- 1National Technology Innovation Center of Synthetic Biology, Tianjin, China
- 2Biodesign Center, Key Laboratory of Systems Microbial Biotechnology, Tianjin Institute of Industrial Biotechnology, Chinese Academy of Sciences, Tianjin, China
- 3Key Laboratory of Systems Microbial Biotechnology, Tianjin Institute of Industrial Biotechnology, Chinese Academy of Sciences, Tianjin, China
The systematical characterization and understanding of the metabolic behaviors are the basis of the efficient plant metabolic engineering and synthetic biology. Genome-scale metabolic networks (GSMNs) are indispensable tools for the comprehensive characterization of overall metabolic profile. Here we first constructed a GSMN of tobacco, which is one of the most widely used plant chassis, and then combined the tobacco GSMN and multiomics analysis to systematically elucidate the impact of in-vitro cultivation on the tobacco metabolic network. In-vitro cultivation is a widely used technique for plant cultivation, not only in the field of basic research but also for the rapid propagation of valuable horticultural and pharmaceutical plants. However, the systemic effects of in-vitro cultivation on overall plant metabolism could easily be overlooked and are still poorly understood. We found that in-vitro tobacco showed slower growth, less biomass and suppressed photosynthesis than soil-grown tobacco. Many changes of metabolites and metabolic pathways between in-vitro and soil-grown tobacco plants were identified, which notably revealed a significant increase of the amino acids content under in-vitro condition. The in silico investigation showed that in-vitro tobacco downregulated photosynthesis and primary carbon metabolism, while significantly upregulated the GS/GOGAT cycle, as well as producing more energy and less NADH/NADPH to acclimate in-vitro growth demands. Altogether, the combination of experimental and in silico analyses offers an unprecedented view of tobacco metabolism, with valuable insights into the impact of in-vitro cultivation, enabling more efficient utilization of in-vitro techniques for plant propagation and metabolic engineering.
Introduction
Plant metabolic characterization and plant engineering are usually incomprehensive and inefficient, partly because of insufficient information on metabolic flux distributions and poor understanding of metabolic behaviors at the network level (Arya et al., 2020; Lynch et al., 2021). To systematically and globally characterize the metabolic behaviors of plants, it is necessary to reconstruct a genome-scale metabolic networks (GSMNs) that incorporate comprehensive catalytic transformations., which have been utilized to elucidate cellular metabolism and guide metabolic engineering to overproduce desired compounds (Chatsurachai et al., 2012; Kim et al., 2015a; Zhang et al., 2017). In the last decade, many efforts have been made to construct GSMNs for the important plant species, such as Arabidopsis (Cheung et al., 2013; Robaina et al., 2017; Scheunemann et al., 2018), rice(Lakshmanan et al., 2013; Chatterjee and Kundu, 2015; Chatterjee et al., 2017), maize (Simons et al., 2014; Seaver et al., 2015; Bogart and Myers, 2016), tomato (Colombié et al., 2015; Yuan et al., 2016; Shameer et al., 2020), and potato (Botero et al., 2018). However, there is still no network model of tobacco, although it is one of the most important economic crops and a model for fundamental biology as well as an excellent chassis for plant synthetic biology. Tobacco has many advantages, such as high biomass, versatile metabolism (more than 4,000 producible chemical compounds), high protein expression (up to more than 40% of its biomass), mature agricultural and laboratory culture systems, as well as efficient techniques for rapid transient expression and chloroplast transformation (Bock, 2014; Azizi et al., 2015). Tobacco has been widely used for the heterologous synthesis of dozens of natural plant compounds, such as artemisinin, anthocyanins, etoposide aglycone, and taxadiene-5-ol (Farhi et al., 2011; Lau and Sattely, 2015; Appelhagen et al., 2018; Li et al., 2019; Zhu et al., 2021). In spite of its importance, to our best knowledge, no GSMN of tobacco has been published to date. Tobacco GSMN is desirable and will help to systematically understand and engineer tobacco metabolism. In this study, our first aim was to initially construct a high-quality GSMN for tobacco.
In vitro culture is a widely used technique for plant cultivation. It is an effective means for rapid clonal propagation of many valuable horticultural and pharmaceutical plants, while also offering a conventional and effective experimental system for plant physiology research. However, multiple specific effects of in vitro system on plant physiology could easily be overlooked or underestimated, such as the exogenous sugar supply, high humidity in compact and closed culture vessel, inefficient gas exchange, and sometimes low irradiance (Desjardins, 1995; Pospóšilová et al., 1999; Pospà et al., 2007; Desjardins et al., 2009; Kozai, 2010; Yaseen et al., 2013). The compact space and high humidity will affect the water potential, transpiration rate, stomatal development and function, while sometimes also inducing stress and defense responses (Hsiao, 1973; Casanova et al., 2008; Ivanova and Van Staden, 2010). The poor ventilation can affect the carbon and oxygen balance, photosynthesis and respiration, as well as the accumulation of volatile secondary metabolites (Cournac et al., 1991; De Souza et al., 2008; De Oliveira et al., 2021). The low irradiance could affect light energy capture and utilization, the synthesis of photosynthetic pigments, photomorphogenesis, and biosynthesis of secondary metabolites (Kitaya et al., 1995; Kadleček et al., 2003; Eckstein et al., 2012). The exogenous sugar supply could affect the carbon, energy and osmotic balance, while also serve as the important signaling molecule to alter plant metabolism (Chanemougasoundharam et al., 2004; Choi et al., 2004; Badr et al., 2011; Xiao et al., 2011; Ševčíková et al., 2019). Therefore, the impact of in vitro cultivation on plant metabolism is a complex and multifaceted topic. Many factors and their interactions in in-vitro system could cause metabolic alterations or disturbances, and subsequently influence the overall metabolic profile of the plant. However, few studies embarked on a systemic and global elucidation of overall plant metabolic variations. Therefore, it is unclear what impact in vitro cultivation may have on overall plant metabolism. Genome-scale metabolic networks (GSMNs) are indispensable tools for the systematic characterization of metabolic behaviors. Metabolome and transcriptome analyses could globally elucidate overall metabolic changes and the underlying regulatory mechanisms. Therefore, combination of genome-scale metabolic networks and omics analysis is an excellent method for systematically elucidating the impact of in vitro cultivation on plant metabolism. In plants, omics data were successfully integrated with the Arabidopsis GSMN and rice GSMN to illustrate their metabolite acclimation profiles under different conditions (Töpfer et al., 2013; Töpfer et al., 2014; Lakshmanan et al., 2015). Similarly, we aimed to combine GSMN with omics data to uncover the metabolic heterogeneity across various in vitro culture conditions.
The aim of this research is to systematically elucidate the impact of in vitro cultivation on tobacco metabolism through GSMN-integrated multiomics analysis. Firstly, we initially constructed a genome-scale metabolic model of tobacco. Secondly, we detected the growth and photosynthetic differences between soil-grown tobacco and in-vitro tobacco. Thirdly, metabolome and transcriptome analyses were performed to identify the variations in metabolites and metabolic pathways between in-vitro and soil-grown tobacco plants. To systematically elucidate the impact of in-vitro cultivation on tobacco metabolic network, we combined model and omics data to elucidated metabolic network signatures of in-vitro tobacco plants, including photosynthesis, the Calvin and TCA cycle, as well as sucrose and N metabolism. This work allows us to systematically understand tobacco metabolism and the impact of in vitro cultivation, enabling us to efficiently utilize in-vitro techniques for plant cultivation and metabolic engineering.
Results and discussion
A genome-scale metabolic model of tobacco
To systematically understand and engineer tobacco metabolism, we firstly had to reconstruct a genome-scale metabolic model (GSMN) for tobacco. To our best knowledge, this is the first reported tobacco model. The metabolic network was based on information from Ntabacum_Tn90Cyc (Plant Metabolic Network; https://plantcyc.org/content/ntabacumtn90cyc-3.0), Kyoto Encyclopedia of Genes and Genomes (KEGG), and other literature sources. Our tobacco GEM, named iJTC6240, accounts for 6,240 genes, 2,887 reactions, and 2,905 metabolites localized across five intracellular compartments, including cytosol, mitochondrion, plastid, vacuole, and peroxisome (Figure 1A). Among the 2,887 reactions, 2,619 are involved in biochemical conversions, while 199 are responsible for metabolite transport between the subcellular compartments, and there are 22 reactions that mediate the exchange with the environment. The inclusion of GPR associations in iJTC6240 allowed us to map the transcriptome data to contextualize the model for further analysis.
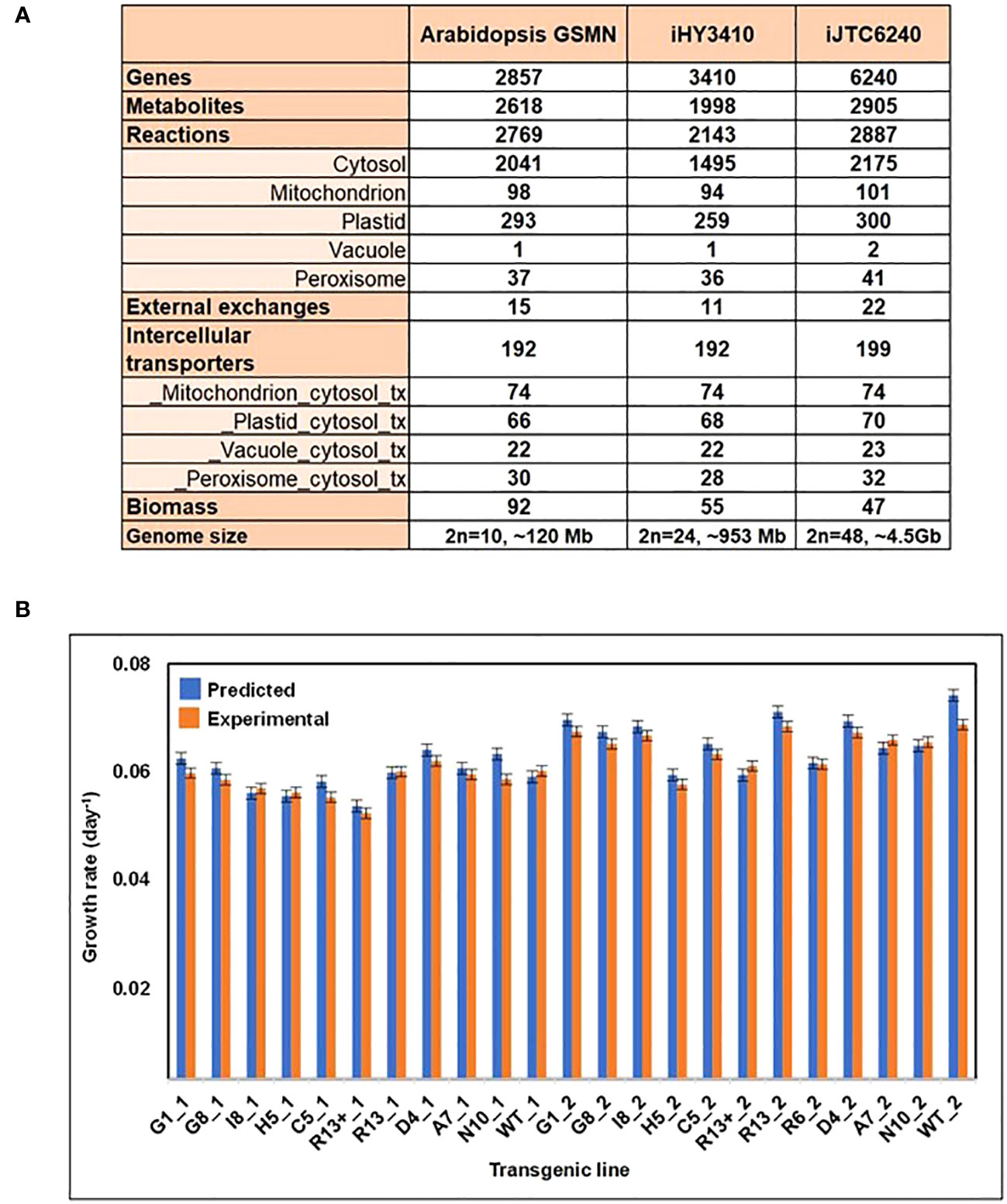
Figure 1 Overview and phenotype predictions of iJTC6240. (A) Comparison of model characteristics and reaction compartmentalization assignments between the Arabidopsis GSMN, iHY3410 and iJTC6240. (B) Comparison of tobacco transgenic line-specific phenotype predictions and experimentally observed growth rates. ‘_1’ and ‘_2’ as suffixes to the transgenic line names indicate that was obtained in 2012 and 2013 respectively. The experimental data was obtained from Jiang et al. (2018).
