- 1University of Brasilia, Departament of Plant Pathology, Brasília, Brazil
- 2Embrapa Instrumentação, São Carlos, Brazil
- 3Embrapa Hortaliças, Brasília, Brazil
Food security, a critical concern amid global population growth, faces challenges in sustainable agricultural production due to significant yield losses caused by plant diseases, with a multitude of them caused by seedborne plant pathogen. With the expansion of the international seed market with global movement of this propagative plant material, and considering that about 90% of economically important crops grown from seeds, seed pathology emerged as an important discipline. Seed health testing is presently part of quality analysis and carried out by seed enterprises and governmental institutions looking forward to exclude a new pathogen in a country or site. The development of seedborne pathogens detection methods has been following the plant pathogen detection and diagnosis advances, from the use of cultivation on semi-selective media, to antibodies and DNA-based techniques. Hyperspectral imaging (HSI) associated with artificial intelligence can be considered the new frontier for seedborne pathogen detection with high accuracy in discriminating infected from healthy seeds. The development of the process consists of standardization of methods and protocols with the validation of spectral signatures for presence and incidence of contamined seeds. Concurrently, epidemiological studies correlating this information with disease outbreaks would help in determining the acceptable thresholds of seed contamination. Despite the high costs of equipment and the necessity for interdisciplinary collaboration, it is anticipated that health seed certifying programs and seed suppliers will benefit from the adoption of HSI techniques in the near future.
Introduction
Food security is currently the focus of agricultural studies in search for sustainable production solutions that keep up with population growth. It is estimated that by 2050 the world will have approximately 10 billion inhabitants (Godfray et al., 2010; Tilman et al., 2011), which makes the demand for food a current challenge. One of the most important limitation for food production are plant diseases which can cause significant losses in productivity and food quality around the world (Savary et al., 2019). Fungal and bacterial pathogens can reduce crop yields by around 15% and plant viruses by a range of 3% to 7%, under favorable conditions such microorganisms can cause losses of up to 100% (Oerke and Dehne, 2004).
Around 90% of crops of economic and food importance are grown by seeds (Maude, 1996), therefore, the seed health is fundamental for agricultural production. Seeds are a primary source of plant pathogen contamination in crop fields and specific tools for identification and early diagnosis of pathogens in seeds are essential to mediate disease management in crops and avoid losses caused by them (Gitaitis and Walcott, 2007; Dutta et al., 2014). Some requirements are desired in the selection of seed health diagnosis methods, such as specificity; sensitivity; efficiency/speed; simplicity; a satisfactory cost-effectiveness; and reliability (Marcinkowska, 2002).
The application of molecular and serological detection methods, despite presenting specificity and sensitivity, are destructive, mostly limited to laboratory application, requiring qualified labor (Kumar and Gupta, 2020). In addition, it does not make it possible to monitor the progress of the disease in the plant or even the action of compounds applied to the crop to control the spread of the pathogen (Mishra et al., 2020). The step forward for a more efficient detection of plant pathogens, focused on quantification and distribution would be the use of hyperspectral images, which could be associated with artificial intelligence, for a faster and more reliable streategy. This article is a state-of-the-art review on the detection of plant pathogens in seeds, emphasizing the recent advances with the use of hyperspectral images as a resource in the diagnosis of phytopathogens disseminated by seeds.
Conventional methods for detecting plant pathogens in seeds
Initially, the detection of pathogens in seeds was carried out using visual diagnosis methods. Some pathogens, mainly fungi, cause external symptoms in seeds that can be naked-eye visualized or with the aid of a microscope (Gaur et al., 2020). The presence of certain symptoms in seeds may be common among plant pathogens or even the signs may not be visible in the seed tissues (Kumar et al., 2020). Furthermore, the symptoms in seeds may also be of abiotic origins, such as environmental stresses and nutrition to the seed crops or mechanical injuries during the seed harvesting and processing (Bala, 2020).
Directed detection by pathogen cultivation by plaquing the seed on semi-selective or enriched culture media is one of the most used techniques for detecting plant pathogens in seeds (Mancini et al., 2016). Although efficient, they are restricted to the detection of only cultivable microorganisms. After the pathogen growth, identification can be performed by morphology, biochemical and/or molecular tests (Kumar et al., 2020).
ELISA (Enzyme-linked immunosorbent assays), a sorologial method which demands species-specific antibodies in order not to cause cross-reactions between related and unrelated species which could lead to false negative results (Dewey et al., 1997; Mancini et al., 2016). Moreover, these tests often yield false positives by detecting non-viable microorganisms. This impacts analysis interpretation and directly affects diagnostic accuracy (Walcott, 2003; Afouda et al., 2009).
Molecular techniques, such as nucleic acid amplification using DNA and RNA, offer enhanced sensitivity and specificity in pathogen detection. PCR (Polymerase chain reaction), a pivotal advancement in molecular biology by Mullis et al. (1986), has evolved into various forms, including Nested PCR, BIO-PCR, qPCR (Quantitative Polymerase Chain Reaction), RT-qPCR (Reverse Transcription Quantitative Polymerase Chain Reaction), and LAMP (Loop-mediated isothermal amplification) (Massung et al., 1998; Notomi et al., 2000; Nazarenko et al., 2002; Bookout et al., 2006).
Conventional PCR is applied in several studies involving the detection of plant pathogens transmitted by seeds. However, these reproductive units are often infected by pathogens at a very low concentrations, which makes detection by PCR unfeasible due to insufficient amount of the target DNA. Based on this problem, BIO-PCR, proven to be effective for bacteria and fungi detection in seeds (Munkvold, 2009) as it allows the pathogen growth by incubation with semi-selective media followed by PCR (Schaad et al., 1995). Compared to conventional PCR, BIO-PCR is more advantageous due to the greater sensitivity, absence of PCR inhibitors (from plant or seed tissues), and detection of only viable cells, eliminating false positives (Marcinkowska, 2002). However, it may have higher costs due to the use of semi-selective media with expensive antibiotics, and delays from pathogen growth in the culture medium (Walcott, 2003; Schena et al., 2004).
Nested PCR is a very sensitive PCR modification, which can be completed in a 24-hour period (Chen et al., 2007). However, it demands additional time and reagents due the two rounds of PCR, and is more prone to contamination than conventional PCR (McCartney et al., 2003; Atkins and Clark, 2004). qPCR and RT-qPCR are other types of PCR which have been used for detecting seed-transmitted plant pathogens due to their sensitivity and speed. While effective, this technique may yield false positives from occasional spikes in background fluorescence or minimal DNA cross-contamination (Robène et al., 2015). Additionally, PCR-based analyses demand specialized and trained technical personnel and expensive equipment, usually being restricted to laboratory settings (Kaur et al., 2020). PCR techniques, including PCR, Nested-PCR, BIO-PCR, qPCR, RT-qPCR and others may be used as a multiplex, capable of detecting simultaneously more than one seed-borne pathogens with greater sensitivity than conventional and individual PCR (Ha et al., 2009; Robène-Soustrade et al., 2010).
Derived from PCR, Loop-mediated isothermal amplification (LAMP) based technique efficiently detects plant pathogens in seeds (Choudhary et al., 2022), offering several advantages such as: it operates under isothermal conditions, allows direct visualization of amplification results, and can be applied in the field (Notomi et al., 2000). The LAMP assay is more sensitive and specific than PCR, mainly due to the addition of loop primers (Nagamine et al., 2002). However, the handling of samples when adding interleaved dyes, which allow visualization of the result, can influence the occurrence of false positives, due to the release of aerosols of target nucleic acid fragments into the environment (Hardinge and Murray, 2019). Also, as other molecular tests, it may detect not only the living microrganisms but also any remaining pathogen DNA within the sample.
