- 1Key Laboratory of Integrated Regulation and Resource Development on Shallow Lakes, Ministry of Education, College of Environment, Hohai University, Nanjing, China
- 2College of Agriculture, Nanjing Agricultural University, Nanjing, China
- 3College of Physics and Optoelectronic Engineering, Shenzhen University, Shenzhen, China
- 4Department of Agronomy, Iowa State University, Ames, IA, United States
Recently, a rapid advancement in using unmanned aerial vehicles (UAVs) for yield prediction (YP) has led to many YP research findings. This study aims to visualize the intellectual background, research progress, knowledge structure, and main research frontiers of the entire YP domain for main cereal crops using VOSviewer and a comprehensive literature review. To develop visualization networks of UAVs related knowledge for YP of wheat, maize, rice, and soybean (WMRS) crops, the original research articles published between January 2001 and August 2023 were retrieved from the web of science core collection (WOSCC) database. Significant contributors have been observed to the growth of YP-related research, including the most active countries, prolific publications, productive writers and authors, the top contributing institutions, influential journals, papers, and keywords. Furthermore, the study observed the primary contributions of YP for WMRS crops using UAVs at the micro, meso, and macro levels and the degree of collaboration and information sources for YP. Moreover, the policy assistance from the People’s Republic of China, the United States of America, Germany, and Australia considerably advances the knowledge of UAVs connected to YP of WMRS crops, revealed under investigation of grants and collaborating nations. Lastly, the findings of WMRS crops for YP are presented regarding the data type, algorithms, results, and study location. The remote sensing community can significantly benefit from this study by being able to discriminate between the most critical sub-domains of the YP literature for WMRS crops utilizing UAVs and to recommend new research frontiers for concentrating on the essential directions for subsequent studies.
1 Introduction
Wheat, maize, and rice were responsible for 30% of the world’s crop production by the year 2020. Furthermore, when it came to the exports of the commodities that were produced from cereal crops, wheat, maize, and rice contributed 45%, 32%, and 9% respectively. Simultaneously, soybean contributed 28% of oil production (FAO, 2022). These crops are of keen importance to address the food security issue. Hence, the global research developments can be examined to update and for self-evaluation in fulfilling the current food demands. By delving deeply into a particular study’s academic history and knowledge structure, it is possible to identify the research themes, knowledge base, research frontiers, and hotspots worldwide. The extensive background of scientific investigations can be categorized using a range of distinct specialties, including institutions, collaborating authors, countries, co-occurring keywords, publications, hot research topics, cited references, and knowledge clusters. Leveraging the co-occurring keywords in articles from databases to conduct a literature clustering helps to reveal the knowledge structure and domains (Qian et al., 2019; Chen and Liu, 2020). These maps can be represented as networks to create a structure of these analyses known as bibliometric (or scientormetric) analysis (Ahmed et al., 2021; Azam et al., 2021; Hussain et al., 2023). Consequently, this review systematically interprets the intellectual history of a research topic or scientific literature through this novel approach. Moreover, it provides insight into new breakthroughs relevant for researchers, business investors, engineers, and the author or institutional collaboration knowledge structures and emerging research topics. This review article focusses on the use of UAVs in yield prediction (YP), and particularly with applications to wheat, maize, rice and soybean (WMRS) cropping systems: given its importance for food security at global scale.
Numerous approaches are being made for WMRS’s sustainable production, protection, monitoring, and estimation, and one use is UAVs. YP analysis has been increasingly popular as big data technologies have advanced (Barnetson et al., 2020). Consulting firms and policymakers frequently employ such models when developing effective strategies (Astor et al., 2020). One of the most critical challenges in agriculture is grain yield regarding personal living standards and food security. The quantity of crop produced in a certain year is referred as the yield (Fischer et al., 2014). Several factors affect here, like genetic features, soil, weather, cultivation, and a wide range of varietals crop yields. Remote sensing (RS), a relatively new technique is expected to help calculate rice yield, especially on a regional scale. With the emergence of UAVs, a unique strategy for RS has been provided, and high spatiotemporal resolution imaging on a regional scale is now possible (Zheng et al., 2019). However, it wasn’t till 2000s that the UAV technology took off in the agricultural sector, thanks to ground-breaking innovations that made the technology more accessible, user-friendly, and affordable. Examples of agricultural-grade UAVs with sensors available in 2019 include the Matrice 100 (Dà-Jiang Innovations, Shenzhen, China) and MicaSense Red Edge MX (Seattle, Washington) as well as the Pix4D Fields (Lausanne, Switzerland) and hardware from Pix4D (Puget Systems, 2021) could be purchased for less than $30,000. In addition, using UAVs' sensors has facilitated the collection of visual data, which can now be analyzed through cloud-based computing services. This advancement has decreased the requirement for costly image processing software and hardware that formerly had to be present on-site. Notably, Pix4D Fields, a software developed by Pix4D in Lausanne, Switzerland, is an example of such cloud-based computing services. Typically, the acquisition of UAVs-based data involves several stages: the formulation of a mission plan, the collection of imagery during the operation, the subsequent stitching, processing, and extraction of the data, and ultimately, the uploading of the resulting data output to precision agriculture machines or for statistical analysis in the context of research. Figure 1 demonstrates a conventional method for acquiring quantifiable and actionable data from multispectral and hyperspectral UAVs.
Additionally, satellites, manned airplanes, and handheld sensors can all be used for RS (Melesse et al., 2007). However, satellites’ drawbacks include lesser resolutions, cloud blockage, scheduling, and location issues. Manned aircraft can cover large areas, but the expense is overpriced. While handheld sensors are highly accurate, they have far smaller coverage areas than aerial RS. UAVs are appealing for agricultural applications due to their ability to efficiently cover large areas, unaffected by cloud interference, unrestricted by location or timing constraints, and reasonably cost-effective (Muchiri and Kimathi, 2022). However, some disadvantages of UAVs-based RS are data degradation due to lighting conditions, data collection’s tendency to occur close to solar noon, airspace restrictions, and poor weather grounding. Regulations regarding the usage of UAVs vary greatly throughout countries and often encompass limitations on velocity and elevation, nocturnal operations, visual range, proximity to airports, and densely populated regions. Additional issues to take into account during secure flight operations include the presence of other manned aircraft, prey birds, and disruptions in controller communication that could result in out of control.
Crop improvement through genetics and plant breeding and the use of precision technologies such as UAVs and remote sensors are potential solutions to meet this demand (Yang et al., 2017). Such technologies are vital for strategic management and can lead to specific breeding decisions and ensure the maximum agricultural outputs (Tilman et al., 2011). The technologies like UAVs in RS, enable rapid collection of phenotypic data with an efficient and non-destructive way for agronomists and plant breeders (Yang et al., 2017). Examples include estimating leaf color (Graham et al., 2009), lodging (Zhao et al., 2019), plant height (Han et al., 2018), stand count (Zhao et al., 2018), canopy cover (Lee and Lee, 2011), fruit count (Dorj et al., 2017) and flower count (Adamsen et al., 2000). The spectral sensors can be used to calculate yield (Shanahan et al., 2001), leaf area index (Boegh et al., 2013), leaf chlorophyll content, indirect leaf nitrogen content, and plant biomass. Finally, thermal sensors gather information to calculate canopy temperature, stomatal conductance, plant water potential and water use efficiency (Santesteban et al., 2017).
Phenotyping estimations rely significantly on the duration of sensing, and as crops reach maturity, phenotyping assessments improve in accuracy. In most cases, the difference between the terrain model and the surface model must be calculated to predict crop height; this yields the so-called digital crop model (Han et al., 2018). Low-resolution cameras (3 megapixels) cannot catch the fine details of complex crop surfaces, hence only cameras with superior resolution (>10 megapixels) should be utilized. Additionally, it has been demonstrated that the “Scale Constraint” function of some imaging processing software and ground control points improves crop height estimates. Refraining from conducting sensing activities on days with wind speeds over 1-10 kilometers per hour is advisable (Sziroczak et al., 2022). This precaution is recommended due to the propensity of wind to induce movement in plants and result in image blur, hence diminishing the precision of crop height estimation.
1.1 Background for yield assessment
Numerous parties rely on crop output and quality estimates, including consultants, producers, academics, insurance agents, commodities merchants, governments, and non-governmental organizations (Rembold et al., 2013). This data helps to determine how much crop insurance to purchase, how much to deliver, when to harvest to maximize quality, how much space is needed for storage, and how much money will be needed. Traditionally, managers have relied on historical yield data and seasonal variables to inform their yield and quality assessments for the remaining season (Raun et al., 2001). In contrast, the ultimate outcomes of crop production and the resulting quality are characterized by a lack of predictability and often subject to factors such as crop genetics, weather conditions, soil composition, proficiency, and choices made in crop management. The assessment of crop production and quality through the utilization of UAVs technologies commonly depends on data acquired from color and spectral sensors. The utilization of UAVs imagery, particularly in conjunction with machine learning (ML) techniques, currently holds the capacity to enhance assessment precision and potentially diminish or eradicate the need for terrestrial surveys. The accurate estimation of crop yield and quality is contingent upon the ability to effectively sense time, as the accuracy of estimation tends to improve as the crop progresses through its life cycle (Ballester et al., 2017; Zhou et al., 2017).
The green normalized difference vegetation index (GNDVI) yielded more precise biomass estimations at the stages of anthesis and full crop development than at earlier stages of development (Ostos-Garrido et al., 2019). According to other studies, it has been observed that the GNDVI yielded more accurate estimations of crop production at the early stage (5 weeks) of a crop’s growth cycle (Wahab et al., 2018). Consequently, great care must be taken in the selection of factors (color component and saturation indices), as this choice greatly influences the efficacy and precision of crop yield and quality estimates using spectral reflectance obtained at peak sites.
However, environmental factors and crop water stress are likely to hinder the performance reliability of spectral indices where NDVI (Normalized Difference Vegetation Index) and RENDVI (Red Edge Normalized Vegetation Difference Index), based on physiological behavior measured in lab conditions rather than actual meteorological variations under field environments for predicting wheat production accurately (Hassan et al., 2019). Consequently, forthcoming investigations will examine these elements within stress-controlled experiments to cultivate further understanding. Incorporating correction factors for quality and yield assessments may be seen as a potential approach to enhance the accuracy of spectrum consumption measurements in indices. The application of ML, namely artificial neural networks, to spectral bands has demonstrated potential (Sarkar et al., 2018). For instance, these protocols have successfully predicted the grain protein content of rice (Oryza sativa) and the total soluble solids content of grapes (Vitis). Relatively inexpensive color cameras have been utilized to capture color characteristics, proving to be a dependable method for generating accurate yield estimations (Kefauver et al., 2017). This approach offers a feasible alternative to utilizing more expensive sensors. However, previous investigations that have employed color cameras have yielded unsatisfactory outcomes (Bura et al., 2018). This could be attributed to the inadequate development of yield estimation protocols, or the studies conducted under unfavorable environmental conditions (Bura et al., 2018). In conclusion, numerous studies have been undertaken to estimate crop production (Zhou et al., 2017; Bura et al., 2018; Hassan et al., 2019; Zheng et al., 2019). However, numerous studies have shown the concerns regarding accuracy, reliability, and scalability for the analysis of the UAVs data for different crop phonemics based studies.
1.1.1 Accuracy
1.1.1.1 Sensor restrictions
The UAVs usually have thermal, multispectral, or hyperspectral sensors (Fei et al., 2023). Even though these sensors can record much information about crops, they might not be precise enough for analyzing individual cereal crops, especially in environments with varying lighting and weather conditions (Lee et al., 2010).
1.1.1.2 Weather and flight stability
Cloud cover, wind, and fluctuating sunlight are external elements that might add inaccuracies into sensor data (Aasen et al., 2018), impacting YP’s accuracy.
1.1.1.3 Data quality
UAVs gather information at different speeds and altitudes, which could cause inconsistencies in the resolution and quality of the data collected from the sensors (Pádua et al., 2017). YP and analyses that rely on this ambiguity may be imperfect.
1.1.2 Reliability
UAVs express difficulty flying in unstable air and are vulnerable to bad weather like wind, rain, and fog, which can delay data collection and even equipment damage (Ubina and Cheng, 2022). This unpredictability may make YP models less reliable (Müller et al., 2016).
1.1.2.1 Data processing and integration
UAVs produce massive amounts of data that must be processed correctly to conduct real-time analyses (Guimarães et al., 2020). Because of inherent variances in data structure and format, integrating UAV data with other data sources, including weather, soil, and ground truth measures is not easy (Samaras et al., 2019).
1.1.3 Scalability
1.1.3.1 Field coverage
UAVs have trouble collecting data at scale due to their short flight times and short battery lives, which limits their capacity to survey vast cereal crop fields in a single flight (Mohsan et al., 2023).
1.1.3.2 Data storage, integration and management
For analyses that span several growing seasons or include long-term monitoring, it is necessary to have a robust infrastructure to store and manage massive datasets acquired by UAVs (Nabwire et al., 2021). When applied to new locations with varied soil types, temperatures, and crop types, ML models built on data from one field or area might not perform as well as when trained on data from another (Benos et al., 2021). Hence, it limits the models’ ability to be applied at various agricultural scales.
1.1.4 Addressing challenges
Diverse methods can be considered to overcome these previously mentioned difficulties.
1.1.4.1 Sensor calibration and data fusion
The application of advanced filtering techniques and regularly calibrating sensors can help to increase the quality of data (Concas et al., 2021). A more complete picture of crop conditions and improved yield projections can be achieved through data fusion and integration (Ahmad et al., 2022), which involves combining data from UAV with data from other sources (such as satellite imaging and ground-based sensors).
1.1.4.2 Hybrid machine learning models
By integrating ML with more conventional statistical approaches, scientists can create more robust models and generalize to new datasets (Elavarasan et al., 2018).
1.1.4.3 Improved technology for UAV
Longer flight times and more automated flight controls are just two examples of how UAV technology is constantly evolving to better cover more ground and collect more data (Chaurasia and Mohindru, 2021).
1.1.4.4 Data management solutions with cloud computing
Using the cloud to store and analyses data can solve scalability problems and make data analysis more effective (Delgado et al., 2019). The background reveals proof of adaptation of the multiple approaches for YP in different cereal crops.
This study employs UAVs for YP and provides a bibliometric analysis of existing research conducted at WMRS crops. The WOSCC extracted pertinent scientific information published between 2005 and 2023 in high caliber publications. VOSviewer was used for co-occurrence, co-citation, and co-authorship analyses. The following are the goals of the current study: (a) To establish a knowledge structure for WMRS crops using UAVs for the YP (b) To evaluate the use of ML, different sensors and limitations for WMRS crops. (c) To identify the knowledge gaps and research frontiers for YP in WMRS crops employing UAVs. The article’s structure includes an introduction to WMRS crops and their importance, publication collection, a description of the results of the bibliometric analysis, review on WMRS crops, constraints and prospects for future study, and contribution of the current study for the scientific community.
