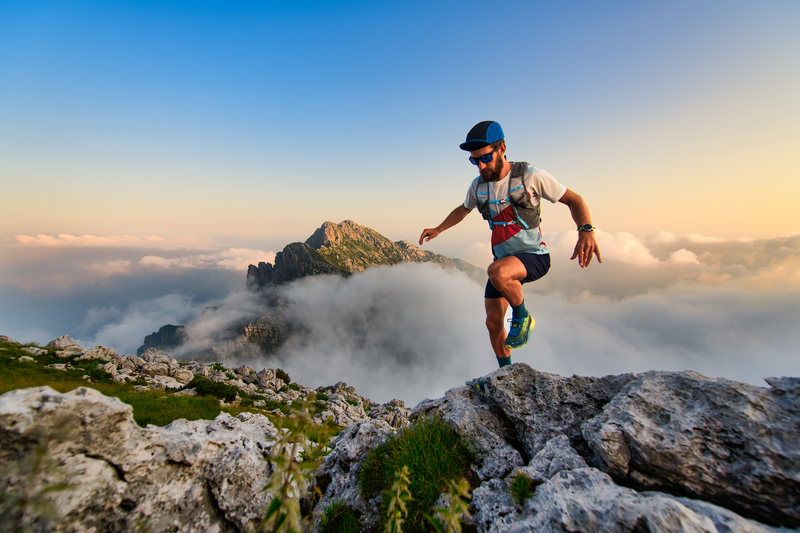
94% of researchers rate our articles as excellent or good
Learn more about the work of our research integrity team to safeguard the quality of each article we publish.
Find out more
ORIGINAL RESEARCH article
Front. Plant Sci. , 11 August 2023
Sec. Plant Breeding
Volume 14 - 2023 | https://doi.org/10.3389/fpls.2023.1229495
Soybean (Glycine max [L.] Merr.) is one of the most significant crops in the world in terms of oil and protein. Owing to the rising demand for soybean products, there is an increasing need for improved varieties for more productive farming. However, complex correlation patterns among quantitative traits along with genetic interactions pose a challenge for soybean breeding. Association studies play an important role in the identification of accession with useful alleles by locating genomic sites associated with the phenotype in germplasm collections. In the present study, a genome-wide association study was carried out for seven agronomic and yield-related traits. A field experiment was conducted in 2015/2016 at two locations that include 155 diverse soybean germplasm. These germplasms were genotyped using SoySNP50K Illumina Infinium Bead-Chip. A total of 51 markers were identified for node number, plant height, pods per plant, seeds per plant, seed weight per plant, hundred-grain weight, and total yield using a multi-locus linear mixed model (MLMM) in FarmCPU. Among these significant SNPs, 18 were putative novel QTNs, while 33 co-localized with previously reported QTLs. A total of 2,356 genes were found in 250 kb upstream and downstream of significant SNPs, of which 17 genes were functional and the rest were hypothetical proteins. These 17 candidate genes were located in the region of 14 QTNs, of which ss715580365, ss715608427, ss715632502, and ss715620131 are novel QTNs for PH, PPP, SDPP, and TY respectively. Four candidate genes, Glyma.01g199200, Glyma.10g065700, Glyma.18g297900, and Glyma.14g009900, were identified in the vicinity of these novel QTNs, which encode lsd one like 1, Ergosterol biosynthesis ERG4/ERG24 family, HEAT repeat-containing protein, and RbcX2, respectively. Although further experimental validation of these candidate genes is required, several appear to be involved in growth and developmental processes related to the respective agronomic traits when compared with their homologs in Arabidopsis thaliana. This study supports the usefulness of association studies and provides valuable data for functional markers and investigating candidate genes within a diverse germplasm collection in future breeding programs.