The model properties of iJTC6240 were compared with those of two plant GEMs, iHY3410 (Yuan et al., 2016) and Arabidopsis GEM (Cheung et al., 2013). Figure 1A summarizes the overall comparison of model characteristics and compartmental reactions between models. iJTC6240 collected more reactions, metabolites, and especially more genes than the other two models, which may be due to the larger genome of tobacco. More metabolic reactions are distributed in cytosol, mitochondria, plastid, vacuole and peroxisome compartments with various proportions. In addition to a single vacuole reaction found in both of iHY3410 and Arabidopsis GEM, also the same one, iJTC6240 collected one more vacuole reaction, whose subcellular location was validated in the literature. The reaction ‘RXN-10938_v’ describes the hydrolysis of phosphatidylinositol 3,5-bisphosphate (PtdIns(3,5)P2), which is essential for maintaining vacuolar morphology and function (Bonangelino et al., 2002). Consistently, the phosphoinositide phosphatase that catalyzes this reaction was reported to be localized to the vacuolar membrane (Rudge et al., 2004). A great number of metabolites and reactions unique to our tobacco model, that have no counterpart in Arabidopsis or tomato, including nornicotine and methylnicotinamide involved in nicotine metabolism. In tobacco, nicotine accounts for 95% of the total alkaloid content; tomatine, solanine and esculeoside, which were known as steroidal glycoalkaloids (SGAs) that are specialized metabolites produced by Solanaceae species.
After the GEM reconstruction, we performed several tests to validate its predictive capabilities. Firstly, the model was evaluated by simulating known metabolic functions, including absorption of PSI and PSII photons during photosynthesis, as well as the biosynthesis and degradation of secondary metabolites, such as alkaloids and terpenoids. Secondly, the ability of iJTC6240 to predict tobacco growth rates was tested. We utilized experimental photosynthetic capacity (Jiang et al., 2018) to constrain the model, and the model was validated by fairly correlation between the predicted and experimental growth rates (Figure 1B).
In-vitro tobacco showed slower growth and less biomass than soil-grown plants
To reflect the general impact of in-vitro cultivations on tobacco, we selected the most common conditions to cultivate tobacco plants in vitro and in soil (as described in the methods section). The seeds germinated approximately 1 week after sowing (WAS), and the germinated seedlings were transplanted into soil or in vitro. The in-vitro plants grew much slower than that in soil, but the true leaf number of both tobacco plants was almost the same during all stages of development (Figures 2A, B). We compared a number of growth parameters between in-vitro and soil-grown tobacco plants at 10 WAS, at which time the top of the in-vitro plants reached the cap of the culture vessel. In-vitro tobacco was much smaller and its fresh weight was approximately one fifth of the soil-grown fresh weight. Moreover, the in-vitro dry weight was only one twelfth of the soil-grown dry weight, indicating that the water content of the in-vitro plants was much higher than that of soil-grown plants. The numbers of true leaves were similar between in-vitro and soil-grown tobacco plants at 10 WAS, and none of the plants developed flower buds during the study period (Figure 2B).
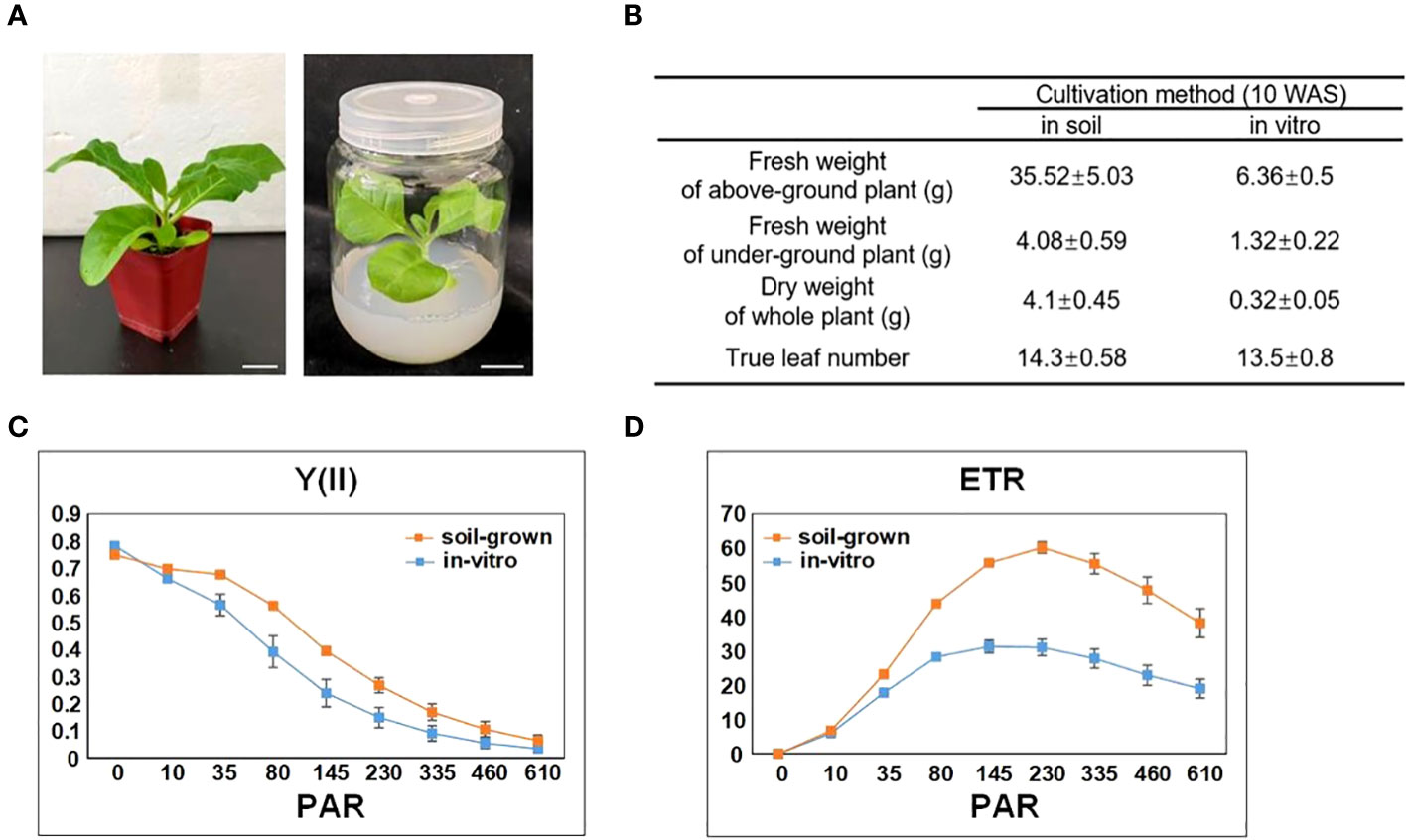
Figure 2 Impact of in vitro cultivation on tobacco growth and photosynthesis. (A) Tobacco plants cultivated in soil and in vitro (with 3% sucrose in the medium), both with 7 true leaves (approximately 5 WAS). Scale bars: 2 cm. (B) The growth difference between soil-grown and in-vitro cultivated tobacco plants. (C) The effective quantum yield of photochemical energy conversion in PSII (Y(II)). (D) The relative electron transport rate (ETR) of PSII. PAR denotes photosynthetically active radiation. Data are the means ± SE (n = 6). The orange color indicates the photosynthetic parameters of soil-grown leaves, while the blue color indicates the photosynthetic parameters of in-vitro leaves.
Suppressed photosynthesis in in-vitro tobacco plants
We measured the leaf chlorophyll fluorescence parameters to explore the photosynthetic system of in-vitro and soil-grown plants. The Fv/Fm, ratio which represents maximum quantum yield of PS II photochemistry, also indicates the stress level of the plants. According to this measure, the in-vitro and soil-grown plants had a similar photosynthetic capacity (0.78 ± 0.01 in soil-grown vs. 0.75 ± 0.005 in in-vitro plants; n=6). To further assess the differences in the photosynthetic efficiency, the effective quantum yield of photochemical energy conversion in PS II (Y(II)) and relative electron transport rate (ETR) were calculated for different light intensities (PAR). Figure 2C showed the Y (II) curve was generally lower in in-vitro tobacco plants than that in in-soi ones, and gradually decreased to nearly 0 µmol at 610 µmol photons m-2s-1 following light intensity increased. The ETR curve under in-vitro condition was also lower than that under soil-grown condition (Figure 2D), and it gradually increased from 0 to nearly 60.22 µmol in soil-grown and 31.04 µmol in in-vitro at 230 µmol photons m-2s-1 (PAR), once reached this level, ETR decreased. Collectively, these results indicated the suppressed photosynthetic capacity under in-vitro condition.
Metabolic variations between in-vitro and soil-grown tobacco plants revealed by metabolome, transcriptome and amino acid data
The above results showed that in-vitro cultivation inhibited the growth of tobacco compared to soil-grown cultivation. To study the impact of in-vitro cultivation on tobacco metabolism and globally detect the metabolic variations between in-vitro and soil-grown tobacco plants, we performed metabolome and transcriptome analyses for 10-WAS in-vitro and soil-grown tobacco plants.
We generated transcriptome sequencing data for in-vitro tobacco and soil-grown tobacco plants, each with three biological replicates. After filtering out low-quality reads from the raw data, a total of 37.77 GB clean data was generated, and 5.64−6.74 GB for each sample was obtained. The GC content was ranging from 43.20% to 43.50%, and the percentages of Q30 bases were over 92.75%. The mapped efficiency of different samples with reference genome ranged from 94.64% to 95.12% (Table S1). The assembly resulted in 88,878 unigenes, of which 13,890 differentially expressed genes (DEGs) were identified between in-vitro and soil-grown plants (8,519 downregulated, 5,371 upregulated) were identified from transcriptomic data, and their GO functional annotation is shown in Supplementary Figure S1.
To detect the difference in metabolite abundance and type between in-vitro and soil-grown tobacco plants, a widely targeted metabolomics analysis was conducted in triplicates. From the overlapping map of total ion current (TIC) of the quality control (QC) sample, it could be observed that there was a high overlap in the curve of the total ion flow, and the retention time and peak intensity remained consistent. This suggested that the signal stability for the same sample was good when the mass spectrometry was detected at different times (Supplementary Figure S2). In this study, a total of 680 metabolites were identified across all samples. All metabolites can be categorized into 12 classes, including lipids (131), phenolic acids (91), flavonoids (89), alkaloids (77), amino acids and derivatives (69), organic acids (47), nucleotides and derivatives (40), lignans and coumarins (25), terpenoids (22), tannins (5), quinones (3) and other metabolites (81). UPLC-ESI-MS/MS-based metabolomic and OPLS-DA (orthogonal partial least squares-discriminant) analyses (Supplementary Figure S3) identified 390 differentially accumulated metabolites (DAMs), 207 downregulated and 183 upregulated.
