- 1Institute of Bioinformatics and Institute of Crop Science, College of Agriculture and Biotechnology, Zhejiang University, Hangzhou, Zhejiang, China
- 2Key Laboratory of Tobacco Biotechnological Breeding, National Tobacco Genetic Engineering Research Center, Yunnan Academy of Tobacco Agricultural Sciences, Kunming, Yunnan, China
- 3Department of Biostatistics, University of Florida, Gainesville, FL, United States
Tobacco (Nicotiana tabacum L.) use is the leading cause of preventable death, due to deleterious chemical components and smoke from tobacco products, and therefore reducing harmful chemical components in tobacco is one of the crucial tobacco breeding targets. However, due to complexity of tobacco smoke and unavailability of high-density genetic maps, the genetic architecture of representative hazardous smoke has not been fully dissected. The present study aimed to explore the genetic architecture of nine hazardous component traits of mainstream smoke through QTL mapping using 271 recombinant inbred lines (RILs) derived from K326 and Y3 in multiple environments. The analysis of genotype and genotype by environment interaction (GE) revealed substantially greater heritability over 95% contributed mostly by GE interaction effects. We also observed strong genetic correlations among most studied hazardous smoke traits, with the highest correlation coefficient of 0.84 between carbon monoxide and crotonaldehyde. Based on a published high-density genetic map, a total of 19 novel QTLs were detected for eight traits using a full QTL model, of which 17 QTLs showed significant additive effects, six showed significant additive-by-environment interaction effects, and one pair showed significant epistasis-by-environment interaction effect. Bioinformatics analysis of sequence in QTL region predicted six genes as candidates for four traits, of which Nt21g04598.1, Nt21g04600.1, and Nt21g04601.1 had pleiotropic effects on PHE and TAR.
Introduction
Tobacco smoking is the world’s leading cause of avoidable premature mortality (World Health Organization, 2023). Cigarette smoke is a complex, dynamic and reactive mixture that consists of more than 8000 chemical compounds (Rodgman and Green, 2003). When a cigarette is lit, the hot carbonaceous coal within the burning cigarette can reach peak temperatures exceeding 900°C during a puff (McAdam et al., 2016). Adjacent to the hot coal, thermolytic processes (including distillation, pyrolysis and combustion) act on the components of the tobacco to form various smoke constituents, which are released as mainstream smoke (Schwanz et al., 2019).
Due to complexity of tobacco smoke, the mechanism underlying harmfulness of smoke has not been fully understood. Therefore, various lists of toxicants have been proposed in an effort to identify the most relevant constituents responsible for smoking-related diseases (Smith and Hansch, 2000; Liu et al., 2012), including lists of analytes from Hoffman (Hoffmann et al., 1997, 2001), Rodgman and Green (Rodgman and Green, 2003), Talhout (Talhout et al., 2011) and FDA (Food and Drug Administration, 2012). Based on the previous lists and toxicological test methods, a simplified evaluation system was established by Xie et al. (Xie et al., 2009). Xie and his colleagues analyzed 29 hazardous constituents in smoke and four pharmacologic indexes for 163 cigarette samples sold in China. Through statistical analysis, seven smoke constituents, including hydrogen cyanide (HCN), ammonia (NH3), phenol (PHE), benzo[α]pyrene (B[a]P), carbon monoxide (CO), crotonaldehyde (CRO), and 4-(methylnitrosamino)-1-(3-pyridyl)-1-butanone (NNK), were selected out to establish a novel hazard index (HI). In addition, given the extreme harm of tar (TAR) in mainstream cigarette smoke to human health, tobacco industry has integrated TAR and HI for comprehensive assessments of hazardous smoke. Efforts are being made to minimize harm, with a focus on tar and the seven representative harmful substances.
There have been reports on the emission of smoke toxicants attributable to several factors such as the variety of chemical compositions in tobacco leaf and the reduction of harmful chemical components within the tobacco leaf is considered as a critical objective in tobacco breeding initiatives (Julio et al., 2006). However, little is currently known about the genetic architecture underlying these smoke-related traits which are considered as quantitative traits. Julio (Julio et al., 2006) was the first to show interest in quantitative trait locus (QTL) mapping and several QTLs of smoke properties (tar, benzo[α]pyrene and CO) were detected in a recombinant inbred line (RIL) population with a partial genetic map. No novel QTL was reported until Tong’s study (Tong et al., 2021) for seven smoke substances, including benzo[a]pyrene, hydrocyanic acid, phenol, carbon monoxide, tar, nicotine and total particle matter, using a high-density genetic map constructed by single nucleotide polymorphisms (SNPs) in RIL population, mapping more QTLs on smoke hazardous components and understanding their interactions with environments is still necessary for efficient molecular genetic improvement of the traits. In this study, based on an integrated high-density linkage map and multi-environment phenotypic data of the RIL population, QTL mapping was conducted for nine hazardous smoke traits; the detected main-effect QTLs, epistastic QTLs and their interactions with environments will provide more insights into the genetic architecture of the traits and greatly facilitate the molecular improvements of breeding low-hazard tobacco varieties.
Materials and methods
Plant materials and field trial
The RILs were generated from two elite flue-cured tobacco parents Y3 and K326. Y3 is a backbone cultivated variety that originated from Zimbabwe with elite agronomic traits and complicated parental sources. K326, whose genome has been assembled (Edwards et al., 2017), was introduced from America with high commercial quality and disease resistance but moderate agronomic performance. A total of 274 RILs were employed in this study, consisting of two parents, one F1 generation hybrid (YKF1; Y3 K326) and 271 F7 generation individuals. The materials were planted at Shilin (N: 23.46; E: 103.17) field experiment stations using complete random design with 5 replications, and were cultivated according to local technical measures for quality tobacco production. The eight hazardous substances, including benzo[α]pyrene (B[a]P), carbon monoxide (CO)¸ crotonaldehyde (CRO), hydrogen cyanide (HCN), ammonia (NH3), 4-(methylnitrosamino)-1-(3-pyridyl)-1-butanone (NNK), phenol (PHE) and tar (TAR) and were collected in the mainstream smoke of cigarettes produced using tobacco planted at Shilin in 2018, 2019 and 2020; and calculated hazard index (HI). Three combinations of location and year were treated as environments denoted as E1 (2018 Shilin), E2 (2019 Shilin) and E3 (2020 Shilin).