2 Retrieval of publications’ information and outline for bibliometric methods
2.1 Publications’ information retrieval from web of science
The most reliable literature-indexing site is the Web of Science (WOS), which includes scientific, social, health, and economic information. Therefore, it is acknowledged that the global WOS is the ideal source for gathering data for bibliometric analysis (Chen and Liu, 2020). The pertinent information was gathered from the WOS core collection (WOSCC) databases. Many iterations were employed to find the best keyword search code to download the most pertinent RS-related publications for YP in WMRS crops utilizing UAVs. Table 1A shows a list of more specific keyword codes that can be used to probe the WOS database systematically. The most useful search keywords were as follows: (“UAV” OR “multispectral” OR “hyperspectral” OR “RGB”) (Topic) and (“yield prediction” OR “yield estimation”) (Topic) and (“maize” OR “rice” OR “wheat” OR “soybean”). Be noted that the published articles were examined for the presence of words within their titles, abstracts, or keywords. All searched documents were validated for relevant material, and manually irrelevant articles were removed. The extraction process involved selecting solely peer-reviewed, original research publications that were published in the English language. The data acquiring period spanned from January 1, 2000, to August 3, 2023 (Table 1B), and the relevant scholarly material and the scope of inquiry were restricted to science and technology.
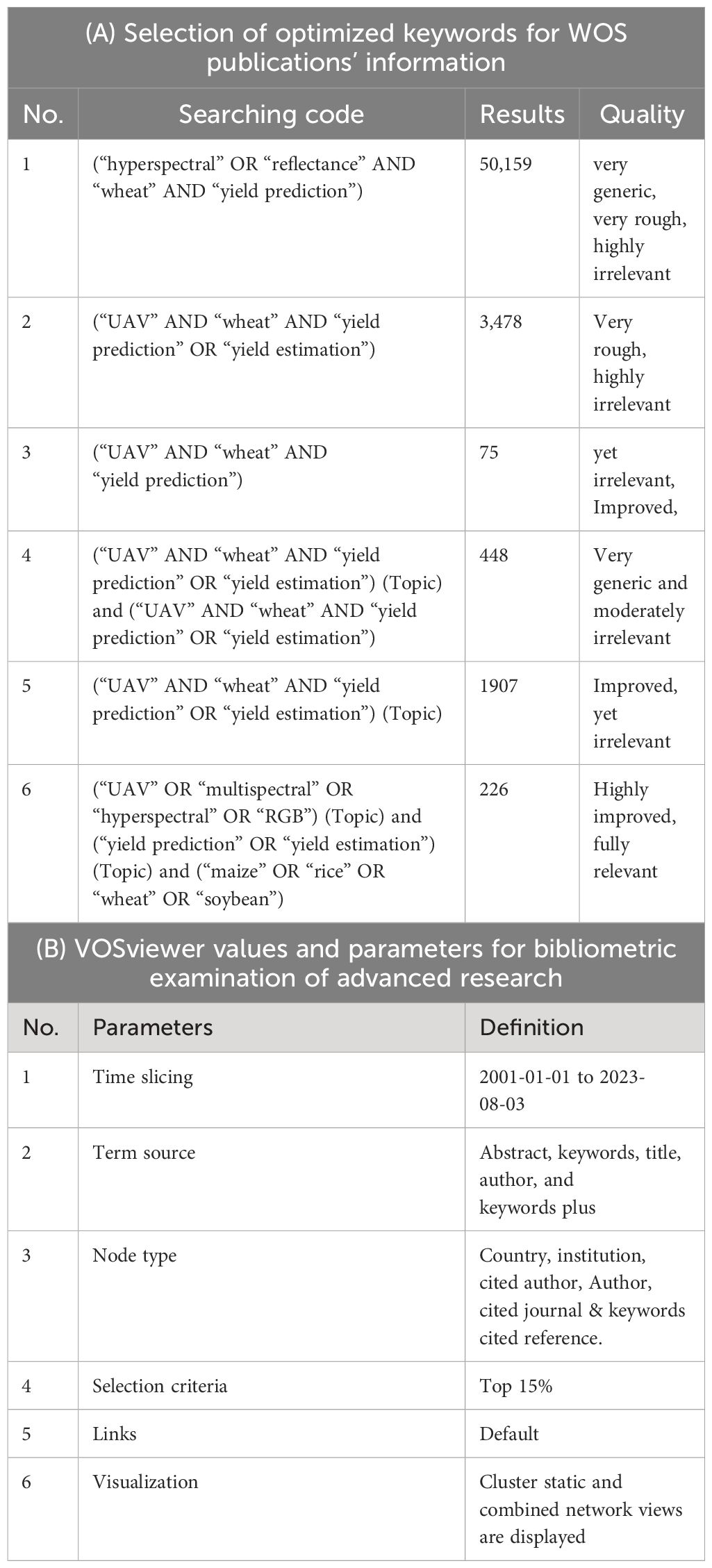
Table 1 Selection of optimized keywords for WOS publications’ information, and VOSviewer values and parameters for bibliometric examination for yield prediction in WMRS crops utilizing UAVs.
2.2 Work flow for the study
An overall number of 226 original research publications were retrieved using the methods described above. The entire document and all referenced materials were saved as “Tab-delimited” as the preferred file type. The schematic shows the actions performed to carry out the investigation in Figure 2.
2.3 VOSviewer based bibliometric analysis
A map or network analysis and the visualization of scientific literature are examples of more advanced bibliometric analysis skills. Nees Jan van Eck and Ludo Waltman developed a robust program called VOSviewer (Version 1.6.18) for investigating and visualizing bibliometric networks (Van Eck and Waltman, 2010). It makes it possible to do analyses such as co-citation analysis, cluster analysis, and bibliometric mapping, all of which highlight the current research collaborations and patterns. The scientific community relies heavily on VOSviewer to visualize and project their data. The scientific community can learn more and get the program at http://www.vosviewer.com.
As per the specified parameters, utilizing VOSviewer facilitated the execution of co-citation analysis and keywords co-occurrence analysis. These analyses generated networks visually representing the co-citations seen among authors, documents, journals, and keywords. Furthermore, these networks provided insights into prominent study themes and emerging areas of investigation. In conclusion, the relevant data and mapping networks were thoroughly analyzed, and the results of the visualization inquiry in the present research study were presented and discussed.
3 Interpretation and discussion of the bibliometric results
3.1 Publications’ information analyses retrieved from web of science
The distribution of citations and matching publications in the domain of YP using RS in WMRS crops utilizing UAVs throughout the years 2005-01-01 to 2023-08-03 is shown in Figure 3A. It can be seen that over the first ten years (from 2005 to 2011), there was a prominently gradual increase in the number of notable publications. In 2012-2014 there was a decrease in publications. However, from 2014 to onward, there is a continuous and gradual increase in publications. In total, 44 research were published in 2022, which is the highest. Of the whole, 60.25% of the total publications are published from 2019-2022, 19.89% only in 2022, while 2023 has contributed 10.52% so far. The concerns about the research dramatically increased starting from 2019 to onward. This can be related to shifting funding preferences or research priorities during that time. The number of citations for publications remains gradually growing, while it has shown a fall in 2023, which might be due to the incompletion of this year. The highest citations (2291) have been reported in 2022, reflecting the impact of the research in this field. This upsurge could be attributed to developments in ML and UAV technology, which facilitate the conduct of creative and significant research.
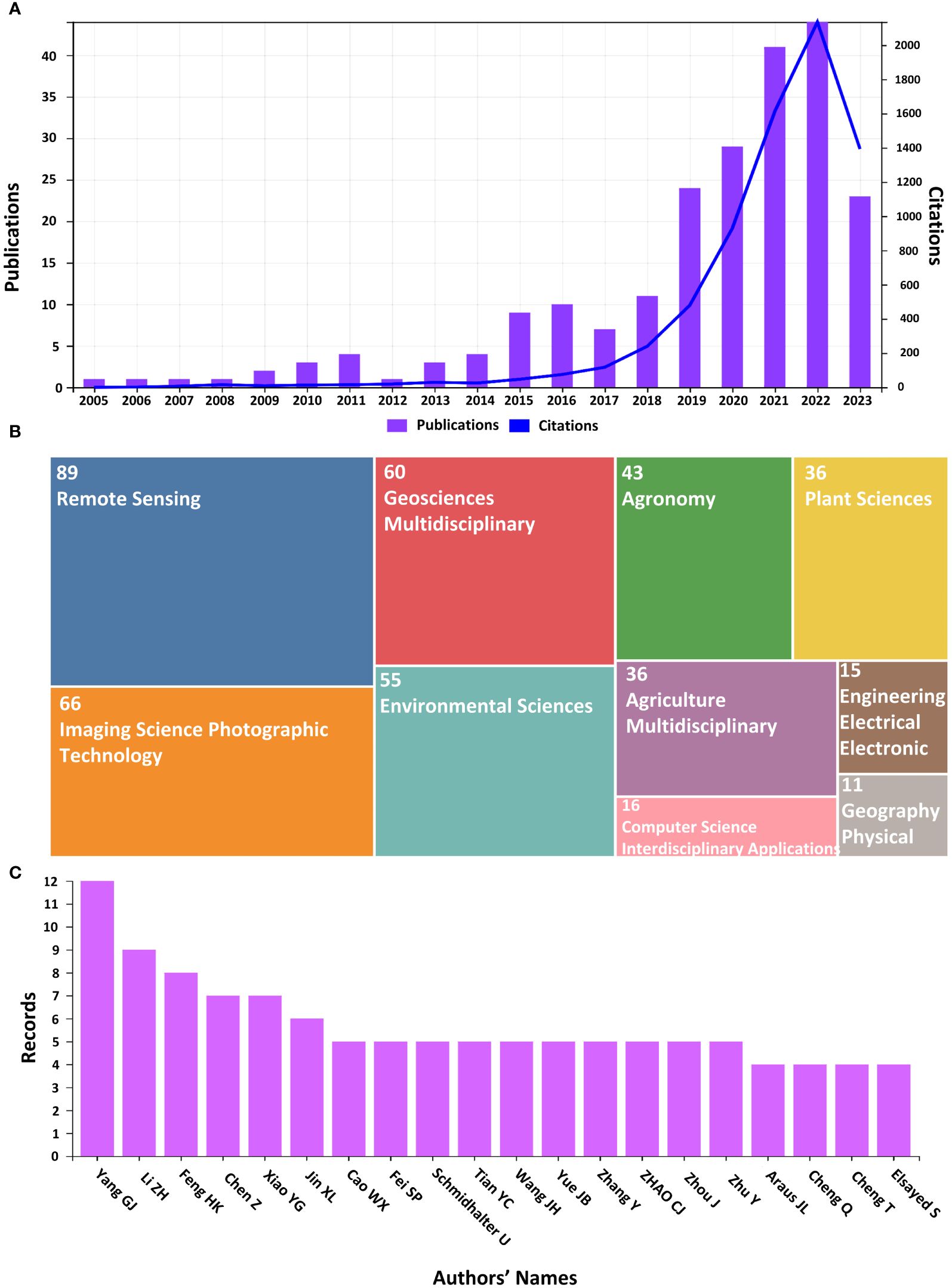
Figure 3 (A) Illustrates the number of publications and citations, (B) Number of publications according to subject categories, (C) Number of records for different authors.
The WOS based subject category area (Figure 3B) shows the publication distribution in the top 10 scientific study areas. Where “Remote Sensing” is leading than all others, having 39.04% (89 Articles) of total articles. While Agriculture, Imaging Science, Photographic Technology, Geosciences Multidisciplinary, Environmental Sciences, Agronomy and Plant Sciences share 89, 66, 60, 55, 43 and 38 publications, respectively. This diversity draws attention to the research’s interdisciplinary nature. The more influenced 15 authors for the YP in WMRS crops utilizing UAVs are ranked in Figure 3C where Yang GJ ranked at top with the maximum number of records in the acquired publications’ dataset. Given their prominence, it appears that they have given this field of study a lot of attention in their work.
Table 2A lists the top 15 journals in which the most articles relevant to the research area of YP in WMRS crops utilizing UAVs are published, along with the number of publications and their percentage contribution. Table 2B lists the top 15 research institutions for YP in WMRS crops utilizing UAVs. Supplementary Tables S1, S2 indicate the 15 most important countries and prolific writers with the most studies in the specified research subject, respectively. Supplementary Table S3 shows the distribution of the top fifteen funding agencies involved in relevant publications collected from the WOS in YP research utilizing UAVs. This systematic methodology provides insightful information and enables researchers to narrow down areas of potential studies for future.
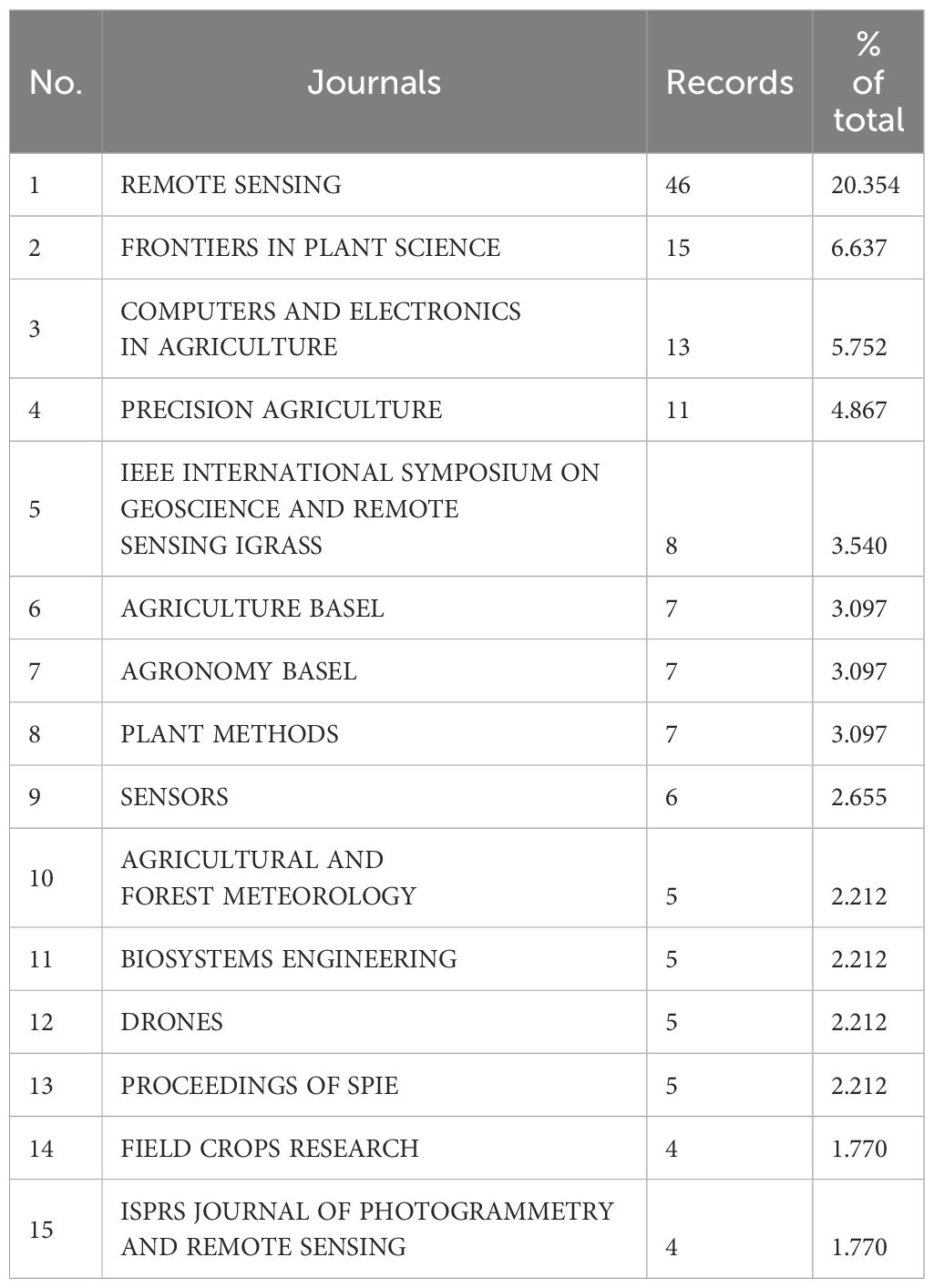
Table 2A The 15 best journals for publishing research on yield prediction in WMRS crops utilizing UAVs.