Cultivation-condition-specific metabolites
Among the 183 upregulated DAMs, 25.7% exhibited abundance in in-vitro tobacco plants but were not detected in soil-grown ones. Some of these in-vitro-specific metabolites were environmental stress markers or stress response compounds, such as malate and tomatidine (Table S2). The dicarboxylic acid malate has a multitude of functions in plant physiology and metabolism, and it was characterized as a major constituents of soluble carbon fraction involved in osmotic adjustment (Finkemeier and Sweetlove, 2009). The high accumulation of malate may help to cope with the ambient osmotic potential of in-vitro culture media. It is reported that the increased levels of tomatidine resembled toxic phenotypes, such as growth retardation (Itkin et al., 2011).
Among 207 downregulated DAMs, 16.4% exhibited abundance only in soil-grown tobacco plants, some of which may reflect the specific culture condition and metabolic responses, such as capsianosides and planteose. Capsianosides were pinpointed as metabolites related to insect resistance (Macel et al., 2019), reminiscent of the flying insects in the climate chamber. Planteose is regarded as a temporary sugar storage compound in plants (Klages et al., 2004). The condition-specific accumulation of planteose may indicate that soil-grown tobacco plants could fix excess carbohydrates through photosynthesis, while in-vitro tobacco plants could not, implying a suppression of photosynthesis in the in-vitro tobacco plants.
Cultivation-condition-specific enriched metabolic pathways and metabolite classes
To further identify cultivation-condition-specific metabolic pathways, KEGG pathway enrichment analysis of DEGs was performed (Figure 3). Many amino acid metabolism related pathways were upregulated, supported by enriched upregulated DEGs related to aminoacyl-tRNA synthesis and amino acids biosynthesis (Figure 3A). The metabolic pathways of alpha-linolenic acid, glycerophospholipid, glycerolipid and fatty acid, which are involved in lipid metabolism, were enriched among the downregulated DEGs (Figure 3B). The primary carbon metabolism was generally downregulated in in-vitro tobacco plants compared to soil-grown ones, since the photosynthesis, glycolysis/gluconeogenesis, pentose phosphate pathway (PPP) and TCA cycle, as well as its closely related pathways, such as the metabolism of starch, sucrose, fructose, mannose, pentose, galactose and pyruvate were all downregulated. At the same time, many downregulated plant secondary metabolic pathways were enriched under in-vitro condition, including the phosphatidylinositol signaling system, flavonoid biosynthesis, and phenylpropanoid biosynthesis. Collectively, enrichment analysis of DEGs suggested that in in-vitro tobacco plants amino acid metabolism was up regulated, while lipid metabolism was attenuated, and the growth inhibition was likely due to the lower activity of primary carbon metabolism and related pathways. In addition, the secondary metabolism of in-vitro tobacco plants was also weakened compared to soil-grown ones.
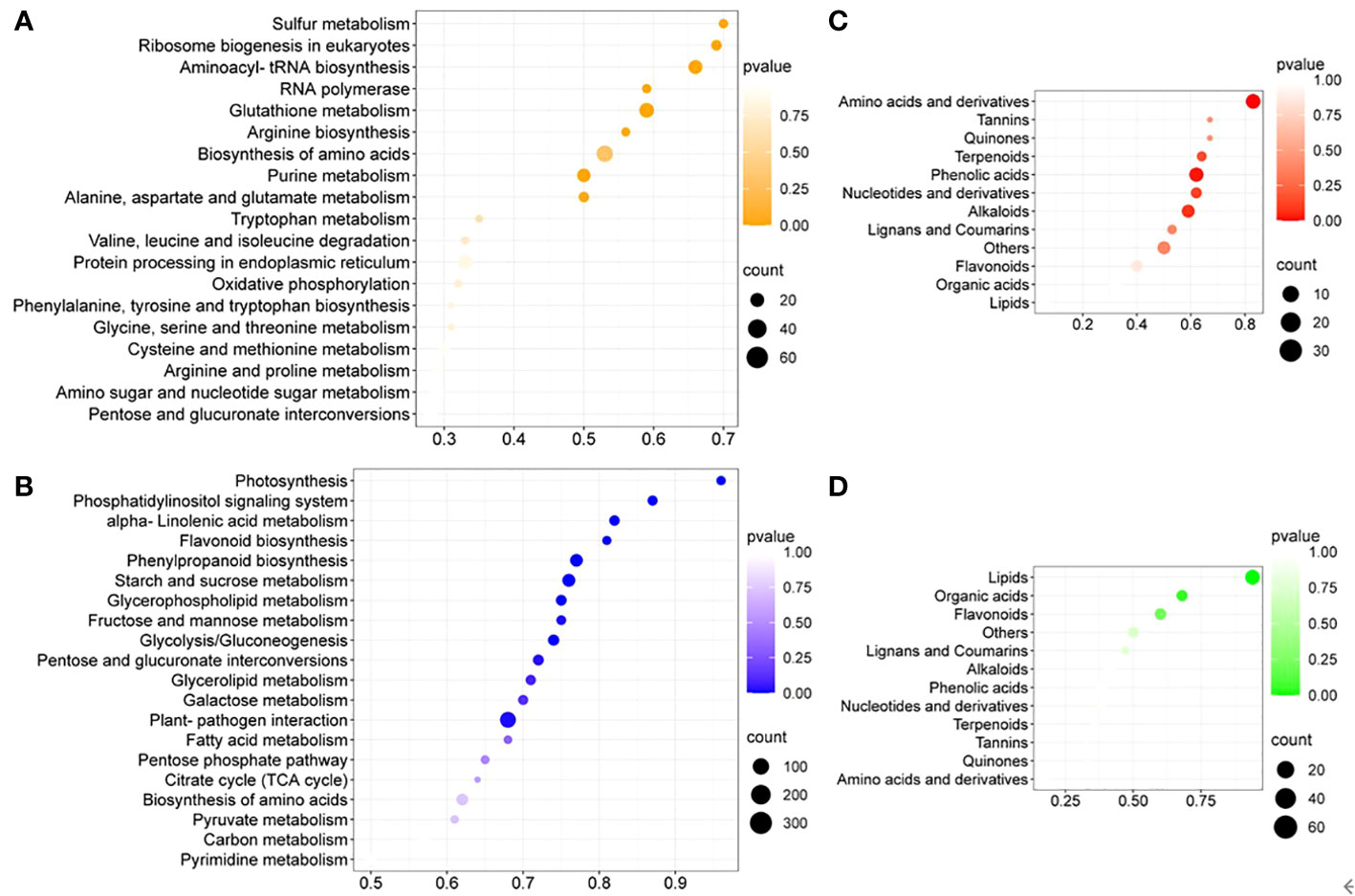
Figure 3 The enrichment analysis of DEGs and DAMs between different culture conditions. (A) The top twenty KEGG pathways enriched among upregulated DEGs, (B) the top twenty KEGG pathways enriched among downregulated DEGs; (C) The enrichment analysis of UpSet DAMs, (D) the enrichment analysis of DownSet DAMs.
DAMs were utilized for the enrichment analysis based on the metabolic classification. The upregulated amino acids and derivatives were enriched among the DAMs with the greatest enrichment degree (Figure 3C). In addition, lipids were enriched among the DownSet of DAMs with the greatest enrichment degree (Figure 3D).
The content of amino acids increases significantly under in-vitro tobacco plants
Compared to soil-grown tobacco, a majority of primary metabolic pathways were downregulated in in-vitro tobacco plants, but the synthesis and accumulation of amino acids was upregulated indicted by analysis of metabolome and transcriptome, which were relative quantification analyses. A total of 5 amino acids and derivatives exhibited significant abundance in in-vitro plants but were not detected in soil-grown tobacco, such as homocysteine. Homocysteine is a non-proteinogenic amino acid and is considered to response to environmental stress (Watanabe et al., 2021). Only one metabolite in the term of amino acid and derivatives, L-theanine, exhibited significant abundance in soil-grown tobacco while it was not detected in in-vitro plants. It was reported that theanine content significantly increased when attacked by Ectropis grisescens (Liao et al., 2019), indicating that the soil-grown plants may have been exposed to insects. Relative quantification results based on metabolome showed the overall levels of the 20 common proteinogenic amino acids were higher in in-vitro tobacco plants (Table S2).
To further quantitatively compare the common amino acids in plants from different culture conditions, automatic amino acid analyzer was utilized to determine the absolute concentrations of free common amino acids. The overall level of free amino acids under in-vitro condition was higher than soil-grown condition, which revealed approximately 2-fold higher amino acid levels in the in-vitro plants (Figure 4). The top 5 most highly accumulated free amino acids, accounting for over 80% of the total content of common amino acids, were distributed in different metabolic branches. For example, Gln and Pro are derived from α-ketoglutarate, Asn is derived from oxaloacetate, Ser is derived from 3-phospho-glycerate and Val is derived from pyruvate. Additionally, four of the top 5 most highly accumulated amino acids were more abundant in in-vitro than in soil-grown plants, including Gln (4.92-fold), Asn (1.92-fold), Ser (1.21-fold), and Val (1.19-fold), but not Pro (0.82-fold). Among these, Ser, Val and Pro were also detected in the metabolomic data, and their metabolic peak areas were consistent with the measured absolute concentrations. Notably, the concentration of Gln, which was not detected in the metabolomic data, was much higher than that of the other amino acids and was primarily responsible for the significant upregulation of amino acids in in-vitro tobacco plants.
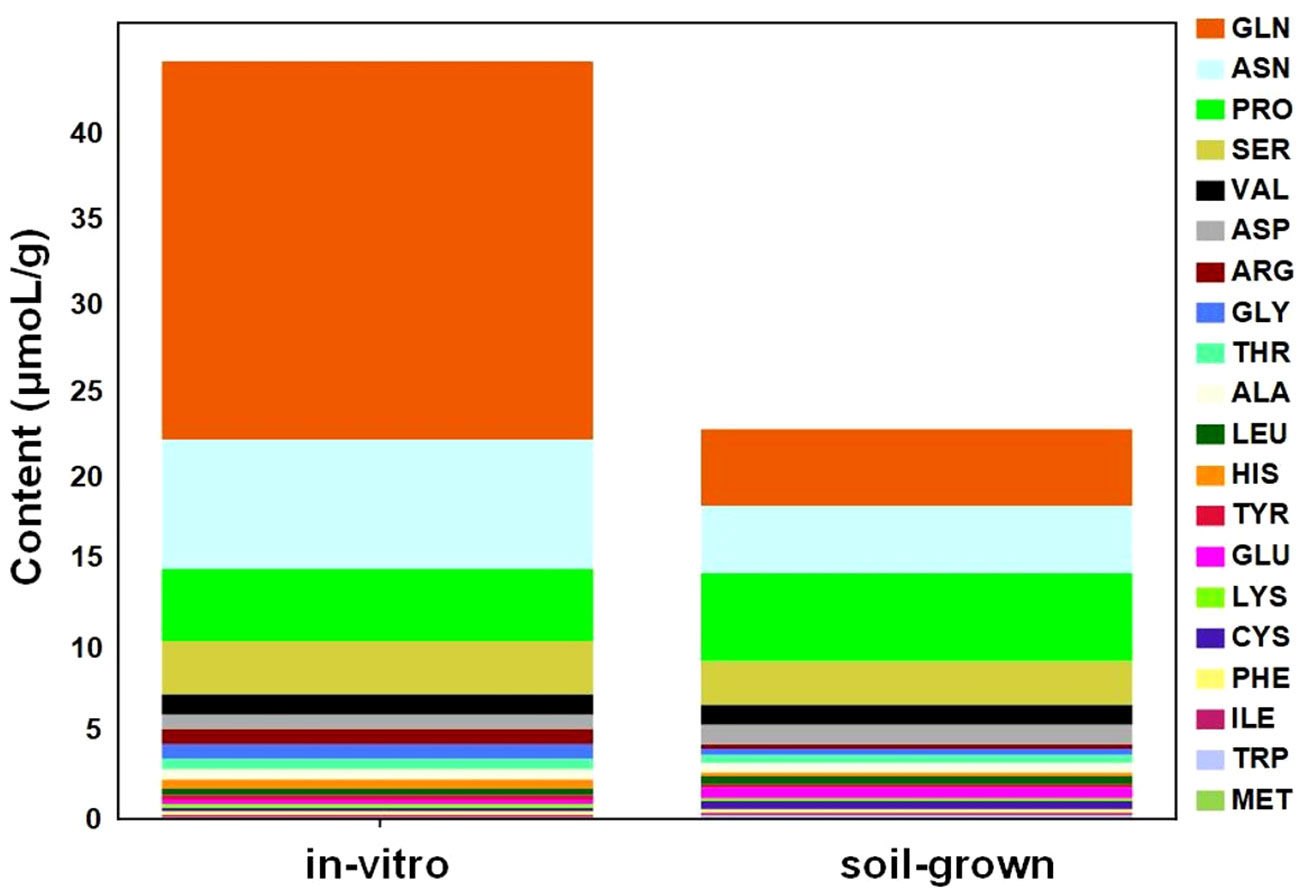
Figure 4 Changes in the absolute concentrations of common amino acids between in-vitro and soil-grown conditions.