The human population is rapidly growing and is expected to reach 10 billion in the next 30 years (Hickey et al., 2019). Arable land for agriculture is decreasing, which poses a threat to food and nutritional security due to climate change causing different biotic and abiotic stresses (Dita et al., 2006; Eltaher et al., 2021; Rani et al., 2023a). However, global food security can be met by the cultivation of legume crops, such as soybean (Glycine max L. Merr.), which improves soil fertility through nitrogen fixation (Pandey et al., 2016). Soybean consumption is linked to physiological and health benefits, including the reduction of menopausal symptoms, diabetes mellitus, cancer, and the inhibition of cardiovascular illnesses (Messina, 1999; Messina, 2016; Karikari et al., 2020). However, the overall production of soybean is lagging in many underdeveloped nations, including Pakistan, and this presents a significant issue. Therefore, the per-unit yield of soybeans must be substantially increased. Given that the conditions in Pakistan are extremely beneficial for crop development, the country’s soybean breeding program has recently concentrated on introducing soybean varieties with high grain yields. Diverse genetic resources provide plant breeders with a better chance of creating new improved cultivars with desirable traits (Rani et al., 2023b). Identification of genomic regions associated with yield-attributing traits will help to improve the yield potential of soybean.
Seed weight is an important factor in determining soybean production, seed consumption, and evolutionary fitness (Cui et al., 2004; Gandhi, 2009; Li et al., 2019). To select cultivars with a variety of end uses, soybean breeders must generate a large variability in seed weight. In some particular edamame types (accessions), the soybean hundred seed weight can reach as high as 60 g, whereas in wild types (Glycine soja Sieb. et Zucc.) it does not exceed 1 g. Therefore, the domestication of soybeans also focused on improving seed weight (Lee et al., 2011; Zhou et al., 2015; Han et al., 2016; Wang et al., 2016). Seed weight is regarded as a complex quantitative trait controlled by a large number of important genes and loci, as well several undetectable loci with minimal impacts; as a result, these polygenes interact with the environment. SoyBase (www.soybase.org) contains more than 300 quantitative trait loci (QTLs) for seed weight. However, it is challenging to utilize these QTLs in breeding programs due to the higher confidence interval and lower genetic variation of linkage mapping data (Gupta et al., 2005). Therefore, linkage disequilibrium-based marker-trait association has been used to take advantage of all recombination events occurring in a natural population (Asins, 2002; Rafalski, 2002).
Genome-wide association study (GWAS) is one of the promising approaches for identifying genetic variations responsible for particular traits (Contreras-Soto et al., 2017). Although GWAS is still a relatively new approach in the fields of molecular biology and plant breeding, it has been widely used in crops such as Capsicum, maize, Sorghum, and soybean (Wang et al., 2012; Morris et al., 2013; Zhang et al., 2015b; Contreras-Soto et al., 2017; Han et al., 2018). According to reports, GWAS is more accurate than well-established methods, such as bi-parental QTL mapping, at identifying candidate genes (Qi et al., 2020). For instance, Miao et al. (2020) recently identified GmSWEET39 (Glyma.15 g049200/Glyma15g05470) utilizing regional association mapping for seed oil. When this gene was overexpressed in Arabidopsis, the quantity of seed oil rose by at least 10%. On all 20 chromosomes of soybean, many QTNs have been discovered and reported through GWAS (Sun et al., 2012; Chaudhary et al., 2015; Zhang et al., 2015a; Wang et al., 2016; Zhang et al., 2016; Fang et al., 2017; Yan et al., 2017b; Copley et al., 2018; Wen et al., 2018; Assefa et al., 2019; Jiang et al., 2019; Zhao et al., 2019). However, population type, size, and the GWAS approach can all lead to differing mapping results. Single-marker genome-wide scan models, such as the mixed linear model (MLM) and general linear model (GLM), are most frequently used for mapping loci related to seed weight in soybean (Wen et al., 2018). The problem of multiple test correction for the threshold significant value as well as mapping power are a couple of the drawbacks of these models. Different multi-locus models, like those for soybean, have been developed and employed in recent GWAS studies.
