- 1Departamento de Ciencias Vegetales, Facultad de Agronomía y Sistemas Naturales, Pontificia Universidad Católica de Chile, Santiago, Chile
- 2Centro de Estudios en Alimentos Procesados (CEAP), Talca, Chile
- 3Department of Soil, Plant and Food Sciences, University of Bari Aldo Moro, Bari, Italy
- 4Instituto de Investigaciones Agropecuarias (INIA), Centro Regional Quilamapu, Chillán, Chile
- 5Instituto de Investigaciones Agropecuarias (INIA), Centro Regional Rayantue, Rancagua, Chile
Wild emmer (Triticum turgidum ssp. dicoccoides) genotypes were studied for their high-nutritional value and good tolerance to various types of stress; for this reason, several QTL (quantitative trait loci) studies have been conducted to find favorable alleles to be introgressed into modern wheat cultivars. Given the complexity of the QTL nature, their interaction with the environment, and other QTLs, a small number of genotypes have been used in wheat breeding programs. Meta-QTL (MQTL) analysis helps to simplify the existing QTL information, identifying stable genomic regions and possible candidate genes for further allele introgression. The study aimed to identify stable QTL regions across different environmental conditions and genetic backgrounds using the QTL information of the past 14 years for different traits in wild emmer based upon 17 independent studies. A total of 41 traits were classified as quality traits (16), mineral composition traits (11), abiotic-related traits (13), and disease-related traits (1). The analysis revealed 852 QTLs distributed across all 14 chromosomes of wild emmer, with an average of 61 QTLs per chromosome. Quality traits had the highest number of QTLs (35%), followed by mineral content (33%), abiotic-related traits (28%), and disease-related traits (4%). Grain protein content (GPC) and thousand kernel weight (TKW) were associated with most of the QTLs detected. A total of 43 MQTLs were identified, simplifying the information, and reducing the average confidence interval (CI) from 22.6 to 4.78 cM. These MQTLs were associated with multiple traits across different categories. Nine candidate genes were identified for several stable MQTLs, potentially contributing to traits such as quality, mineral content, and abiotic stress resistance. These genes play essential roles in various plant processes, such as carbohydrate metabolism, nitrogen assimilation, cell wall biogenesis, and cell wall extensibility. Overall, this study underscores the importance of considering MQTL analysis in wheat breeding programs, as it identifies stable genomic regions associated with multiple traits, offering potential solutions for improving wheat varieties under diverse environmental conditions.
1 Introduction
Due to the domestication process and systematic breeding selection, the genetic variability of bread wheat (Triticum aestivum L.) and durum wheat (Triticum turgidum L. ssp. durum) is relatively small compared to the diversity found in their tetraploid progenitor wild emmer (Triticum turgidum ssp. dicoccoides; 2n = 4x = 28 chromosomes; AB-genomes) (Abbo et al., 2014; Golan et al., 2015). Historically, yield and yield components have been the main selection criteria in breeding programs for any staple crop, including wheat. Generally, there is a negative correlation among yield components and quality traits (Blanco et al., 2012). In fact, some studies have found that durum and bread wheat decreased over selection time in grain protein content (GPC) (Subira et al., 2014), mineral content (Cakmak et al., 2010), and resistance to abiotic and biotic stressors in favor of higher yields, test weight, and thousand kernel weight (TKW) (Peng et al., 2011). Due to the impacts of climate change and the environmental effect on various traits, it is imperative to incorporate tolerance to environmental stressors and diseases into breeding programs, particularly for crops such as wheat. This necessity extends to the inclusion of crops that are both healthier and more nutritious while also avoiding intensive production practices (Longin et al., 2016).
Because of its nutritional values and tolerance to abiotic and biotic stressors, wild emmer has been used as an alternative crop for the introgression of favorable alleles into modern wheat genotypes, expanding the genetic diversity within ongoing wheat breeding programs (Cakmak et al., 2004; Kuznetsova et al., 2019), particularly in the cultivation of the crop under adverse environmental conditions (Beres et al., 2020). The introgression of specific wild emmer genes into modern wheats has been carried out for the principal traits important for breeders, the food industry, and the consumers (Merchuk-Ovnat et al., 2016a, 2017; Kumar et al., 2020; Liu et al., 2021). Joppa and Cantrell (1990) identified the first QTL (quantitative trait loci) for GPC in wild emmer lines “FA-15-3” and “F-28-8-3” from Israel, called Gpc-B1 on chromosome 6B. This genetic locus increased the overall GPC to 18%, 2% higher than the GPC in the recurrent durum wheat parent “Langdon” (LDN), which had 16.8% (Joppa et al., 1991). The gene Gpc-B1 encodes for a NO APICAL MERISTEM-B1 (NAM-B1), which increases nutrient remobilization from leaves to grains related to the final GPC and mineral composition. However, it was not functional in modern wheat cultivars (Uauy et al., 2006; Avni et al., 2014). Since the discovery of Gpc-B1, several other QTLs have been identified; for example, Peleg et al. (2009a) and Fatiukha et al. (2019) mapped several QTLs associated with plant productivity and drought-adaptive traits in a RIL population derived from a cross between durum wheat (LDN) and wild emmer (“G18-16”). Subsequently, some of these wild QTLs were introgressed from G18-16 into an elite Israeli durum material (“Uzan”) on chromosomes 1B and 2B and bread wheat (“Bar Nir” and “Zahir”) on chromosome 7A via marker-assisted selection. The introgressed QTL improved grain yield, biomass, photosynthetic capacity, and root development across different environments, particularly under drought conditions (Merchuk-Ovnat et al., 2016a, b, 2017).
Several studies have identified QTLs in wild emmer in different mapping populations and environments. Meta-QTL (MQTL) analysis developed by Goffinet and Gerber (2000) is an alternative to (i) facilitate the compilation of information regarding consensus QTLs, narrowing the QTL regions for a particular trait across different environments and genetic backgrounds; (ii) allow the study of many traits at once defining their reliable location and effects across different genetic backgrounds and environments determining the molecular markers valuable for marker-assisted selection (MAS) (Goffinet and Gerber, 2000; Khahani et al., 2021; Soriano et al., 2021); (iii) allow the identification of QTLs that have pleotropic effects by determining regions of the genome (MQTL) that contain QTLs for different traits (Said et al., 2013); (iv) the given MQTL information has been used for candidate genes detection and for future cloning of the genes (Colasuonno et al., 2021; Saini et al., 2021).
Meta-QTL analysis has been successfully performed in bread wheat for yield and related traits (Zhang et al., 2010; Liu et al., 2020; Miao et al., 2022; Saini et al., 2022a), grain quality traits (Tyagi et al., 2015), mineral content (Singh et al., 2022), fusarium head blight (FHB) resistance (Löffler et al., 2009; Venske et al., 2019; Saini et al., 2022b), and abiotic resistance (Xu et al., 2017; Guo et al., 2023). In durum wheat, MQTL has been conducted for yield and related traits (Arriagada et al., 2022); quality traits, abiotic and biotic stressors (Soriano et al., 2021); pasta making quality (Roselló et al., 2018); GPC (Saini et al., 2022c); and ortho-MQTL analysis for quality traits (Marcotuli et al., 2022). To the best of our knowledge, the only MTQL study performed using wild emmer was carried out by Avni et al. (2018) for grain weight. However, no MQTL studies have been conducted using different quality traits, mineral composition, abiotic stressors, or diseases such as FHB in wild emmer. Considering the helpful information that can be obtained from MQTL analysis from wild emmer grown in different environments, the aim of the study was to examine all the existing QTL information published in the last 14 years in different environments regarding agronomical and chemical quality traits, mineral composition, abiotic-related traits, and diseases-related traits to identify MQTL regions and candidate genes in wild emmer that can be further used in modern wheats breeding programs in the world.
2 Materials and methods
The MQTL analysis for quality traits, mineral composition traits, abiotic-related traits, and disease-related traits involved three main steps: first, a complete compilation of all QTL data associated with quality, mineral composition, abiotic, and diseases already reported for wild emmer. A list of traits that belongs to each category is reported in Table 1. Second, the creation of a consensus map where the QTLs previously collected from the literature were projected. Third, the MQTL identification through MQTL analysis.
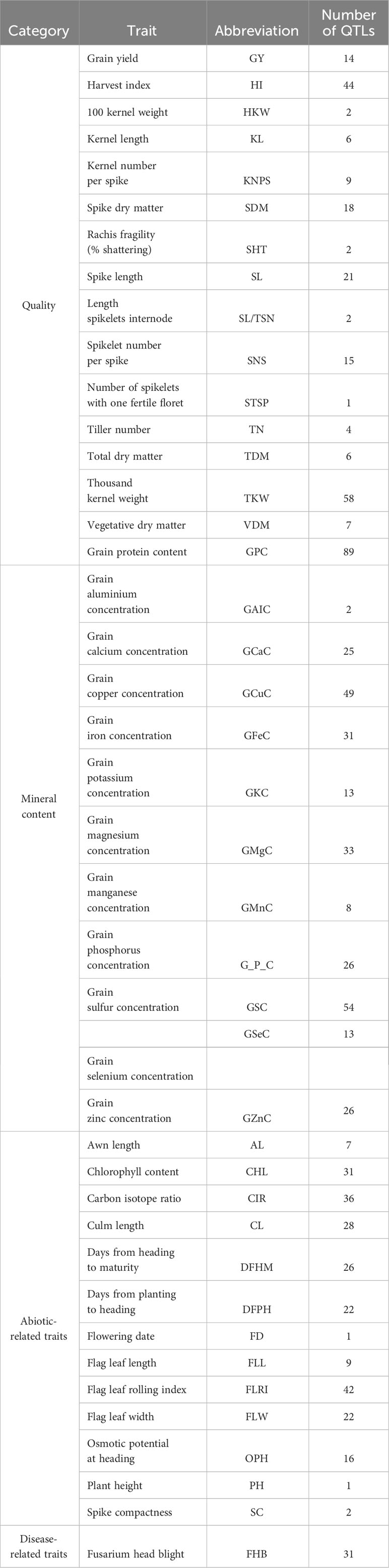
Table 1 Abbreviations and numbers of QTLs for quality, mineral composition, abiotic, and disease-related traits categories reported in the QTL studies for wild emmer.