Measurement and calculation of nine hazard constituents in mainstream smoke
B[a]P was analyzed using the gas chromatography mass spectrometry (GC-MS) method, as described by the Chinese standard method GB/T 21130-2007 (Standardization Administration of China, 2007). CO was determined in the vapor phase using a non-dispersive infrared analyzer, as described by the Chinese standard method GB/T 23356-2009 (Standardization Administration of China, 2009). CRO was analyzed using the high-performance liquid chromatography (HPLC) method, as described by the Chinese tobacco industry standard method YC/T 254–2008 (Standardization Administration of China, 2008a). HCN was quantified using Ion Chromatography, the method as described by the Chinese tobacco industry standard method YC/T 403–2011 (Standardization Administration of China, 2011). NH3 was analyzed using Ion Chromatography, the method as described by the Chinese tobacco industry standard method YC/T 377–2017 (Standardization Administration of China, 2017). NNK analysis was carried out using GC-TEA method, as described by the Chinese standard method GB/T 23228-2008 (Standardization Administration of China, 2008b). PHE was analyzed using HPLC, the method as described by the Chinese tobacco industry standard method YC/T 255–2008 (Standardization Administration of China, 2008c). TAR was analyzed using a routine analytical smoking machine, as described by the Chinese standard method GB/T 19609-2004 (Standardization Administration of China, 2004). HI was calculated by the following formula, (Xie et al., 2009), where represents emission level of CO (mg/cigarette), for HCN (μg/cigarette), for NNK (ng/cigarette), for NH3 (μg/cigarette), for B[a]P (ng/cigarette), for PHE (μg/cigarette) and for CRO (μg/cigarette). Three biological replicates were used for each assessment.
Statistical analysis of phenotypes
Variance components analysis and heritability estimation were performed based on the following linear model,
where is the phenotypic value of the i-th replication of the -th line in the -th environment; is the population mean; is the genotypic value of the -th genotype, random effect, ; is the effect of the -th environment, random, ; is the interaction effect between the -th genotype and the -th environment, random, ; is the residual effect of the individual, random, .
The mmer module of sommer R package (Covarrubias-Pazaran, 2016) was applied to estimate the variances of random effects () and to predict the random effects by BLUPs (best linear unbiased predictions, ) by solving the mixed model equation (MME). Heritability was estimated with the formula and , where was the estimated genotypic variance, was the estimated variance due to gene-by-environment interaction, and was the estimated residual variance. The rcorr module of Hmisc R package (https://cran.r-project.org/web/packages/Hmisc/index.html) was employed to calculate Pearson correlation coefficients between six studied traits: (1) phenotypic correlation coefficients with for each environment, respectively; (2) genetic correlation coefficients with . (, where is the adjusted genotypic value of the -th line by the environment effects, is the estimated population mean, and is the genotypic value of the -th line predicted by BLUP).
Genetic linkage map
The high-resolution linkage map (Tong et al., 2023) that contained 46,324 markers, which were classified into 7,107 bins, a group of markers with least genotype missing rate and same genetic distance, distributed on the 24 linkage groups (LGs) and covered 3334.88 cM with an average genetic distance of 0.469 cM, was employed in this study.
Genetic model and statistical methods for QTL mapping
A QTL full model was adopted for modeling the genetic architecture of complex traits from multi-environment trials, which includes additive effect (a) of each QTL, additive-by-additive epistatic effect (aa) of each pair of epistatic QTL, treated as fixed effects, and their corresponding environment interaction effects (ae and aae) as random effects. Suppose a trait is controlled by segregating QTLs, of which pairs of QTLs are involved in epistasis. Then, the phenotypic value of the m-th replication of the -th genotype in the -th environment () can be expressed by the following mixed linear model (Yang et al., 2007),
where, is the population mean; is the additive effect of the-th QTL with indicator variable , fixed effect; is the additive-by-additive epistatic effect of the -th QTL and the -th QTL with indicator variable , fixed effect; is the effect of the -th environment, random effect, ; is the additive-by-environment interaction effect of the -th QTL and the -th environment with observation (), random effect, ; is the interaction effect of the and the -th environment with observation (), random effect, ; and is the residual effect of the individual, random, .
QTLNetwork 2.0 software were employed to detect QTLs by the mixed-linear-model-based composite interval mapping (MCIM) method (Yang et al., 2008). One- and two-dimensional genome scans for QTLs were performed with configurations of 10 cM testing window, 1 cM walking step and 10 cM filtration window size. To control the experiment-wise type I error rate, a critical F-value based on the Henderson III method was determined by the permutation test with 1,000 times for each tested locus at the significance level of 0.05. Based on the significant QTL, a QTL full model was established and used to estimate each parameter based on the samples generated by Markov chain Monte Carlo (MCMC) with 20,000 Gibbs sampler iterations.
Candidate genes prediction
The physical positions of the marker interval with QTL were determined using Nucleotide BLAST module of NCBI (https://blast.ncbi.nlm.nih.gov/Blast.cgi), which utilized sequence information of two adjacent bin markers in the linkage map. Variants including SNPs and Indels located within the QTL regions were selected for subsequent filtration. These variants were annotated by software SnpEff (http://pcingola.github.io/SnpEff/) based on K326 reference genome (https://solgenomics.net/ftp/genomes/Nicotiana_tabacum/edwards_et_al_2017/assembly/Nitab-v4.5_genome_Chr_Edwards2017.fasta ), and those showing HIGH or MODERATE impact on related protein effectiveness were retained, according to annotation results. Then, the eligible variants with P value less than 0.05 were identified by performing single-marker regressions with as response variable (as mentioned in the Statistical analysis of phenotypes). Before performing the enrichment analysis of genes with variants above, the protein sequences of the K326 reference genome (https://solgenomics.net/ftp/genomes/Nicotiana_tabacum/edwards_et_al_2017/annotation/Nitab-v4.5_proteins_Edwards2017.fasta) should be uploaded to the eggNOG-mapper website (http://eggnog-mapper.embl.de/) for functional annotation. Gene Ontology (GO) and KEGG pathway enrichment analyses were carried out using the clusterProfiler R package (https://github.com/YuLab-SMU/clusterProfiler) as criteria for predicting candidate genes.
Results
Phenotypic performance of nine smoke-related traits
For the nine smoke-related traits, the estimated heritability of genetic effects ranged from 11.03% for CRO to 20.00% for CO, displaying a limited stability across environments for these traits (Table 1). Furthermore, gene-by-environment effects were found to contribute significantly more to phenotypic variation with most estimated interaction heritability () nearly over 80%, indicating selection of these traits should design specific strategy for different environment conditions. Most phenotypic correlations of the traits were positive and reached statistical significance (), for example, with coefficients over 0.8 between CO and CRO in three environments (Figures 1A-C). Nevertheless, strong negative correlations were observed between NNK and other three constituents: B[a]P, CO, and CRO, while, weak negative correlations between B[a]P and PHE, and between NNK and TAR. In general, the phenotypic correlations in three environments showed similar pattern as well as the genetic correlations (Figure 1D), indicating a strong and reliable underlying genetic relationship among these traits that make further exploration necessary. However, inconsistencies in the performance of the traits across three environments could be observed, with E2 exhibiting a higher frequency of exceptions. For instance, the phenotypic correlations between CO and PHE were significantly positive in E1 (Figure 1A) and E3 (Figure 1C), but significantly negative in E2 (Figure 1B). The genetic correlation coefficients, calculated using the estimated genotypic values, exhibited the highest correlation of 0.84 between CO and CRO, followed by 0.79 between NH3 and HI, and several other strong correlations above 0.7, such as NH3 and PHE (Figure 1D). The existence of high underlying genetic correlations between these traits might help to explain their strong phenotypic correlations. Further, correlations between HI and the other traits were all displayed significantly positive except B[a]P, which is consistent with the fact that HI was a composite index comprising seven representative smoke traits.