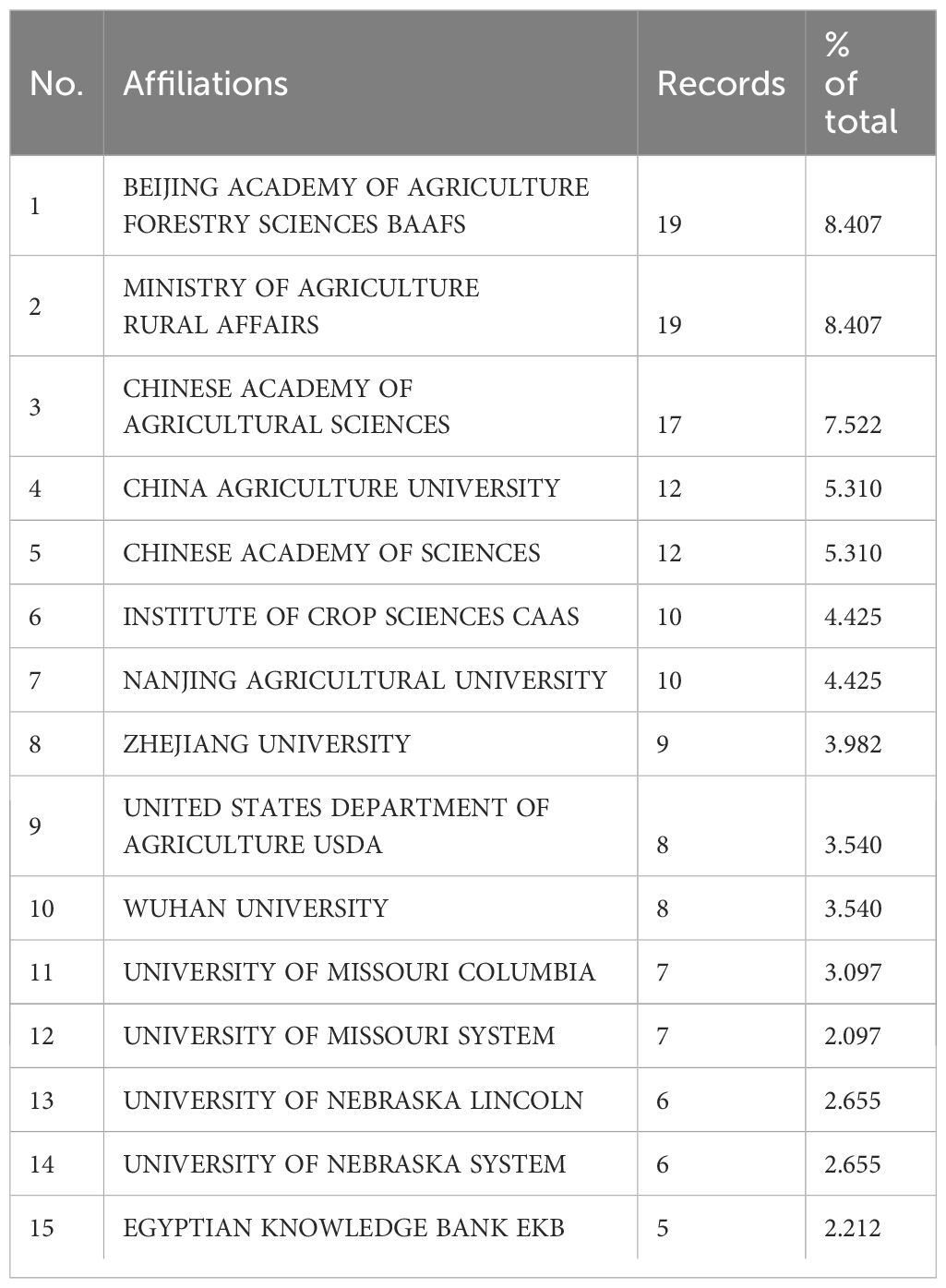
Table 2B The 15 best institutions for publishing research on yield prediction in WMRS crops utilizing UAVs.
3.2 Citations’ analyses
When a third author or document cites two or more authors or documents simultaneously, this is called a co-citation link (Chang et al., 2015). VOSviewer uses three main types of co-citation analyses to show how documents, writers who cite each other, and journals are connected and how they map to each other. Co-citation analysis is a good way to determine how many connections between journals, authors, and papers. It builds a mapping framework and tracks how scientific research areas change over time (Behrend and Eulerich, 2019).
3.2.1 Co-citation analysis for documents
The documents or articles are the most important parts of the knowledge repository or database in the area of predicting crop yields using UAVs for WMRS crops. Reference co-citation analysis, also called document co-citation analysis, is a good way to look at how a study area has grown and changed over time (Liao et al., 2018). After the scientometric study in VOS viewer was done, a network for displaying cited documents was made (Figure 4). Every node represents a reference or article cited, and the links between the nodes demonstrate how the mentioned references and articles relate to one another. Larger nodes represent more essential documents, and documents often referenced by other documents are close to each other. Figure 4 shows the most important works completed in this field are (Zhou et al., 2017) (Haboudane et al., 2004), and (Maimaitijiang et al., 2020).
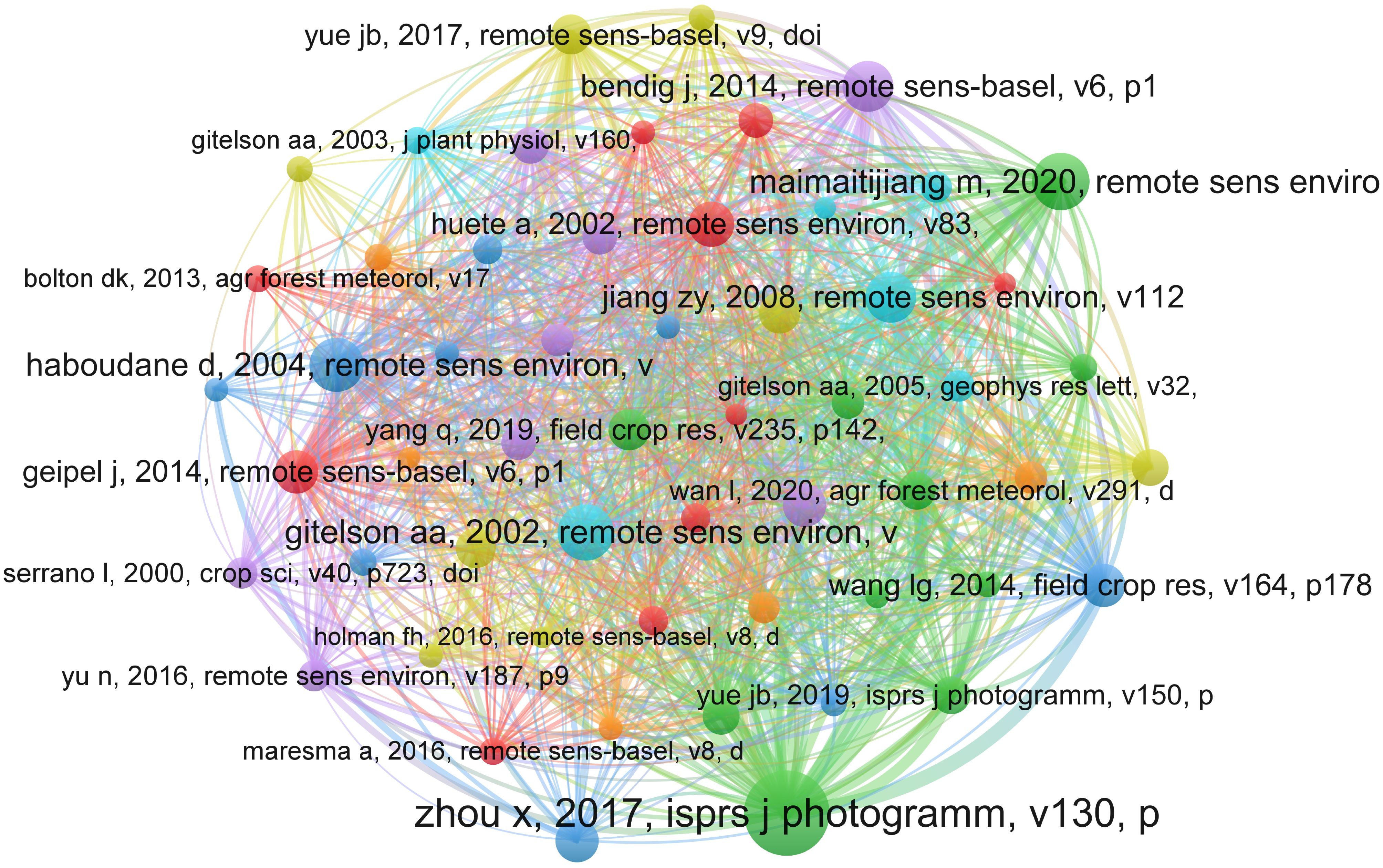
Figure 4 The document co-citation network visualization map for research on yield prediction in WMRS crops utilizing UAVs.
3.2.2 Co-citation analysis for authors
The distribution of authors with more citations in a particular field of study is also examined by the co-citation analysis for the author, which is used to identify the most productive writers in that subject. The co-citation analysis also makes the visualization of associated writers’ subject areas and research interests feasible. The visualization network that was produced after the author’s co-citation analysis of the study into YP in WMRS crops using UAVs is shown in Figure 5. The nodes represent authors, the connecting lines between two nodes indicate their relationship regarding co-citations. An author’s importance is increased when a node gets larger because that particular author makes more citations.
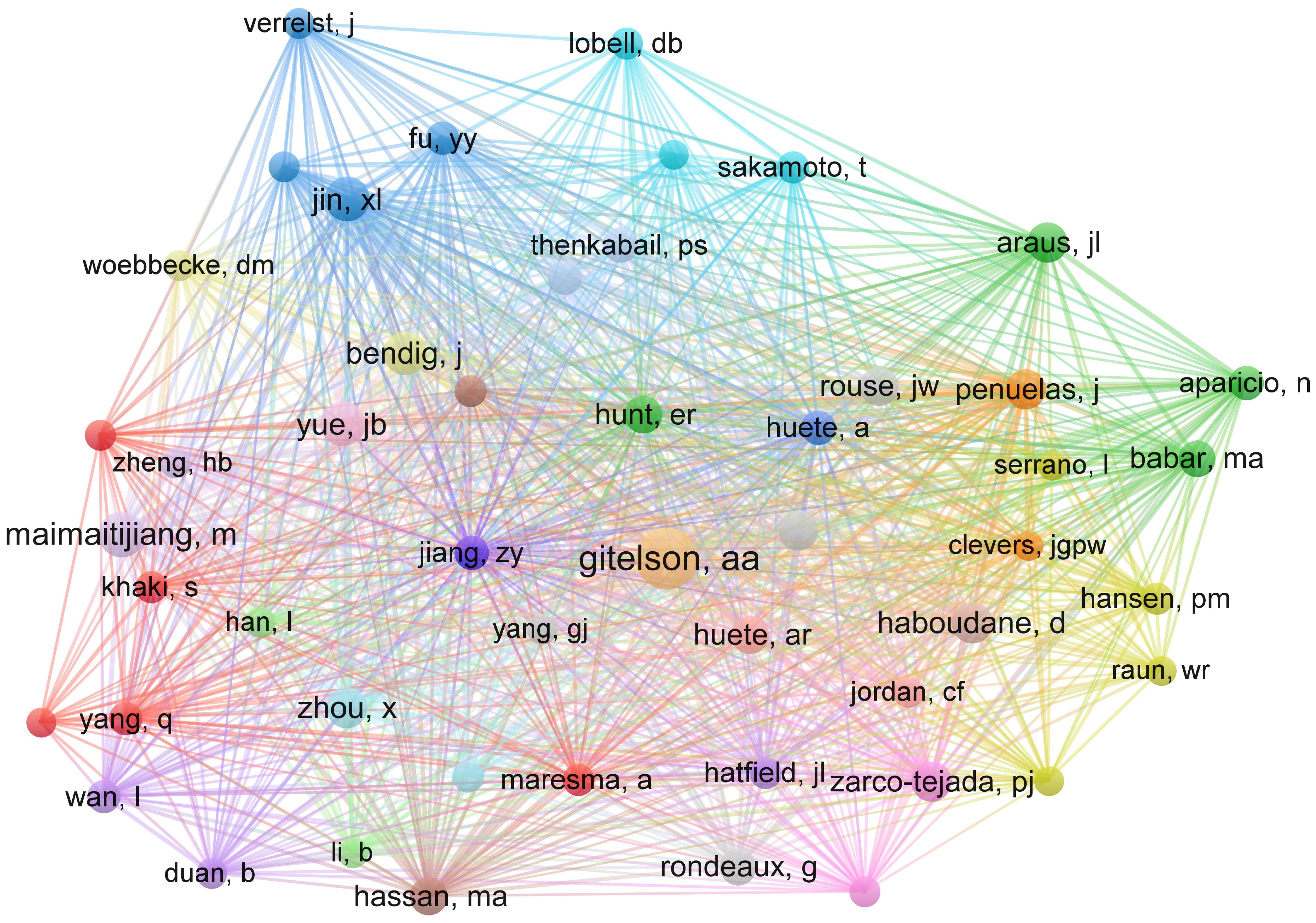
Figure 5 The authors’ co-citation network visualization map for research on yield prediction in WMRS crops utilizing UAVs.