2.1 Data collection and consensus genetic map construction
A comprehensive bibliographic search on the Web of Science, Google Scholar, and PubMed was conducted to find the QTL studies associated with quality traits, mineral composition traits, abiotic-related traits, and diseases in wild emmer. At the end of an exhaustive search, a total of 17 independent studies from 2009 to 2022 that included F2 mapping populations, recombinant inbred lines (RILs), recombinant inbred chromosome lines (RICs), and backcross (BC) were used. The selected QTL studies must contain the following:
i. a map for the population that includes the wild emmer parental wheat information
ii. the position of QTL, such as peak position (Pos) and CIs
iii. logarithm of the odds score (LOD) for each QTL
iv. phenotypic variance percentage for each QTL (PVE or r2)
Any QTL study that did not meet these criteria was not considered in our work. The only disease that met the analysis requirements was FHB; therefore, it was the only trait considered in this category.
From the studies, 41 traits were identified. The number of traits per category was 16 for quality traits, 11 for mineral composition traits, 13 for abiotic-related traits, and one for disease-related traits. The name of each trait per category and the number of QTLs per trait are included in Table 1. The studies and the QTLs used are available in Table 2. The environmental information per study is in the Supplementary Table 1.
Each QTL was treated as independent, even if some of them were detected in multiple environments or genetic backgrounds. If the CI (95%) for the QTL was not reported, it was calculated using the following equations described by Guo et al. (2006):
where N is the population size and R2 is the proportion of the phenotypic variance explained by the QTL.
The collected genetic maps containing their chromosomes, markers, and positions in cM were integrated individually onto the durum wheat reference map developed by Maccaferri et al. (2015). Maccaferri’s map consisted of 30,144 markers spanning 2,631 cM with 11 markers per cM density. The consensus map was constructed using BioMercator 4.2.3 software (Arcade et al., 2004).
2.2 Projection of QTL and meta-QTL analysis
The original QTL data from the 17 studies were projected individually onto the created consensus map by following the homothetic approach described by Chardon et al. (2004). A total of 14 studies were projected, followed by the MQTL analysis that was performed per chromosome using the BioMercator V4.2.3 software (Arcade et al., 2004). If the QTL number per chromosome was higher than 10, the Veyrieras algorithm available in the software was used for the MQTL analysis (Veyrieras et al., 2007). The first part of the Veyrieras algorithm indicated the number of MTQL models generated by different criteria. The criteria were the Akaike Information Criterion (AIC), the corrected AIC (AICc), the modified AIC with factor 3 (AIC3), the Bayesian Information Criterion (BIC), and the Average Weight of Evidence (AWE). The best MQTL model was selected based on the lowest number in at least three of the five criteria (Soriano and Alvaro, 2019). Conversely, if the QTL number per chromosome was ≤ 10, the Goffinet and Gerber approach was performed for the MQTL analysis (Goffinet and Gerber, 2000). The final identification of the number of MQTL per chromosome was based on a delta value ≥ 0.9 value in at least two studies.
2.3 Identification of candidate genes
Gene annotations for the most important marker-trait associations (MTAs) were performed using the high-confidence genes reported for the wheat genome sequence (Svevo browser), available at https://wheat.pw.usda.gov/GG3/jbrowse_Durum_Svevo. The marker locations were defined by flanking marker positions and the CI of the MQTL.
3 Results
3.1 Features for the studied QTLs under diverse growing environments
The 17 studies comprised 19 biparental populations and ten wild emmer accessions. In our study, only the QTLs belonging to the wild emmer parent were used. A total of 852 QTLs, distributed across all 14 chromosomes (A and B genomes) of wild emmer, for the 41 selected traits were collected (Table 2). The number of QTLs ranged from 24 on chromosome 1B to 96 on chromosome 5A, averaging 61 QTLs per chromosome (Figure 1A). Overall, quality was the category with the highest number of QTLs, representing 35% (298 QTLs), followed by mineral content with 33% (280 QTLs), abiotic-related traits with 28% (243 QTLs), and disease-related traits with 4% (31 QTLs) (Figure 1B). GPC was the trait with the largest number of QTLs (86), followed by TKW (58 QTLs) (Table 1).
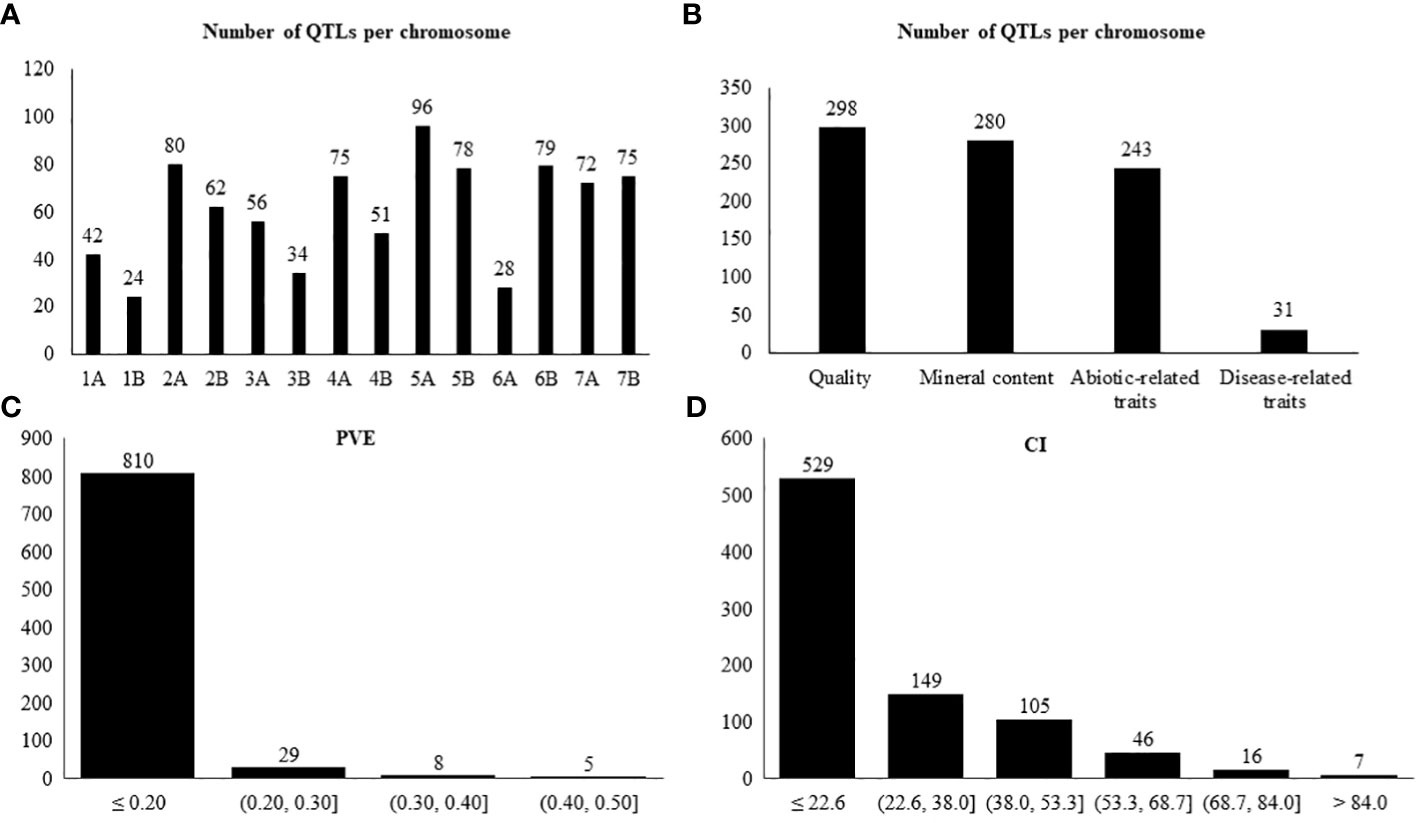
Figure 1 Number of QTLs per: (A) chromosome, (B) trait category, (C) PVE, (D) confidence interval based on 852 collected QTLs.
The PVE for each QTL ranged from 0.001 to 0.63, with an average of 0.08 (Figure 1C). Per category, the PVE ranged as follows: abiotic-related traits from 0.001 to 0.46; disease-related traits from 0.02 to 0.26; mineral content from 0.005 to 0.24; and quality from 0.006 to 0.63 (Supplementary Figure S1). A total of 94%, 74%, 99%, and 95% of the QTLs for abiotic-related traits, disease-related traits, mineral content, and quality had PVE ≤ 0.20, respectively.
The CIs ranged from 0.1 to 234.5 cM, with an average of 22.6 cM. A total of 529 QTLs, corresponding to 62% of the studied QTLs, were located between 0.1 and 22.6 cM (Figure 1D). Of those 529 QTLs, 144 corresponded to abiotic-related traits, 23 to disease-related traits, 164 to mineral content, and 205 to quality (Supplementary Figure S2).