Hyperspectral imaging and artificial intelligence
The human eye can detect only a small portion of the electromagnetic spectrum, distinguishing the spectral responses of images/objects in a restricted range of the visible spectrum between 400 nm and 700 nm (Zwinkels, 2015). To overcome this limitation, optical remote sensing has emerged as a technique capable of obtaining representative data in ranges beyond infrared, typically between 400 nm and 2500 nm (Oliveira et al., 2020). The possibility of capturing the spectral signal in a larger range allows the structuring of reflectance profiles and the detection of patterns that differentiate features between different targets far beyond the visual range (Shanmugapriya et al., 2019).
In recent decades, several studies have involved the application of remote sensing in agriculture both in field and laboratory. The use of optical remote sensing through hyperspectral images is an example (Demattê et al., 2010; Feng et al., 2019; Abdulridha et al., 2020b). These sensors measure the reflectance of an object, such as plant leaves, and identify any changes, such as those caused by a plant pathogen, as any disturbance to the leaf region would alter the reflectance and direction of the light (Xie et al., 2008).
A HSI (hyperspectral imaging) combines conventional imaging system with spectroscopy. Unlike the RGB (red, green and blue) color system, HSI corresponds to a set of techniques capable of capturing wavelengths beyond red, green and blue (Li et al., 2014). HSI consists of a spectrograph capable of capturing reflectance in a wide range of the spectrum, such as visible (VIS), ultraviolet (UV), near-infrared (NIR), and short-wave infrared (SWIR) (Bock et al., 2010). Such images are three-dimensional (3D), formed by two spatial dimensions (x and y) and one spectral dimension (z). By combining conventional imaging with spectroscopy, HSI obtains complementary information from both fields (Mishra et al., 2017). An example of such a combination is that while spectroscopy collects data about plant physiology, conventional imaging system gathers information on plant structural dynamics (Montes et al., 2007; Bucksch et al., 2014).
Hyperspectral images have hundreds of wavelength bands close together in a spectral range, producing a dense colorfull cube of information, with spatial resolution capable of obtaining several pixels per target (Lowe et al., 2017). The spectral portion generally applied to the study of hyperspectral images of plants ranges from UV starting at ~250 nm to SWIR up to ~2500 nm. The most useful spectral band for plant analysis are the visible and near infrared (Lowe et al., 2017). In the range of 400-700 nm, it is possible to capture changes in leaf pigmentation, whereas at 700-1300 nm range, changes in the cellular structure of the mesophyll. Wider spectral ranges (1300-2500 nm) are needed, for example, for water content in a plant (Peñuelas and Filella, 1998).
The greatest advantage of the application of HSI is that they are techniques capable of detecting and differentiating a disease even in an asymptomatic plant (Martinelli et al., 2015; Whetton et al., 2018). The possibility of early detection through the use of hyperspectral sensors can contribute to the early and efficient management of diseases, preventing spread in the field (Abdulridha et al., 2020a). Furthermore, hyperspectral imaging technology is non-invasive/destructive, which is advantageous when compared to molecular analysis, where the sample cannot be reused (Sankaran et al., 2010; Golhani et al., 2018).
The data generated is quite collinear and requires different statistical tools to extract information and model patterns (Mishra et al., 2017), such as for artificial intelligence. The application of artificial intelligence using machine learning and deep learning combined with hyperspectral images has been promising in several areas of agricultural management (Demattê et al., 2010; El-Mesery et al., 2019; Feng et al., 2019; Abdulridha et al., 2020b). Artificial intelligence has different definitions, succinctly, it refers to the ability of a machine to perform a function in a similar way to the human mind for “learning” and “problem solving”. Machine learning consists of computational algorithms that learn from input data and can perform classification or clustering tasks, which are ideal for finding patterns and trends in hyperspectral data (Dhakal et al., 2023).
Machine learning is a subfield of artificial intelligence and deep machine learning is a subset that integrates machine learning (Nguyen et al., 2019). Deep learning is excellent for analyzing and extracting useful data in large quantities or collected from different sources (Zhang et al., 2020). The analysis of hyperspectral images using deep learning with convolutional neural networks has emerged as a favorable methodology for evaluating and managing various conditions in agricultural crops, mainly aimed at disease diagnostics. Neural networks are designed to learn the spatial features that best classify or quantify a target/object, in the case of plant diseases, classifying and distinguishing, for example, healthy leaves from those infected by pathogens (Kattenborn et al., 2021).
In addition to deep learning, other algorithmic models based on machine learning are applied in hyperspectral data processing. Examples are: PCA (Principal Component Analysis) and LDA (Linear Discriminant Analysis) used for extracting features of interest, similarities, and dissimilarities in the data (Dua et al., 2020; Hsieh and Kiang, 2020), and SVM (Support Vector Machine), for solving linear classification problems, which are widely employed for HSI data classification due to their ability to effectively separate heterogeneous samples on the mapped plane (Kale et al., 2017). Although SVM was designed to decipher linear datasets, its usage can be extended to non-linear data when combined with kernel methods (Melgani and Bruzzone, 2004).
Segmentation, an important step before delivering the data cube to test a model, is applied for noise removal from the images, extraction of useful and non-redundant features and establishing the relationship between similar data points in the sample. Therefore, enabling the selection of regions of interest (ROIs), thereby allowing for more accurate data classification. However, segmentation requires the use of methods that demonstrate satisfactory performance for ROI selection. There are algorithms that can assist in this aspect, each with its own peculiarities. Furthermore, some studies clarify which algorithms exhibit better performance according to the type of HSI data to be addressed (Grewal et al., 2023). For seeds, few publications describe the issues associated to those plant material, as the variance of reflectance on each seed, due surface deformities and specular reflection, increasing the difficulties of segmentation (Barbedo et al., 2015).
Another importante step is the fusion of spectral and spatial data, which allows for the enhancement of spatial resolution in HSI images, while preserving spectral quality. However, the fusion process is complex, as there is typically a trade-off between preserving spatial data and spectral quality (Mookambiga and Gomathi, 2016). It is from this perspective that artificial intelligence has proven to be efficient in solving problems related to HSI data fusion, such as deep learning, which is ideal for extracting characteristics from complex and abundant data (Li et al., 2022). Additionally, there is commercial software available that can integrate these steps, simplifying the process.
Detection of plant pathogens in seeds by hyperspectral imaging
The detection of plant pathogens in seeds using hyperspectral images associated with artificial intelligence mechanisms is described for different pathosystems (Table 1). A limited number of studies have been published specifically focusing on the detection of plant pathogens on seeds. The majority of these studies utilize prediction models for data processing, given that alterations on these organs may not be as visually discernible as those on fully grown plants, such as symptoms of necrosis or others. The low number of publications (22) and erratic distribution along the years reveals that it is still an open field to be explored, which probably concerns to the necessity of deep knowledge on the machine and algorithmic possibilities and their high costs.