Population structure, kinship, and the level of linkage disequilibrium (LD) have the greatest effects on the precision and effectiveness of QTLs discovered by GWAS (Neale and Savolainen, 2004; Weir, 2010; Korte and Farlow, 2013). However, biasness in GWAS created by the aforementioned factors can be removed by adjusting the false discovery rate (FDR), via modifications to the model, and the use of population structure matrices and modified kinship (Kang et al., 2008; Vanraden, 2008; Wang et al., 2012; Li et al., 2013; Brzyski et al., 2017). Such modifications in GWAS designs can lead to more accurate identification of significant marker-trait associations, which is reinforced by more recent improvements in computational approaches (Takeuchi et al., 2013; Tang et al., 2016; Kichaev et al., 2019; Qi et al., 2020). The use of bioinformatics techniques has increased the possible identification of potential genes for target QTL. One such methods is to use a co-expression network, which gives genes with similar functions priority. Numerous crops, including maize (Schaefer et al., 2018), rice (Sarkar et al., 2014), peanuts (Zhang et al., 2019), Arabidopsis (Angelovici et al., 2017), and soybean (Wu et al., 2019; Yang et al., 2019; Razzaq et al., 2023), have effectively benefited from its application. Through the incorporation of co-expression network analysis, Palumbo et al. (2014) found a class of hub genes that result in considerable transcriptome reprogramming throughout grapevine development. Hub genes (genes with strong connectivity) may provide information about the function of a gene in the network (Das et al., 2017).
The current study was conducted using genotypes from the USDA-ARS with the aim of identifying molecular markers and candidate genes that are related to yield and other important agronomic traits using GWAS. To our knowledge, this is the first study to describe the identification of genetic factors regulating grain yield, as well as high-performing genotypes, in a Pakistani environment.
A total of 155 soybean accessions were collected from the USDA-ARS germplasm collection center (Supplementary Table 1). All plant materials were planted at two locations: the National Institute for Biotechnology and Genetic Engineering, Faisalabad (31°’42’N 73°’02’E), and the Nuclear Institute of Agriculture, Tando Jam (25°’60’N 68°’60’E), during August 2015/2016. A field experiment was conducted using a single-row plot randomized complete block design with three repetitions for the tested conditions in four environments (two locations × 2 years). Seedbeds were prepared by ploughing once with a cultivator, then planking and ploughing twice with a rotavator. Sowing was carried out with the use of a dibbler to keep a spacing of 3 inches between plants. For appropriate emergence, a row-to-row gap of 30 cm and a seed depth of 1–2 inches were maintained. For each soybean accession, three 2.43m rows were used. Weather conditions, including temperature, rainfall, and humidity, during the growing period in 2015/2016 at both locations were obtained from https://www.worldweatheronline.com/(Figure 1).
Figure 1 Weather conditions for the soybean genotypes growth period during 2015 and 2016. Monthly rainfall (mm) (left x-axis) and relative humidity (%) (right x-axis).
Plants from each row were randomly chosen to record phenotypic data at full maturity in the years 2015 and 2016. Plant height (PH) was measured from above the surface of the soil to the tip of the main stem. The number of nodes (NN) were counted on the main stem of each plant. Likewise, pods per plant (PPP) were counted on each plant. Seeds per plant (SDPP) were measured by counting the number of seeds on each plant. Seed weight per plant (SWPP) was measured by calculating the weight of all the seeds harvested from a single plant. For hundred grain weight (HGW), 100 seeds were selected from each genotype to calculate seed weight. The total yield (TY) of each genotype was calculated on a plot-by-plot basis after harvesting.
Combined analysis of variance (ANOVA) was used to estimate the genotype, environment, and genotype environment interaction for 2015/2016. The soybean accessions of the GWAS panel were considered as a fixed effect, whereas environment and block were considered as a random effect. Correlations between PH, NN, PP, SDPP, SWPP, HGW, and TY were observed by using mean data of all the traits in the R package “Performance Analytics” to draw the correlation matrix.