3.2 Projection of the QTLs on the consensus map
The projection of the QTLs was made using the 17 studies collected previously indicated; however, only 14 studies were projected. Of the 852 QTLs available, only 712 were successfully projected on the consensus map (Figure 2); the remaining 140 QTLs could not be projected. According to Soriano and Alvaro (2019), the lack of projection is due to a low PVE between the QTLs, causing a larger CI and/or the absence of markers between the original map and the consensus map. The number of QTLs per chromosome ranged from 15 on chromosome 6A to 81 on chromosome 5B (Figure 3A). The trait with the most significant number of projected QTLs was GPC, with 85 QTLs representing 12% of the total QTLs. Plant height (PH), spike compactness (SC), and number of spikelets with one fertile floret (STPS) had the lower number of QTLs projected, with 1 QTL per trait, representing 0.14%, respectively (Supplementary Table S2). The category mineral content had the highest number of QTLs projected with 38%, followed by quality, abiotic-related traits, and disease-related traits with 34%, 23%, and 3.9%, respectively (Figure 3B).
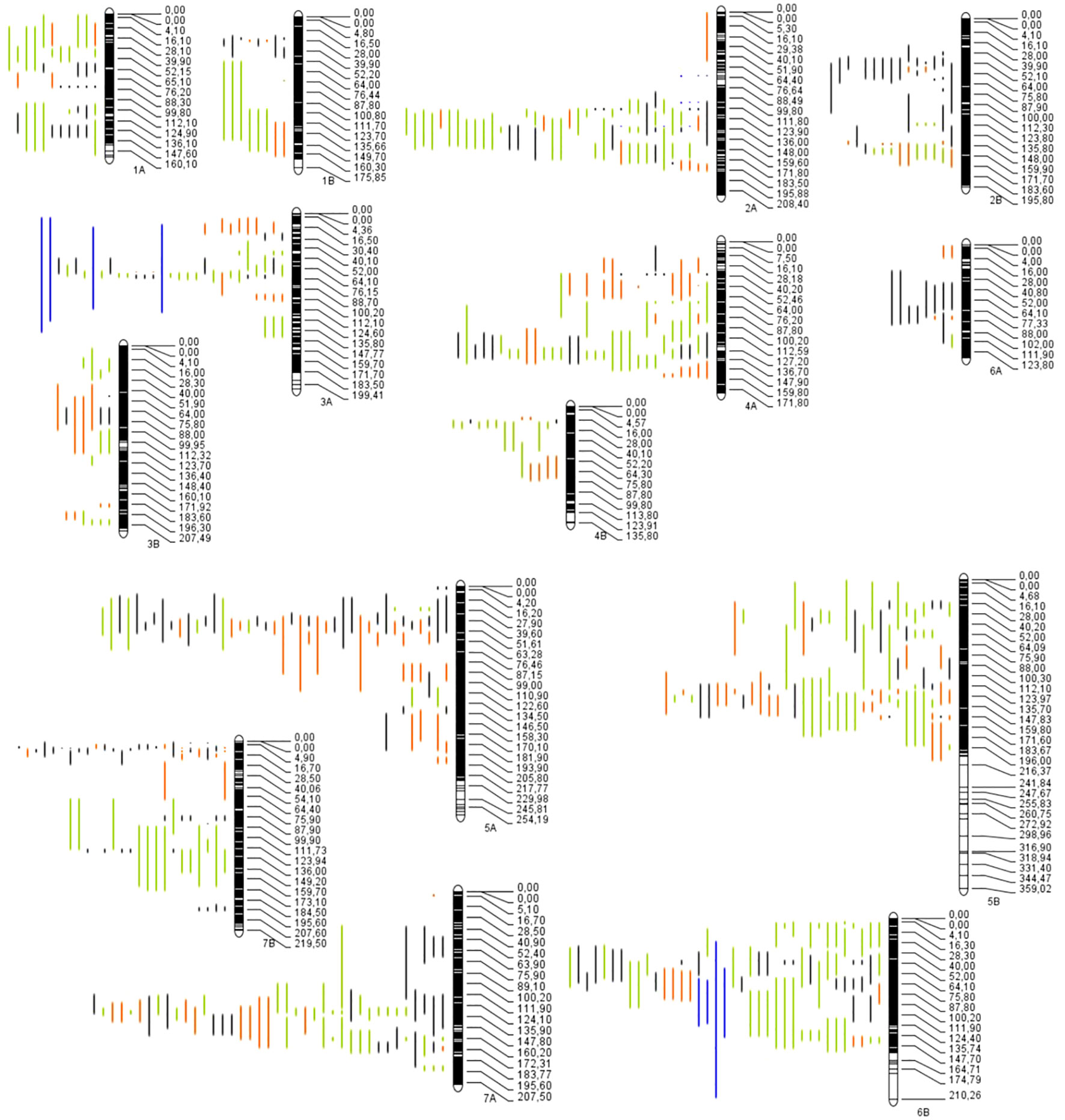
Figure 2 Distribution of the QTLs projected through 14 chromosomes in wild emmer for quality traits (red bars), mineral content (yellow bars), abiotic-related traits (green bars) and fusarium head blight (blue bars).
The consensus map included 31,723 markers, covering a length of 2,879.1 cM. For each chromosome, the size of the genetic map ranged from 131.2 cM on chromosome 6A to 359.0 cM on chromosome 5B. In addition, the number of markers ranged from 1,455 in chromosome 4B to 3,421 in chromosome 2B, with an average of 2,266 marker per chromosome (Supplementary Table S3).
3.3 Meta-QTLs detected for all studied traits
Of the total 712 projected QTLs, only 395 QTLs were grouped into 43 MQTLs, which significantly reduced the total number of projected QTLs (43 MQTLs correspond to equal 11% of 395 QTLs). The remaining 317 QTLs could not be assigned to any MQTL, staying as a single QTL. This could happen because the predicted peaks were not included in any MQTL or the CI was too large. The number of MQTLs ranged from 2 in chromosomes 3B, 4A, 4B, 6A, and 7B to 5 in chromosomes 2A and 5A, with an average of 3.1 MQTLs per chromosome. dicoccoides_MQTL_7A.2 was the MQTL with the highest number of QTLs associated (37 QTLs) vs. dicoccoides_MQTL_1B.2, dicoccoides_MQTL_3B.2, dicoccoides_MQTL_5B.2, dicoccoides_MQTL_6A.2, and dicoccoides_MQTL_7A.1 with the lowest number of QTLs associated (3 QTLs).
Each MQTL was linked to at least one specific trait. For instance, dicoccoides_MQTL_5A.1 and dicoccoides_MQTL_7A.1 were associated with GPC and spike dry matter (SDM), respectively. Notably, dicoccoides_MQTL_7A.2 exhibited associations with ten distinct traits, as detailed in Table 3.
In the overall analysis, three of the 43 MQTLs were identified with associations to FHB on chromosomes 2A, 6A, and 6B, specifically dicoccoides_MQTL_2A.1, dicoccoides_MQTL_6A.1, and dicoccoides_MQTL_6B.2. Furthermore, a subset of four MQTLs out of the total 43 were exclusively associated with quality traits, distributed across chromosomes 2A, 5A, and 7A. Examples include dicoccoides_MQTL_2A.4, dicoccoides_MQTL_2A.3, dicoccoides_MQTL_5A.1, and dicoccoides_MQTL_7A.1. Similarly, two MQTLs, specifically dicoccoides_MQTL_2B.3 and dicoccoides_MQTL_5A.5, associated solely with abiotic-related traits within chromosomes 2B and 5A. Notably, 26 out of the 43 MQTLs associated with mineral content and they also showed connections to one or more quality traits and abiotic-related traits, as outlined in Table 3.
The detected MQTLs exhibited a range of average CI (95%), from 0.01 cM for dicoccoides_MQTL_5A.5 to 28.75 cM for dicoccoides_MQTL_1B.3, with an overall mean of 4.78 cM (as shown in Table 3). Interestingly, this average was 4.7 times smaller than the initial average CI of 22.6 cM. The genomic region delineated for dicoccoides_MQTL_5A.5 (CI: 0.01 cM) exhibited an association with six QTLs originating from distinct studies, one conducted by Buerstmayr et al. (2012) and the other by Fatiukha et al. (2021), as well as two wild emmer accessions (Table 2). Notably, these QTLs exclusively pertained to traits categorized as abiotic related, specifically the carbon isotope ratio (CIR), days from heading to maturity (DFHM), and SC. It is noteworthy that the flanking markers associated with these QTLs were situated external to the defined MQTL region (Table 3). No discernible genetic associations with genes were identified in proximity to these markers.
Per chromosome, the average CI ranged from 1.57 cM in chromosome 7B to 15.47 cM in chromosome 1B.
3.4 Candidate genes
Using the genomic sequences located in the breeding QTL from “Svevo,” nine candidate genes correlated to MQTL were identified (Table 4). The flanking markers were aligned with the genome browsers for both Svevo (durum wheat) reference genome, accessible at https://iwgs.org/, and after excluding transposable elements, a total of 25 genes were identified.
The MQTLs that were associated with candidate genes were located on chromosomes 1A, 2A, 4A, 4B, 6B, and 7B (Table 4). In the case of chromosome 1A, three MQTLs were associated with genes. In detail, dicoccoides_MQTL_1A.1 was associated with beta-1,3-galactosyltransferase, dicoccoides_MQTL_1A.2 was associated with glycosyltransferase STELLO2, and dicoccoides_MQTL_1A.4 was associated with SUPPRESSOR OF PHYA-105 1-like. The chromosome has associated the dicoccoides_MQTL_2A.4 with the kinesin-like protein KIN-14I and the dicoccoides_MQTL_2A.5 cysteine-rich receptor-like protein kinase (CRK) 10. The MTQL for chromosomes 4A, 4B, 6B, and 7B were associated with one gene each.