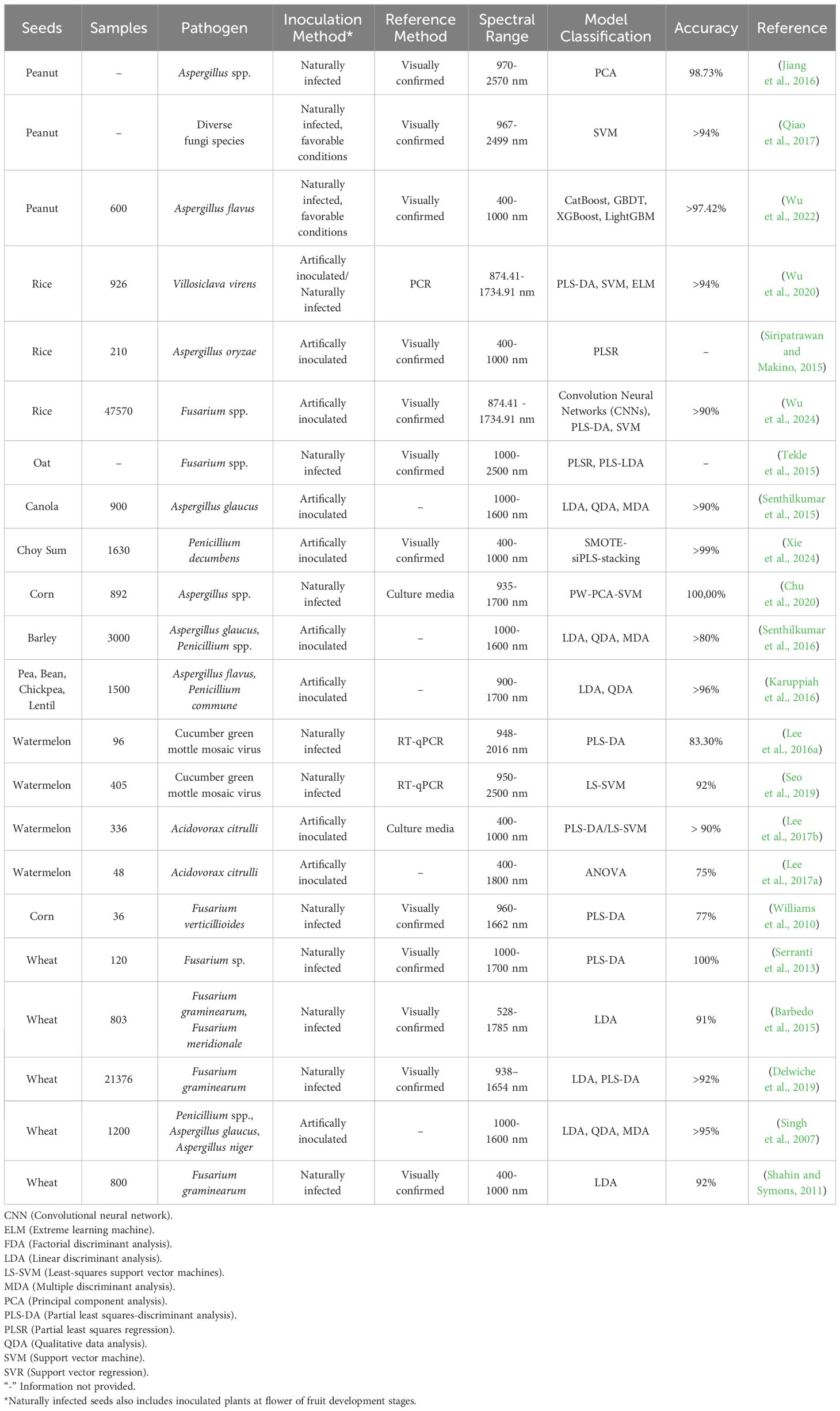
Table 1 Compilation of publications focusing on the utilization of hyperspectral imaging for the detection of plant pathogens in seeds.
The variables tested in these studies include sample size, the method for producing infected seed samples, the reference method for confirming the infection, as well as the wavelengths applied and algorithms utilized. The number of sample size among the publications is variable, from not informing the number of seeds applied in the study up to 47 thousand seeds (Figure 1A). The low number of seeds used to train and test the algorithm can superestimate the accuracy. Several publications utilize artificially inoculated seeds (40,9%), often through the infiltration of propagule suspensions, as positive controls for image generation (Figure 1B), while 54,5% used naturally infected seeds, including those for the detection of mycotoxin-producing fungi. Artificially inoculated or infiltrated seeds are commonly utilized to generate contaminated seed lots. However, this approach may introduce potential inaccuracies in the data, as the concentration of the pathogen could surpass levels typically observed in natural conditions. Wu et al. (2020) states that laboratory-inoculated seeds reflects accurately the detection of field-infected kernels. Nevertheless, such inoculated seeds might not express the impact of infection on the physiology, chemistry, and other compositions of the seed, which could be detected by hyperspectral imaging (HSI), as it can happen to soy seeds infected with Fusarium verticillioides, which may change flavonoids content (Pedrozo and Little, 2017). Further studies are required to validate this data. Additionally, the knowledge regarding the threshold limit of propagules on a seed for disease transmission, from germination to seedlings, potentially resulting in disease outbreaks, is crucial. This knowledge impairs the use of the method in properly detecting potentially harmful infected seeds (those that lead to disease transmission). For reference method, which is a method for confirmation of the pathogen presence within the seed, some publications (22.77%) did not use any kind of evaluation, while 54,5% assessed it visually (Figure 1C) and three publications (13,6%) used molecular methods. Concerning the wavelenghs applied, VIS, NIR, VNIR, SWIR bands (ranging from 400 to 2500 nm) were used in several studies, with a predominancy of NIR, occasionally in combination (Figure 1D).
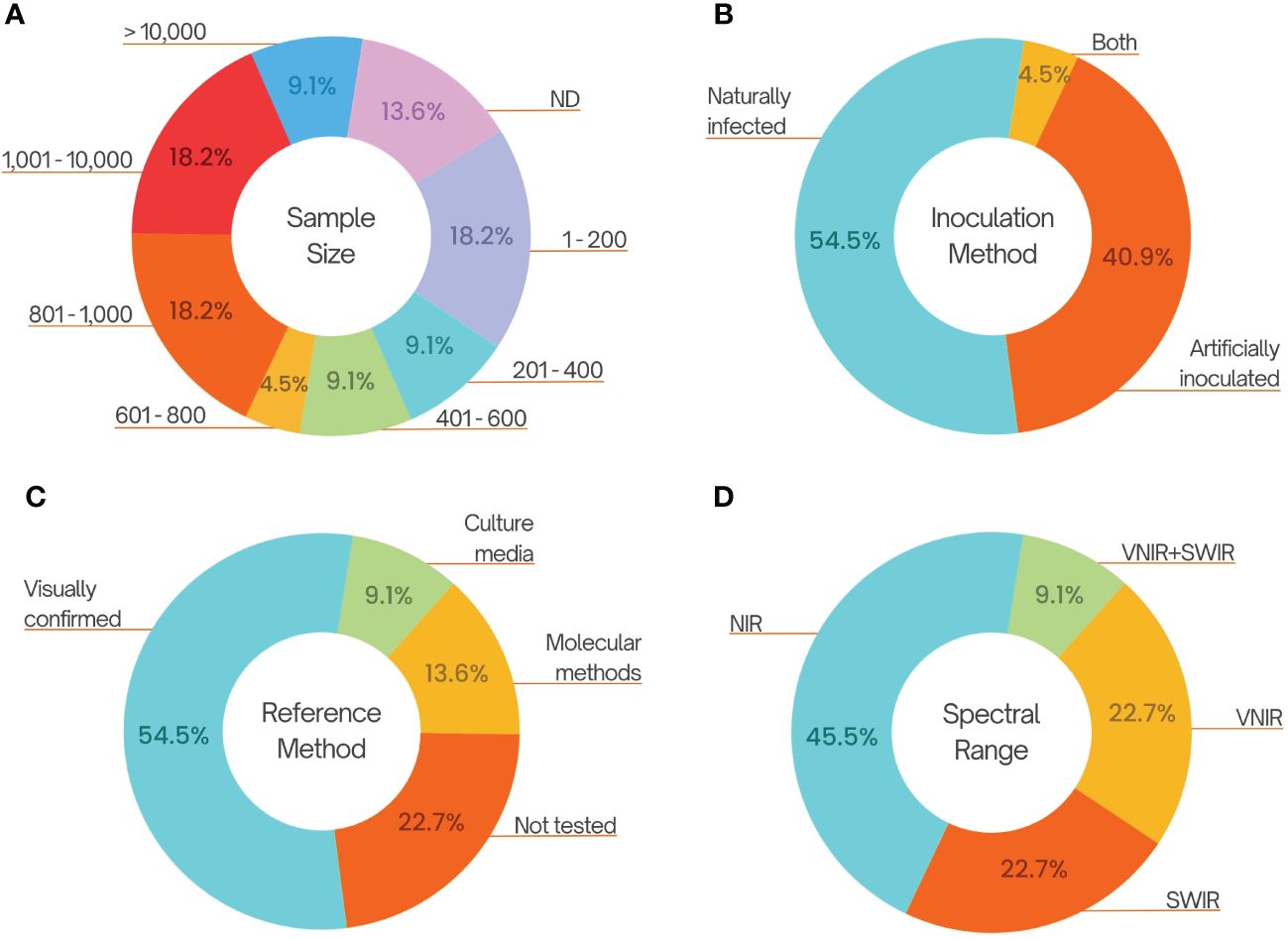
Figure 1 Compilation of cited publications on hyperspectral imaging for the detection of plant pathogens in seeds. (A) Sample size used in each publication. (B) Method of inoculation for the production of infested/infect seeds. (C) Used methods for the confirmation or quantification of the pathogen within seeds. (D) The intervals of wavelenght used for the hyperspectral image production, 400-1000 (VNIR), 700-2500 nm (NIR), 1000-2500 nm (SWIR).