Similarly, the distance between two successive nodes or writers is inversely associated with the amount that one author is cited by the other. The authors’ areas of interest will be more strongly correlated with the size of the distance between the nodes. Author co-authorship analysis confirms the extensive analysis of the visualization network, which shows that most authors collaborate to a very high degree. It offers vital insights into the organization and movement of a research field, aiding researchers in comprehending significant contributors, patterns, and possibilities for collaboration.
The 15 best most co-cited authors are listed in Table 3A along with their respective authors, counts of citations, years of citation counts, and rankings based on the times their scientific literature have been cited. According to the data, the aforementioned authors’ work made a significant contribution to the field of YP in WMRS crops utilizing UAVs, making them significant participators to the future growth of YP research. According to the findings, the authors (Zhou et al., 2017), (Maimaitijiang et al., 2020), and (Yue et al., 2019) were the most prolific in the research field,
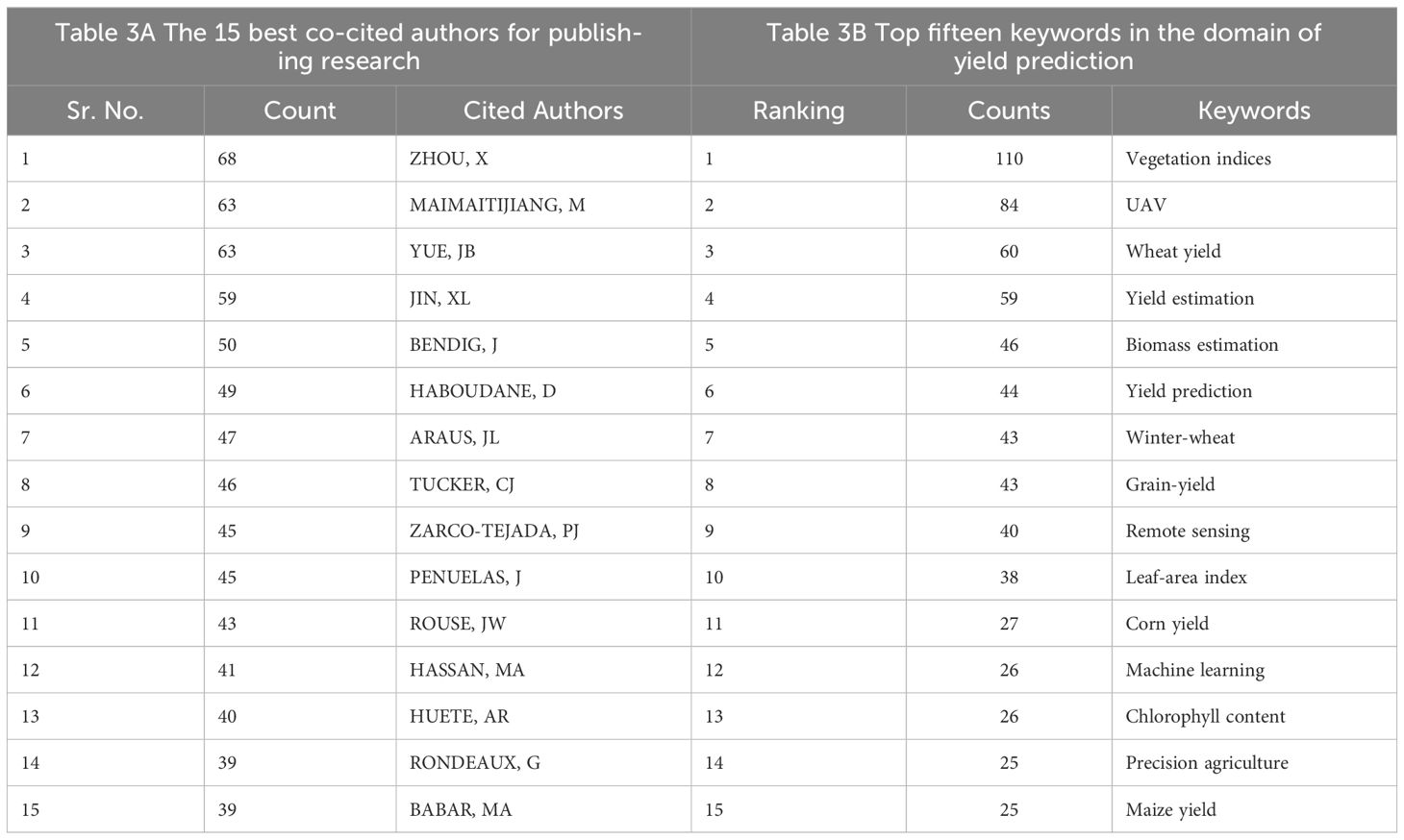
Table 3 The 15 best co-cited authors and top fifteen keywords for research on yield prediction in WMRS crops utilizing UAVs.
3.2.3 Citations analysis for documents
Citation analysis for authors represents a bibliometric approach employed to assess the impact and influence of an individual author’s scholarly output within the academic and relevant research field. This method entails comprehensive scrutiny of the frequency with which an author’s publications have garnered citations in the works of other researchers. Through the examination of citation patterns, this analytical method provides valuable insights into an author’s contributions to their field of study, the level of recognition their research receives, and the extent of their influence within the scholarly and academic community. Figure 6 visualizes the articles with more than 20 citations from different years. The size of nodes represents the highly cited documents, like (Chlingaryan et al., 2018), (Zhou et al., 2017), (Maes and Steppe, 2019), and (Shanahan et al., 2001) are most prominent with superior size of nodes.
3.3 Keywords’ co-occurrence analysis
In a scholarly context, keywords serve as descriptors that elucidate the precise subject matter or overarching category to which an article pertains. Additionally, they encapsulate the primary information summarized within research papers. In essence, keyword co-occurrence analysis can be employed to discern prevailing focal points and emerging research frontiers. The most rapidly increasing citation rates for individual keywords indicate perennially popular issues or promising avenues for future study. Figure 7 is a visual representation of the VOSviewer keyword co-occurrence analysis results. Nodes represent the keywords, and the size of the nodes represents the frequency with which they occur together.
Table 3B presents a ranking of the top fifteen keywords in the domain of YP in WMRS crops utilizing UAVs. These keywords have been ordered based on their occurrence frequency. The keywords demonstrating the most pronounced co-occurrence frequencies, along with their respective counts, include Vegetation indices (110 occurrences), UAV (84 occurrences), Wheat yield (60 occurrences), and Yield estimation (59 occurrences). Citation frequency analysis offers a concise insight into the prevalence of frequently employed keywords within a specified timeframe. This analysis allows for the temporal depiction of these terms, drawing attention to the time frame in which they were employed and referenced most frequently.
3.4 Co-authorship analysis
Cooperative analysis represents a multifaceted approach that delves into various tiers of investigation, spanning from the broad macro scale to the intricate micro level. Its objective is to unveil the intricate distribution patterns characterizing the collaborative dynamics within scientific research. In this specific context, the formidable tool of choice is VOSviewer, a sophisticated visualization software adept at illuminating the intricate web of collaborative relationships among institutions, countries/regions, and authors operating in the specialized domain of YP in WMRS crops utilizing UAVs. The calculation of the depth of collaborative involvement utilizes both fractional and full counting approaches, where full counting assigns equal weight to each participant.
Within the analytical framework of literature studies, two distinctive counting methodologies come into play: fractional and full counting. In the full counting mode, each contributor is endowed with an equal weight of 1, which is symmetrically distributed in quantifying the depth of their collaborative involvement. VOSviewer, as the computational engine, undertakes the intricate task of computing scores that gauge the interrelationships among knowledge units. Employing the precision of the association strength algorithm, it harmonizes and standardizes the raw data. The result concludes in creating an enlightening visualization map of the literature, encompassing distance-based and graph-based representations, thereby offering a comprehensive insight into the complex web of scholarly interactions.
3.4.1 Co-authorship analysis for authors
The quantification of an author’s productivity within a specific domain is a pivotal metric in assessing their impact within that particular field. The analysis of author collaboration serves as a valuable tool for investigating YP in WMRS crops utilizing UAVs and the associated social networks of cooperation. In Figure 8A, the study presents the author collaboration network within the domain of yield predictionYP in WMRS crops utilizing UAVs, focusing on authors who have contributed for at least two articles.
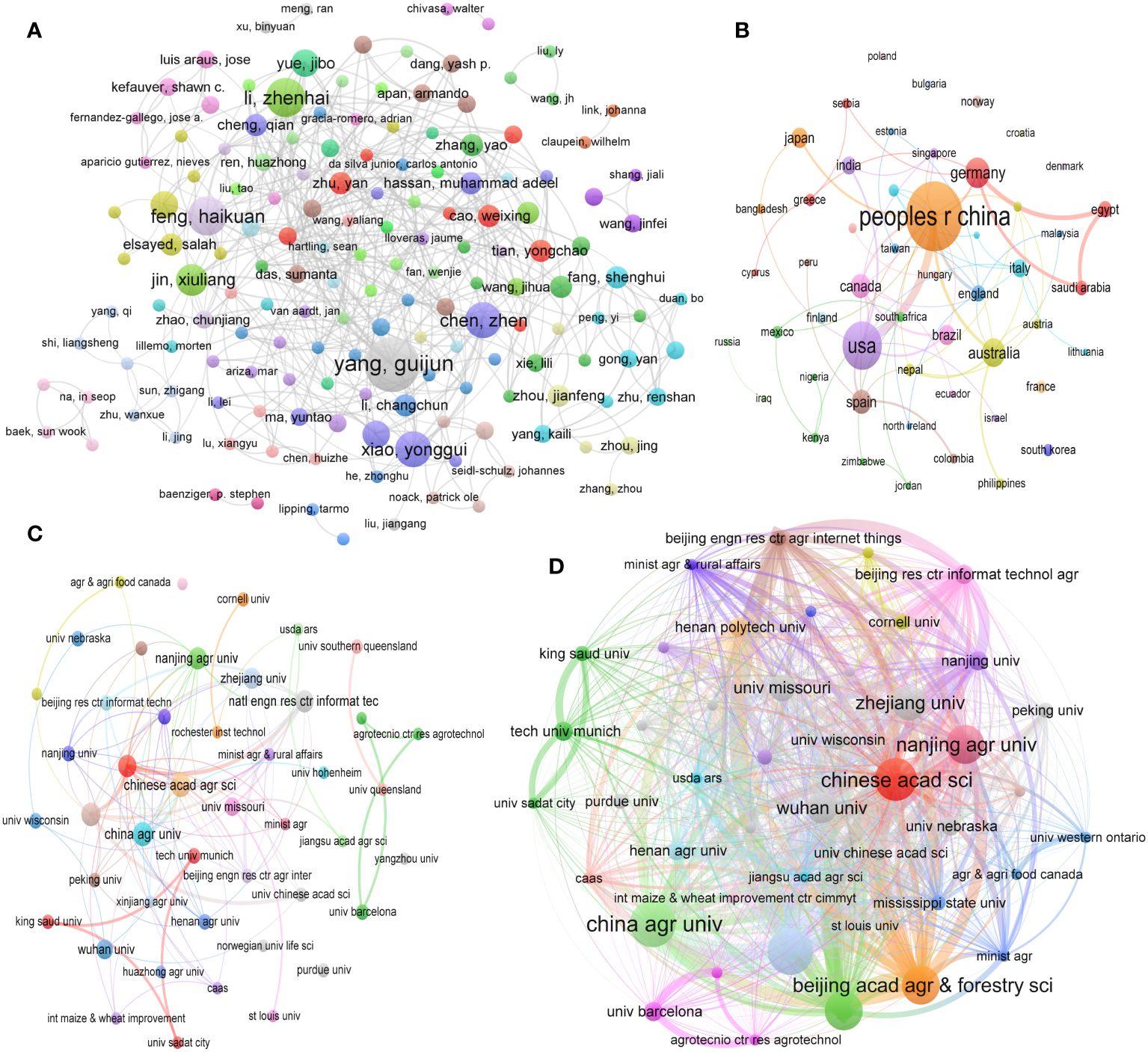
Figure 8 (A) Co-authorship analysis of authors, (B) Co-authorship analysis of regions/countries, (C) Co-authorship analysis of institutions, and (D) Coupling analysis of institutions for yield prediction in WMRS crops utilizing UAVs.
As depicted in Figure 8A, the collaboration landscape in this field exhibits a persistent spirit of cooperation and manifests a small-world effect. It is evident that each academic group maintains the capacity to engage in direct or indirect collaboration with other scientific research teams, illustrating the continuous transmission of information within the network. Notably, within specific clusters, the trajectory of YP in WMRS crops utilizing UAVs is influenced by high-impact authors, such as Yang Guijun, Li Zhenhai, Feng Haikuan, Chen Zhen, Xiao Yonggui and several others (Figure 8A).
3.4.2 Co- authorship analysis for regions/countries
Figure 8B visually represents international cooperation among countries and regions over time, incorporating dynamic elements. In this visualization, the size and color of nodes correspond to the volume of research documents produced and the average publication year within each respective country or region. Furthermore, the thickness of the connecting lines between nodes indicates the level of collaboration between these entities, with thicker links representing more significant cooperation. By analyzing Figure 8B, it is evident that China and the United States are the leading countries in deploying UAVs for YP in WMRS crops. Countries such as Germany, Australia, Spain, Japan, Saudi Arabia and Canada have also actively engaged in this field of research.
It is worth highlighting that China, owing to its remarkable research productivity, has forged robust collaborative ties with Unites States for YP in WMRS crops utilizing UAVs. Looking ahead, both the China and United States are poised to emerge as principal players in the field of RS. Progress in advancing RS for YP in these two nations is of global significance, given the far-reaching benefits it brings. The advancements and collaboration between China and the United States, the two greatest developed and emerging nations in the world, significantly impact the Asia-Pacific region and the global scene. Motivated by same goals including guaranteeing food security and reducing the impact of sustainable crop production, both countries have agreed to make use of crop production innovations.
3.4.3 Co-authorship analysis for institutions
The examination of institutional cooperation yields valuable insights into organizations and groups that make significant contributions within a particular field. This analysis serves as a foundational step towards fostering enhanced future collaboration for organizations with 3 documents as co-authorship. To effectively depict the distribution of organizations and their collaborative relationships, this study employs VOSviewer, a visualization tool, to represent the network of institutional collaboration within the domain of RS for YP in WMRS crops utilizing UAVs, as presented in Figure 8C.