4 Discussion
4.1 The significance of considering MQTL analysis
In recent years, various studies have been conducted on modern wheats, particularly bread wheat, to identify new genetic locations associated with different quality parameters. These parameters include yield and related traits (Maccaferri et al., 2019; Arriagada et al., 2020; Gupta et al., 2020), protein content (Saini et al., 2022c), mineral content (Fatiukha et al., 2020), abiotic-related traits (Bhusal et al., 2018), and biotic-related traits such as FHB (Zhang et al., 2022). Nevertheless, research indicates that intensive breeding practices in modern wheats have led to increased productivity at the expense of diminished nutritional value, protein content, and resistance or tolerance to abiotic and biotic stressors (Cakmak et al., 2010; Peng et al., 2011; Cabas-Lühmann et al., 2023). Various QTL studies were conducted, showing the substantial nutritive value and stress tolerance conferred by wild emmer. These studies primarily aimed to identify alleles or markers associated with nutritional value, particularly concerning iron and zinc content (Peleg et al., 2009a; Liu et al., 2021), protein content (Fatiukha et al., 2019), and tolerance or resistance to abiotic (Peleg et al., 2005) and biotic stressors (Huang et al., 2016). Some of these QTLs have been used in wheat breeding programs to enhance the genotypes by introducing alleles that broaden their genetic diversity, thereby improving nutritional content, protein content, and resistance or tolerance to stressors (Kumar et al., 2018; Fatiukha et al., 2019; Kuznetsova et al., 2019; Cabas-Lühmann et al., 2023). However, despite all these studies on QTLs, wheat breeding programs have used only a limited subset of those genetic resources (Cobb et al., 2019). This limited adoption is primarily because the expression of these QTLs is greatly affected by the environment, the genotype by environment interactions, the mapping population type, and the statistical method used for the QTL identification (Sandhu et al., 2021; Zheng et al., 2021). A Meta-QTL analysis emerges as a powerful approach to consolidate QTL information from diverse populations cultivated in various environments. This method identifies stable QTLs within the plant genome, situated in genomic regions encompassing valuable and diverse genetic material for potential integration into wheat breeding programs.
4.2 Quantitative trait loci for quality traits, mineral content, abiotic-related traits, and disease-related traits
In this study, a total of 852 QTLs were identified across all 14 chromosomes of wild emmer. The QTLs were distributed as quality traits (298 QTLs), mineral content (280 QTLs), abiotic-related traits (243 QTLs), and disease-related traits (31 QTLs). The quality category exhibited the highest QTL count, encompassing yield, yield-related traits, and GPC. Within the quality category, TKW and GPC were the traits with the highest QTL counts, having 58 and 89 QTLs, respectively. The largest number of QTLs associated with TKW and GPC can be linked to the extensive research conducted on these traits, given their significance in both wheat yield and product manufacturing (Avni et al., 2018). For instance, QTL studies on yield in durum wheat often utilize TKW as a pivotal yield component (Liang et al., 2017), influenced by environmental factors during grain filling (Li et al., 2019), that can be used for genotypes that grow under diverse environmental conditions (Gupta et al., 2020; Arriagada et al., 2022; Wang et al., 2022). Since the obtention of high-yield genotypes is necessary for wheat breeding programs, incorporating accumulated loci associated with yield components like TKW becomes essential. TKW is not only influenced by the environment during grain filling, but it is also positively correlated with overall grain and milling yield (Wang and Fu, 2020). On the other hand, wild emmer is known as a valuable genetic reservoir, abundant in allelic variants, offering a substantial alternative to enhance GPC through the introgression of favorable alleles into modern wheat varieties (Fatiukha et al., 2019; Colasuonno et al., 2021). This explains why most of the QTL studies on this ancient wheat are related to GPC. Interestingly, the data highlights that the primary locations for QTLs related to TKW were chromosomes 5A (13 QTLs) and 4A (11 QTLs). In contrast, QTLs for GPC were predominantly localized on chromosomes 6A and 7B (8 QTLs each).
Mineral content was the second-highest category in the number of QTLs, which can be attributed to the substantial genetic diversity inherent in mineral nutrient concentrations associated with wild emmer (Cakmak et al., 2004; Peleg et al., 2008). A known QTL called Gpc-B1 has enhanced both GPC and mineral content upon introgression into modern wheat. Initially identified by Joppa and Cantrell (1990) on chromosome 6B in wild emmer lines FA-15-3 and F-28-8-3 from Israel, Gpc-B1 has proven its efficacy. Research by Distelfeld et al. (2007) has shown that recombinant chromosome substitution lines (RSLs) carrying the wild Gpc-B1 allele from the cross “DIC-6B” x LDN exhibited, on average, 12%, 18%, and 29% higher concentrations of Zn, Fe, and Mn, respectively, in the grain compared to LDN. Furthermore, Peleg et al. (2009a) identified 38 stable QTLs associated with the wild alleles from G18-16 within a RIL population. These QTLs explained variations in grain mineral nutrient concentrations ranging from 0.7% to 19.2%. In the current study, most of the collected QTLs related to mineral content were located on chromosomes 6B (40 QTLs), 2A (34 QTLs), and 7A (32 QTLs).
Abiotic-related traits comprised the next category, followed by disease-related traits with only FHB as a trait of interest. The number of QTLs associated with abiotic-related traits is due to the focus of many studies on identifying genome locations that confer tolerance to drought conditions because of the global warming impact on crop productivity (Shew et al., 2020). Wild emmer can grow under dry and saline conditions and it has promising genes allowing to cope with these environmental constraints (Peng et al., 2003). According to our data, most of the QTLs for abiotic-related traits were on chromosomes 5A (35 QTLs) and 5B (33 QTLs). Peleg et al. (2009b) identified 59 QTLs in the wild emmer accession G18-16 related to better adaptability of the crop grown under drought conditions from an original RIL population (durum LDN × G18-16). The major genomic regions associated with productivity and drought-adaptability traits were identified on chromosomes 2A, 4A, 5A, and 7B.
FHB is a severe fungal disease-causing significant yield losses, quality deterioration, and mycotoxin contamination in small grains, particularly in crops like wheat, with a pronounced impact on durum wheat. Breeding for FHB resistance poses challenges due to the polygenic nature of the trait and the substantial influence of genotype by environment interaction. Burstmayr et al. (2020) underscore the importance of considering genetic variation and employing appropriate tools for identifying genotypes for this trait in the wheat breeding process. Two distinct types of resistance have been identified: Type 1, which addresses initial infection resistance, and Type 2, which is the resistance of the spread within the spike. Type 2 resistance is considered less susceptible to environmental variations and a more reliable indicator of FHB resistance (Buerstmayr et al., 2013). Wild emmer QTLs have been related to both FHB resistance (Otto et al., 2002; Chen et al., 2007; Gladysz et al., 2007; Buerstmayr et al., 2013) and susceptibility (Garvin et al., 2009), serving as a potential resource for enhancing durum wheat genotypes.
Our study showed that the majority of FHB QTLs were situated on chromosome 2A (15 QTLs), followed by chromosome 6B (six QTLs), and chromosome 3A (four QTLs). On chromosome 3A, the QTL Qfhs.ndsu-3AS derived from wild emmer “Israel A” (Otto et al., 2002; Chen et al., 2007), “Mt. Hermon” (Gladysz et al., 2007), and “Mt. Gerizim #36” (Buerstmayr et al., 2013) imparted Type 2 resistance. All three accessions exhibited a peak position near the Xgwm2 microsatellie marker. Soresi et al. (2017) validated the effectiveness of Qfhs.ndsu-3AS resistance in two Argentinean durum wheat cultivars. Qfhs.ndsu-3A demonstrated a dominant allele interaction, enhancing resistance by 50% for both homozygous and heterozygous genotypes.
Garvin et al. (2009) identified specific QTLs on the long arm of chromosome 2A within the wild emmer accession “Israel A,” precisely located between Xgwm558 and Xgwm445, covering an approximate distance of 22 cM. Their findings suggested that chromosome 2A contributes to increased susceptibility to FHB. One hypothesis proposed by those researchers was that the genes present on chromosome 2A might mitigate or suppress the effect of FHB resistance conditioned by Qfhs.ndsu 3AS. In a related study, Faris et al. (2014), exploring the association between spike morphology and FHB in wild emmer, reported a QTL for spikelet numbers per spike (QSpn.fcu-2A) that overlapped with the CI of the QTL for FHB susceptibility described by Garvin et al. (2009) on chromosome 2A. It was noted, however, that although they shared the same genomic region, the QTL for spikelet numbers per spike (QSpn.fcu-2A) was excluded as a determinant of FHB susceptibility due to its consideration as an attribute of FHB resistance.
4.3 Meta-QTL and for quality traits, mineral content, abiotic-related traits, and disease-related traits
In this study, we conducted the first MQTL analysis for multiple polygenic traits in wild emmer, and we grouped a total of 395 previously identified QTLs into 43 MQTLs. The results demonstrated a reduction of existing QTL information for polygenic traits, aligning with findings by Khahani et al. (2021), indicating that MQTL analysis enhances precision in detecting candidate genes by narrowing down the genomic regions for traits of interest. The average CI of the MQTLs was much narrower than the average CI of the known QTLs. Specifically, the average CI of the MQTLs was 4.7-fold lower than the initial average CI (22.6 cM). This reduction in CI means increased accuracy and facilitates a more focused exploration of promising candidate genes within each MQTL (Sandhu et al., 2021). This refined approach provides a valuable resource for further allele introgression in wheat breeding.