Most studies focused on the detection of fungi, particularly those associated with the production of mycotoxins, such as Fusarium and Aspergillus species (Table 1). Mycotoxin accumulation renders grains unfit for human and animal consumption. Few studies address the dispersion of fungi by seeds, which could introduce the pathogen and/or cause an outbreak on production fields. Notably, groups of pathogens such as bacteria and viruses received limited attention, despite the fact that several important diseases transmitted by seeds fall within these categories. In the case of bacterial pathogens, Lee et al. (2017b) applied hyperspectral imaging in the near-infrared range with two models to classify healthy watermelon seeds and those infected with Acidovorax avenae subsp. citrulli. The accuracy of both PLS-DA and LS-SVM models was of 91.7% and 90.5%, respectively. Using the Raman hyperspectral imaging technique, they achieved 75% of classification accuracy (Lee et al., 2017a). On its turn, for viruses, using the PLS-DA model, Lee et al. (2016a) distinguished watermelon seeds infected by Cucumber green mottle mosaic virus (CGMMV) with an accuracy of 83.3% while Seo et al. (2019) obtained 92% accuracy using the LS-SVM (Least squares support vector machine) model.
For plant pathogenic fungi, numerous studies have investigated seed detection using hyperspectral images. Notably, some have demonstrated high accuracy in classification. For instance, Chu et al. (2020) achieved 100% accuracy using PCA and SVM models to classify hyperspectral data from both healthy and Aspergillus spp. infected corn seeds. Wu et al. (2022) utilized hyperspectral images in the NIR range to identify peanut seeds naturally infected with Aspergillus flavus, reaching an accuracy exceeding 97% with three distinct classification models. In Wu et al. (2024) study, the combination of hyperspectral data acquisition and sample classification using convolutional neural networks yielded an accuracy of over 98% in identifying rice seeds infected with Fusarium spp. Additionally, employing other learning models like PLS-DA and SVM resulted in accuracies surpassing 90%.
The detection of nematodes in seeds involving hyperspectral is still an open area, as no publication has been found, probably due to the fact that most plant nematode are transmited by vegetative materials, such as potato tubers, with few species capable of infecting botanic seeds. Žibrat et al. (2021) and Lapajne et al. (2022) evaluated the use of hyperspectral imaging and the application of different algorithmic models and obtained an accuracy of around 100% in the classification of infected seed potato tubers with Meloidogyne luci.
Final comments and perspectives
Due to its non-destructive nature, hyperspectral imaging might be applied to 100% of seeds without the need for sampling or destruction, as the case of molecular methods. Moreover, the utilization of hyperspectral imaging for detection does not preclude the use of molecular diagnosis as shown by Wu et al., 2020; instead, the integration of these methods enhances reliability and specificity in pathogen detection. The application of hyperspectral imaging diagnostics in seeds can expedite the detection of microorganisms, thereby lowering the chances of the introduction of new plant pathogens or variants in a site. However, much remains to be studied in order to enhance the reliability of this technology, aligning it with the standards observed in other scientific domains that already uses hyperspectral imaging.
Several difficulties in this area serve as deterrents for the adoption of this technology, including the high costs of equipment, complex software and methods. Furthermore, the involvement of a multidisciplinary team including plant pathologists, along with electronic engineers, physicists, and software engineers, is strongly recommended to deal with this new research frontier. Unfortunately, it can be observed in the recent published publications that plant pathologists are not present as part of their research teams. With the knowledge of epidemics, agricultural producing systems and seed-borne pathogen infection and detection, such especialist could shorten the period for technology application and adoption by the producing chaim authors.
Concerning the generation of contaminated seed lots for the studies, prior to the development of the hyperspectral imaging protocol, assays should be carried out to simulate the most natural conditions of an infected seed. Alternatively, naturally infected seeds obtained through either natural or artificial infection of plants could be utilized. However, this alternative approach introduces a different challenge: determining the method to validate the presence of the plant pathogen on a specific seed. Another issue is the lack of information regarding the spectral signature of the putative alterations caused to the seed by the presence of the pathogen and its successful transmission to the plant. Besides, sample standards need to be defined for each crop species.
Despite these aspects, the analyzed publications collectively highlighted important approaches that should be considered for the establishment of protocols for hyperspectral imaging detection technologies. Therefore, a seven-step scheme is proposed and recommended here as a template for future studies (Figure 2): obtention of contaminated seeds, preferably naturally infected; images capture; the data cube generation; assessment of the infection status of each seed; segmentation or extraction of the region of interest; model selection and classification based on sample size and accuracy; and finally, testing with a larger sample.