Each node’s size in the below illustration represents the total number of scholarly articles published by that institution. The thickness of the connecting lines represents the degree of collaboration between universities. Nodes sharing the same color signify a higher level of cooperation than nodes with distinct colors. This analysis reveals notable collaborative connections among universities and colleges. For instance, the Chinese Academy of Sciences with China Agriculture University, and Nanjing Agricultural University with Zhejiang University exhibit a pronounced cooperative association, as evidenced by their placement within the red and green clusters. Additionally, in this study, we observe a strong collaborative relationship between King Saud University and the University of Sadat within the red cluster. Several other clusters have also merged around productive institutions, thereby contributing to forming a diversified and expansive cooperation network within the landscape of RS for YP in WMRS crops utilizing UAVs.
3.4.4 Coupling analysis for organizations
Organizations’ coupling analysis is a sophisticated bibliometric method used to examine the interconnections and relationships between different organizations or institutions within the context of scientific research and publication activities. It primarily focuses on quantifying and understanding the collaborative patterns and knowledge exchange dynamics among these entities, often in the context of specific research fields or disciplines. The insights gained from organizational coupling analysis can inform policymakers, funding agencies, and researchers about the structure and dynamics of collaborative networks in specific research domains. This information is valuable for fostering interdisciplinary collaboration, optimizing research investments, and advancing scientific progress by promoting effective knowledge exchange among organizations. The organizations with a minimum 3 documents together are illustrated in Figure 8D, Chinese Academy, Nanjing Agricultural University, China Agricultural University, and Beijing Academy of Agriculture and Forestry Sciences are more prominent in respective clusters and have shown a prominent connection in connecting lines and node size. By highlighting collaborative networks and influencing choices on research investments and interdisciplinary collaboration, the results of this analysis can direct researchers, politicians, and funding agencies.
4 Recent studies on WMRS crops for UAV-based yield prediction
In agricultural research, precise early YP is paramount for individual farmers and the broader agricultural sector. UAVs have demonstrated commendable efficacy in enhancing YP accuracy through the utilization of various data sources (Hassan et al., 2019; Zheng et al., 2019; Kumar et al., 2023). For instance, such accuracy has been achieved by harnessing metrics such as RGB-derived plant height and canopy cover (Chu et al., 2016), VIs (Gracia-Romero et al., 2017), and multispectral imagery (Kyratzis et al., 2017; Zheng et al., 2019; Su et al., 2023). It is worth noting that the temporal dimension plays a pivotal role in optimizing YPs, with multitemporal VIs, including those accumulated throughout the crop’s growing season, exhibiting superior performance compared to single measurements (Zhou et al., 2017).The investigations conducted using UAVs for YP have predominantly adjusted on experimental fields characterized by substantial variations in final yield due to factors such as nitrogen levels (Zhou et al., 2017), phosphorous concentrations (Gracia-Romero et al., 2017), or irrigation practices (Vega et al., 2015). However, it remains imperative to scrutinize the efficacy of these methods within the context of precision agriculture conditions, where variations are predominantly driven by edaphic and microclimatic factors and are comparatively less extreme. Furthermore, it is crucial to acknowledge that prevailing UAV-based YP studies have primarily revolved around developing empirical regression models. While these models serve the purpose of extrapolating yield estimates to encompass entire fields, it is imperative to recognize their inherent limitation – regression coefficients derived from one year’s data may not be transferrable to subsequent years at the same location, nor to different locations within the same year (Rembold et al., 2013).An alternative approach in this domain involves the estimation of yield based on crop growth models. For instance, the GRAMI growth model was successfully applied to rice YP using UAVs GRAMI growth model utilizing UAV-derived data (Kim et al., 2017). Nevertheless, it remains evident that substantial research endeavors are indispensable to delineate the optimal sensor configurations, flight timing, and refinement of crop growth models to harness UAVs information most effectively and reliably for YP purposes. Numerous studies conducted since 2020 are shown in Table 4 as a summary for YP in WMRS crops utilizing UAVs.
4.1 Utilization of machine learning techniques and factors influencing yield prediction
Various methodologies and techniques have been employed in previous findings for YP. Table 4 provides details of the ML approaches applied in recent studies. The RF technique is widely employed in the domain of YP in WMRS crops, as it is a commonly utilized method in technical approaches. The studies have used numerous techniques, i.e. SVM, KNN, PCA, LR, MLR, MMDL, RR, ANN, PLSR DCN, etc. SVM can be used with statistical techniques like ANOVA to estimate agricultural yield (Li et al., 2018). In wheat crop SVM manifested R2 = 0.93 using RGB images acquired by UAVs (Wang et al., 2021a). Another study established the R2 = 0.87 for wheat crops through MS images by UAVs calculating WIs and VIs using the LRM technique (Song et al., 2021). The MLR showed R2 = 0.81 for wheat crop (Han et al., 2021) and R2 = 0.95 for rice crop through MS images (Perros et al., 2021). Accordingly, different ML techniques have shown interesting results using raw image data acquired using UAVs (Table 4). The details of the abbreviations is given in Table 5.
The authors give similar importance to each factor for inclusion into the ML model (Zhang et al., 2019; Mustafa et al., 2022b). Osco et al. (2020) consider the significance of several elements like meteorological conditions, geographic location, and radiometric calibration. Each location’s meteorological variables (daily daylight hours, daily solar radiation, daily temperature sum, and daily wind speed) were employed, along with field-specific rainfall data. They found that the number of neural layers and the learning rate of an ANN affect the prediction. As a result, the prediction model performs worse when too many or too few parameters are added (Adisa et al., 2019). The conclusion highlights the significance of neural networks in creating prediction models. On the other hand, by including irrelevant features to the model, its complexity may increase, leading to a drop in prediction accuracy concerning time complexity and irrelevant results (Kanning et al., 2018). The study Eugenio et al. (2020) indicates that the UAV is the most effective method for gathering image data from the location. They performed their experiment accounting for distinct weather scenarios. Overall performance shows the accuracy of the model, although this study has indicated that the inclusion of either an excessive number or a small number of factors causes data sparsity. The main problem this study highlighted is that they employ constant weather conditions for every season. Because of this, a realistic strategy is required, considering the various weather-related influencing factors throughout the year. Peng et al. (2019) has mentioned that ML technology is typically used in precision agriculture to leverage the vast amounts of collected data. ML can estimate certain crop growth rate-related metrics, identify and differentiate objects in photos, and even detect diseases. To take advantage of the data, ML techniques such as CNN, ANN, regression modeling, RF, and deep learning have been employed (Islam et al., 2018). The use of ML has grown dramatically in recent years, partly because deep learning is developing at a rapid pace (Guo et al., 2019; Mustafa et al., 2022a, 2022; Hussain et al., 2023; Mustafa et al., 2024).
4.2 Emerging trends and the current research landscape in the domain of yield prediction in WMRS crops utilizing UAVs
Some of this study’s most substantial implications are as follows:
● Regarding YP in WMRS crops utilizing UAVs research, Zhou X, Maimaitijiang M, Yue JB, Jin XL, Bendig J, Haboudane D, and other writers are the most productive at the micro level. Researchers like Shanahan et al. (2001); Chlingaryan et al. (2018); Maes and Steppe (2019); Zheng et al. (2019), and others have been referenced extensively in YP for WMRS crops utilizing UAVs.
● Beijing Academy of Agriculture Forestry Science, Ministry of Agriculture Rural Affairs, Chinese Academy of Agricultural Sciences and China Agriculture University are the most dynamic and productive research for YP in WMRS crops utilizing UAVs at the meso scale.
● China, the United States, Germany, Australia, Canada, Spain, and Brazil are the most active and productive contributors to YP in WMRS crops using UAVs at the macro level. Compared to the other countries on the list, China and the United States presumably have more publications because their governments provide more extensive financial support policies to the scientific community.
● The National Natural Science Fund of China, the National Key Research and Development Program of China, the National Science Foundation, the Fundamental Research Funds for The Central Universities, and others have provided the bulk of the funding for YP in WMRS crops using UAVs.
● Precision Agriculture, Computers and Electronics in Agriculture, Remote Sensing, and Frontiers in Plant Science supported the main journals most.
● Although promising progress has been made in predicting crop yields with WMRS employing UAVs, more definitive results are urgently needed.
These findings provide important insight into the state of research and development in YP in WMRS crops using UAVs, and they highlight the most recent advancements in the field.
5 Constraints and prospects for the future
Despite the extensive research performed on YP of WMRS crops using RS, a definitive technique or technological configuration can still be universally applied. Due to variations in ML approaches, the selected or retrieved specific features such as vegetation indices, color, and spectral properties exhibit fluctuations (Zheng et al., 2019; Baio et al., 2022). Table 4 displays the suitability and chaos of several algorithms. As a result, the primary future vision is to concentrate on certain methodology that can be generalized for accurate quantification and YP.
Examining complex interactions among many environmental conditions will enable more precise and real-time crop YPs (Jung et al., 2021; Mia et al., 2023). A more thorough dataset for enhancing prediction models can be produced by establishing data standards protocols and encouraging data exchange between farmers and researchers; collaboration can result in more accurate insights and forecasts (Wolfert et al., 2017). Additionally, efforts to make UAVs technology less expensive and sophisticated will enhance its use, which will be advantageous to small-scale farmers. While incentives and subsidies provided by the government may be helpful. Crop YP and agriculture management benefit from deep and ML. These new algorithms can more effectively analyze complicated environmental interactions and massive datasets than older methods, resulting in more accurate and real-time yield estimates. Deep learning algorithms can identify complex data patterns for better predictions and insights (Maimaitijiang et al., 2020; Bellis et al., 2022; Mia et al., 2023; Yu et al., 2023).
Additionally, improvements in sensor technology (hyperspectral and LiDAR sensors) can offer even more precise and detailed information, enabling crop health and yield potential (Maes and Steppe, 2019). Also, it will be necessary to push for simplified laws that balance safety issues and the potential advantages of UAVs technology in agriculture. This may encourage a broader uptake of UAVs (McCarthy et al., 2023). Most significantly, maximizing the effectiveness of UAVs solutions for certain crops and areas, customized sensors and data analysis methods can give farmers and researchers more pertinent information.
6 Contribution of the study for the scientific community
An in-depth scientometric analysis of academic literature from 2001 to 2023 highlights research trends, boundaries and institutional relationships. The study identifies critical gaps, such as reliance on English-language articles and the need for transferable culture-specific features between sensors and algorithms. It proposes standardized data protocols for consistency and comparability, advanced hybrid models combining multiple machine learning (ML) algorithms and remote sensing (RS) techniques, and real-time monitoring systems using machine learning and deep learning to gain immediate insights. In addition, it recommends integrating advanced sensors such as hyperspectral and LiDAR with UAVs, promoting collaboration and data sharing between stakeholders, and advocating balanced government incentives and regulations for UAVs. These contributions aim to mitigate current limitations, ensure more accurate, reliable and widely applicable yield forecasts, and ultimately improve global agricultural productivity.
7 Conclusion
This study conducted a scientometric analysis of the academic literature pertaining to the use of unmanned aerial vehicles (UAVs) for yield prediction (YP) in Wheat, Maize, Rice, and Soybean (WMRS) crops. The retrieval of research published between 2001 and 2023 was conducted using co-citation, co-authorship, and co-occurrence analysis of phrases, utilizing the Web of Science (WOS) database. Research frontiers, trending issues, authors, innovative knowledge systems, and institutional relationships were all taken into account. Although the results of the present study’s graphical analysis of related papers are outstanding, the study has many shortcomings. Since the databases that make up the WOS core collection only include English-language articles, the resulting reference footprint is limited. Although remote sensing can predict yields with high accuracy, more research is needed, focusing on identifying transferable crop-specific traits across sensors and algorithms. This would make it possible to estimate yields more precisely and consistently, enhancing crop management techniques, reducing financial losses, and guarantee food security. Thus, research must concentrate on extracting quantitative knowledge on various crop stages utilizing various data types to construct comprehensive decision support systems. These advancements in remote sensing and YP have the potential to increase agricultural productivity across the globe significantly. Moreover, application of the advanced hybrid models, data protocols and feature selection are appealing indicators in conjunction with ML and RS for WMRS crops’ yield estimation. Government incentives for farmers, real time monitoring using ML and deep learning, hyperspectral and Lidar sensors, and UAV regulations can enhance the YP investigations.
Data availability statement
The raw data supporting the conclusions of this article will be made available by the authors, without undue reservation.
Author contributions
GM: Writing – original draft, Writing – review & editing. IK: Formal analysis, Methodology, Writing – original draft. SH: Project administration, Validation, Writing – review & editing. YJ: Methodology, Software, Supervision, Writing – original draft. JL: Data curation, Formal analysis, Investigation, Validation, Writing – review & editing. SA: Resources, Visualization, Writing – review & editing. RO: Software, Validation, Supervision, Writing – original draft. YL: Supervision, Writing – review & editing.
Funding
The author(s) declare financial support was received for the research, authorship, and/or publication of this article. National Key Research and Development Program (2024YFE0103100) and National Natural Science Foundation of China: 3170474.
Acknowledgments
Thanks to all co-authors for best participation in literature review.
Conflict of interest
The authors declare that the research was conducted in the absence of any commercial or financial relationships that could be construed as a potential conflict of interest.
The reviewer HZ declared a past co-authorship with the author GM to the handling editor.
Publisher’s note
All claims expressed in this article are solely those of the authors and do not necessarily represent those of their affiliated organizations, or those of the publisher, the editors and the reviewers. Any product that may be evaluated in this article, or claim that may be made by its manufacturer, is not guaranteed or endorsed by the publisher.