The MQTL, specifically dicoccoides_MQTL_7A.2, demonstrated clear strength by encompassing the largest number of associated QTLs (37) from diverse environmental backgrounds. In general, only a limited number of MQTLs were specifically linked to a particular category; for quality, there were four of 43 MQTLs, and for abiotic-related traits, two of 43 MQTLs exhibited category specificity. The remaining 37 MQTLs exhibited a polygenic trait from various categories. Interestingly, all MQTLs involving mineral content traits were associated with traits from other categories, primarily quality and abiotic-related traits. This information holds significance for wheat breeding programs, as the identified MQTLs were linked to multiple polygenic traits. This versatility is important for potential enhancements in productivity and overall quality, addressing key and challenging traits in wheat breeding.
Previous MQTL studies in durum wheat have shown similar co-localization compared to the ones generated in this research. Nine MQTLs found by Soriano et al. (2021) were closer to or overlapped the positions of the ones found in this study in chromosomes 1A (one MQTL; peak 46.1 cM), 1B (one MQTL; peak 29.3 cM), 2B (two MQTLs; peaks 59.0 and 147.5 cM), 4B (one MQTL; peak 17.1 cM), 5A (two MQTLs; peaks 48.6 and 143.2 cM), and 5B (two MQTLs; peaks 122.2 and 139.0 cM). Ten MTQLs identified by Arriagada et al. (2022) were closer to or overlapped the positions of the ones encountered in this research in chromosomes 1A (one MQTL; peak 119.2 cM), 1B (one MQTL; peak 71.5 cM), 2A (two MQTLs; peak 139.1 and 139.3 cM), 2B (two MQTLs; peak 56.1 and 51.04 cM), 3A (one MQTL; peak 75.8 cM), 5A (two MTQL; peak 36.6 cM and 102.83), 5B (one MQTL; peak 44.5 cM), and 6B (one MQTL; peak 74.1 cM). Arriagada et al. (2022) identified some of these MQTLs under rainfed and/or irrigated conditions of durum wheat. Except for the MQTL found in chromosomes 2A (one MQTL), 2B (one MTQL), 3A (one MQTL), and 5B (one MTQL), the rest were located under rainfed conditions, and all of them related to yield traits. One genomic region in chromosome 2B was similar and closer in all three studies reported by Soriano et al. (2021), durumMQTL2B.2 (peak 59.0 cM) that was associated with grain selenium yield and GPC; by Arriagada et al. (2022), yield_MQTL2B.1_I (peak 56.12 cM) that was associated with TKW and HI; and in the present study, dicoccoides_MQTL_2B.1 (peak 50.99 cM), which associated with HI, GY, and CL. Although no similar genes were identified in this genomic region, the MQTLs with abiotic and yield-related traits under adverse growing conditions can be considered strong genomic regions for wheat breeding programs to maintain or increase yield under drought conditions, being a powerful resource in the current worldwide scenario of climate change.
4.4 Candidate genes identified for the stable MQTLs
Since this is the first work that tackles multiple traits in wild emmer, the obtained genes of interest are valuable for their study and potential use by utilizing the durum wheat reference genome.
In particular, on chromosome 1A, the MQTL_1A.1 for GCaC, GFeC, and FLRI was associated with a beta-1,3-galactosyltransferase gene involved in the transfer of a galactose to a terminal GlcNAc residue in β-1,3-linkage (Rathan et al., 2022). Another association with genes involved in the cell wall composition, the glycosyltransferase STELLO2, was detected again on chromosome 1A associated with MQTL_1A.2 (Gómez-Espejo et al., 2022). On chromosome 1A, a gene was identified, the suppressor of the PHYTOCHROME A (PHYA), which is involved in regulating mature plant development and related to TKW and GKC (Garg et al., 2006). Another correlation with TKW and GKC was reported with MQTL on chromosome 2A and the gene kinesin-like protein KIN-14l, which regulates grain length and PH by affecting expression levels of genes involved in GA synthesis and response (Wu et al., 2014). An important association was detected on chromosome 2A with the CRK and FLRI abiotic-related trait. Many studies suggest the involvement of CRK proteins in plant development, cell death, immunity, and responses to abiotic stresses (Shumayla et al., 2019). A gene related to carbohydrate metabolism, the alpha-L-fucosidase 2-like was detected on chromosome 4A and co-localized with MQTL for wheat quality. The literature reported the importance of this gene in influencing floral organ differentiation, cell wall biogenesis and degradation, and spike differentiation (Zhu et al., 2016). A BLAST search revealed an association with a candidate gene, aminopeptidase M1-B, involved in plant developmental processes such as embryonic, vegetative, and reproductive development in agreement with Maulana et al. (2021) in wheat.
Another relevant association was detected on chromosome 6B between a MQTL controlling different traits and the glucose-6-phosphate 1-dehydrogenase gene, which is involved in regulating the oxidative pentose phosphate pathway. This gene plays a central role during nitrate assimilation in heterotrophic tissues, contributing to nitrogen metabolism (Esposito, 2016).
The last association was reported on chromosome 7B between the xyloglucan endotransglucosylase/hydrolase protein 24-like gene and GPC, GMgC, and GSC. The xyloglucan endoglycosylases/hydrolases proteins are involved in constructing and remodeling cell wall structures and play an essential role in regulating cell wall extensibility (Han et al., 2023).
5 Conclusions
This comprehensive study was the first work that analyzed 852 already known QTLs across 19 biparental populations of wild emmer, shedding light on the genetic basis of 41 selected traits. The traits were reported according to their category as quality traits, mineral content, abiotic-related traits, and disease-related traits. These QTLs were distributed across all 14 chromosomes and exhibited a wide range of phenotypic variation (0.001 to 0.63), with GPC and TKW emerging as the traits with more QTLs reported in the literature.
Overall, 43 MQTLs were determined, providing a condensed view of stable genomic regions associated with polygenic traits. These MQTLs exhibited narrower CIs than known QTLs, with an average of 4.7-folds lower. The dicoccoides_MQTL_7A.2 had the most QTLs associated with a total of 37 QTLs from different environmental backgrounds, reflecting the strength of the found MQTLs. On the other hand, there was a genomic region, the dicoccoides_MQTL_2B.1 in chromosome 2B, similar to two other studies: Soriano et al. (2021), durumMQTL2B.2 (peak 59.0 cM) and Arriagada et al. (2022), yield_MQTL2B.1_I (peak 56.12 cM). This offers valuable insights for wheat breeding programs since MTQLs with abiotic and yield-related traits can be considered strong genomic regions to maintain or increase yield under stressful conditions. The obtained MQTLs represent regions rich in candidate genes, including those related to carbohydrate metabolism, plant development, and stress responses. This research underscores the significance of MQTL analysis in harnessing the genetic potential of wild emmer to enhance wheat productivity and quality in the face of evolving sustainable agricultural challenges and climate change.
Data availability statement
The original contributions presented in the study are included in the article/Supplementary Material. Further inquiries can be directed to the corresponding authors.
Author contributions
PC: Conceptualization, Data curation, Formal Analysis, Investigation, Writing – original draft, Writing – review & editing. AS: Conceptualization, Funding acquisition, Supervision, Writing – review & editing. OA: Data curation, Formal Analysis, Writing – review & editing. IvM: Writing – review & editing. IM: Writing – review & editing. CA: Writing – review & editing. AG: Writing – review & editing.
Funding
The author(s) declare financial support was received for the research, authorship, and/or publication of this article. This article was funded by the Agencia Nacional de Investigación y Desasrrollo de Chile (ANID) grant number 1210092, Fondecyt Regular (AS, IvM, and CA).
Conflict of interest
The authors declare that the research was conducted in the absence of any commercial or financial relationships that could be construed as a potential conflict of interest.
Publisher’s note
All claims expressed in this article are solely those of the authors and do not necessarily represent those of their affiliated organizations, or those of the publisher, the editors and the reviewers. Any product that may be evaluated in this article, or claim that may be made by its manufacturer, is not guaranteed or endorsed by the publisher.