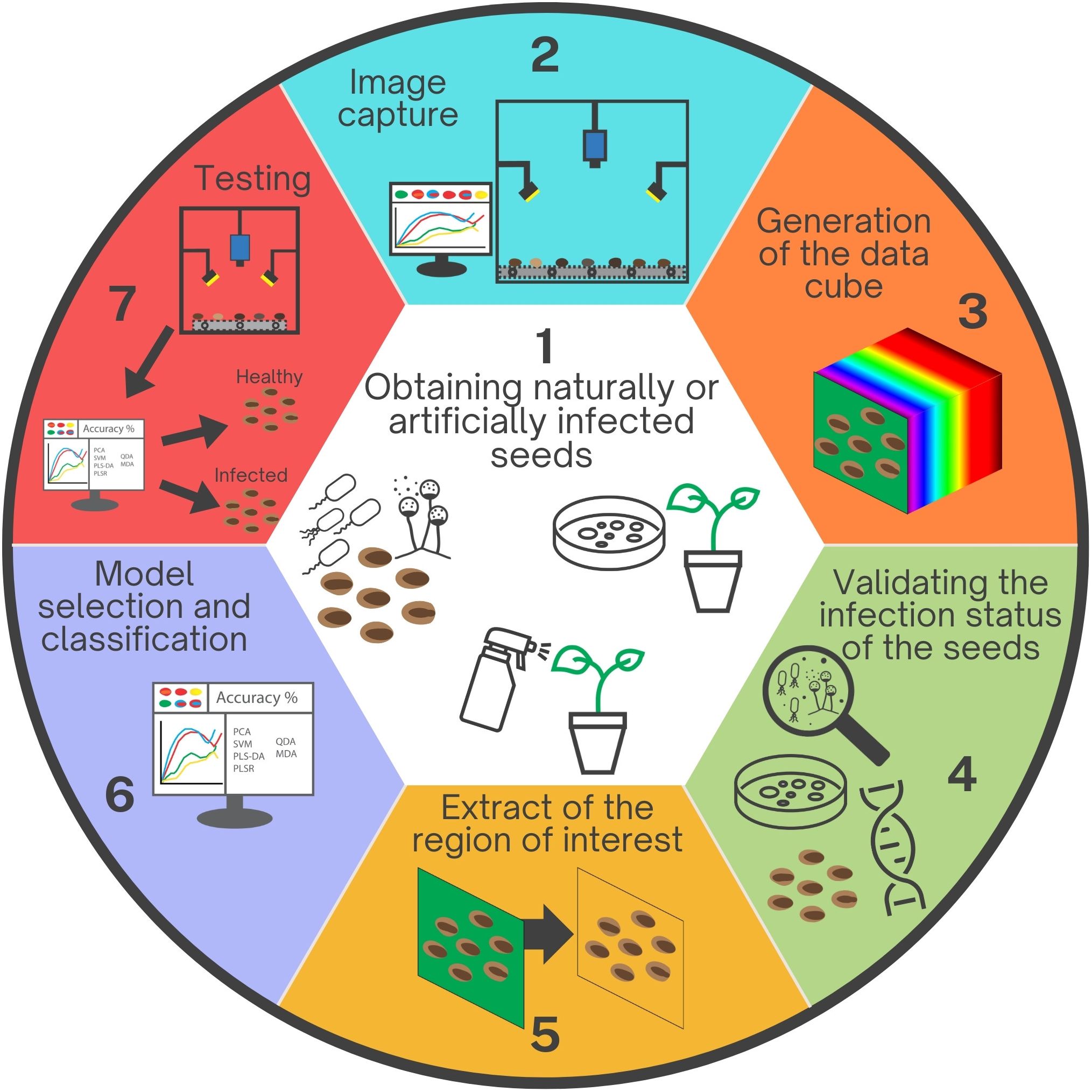
Figure 2 Sequential stages involved in developing hyperspectral imaging for detecting plant pathogens within seeds. 1. Production or acquisition of seeds naturally or artificially infected by inoculating seeds, flowers, or fruits. 2. Image capture utilizing a hyperspectral camera. 3. Data cube generation by integrating multiple wavelength readings. 4. Validation of the infection status of each seed through various detection methods, such as culture media or molecular techniques. 5. Background removal and selection of the region of interest. 6. Selection of machine learning/deep learning models based on the seeds identified in step 4, taking into account the accuracy of each model. 7. Testing the developed protocol and algorithm to validate the technique efficacy.
Hyperspectral imaging studies for seed detection are relatively recent, but they hold significant potential for the development of new protocols, particularly in the context of the prevailing era of robotics and artificial intelligence. The adoption of hyperspectral imaging detection technologies stands to offer substantial advantages in the production of healthy seeds, particularly in terms of enhanced reliability and practicality. This technology holds great promise for certification authorities and has the potential to bolster the confidence of farmers in this agricultural inputs, if a substantial amount of them can be checked for the presence of important seed-borne pathogens.
Author contributions
LF: Data curation, Writing – original draft. IC: Writing – review & editing. LJ: Writing – review & editing. AQ: Writing – review & editing. MR: Conceptualization, Funding acquisition, Supervision, Writing – review & editing.
Funding
The author(s) declare financial support was received for the research, authorship, and/or publication of this article. This research received funding from the Fundação de Apoio à Pesquisa do Distrito Federal (FAPDF), under grant number 00193-00001058/2021-57.
Conflict of interest
The authors declare that the research was conducted in the absence of any commercial or financial relationships that could be construed as a potential conflict of interest.
Publisher’s note
All claims expressed in this article are solely those of the authors and do not necessarily represent those of their affiliated organizations, or those of the publisher, the editors and the reviewers. Any product that may be evaluated in this article, or claim that may be made by its manufacturer, is not guaranteed or endorsed by the publisher.
References
Abdulridha, J., Ampatzidis, Y., Kakarla, S. C., Roberts, P. (2020a). Detection of target spot and bacterial spot diseases in tomato using UAV-based and benchtop-based hyperspectral imaging techniques. Precis. Agric. 21, 955–978. doi: 10.1007/s11119-019-09703-4
Abdulridha, J., Ampatzidis, Y., Qureshi, J., Roberts, P. (2020b). Laboratory and UAV-based identification and classification of tomato yellow leaf curl, bacterial spot, and target spot diseases in tomato utilizing hyperspectral imaging and machine learning. Remote Sens. 12 2732. doi: 10.3390/rs12172732
Afouda, L., Wolf, G., Wydra, K. (2009). Development of a sensitive serological method for specific detection of latent infection of macrophomina phaseolina in cowpea. J. @ Phytopathol. 157, 15–23. doi: 10.1111/j.1439-0434.2008.01453.x
Atkins, S. D., Clark, I. M. (2004). Fungal molecular diagnostics: a mini review. J. Appl. Genet. 45, 3–15.