In order to further exclude the influence of developmental status (such as flowering transition) on plant metabolism, 7-WAS tobacco metabolic variations between two cultivations were also tested except for 10-WAS tobacco. We measured the concentrations of amino acid, one of the most characteristic metabolic variations, for 7-WAS tobacco (Supplementary Figure S4), and amino acid concentrations increased significantly under in-vitro condition, the pattern was consistent with the results of 10-WAS plants. These results indicated the metabolic variations we detected were mainly due to the different cultivation systems.
Metabolic network signatures of in-vitro tobacco plants unraveled by omics-based modeling
To further explore how different culture conditions influence growth, we examined the internal flux distributions under soil-grown and in-vitro conditions. The transcriptome data and measured amino acid concentrations under in-vitro and soil-grown conditions were used to calculate constraints to GEM, leading to context specific models. The flux balance analysis results showed that the growth rate of the tobacco plants was lower under in-vitro than under soil-grown. Notably, multiple crucial metabolic pathways were downregulated under in-vitro condition, including photosynthesis, the Calvin cycle, glycolysis, and tricarboxylic acid cycle (Figure 5). The slower growth and less biomass under in-vitro condition may be related to the overall downregulation of primary carbon metabolism. By contrast, the cyclic electron flow (CEF) and the glutamine synthetase (GS)/glutamine-2-oxoglutarate aminotransferase (GOGAT, also known as glutamate synthase) cycle were upregulated under in-vitro condition, and the metabolomic data also showed that the concentration of Gln was higher than that under soil-grown. After normalized by the biomass rate, more ATP was produced by proton pumps (1.5-fold), while less NADH/NADPH (by 12% and 0.18%, respectively) was produced under in-vitro condition.
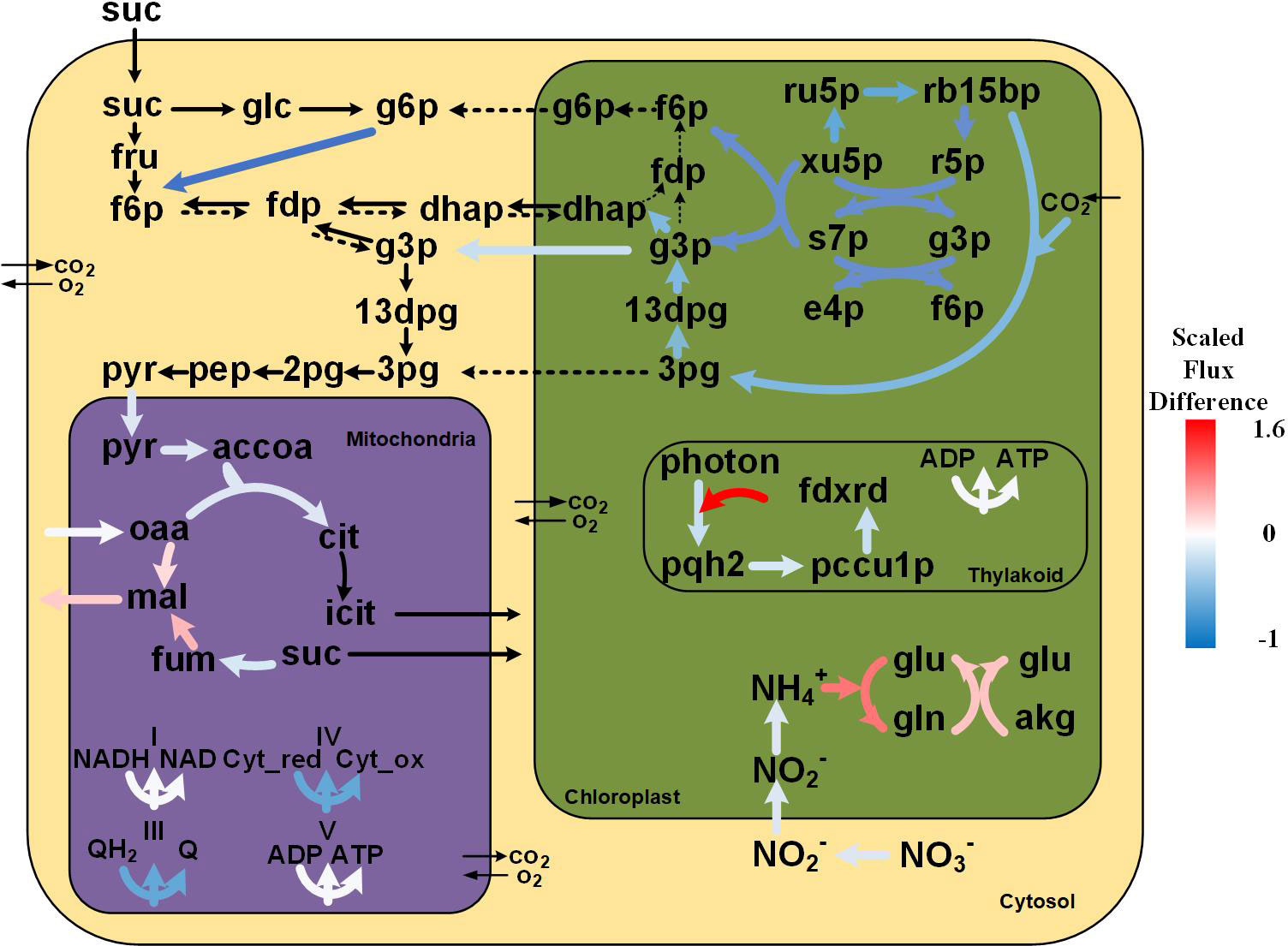
Figure 5 Flux distribution of the primary metabolism of tobacco under soil-grown and in-vitro conditions. The black bold arrows indicate reactions carrying non-zero fluxes in in-vitro plants while having zero fluxes in soil-grown plants; the black dotted arrows indicate reactions carrying non-zero fluxes in soil-grown plants while having zero fluxes in-vitro based on FBA solutions. The differences in the flux values under the two conditions were calculated using the log10 scale fold changes of in-vitro/soil-grown values as a reference. Metabolite abbreviations are as follows: suc, sucrose; glc, glucose; g6p, glucose 6-phosphate; fru, fructose; f6p, fructose 6-phosphate; fdp, fructose 1,6-bisphosphate; dhap, dihydroxyacetone phosphate; g3p, glyceraldehyde 3-phosphate; 13dpg, 3-phospho-glyceroyl phosphate; 3pg, 3-phospho-glycerate; 2pg, glycerate 2-phosphate; pep, phosphoenolpyruvate; pyr, pyruvate; accoa, acetyl-coa; cit, citrate; icit, isocitrate; fum, fumarate; mal, malate; oaa, oxaloacetate; Cyt_red, Cytochromes c_reduced; Cyt_ox, Cytochromes c_oxidized; QH2, ubiquinol; Q, ubiquinone; ru5p, ribulose 5-phosphate; xu5p, xylulose 5-phosphate; s7p, sedoheptulose 7-phosphate; e4p, erythrose 4-phosphate; rb15bp, ribulose 1,5-bisphosphate; r5p, ribose 5-phosphate; pqh2, plastoquinol; pccu1p, plastocyanin reduced; fdxrd, ferredoxins reduced; gln, glutamine; akg, 2-oxoglutarate; glu, glutamate.
Photosynthesis
Photosynthetic organisms use various electron flow pathways to generate redox power from light energy in the form of NADPH. The linear electron flow (LEF) pathway, where the electrons flow from PSII to PSI through Cytochrome b6f complex and ferredoxin NADP+-oxidoreductase, is the most extensively used pathway (Allen, 2002). Additionally, plants also possess other alternative electron flow (AEF) pathways, such as CEF, which provides acclimatory plasticity for the photosynthetic machinery. In the CEF process, electrons are recycled to generate a proton gradient that drives the synthesis of ATP, which is essential for balancing the energy demand. Although the physiological roles of CEF pathways are not clear, they are suggested to function alongside LEF (Allen, 2003).
The simulations revealed that both the LEF and CEF pathways carried noticeable fluxes under soil-grown and in-vitro conditions, suggesting that the CEF pathway is possibly always operational. Interestingly, the predicted rate of photosynthesis under in-vitro condition was much more dependent on the CEF pathway than under soil-grown condition. In fact, the corresponding energy generated by the CEF pathway under in-vitro condition was 42.9-fold higher than in soil-grown plants. The CEF pathway under in-vitro condition, rather than LEF, was used increasingly to generate more energy, that may response to the lower reducing power demand in the Calvin cycle because of low CO2 uptake, as well as the need to scavenge excess reducing power, which is cytotoxic. Additionally, considerable ATP was needed in a multitude of metabolic pathways, including Gln biosynthesis by glutamine synthetase and sucrose import via transmembrane transporters. Generally speaking, the CEF pathway carried the higher fluxes, highlighting its flexibility and also suggesting that the CEF pathway may contribute to the adaptation of energy demand under in-vitro condition.
Calvin cycle
The Calvin cycle exhibited appreciable lower fluxes (by 43%) in in-vitro plants compared to soil-grown plants, which was attibuted to the lower CO2 uptake. In both simulations, the Calvin cycle was driven by the supply of CO2 from cytosol to plastid and was subsequently withdrawn in the form of 3-phosphoglycerate (3PG), that can be transported to cytosol. The 3PG in the cytosol was utilized for energy production through cytosolic glycolysis and the TCA cycle, in agreement with previous reports (Lakshmanan et al., 2013). In this regard, the flux analysis results indicated reduced fluxes (by 76%) under in-vitro condition, whereby the 3PG in the plastid was utilized to produce 1,3-bisphosphoglycerate (13DPG) instead of energy generation, with a decreased flux (by 45%). 13DPG was converted into glyceraldehyde 3-phosphate (G3P) that participated in PPP and gluconeogenesis. Under in-vitro condition, the plastidic dihydroxyacetone phosphate (DHAP) produced from G3P by fructose-bisphosphate aldolase was transported to cytosol to synthesize Fru-1,6-bisP, while under soil-grown condition it was utilized to synthesize plastidic G6P, which was transported to cytosol to enter Embden-Meyerhof-Parnas (EMP) pathway.