Supplementary material
The Supplementary Material for this article can be found online at: https://www.frontiersin.org/articles/10.3389/fpls.2024.1305196/full#supplementary-material
References
Abbo, S., Pinhasi van-Oss, R., Gopher, A., Saranga, Y., Ofner, I., Peleg, Z. (2014). Plant domestication versus crop evolution: A conceptual framework for cereals and grain legumes. Trends Plant Sci. 19, 351–360. doi: 10.1016/j.tplants.2013.12.002
Arcade, A., Labourdette, A., Falque, M., Mangin, B., Chardon, F., Charcosset, A., et al. (2004). BioMercator: integrating genetic maps and QTL towards Discovery of candidate genes. Bioinformatics 20, 2324–2326. doi: 10.1093/bioinformatics/bth230
Arriagada, O., Gadaleta, A., Marcotuli, I., Maccaferri, M., Campana, M., Reveco, S., et al. (2022). A comprehensive meta-QTL analysis for yield-related traits of durum wheat (Triticum turgidum L. var. durum) grown under different water regimes. Front. Plant Sci. 13. doi: 10.3389/fpls.2022.984269
Arriagada, O., Marcotuli, I., Gadaleta, A., Schwember, A. R. (2020). Molecular mapping and genomics of grain yield in durum wheat: a review. Int. J. Mol. Sci. 21, 7021. doi: 10.3390/ijms21197021
Avni, R., Nave, M., Eilam, T., Sela, H., Alekperov, C., Peleg, Z., et al. (2014). Ultra-dense genetic map of durum wheat × wild emmer wheat developed using the 90K iSelect SNP genotyping assay. Mol. Breed. 34, 1549–1562. doi: 10.1007/s11032-014-0176-2
Avni, R., Oren, L., Shabtay, G., Assili, S., Pozniak, C., Hale, I., et al. (2018). Genome based meta-QTL analysis of grain weight in tetraploid wheat identifies rare alleles of GRF4 associated with larger grains. Genes 9, 636. doi: 10.3390/genes9120636
Beres, B. L., Rahmani, E., Clarke, J. M., Grassini, P., Pozniak, C. J., Geddes, C. M., et al. (2020). A systematic review of durum wheat: Enhancing production systems by exploring genotype, environment, and management (G × E × M) synergies. Front. Plant Sci. 11. doi: 10.3389/fpls.2020.568657
Bhusal, N., Sharma, P., Sareem, S., Sarial, A. K. (2018). Mapping QTLs for chlorophyll content and chlorophyll fluorescence in wheat under heat stress. Biol. Plantarum 62, 721–731. doi: 10.1007/s10535-018-0811-6
Blanco, A., Mangini, G., Giancaspro, A., Give, S., Colasuonno, P., Simeone, R., et al. (2012). Relationships between grain protein content and grain yield components through quantitative trait locus analyses in a recombinant inbred line population derived from two elite durum wheat cultivars. Mol. Breed. 30, 79–92. doi: 10.1007/s11032-011-9600-z
Buerstmayr, M., Alimari, A., Steiner, B., Buerstmayr, H. (2013). Genetic mapping of QTL for resistant to Fusarium head blight spread (type 2 resistance) in a Triticum dicoccoides × Triticum durum backcross-derived population. Theor. Appl. Genet. 126, 2825–2834. doi: 10.1007/s00122-013-2174-x
Buerstmayr, M., Huber, K., Heckmann, J., Steiner, B., Nelson, J. C., Buerstmayr, H. (2012). Mapping of QTL for Fusarium head blight resistance and morphological and developmental traits in three backcross populations derived from Triticum dicoccum x Triticum durum. Theor. Appl. Genet. 125, 1751–1765. doi: 10.1007/s00122-012-1951-2
Burstmayr, M., Steiner, B., Buerstmayr, H. (2020). Breeding for Fusarium head blight resistance in wheat- progress and challenges. Plant Breed. 139, 429–454. doi: 10.1111/pbr.12797
Cabas-Lühmann, P., Arriagada, O., Matus, I., Marcotuli, I., Gadaleta, A., Schwember, A. (2023). Comparison of durum with ancient tetraploid wheats from an agronomical, chemical, nutritional, and genetic standpoints: a review. Euphytica 219, 61. doi: 10.1007/s10681-023-03188-z
Cakmak, I., Pfeiffer, W. H., McClafferty, B. (2010). Biofortification of durum wheat with zinc and iron. Cereal Chem. 12, 452. doi: 10.1094/CCHEM-87-1-0010
Cakmak, I., Torun, A., Millet, E., Feldman, M., Fahima, T., Korol, A., et al. (2004). Triticum dicoccoides: an important genetic resource for increasing zinc and iron concentration in modern cultivated wheat. Soil Sci. Plant Nutr. 50, 1047–1054. doi: 10.1080/00380768.2004.10408573
Chardon, F., Virlon, B., Moreau, L., Falque, M., Joets, J., Decousset, L., et al. (2004). Genetic architecture of flowering time in maize as inferred from quantitative trait loci meta-analysis and synteny conservation with the rice genome. Genetics 168, 2169–2185. doi: 10.1534/genetics.104.032375
Chen, X., Faris, J. D., Hu, J., Stack, R. W., Adhikari, T., Elias, E. M., et al. (2007). Saturation and comparative mapping of a major Fusarium head blight resistance QTL in tetraploid wheat. Mol. Breed. 19, 113–124. doi: 10.1007/s11032-006-9049-7
Chen, L., Li, H., Liu, Y., Hou, Y. (2021). Genetic identification of a major, novel and stably expressed QTL for effective tiller number from tetraploid wheat. Biotechnol. Biotechnol. Equip. 35, 1538–1545. doi: 10.1080/13102818.2021.1996271
Cobb, J. N., Biswas, P. S., Platten, J. D. (2019). Back to the future: Revisiting MAS as a tool for modern plant breeding. Theor. Appl. Genet. 132, 647–667. doi: 10.1007/s00122-018-3266-4
Colasuonno, P., Marcotuli, I., Gadaleta, A., Soriano, J. M. (2021). From genetic maps to QTL cloning: an overview for durum wheat. Plants 10, 315. doi: 10.3390/plants10020315
Deblieck, M., Szilagyi, G., Andrii, F., Saranga, Y., Lauterberg, M., Neumann, K., et al. (2022). Dissection of a grain yield QTL from wild emmer wheat reveals sub-intervals associated with culm length and kernel number. Front. Genet. 13. doi: 10.3389/fgene.2022.955295
Distelfeld, A., Cakmak, I., Peleg, Z., Ozturk, L., Yazici, A. M., Budak, H., et al. (2007). Multiple QTL effects of wheat Gpc-B1 locus on grain protein and micronutrient concentrations. Physiol. Plantarum 129, 635–643. doi: 10.1111/j.1399-3054.2006.00841.x
Esposito, S. (2016). Nitrogen assimilation, abiotic stress and glucose 6-phosphate dehydrogenase: the full circle of reductants. Plants 5, 24. doi: 10.3390/plants5020024
Faris, J. D., Zhang, Z., Garvin, D. F., Xu, S. S. (2014). Molecular and comparative mapping of genes governing spike compactness from wild emmer wheat. Mol. Genet. Genomics 289, 641–651. doi: 10.1007/s00438-014-0836-2
Fatiukha, A., Deblieck, M., Klymiuk, V., Merchuk-Ovnat, L., Peleg, Z., Fahima, T., et al. (2021). Genomic architecture of phenotypic plasticity in response to water stress in tetraploid wheat. Int. J. Mol. Sci. 22, 1723. doi: 10.3390/ijms22041723
Fatiukha, A., Filler, N., Lupo, I., Lidzbarsky, G., Klymiuk, V., Korol, A. B., et al. (2019). Grain protein content and thousand kernel weight QTLs identified in a durum × wild emmer wheat mapping population tested in five environments. Theor. Appl. Genet. 133, 119–131. doi: 10.1007/s00122-019-03444-8
Fatiukha, A., Klymiuk, V., Peleg, Z., Saranga, Y., Cakmak, I., Krugman, T., et al. (2020). Variation in phosphorus and sulfur content shapes the genetic architecture and phenotypic associations within the wheat grain ionome. Plant J. 101, 555–572. doi: 10.1111/tpj.14554
Garg, A. K., Sawers, R. J., Wang, H., Kim, J. K., Walker, J. M., Brutnell, T. P., et al. (2006). Light-regulated overexpression of an Arabidopsis phytochrome A gene in rice alters plant architecture and increases grain yield. Planta 223, 627–636. doi: 10.1007/s00425-005-0101-3
Garvin, D. F., Stack, R., Hansen, J. (2009). Quantitative trait locus mapping of increased Fusarium head blight susceptibility associated with a wild emmer wheat chromosome. Phytopathology 99, 447–452. doi: 10.1094/PHYTO-99-4-0447
Gladysz, C., Lemmens, M., Steiner, B., Buerstmayr, H. (2007). Evaluation and genetic mapping of resistance to Fusarium head blight in Triticum dicoccoides. Isr. J. Plant Sci. 55, 263–266. doi: 10.1560/IJPS.55.3-4.263
Goffinet, B., Gerber, S. (2000). Quantitative trait loci: A meta-analysis. Genetics 155, 463–473. doi: 10.1093/genetics/155.1.463
Golan, G., Oksenberg, A., Peleg, Z. (2015). Genetic evidence for differential selection of grain and embryo weight during wheat evolution under domestication. J. Exp. Bot. 66, 5703–5711. doi: 10.1093/jxb/erv249
Gómez-Espejo, A. L., Sansaloni, C. P., Burgueño, J., Toledo, F. H., Benavides-Mendoza, A., Reyes-Valdés, M. H. (2022). Worldwide selection footprints for drought and heat in bread wheat (Triticum aestivum L.). Plants 11, 2289. doi: 10.3390/plants11172289
Guo, K., Chen, T., Zhang, P., Liu, Y., Chen, Z., Shahinnia, F., et al. (2023). Meta-QTL analysis and in-silico transcriptome assessment for controlling chlorophyll traits in common wheat. Plant Genome 16, e20294. doi: 10.1002/tpg2.20294
Guo, B., Sleper, D. A., Lu, P., Shannon, J. G., Nguyen, H. T., Arelli, P. R. (2006). QTLs associated with resistance to soybean cyst nematode in soybean: Meta-analysis of QTL locations. Crop Sci. 46, 202–208. doi: 10.2135/cropsci2005.04-0036
Gupta, P. K., Balyan, H. S., Sharma, S., Kumar, R. (2020). Genetics of yield, abiotic stress tolerance and biofortification in wheat (Triticum aestivum L.). Theor. Appl. Genet. 133, 1569–1602. doi: 10.1007/s00122-020-03583-3
Han, J., Liu, Y., Shen, Y., Li, W. (2023). A surprising diversity of xyloglucan endotransglucosylase/hydrolase in wheat: new in sight to the roles in drought tolerance. Int. J. Mol. Sci. 24, 9886. doi: 10.3390/ijms24129886
Huang, L., Raats, D., Sela, H., Klymiuk, V., Ladzbarsky, G., Feng, L., et al. (2016). Evolution and adaptation of wild emmer wheat populations to biotic and abiotic stresses. Annu. Rev. Phytopathol. 54, 279–301. doi: 10.1146/annurev-phyto-080614-120254
Joppa, L. R., Cantrell, R. G. (1990). Chromosomal location of genes for grain protein content of wild tetraploid wheat. Crop Sci. 30, 1059–1064. doi: 10.2135/cropsci1990.0011183X003000050021x
Joppa, L. R., Hareland, G. A., Cantrell, R. G. (1991). Quality characteristics of the Langdon durum-dicoccoides chromosome substitution lines. Crop Sci. 31, 1513–1517. doi: 10.2135/cropsci1991.0011183X003100060024x
Khahani, B., Tavakol, E., Shariati, V., Rossini, L. (2021). Meta-QTL and ortho-MQTL analyses identified genomic regions controlling rice yield, yield-related traits and root architecture under water deficit conditions. Sci. Rep. 11, 6942. doi: 10.1038/s41598-021-86259-2
Kumar, A., Jain, S., Elias, E. M., Ibrahim, M., Sharma, L. K. (2018). “An overview of QTL identification and marker-assisted selection for grain protein content in wheat,” in Eco-friendly agro-biological techniques for enhancing crop productivity. Eds. Sengar, R., Singh., A. (Singapore: Springer), 245–274.