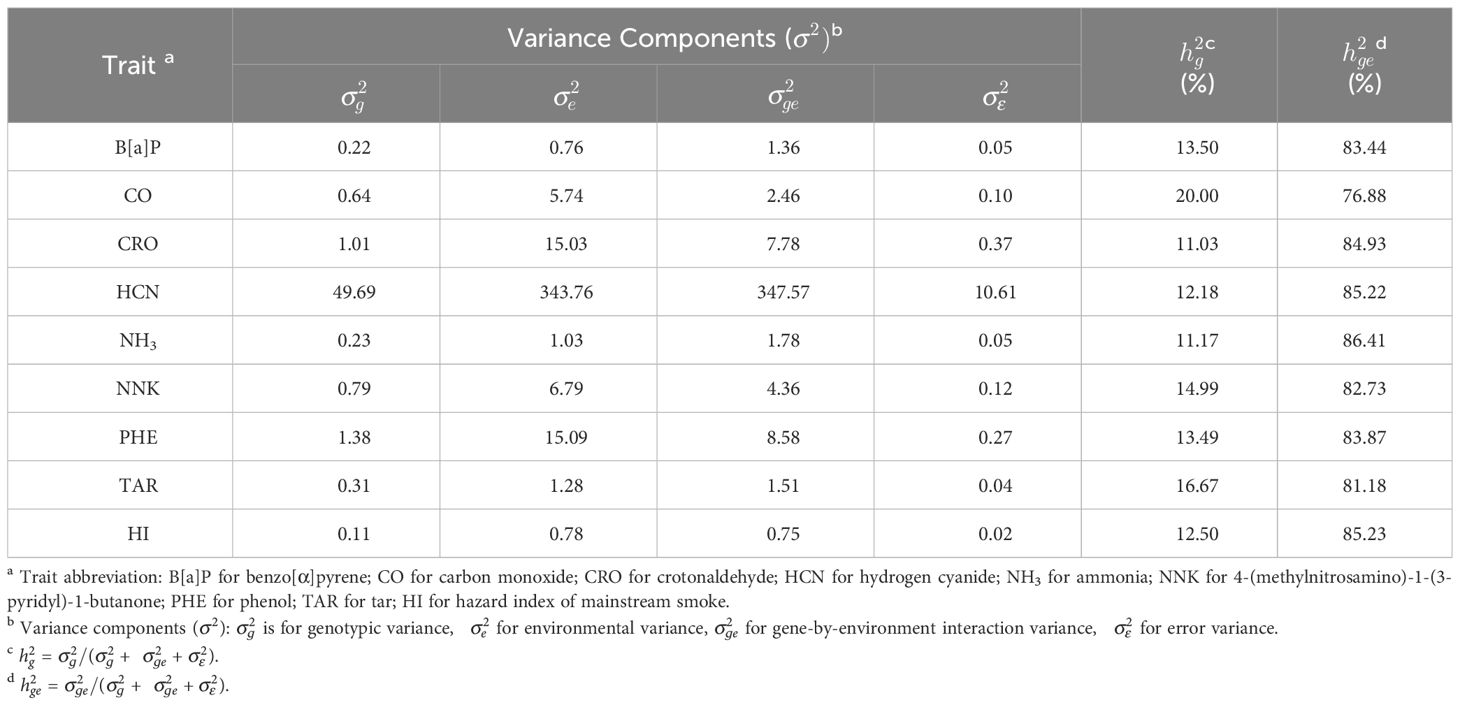
Table 1 Variance components analysis and estimated heritability (%) of genetic effects for smoke-related traits.
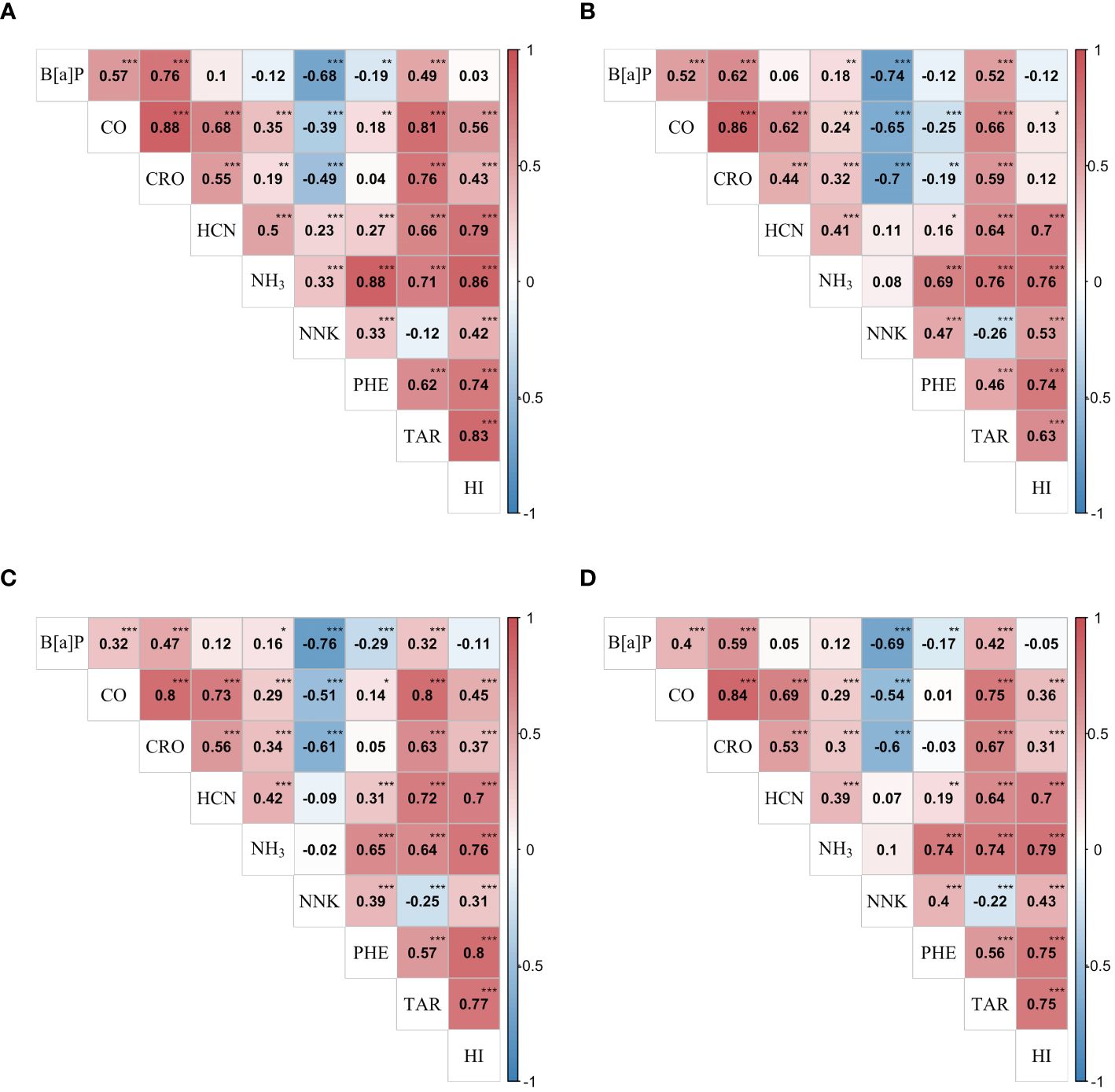
Figure 1 Phenotypic and genetic correlations between studied traits in the RIL population. Heat map (A–C) showed phenotypic correlation coefficients between nine traits in E1 (2018 Shilin), E2 (2019 Shilin), E3 (2020 Shilin) in turn, the heat map (D) showed genetic correlation coefficients between nine traits. *, **, *** denote significance level at 0.05, 0.01 and 0.001, respectively. Traits abbreviations are same as those in Table 1.