TCA cycle and oxidative phosphorylation
The model simulation revealed an incomplete TCA cycle under both conditions. As there are multiple cytosolic isoforms of the enzymes that catalyze the mitochondrial TCA reactions, it stands to reason that some mitochondrial isoforms were not used in our model prediction (Sweetlove et al., 2010), as for example succinyl-CoA synthetase operated in the form of a cytosolic bypass in the prediction. The operation of an incomplete TCA cycle in the light by our tobacco model is supported by a study in French bean leaves (Phaseolus vulgaris), which indicated that the TCA cycle was almost completely inhibited under light (Tcherkez et al., 2005). Similarly, Poolman et al. (2013) reported that the TCA cycle did not function as a conventional cycle in rice under high light (Poolman et al., 2013).
In the simulation of soil-grown tobacco, no flux was observed from citrate to succinate, while under the in-vitro condition, carbon flux enters the cycle at two different points: (1) acetyl-CoA from pyruvate and (2) succinate from cytosol. The TCA cycle of in-vitro tobacco plants was impaired (flux reduction by 77%), with an increase of malate production from fumarate (2.9-fold) and from oxaloacetate (by 68%). The malate highly produced by TCA cycle in mitochondria was then transported to plastids, where it was converted into oxaloacetate by malate dehydrogenase (NAD) to provide reducing power for the GS/GOGAT cycle, while the oxaloacetate was utilized to form Asp.
The fluxes within mitochondrial respiration were relative mild reduced under in-vitro condition (by 92%) with a 90% flux of oxidative NADH as the first step of the respiratory chain. Additionally, succinate dehydrogenase (SDH, also known as Complex II) has a dual function as a component of the TCA cycle and the electron transport chain (ETC). The flux through SDH was comparable with its TCA cycle flux (76.7%).
Sucrose metabolism
Since sucrose (Suc) was imported from the surrounding medium under in-vitro condition, noticeable levels of ATP were consumed for this active transmembrane transport process. The sucrose invertase (INV) was predicted to degrade Suc into fructose and glucose, while that is not an energetically efficient way to break down Suc when compared to the cleavage of Suc by sucrose synthase (SUS) (Magneschi and Perata, 2009). Subsequently, fructose and glucose are converted into G6P, which flows into the EMP pathway, while under soil-grown condition, G6P that drives EMP is supplied by its plastidic isoform and is derived from F6P, which synthesizes via the pentose phosphate pathway and the hydrolysis of fructose-1,6-bisphosphate.
N metabolism
In our model, we considered that tobacco can take up NH4+ and/or NO3- as nitrogen sources. After uptake, nitrate is firstly reduced into nitrite by nitrate reductase (NR) in cytoplasm, after which nitrite is transported into plastid and reduced to ammonium by plastidic nitrite reductase (NiR). In this regard, the flux analysis results indicated that this route exhibited reduced fluxes (by 75%) under in-vitro condition, and the resulting lower consumption of reducing power may be related to its lower supply from photosynthetic light reactions, and a greater proportion were shifted toward energy generation.
Interestingly, ammonium assimilation, by which inorganic N is converted into organic N, was higher under in-vitro condition. The ammonium assimilation in plastid is accomplished by the GS/GOGAT cycle, where GS catalyzes glutamine (Gln) synthesis by incorporating a molecule of ammonium into glutamate (Glu) in an ATP-dependent manner, while GOGAT produces two molecules of Glu by transferring the amide from Gln to 2-oxoglutarate (2-OG). Plants possess two isoforms of GOGAT that differ in their electron donor for catalysis, NADH-GOGAT (EC 1.4.1.1) and ferredoxin (Fd)-GOGAT (EC 1.4.7.1), both of which are located in the plastids (Lea and Blackwell, 1993). Our model predicted that tobacco plants utilize the NADH-GOGAT to generate Glu. The resulting constraint-based flux analysis revealed that the flux through NADH-GOGAT (EC 1.4.1.1) was higher under in-vitro condition than that in soil-grown plants (2.14-fold). In higher plants, there are two types of GS with distinct subcellular localization, cytosolic GS1 and plastidic GS2. Our model predicted that glutamine was only produced by plastidic GS2 in both cases, and the flux was higher in-vitro than in soil-grown plants (4.91-fold). Collectively, it can therefore be seen that the higher activity of the GS/GOGAT cycle under in-vitro condition results in increased Gln synthesis at the expense of Glu, since the flux through GS was higher than through GOGAT. These findings may offer a reasonable explanation for the higher Gln but lower Glu content under in-vitro condition compared to soil-grown plants.
The composition of the culture medium is crucial for in-vitro cultivation. MS medium was originally designed for tobacco callus and is widely used for many plant species nowadays. MS medium contains macronutrients that include high levels of nitrate and organic additives, such as sugar, and vitamins. Sugar provides metabolic energy and carbon skeletons for the biosynthesis of the organic compounds required for cell growth and also act as an osmotic component in the culture medium. Stancato and Tucci (2010) emphasized that the accumulation of dry matter in-vitro plants is positively correlated with the sucrose content in the culture medium. However, high sucrose concentration added to the culture medium can cause starch and sucrose accumulation in the leaves, which can reduce the photosynthetic activity (Schmildt et al., 2015). In our study, the photosynthetic activity of in-vitro tobacco was suppressed, which may be attributed to an abundance supplement of sucrose [3% (w/v)] in the culture medium. The increasing nitrogen supplement could lead to an increase in the total nitrogen content in tissues. Sarimento et al. (1992) revealed that the application of increasing quantities of nitrogen enhances the accumulation of the free amino acids in grapevine explants. In this study, the content of some amino acids also increased significantly in in-vitro tobacco. Furthermore, in silico simulations showed in-vitro tobacco had a higher activity of the GS/GOGAT cycle fixing the substantial amounts of ammonium at the expense of Glu to produce Gln, which may be attributed to an abundance supplement of ammonium salts in the culture medium.
Compared to soil-grown tobacco, although there was additional carbon (sucrose) uptake, we noticed diminished carbon fixation in the Calvin cycle in our in silico investigation, that resulted in lower growth rate and less biomass under in-vitro tobacco. The Calvin cycle was subjected to energy and reducing power. Although ATP production was abundant, the generation of reducing power (NADH/NADPH) is markedly limited indicated by our systematical combined omics-based analysis. Here we gave three suggestions on how to improve the situation under in-vitro condition. Firstly, our model prediction showed a surprisingly high level of cyclic photophosphorylation, resulting in the reduction power being utilized for the generation of abundance energy. We proposed suppressing cyclic photophosphorylation and boosting non-cyclic photophosphorylation activity for this purpose. Additionally, our model survey also predicted increased flux from fumarate to malate but decreased subsequently in the TCA cycle. To improve the flux towards oxaloacetate and consequently the overall TCA cycle, we suggested that supplement the culture medium with appropriate pyruvate or organic acid (such as acetate, citrate) salts, which could potentially elevate the concentration of TCA metabolites intermediates and thereby drive the TCA cycle to generate reducing power. Finally, addition of glycerin into medium was supposed to help to alleviate the deficiency in reductive power.
To improve the suppressed photosynthesis in in-vitro tobacco than soil-grown tobacco, we proposed: Firstly, since we provided sufficient detail in iJTC6240 to define all individual electron transport reactions including photosystem II (PSII), photosystem I (PSI), and the cyclic/non-cyclic electron transfer, both photons utilized in PSII and PSI exhibited lower level of flux (by 74% and 67%, respectively) under in-vitro compared to soil-grown tobacco in our observations. We speculated that maybe an appropriately elevation of effective photons utilization that not inducing photoinhibition would confer improvements. Secondly, light quality serves as a pivotal factor affects various morphological processes in plants, it was reported that in blue light, photosynthesis was up-regulated (Lakshmanan et al., 2015). In that way, it was hypothesized that varying light wavelengths in chamber may modulate the suppressed photosynthetic processes in-vitro.
Materials and methods
Plant material, growth conditions and biomass determination
Tobacco (Nicotiana tabacum cv. Petit Havana) seeds were sterilized with 75% ethanol for 1 min, and then sterilized with 10% sodium hypochlorite for 20 min, rinsed with sterile water three times, and finally germinated on Murashige and Skoog (MS) agar plates containing 3% (w/v) sucrose in a climate chamber at temperature of 25°C with a light/dark cycle of 16 h/8 h and photon fluence of 186 μmol m −2 s −1. The germinated seedling was transplanted into pots containing soil/vermiculite mixture (1:1) or into 500 mL glass culture vessels (covered with gas-permeable caps) containing solidified MS medium (100 mL per vessel) with 3% sucrose. The fresh weight of the tobacco aboveground part was measured using an electronic balance. The dry weight was recorded after drying the samples in an oven at 65°C to constant weight.
Nucleic acids extraction
Genomic DNA was isolated using a Super Plant Genomic DNA Kit (Tiangen Biotech, Beijing). Total RNA was extracted from 10-WAS plants using an RNAprep Pure Plant Plus Kit (Tiangen Biotech, Beijing). DNA and RNA degradation and contamination were monitored on a 1% (w/v) agarose gel. RNA purity and integrity were assessed using the NanoPhotometer® spectrophotometer (IMPLEN, CA, USA) and the RNA Nano 6000 Assay Kit of the Agilent Bioanalyzer 2100 system (Agilent Technologies, CA, USA), respectively. RNA concentration was measured using the Qubit® RNA Assay Kit in the Qubit®2.0 Flurometer (Life Technologies, CA, USA).
Transcriptome analysis
The aboveground parts of three independent plants were collected and pooled as one transcriptomic sample, each sample with three biological replicates. Transcriptome analyses were performed for in-vitro and soil-grown tobacco plants. Qualified RNA from each samples were shipped on dry ice to the Biomarker Biotechnology Corporation (Beijing, China) for cDNA library construction and RNA sequencing. The library was prepared using the VAHTS® Universal V8 RNA-seq Library Prep Kit for Illumina (Vazyme Biotech, Nanjing, China). Subsequently, the cDNA libraries were paired-end sequenced on an Illumina NovaSeq 6000 platform (Illumina, San Diego, CA, USA). Clean reads were obtained by removing reads containing adapters or ploy-N and low-quality reads from the original data. At the same time, the percentage of Q30 bases and GC-content of the clean data were calculated. All downstream analyses were based on clean data of high quality. After performing quality control, we obtained clean reads which were then mapped to the tobacco reference genome Ntab_TN90 (Sierro et al., 2014) using HISAT2 (Kim et al., 2015b). The mapped reads were subsequently assembled using StringTie (Pertea et al., 2015). The assembled genes were aligned against several databases, including Nr, Swiss-Prot, KEGG, COG, GO, KOG, eggNOG and Pfam, with BLASTX at an e-value cutoff of 1e–5 (Altschul et al., 1997). Gene expression levels were calculated based on the read counts and normalized by the fragments per kilobase of transcript per million fragments mapped (FPKM) method (Florea et al., 2013). DEGs were identified using the DESeq2 package (Love et al., 2014) using a false discovery rate (FDR)<0.05 and fold-change (FC) ≥2 or FC ≤ 0.5 as the filtering criteria.
