16. doi: 10.1007/s10681-021-02868-y
Zhang, M., Lai, L., Liu, X., Liu, J., Liu, R., Wang, Y., et al. (2022a). Overexpression of nitrate transporter 1/peptide gene osNPF7.6 increases rice yield and nitrogen use efficiency. Life 12, 1–10. doi: 10.3390/life12121981
Zhang, J., Liu, Y. X., Zhang, N., Hu, B., Jin, T., Xu, H., et al. (2019). NRT1.1B is associated with root microbiota composition and nitrogen use in field-grown rice. Nat. Biotechnol. 37, 676–684. doi: 10.1038/s41587-019-0104-4
Zhang, F., Wu, Z. C., Wang, M. M., Dingkuhn, M., Xu, J. L., Zhou, Y. L., et al. (2017). Genome-wide association analysis identifies resistance loci for bacterial blight in a diverse collection of indica rice germplasm. PloS One 12, 1–17. doi: 10.1371/journal.pone.0174598
Zhang, Z. S., Xia, J. Q., Alfatih, A., Song, Y., Huang, Y. J., Sun, L. Q., et al. (2022b). Rice NIN-LIKE PROTEIN 3 modulates nitrogen use efficiency and grain yield under nitrate-sufficient conditions. Plant Cell Environ. 45, 1520–1536. doi: 10.1111/pce.14294
Zhao, X., Qiu, T., Feng, H., Yin, C., Zheng, X., Yang, J., et al. (2021b). A novel glycine-rich domain protein, GRDP1, functions as a critical feedback regulator for controlling cell death and disease resistance in rice. J. Exp. Bot. 72, 608–622. doi: 10.1093/jxb/eraa450
Zhao, J., Yang, B., Li, W., Sun, S., Peng, L., Feng, D., et al. (2021a). A genome-wide association study reveals that the glucosyltransferase OsIAGLU regulates root growth in rice. J. Exp. Bot. 72, 1119–1134. doi: 10.1093/jxb/eraa512
Zhen, X., Xu, F., Zhang, W., Li, N., Li, X. (2019). Overexpression of rice gene OsATG8b confers tolerance to nitrogen starvation and increases yield and nitrogen use efficiency (NUE) in Arabidopsis. PloS One 14, 1–18. doi: 10.1371/journal.pone.0223011
Zhou, Y., Tao, Y., Tang, D., Wang, J., Zhong, J., Wang, Y., et al. (2017). Identification of QTL associated with nitrogen uptake and nitrogen use efficiency using high throughput genotyped CSSLs in rice (Oryza sativa l.). Front. Plant Sci. 8, 1166. doi: 10.3389/fpls.2017.01166
Zhu, D., Kang, H., Li, Z., Liu, M., Zhu, X., Wang, Y., et al. (2016). A genome-wide association study of field resistance to magnaporthe oryzae in rice. Rice 9, 1–9. doi: 10.1186/s12284-016-0116-3
Zhu, Y., Li, T., Xu, J., Wang, J., Wang, L., Zou, W., et al. (2020). Leaf width gene LW5/D1 affects plant architecture and yield in rice by regulating nitrogen utilization efficiency. Plant Physiol. Biochem. 157, 359–369. doi: 10.1016/j.plaphy.2020.10.035
Zhu, X. F., Wang, Z. W., Wan, J. X., Sun, Y., Wu, Y. R., Li, G. X., et al. (2015). Pectin enhances rice (Oryza sativa) root phosphorus remobilization. J. Exp. Bot. 66, 1017–1024. doi: 10.1093/jxb/eru461
Keywords: sustainability, high-throughput phenotyping, genomics, genomic selection, organic rice, low-input agriculture
Citation: Jyoti SD, Singh G, Pradhan AK, Tarpley L, Septiningsih EM and Talukder SK (2024) Rice breeding for low input agriculture. Front. Plant Sci. 15:1408356. doi: 10.3389/fpls.2024.1408356
Received: 28 March 2024; Accepted: 24 May 2024;
Published: 21 June 2024.
Edited by:
Dayun Tao, Yunnan Academy of Agricultural Sciences, ChinaReviewed by:
Parameswaran C, Indian Council of Agricultural Research (ICAR), IndiaAnandan Annamalai, Indian Institute of Seed Science, India
Copyright © 2024 Jyoti, Singh, Pradhan, Tarpley, Septiningsih and Talukder. This is an open-access article distributed under the terms of the Creative Commons Attribution License (CC BY). The use, distribution or reproduction in other forums is permitted, provided the original author(s) and the copyright owner(s) are credited and that the original publication in this journal is cited, in accordance with accepted academic practice. No use, distribution or reproduction is permitted which does not comply with these terms.
*Correspondence: Shyamal K. Talukder, shyamal.talukder@ag.tamu.edu