Bala, A. (2020). “Non-parasitic seed disorders of major agricultural crops,” in Seed-Borne Diseases of Agricultural Crops: Detection, Diagnosis & Management. Eds. Kumar, R., Gupta, A. (Springer Nature Pte Ltd, Singapore), 809–820. doi: 10.1007/978-981-32-9046-4_28
Barbedo, J., Tibola, C., Fernandes, M. (2015). Detecting Fusarium head blight in wheat kernels using hyperspectral imaging. Biosyst. Eng. 131, 65–76. doi: 10.1016/j.biosystemseng.2015.01.003
Bock, C. H., Poole, G. H., Parker, P. E., Gottwald, T. R. (2010). Plant disease severity estimated visually, by digital photography and image analysis, and by hyperspectral imaging. CRC. Crit. Rev. Plant Sci. 29, 59–107. doi: 10.1080/07352681003617285
Bookout, A. L., Cummins, C. L., Mangelsdorf, D. J., Pesola, J. M., Kramer, M. F. (2006). High-throughput real-time quantitative reverse transcription PCR. Curr. Protoc. Mol. Biol. 73, 1–15. doi: 10.1002/0471142727.mb1508s73
Bucksch, A., Burridge, J., York, L. M., Das, A., Nord, E., Weitz, J. S., et al. (2014). Image-based high-throughput field phenotyping of crop roots. Plant Physiol. 166, 470–486. doi: 10.1104/pp.114.243519
Chen, Y. Y., Conner, R. L., Gillard, C. L., Boland, G. J., Babcock, C., Chang, K. F., et al. (2007). A specific and sensitive method for the detection of colletotrichum lindemuthianum in dry bean tissue. Plant Dis. 91, 1271–1276. doi: 10.1094/PDIS-91-10-1271
Choudhary, P., Goswami, S. K., Chakdar, H., Verma, S., Thapa, S., Srivastava, A. K., et al. (2022). Colorimetric loop-mediated isothermal amplification assay for detection and ecological monitoring of Sarocladium oryzae, an important seed-borne pathogen of rice. Front. Plant Sci. 13. doi: 10.3389/fpls.2022.936766
Chu, X., Wang, W., Ni, X., Li, C., Li, Y. (2020). Classifying maize kernels naturally infected by fungi using near-infrared hyperspectral imaging. Infrared Phys. Techn. 105, 103242. doi: 10.1016/j.infrared.2020.103242
Demattê, J. A. M., Fiorio, P. R., Araújo, S. R. (2010). Variation of routine soil analysis when compared with hyperspectral narrow band sensing method. Remote Sens. 2, 1998–2016. doi: 10.3390/rs2081998
Delwiche, S. R., Rodriguez, I. T., Rausch, S. R., Graybosch, R. A. (2019). Estimating percentages of fusarium-damaged kernels in hard wheat by near-infrared hyperspectral imaging. J. Cereal Sci. 87, 18–24. doi: 10.1016/j.jcs.2019.02.008
Dewey, F. M., Thornton, C. R., Gilligan, C. A. (1997). Use of monoclonal antibodies to detect, quantify and visualize fungi in soils. Adv. Bot. Res. 24, 275–308. doi: 10.1016/S0065-2296(08)60076-2
Dhakal, K., Sivaramakrishnan, U., Zhang, X., Belay, K., Oakes, J., Wei, X., et al. (2023). Machine learning analysis of hyperspectral images of damaged wheat kernels. Sensors 23, 3523. doi: 10.3390/s23073523
Dua, Y., Kumar, V., Singh, R. S. (2020). Comprehensive review of hyperspectral image compression algorithms. Opt. Eng. 59, 090902–090902. doi: 10.1117/1.OE.59.9.090902
Dutta, B., Gitaitis, R., Smith, S., Langston, D. (2014). Interactions of seedborne bacterial pathogens with host and non-host plants in relation to seed infestation and seedling transmission. PLoS One 9, e99215. doi: 10.1371/journal.pone.0099215
El-Mesery, H. S., Mao, H., Abomohra, A. E. F. (2019). Applications of non-destructive technologies for agricultural and food products quality inspection. Sensors 2019 19, 846. doi: 10.3390/s19040846
Feng, L., Zhu, S., Liu, F., He, Y., Bao, Y., Zhang, C. (2019). Hyperspectral imaging for seed quality and safety inspection: A review. Plant Methods 15, 1–25. doi: 10.1186/s13007-019-0476-y
Gaur, A., Kumar, A., Kiran, R., Kumari, P. (2020). “Importance of seed-borne diseases of agricultural crops: Economic losses and impact on society,” in Seed-Borne Diseases of Agricultural Crops: Detection, Diagnosis & Management. Eds. Kumar, R., Gupta, A. (Springer Nature Pte Ltd, Singapore), 3–23. doi: 10.1007/978-981-32-9046-4_1
Gitaitis, R., Walcott, R. (2007). The epidemiology and management of seedborne bacterial diseases. Annu. Rev. Phytopathol. 45, 371–397. doi: 10.1146/annurev.phyto.45.062806.094321
Godfray, H. C. J., Crute, I. R., Haddad, L., Lawrence, D., Muir, J. F., Nisbett, N., et al. (2010). The future of the global food system. Philos. Trans. R. Soc Lond. B. Biol. Sci. 365, 2769–2777. doi: 10.1098/rstb.2010.0180
Golhani, K., Balasundram, S. K., Vadamalai, G., Pradhan, B. (2018). A review of neural networks in plant disease detection using hyperspectral data. Inf. Process. Agric. 5, 354–371. doi: 10.1016/j.inpa.2018.05.002
Grewal, R., Kasana, S. S., Kasana, G. (2023). Hyperspectral image segmentation: a comprehensive survey. Multimed. Tools Appl. 82, 20819–20872. doi: 10.1007/s11042-022-13959-w
Ha, Y., Fessehaie, A., Ling, K. S., Wechter, W. P., Keinath, A. P., Walcott, R. R. (2009). Simultaneous detection of Acidovorax avenae subsp. citrulli and Didymella bryoniae in cucurbit seedlots using magnetic capture hybridization and real-time polymerase chain reaction. Phytopathology 99, 666–678. doi: 10.1094/PHYTO-99-6-0666
Hardinge, P., Murray, J. A. H. (2019). Reduced false positives and improved reporting of loop-mediated isothermal amplification using quenched fluorescent primers. Sci. Rep. 9, 1–13. doi: 10.1038/s41598-019-43817-z
Hsieh, T. H., Kiang, J. F. (2020). Comparison of CNN algorithms on hyperspectral image classification in agricultural lands. Sensors 20, 1734. doi: 10.3390/s20061734
Jiang, J., Qiao, X., He, R. (2016). Use of Near-Infrared hyperspectral images to identify moldy peanuts. J. Food Eng. 169, 284–290. doi: 10.1016/j.jfoodeng.2015.09.013
Kale, K. V., Solankar, M. M., Nalawade, D. B., Dhumal, R. K., Gite, H. R. (2017). A research review on hyperspectral data processing and analysis algorithms. Proc. Natl. Acad. sciences India section: Phys. Sci. 87, 541–555. doi: 10.1007/s40010-017-0433-y
Karuppiah, K., Senthilkumar, T., Jayas, D. S., White, N. D. G. (2016). Detection of fungal infection in five different pulses using near-infrared hyperspectral imaging. J. Stored Prod. Res. 65, 13–18. doi: 10.1016/j.jspr.2015.11.005
Kattenborn, T., Leitloff, J., Schiefer, F., Hinz, S. (2021). Review on Convolutional Neural Networks (CNN) in vegetation remote sensing. ISPRS J. Photogramm. Remote Sens. 173, 24–49. doi: 10.1016/j.isprsjprs.2020.12.010
Kaur, S. I., Kashyap, P. L., Kang, S. S., Sharma, A. (2020). “Detection and diagnosis of seed-borne viruses and virus-like pathogens,” in Seed-Borne Diseases of Agricultural Crops: Detection, Diagnosis & Management. Eds. Kumar, R., Gupta, A. (Springer Nature Pte Ltd, Singapore), 169–199. doi: 10.1007/978-981-32-9046-4_7
Kumar, R., Gupta, A. (2020). Seed-borne diseases of agricultural crops: Detection, diagnosis & management (Springer Singapore). doi: 10.1007/978-981-32-9046-4
Kumar, R., Gupta, A., Srivastava, S., Devi, G., Singh, V. K., Goswami, S. K., et al. (2020). “Diagnosis and detection of seed-borne fungal phytopathogens,” in Seed-Borne Diseases of Agricultural Crops: Detection, Diagnosis & Management. Eds. Kumar, R., Gupta, A. (Singapore: Springer Nature Pte Ltd), 107–142. doi: 10.1007/978-981-32-9046-4_5
Lapajne, J., Knapič, M., Žibrat, U. (2022). Comparison of selected dimensionality reduction methods for detection of root-knot nematode infestations in potato tubers using hyperspectral imaging. Sensors 22, 367. doi: 10.3390/s22010367
Lee, H., Kim, M. S., Lim, H. S., Park, E., Lee, W. H., Cho, B. K. (2016a). Detection of cucumber green mottle mosaic virus-infected watermelon seeds using a near-infrared (NIR) hyperspectral imaging system: Application to seeds of the “Sambok Honey” cultivar. Biosyst. Eng. 148, 138–147. doi: 10.1016/j.biosystemseng.2016.05.014
Lee, H., Kim, M. S., Qin, J., Park, E., Song, Y. R., Oh, C. S., et al. (2017a). Raman hyperspectral imaging for detection of watermelon seeds infected with Acidovorax citrulli. Sensors 17, 2188. doi: 10.3390/s17102188
Lee, H., Kim, M. S., Song, Y. R., Oh, C. S., Lim, H. S., Lee, W. H., et al. (2017b). Non-destructive evaluation of bacteria-infected watermelon seeds using visible/near-infrared hyperspectral imaging. J. Sci. Food Agric. 97, 1084–1092. doi: 10.1002/jsfa.7832
Li, J., Hong, D., Gao, L., Yao, J., Zheng, K., Zhang, B., et al. (2022). Deep learning in multimodal remote sensing data fusion: A comprehensive review. Int. J. Appl. Earth Obs. Geoinf. 112, 102926. doi: 10.1016/j.jag.2022.102926
Li, L., Zhang, Q., Huang, D. (2014). A review of imaging techniques for plant phenotyping. Sensors 14, 20078–20111. doi: 10.3390/s141120078
Lowe, A., Harrison, N., French, A. P. (2017). Hyperspectral image analysis techniques for the detection and classification of the early onset of plant disease and stress. Plant Methods 13, 1–12. doi: 10.1186/s13007-017-0233-z
Mancini, V., Murolo, S., Romanazzi, G. (2016). Diagnostic methods for detecting fungal pathogens on vegetable seeds. Plant Pathol. 65, 691–703. doi: 10.1111/ppa.12515
Marcinkowska, J. Z. (2002). Methods of finding and identyfication of pathogens in seeds. Plant Breed. Seed Sci. 46, 31–48.