Supplementary material
The Supplementary Material for this article can be found online at: https://www.frontiersin.org/articles/10.3389/fpls.2024.1401246/full#supplementary-material
References
Aasen, H., Honkavaara, E., Lucieer, A., Zarco-Tejada, P. J. (2018). Quantitative remote sensing at ultra-high resolution with UAV spectroscopy: a review of sensor technology, measurement procedures, and data correction workflows. Remote Sens. 10, 1091. doi: 10.3390/rs10071091
Adamsen, F., Coffelt, T., Nelson, J. M., Barnes, E. M., Rice, R. C. (2000). Method for using images from a color digital camera to estimate flower number. Crop Sci. 40, 704–709. doi: 10.2135/cropsci2000.403704x
Adewopo, J., Peter, H., Mohammed, I., Kamara, A., Craufurd, P., Vanlauwe, B. (2020). Can a combination of UAV-derived vegetation indices with biophysical variables improve yield variability assessment in smallholder farms? Agronomy 10, 1934. doi: 10.3390/agronomy10121934
Adisa, O. M., Botai, J. O., Adeola, A. M., Hassen, A., Botai, C. M., Darkey, D., et al. (2019). Application of artificial neural network for predicting maize production in South Africa. Sustainability 11, 1145. doi: 10.3390/su11041145
Ahmad, U., Nasirahmadi, A., Hensel, O., Marino, S. (2022). Technology and data fusion methods to enhance site-specific crop monitoring. Agronomy 12, 555. doi: 10.3390/agronomy12030555
Ahmed, A., Azam, A., Bhutta, M. M. A., Khan, F. A., Aslam, R., Tahir, Z. (2021). Discovering the technology evolution pathways for 3D printing (3DP) using bibliometric investigation and emerging applications of 3DP during COVID-19. Clean. Environ. Syst. 3, 100042. doi: 10.1016/j.cesys.2021.100042
Astor, T., Dayananda, S., Nautiyal, S., Wachendorf, M. (2020). Vegetable crop biomass estimation using hyperspectral and RGB 3D UAV data. Agronomy 10, 1600. doi: 10.3390/agronomy10101600
Azam, A., Ahmed, A., Wang, H., Wang, Y., Zhang, Z. (2021). Knowledge structure and research progress in wind power generation (WPG) from 2005 to 2020 using CiteSpace based scientometric analysis. J. Clean. Product. 295, 126496. doi: 10.1016/j.jclepro.2021.126496
Baio, F. H. R., Santana, D. C., Teodoro, L. P. R., de Oliveira, I. C., Gava, R., de Oliveira, J. L. G., et al. (2022). Maize yield prediction with machine learning, spectral variables and irrigation management. Remote Sens. 15, 79. doi: 10.3390/rs15010079
Ballester, C., Hornbuckle, J., Brinkhoff, J., Smith, J., Quayle, W. (2017). Assessment of in-season cotton nitrogen status and lint yield prediction from unmanned aerial system imagery. Remote Sens. 9, 1149. doi: 10.3390/rs9111149
Barnetson, J., Phinn, S., Scarth, P. (2020). Estimating plant pasture biomass and quality from UAV imaging across Queensland’s Rangelands. AgriEngineering 2, 523–543. doi: 10.3390/agriengineering2040035
Bascon, M. V., Nakata, T., Shibata, S., Takata, I., Kobayashi, N., Kato, Y., et al. (2022). Estimating yield-related traits using uav-derived multispectral images to improve rice grain yield prediction. Agriculture 12, 1141. doi: 10.3390/agriculture12081141
Behrend, J., Eulerich, M. (2019). The evolution of internal audit research: a bibliometric analysis of published documents, (1926–2016). Account. History Rev. 29, 103–139. doi: 10.1080/21552851.2019.1606721
Bellis, E. S., Hashem, A. A., Causey, J. L., Runkle, B. R., Moreno-García, B., Burns, B. W., et al. (2022). Detecting intra-field variation in rice yield with unmanned aerial vehicle imagery and deep learning. Front. Plant Sci. 13, 716506. doi: 10.3389/fpls.2022.716506
Benos, L., Tagarakis, A. C., Dolias, G., Berruto, R., Kateris, D., Bochtis, D. (2021). Machine learning in agriculture: A comprehensive updated review. Sensors 21, 3758. doi: 10.3390/s21113758
Bhandari, M., Baker, S., Rudd, J. C., Ibrahim, A. M., Chang, A., Xue, Q., et al. (2021). Assessing the effect of drought on winter wheat growth using unmanned aerial system (UAS)-based phenotyping. Remote Sens. 13, 1144. doi: 10.3390/rs13061144
Bian, C., Shi, H., Wu, S., Zhang, K., Wei, M., Zhao, Y., et al. (2022). Prediction of field-scale wheat yield using machine learning method and multi-spectral UAV data. Remote Sens. 14, 1474. doi: 10.3390/rs14061474
Boegh, E., Houborg, R., Bienkowski, J., Braban, C. F., Dalgaard, T., Van Dijk, N., et al. (2013). Remote sensing of LAI, chlorophyll and leaf nitrogen pools of crop-and grasslands in five European landscapes. Biogeosciences 10, 6279–6307. doi: 10.5194/bg-10-6279-2013
Bukowiecki, J., Rose, T., Kage, H. (2021). Sentinel-2 data for precision agriculture?—a uav-based assessment. Sensors 21, 2861. doi: 10.3390/s21082861
Bura, R. O., Apriyani, S. W., Ariwibawa, K., Adharian, E. (2018). “UAV application for oil palm harvest prediction.” in Journal of Physics: Conference Series, Volume 1130, 6th International Seminar of Aerospace Science and Technology 25–26 September 2018 Jakarta, Indonesia, 012001. doi: 10.1088/1742-6596/1130/1/012001
Chang, Y.-W., Huang, M.-H., Lin, C.-W. (2015). Evolution of research subjects in library and information science based on keyword, bibliographical coupling, and co-citation analyses. Scientometrics 105, 2071–2087. doi: 10.1007/s11192-015-1762-8
Chaurasia, R., Mohindru, V. (2021). Unmanned aerial vehicle (UAV): A comprehensive survey. Unmanned Aerial Vehicles Internet Things (IoT) Concepts Techn. Appl. 1–27. doi: 10.1002/9781119769170.ch1
Chen, X., Liu, Y. (2020). Visualization analysis of high-speed railway research based on CiteSpace. Trans. Policy 85, 1–17. doi: 10.1016/j.tranpol.2019.10.004
Chivasa, W., Mutanga, O., Burgueno, J. (2021). UAV-based high-throughput phenotyping to increase prediction and selection accuracy in maize varieties under artificial MSV inoculation. Comput. Electron. Agric. 184, 106128. doi: 10.1016/j.compag.2021.106128
Chlingaryan, A., Sukkarieh, S., Whelan, B. (2018). Machine learning approaches for crop yield prediction and nitrogen status estimation in precision agriculture: A review. Comput. Electron. Agric. 151, 61–69. doi: 10.1016/j.compag.2018.05.012
Chu, T., Chen, R., Landivar, J. A., Maeda, M. M., Yang, C., Starek, M. J. (2016). Cotton growth modeling and assessment using unmanned aircraft system visual-band imagery. J. Appl. Remote Sens. 10, 036018–036018. doi: 10.1117/1.JRS.10.036018
Concas, F., Mineraud, J., Lagerspetz, E., Varjonen, S., Liu, X., Puolamäki, K., et al. (2021). Low-cost outdoor air quality monitoring and sensor calibration: A survey and critical analysis. ACM Trans. Sensor Networks (TOSN) 17, 1–44. doi: 10.1145/3446005
Costa, L., McBreen, J., Ampatzidis, Y., Guo, J., Gahrooei, M. R., Babar, M. A. (2022). Using UAV-based hyperspectral imaging and functional regression to assist in predicting grain yield and related traits in wheat under heat-related stress environments for the purpose of stable yielding genotypes. Precis. Agric. 23, 622–642. doi: 10.1007/s11119-021-09852-5
Danilevicz, M. F., Bayer, P. E., Boussaid, F., Bennamoun, M., Edwards, D. (2021). Maize yield prediction at an early developmental stage using multispectral images and genotype data for preliminary hybrid selection. Remote Sens. 13, 3976. doi: 10.3390/rs13193976
Das, S., Christopher, J., Apan, A., Choudhury, M. R., Chapman, S., Menzies, N. W., et al. (2021). Evaluation of water status of wheat genotypes to aid prediction of yield on sodic soils using UAV-thermal imaging and machine learning. Agric. For. Meteorol. 307, 108477. doi: 10.1016/j.agrformet.2021.108477
Delgado, J. A., Short, N. M., Jr., Roberts, D. P., Vandenberg, B. (2019). Big data analysis for sustainable agriculture on a geospatial cloud framework. Front. Sustain. Food Syst. 3, 54. doi: 10.3389/fsufs.2019.00054
Dorj, U.-O., Lee, M., Yun, S. (2017). An yield estimation in citrus orchards via fruit detection and counting using image processing. Comput. Electron. Agric. 140, 103–112. doi: 10.1016/j.compag.2017.05.019
Duan, B., Fang, S., Gong, Y., Peng, Y., Wu, X., Zhu, R. (2021). Remote estimation of grain yield based on UAV data in different rice cultivars under contrasting climatic zone. Field Crops Res. 267, 108148. doi: 10.1016/j.fcr.2021.108148
Elavarasan, D., Vincent, D. R., Sharma, V., Zomaya, A. Y., Srinivasan, K. (2018). Forecasting yield by integrating agrarian factors and machine learning models: A survey. Comput. Electron. Agric. 155, 257–282. doi: 10.1016/j.compag.2018.10.024
Eugenio, F. C., Grohs, M., Venancio, L. P., Schuh, M., Bottega, E. L., Ruoso, R., et al. (2020). Estimation of soybean yield from machine learning techniques and multispectral RPAS imagery. Remote Sens. Applications: Soc. Environ. 20, 100397. doi: 10.1016/j.rsase.2020.100397
Fei, S., Hassan, M. A., He, Z., Chen, Z., Shu, M., Wang, J., et al. (2021a). Assessment of ensemble learning to predict wheat grain yield based on UAV-multispectral reflectance. Remote Sens. 13, 2338. doi: 10.3390/rs13122338
Fei, S., Hassan, M. A., Ma, Y., Shu, M., Cheng, Q., Li, Z., et al. (2021b). Entropy weight ensemble framework for yield prediction of winter wheat under different water stress treatments using unmanned aerial vehicle-based multispectral and thermal data. Front. Plant Sci. 12,730181. doi: 10.3389/fpls.2021.730181
Fei, S., Hassan, M. A., Xiao, Y., Su, X., Chen, Z., Cheng, Q., et al. (2023). UAV-based multi-sensor data fusion and machine learning algorithm for yield prediction in wheat. Precis. Agric. 24, 187–212. doi: 10.1007/s11119-022-09938-8
Feng, H., Tao, H., Fan, Y., Liu, Y., Li, Z., Yang, G., et al. (2022). Comparison of winter wheat yield estimation based on near-surface hyperspectral and UAV hyperspectral remote sensing data. Remote Sens. 14, 4158. doi: 10.3390/rs14174158
Fischer, R., Byerlee, D., Edmeades, G. (2014). Crop yields and global food security. ACIAR: Canberra ACT, 8–11. doi: 10.1093/erae/jbv034
Fu, Z., Jiang, J., Gao, Y., Krienke, B., Wang, M., Zhong, K., et al. (2020). Wheat growth monitoring and yield estimation based on multi-rotor unmanned aerial vehicle. Remote Sens. 12, 508. doi: 10.3390/rs12030508
Fu, Y., Yang, G., Song, X., Li, Z., Xu, X., Feng, H., et al. (2021). Improved estimation of winter wheat aboveground biomass using multiscale textures extracted from UAV-based digital images and hyperspectral feature analysis. Remote Sens. 13, 581. doi: 10.3390/rs13040581
García-Martínez, H., Flores-Magdaleno, H., Ascencio-Hernández, R., Khalil-Gardezi, A., Tijerina-Chávez, L., Mancilla-Villa, O. R., et al. (2020). Corn grain yield estimation from vegetation indices, canopy cover, plant density, and a neural network using multispectral and RGB images acquired with unmanned aerial vehicles. Agriculture 10, 277. doi: 10.3390/agriculture10070277
Ge, H., Ma, F., Li, Z., Du, C. (2021). Grain yield estimation in rice breeding using phenological data and vegetation indices derived from UAV images. Agronomy 11, 2439. doi: 10.3390/agronomy11122439
Gilliot, J.-M., Michelin, J., Hadjard, D., Houot, S. (2021). An accurate method for predicting spatial variability of maize yield from UAV-based plant height estimation: A tool for monitoring agronomic field experiments. Precis. Agric. 22, 897–921. doi: 10.1007/s11119-020-09764-w
Gracia-Romero, A., Kefauver, S. C., Vergara-Díaz, O., Zaman-Allah, M. A., Prasanna, B. M., Cairns, J. E., et al. (2017). Comparative performance of ground vs. aerially assessed RGB and multispectral indices for early-growth evaluation of maize performance under phosphorus fertilization. Front. Plant Sci. 8, 2004. doi: 10.3389/fpls.2017.02004
Gracia-Romero, A., Rufo, R., Gómez-Candón, D., Soriano, J. M., Bellvert, J., Yannam, V. R. R., et al. (2023). Improving in-season wheat yield prediction using remote sensing and additional agronomic traits as predictors. Front. Plant Sci. 14, 1063983. doi: 10.3389/fpls.2023.1063983
Graham, E. A., Yuen, E. M., Robertson, G. F., Kaiser, W. J., Hamilton, M. P., Rundel, P. (2009). Budburst and leaf area expansion measured with a novel mobile camera system and simple color thresholding. Environ. Exp. Bot. 65, 238–244. doi: 10.1016/j.envexpbot.2008.09.013
Guimarães, N., Pádua, L., Marques, P., Silva, N., Peres, E., Sousa, J. J. (2020). Forestry remote sensing from unmanned aerial vehicles: A review focusing on the data, processing and potentialities. Remote Sens. 12, 1046. doi: 10.3390/rs12061046
Guo, C., Tang, Y., Lu, J., Zhu, Y., Cao, W., Cheng, T., et al. (2019). Predicting wheat productivity: Integrating time series of vegetation indices into crop modeling via sequential assimilation. Agric. For. Meteorol. 272, 69–80. doi: 10.1016/j.agrformet.2019.01.023