Additive and additive-by-environment interaction effects
A total of 19 QTLs were identified responsible for the nine smoke-related traits. Among them, NH3 had four QTLs; CO, NNK, PHE and TAR each had three QTLs; CRO, HCN and HI each only detected one QTL (Table 2). These QTLs distributed on seven linkage groups (LGs), with LG15 containing the highest number of QTLs (7 QTLs), followed by LG6 (6 QTLs) and LG1 (2 QTLs), while each of LG4, LG5, LG10 and LG19 only harbored one QTL.
It is noteworthy that qPHE1 and qTAR1 located in the same marker interval with flanking marker PT61201 and SNP_0912209_288, similarly, qPHE6 and qTAR6 in the same marker interval ranged by SNP_0002499_215080 and SNP_0011326_16206, qNH315-2 and qTAR15 in the same interval ranged by SNP_0002539_133 and SNP_0000535_1549 (Table 2). These co-location indicated the potential pleiotropic effect of QTL on PHE and TAR or on NH3 and TAR, which requires further investigation for revealing the molecular mechanism of genetic correlation between traits. Our inference on existence of pleiotropic QTL was enhanced by the significant and relatively high genetic correlations estimated between the studied traits (Figure 1), which were 0.74 between NH3 and TAR, and 0.56 between PHE and TAR as we mentioned above.
Totally, 17 QTLs with additive (a) main effects were detected for nine traits, of which six QTLs also showed additive-by-environment interaction (ae) effects (Table 3). Most of the QTLs exhibited small additive effects, which were regarded as minor-effect QTLs and accounted for approximately 2% phenotypic variance. The average proportion of phenotypic variance explained by individual QTL () was 2.28%. Moreover, around 70% of all QTLs each explained less than 3% of the phenotypic variance, the rest QTLs taking relatively larger effects on five traits, qHCN6 (=4.99%), qHI6 (=3.39%), qTAR6 (=3.14%), qCO15 (=3.22%), qNH315-2 (=3.21%), located mainly on LG6 and LG15. Most QTLs, whose homozygous genotypes of the alleles from the parent K326 (QQ), contributed positive additive effects (i.e., increasing the trait value) (Table 4); in contrast, the other QTL genotype with alleles from the parent Y3 (qq) contributed negative additive effects.
Significant additive by environment interaction effects (ae) were found for less than half of QTLs, and their contributions to phenotypic variation () were around 1%, with most being lower than their corresponding additive effects. However, there was an exception that the interaction between qNNK6 and two environments (E1, E2) accounted for 1.17% of the phenotypic variance, which was higher than 0.80% explained by corresponding additive effects. Moreover, the ae effects of QTL could take same or opposite effect direction as their main effects (a), and also showed different effect direction across environments. However, the ae effects of different QTLs for same trait may show consistent effect direction in same environment. For example, the ae effects of qNNK6 and qNNK15-1 both contributed negative effects in E1. It is widely recognized that QTLs with no significant ae effects have crucial application potential in breeding new varieties with strong environment adaptability. Of significance, the absence of ae effects of QTLs for HCN, NH3, TAR, and HI suggested that these four traits may exhibit stable performance across various environments.
Additive-by-additive epistasis and epistasis-by-environment interaction effects
Two-dimensional genome scan found a digenic epistatic QTL pair (qNH35- qNH310) with epistasis-by-environment interaction effects (aae) for NH3, but no paired epistatic QTLs for other traits (Table 3). This epistatic QTLs didn’t contribute additive-additive epistatic effects (aa), only positive aae effects in E1 and negative in E2, accounting for 1.51% of total phenotypic variation. Additionally, the epistatic QTL pair qNH35 on LG5 and qNH310 on LG10, each had no individual additive effect.
Overall, environmental effect (E) explained the largest part of the phenotypic variance which ranged from 26.23% to 53.83%, followed by genetic main effect (G) and gene-by-environment interaction effect (GE) (Supplementary Table S1). For all traits, the G effects, which were entirely composed of additive effects of one to three QTLs, explained 1.04% to 8.00% of the phenotypic variance. Besides, for CO, CRO, NH3, NNK, PHE, the contribution of GE to the total variation was small, with some in the form of ae effects and others in the form of aae effects. In summary, our analysis showed that these traits were primarily controlled by a single major gene or polygenes.
Prediction of superior genotype for smoke-related traits
To explore the potential of the identified QTLs in improving smoke-related traits through genetic and molecular manipulation, we undertook QTL genotype design and assessed the trait values potentially achieved by the general superior homozygous line (GSL(-)) and the superior homozygous line for each year (SL(-)) based on the genetic effects of these QTLs. Notably, for the HCN, TAR, and HI, there are no QTL involved in interaction with environment, the designed superior genotypes GSL(-) and SL(-) of each trait remained consistent across all environments (Table 4). On the CO, CRO, NH3, NNK, and PHE, although they were influenced by gene-environment effects, their superior lines (GSL, SL) have identical homozygous genotypes for each trait across three environments, respectively, except the SL(-)1 with QQ at qNNK6 for NNK. In particular, the superior lines of HCN, NNK and PHE were constructed by all homozygous genotypes with alleles from the parent Y3 (qq) at QTLs except qNNK6 for NNK, which indicated that the pyramid of genes from Y3 at these QTLs can effectively decrease the levels of hazardous smoke constituents, HCN, NNK, and PHE. Meanwhile, this advantageous genotype remained valid under all environments. This discovery provided valuable indication for efficient simultaneous improvements of HCN, NNK, and PHE by utilization of elite genes in Y3.