Martinelli, F., Scalenghe, R., Davino, S., Panno, S., Scuderi, G., Ruisi, P., et al. (2015). Advanced methods of plant disease detection. A review Advanced methods of plant disease detection. A review. Agron. Sustain. De. 35, 1–25. doi: 10.1007/s13593-014-0246-1
Massung, R. F., Slater, K., Owens, J. H., Nicholson, W. L., Mather, T. N., Solberg, V. B. (1998). Nested PCR assay for detection of granulocytic ehrlichiae. J. Clin. Microbiol. 36, 1090–1095. doi: 10.1128/JCM.36.4.1090-1095.1998
Maude, R. (1996). Seedborne diseases and their control: principles and practice (Wallingford, UK: CAB International).
McCartney, H. A., Foster, S. J., Fraaije, B. A., Ward, E. (2003). Molecular diagnostics for fungal plant pathogens. Pest. Manage. Sci. 59, 129–142. doi: 10.1002/ps.575
Melgani, F., Bruzzone, L. (2004). Classification of hyperspectral remote sensing images with support vector machines. IEEE Trans. Geosci. Remote Sens. 42, 1778–1790. doi: 10.1109/TGRS.2004.831865
Mishra, P., Asaari, M. S. M., Herrero-Langreo, A., Lohumi, S., Diezma, B., Scheunders, P. (2017). Close range hyperspectral imaging of plants: A review. Biosyst. Eng. 164, 49–67. doi: 10.1016/j.biosystemseng.2017.09.009
Mishra, P., Polder, G., Vilfan, N. (2020). Close range spectral imaging for disease detection in plants using autonomous platforms: a review on recent studies. Curr. Robot. Rep. 1, 43–48. doi: 10.1007/s43154-020-00004-7
Montes, J. M., Melchinger, A. E., Reif, J. C. (2007). Novel throughput phenotyping platforms in plant genetic studies. Trends Plant Sci. 12, 433–436. doi: 10.1016/j.tplants.2007.08.006
Mookambiga, A., Gomathi, V. (2016). Comprehensive review on fusion techniques for spatial information enhancement in hyperspectral imagery. Multidimens. Syst. Signal Process. 27, 863–889. doi: 10.1007/s11045-016-0415-2
Mullis, K., Faloona, F., Scharf, S., Saiki, R., Horn, G., Erlich, H. (1986). Specific enzymatic amplification of DNA in vitro: the polymerase chain reaction. Cold Spring Harb. Symp. Quant. Biol. 51, 263–273. doi: 10.1101/SQB.1986.051.01.032
Munkvold, G. P. (2009). Seed pathology progress in academia and industry. Annu. Rev. Phytopathol. 47, 285–311. doi: 10.1146/annurev-phyto-080508-081916
Nagamine, K., Hase, T., Notomi, T. (2002). Accelerated reaction by loop-mediated isothermal amplification using loop primers. Mol. Cell. Probes 16, 223–229. doi: 10.1006/mcpr.2002.0415
Nazarenko, I., Lowe, B., Darfler, M., Ikonomi, P., Schuster, D., Rashtchian, A. (2002). Multiplex quantitative PCR using self-quenched primers labeled with a single fluorophore. Nucleic Acids Res. 30, 37. doi: 10.1093/nar/30.9.e37
Nguyen, G., Dlugolinsky, S., Bobák, M., Tran, V., García, A. L., Heredia, I., et al. (2019). Machine Learning and Deep Learning frameworks and libraries for large-scale data mining: a survey. Artif. Intell. Rev. 52, 77–124. doi: 10.1007/s10462-018-09679-z
Notomi, T., Okayama, H., Masubuchi, H., Yonekawa, T., Watanabe, K., Amino, N., et al. (2000). Loop-mediated isothermal amplification of DNA. Nucleic Acids Res. 28, 63. doi: 10.1093/nar/28.12.e63
Oerke, E. C., Dehne, H. W. (2004). Safeguarding production—losses in major crops and the role of crop protection. Crop Prot. 23, 275–285. doi: 10.1016/j.cropro.2003.10.001
Oliveira, M. R. R. D., Ribeiro, S. G., Mas, J. F., Teixeira, A. D. S. (2021). Advances in hyperspectral sensing in agriculture: a review. Cienc. Agron. 51, e20207739. doi: 10.5935/1806-6690.20200096
Pedrozo, R., Little, C. R. (2017). Fusarium verticillioides inoculum potential influences soybean seed quality. Eur. J. Plant Pathol. 148, 749–754. doi: 10.1007/s10658-016-1127-z
Peñuelas, J., Filella, I. (1998). Visible and near-infrared reflectance techniques for diagnosing plant physiological status. Trends Plant Sci. 3, 151–156. doi: 10.1016/S1360-1385(98)01213-8
Qiao, X., Jiang, J., Qi, X., Guo, H., Yuan, D. (2017). Utilization of spectral-spatial characteristics in shortwave infrared hyperspectral images to classify and identify fungi-contaminated peanuts. Food Chem. 220, 393–399. doi: 10.1016/j.foodchem.2016.09.119
Robène, I., Perret, M., Jouen, E., Escalon, A., Maillot, M. V., Chabirand, A., et al. (2015). Development and validation of a real-time quantitative PCR assay to detect Xanthomonas axonopodis pv. allii from onion seed. J. Microbiol. Methods 114, 78–86. doi: 10.1016/j.mimet.2015.04.017
Robène-Soustrade, I., Legrand, D., Gagnevin, L., Chiroleu, F., Laurent, A., Pruvost, O. (2010). Multiplex Nested PCR for Detection of Xanthomonas axonopodis pv. allii from Onion Seeds. Appl. Environ. Microbiol. 76, 2697. doi: 10.1128/AEM.02697-09
Sankaran, S., Mishra, A., Ehsani, R., Davis, C. (2010). A review of advanced techniques for detecting plant diseases. Comput. Electron. Agric. 72, 1–13. doi: 10.1016/j.compag.2010.02.007
Savary, S., Willocquet, L., Pethybridge, S. J., Esker, P., McRoberts, N., Nelson, A. (2019). The global burden of pathogens and pests on major food crops. Nat. Ecol. Evol. 3, 430–439. doi: 10.1038/s41559-018-0793-y
Schaad, N. W., Cheong, S. S., Tamaki, S., Hatziloukas, E., Panopoulos, N. J. (1995). A combined biological and enzymatic amplification (BIO-PCR) technique to detect Pseudomonas syringae pv. phaseolicola in bean seed extracts. Phytopathology 85, 243–248. doi: 10.1094/Phyto-85-243
Schena, L., Nigro, F., Ippolito, A., Gallitelli, D. (2004). Real-time quantitative PCR: A new technology to detect and study phytopathogenic and antagonistic fungi. Eur. J. Plant Pathol. 110, 893–908. doi: 10.1007/s10658-004-4842-9
Senthilkumar, T., Jayas, D. S., White, N. D. G. (2015). Detection of different stages of fungal infection in stored canola using near-infrared hyperspectral imaging. J. Stored Prod. Res. 63, 80–88. doi: 10.1016/j.jspr.2015.07.005