Guo, Y., Wang, H., Wu, Z., Wang, S., Sun, H., Senthilnath, J., et al. (2020). Modified red blue vegetation index for chlorophyll estimation and yield prediction of maize from visible images captured by UAV. Sensors 20, 5055. doi: 10.3390/s20185055
Haboudane, D., Miller, J. R., Pattey, E., Zarco-Tejada, P. J., Strachan, I. B. (2004). Hyperspectral vegetation indices and novel algorithms for predicting green LAI of crop canopies: Modeling and validation in the context of precision agriculture. Remote Sens. Environ. 90, 337–352. doi: 10.1016/j.rse.2003.12.013
Han, X., Thomasson, J. A., Bagnall, G. C., Pugh, N. A., Horne, D. W., Rooney, W. L., et al. (2018). Measurement and calibration of plant-height from fixed-wing UAV images. Sensors 18, 4092. doi: 10.3390/s18124092
Han, X., Wei, Z., Chen, H., Zhang, B., Li, Y., Du, T. (2021). Inversion of winter wheat growth parameters and yield under different water treatments based on UAV multispectral remote sensing. Front. Plant Sci. 12, 609876. doi: 10.3389/fpls.2021.609876
Hassan, M. A., Yang, M., Rasheed, A., Yang, G., Reynolds, M., Xia, X., et al. (2019). A rapid monitoring of NDVI across the wheat growth cycle for grain yield prediction using a multi-spectral UAV platform. Plant Sci. 282, 95–103. doi: 10.1016/j.plantsci.2018.10.022
Herrero-Huerta, M., Rodriguez-Gonzalvez, P., Rainey, K. M. (2020). Yield prediction by machine learning from UAS-based multi-sensor data fusion in soybean. Plant Methods 16, 1–16. doi: 10.1186/s13007-020-00620-6
Herrmann, I., Bdolach, E., Montekyo, Y., Rachmilevitch, S., Townsend, P. A., Karnieli, A. (2020). Assessment of maize yield and phenology by drone-mounted superspectral camera. Precis. Agric. 21, 51–76. doi: 10.1007/s11119-019-09659-5
Hussain, S., Mustafa, G., Haider Khan, I., Liu, J., Chen, C., Hu, B., et al. (2023). Global trends and future directions in agricultural remote sensing for wheat scab detection: insights from a bibliometric analysis. Remote Sens. 15, 3431. doi: 10.3390/rs15133431
Islam, T., Chisty, T. A., Chakrabarty, A. (2018). “A deep neural network approach for crop selection and yield prediction in Bangladesh,” in 2018 IEEE Region 10 Humanitarian Technology Conference (R10-HTC), Malambe, Sri Lanka, 1–6. doi: 10.1109/R10-HTC.2018.8629828
Jung, J., Maeda, M., Chang, A., Bhandari, M., Ashapure, A., Landivar-Bowles, J. (2021). The potential of remote sensing and artificial intelligence as tools to improve the resilience of agriculture production systems. Curr. Opin. Biotechnol. 70, 15–22. doi: 10.1016/j.copbio.2020.09.003
Kanning, M., Kühling, I., Trautz, D., Jarmer, T. (2018). High-resolution UAV-based hyperspectral imagery for LAI and chlorophyll estimations from wheat for yield prediction. Remote Sens. 10, 2000. doi: 10.3390/rs10122000
Kavaliauskas, A., Žydelis, R., Castaldi, F., Auškalnienė, O., Povilaitis, V. (2023). Predicting maize theoretical methane yield in combination with ground and UAV remote data using machine learning. Plants 12, 1823. doi: 10.3390/plants12091823
Kefauver, S. C., Vicente, R., Vergara-Díaz, O., Fernandez-Gallego, J. A., Kerfal, S., Lopez, A., et al. (2017). Comparative UAV and field phenotyping to assess yield and nitrogen use efficiency in hybrid and conventional barley. Front. Plant Sci. 8, 1733. doi: 10.3389/fpls.2017.01733
Kim, M., Ko, J., Jeong, S., Yeom, J., Kim, H. (2017). Monitoring canopy growth and grain yield of paddy rice in South Korea by using the GRAMI model and high spatial resolution imagery. GIScience Remote Sens. 54, 534–551. doi: 10.1080/15481603.2017.1291783
Kumar, C., Mubvumba, P., Huang, Y., Dhillon, J., Reddy, K. (2023). Multi-stage corn yield prediction using high-resolution UAV multispectral data and machine learning models. Agronomy 13, 1277. doi: 10.3390/agronomy13051277
Kurihara, J., Nagata, T., Tomiyama, H. (2023). Rice yield prediction in different growth environments using unmanned aerial vehicle-based hyperspectral imaging. Remote Sens. 15, 2004. doi: 10.3390/rs15082004
Kyratzis, A. C., Skarlatos, D. P., Menexes, G. C., Vamvakousis, V. F., Katsiotis, A. (2017). Assessment of vegetation indices derived by UAV imagery for durum wheat phenotyping under a water limited and heat stressed Mediterranean environment. Front. Plant Sci. 8, 1114. doi: 10.3389/fpls.2017.01114
Lee, W.-S., Alchanatis, V., Yang, C., Hirafuji, M., Moshou, D., Li, C. (2010). Sensing technologies for precision specialty crop production. Comput. Electron. Agric. 74, 2–33. doi: 10.1016/j.compag.2010.08.005
Lee, K.-J., Lee, B.-W. (2011). Estimating canopy cover from color digital camera image of rice field. J. Crop Sci. Biotechnol. 14, 151–155. doi: 10.1007/s12892-011-0029-z
Li, Z., Chen, Z., Cheng, Q., Duan, F., Sui, R., Huang, X., et al. (2022). UAV-based hyperspectral and ensemble machine learning for predicting yield in winter wheat. Agronomy 12, 202. doi: 10.3390/agronomy12010202
Li, C., Ma, C., Cui, Y., Lu, G., Wei, F. (2021). Uav hyperspectral remote sensing estimation of soybean yield based on physiological and ecological parameter and meteorological factor in China. J. Indian Soc. Remote Sens. 49, 873–886. doi: 10.1007/s12524-020-01269-3
Li, Z., Wang, J., Liu, C., Song, X., Xu, X., Li, Z. (2018). “Predicting grain protein content in winter wheat using hyperspectral and meteorological factor,“ in 2018 7th International Conference on Agro-geoinformatics (Agro-geoinformatics), Hangzhou, China, 1–4. doi: 10.1109/Agro-Geoinformatics.2018.8476098
Liao, H., Tang, M., Luo, L., Li, C., Chiclana, F., Zeng, X.-J. (2018). A bibliometric analysis and visualization of medical big data research. Sustainability 10, 166. doi: 10.3390/su10010166
Liu, Y., Nie, C., Zhang, Z., Wang, Z., Ming, B., Xue, J., et al. (2023). Evaluating how lodging affects maize yield estimation based on UAV observations. Front. Plant Sci. 13, 979103. doi: 10.3389/fpls.2022.979103
Liu, J., Zhu, Y., Tao, X., Chen, X., Li, X. (2022). Rapid prediction of winter wheat yield and nitrogen use efficiency using consumer-grade unmanned aerial vehicles multispectral imagery. Front. Plant Sci. 13, 1032170. doi: 10.3389/fpls.2022.1032170
Longfei, Z., Ran, M., Xing, Y., Yigui, L., Zehua, H., Zhengang, L., et al. (2023). Improved yield prediction of ratoon rice using unmanned aerial vehicle-based multi-temporal feature method. Rice Sci. 30, 247–256. doi: 10.1016/j.rsci.2023.03.008
Luo, S., Jiang, X., Jiao, W., Yang, K., Li, Y., Fang, S. (2022). Remotely sensed prediction of rice yield at different growth durations using UAV multispectral imagery. Agriculture 12, 1447. doi: 10.3390/agriculture12091447
Ma, J., Liu, B., Ji, L., Zhu, Z., Wu, Y., Jiao, W. (2023). Field-scale yield prediction of winter wheat under different irrigation regimes based on dynamic fusion of multimodal UAV imagery. Int. J. Appl. Earth Observ. Geoinform. 118, 103292. doi: 10.1016/j.jag.2023.103292
Maes, W. H., Steppe, K. (2019). Perspectives for remote sensing with unmanned aerial vehicles in precision agriculture. Trends Plant Sci. 24, 152–164. doi: 10.1016/j.tplants.2018.11.007
Maimaitijiang, M., Sagan, V., Sidike, P., Hartling, S., Esposito, F., Fritschi, F. B. (2020). Soybean yield prediction from UAV using multimodal data fusion and deep learning. Remote Sens. Environ. 237, 111599. doi: 10.1016/j.rse.2019.111599
Marino, S., Alvino, A. (2020). Agronomic traits analysis of ten winter wheat cultivars clustered by UAV-derived vegetation indices. Remote Sens. 12, 249. doi: 10.3390/rs12020249
McCarthy, C., Nyoni, Y., Kachamba, D. J., Banda, L. B., Moyo, B., Chisambi, C., et al. (2023). Can drones help smallholder farmers improve agriculture efficiencies and reduce food insecurity in sub-Saharan Africa? Local perceptions from Malawi. Agriculture 13, 1075. doi: 10.3390/agriculture13051075
Meiyan, S., Mengyuan, S., Qizhou, D., Xiaohong, Y., Baoguo, L., Yuntao, M. (2022). Estimating the maize above-ground biomass by constructing the tridimensional concept model based on UAV-based digital and multi-spectral images. Field Crops Res. 282, 108491. doi: 10.1016/j.fcr.2022.108491
Melesse, A. M., Weng, Q., Thenkabail, P. S., Senay, G. B. (2007). Remote sensing sensors and applications in environmental resources mapping and modelling. Sensors 7, 3209–3241. doi: 10.3390/s7123209
Mia, M. S., Tanabe, R., Habibi, L. N., Hashimoto, N., Homma, K., Maki, M., et al. (2023). Multimodal deep learning for rice yield prediction using UAV-based multispectral imagery and weather data. Remote Sens. 15, 2511. doi: 10.3390/rs15102511
Mohsan, S. A. H., Othman, N. Q. H., Li, Y., Alsharif, M. H., Khan, M. A. (2023). Unmanned aerial vehicles (UAVs): Practical aspects, applications, open challenges, security issues, and future trends. Intell. Serv. Robot. 16, 109–137. doi: 10.1007/s11370-022-00452-4
Muchiri, G., Kimathi, S. (2022). “A review of applications and potential applications of UAV,” in Proceedings of the Sustainable Research and Innovation Conference, 280–283.
Müller, B., Hardt, L., Armbruster, A., Kiefer, K., Reise, C. (2016). Yield predictions for photovoltaic power plants: empirical validation, recent advances and remaining uncertainties. Prog. Photovol.: Res. Appl. 24, 570–583. doi: 10.1002/pip.2616
Mustafa, G., Zheng, H., Khan, I. H., Tian, L., Jia, H., Li, G., et al. (2022a). Hyperspectral reflectance proxies to diagnose in-field fusarium head blight in wheat with machine learning. Remote Sens. 14, 2784. doi: 10.3390/rs14122784
Mustafa, G., Zheng, H., Khan, I. H., Zhu, J., Yang, T., Wang, A., et al. (2024). Enhancing fusarium head blight detection in wheat crops using hyperspectral indices and machine learning classifiers. Comput. Electron. Agric. 218, 108663. doi: 10.1016/j.compag.2024.108663
Mustafa, G., Zheng, H., Li, W., Yin, Y., Wang, Y., Zhou, M., et al. (2022b). Fusarium head blight monitoring in wheat ears using machine learning and multimodal data from asymptomatic to symptomatic periods. Front. Plant Sci. 13. doi: 10.3389/fpls.2022.1102341
Nabwire, S., Suh, H.-K., Kim, M. S., Baek, I., Cho, B.-K. (2021). Application of artificial intelligence in phenomics. Sensors 21, 4363. doi: 10.3390/s21134363
Osco, L. P., Junior, J. M., Ramos, A. P. M., Furuya, D. E. G., Santana, D. C., Teodoro, L. P. R., et al. (2020). Leaf nitrogen concentration and plant height prediction for maize using UAV-based multispectral imagery and machine learning techniques. Remote Sens. 12, 3237. doi: 10.3390/rs12193237
Ostos-Garrido, F. J., De Castro, A. I., Torres-Sánchez, J., Pistón, F., Peña, J. M. (2019). High-throughput phenotyping of bioethanol potential in cereals using UAV-based multi-spectral imagery. Front. Plant Sci. 10, 948. doi: 10.3389/fpls.2019.00948
Pádua, L., Vanko, J., Hruška, J., Adão, T., Sousa, J. J., Peres, E., et al. (2017). UAS, sensors, and data processing in agroforestry: A review towards practical applications. Int. J. Remote Sens. 38, 2349–2391. doi: 10.1080/01431161.2017.1297548
Panday, U. S., Shrestha, N., Maharjan, S., Pratihast, A. K., Shahnawaz, Shrestha, K. L., et al. (2020). Correlating the plant height of wheat with above-ground biomass and crop yield using drone imagery and crop surface model, a case study from Nepal. Drones 4, 28. doi: 10.3390/drones4030028
Peng, X., Han, W., Ao, J., Wang, Y. (2021). Assimilation of LAI derived from UAV multispectral data into the SAFY model to estimate maize yield. Remote Sens. 13, 1094. doi: 10.3390/rs13061094
Peng, Y., Li, Y., Dai, C., Fang, S., Gong, Y., Wu, X., et al. (2019). Remote prediction of yield based on LAI estimation in oilseed rape under different planting methods and nitrogen fertilizer applications. Agric. For. Meteorol. 271, 116–125. doi: 10.1016/j.agrformet.2019.02.032
Perros, N., Kalivas, D., Giovos, R. (2021). Spatial analysis of agronomic data and uav imagery for rice yield estimation. Agriculture 11, 809. doi: 10.3390/agriculture11090809
Prey, L., Hanemann, A., Ramgraber, L., Seidl-Schulz, J., Noack, P. O. (2022). UAV-Based estimation of grain yield for plant breeding: Applied strategies for optimizing the use of sensors, vegetation indices, growth stages, and machine learning algorithms. Remote Sens. 14, 6345. doi: 10.3390/rs14246345
Prey, L., Ramgraber, L., Seidl-Schulz, J., Hanemann, A., Noack, P. O. (2023). The transferability of spectral grain yield prediction in wheat breeding across years and trial locations. Sensors 23, 4177. doi: 10.3390/s23084177
Puget Systems (2021). Recommended Systems for Pix4D. Available online at: https://www.pugetsystems.com/recommended/Recommended-Systems-for-Pix4D-207.