Metabolome analysis
Metabolite profiling was carried out using a widely targeted metabolomic method by Metware Biotechnology (Wuhan, China) for the same samples as transcriptome. Briefly, the freeze-dried plant samples were crushed using a mixer mill (MM 400, Retsch) and extracted with 70% methanol extraction solution. After centrifugation, the supernatant was filtrated and then analyzed using a UPLC-ESI-MS/MS system in conjunction with UPLC (Shim-pack UFLC Shimadzu CBM30A system) and MS (Applied Biosystems 4500 Q TRAP). The analytical parameters were set as the same as Shi et al., 2021. For metabolomics data, the primary results were identified by comparing the fragmentation patterns, accurate m/z value and retention time to the standards in the self-compiled database (MetWare, Wuhan, China) and the public databases (Metlin and Massbank). The characteristic ions of each metabolite were obtained by triple four-stage rod screening, the signal intensity of the characteristic ions was obtained in the detector, and MultiQuant software was used to integrate and correct the chromatographic peaks. All integration data for the chromatographic peak areas were exported and saved. To detect the repeatability of the samples, QC samples (mixed sample extracts) were inserted in every 10 test samples during the analysis. The accuracy and reproducibility of metabolite detection could be determined by using the overlapping display analysis of mass spectrometry TIC of different QC samples. Combined FC of metabolite difference and the variable importance in projection (VIP) value of the orthogonal partial least squares discriminant analysis (OPLS-DA) were used to screen DAMs. The FC ≥ 2 or FC ≤ 0.5, and VIP ≥ 1 were considered as the standard of screening. KEGG pathway database was used to annotate the DAMs.
Extraction and quantification of free amino acids
An automatic amino acid analyzer (Hitachi L-8900, Tokyo, Japan) was used for the determination of free amino acids. The samples were diluted with 10 mL of boiling distilled water and incubated in a water bath at 95°C for 10 min. The extraction was repeated three times and the supernatant was obtained after centrifugation. After adding 10% sulfosalicylic acid, the mixture was centrifuged at 13400 g for 10 min, then dissolved in 0.02 M HCl, and passed through a 0.22-µm pore-size filter membrane into a brown sample bottle for further testing. An autoanalyzer based on the ninhydrin method was used to quantify the free amino acids according to the method described by Ye et al. (2022) (Ye et al., 2022). The amino acid content in each sample was calculated by comparing the peak area of the detected amino acid with that of the standard. All determinations were carried out in triplicate.
Chlorophyll fluorescence analysis
Chlorophyll fluorescence parameters were measured at room temperature using a pulse-amplitude-modulated (PAM) fluorometer (Imaging-PAM M-series, Walz, Germany). The expanded leaves were dark-adapted for 20 min before measurement. Six different areas of interest (AOI) were randomly selected on each leaf. The maximal quantum yield of photosystem II (Fv/Fm) was calculated as (Fm – Fo)/Fm, where Fm is the maximum chlorophyll fluorescence, measured immediately after a saturating light pulse, while Fo, the steady-state chlorophyll fluorescence, was measured after dark acclimation before a saturating light pulse. The effective PS II quantum yield of the illuminated samples was calculated using the formula Y(II) = (Fm’-F)/Fm’. The trend of Y(II) and the chloroplast electron transport rate (ETR) parameter was determined on the basis of a rapid light curve (RLC) at PAR of 0, 10, 35, 80, 145, 230, 335, 460 and 610 µmol photons m−2 s−1.
Construction and curation of the tobacco genome-scale metabolic model
Construction of a draft model
An initial reaction set for tobacco (Nicotiana tabacum) metabolism was obtained from Ntabacum_Tn90Cyc and KEGG (Kanehisa and Goto, 2000). Some spontaneous as well as non-gene-associated reactions, including metabolite transport, were also incorporated into the model. A total of 2,116 reactions were extracted from these databases, along with genes, pathways, and empirical formulas of most metabolites as our initial raw data set. To model the inputs and outputs of the metabolic system, 22 extracellular exchange reactions and 47 biomass drain reactions were respectively defined using ‘_Cyto_tx’ and ‘_biomass’ as the subscripts. Extracellular exchange reactions include the import of various energy sources (Glc, Suc, starch, and photons) and mineral nutrients (e.g. NO3-, NH4+, SO42-, Pi, Ca, Fe, Mg, K) as inputs along with the exchange of CO2, oxygen, and water with the environment.
Subcellular compartmentalization
The inclusion of gene–protein-reaction (GPR) associations was utilized to localize the individual reactions in the draft model to appropriate subcellular compartments by querying tobacco gene sequences against UniProt database. If it was not available in UniProt, the compartmental information from the GEM (Yuan et al., 2016) of a phylogenetically closely related species, tomato, was used to predict putative cellular compartment for the same reaction. If none of the case above, the WoLF PSORT (Horton et al., 2007) localization prediction software was applied to predict the reaction compartmentalization. Five subcellular compartments, namely cytosol (_c), mitochondria (_m), plastid (_p), peroxisome (_x), and vacuole (_v), are included in the tobacco model, whereby respective suffixes were used at the end of the reaction and metabolite identifiers to distinguish one compartment from another. The cytosol is the default compartment, and any metabolic exchanges between compartments went through the cytosol using intracellular exchange reactions between the cytosol and other compartments denoted with the subscripts _pc, _mc, _xc, and _vc for plastidic, mitochondrial, peroxisomal, and vacuolar exchanges, respectively. The information on intracellular transporters and the energy costs associated with transport was obtained from a published Arabidopsis genome-scale metabolic model that has undergone extensive curation (Cheung et al., 2013).
Model curation
In order to obtain accurate predictions of metabolic fluxes, genome-scale metabolic models should be curated. Firstly, we revised the directionality and reversibility of reactions based on an Arabidopsis (Arabidopsis thaliana) genome-scale metabolic model (Cheung et al., 2013) and the defined reversibility of the reactions (Ma and Zeng, 2003). After that, errors of infinite reducing power/energy production were excluded. The protons pumped out from electron transfer reactions of respiratory chains were compartmentalized to the mitochondrial membrane and the proper P/O ratio was guaranteed (the mitochondrial NADH and succinate ratios were 1.875 and 1.125 respectively). Finally, gaps (missing reactions) were identified in biomass components and the biosynthesis pathways of 20 common products (including pyruvate, oxaloacetate and glycerone phosphate) and were filled according to the methods of Luo et al. (2022) (Luo et al., 2022). Generally, the weight-added pFBA gap-filling algorithm was used to introduce the minimal number of reactions from MetaCyc into the model. Each biomass component or common product synthesis pathway was manually curated to make sure its production was less than its theoretical maximum yield, which was calculated according to the ratio of the reduction degrees of substrate to product. Finally, the molar mass of biomass in the biomass production reaction should be 1 g/mmol, and deviations from this value will result in errors in the calculated specific growth rate. Using the BiomassMW algorithm (Chan et al., 2017), we examined the biomass equation of the model and corrected the coefficients of all components.
Model validation
The model was checked via constraint‐based flux balance analysis (FBA) (Orth et al., 2010) using the COBRA Toolbox (Heirendt et al., 2019). The ability of iJTC6240 to predict growth rates of transgenic tobacco lines with different photosynthetic capacity grown under field conditions (Jiang et al., 2018) was investigated. In each transgenic line condition, photon influxes were allowed from the photon input reaction with active photosynthesis and with all organic sources of energy set to zero. As most plants uptake nitrogen in the form of nitrate and ammonium, nitrogen input was set to be 50% nitrate and 50% ammonium for the simulation. The rate of CO2 fixed was constrained according to the photosynthetic capacity of the respective experimental transgenic lines with biomass maximization as the objective function. The linear programming was mathematically represented as follows:
where Sij is the stoichiometric coefficient of metabolite i in reaction j, n is the set of all reactions in the model, V is a vector of reaction flux, m is the transporter reaction of each biomass component, and t is the corresponding transporter flux of the biomass component.
Incorporation of transcriptomic and free amino acid measurement data into the metabolic model
The E‐Flux algorithm (Colijn et al., 2009) was used to contextualize the model by integrating the corresponding transcriptome data of in-vitro/soil-grown plants. E‐Flux utilizes gene expression data to set the upper and lower bounds of reactions using gene–protein–reaction (GPR) associations from the model. In detail, the transcriptome data were transformed by log2(FPKM+1) using the mean values of three duplicates. The mathematical operations utilized to calculate the numerical values were the sum of “OR” expressions. Finally, the GPR rule values were normalized, divided by the maximum value in each condition. The normalized values were used to establish the new lower and upper reaction bounds. Exchange reactions corresponding to each growth environment were set as −1/1. After that, the condition‐specific models were obtained with specific reaction bounds.
The free amino acid data was incorporated into the model as the biomass component. Under soil-grown condition, photon influxes were allowed from the photon input reaction with active photosynthesis and with all organic sources of energy set to zero. The in-vitro condition was modeled by allowing photon influx with active photosynthesis and sucrose as the carbon and energy input (Sucrose_Cyto_tx), with all other constraints the same as the soil-grown one. Constraint-based FBA was run with the objective function of biomass maximization.
Statistical analysis
For quantitative analysis, values were expressed as the means ± standard errors of three independent biological replicates. Statistical significance analysis was performed using a Student’s t-test in Microsoft Excel. A value of p< 0.05 was statistically significant.
Data availability statement
The original contributions presented in the study are publicly available. This data can be found here: https://www.ncbi.nlm.nih.gov/bioproject, PRJNA1014250; https://www.ebi.ac.uk/metabolights, MTBLS8557.
Author contributions
JY: Formal Analysis, Writing – original draft, Writing – review & editing. XW: Formal Analysis, Writing – original draft, Writing – review & editing. QY: Formal Analysis, Writing – original draft. JS: Formal Analysis. JC: Formal Analysis. ZL: Conceptualization, Writing – original draft, Writing – review & editing, Supervision, Funding acquisition. HM: Conceptualization, Writing – review & editing, Supervision, Funding acquisition.
Funding
The author(s) declare financial support was received for the research, authorship, and/or publication of this article. This study was supported by the National Key R&D Program of China (grant no. 2018YFA0900300), Tianjin Synthetic Biotechnology Innovation Capacity Improvement Project (grant no. TSBICIP-PTJS-001, TSBICIP-CXRC-001), and Key project at central government level: The ability establishment of sustainable use for valuable Chinese medicine resources [2060302].
Acknowledgments
We thank Dr. Lei Zhao to helped us in chlorophyll fluorescence experiments and analysis.
Conflict of interest
The authors declare that the research was conducted in the absence of any commercial or financial relationships that could be construed as a potential conflict of interest.
Publisher’s note
All claims expressed in this article are solely those of the authors and do not necessarily represent those of their affiliated organizations, or those of the publisher, the editors and the reviewers. Any product that may be evaluated in this article, or claim that may be made by its manufacturer, is not guaranteed or endorsed by the publisher.
Supplementary material
The Supplementary Material for this article can be found online at: https://www.frontiersin.org/articles/10.3389/fpls.2023.1281348/full#supplementary-material
Supplementary Data Sheet 1 | Genome-scale metabolic model of iJTC6240 in SBML format.
Supplementary Data Sheet 2 | Genome-scale metabolic model of iJTC6240 in Microsoft Excel format.