Senthilkumar, T., Jayas, D. S., White, N. D. G., Fields, P. G., Gräfenhan, T. (2016). Detection of fungal infection and Ochratoxin A contamination in stored barley using near-infrared hyperspectral imaging. Biosyst. Eng. 147, 162–173. doi: 10.1016/j.biosystemseng.2016.03.010
Seo, Y., Lee, H., Bae, H. J., Park, E., Lim, H. S., Kim, M. S., et al. (2019). Optimized multivariate analysis for the discrimination of cucumber green mosaic mottle virus-infected watermelon seeds based on spectral imaging. Biosyst. Eng. 44, 95–102. doi: 10.1007/s42853-019-00019-9
Serranti, S., Cesare, D., Bonifazi, G. (2013). The development of a hyperspectral imaging method for the detection of Fusarium-damaged, yellow berry and vitreous Italian durum wheat kernels. Biosyst. Eng. 115, 20–30. doi: 10.1016/j.biosystemseng.2013.01.011
Shahin, M. A., Symons, S. J. (2011). Detection of Fusarium damaged kernels in Canada Western Red Spring wheat using visible/near-infrared hyperspectral imaging and principal component analysis. Comput. Electron. Agric. 75, 107–112. doi: 10.1016/j.compag.2010.10.004
Shanmugapriya, P., Rathika, S., Ramesh, T., Janaki, P. (2019). Applications of remote sensing in agriculture - A review. Int. J. Curr. Microbiol. Appl. Sci. 8, 2270–2283. doi: 10.20546/ijcmas.2019.801.238
Singh, C. B., Jayas, D. S., Paliwal, J., White, N. D. G. (2007). Fungal detection in wheat using near-infrared hyperspectral imaging. Trans. ASABE 50, 2171–2176. doi: 10.13031/2013.24077
Siripatrawan, U., Makino, Y. (2015). Monitoring fungal growth on brown rice grains using rapid and non-destructive hyperspectral imaging. Int. J. Food Microbiol. 199, 93–100. doi: 10.1016/j.ijfoodmicro.2015.01.001
Tekle, S., Mage, I., Segtnan, V. H., Bjornstad, A. (2015). Near-infrared hyperspectral imaging of fusarium-damaged oats (Avena sativa L.). Cereal Chem. 92, 73–80. doi: 10.1094/CCHEM-04-14-0074-R
Tilman, D., Balzer, C., Hill, J., Befort, B. L. (2011). Global food demand and the sustainable intensification of agriculture. Proc. Natl. Acad. Sci. U.S.A. 108, 20260–20264. doi: 10.1073/pnas.1116437108
Walcott, R. R. (2003). Detection of seedborne pathogens. Horttechnology 13, 40–47. doi: 10.21273/HORTTECH.13.1.0040
Whetton, R. L., Hassall, K. L., Waine, T. W., Mouazen, A. M. (2018). Hyperspectral measurements of yellow rust and fusarium head blight in cereal crops: Part 1: Laboratory study. Biosyst. Eng. 166, 101–115. doi: 10.1016/j.biosystemseng.2017.11.008
Williams, P., Manley, M., Fox, G., Geladi, P. (2010). Indirect Detection of Fusarium Verticillioides in Maize (Zea mays L.) Kernels by near Infrared Hyperspectral Imaging. J. Near Infrared Spectroc. 18, 49–58. doi: 10.1255/jnirs.858
Wu, N., Jiang, H., Bao, Y., Zhang, C., Zhang, J., Song, W., et al. (2020). Practicability investigation of using near-infrared hyperspectral imaging to detect rice kernels infected with rice false smut in different conditions. Sens. Actuators B Chem. 308, 127696. doi: 10.1016/j.snb.2020.127696
Wu, N., Weng, S., Xiao, Q., Jiang, H., Zhao, Y., He, Y. (2024). Rapid and accurate identification of bakanae pathogens carried by rice seeds based on hyperspectral imaging and deep transfer learning. Spectrochim Acta A Mol. Biomol. Spectrosc. 311, 123889. doi: 10.1016/j.saa.2024.123889
Wu, Q., Xu, L., Zou, Z., Wang, J., Zeng, Q., Wang, Q., et al. (2022). Rapid nondestructive detection of peanut varieties and peanut mildew based on hyperspectral imaging and stacked machine learning models. Front. Plant Sci. 13. doi: 10.3389/fpls.2022.1047479
Xie, B., Chen, B., Ma, J., Chen, J., Zhou, Y., Han, X., et al. (2024). Rapid identification of Choy sum seeds infected with Penicillium decumbens based on hyperspectral imaging and stacking ensemble learning. Food Anal. Methods 17, 1–10. doi: 10.1007/s12161-024-02574-0
Xie, Y., Sha, Z., Yu, M. (2008). Remote sensing imagery in vegetation mapping: a review. J. Plant Ecol. 1, 9–23. doi: 10.1093/jpe/rtm005
Zhang, N., Yang, G., Pan, Y., Yang, X., Chen, L., Zhao, C. (2020). A review of advanced technologies and development for hyperspectral-based plant disease detection in the past three decades. Remote Sens 12, 3188. doi: 10.3390/rs12193188
Žibrat, U., Gerič Stare, B., Knapič, M., Susič, N., Lapajne, J., Širca, S. (2021). Detection of root-knot nematode meloidogyne luci infestation of potato tubers using hyperspectral remote sensing and real-time pcr molecular methods. Remote Sens 13, 1996. doi: 10.3390/rs13101996
Keywords: artificial intelligence, phytopathogen, machine learning, seedborne, bacteria, fungus, virus, nematode
Citation: Ferreira LdC, Carvalho ICB, Jorge LAdC, Quezado-Duval AM and Rossato M (2024) Hyperspectral imaging for the detection of plant pathogens in seeds: recent developments and challenges. Front. Plant Sci. 15:1387925. doi: 10.3389/fpls.2024.1387925
Received: 18 February 2024; Accepted: 27 March 2024;
Published: 11 April 2024.
Edited by:
Jakub Nalepa, Silesian University of Technology, PolandReviewed by:
Leiqing Pan, Nanjing Agricultural University, ChinaCopyright © 2024 Ferreira, Carvalho, Jorge, Quezado-Duval and Rossato. This is an open-access article distributed under the terms of the Creative Commons Attribution License (CC BY). The use, distribution or reproduction in other forums is permitted, provided the original author(s) and the copyright owner(s) are credited and that the original publication in this journal is cited, in accordance with accepted academic practice. No use, distribution or reproduction is permitted which does not comply with these terms.
*Correspondence: Maurício Rossato, bWF1cmljaW8ucm9zc2F0b0B1bmIuYnI=