Qian, J., Law, R., Wei, J. (2019). Knowledge mapping in travel website studies: A scientometric review. Scandinavian J. Hospital. Tourism 19, 192–209. doi: 10.1080/15022250.2018.1526113
Ramos, A. P. M., Osco, L. P., Furuya, D. E. G., Gonçalves, W. N., Santana, D. C., Teodoro, L. P. R., et al. (2020). A random forest ranking approach to predict yield in maize with uav-based vegetation spectral indices. Comput. Electron. Agric. 178, 105791. doi: 10.1016/j.compag.2020.105791
Raun, W. R., Solie, J. B., Johnson, G. V., Stone, M. L., Lukina, E. V., Thomason, W. E., et al. (2001). In-season prediction of potential grain yield in winter wheat using canopy reflectance. Agron. J. 93, 131–138. doi: 10.2134/agronj2001.931131x
Rembold, F., Atzberger, C., Savin, I., Rojas, O. (2013). Using low resolution satellite imagery for yield prediction and yield anomaly detection. Remote Sens. 5, 1704–1733. doi: 10.3390/rs5041704
Roy Choudhury, M., Das, S., Christopher, J., Apan, A., Chapman, S., Menzies, N. W., et al. (2021). Improving biomass and grain yield prediction of wheat genotypes on sodic soil using integrated high-resolution multispectral, hyperspectral, 3D point cloud, and machine learning techniques. Remote Sens. 13, 3482. doi: 10.3390/rs13173482
Samaras, S., Diamantidou, E., Ataloglou, D., Sakellariou, N., Vafeiadis, A., Magoulianitis, V., et al. (2019). Deep learning on multi sensor data for counter UAV applications—A systematic review. Sensors 19, 4837. doi: 10.3390/s19224837
Santesteban, L., Di Gennaro, S., Herrero-Langreo, A., Miranda, C., Royo, J., Matese, A. (2017). High-resolution UAV-based thermal imaging to estimate the instantaneous and seasonal variability of plant water status within a vineyard. Agric. Water Manage. 183, 49–59. doi: 10.1016/j.agwat.2016.08.026
Sapkota, S., Paudyal, D. R. (2023). Growth monitoring and yield estimation of maize plant using unmanned aerial vehicle (UAV) in a hilly region. Sensors 23, 5432. doi: 10.3390/s23125432
Saravia, D., Salazar, W., Valqui-Valqui, L., Quille-Mamani, J., Porras-Jorge, R., Corredor, F.-A., et al. (2022). Yield predictions of four hybrids of maize (Zea mays) using multispectral images obtained from UAV in the Coast of Peru. Agronomy 12, 2630. doi: 10.3390/agronomy12112630
Sarkar, T. K., Ryu, C.-S., Kang, Y.-S., Kim, S.-H., Jeon, S.-R., Jang, S.-H., et al. (2018). Integrating UAV remote sensing with GIS for predicting rice grain protein. J. Biosyst. Eng. 43, 148–159. doi: 10.5307/JBE.2018.43.2.148
Shafiee, S., Lied, L. M., Burud, I., Dieseth, J. A., Alsheikh, M., Lillemo, M. (2021). Sequential forward selection and support vector regression in comparison to LASSO regression for spring wheat yield prediction based on UAV imagery. Comput. Electron. Agric. 183, 106036. doi: 10.1016/j.compag.2021.106036
Shafiee, S., Mroz, T., Burud, I., Lillemo, M. (2023). Evaluation of UAV multispectral cameras for yield and biomass prediction in wheat under different sun elevation angles and phenological stages. Comput. Electron. Agric. 210, 107874. doi: 10.1016/j.compag.2023.107874
Shanahan, J. F., Schepers, J. S., Francis, D. D., Varvel, G. E., Wilhelm, W. W., Tringe, J. M., et al. (2001). Use of remote-sensing imagery to estimate corn grain yield. Agron. J. 93, 583–589. doi: 10.2134/agronj2001.933583x
Shen, Y., Mercatoris, B., Cao, Z., Kwan, P., Guo, L., Yao, H., et al. (2022). Improving wheat yield prediction accuracy using LSTM-RF framework based on UAV thermal infrared and multispectral imagery. Agriculture 12, 892. doi: 10.3390/agriculture12060892
Song, Y., Wang, J., Shan, B. (2021). Estimation of winter wheat yield from UAV-based multi-temporal imagery using crop allometric relationship and SAFY model. Drones 5, 78. doi: 10.3390/drones5030078
Song, Y., Wang, J., Shang, J., Liao, C. (2020). Using UAV-based SOPC derived LAI and SAFY model for biomass and yield estimation of winter wheat. Remote Sens. 12, 2378. doi: 10.3390/rs12152378
Su, X., Wang, J., Ding, L., Lu, J., Zhang, J., Yao, X., et al. (2023). Grain yield prediction using multi-temporal UAV-based multispectral vegetation indices and endmember abundance in rice. Field Crops Res. 299, 108992. doi: 10.1016/j.fcr.2023.108992
Sunoj, S., Cho, J., Guinness, J., van Aardt, J., Czymmek, K. J., Ketterings, Q. M. (2021). Corn grain yield prediction and mapping from Unmanned Aerial System (UAS) multispectral imagery. Remote Sens. 13, 3948. doi: 10.3390/rs13193948
Sziroczak, D., Rohacs, D., Rohacs, J. (2022). Review of using small UAV based meteorological measurements for road weather management. Prog. Aerospace Sci. 134, 100859. doi: 10.1016/j.paerosci.2022.100859
Tao, H., Feng, H., Xu, L., Miao, M., Yang, G., Yang, X., et al. (2020). Estimation of the yield and plant height of winter wheat using UAV-based hyperspectral images. Sensors 20, 1231. doi: 10.3390/s20041231
Tavares, C. J., Ribeiro Junior, W. Q., Ramos, M. L. G., Pereira, L. F., Casari, R. A., das, C. N., et al. (2022). Water stress alters morphophysiological, grain quality and vegetation indices of soybean cultivars. Plants 11, 559. doi: 10.3390/plants11040559
Teodoro, P. E., Teodoro, L. P. R., Baio, F. H. R., da Silva Junior, C. A., dos Santos, R. G., Ramos, A. P. M., et al. (2021). Predicting days to maturity, plant height, and grain yield in soybean: A machine and deep learning approach using multispectral data. Remote Sens. 13, 4632. doi: 10.3390/rs13224632
Tian, Z., Zhang, Y., Liu, K., Li, Z., Li, M., Zhang, H., et al. (2022). UAV remote sensing prediction method of winter wheat yield based on the fused features of crop and soil. Remote Sens. 14, 5054. doi: 10.3390/rs14195054
Tilman, D., Balzer, C., Hill, J., Befort, B. L. (2011). Global food demand and the sustainable intensification of agriculture. Proc. Natl. Acad. Sci. 108, 20260–20264. doi: 10.1073/pnas.1116437108
Ubina, N. A., Cheng, S.-C. (2022). A review of unmanned system technologies with its application to aquaculture farm monitoring and management. Drones 6, 12. doi: 10.3390/drones6010012
Van Eck, N., Waltman, L. (2010). Software survey: VOSviewer, a computer program for bibliometric mapping. scientometrics 84, 523–538. doi: 10.1007/s11192-009-0146-3
Vega, F. A., Ramirez, F. C., Saiz, M. P., Rosúa, F. O. (2015). Multi-temporal imaging using an unmanned aerial vehicle for monitoring a sunflower crop. Biosyst. Eng. 132, 19–27. doi: 10.1016/j.biosystemseng.2015.01.008
Wahab, I., Hall, O., Jirström, M. (2018). Remote sensing of yields: Application of uav imagery-derived ndvi for estimating maize vigor and yields in complex farming systems in sub-saharan africa. Drones 2, 28. doi: 10.3390/drones2030028
Wan, L., Cen, H., Zhu, J., Zhang, J., Zhu, Y., Sun, D., et al. (2020). Grain yield prediction of rice using multi-temporal UAV-based RGB and multispectral images and model transfer–a case study of small farmlands in the South of China. Agric. For. Meteorol. 291, 108096. doi: 10.1016/j.agrformet.2020.108096
Wang, D., Li, R., Zhu, B., Liu, T., Sun, C., Guo, W. (2022a). Estimation of wheat plant height and biomass by combining uav imagery and elevation data. Agriculture 13, 9. doi: 10.3390/agriculture13010009
Wang, Z., Wang, S., Wang, H., Liu, L., Li, Z., Zhu, Y., et al. (2022b). Field-scale rice yield estimation based on UAV-based MiniSAR data with Ku band and modified water-cloud model of panicle layer at panicle stage. Front. Plant Sci. 13, 1001779. doi: 10.3389/fpls.2022.1001779
Wang, F., Yao, X., Xie, L., Zheng, J., Xu, T. (2021a). Rice yield estimation based on vegetation index and florescence spectral information from UAV hyperspectral remote sensing. Remote Sens. 13, 3390. doi: 10.3390/rs13173390
Wang, F., Yi, Q., Hu, J., Xie, L., Yao, X., Xu, T., et al. (2021b). Combining spectral and textural information in UAV hyperspectral images to estimate rice grain yield. Int. J. Appl. Earth Observ. Geoinform. 102, 102397. doi: 10.1016/j.jag.2021.102397
Wolfert, S., Ge, L., Verdouw, C., Bogaardt, M.-J. (2017). Big data in smart farming–a review. Agric. Syst. 153, 69–80. doi: 10.1016/j.agsy.2017.01.023
Yang, S., Hu, L., Wu, H., Ren, H., Qiao, H., Li, P., et al. (2021a). Integration of crop growth model and random forest for winter wheat yield estimation from UAV hyperspectral imagery. IEEE J. Select. Topics Appl. Earth Observ. Remote Sens. 14, 6253–6269. doi: 10.1109/JSTARS.2021.3089203
Yang, G., Liu, J., Zhao, C., Li, Z., Huang, Y., Yu, H., et al. (2017). Unmanned aerial vehicle remote sensing for field-based crop phenotyping: current status and perspectives. Front. Plant Sci. 8, 1111. doi: 10.3389/fpls.2017.01111
Yang, W., Nigon, T., Hao, Z., Paiao, G. D., Fernández, F. G., Mulla, D., et al. (2021b). Estimation of corn yield based on hyperspectral imagery and convolutional neural network. Comput. Electron. Agric. 184, 106092. doi: 10.1016/j.compag.2021.106092
Yang, J., Xing, M., Tan, Q., Shang, J., Song, Y., Ni, X., et al. (2023). Estimating effective leaf area index of winter wheat based on UAV point cloud data. Drones 7, 299. doi: 10.3390/drones7050299
Yang, B., Zhu, W., Rezaei, E. E., Li, J., Sun, Z., Zhang, J. (2022). The optimal phenological phase of maize for yield prediction with high-frequency UAV remote sensing. Remote Sens. 14, 1559. doi: 10.3390/rs14071559
Yu, D., Zha, Y., Sun, Z., Li, J., Jin, X., Zhu, W., et al. (2023). Deep convolutional neural networks for estimating maize above-ground biomass using multi-source UAV images: A comparison with traditional machine learning algorithms. Precis. Agric. 24, 92–113. doi: 10.1007/s11119-022-09932-0
Yuan, N., Gong, Y., Fang, S., Liu, Y., Duan, B., Yang, K., et al. (2021). UAV remote sensing estimation of rice yield based on adaptive spectral endmembers and bilinear mixing model. Remote Sens. 13, 2190. doi: 10.3390/rs13112190
Yue, J., Feng, H., Li, Z., Zhou, C., Xu, K. (2021). Mapping winter-wheat biomass and grain yield based on a crop model and UAV remote sensing. Int. J. Remote Sens. 42, 1577–1601. doi: 10.1080/01431161.2020.1823033
Yue, J., Yang, G., Tian, Q., Feng, H., Xu, K., Zhou, C. (2019). Estimate of winter-wheat above-ground biomass based on UAV ultrahigh-ground-resolution image textures and vegetation indices. ISPRS J. Photogram. Remote Sens. 150, 226–244. doi: 10.1016/j.isprsjprs.2019.02.022
Zeng, L., Peng, G., Meng, R., Man, J., Li, W., Xu, B., et al. (2021). Wheat yield prediction based on unmanned aerial vehicles-collected red–green–blue imagery. Remote Sens. 13, 2937. doi: 10.3390/rs13152937
Zhang, J., Liu, X., Liang, Y., Cao, Q., Tian, Y., Zhu, Y., et al. (2019). Using a portable active sensor to monitor growth parameters and predict grain yield of winter wheat. Sensors 19, 1108. doi: 10.3390/s19051108
Zhang, M., Zhou, J., Sudduth, K. A., Kitchen, N. R. (2020). Estimation of maize yield and effects of variable-rate nitrogen application using UAV-based RGB imagery. Biosyst. Eng. 189, 24–35. doi: 10.1016/j.biosystemseng.2019.11.001
Zhao, X., Yuan, Y., Song, M., Ding, Y., Lin, F., Liang, D., et al. (2019). Use of unmanned aerial vehicle imagery and deep learning unet to extract rice lodging. Sensors 19, 3859. doi: 10.3390/s19183859
Zhao, B., Zhang, J., Yang, C., Zhou, G., Ding, Y., Shi, Y., et al. (2018). Rapeseed seedling stand counting and seeding performance evaluation at two early growth stages based on unmanned aerial vehicle imagery. Front. Plant Sci. 9, 1362. doi: 10.3389/fpls.2018.01362
Zhaosheng, Y., Tao, L., Tianle, Y., Chengxin, J., Chengming, S. (2022). Rapid detection of wheat ears in orthophotos from unmanned aerial vehicles in fields based on YOLOX. Front. Plant Sci. 13, 851245. doi: 10.3389/fpls.2022.851245
Zheng, H., Cheng, T., Zhou, M., Li, D., Yao, X., Tian, Y., et al. (2019). Improved estimation of rice aboveground biomass combining textural and spectral analysis of UAV imagery. Precis. Agric. 20, 611–629. doi: 10.1007/s11119-018-9600-7
Zheng, H., Ji, W., Wang, W., Lu, J., Li, D., Guo, C., et al. (2022). Transferability of models for predicting rice grain yield from unmanned aerial vehicle (UAV) multispectral imagery across years, cultivars and sensors. Drones 6, 423. doi: 10.3390/drones6120423
Zhou, J., Beche, E., Vieira, C. C., Yungbluth, D., Zhou, J., Scaboo, A., et al. (2022). Improve soybean variety selection accuracy using UAV-based high-throughput phenotyping technology. Front. Plant Sci. 12, 768742. doi: 10.3389/fpls.2021.768742
Zhou, X., Kono, Y., Win, A., Matsui, T., Tanaka, T. S. (2021b). Predicting within-field variability in grain yield and protein content of winter wheat using UAV-based multispectral imagery and machine learning approaches. Plant Product. Sci. 24, 137–151. doi: 10.1080/1343943X.2020.1819165
Zhou, X., Zheng, H., Xu, X., He, J., Ge, X., Yao, X., et al. (2017). Predicting grain yield in rice using multi-temporal vegetation indices from UAV-based multispectral and digital imagery. ISPRS J. Photogram. Remote Sens. 130, 246–255. doi: 10.1016/j.isprsjprs.2017.05.003
Keywords: yield prediction, unmanned aerial vehicles, VOSviewer, wheat, maize, rice, soybean heading 3, left
Citation: Mustafa G, Liu Y, Khan IH, Hussain S, Jiang Y, Liu J, Arshad S and Osman R (2024) Establishing a knowledge structure for yield prediction in cereal crops using unmanned aerial vehicles. Front. Plant Sci. 15:1401246. doi: 10.3389/fpls.2024.1401246
Received: 15 March 2024; Accepted: 15 July 2024;
Published: 09 August 2024.
Edited by:
Parvathaneni Naga Srinivasu, Amrita Vishwa Vidyapeetham University, IndiaCopyright © 2024 Mustafa, Liu, Khan, Hussain, Jiang, Liu, Arshad and Osman. This is an open-access article distributed under the terms of the Creative Commons Attribution License (CC BY). The use, distribution or reproduction in other forums is permitted, provided the original author(s) and the copyright owner(s) are credited and that the original publication in this journal is cited, in accordance with accepted academic practice. No use, distribution or reproduction is permitted which does not comply with these terms.
*Correspondence: Yuhong Liu, eWhsaXVAaGh1LmVkdS5jbg==