Kumar, A., Saripalli, G., Jan, I., Kumar, K., Sharma, P. K., Balyan, H. S., et al. (2020). Meta-QTL analysis and identification of candidate genes for drought tolerance in bread wheat (Triticum aestivum L.). Physiol. Mol. Biol. Plants 26, 1713–1725. doi: 10.1007/s12298-020-00847-6
Kuznetsova, E., Shayapova, L., Klimova, E., Nasrullaeva, G., Brindza, J., Stolyarov, M., et al. (2019). Composition, quality characteristics and microstructure of the grain Triticum dicoccum. Slovak J. Food Sci. 13, 933–940. doi: 10.5219/1174
Li, J., Zhang, Z., Liu, Y., Yao, C., Song, W., Xu, X., et al. (2019). Effects of micro sprinkling with different irrigation amount on grain yield and water use efficiency of winter wheat in the North China Plain. Agric. Water Manage. 224, 105736. doi: 10.1016/j.agwat.2019.105736
Liang, W., Zhang, Z., Wen, X., Liao, Y., Liu, Y. (2017). Effect of non-structural carbohydrate accumulation in the stem pre-anthesis on grain filling of wheat inferior grain. Field Crop Res. 211, 66–76. doi: 10.1016/j.fcr.2017.06.016
Liu, J., Huang, L., Li, T., Liu, Y., Yan, Z., Tang, G., et al. (2021). Genome-wide association study for grain micronutrient concentrations in wheat advanced lines derived from wild emmer. Front. Plant Sci. 12. doi: 10.3389/fpls.2021.651283
Liu, H., Mullan, D., Zhang, C., Zhao, S., Li, X., Zhang, A., et al. (2020). Major genomic regions responsible for wheat yield and its components as revealed by meta-QTL and genotype–phenotype association analyses. Planta 252, 4. doi: 10.1007/s00425-020-03466-3
Löffler, M., Schoen, C. C., Miedaner, T. (2009). Revealing the genetic architecture of FHB resistance in hexaploid wheat (Triticum aestivum L.) by QTL meta-analysis. Mol. Breed. 23, 473–488. doi: 10.1007/s11032-008-9250-y
Longin, C. F. H., Ziegler, J., Schweiggert, R., Koehler, P., Carle, R., Würschum, T. (2016). Comparative study of hulled (einkorn, emmer, and spelt) and naked wheats (durum and bread wheat): agronomic performance and quality traits. Crop Sci. 56, 302–311. doi: 10.2135/cropsci2015.04.0242
Maccaferri, M., Harris, N. S., Twardziok, S. O., Pasam, R. K., Gundlach, H., Spannagl, M., et al. (2019). Durum wheat genome highlights past domestication signatures and future improvement targets. Nat. Genet. 51, 885–895. doi: 10.1038/s41588-019-0381-3
Maccaferri, M., Ricci, A., Salvi, S., Milner, S. G., Noli, E., Martelli, P. L., et al. (2015). A high-density, SNP-based consensus map of tetraploid wheat as a bridge to integrate durum and bread wheat genomics and breeding. Plant Biotechnol. J. 13, 648–663. doi: 10.1111/pbi.12288
Marcotuli, I., Soriano, J. M., Gadaleta, A. (2022). A consensus map for quality traits in durum wheat based on genome-wide association studies and detection of ortho-meta QTL across cereal species. Front. Genet. 13. doi: 10.3389/fgene.2022.982418
Maulana, F., Huang, W., Anderson, J. D., Kumssa, T. T., Ma, X.-F. (2021). Genome-wide association mapping of seedling vigor and regrowth vigor in winter wheat. Crops 1, 153–165. doi: 10.3390/crops1030015
Merchuk-Ovnat, L., Barak, V., Fahima, T., Ordon, F., Lidzbarsky, G. A., Krugman, T., et al. (2016a). Ancestral QTL alleles from wild emmer wheat improve drought resistance and productivity in modern wheat cultivars. Front. Plant Sci. 7. doi: 10.3389/fpls.2016.00452
Merchuk-Ovnat, L., Fahima, T., Ephrath, J. E., Krugman, T., Saranga, Y. (2017). Ancestral QTL alleles from wild emmer wheat enhance root development under drought in modern wheat. Front. Plant Sci. 8. doi: 10.3389/fpls.2017.00703
Merchuk-Ovnat, L., Fahima, T., Krugman, T., Saranga, Y. (2016b). Ancestral QTL alleles from wild emmer wheat improve grain yield, biomass and photosynthesis across environments in modern wheat. Plant Sci. 251, 23–34. doi: 10.1016/j.plantsci.2016.05.003
Miao, Y., Jing, F., Ma, J., Liu, Y., Zhang, P., Chen, T., et al. (2022). Major genomic regions for wheat grain weight as revealed by QTL linkage mapping and meta-analysis. Front. Plant Sci. 13. doi: 10.3389/fpls.2022.802310
Mo, Z., Zhu, J., Wei, J., Zhou, J., Xu, Q., Tang, H., et al. (2021). The 55K SNP-Based exploration of QTLs for spikelet number per spike in a tetraploid wheat (Triticum turgidum L.) population: chinese landrace “Ailanmai” × wild emmer. Front. Plant Sci. 12, 732837. doi: 10.3389/fpls.2021.732837
Otto, C. D., Kianian, S. F., Elias, E. M., Stack, R. W., Joppa, L. R. (2002). Genetic dissection of a major Fusarium head blight QTL in tetraploid wheat. Plant Mol. Biol. 48, 625–632. doi: 10.1023/A:1014821929830
Peleg, Z., Cakmak, I., Ozturk, L., Yazici, A., Jun, Y., Budak, H., et al. (2009a). Quantitative trait loci conferring grain mineral nutrient concentrations in durum wheat × wild emmer wheat RIL population. Theor. Appl. Genet. 119, 353–369. doi: 10.1007/s00122-009-1044-z
Peleg, Z., Fahima, T., Abbo, S., Krugman, T., Nevo, E., Yakir, D., et al. (2005). Genetic diversity for drought resistance in wild emmer wheat and its ecogeographical associations. Plant Cell Environ. 28, 176–191. doi: 10.1111/j.1365-3040.2005.01259.x
Peleg, Z., Fahima, T., Korol, A., Abbo, S., Saranga, Y. (2011). Genetic analysis of wheat domestication and evolution under domestication. J. Exp. Bot. 62, 5051–5061. doi: 10.1093/jxb/err206
Peleg, Z., Fahima, T., Krugman, T., Abbo, S., Yakir, D. A. N., Korol, A. B., et al. (2009b). Genomic dissection of drought resistance in durum wheat× wild emmer wheat recombinant inbreed line population. Plant Cell Environ. 32, 758–779. doi: 10.1111/j.1365-3040.2009.01956.x
Peleg, Z., Saranga, Y., Suprunova, T., Ronin, Y., Röder, M., Kilian, A., et al. (2008). High-density genetic map of durum wheat × wild emmer wheat based on SSR and DArT markers. Theor. Appl. Genet. 117, 103–115. doi: 10.1007/s00122-008-0756-9
Peng, J., Sun, D., Nevo, E. (2011). Wild emmer wheat, Triticum dicoccoides, occupies a pivotal position in wheat domestication process. Aust. J. Crop Sci. 5, 1127–1143.