References
Allen, J. (2002). Photosynthesis of ATP-electrons, proton pumps, rotors, and poise. Cell 110, 273–276. doi: 10.1016/s0092-8674(02)00870-x
Allen, J. F. (2003). Cyclic, pseudocyclic and noncyclic photophosphorylation: new links in the chain. Trends Plant Sci. 8, 15–19. doi: 10.1016/s1360-1385(02)00006-7
Altschul, S. F., Madden, T. L., Schäffer, A. A., Zhang, J., Zhang, Z., Miller, W., et al. (1997). Gapped BLAST and PSI-BLAST: a new generation of protein database search programs. Nucleic Acids Res. 25, 3389–3402. doi: 10.1093/nar/25.17.3389
Appelhagen, I., Wulff, A. K., Wendell, M., Hvoslef, A. K., Russell, J., Oertel, A., et al. (2018). Colour bio-factories: Towards scale-up production of anthocyanins in plant cell cultures. Metab. Eng. 48, 218–232. doi: 10.1016/j.ymben.2018.06.004
Arya, S. S., Rookes, J. E., Cahill, D. M., Lenka, S. K. (2020). Next-generation metabolic engineering approaches towards development of plant cell suspension cultures as specialized metabolite producing biofactories. Biotechnol. Adv. 45, 107635–107649. doi: 10.1016/j.biotechadv.2020.107635
Azizi, P., Rafii, M. Y., Maziah, M., Abdullah, S. N., Hanafi, M. M., Latif, M. A., et al. (2015). Understanding the shoot apical meristem regulation: a study of the phytohormones, auxin and cytokinin, in rice. Mech. Dev. 135, 1–15. doi: 10.1016/j.mod.2014.11.001
Badr, A., Angers, P., Desjardins, Y. (2011). Metabolic profiling of photoautotrophic and photomixotrophic potato plantlets (Solanum tuberosum) provides new insights into acclimatization. PCTOC 107, 13–24. doi: 10.1007/s11240-011-9951-5
Bock, R. (2014). Genetic engineering of the chloroplast: novel tools and new applications. Curr. Opin. Biotechnol. 26, 7–13. doi: 10.1016/j.copbio.2013.06.004
Bogart, E., Myers, C. R. (2016). Multiscale metabolic modeling of C4 plants: connecting nonlinear genome-scale models to leaf-scale metabolism in developing maize Leaves. PloS One 11, e0151722. doi: 10.1371/journal.pone.0151722
Bonangelino, C. J., Nau, J. J., Duex, J. E., Brinkman, M., Wurmser, A. E., Gary, J. D., et al. (2002). Osmotic stress-induced increase of phosphatidylinositol 3,5-bisphosphate requires Vac14p, an activator of the lipid kinase Fab1p. J. Cell Biol. 156, 1015–1028. doi: 10.1083/jcb.200201002
Botero, K., Restrepo, S., Pinzón, A. (2018). A genome-scale metabolic model of potato late blight suggests a photosynthesis suppression mechanism. BMC Genomics 19, 863–877. doi: 10.1186/s12864-018-5192-x
Casanova, E., Moysset, L., Trillas, M. I. (2008). Effects of agar concentration and vessel closure on the organogenesis and hyperhydricity of adventitious carnation shoots. Biol. Plantarum. 52, 1–8. doi: 10.1007/s10535-008-0001-z
Chan, S. H. J., Cai, J., Wang, L., Simons, M. N., Maranas, C. D. (2017). Standardizing biomass reactions and ensuring complete mass balance in genome-scale metabolic models. Bioinformatics 33, 3603–3609. doi: 10.1093/bioinformatics/btx453
Chanemougasoundharam, A., Sarkar, D., Pandey, S., Alb, F., Helali, O., Minhas, J. (2004). Culture tube closure-type affects potato plantlets growth and chlorophyll contents. Biol. Plantarum. 48, 7–11. doi: 10.1023/B:BIOP.0000024268.20248.33
Chatsurachai, S., Furusawa, C., Shimizu, H. (2012). An in silico platform for the design of heterologous pathways in nonnative metabolite production. BMC Bioinf. 13, 93–105. doi: 10.1186/1471-2105-13-93
Chatterjee, A., Huma, B., Shaw, R., Kundu, S. (2017). Reconstruction of Oryza sativa indica genome scale metabolic model and its responses to varying RuBisCO activity, light intensity, and enzymatic cost conditions. Front. Plant Sci. 8. doi: 10.3389/fpls.2017.02060
Chatterjee, A., Kundu, S. (2015). Revisiting the chlorophyll biosynthesis pathway using genome scale metabolic model of Oryza sativa japonica. Sci. Rep. 5, 14975–14989. doi: 10.1038/srep14975
Cheung, C. Y., Williams, T. C., Poolman, M. G., Fell, D. A., Ratcliffe, R. G., Sweetlove, L. J. (2013). A method for accounting for maintenance costs in flux balance analysis improves the prediction of plant cell metabolic phenotypes under stress conditions. Plant J. 75, 1050–1061. doi: 10.1111/tpj.12252
Choi, S. R., Kim, M. J., Eun, J. S., Ahn, M. S., Lim, H. C., Ryu, J., et al. (2004). Effects of membrane filter and sucrose concentrations on the growth of balloon flower (Platycodon grandiflorum A. DC.) plantlets in vitro. J. Plant Biotechnol. 31, 209–217. doi: 10.5010/JPB.2004.31.3.209
Colijn, C., Brandes, A., Zucker, J., Lun, D. S., Weiner, B., Farhat, M. R., et al. (2009). Interpreting expression data with metabolic flux models: predicting Mycobacterium tuberculosis mycolic acid production. PloS Comput. Biol. 5, e1000489. doi: 10.1371/journal.pcbi.1000489
Colombié, S., Nazaret, C., Bénard, C., Biais, B., Mengin, V., Solé, M., et al. (2015). Modelling central metabolic fluxes by constraint-based optimization reveals metabolic reprogramming of developing Solanum lycopersicum (tomato) fruit. Plant J. 81, 24–39. doi: 10.1111/tpj.12685
Cournac, L., Dimon, B., Carrier, P., Lohou, A. E., Chagvardieff, P. (1991). Growth and photosynthetic characteristics of Solanum tuberosum plantlets cultivated in vitro in different conditions of aeration, sucrose supply, and CO2 enrichment. Plant Physiol. 97, 112–117. doi: 10.1104/pp.97.1.112
De Oliveira, T., Balduino, M. C. M., De Carvalho, A. A., Bertolucci, S. K. V., Cossa, M. C., Coelho, A. D., et al. (2021). The effect of alternative membrane system, sucrose, and culture methods under photosynthetic photon flux on growth and volatile compounds of mint in vitro. IVCDB-. Plant 57, 529–540. doi: 10.1007/s11627-020-10147-z
Desjardins, Y. (1995). “Photosynthesis in vitro – on the factors regulating CO2 assimilation in micropropagation systems,” in Acta horticulturae (Leuven, Belgium: International Society for Horticultural Science (ISHS), 45–62. doi: 10.17660/ActaHortic.1995.393.5
Desjardins, Y., Dubuc, J. F., Badr, A. (2009). “In vitro culture of plants: a stressful activity,” in Acta horticulturae (Leuven, Belgium: International Society for Horticultural Science (ISHS), 29–50. doi: 10.17660/ActaHortic.2009.812.1
De Souza, A. P., Gaspar, M., Da Silva, E. A., Ulian, E. C., Waclawovsky, A. J., Nishiyama, M. Y., Jr., et al. (2008). Elevated CO2 increases photosynthesis, biomass and productivity, and modifies gene expression in sugarcane. Plant Cell Environ. 31, 1116–1127. doi: 10.1111/j.1365-3040.2008.01822.x
Eckstein, A., Zięba, P., Gabryś, H. (2012). Sugar and light effects on the condition of the photosynthetic apparatus of Arabidopsis thaliana cultured in vitro. J. Plant Growth Regulation. 31, 90–101. doi: 10.17660/ActaHortic.2009.812.1
Farhi, M., Marhevka, E., Ben, J., Algamas, A., Liang, Z., Zeevi, V., et al. (2011). Generation of the potent anti-malarial drug artemisinin in tobacco. Nat. Biotechnol. 29, 1072–1074. doi: 10.1038/nbt.2054
Finkemeier, I., Sweetlove, L. J. (2009). The role of malate in plant homeostasis. Biol. Rep. 1, 47–56. doi: 10.3410/b1-47
Florea, L., Song, L., Salzberg, S. L. (2013). Thousands of exon skipping events differentiate among splicing patterns in sixteen human tissues. Res 2, 188–192. doi: 10.12688/f1000research.2-188.v2
Heirendt, L., Arreckx, S., Pfau, T., Mendoza, S. N., Richelle, A., Heinken, A., et al. (2019). Creation and analysis of biochemical constraint-based models using the COBRA Toolbox v.3.0. Nat. Protoc. 14, 639–702. doi: 10.1038/s41596-018-0098-2
Horton, P., Park, K. J., Obayashi, T., Fujita, N., Harada, H., Adams, C. J., et al. (2007). WoLF PSORT: protein localization predictor. Nucleic Acids Res. 35, 585–587. doi: 10.1093/nar/gkm259
Hsiao, T. C. (1973). Plant responses to water stress. Annu. Rev. Plant Physiol. 24, 519–570. doi: 10.1146/annurev.pp.24.060173.002511
Itkin, M., Rogachev, I., Alkan, N., Rosenberg, T., Malitsky, S., Masini, L., et al. (2011). Glycoalkaloid metabolism is required for steroidal alkaloid glycosylation and prevention of phytotoxicity in tomato. Plant Cell. 23, 4507–4525. doi: 10.1105/tpc.111.088732
Ivanova, M., Van Staden, J. (2010). Natural ventilation effectively reduces hyperhydricity in shoot cultures of Aloe polyphylla Schönland ex Pillans. Plant Growth Regulation. 60, 143–150. doi: 10.1007/s10725-009-9430-8
Jiang, Z., Kempinski, C., Kumar, S., Kinison, S., Linscott, K., Nybo, E., et al. (2018). Agronomic and chemical performance of field-grown tobacco engineered for triterpene and methylated triterpene metabolism. Plant Biotechnol. J. 16, 1110–1124. doi: 10.1111/pbi.12855
Kadleček, P., Rank, B., Tichá, I. (2003). Photosynthesis and photoprotection in Nicotiana tabacum L. in vitro-grown plantlets. J. Plant Physiol. 160, 1017–1024. doi: 10.1078/0176-1617-00980
Kanehisa, M., Goto, S. (2000). KEGG: kyoto encyclopedia of genes and genomes. Nucleic Acids Res. 28, 27–30. doi: 10.1093/nar/28.1.27
Kim, B., Kim, W. J., Kim, D. I., Lee, S. Y. (2015a). Applications of genome-scale metabolic network model in metabolic engineering. J. Ind. Microbiol. Biotechnol. 42, 339–348. doi: 10.1007/s10295-014-1554-9
Kim, D., Langmead, B., Salzberg, S. L. (2015b). HISAT: a fast spliced aligner with low memory requirements. Nat. Methods 12, 357–360. doi: 10.1038/nmeth.3317
Kitaya, Y., Fukuda, O., Kozai, T., Kirdmanee, C. (1995). Effects of light intensity and lighting direction on the photoautotrophic growth and morphology of potato plantlets in vitro. Sci. Horticult. 62, 15–24. doi: 10.1016/0304-4238(94)00760-D
Klages, K. U., Boldingh, H. L., Cooney, J. M., Macrae, E. A. (2004). Planteose is a short-term storage carbohydrate in Actinidia leaves. Funct. Plant Biol. 31, 1205–1214. doi: 10.1071/fp04126
Kozai, T. (2010). Photoautotrophic micropropagation-environmental control for promoting photosynthesis. Prop. Ornam. Plants. 10, 188–204.