Enrichment analysis and prediction of candidate gene for smoke-related traits
Annotation of variants (SNP/Indel) was conducted using SnpEff based on the K326 reference genome, and 559,513 variants were identified within the putative physical positions of the 17 additive-QTL regions. Among these, only variants with a HIGH or MODERATE impact on protein function were retained, as they were considered potentially functional in candidate genes, as a result, 5,268 variants were selected in total. Then simple regression analysis of the predicted genetic values on these variants was performed, and 600 significant variants in 76 genes were selected () (Supplementary Table S2). These prioritized genes demonstrated enrichment in five GO biological processes, four GO molecular functions, and two KEGG pathways. Specifically, the enrichment was observed in cellular processes (biological process), catalytic activity (molecular function), and signaling molecules metabolic pathways (Table 5). For instance, “callose deposition in cell wall”, “polysaccharide localization” and “callose localization” were associated with carbohydrate biosynthesis.
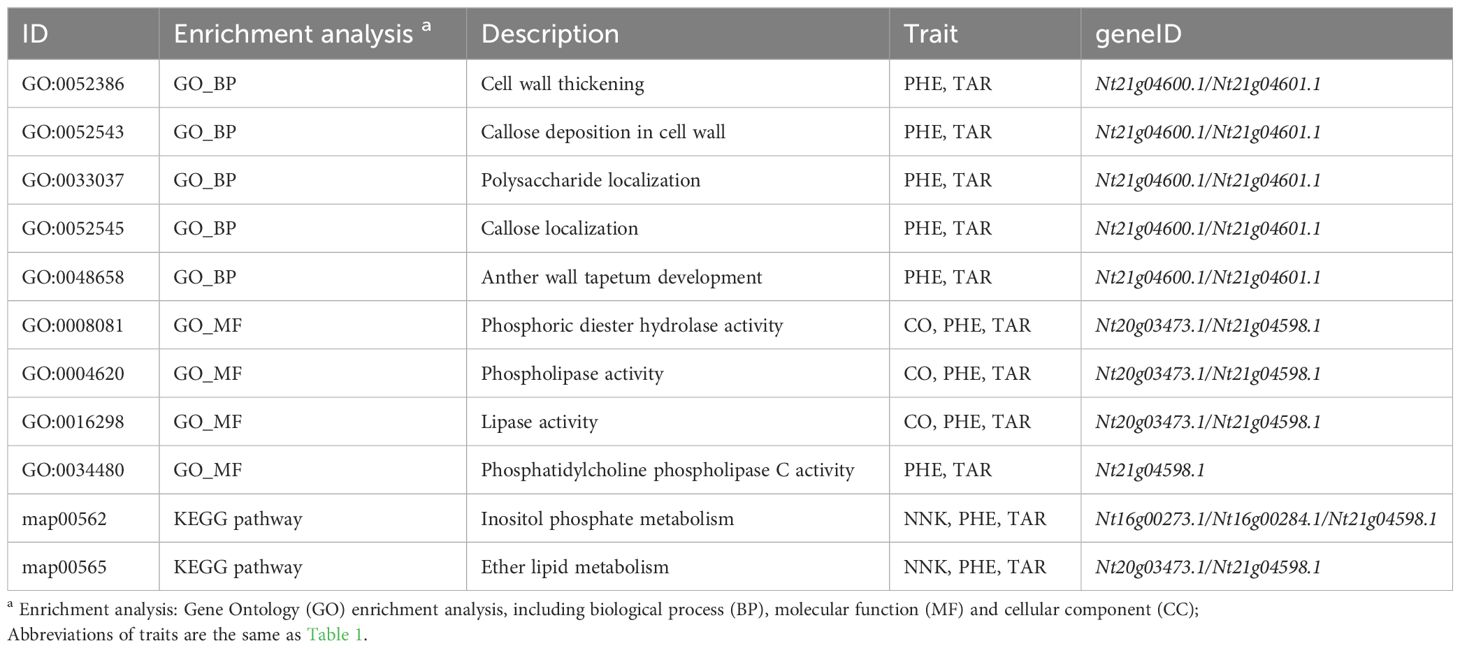
Table 5 Significantly enriched Gene Ontology (GO) terms and enriched KEGG pathway terms of potential genes.
The genes Nt21g04598.1, Nt21g04600.1 and Nt21g04601.1 were predicted as potential pleiotropic candidate genes for qPHE1 (PHE) and qTAR1 (TAR), BLASTP results indicated their putative functions. Nt21g04598.1 was shown to encode a protein highly homologous to non-specific phospholipase C4 in Arabidopsis thaliana, which functioned as plasma membrane bound and promoted tolerance to phosphate deficiency and hyperosmotic stress (Nakamura et al., 2005; Peters et al., 2010; Wimalasekera et al., 2010; Kocourková et al., 2011; Pejchar et al., 2015; Yang et al., 2021). Nt21g04600.1 and Nt21g04601.1 were predicted to code a protein with high homology to transcription factor bHLH91 in Arabidopsis thaliana, which regulated the transcriptional expression, thereby regulating the plant’s adaptive responses (Qian et al., 2021). The gene Nt20g03473.1 was pinpointed as a candidate gene for qCO6 (CO), which encoded a protein highly homologous to phospholipase D (PLD) delta in Arabidopsis thaliana. PLD delta has been proposed to play a role in many cellular processes such as signal transduction, membrane trafficking, cytoskeletal rearrangements, and membrane degradation (Distéfano et al., 2012; Guo et al., 2012; Uraji et al., 2012; Jia et al., 2013); for example, it was involved in H2O2 and abscisic acid (ABA)-induced stomatal closure, nitric oxide (NO) signaling and ABA-promoted senescence. Moreover, gene Nt16g00273.1 and Nt16g00284.1 have been identified as candidate genes for qNNK6 (NNK), which have been predicted to encode uncharacterized serine-rich protein C215.13 and type II inositol polyphosphate 5-phosphatase 15 isoform X2 in Nicotiana tabacum, respectively, awaiting further annotation in the future.
Discussion
In comparison to traditional technologies used to reduce harmful ingredients in cigarette smoke, targeting the molecular mechanisms underlying the production of hazardous substances would be an innovative approach to improve safety and quality. Julio et al. (Julio et al., 2006) was the first to apply QTL analysis to explore smoke toxicants. They constructed a partial genetic map using 138 low-throughput markers, including amplified fragment length polymorphism (AFLP), inter simple sequence repeat (ISSR), sequence specific amplified polymorphism (SSAP) and sequence characterized amplified region (SCAR), which were assigned to 18 linkage groups. Then, a total of five QTLs were identified for TAR, CO, and B[a]P in a RIL population. Furthermore, Tong (Tong et al., 2021) conducted QTL studies using a high-density genetic map with 45,081 SNPs, which was constructed by whole-genome sequencing data of a tobacco population of 274 individuals. They detected several major QTLs of PHE, CO and TAR distributed in LG6 from 123.28 to 158.72 cM, and the close linkage of these QTLs were in accord with the strong positive correlations among these traits. Similarly, our study also detected some QTLs of PHE and TAR in the same region, pleiotropic effects or linkage of which may lead to high genetic correlation between the traits.