For genotyping the population, Illumina Infinium SoySNP50K Bead Chip data from the Soybase database (https://www.soybase.org/snps/) was downloaded to enable genotyping of the population using the Illumina Infinium SoySNP50K Bead Chip. A total of 42,291 SNPs were found for the selected genotypes, of which 211 that were found in unanchored sequence scaffolds were eliminated before further investigation. In TASSEL v5.0, the remaining 42,080 SNPs were imported. Monomorphic SNPs, SNPs with more than 20% of the genotype’s data missing, SNPs with more than 10% heterozygosity, and SNPs with a minor allele frequency of less than 5% were removed from the data. Finally, the remaining 35,110 SNPs were employed for the GWAS study and diversity analysis. An SNP density plot was constructed using the R package CMplot.
Population structures of 155 diverse genotypes were investigated using STRUCTURE 2.3.1 software. The number of subgroups (K) was set from one to 10, with three replications. The length of the burn-in period and number of Monto Carlo Markov chain (MCMC) replication were both set to 10,000 replicates. An admixture model along with a correlated allele frequency model (independent of each run) was used to analyze the population structure (Shi et al., 2016). STRUCTURE HARVESTER was used to estimate the best-suited K in this population.
Fixed and random model Circulating Probability (FarmCPU) implemented in the R package was used for GWAS. The FarmCPU model incorporates significant markers as covariates in a stepwise regression model MLM and uses a multiple locus linear mixed model (MLMM) to largely minimize the confusion between tested markers and kinship (Liu et al., 2016). Average data of all the traits in each year was used as phenotype, whereas 35,110 SNPs obtained from the 50K SNP chip from SoyBase were taken as genotype for GWAS analysis. The SNPs associated with traits with P ≤ 1.2 × 10-4 (-log10P = 3.92) were identified as significant SNPs.
By using TASSEL 5.0 software, pairwise LD between the markers was estimated using the squared coefficient (r2) of alleles. Average r2 dropped to half of its maximum value when the decay rate of LD was plotted as the chromosomal distance between markers. The critical value of r2 beyond which LD was likely to be caused by linkage was set at r2 = 0.1.
The putative genes underlying the ±250 Kb genomic region of significant SNPs were searched using G.max Williams 82.a2 as the reference genome in SoyBase (https://www.soybase.org/snps/). Additionally, functional annotation of each gene was investigated using SoyBase to find the potential candidate genes. The following criteria were used to choose candidate genes: (i) genes with known functions in soybean associated with a trait of interest; (ii) genes located by significant SNPs; and (iii) genes with known functions in Arabidopsis orthologs associated with the desired trait. The enrichment of Gene Ontology (GO) terms was calculated by comparing all the genes included in each QTN to the number of genes annotated in each GO term using ShinyGO 0.76 web software (Ge et al., 2020). For the identified genes, enrichment analysis was performed to check whether the set is enriched with the genes of a certain pathway or functional category. Genes annotated in the interval were compared with their orthologs in other plant species using The Arabidopsis Information Resource (TAIR). The validity of potential candidate genes was then investigated in the literature.
The results obtained from combined ANOVA showed that environment was the main influence on all the traits except hundred grain weight, which is mainly influenced by G × E interaction, i.e., 39% (Table 1). A correlation matrix of average data for PH, NN, PPP, SDPP, SWPP, HGW, and TY showed that the traits were positively correlated (Figure 2). TY showed a high level of positive correlation with PPP, SDPP, and SWPP but a low level of positive correlation with PH, NN, and HGW. PH and HGW showed a low level of positive correlation with all the traits. The correlation observed for PPP was positive but high with NN, SDPP, SWPP, and TY and slightly low with PH and HGW. SDPP showed a moderate positive correlation with SWPP and TY. The correlation observed for HGW was positive but low with all the traits. The frequency distributions of the phenotypic data for the quantitative characteristics PH, NN, PPP, SDPP, SWPP, HGW, and TY revealed a continuous distribution (Figure 3).