Peng, J. H., Ronin, Y., Fahima, T., Röder, M. S., Li, Y., Nevo, E., et al. (2003). Domestication quantitative trait loci in Triticum dicoccoides, the progenitor of wheat. Proc. Natl. Acad. Sci. U. S. A. 100, 2489–2494. doi: 10.1073/pnas.252763199
Rathan, N. D., Krishna, H., Ellur, R. K., Sehgal, D., Govindan, V., Ahlawat, A., et al. (2022). Genome-wide association study identifies loci and candidate genes for grain micronutrients and quality traits in wheat (Triticum aestivum L.). Sci. Rep. 12, 7037. doi: 10.1038/s41598-022-10618-w
Roselló, M., Royo, C., Álvaro, F., Villegas, D., Nazco, R., Soriano, J. M. (2018). Pasta-making quality qtlome from mediterranean durum wheat landraces. Front. Plant Sci. 9. doi: 10.3389/fpls.2018.01512
Said, J. I., Lin, Z., Zhang, X., Song, M., Zhang, J. (2013). A comprehensive meta QTL analysis for fiber quality, yield, yield related and morphological traits, drought tolerance, and disease resistance in tetraploid cotton. BMC Genomics 14, 776. doi: 10.1186/1471-2164-14-776
Saini, D. K., Chahal, A., Pal, N., Srivastava, P., Gupta, P. K. (2022b). Meta-analysis reveals consensus genomic regions associated with multiple disease resistance in wheat (Triticum aestivum L.). Mol. Breed. 42, 11. doi: 10.1007/s11032-022-01282-z
Saini, D. K., Chopra, Y., Pal, N., Chahal, A., Srivastava, P., Gupta, P. K. (2021). Meta-QTL, ortho-MQTL and candidate genes for nitrogen use efficiency and root system architecture in bread wheat (Triticum aestivum L.). Physiol. Mol. Biol. Plants 27, 2245–2267. doi: 10.1007/s12298-021-01085-0
Saini, D. K., Chopra, Y., Singh, J., Sandhu, K. S., Kumar, A., Bazzer, S., et al. (2022a). Comprehensive evaluation of mapping complex traits in wheat using genome-wide association studies. Mol. Breed. 42, 1. doi: 10.1007/s11032-021-01272-7
Saini, P., Sheikh, I., Saini, D. K., Mir, R. R., Dhaliwal, H. S., Tyagi, V. (2022c). Consensus genomic regions associated with grain protein content in hexaploid and tetraploid wheat. Front. Genet. 13. doi: 10.3389/fgene.2022.1021180
Sandhu, N., Pruthi, G., Prakash Raigar, O., Singh, M. P., Phagna, K., Kumar, A., et al. (2021). Meta-qtl analysis in rice and cross-genome talk of the genomic regions controlling nitrogen use efficiency in cereal crops revealing phylogenetic relationship. Front. Genet. 12. doi: 10.3389/fgene.2021.807210
Shew, A. M., Tack, J. B., Nalley, L. L., Chaminuka, P. (2020). Yield reduction under climate warming varies among wheat cultivars in South Africa. Nat. Commun. 11:4408. doi: 10.1038/s41467-020-18317-8
Shumayla, T. S., Upadhyay, S.K. (2019). “Receptor-like kinases and environmental stress in plants,” in Molecular approaches in plant biology and environmental challenges. Eds. Singh, S., Upadhyay, S., Pandey, A., Kumar, S. (Springer, Singapore). Energy, environment, and sustainability.
Singh, R., Saripalli, G., Gautam, T., Kumar, A., Batra, R., Kumar, J., et al. (2022). Meta-QTL, ortho-MetaQTL and candidate genes for grain Fe and Zn contents in wheat (Triticum aestivum L.). Physiol. Mol. Biol. Plants 28, 637–650. doi: 10.1007/s12298-022-01149-9
Soresi, D., Zappacosta, D., Garayalde, A., Irigoyen, I., Basualdo, J., Carrera, A. (2017). A valuable QTL for Fusarium head blight resistance from Triticum turgidum L. ssp. dicoccoides has a stable expression in durum wheat cultivars. Cereal Res. Commun. 45, 234–247. doi: 10.1556/0806.45.2017.007
Soriano, J. M., Alvaro, F. (2019). Discovering consensus genomic regions in wheat for root-related traits by QTL meta-analysis. Sci. Rep. 9. doi: 10.3389/s41598-019-47038-2
Soriano, J. M., Colasuonno, P., Marcotuli, I., Gadaleta, A. (2021). Meta-QTL analysis and identification of candidate genes for quality, abiotic and biotic stress in durum wheat. Sci. Rep. 11, 11877. doi: 10.1038/s41598-021-91446-2
Subira, J., Peña, R. J., Álvaro, F., Ammar, K., Ramdani, A., Royo, C. (2014). Breeding progress in the pasta–making quality of durum wheat cultivars released in Italy and Spain during the 20th Century. Crop Pasture Sci. 65, 16–26. doi: 10.1071/CP13238
Thanh, P. H., Vladutu, C., Kianian, S. F., Thanh, P. T., Ishii, T., Nitta, M., et al. (2013). Molecular genetic analysis of domestication traits in emmer wheat. i: map construction and QTL analysis using an F2 population. Biotechnol. Biotechnol. Equip. 27, 3627–3637. doi: 10.5504/BBEQ.2013.0008
Tyagi, S., Mir, R. R., Balyan, H. S., Gupta, P. K. (2015). Interval mapping and meta-QTL analysis of grain traits in common wheat (Triticum aestivum L.). Euphytica 201, 367–380. doi: 10.1007/s10681-014-1217-y
Uauy, C., Distelfeld, A., Fahima, T., Blechl, A., Dubcovsky, J. (2006). A NAC gene regulating senescence improves grain protein, zinc, and iron content in wheat. Science 314, 1298–1301. doi: 10.1126/science.1133649
Velu, G., Tutus, Y., Gomez-Becerra, H. F., Hao, Y., Demir, L., Kara, R., et al. (2017). QTL mapping for grain zinc and iron concentrations and zinc efficiency in a tetraploid and hexaploid wheat mapping populations. Plant Soil 411, 81–99. doi: 10.1007/s11104-016-3025-8
Venske, E., dos Santos, R. S., da Farias, D. R., Rother, V., da Maia, L. C., Pegoraro, C., et al. (2019). Meta-analysis of the QTLome of fusarium head blight resistance in bread wheat: refining the current puzzle. Front. Plant Sci. 10. doi: 10.3389/fpls.2019.00727
Veyrieras, J. B., Goffinet, B., Charcosset, A. (2007). MetaQTL: A package of new computational methods for the meta-analysis of QTL mapping experiments. BMC Bioinf. 8, 49. doi: 10.1186/1471-2105-8-49
Wang, Z., Dhakal, S., Cerit, M., Wang, S., Rauf, Y., Yu, S., et al. (2022). QTL mapping of yield components and kernel traits in wheat cultivars TAM 112 and Duster. Front. Plant Sci. 13. doi: 10.3389/fpls.2022.1057701
Wang, K., Fu, B. (2020). Inter-relationships between test weight, thousand kernel weight, kernel size distribution and their effects on durum wheat milling, semolina composition and pasta processing quality. Foods 9, 1308. doi: 10.3390/foods9091308
Wu, T., Shen, Y., Zheng, M., Yang, C., Chen, Y., Feng, Z., et al. (2014). Gene SGL, encoding a kinesin-like protein with transactivation activity, is involved in grain length and plant height in rice. Plant Cell Rep. 33, 235–244. doi: 10.1007/s00299-013-1524-0
Xu, Y.-F., Li, S.-S., Li, L.-H., Ma, F.-F., Fu, X.-Y., Shi, Z.-L., et al. (2017). QTL mapping for yield and photosynthetic related traits under different water regimes in wheat. Mol. Breed. 37, 1–18. doi: 10.1007/s11032-016-0583-7
Yan, J., Xue, W.-T., Yang, R.-Z., Qin, H.-B., Fahima, T., Cheng, J.-P. (2018). Quantitative trait loci conferring grain selenium nutrient in durum wheat × wild emmer wheat RIL population. Czech J. Genet. Plant Breed. 54, 52–58. doi: 10.17221/112/2016-CJGPB
Zhang, J. F., Gill, H. S., Halder, J., Brar, N. K., Ali, S., Bernardo, A., et al. (2022). Multi-locus genome wide association studies to characterize fusarium head blight (FHB) resistance in hard Winter wheat. Front. Plant Sci. 13. doi: 10.3389/fpls.2022.946700
Zhang, L., Liu, D. C., Guo, X. L., Yang, W. L., Sun, J. Z., Wang, D. W., et al. (2010). Genomic distribution of quantitative trait loci for yield and yield-related traits in common wheat. J. Integr. Plant Biol. 52, 996–1007. doi: 10.1111/j.1744-7909.2010.00967.x
Zheng, T., Hua, C., Li, L., Sun, Z., Yuan, M., Bai, G., et al. (2021). Integration of meta-QTL discovery with omics: Towards a molecular breeding platform for improving wheat resistance to Fusarium head blight. Crop J. 9, 739–749. doi: 10.1016/j.cj.2020.10.006
Zhou, J., Li, C., You, J., Tang, H., Mu, Y., Jiang, Q., et al. (2021). Genetic identification and characterization of chromosomal regions for kernel length and width increase from tetraploid wheat. BMC Genomics 22, 706. doi: 10.1186/s12864-021-08024-z
Keywords: meta-QTL analysis, QTL, wild emmer, quality traits, mineral composition, abiotic-related traits, disease-related traits
Citation: Cabas-Lühmann P, Schwember AR, Arriagada O, Marcotuli I, Matus I, Alfaro C and Gadaleta A (2024) Meta-QTL analysis and candidate genes for quality traits, mineral content, and abiotic-related traits in wild emmer. Front. Plant Sci. 15:1305196. doi: 10.3389/fpls.2024.1305196
Received: 10 October 2023; Accepted: 26 February 2024;
Published: 14 March 2024.
Edited by:
Satish Kumar, Indian Council of Agricultural Research (ICAR), IndiaReviewed by:
Hossein Sabouri, Gonbad Kavous University, IranJindong Liu, Chinese Academy of Agricultural Sciences, China
Satinder Kaur, Punjab Agricultural University, India
Copyright © 2024 Cabas-Lühmann, Schwember, Arriagada, Marcotuli, Matus, Alfaro and Gadaleta. This is an open-access article distributed under the terms of the Creative Commons Attribution License (CC BY). The use, distribution or reproduction in other forums is permitted, provided the original author(s) and the copyright owner(s) are credited and that the original publication in this journal is cited, in accordance with accepted academic practice. No use, distribution or reproduction is permitted which does not comply with these terms.
*Correspondence: Andrés R. Schwember, aschwember@uc.cl; Agata Gadaleta, agata.gadaleta@uniba.it