Compared with previous QTL studies on hazardous smoke-related traits, our study possesses following advantages. First, we utilized a up-to-date published integrated linkage map with 46,324 polymorphic markers, including SNPs, Indels and SSRs, distributed on 24 linkage groups and covered 3334.88 cM with an average genetic distance of 0.469 cM (Tong et al., 2023). This high-resolution linkage map represents the most comprehensive map of tobacco to date. Second, we employed a QTL full model with effects of additive, additive-additive epistasis, and their interaction with environments, which is more rational to depict the genetic properties of quantitative traits where gene-gene and gene-environment interaction are mostly involved. As a result, we identified a total of 19 QTLs for the studied hazardous smoke-related traits, of which 17 QTLs showed significant additive effects, six showed significant additive-by-environment interaction effects, one pair showed significant epistasis-by-environment interaction effects for NH3. Notably, only one QTL was detected for CRO, HCN and HI, but more QTLs for CO, NH3, PHE and TAR, respectively, probably due to relatively lower general heritability () and interaction heritability () of CRO, HCN and HI.
Besides, we included the comprehensive index HI in QTL analysis to explore whether any pleiotropic QTLs could be detected with the eight direct smoke-related traits. It turned out that qHI6 was found to be located on the same linkage group as qNNK6, qCO6, qHCN6 and qPHE6. This is in concert with the high genetic correlations over 0.7 between HI and HCN, NH3 and PHE. In addition, considering of no QTL detected for B[a]P and its relatively small genotypic variance and genotype by environment interaction variance, we inferred that the B[a]P may be controlled by minor-effect QTLs which couldn’t be detected by the program because of too small effect magnitude, unlike major QTLs with relatively large effects that are more likely to be detected (Heffner et al., 2009; Beavis, 2019).
Our investigation pinpointed the qPHE1 and the qTAR1 were co-localized in the same chromosome, demarcated by the genetic markers PT61201 and SNP_0912209_288. Similarly, qPHE6 and qTAR6 were found to co-localize in another region, flanked by SNP_0002499_215080 and SNP_0011326_16206. These findings suggested the possibility that pleiotropic genes, may be nested within these genomic segments. Furthermore, our hypothesis on existence of pleiotropic gene was reinforced by the substantial genetic correlation with coefficient of 0.56 between the corresponding traits, PHE and TAR. The strong and stable trait correlation across environments further indicated the existence of shared pleiotropic candidate genes in these identified genomic regions. With subsequent comprehensive bioinformatics analyses, Nt21g04598.1, Nt21g04600.1 and Nt21g04601.1 were anchored at and predicted as pleiotropic candidate genes for qPHE1 and qTAR1. According to the results of NCBI-BLASTP, Nt21g04598.1 was predicted to encode a protein highly homologous to non-specific phospholipase C4 in Arabidopsis thaliana, Nt21g04600.1 and Nt21g04601.1 were predicted to code a protein with high homology to transcription factor bHLH91 in Arabidopsis thaliana. Coupled with results of GO and KEGG enrichment analyses, we speculated that these candidate genes might be involved in the signaling or regulatory processes of carbohydrate biosynthesis and transformation, leading to different performances of PHE and TAR.
In our study, identifying candidate genes relied on the rough physical positions of QTL intervals, which might encompass hundreds or thousands of genes. In other words, distinguishing target trait genes from annotated genes within a QTL remains challenging for primary mapping populations such as RILs used in our study. Therefore, fine mapping using secondary mapping populations (e.g., SSSLs, NILs, CSSLs), are needed to narrow down target QTLs, eliminating genetic background interference for accurate candidate gene prediction. Additionally, further studies are needed for functional validation of these candidate genes with cutting-edge molecular biology techniques on the levels of gene expressions, proteins and metabolites, so that, the pleiotropic genes could be effectively used in synchronous improvement of PHE and TAR.
Data availability statement
The original contributions presented in the study are included in the article/Supplementary Material. Further inquiries can be directed to the corresponding authors.
Author contributions
MX: Data curation, Writing – original draft. ZT: Conceptualization, Funding acquisition, Investigation, Project administration, Supervision, Writing – original draft. CJ: Data curation, Validation, Writing – original draft. QZ: Data curation, Methodology, Software, Writing – original draft. FL: Methodology, Software, Writing – original draft. DF: Data curation, Investigation, Writing – original draft. XC: Data curation, Investigation, Writing – original draft. TZ: Methodology, Software, Validation, Writing – original draft. XL: Methodology, Writing – review & editing. BX: Conceptualization, Funding acquisition, Project administration, Resources, Writing – review & editing. HX: Conceptualization, Methodology, Project administration, Supervision, Validation, Writing – review & editing.
Funding
The author(s) declare that financial support was received for the research, authorship, and/or publication of this article. This work was supported by the grants from China National Tobacco Company (110202101038 (JY-15) and 110202101002 (JY-02)), the Yunnan Tobacco Company (2022530000241009 and 2021530000241013), and the NSFC (31871707, 31961143016).
Conflict of interest
The authors declare that the research was conducted in the absence of any commercial or financial relationships that could be construed as a potential conflict of interest.
This study received funding from China National Tobacco Company, the Yunnan Academy of Tobacco Agricultural Sciences and the NSFC. The funders had the following involvement with the study: performed field trials and provided data including genotypic and phenotypic data.
Publisher’s note
All claims expressed in this article are solely those of the authors and do not necessarily represent those of their affiliated organizations, or those of the publisher, the editors and the reviewers. Any product that may be evaluated in this article, or claim that may be made by its manufacturer, is not guaranteed or endorsed by the publisher.