Lakshmanan, M., Lim, S. H., Mohanty, B., Kim, J. K., Ha, S. H., Lee, D. Y. (2015). Unraveling the light-specific metabolic and regulatory signatures of rice through combined in Silico modeling and multiomics analysis. Plant Physiol. 169, 3002–3020. doi: 10.1104/pp.15.01379
Lakshmanan, M., Zhang, Z., Mohanty, B., Kwon, J. Y., Choi, H. Y., Nam, H. J., et al. (2013). Elucidating rice cell metabolism under flooding and drought stresses using flux-based modeling and analysis. Plant Physiol. 162, 2140–2150. doi: 10.1104/pp.113.220178
Lau, W., Sattely, E. S. (2015). Six enzymes from mayapple that complete the biosynthetic pathway to the etoposide aglycone. Science 349, 1224–1228. doi: 10.1126/science.aac7202
Lea, P. J., Blackwell, R. D. (1993). “Ammonia assimilation, photorespiration and amino acid biosynthesis,” in Photosynthesis and Production in a Changing Environment: A field and laboratory manual. Eds. Hall, D. O., Scurlock, J. M. O., Bolhàr, H. R., Leegood, R. C., Long, S. P. (Dordrecht: Springer Netherlands), 313–336. doi: 10.1007/978-94-011-1566-7_20
Li, J., Mutanda, I., Wang, K., Yang, L., Wang, J., Wang, Y. (2019). Chloroplastic metabolic engineering coupled with isoprenoid pool enhancement for committed taxanes biosynthesis in Nicotiana benthamiana. Nat. Commun. 10, 4850–4861. doi: 10.1038/s41467-019-12879-y
Liao, Y., Yu, Z., Liu, X., Zeng, L., Cheng, S., Li, J., et al. (2019). Effect of major tea insect attack on formation of quality-related nonvolatile specialized metabolites in tea (Camellia sinensis) leaves. J. Agric. Food Chem. 67, 6716–6724. doi: 10.1021/acs.jafc.9b01854
Love, M. I., Huber, W., Anders, S. (2014). Moderated estimation of fold change and dispersion for RNA-seq data with DESeq2. Genome Biol. 15, 550–561. doi: 10.1186/s13059-014-0550-8
Luo, J., Yuan, Q., Mao, Y., Wei, F., Zhao, J., Yu, W., et al. (2022). Reconstruction of a genome-scale metabolic network for Shewanella oneidensis MR-1 and analysis of its metabolic potential for bioelectrochemical systems. Front. Bioeng. Biotechnol. 10. doi: 10.3389/fbioe.2022.913077
Lynch, J. H., Huang, X. Q., Dudareva, N. (2021). Silent constraints: the hidden challenges faced in plant metabolic engineering. Curr. Opin. Biotechnol. 69, 112–117. doi: 10.1016/j.copbio.2020.12.014
Ma, H., Zeng, A. P. (2003). Reconstruction of metabolic networks from genome data and analysis of their global structure for various organisms. Bioinformatics 19, 270–277. doi: 10.1093/bioinformatics/19.2.270
Macel, M., Visschers, I. G. S., Peters, J. L., Kappers, I. F., De Vos, R. C. H., Van Dam, N. M. (2019). Metabolomics of thrips resistance in pepper (Capsicum spp.) reveals monomer and dimer acyclic diterpene glycosides as potential chemical defenses. J. Chem. Ecol. 45, 490–501. doi: 10.1007/s10886-019-01074-4
Magneschi, L., Perata, P. (2009). Rice germination and seedling growth in the absence of oxygen. Ann. Bot. 103, 181–196. doi: 10.1093/aob/mcn121
Orth, J. D., Thiele, I., Palsson, B. (2010). What is flux balance analysis? Nat. Biotechnol. 28, 245–248. doi: 10.1038/nbt.1614
Pertea, M., Pertea, G. M., Antonescu, C. M., Chang, T. C., Mendell, J. T., Salzberg, S. L. (2015). StringTie enables improved reconstruction of a transcriptome from RNA-seq reads. Nat. Biotechnol. 33, 290–295. doi: 10.1038/nbt.3122
Poolman, M. G., Kundu, S., Shaw, R., Fell, D. A. (2013). Responses to light intensity in a genome-scale model of rice metabolism. Plant Physiol. 162, 1060–1072. doi: 10.1104/pp.113.216762
PospÃ, J., Synková, H., Haisel, D., SemoráDová, S. (2007). “Acclimation of plantlets to ex vitro conditions: effects of air humidity, irradiance, CO2 concentration and abscisic acid (a review),” in Acta horticulturae (Leuven, Belgium: International Society for Horticultural Science (ISHS), 29–38. doi: 10.17660/ActaHortic.2007.748.2
Pospóšilová, J., Tichá, I., Kadleček, P., Haisel, D., Plzáková, Š. (1999). Acclimatization of micropropagated plants to ex vitro conditions. Biol. Plantarum. 42, 481–497. doi: 10.1023/A:1002688208758
Robaina, S., Daloso, D. M., Zhang, Y., Fernie, A. R., Nikoloski, Z. (2017). Resolving the central metabolism of Arabidopsis guard cells. Sci. Rep. 7, 8307–8317. doi: 10.1038/s41598-017-07132-9
Rudge, S. A., Anderson, D. M., Emr, S. D. (2004). Vacuole size control: regulation of PtdIns(3,5)P2 levels by the vacuole-associated Vac14-Fig4 complex, a PtdIns(3,5)P2-specific phosphatase. Mol. Biol. Cell. 15, 24–36. doi: 10.1091/mbc.e03-05-0297
Sarimento, R., Villegas, A., Mazuelos, C., Garcia, J. L., Troncoso, A. (1992). Influence of the nitrogen source and concentration on N fractions and free amino acid levels of grape vine explants. Plant Soil. 144, 255–258. doi: 10.1007/BF00012882
Scheunemann, M., Brady, S. M., Nikoloski, Z. (2018). Integration of large-scale data for extraction of integrated Arabidopsis root cell-type specific models. Sci. Rep. 8, 7919–7927. doi: 10.1038/s41598-018-26232-8
Schmildt, O., Netto, A. T., Schmildt, E. R., Carvalho, V. S., Otoni, W.c., Campostrini, E., et al. (2015). Photosynthetic capacity, growth and water relations in ‘Golden’ papaya cultivated in vitro with modifications in light quality, sucrose concentration and ventilation. Theor. Exp. Plant Physiol. 27, 7–18. doi: 10.1007/s40626-014-0026-y
Seaver, S. M., Bradbury, L. M., Frelin, O., Zarecki, R., Ruppin, E., Hanson, A. D., et al. (2015). Improved evidence-based genome-scale metabolic models for maize leaf, embryo, and endosperm. Front. Plant Sci. 6. doi: 10.3389/fpls.2015.00142
Ševčíková, H., Lhotáková, Z., Hamet, J., Lipavská, H. (2019). Mixotrophic in vitro cultivations: the way to go astray in plant physiology. Physiol. Plant 167, 365–377. doi: 10.1111/ppl.12893
Shameer, S., Vallarino, J. G., Fernie, A. R., Ratcliffe, R. G., Sweetlove, L. J. (2020). Flux balance analysis of metabolism during growth by osmotic cell expansion and its application to tomato fruits. Plant J. 103, 68–82. doi: 10.1111/tpj.14707
Shi, J., Ma, W. J., Wang, C. P., Wu, W. L., Tian, J., Zhang, Y., et al. (2021). Impact of various microbial-fermented methods on the chemical profile of dark tea using a single raw tea material. J. Agric. Food Chem. 69, 4210–4222. doi: 10.1021/acs.jafc.1c00598
Sierro, N., Battey, J. N., Ouadi, S., Bakaher, N., Bovet, L., Willig, A., et al. (2014). The tobacco genome sequence and its comparison with those of tomato and potato. Nat. Commun. 5, 3833–3851. doi: 10.1038/ncomms4833
Simons, M., Saha, R., Amiour, N., Kumar, A., Guillard, L., Clément, G., et al. (2014). Assessing the metabolic impact of nitrogen availability using a compartmentalized maize leaf genome-scale model. Plant Physiol. 166, 1659–1674. doi: 10.1104/pp.114.245787
Stancato, G. C., Tucci, M. L. (2010). Monitoring the end of the in vitro phase of Anthurium andreanum Lindl. plantlets. Braz. J. Plant Physsiol. 22, 61–68. doi: 10.1590/S1677-04202010000100007
Sweetlove, L. J., Beard, K. F., Nunes, A., Fernie, A. R., Ratcliffe, R. G. (2010). Not just a circle: flux modes in the plant TCA cycle. Trends Plant Sci. 15, 462–470. doi: 10.1016/j.tplants.2010.05.006
Tcherkez, G., Cornic, G., Bligny, R., Gout, E., Ghashghaie, J. (2005). In vivo respiratory metabolism of illuminated leaves. Plant Physiol. 138, 1596–1606. doi: 10.1104/pp.105.062141
Töpfer, N., Caldana, C., Grimbs, S., Willmitzer, L., Fernie, A. R., Nikoloski, Z. (2013). Integration of genome-scale modeling and transcript profiling reveals metabolic pathways underlying light and temperature acclimation in Arabidopsis. Plant Cell. 25, 1197–1211. doi: 10.1105/tpc.112.108852
Töpfer, N., Scossa, F., Fernie, A., Nikoloski, Z. (2014). Variability of metabolite levels is linked to differential metabolic pathways in Arabidopsis's responses to abiotic stresses. PloS Comput. Biol. 10, e1003656. doi: 10.1371/journal.pcbi.1003656
Watanabe, M., Chiba, Y., Hirai, M. Y. (2021). Metabolism and regulatory functions of O-acetylserine, S-adenosylmethionine, homocysteine, and serine in plant development and environmental responses. Front. Plant Sci. 12. doi: 10.3389/fpls.2021.643403
Xiao, Y., Niu, G., Kozai, T. (2011). Development and application of photoautotrophic micropropagation plant system. PCTOC 105, 149–158. doi: 10.1007/s11240-010-9863-9
Yaseen, M., Ahmad, T., Sablok, G., Standardi, A., Hafiz, I. A. (2013). Review: role of carbon sources for in vitro plant growth and development. Mol. Biol. Rep. 40, 2837–2849. doi: 10.1007/s11033-012-2299-z
Ye, H., Hu, J., Peng, S., Zong, W., Zhang, S., Tong, L., et al. (2022). Determination of the chemical compounds of Shuchazao tea flowers at different developmental stages and in young shoots using 1H NMR-based metabolomics. Monatshefte. für. Chemie. - Chem. Monthly. 153, 409–417. doi: 10.1007/s00706-022-02928-6
Yuan, H., Cheung, C. Y., Poolman, M. G., Hilbers, P. A., Van Riel, N. A. (2016). A genome-scale metabolic network reconstruction of tomato (Solanum lycopersicum L.) and its application to photorespiratory metabolism. Plant J. 85, 289–304. doi: 10.1111/tpj.13075
Zhang, Y., Cai, J., Shang, X., Wang, B., Liu, S., Chai, X., et al. (2017). A new genome-scale metabolic model of Corynebacterium glutamicum and its application. Biotechnol. Biofuels. 10, 169–173. doi: 10.1186/s13068-017-0856-3
Keywords: genome-scale metabolic model, tobacco, flux balance analysis, in vitro cultivation, multiomics analysis
Citation: Yu J, Wang X, Yuan Q, Shi J, Cai J, Li Z and Ma H (2023) Elucidating the impact of in vitro cultivation on Nicotiana tabacum metabolism through combined in silico modeling and multiomics analysis. Front. Plant Sci. 14:1281348. doi: 10.3389/fpls.2023.1281348
Received: 22 August 2023; Accepted: 16 October 2023;
Published: 03 November 2023.
Edited by:
Peijian Cao, Zhengzhou Tobacco Research Institute of CNTC, ChinaReviewed by:
Xusheng Wang, University of Tennessee Health Science Center (UTHSC), United StatesYongxia Yang, Henan Agricultural University, China
Copyright © 2023 Yu, Wang, Yuan, Shi, Cai, Li and Ma. This is an open-access article distributed under the terms of the Creative Commons Attribution License (CC BY). The use, distribution or reproduction in other forums is permitted, provided the original author(s) and the copyright owner(s) are credited and that the original publication in this journal is cited, in accordance with accepted academic practice. No use, distribution or reproduction is permitted which does not comply with these terms.
*Correspondence: Zhichao Li, Lizhch@tib.cas.cn; Hongwu Ma, ma_hw@tib.cas.cn
†These authors have contributed equally to this work