Supplementary material
The Supplementary Material for this article can be found online at: https://www.frontiersin.org/articles/10.3389/fpls.2024.1358953/full#supplementary-material
Abbreviations
ABA, Abscisic acid; AFLP, Amplified fragment length polymorphism; B[a]P, Benzo[α]pyrene; BLAST, Basic local alignment search tool; BLASTP, Protein BLAST; BLUP, Best linear unbiased prediction; CO, Carbon monoxide; CRO, Crotonaldehyde; E, Environment/Environmental; G, Genetic; GB/T, GuoBiao/Tuijian (recommended national standard in China); GC-MS, Gas chromatography - mass spectrometry; GC-TEA, Gas chromatography - thermal energy analyser; GE, Gene-by-environment interaction; GO, Gene ontology; GSL, General superior line; HCN, Hydrogen cyanide; HI, Hazard index; HPLC, High performance liquid chromatography; Indel, Insertion or deletion; ISSR, Inter simple sequence repeat; KEGG, Kyoto encyclopedia of genes and genomes; LG, Linkage group; MAS, Marker-assisted selection; MCIM, Mixed-linear-model-based composite interval mapping; MCMC, Markov chain Monte Carlo; MME, Mixed model equation; NCBI, National center for biotechnology information; NH3, Ammonia; NNK, 4-(methylnitrosamino)-1-(3-pyridyl)-1-butanone; NO, Nitric oxide; PHE, Phenol; PLD, Phospholipase D; QTL, Quantitative trait locus; RIL, Recombinant inbred lines; SCAR, Sequence characterized amplified region; SL, Superior line; SNP, Single-nucleotide polymorphism; SSAP, Sequence specific amplified polymorphism; SSR, Simple sequence repeat; TAR, Tar; YC/T: YanCao/Tuijian (recommended tobacco industry standard in China).
References
Beavis, W. D. (2019). “QTL Analyses: Power, Precision, and Accuracy,” in Molecular Dissection of Complex Traits (Boca Raton, Fl: CRC Press), 18. doi: 10.1201/9780429117770-10
Covarrubias-Pazaran, G. (2016). Genome-Assisted prediction of quantitative traits using the r package sommer. PloS One 11 (6). doi: 10.1371/journal.pone.0156744
Distéfano, A. M., Scuffi, D., García-Mata, C., Lamattina, L., Laxalt, A. M. (2012). Phospholipase Dδ is involved in nitric oxide-induced stomatal closure. Planta 236 (6), 1899–907. doi: 10.1007/s00425-012-1745-4
Edwards, K. D., Fernandez-Pozo, N., Drake-Stowe, K., Humphry, M., Evans, A. D., Bombarely, A., et al. (2017). A reference genome for Nicotiana tabacum enables map-based cloning of homeologous loci implicated in nitrogen utilization efficiency. BMC Genomics 18 (1), 448. doi: 10.1186/s12864-017-3791-6
Food and Drug Administration (2012) Harmful and Potentially Harmful Constituents in Tobacco Products and Tobacco Smoke. Available online at: https://www.fda.gov/tobacco-products/rules-regulations-and-guidance/harmful-and-potentially-harmful-constituents-tobacco-products-and-tobacco-smoke-established-list (Accessed November 15, 2023).
Guo, L., Devaiah, S. P., Narasimhan, R., Pan, X., Zhang, Y., Zhang, W., et al. (2012). Cytosolic glyceraldehyde-3-phosphate dehydrogenases interact with phospholipase Dδ to transduce hydrogen peroxide signals in the arabidopsis response to stress. Plant Cell 24 (5), 2200–2212. doi: 10.1105/tpc.111.094946
Heffner, E. L., Sorrells, M. E., Jannink, J. L. (2009). Genomic selection for crop improvement. Crop Sci. 49. doi: 10.2135/cropsci2008.08.0512
Hoffmann, D., Djordjevic, M. V., Hoffmann, I. (1997). The changing cigarette. Prev. Med. (Baltim) 26. doi: 10.1006/pmed.1997.0183
Hoffmann, D., Hoffmann, I., El-Bayoumy, K. (2001). The less harmful cigarette: A controversial issue. A tribute to Ernst L. Wynder. Chem. Res. Toxicol. 14 (7), 767–790. doi: 10.1021/tx000260u
Jia, Y., Tao, F., Li, W. (2013). Lipid profiling demonstrates that suppressing arabidopsis phospholipase Dδ Retards ABA-promoted leaf senescence by attenuating lipid degradation. PloS One 8 (6). doi: 10.1371/journal.pone.0065687
Julio, E., Denoyes-Rothan, B., Verrier, J. L., Dorlhac De Borne, F. (2006). Detection of QTLs linked to leaf and smoke properties in Nicotiana tabacum based on a study of 114 recombinant inbred lines. Mol. Breed. 18 (1), 69–91. doi: 10.1007/s11032-006-9019-0
Kocourková, D., Krčková, Z., Pejchar, P., Veselková, Š., Valentová, O., Wimalasekera, R., et al. (2011). The phosphatidylcholine-hydrolysing phospholipase C NPC4 plays a role in response of Arabidopsis roots to salt stress. J. Exp. Bot. 62 (11), 3753–3763. doi: 10.1093/jxb/err039
Liu, C., McAdam, K., Perfetti, T. (2012). Some recent topics in cigarette smoke science. Mini Rev. Org. Chem. 8 (4), 349–359. doi: 10.2174/157019311797440272
McAdam, K., Eldridge, A., Fearon, I. M., Liu, C., Manson, A., Murphy, J., et al. (2016). Influence of cigarette circumference on smoke chemistry, biological activity, and smoking behaviour. Regul. Toxicol. Pharmacol. 82, 111–126. doi: 10.1016/j.yrtph.2016.09.010
Nakamura, Y., Awai, K., Masuda, T., Yoshioka, Y., Takamiya, K. I., Ohta, H. (2005). A novel phosphatidylcholine-hydrolyzing phospholipase C induced by phosphate starvation in Arabidopsis. J. Biol. Chem. 280 (9), 7469–7476. doi: 10.1074/jbc.M408799200
Pejchar, P., Potocký, M., Krčková, Z., Brouzdová, J., Daněk, M., Martinec, J. (2015). Non-specific phospholipase C4 mediates response to aluminum toxicity in Arabidopsis thaliana. Front. Plant Sci. 6, 66. doi: 10.3389/fpls.2015.00066
Peters, C., Li, M., Narasimhan, R., Roth, M., Welti, R., Wang, X. (2010). Nonspecific phospholipase C NPC4 promotes responses to abscisic acid and tolerance to hyperosmotic stress in Arabidopsis. Plant Cell 22 (8), 2642–2659. doi: 10.1105/tpc.109.071720
Qian, Y., Zhang, T., Yu, Y., Gou, L., Yang, J., Xu, J., et al. (2021). Regulatory mechanisms of bHLH transcription factors in plant adaptive responses to various abiotic stresses. Front. Plant Sci. 12, 67761. doi: 10.3389/fpls.2021.677611
Rodgman, A., Green, C. (2003). Toxic Chemicals in Cigarette Mainstream Smoke - Hazard and Hoopla. Vol. 20. Beiträge zur Tabakforschung International/Contributions to Tobacco Research . doi: 10.2478/cttr-2013-0764
Schwanz, T. G., Bokowski, L. V. V., Marcelo, M. C. A., Jandrey, A. C., Dias, J. C., Maximiano, D. H., et al. (2019). Analysis of chemosensory markers in cigarette smoke from different tobacco varieties by GC×GC-TOFMS and chemometrics. Talanta 202, 74–89. doi: 10.1016/j.talanta.2019.04.060
Smith, C. J., Hansch, C. (2000). The relative toxicity of compounds in mainstream cigarette smoke condensate. Food Chem. Toxicol. 38 (7), 637–646. doi: 10.1016/S0278-6915(00)00051-X
Standardization Administration of China (2004) Cigarette Determination of total and nicotine-free dry particulate matter using a routine analytical smoking machine. Available online at: https://openstd.samr.gov.cn/bzgk/gb/newGbInfo?hcno=232998A21D07A0AC6AF73B72B7FA76DA (Accessed November 15, 2023).
Standardization Administration of China (2007) Cigarettes - Determination of Benzo[a]pyrene in total particulate matter. Available online at: https://openstd.samr.gov.cn/bzgk/gb/newGbInfo?hcno=508185313FC16FE2980BD0B6EC3E5325 (Accessed November 15, 2023).
Standardization Administration of China (2008a) Cigarettes - Determination of major carbonyls in mainstream cigarette smoke - High performance liquid chromatographic method. Available online at: https://hbba.sacinfo.org.cn/stdDetail/0aa1c0a086cb877109a5819553444d1f (Accessed November 15, 2023).
Standardization Administration of China (2008b) Cigarette - Determination of tobacco specific N-Nitrosamines in total particulate matter of mainstream cigarette smoke - GC-TEA method. Available online at: https://openstd.samr.gov.cn/bzgk/gb/newGbInfo?hcno=6775A00A9373F7FB80337741B2F8E3F9 (Accessed November 15, 2023).
Standardization Administration of China (2008c) Cigarettes - Determination of major phenolic compounds in mainstream cigarette smoke - High performance liquid chromatographic method. Available online at: https://hbba.sacinfo.org.cn/stdDetail/059e5e75fa2a52406e7f83c4c746f6f5 (Accessed November 15, 2023).
Standardization Administration of China (2009) Cigarettes - Determination of carbon monoxide in the vapour phase of cigarette smoke - NDIR method. Available online at: https://openstd.samr.gov.cn/bzgk/gb/newGbInfo?hcno=738000AC5FC18E4C16317A035CD69146 (Accessed November 15, 2023).
Standardization Administration of China (2011) Cigarettes - Determination of hydrogen cyanide in mainstream cigarette smoke - Ion chromatography method. Available online at: https://hbba.sacinfo.org.cn/stdDetail/5f25cf08fee99fe0abf95a3fdf58dd04 (Accessed November 15, 2023).
Standardization Administration of China (2017) Cigarettes - Determination of ammonia in mainstream cigarette smoke - Ion chromatography method. Available online at: https://hbba.sacinfo.org.cn/stdDetail/a3370049ee6992ce8c1a20ef16865675 (Accessed November 15, 2023).
Talhout, R., Schulz, T., Florek, E., van Benthem, J., Wester, P., Opperhuizen, A. (2011). Hazardous compounds in tobacco smoke. Int. J. Environ. Res. Public Health 8, 613–628. doi: 10.3390/ijerph8020613
Tong, Z., Xiu, Z., Ming, Y., Fang, D., Chen, X., Hu, Y., et al. (2021). Quantitative trait locus mapping and genomic selection of tobacco (Nicotiana tabacum L.) based on high-density genetic map. Plant Biotechnol. Rep. 15, 845–854. doi: 10.1007/s11816-021-00713-1
Tong, Z., Xu, M., Zhang, Q., Lin, F., Fang, D., Chen, X., et al. (2023). Construction of a high-density genetic map and dissection of genetic architecture of six agronomic traits in tobacco (Nicotiana tabacum L.). Front. Plant Sci. 14, 1126529. doi: 10.3389/fpls.2023.1126529
Uraji, M., Katagiri, T., Okuma, E., Ye, W., Hossain, M. A., Masuda, C., et al. (2012). Cooperative function of PLDδ and PLDα1 in abscisic acid-induced stomatal closure in arabidopsis. Plant Physiol. 159 (1), 450–460. doi: 10.1104/pp.112.195578
Wimalasekera, R., Pejchar, P., Holk, A., Martinec, J., Scherer, G. F. E. (2010). Plant phosphatidylcholine-hydrolyzing phospholipases C NPC3 and NPC4 with roles in root development and brassinolide signaling in arabidopsis thaliana. Mol. Plant 3 (3), 610–625. doi: 10.1093/mp/ssq005
World Health Organization (2023) WHO report on the global tobacco epidemic 2023: protect people from tobacco smoke. Available online at: https://www.who.int/publications/i/item/9789240077164 (Accessed November 15, 2023).
Xie, J., Liu, H., Zhu, M., Zhong, K., Dai, Y., Du, W., et al. (2009). Development of a novel hazard index of mainstream cigarette smoke and its application on risk evaluation of cigarette products. Tobacco Science & Technology 2, 5–15.
Yang, B., Li, M., Phillips, A., Li, L., Ali, U., Li, Q., et al. (2021). Nonspecific phospholipase C4 hydrolyzes phosphosphingolipids and sustains plant root growth during phosphate deficiency. Plant Cell 33 (3), 766–780. doi: 10.1093/plcell/koaa054
Yang, J., Hu, C., Hu, H., Yu, R., Xia, Z., Ye, X., et al. (2008). QTLNetwork: Mapping and visualizing genetic architecture of complex traits in experimental populations. Bioinformatics 24 (5), 721–723. doi: 10.1093/bioinformatics/btm494
Keywords: tobacco, mixed linear model, QTL mapping, gene-by-environment interactions, pleiotropy
Citation: Xu M, Tong Z, Jin C, Zhang Q, Lin F, Fang D, Chen X, Zhu T, Lou X, Xiao B and Xu H (2024) Dissection of genetic architecture of nine hazardous component traits of mainstream smoke in tobacco (Nicotiana tabacum L.). Front. Plant Sci. 15:1358953. doi: 10.3389/fpls.2024.1358953
Received: 20 December 2023; Accepted: 19 April 2024;
Published: 08 May 2024.
Edited by:
Cheng Shihua, China National Rice Research Institute (CAAS), ChinaReviewed by:
Hanwei Mei, Shanghai Agrobiological Gene Center, ChinaQingzhang Du, Beijing Forestry University, China
Copyright © 2024 Xu, Tong, Jin, Zhang, Lin, Fang, Chen, Zhu, Lou, Xiao and Xu. This is an open-access article distributed under the terms of the Creative Commons Attribution License (CC BY). The use, distribution or reproduction in other forums is permitted, provided the original author(s) and the copyright owner(s) are credited and that the original publication in this journal is cited, in accordance with accepted academic practice. No use, distribution or reproduction is permitted which does not comply with these terms.
*Correspondence: Bingguang Xiao, eGlhb2Jnc3VibWlzc2lvbkAxMjYuY29t; Haiming Xu, aG14dUB6anUuZWR1LmNu
†These authors have contributed equally to this work and share first authorship