Figure 2 Correlation analysis of 155 soybean accessions between seven traits: plant height (PH), number of nodes (NN), pods per plant (PPP), seed per plant (SDPP), seed weight per plant (SWPP), hundred grain weight (HGW), and total yield (TY). One star ('*'), Two stars ('**'), Three stars ('***') denote that the corresponding variable is significant at 10%, 5%, 1% level, respectively. Absence of star denotes no significant variable.
Figure 3 Frequency distribution of the mean data of agronomic traits of soybean accessions across the environments.
STRUCTURE Harvester revealed a delta K peak at K = 2 (Figure 4A), demonstrating the presence of two subpopulations in the panel of 155 soybean natural populations. STRUCTURE 2.3.4 produced a bar plot that displayed two subpopulations with little differentiation but a lot of mixing (Figure 4B). Fst values (mean inbreeding coefficients of the subpopulation relative to the overall population) for subpopulation 1 and subpopulation 2 were 0.1101 and 0.4970, respectively (Supplementary Table 2). Individuals in the same cluster were separated on average by 0.3508 for subpopulation 1 and 0.2385 for subpopulation 2. (Supplementary Table 2). A genotype relating to each cluster was demonstrated in terms of membership proportion, which was found to be 0.4573 and 0.5427 for subpopulation 1 and subpopulation 2, respectively (Supplementary Table 2). Among populations, the average allele frequency divergence observed was 0.126 (Supplementary Table 2).
Figure 4 Population STRUCTURE analysis of 155 soybean accessions for genetic diversity analysis. (A) Bar plot divides the population into two cluster in which red represents cluster 1 and green represents cluster 2. (B) Plot showing the results from STRUCTURE HARVESTER. The delta K peak corresponds to K = 2.
To assess the LD decay for the entire genome, 35,110 SNPs were used. The LD decay with increasing physical distance was shown by a scatter plot of r2 against physical distance. The average genetic distance at which LD declined below r2 of 0.1 was used to calculate the average QTL confidence interval (CI). The whole-genome average maximum r2 value was recorded at 0.44, which decayed to 0.22 at a CI of 479,078 bp for the QTLs (Figure 5). The average SNP density varied over each chromosome, ranging from 40.57 kb per SNP on chromosome 1 to 20.21 kb per SNP on chromosome 18 (Supplementary Table 3; Figure 6). A total of 35,110 high-quality SNPs retained after filtering were used for GWAS analysis. SNPs on each chromosome varied from 1,251 (chromosome 12) to 2,868 (chromosome 18), with an average of 1,755 SNPs per chromosome (Supplementary Table 3).
Figure 5 Genome-wide average linkage disequilibrium (LD) decay rate. The x-axis shows the distance (base pair) between SNPs, and the y-axis shows the LD value.
Figure 6 SNP density plot drawn using Cmplot. The horizontal axis shows the chromosome length (Mb); the color bar exhibits the number of SNPs.
Genome-wide association analysis was performed for the grand mean of phenotypic traits of all the environments and 35,100 SNP markers using the FarmCPU, in which P + K values were used as covariates for reducing the FDR. A total of 51 significant SNPs were identified for PH, NN, PPP, SDPP, SWPP, HGW, and TY (Table 2). Of these 51 significant SNPs, 18 were putatively novel, whereas the remaining 33 SNPs colocalized with previously reported QTLs. Most of these QTNs have a positive effect on the traits (Figure 7). Manhattan plots and associated Q-Q plots are shown in Figure 8. A maximum of 12 SNPs were found to be associated with the PPP, and only two SNPs were significantly associated with NN.
Table 2 SNPs significantly reported for seven soybean traits along with previously reported QTLs in overlapping regions.
Figure 7 Phenotypic differences between accessions carrying different alleles of significant SNPs for hundred grain weight (A), plant height (B), seed weight per plant (C), pods per plant (D), total yield (E), and seed per plant (F).
Figure 8 Genome-wide association analysis Manhattan plots and Q-Q plots of 155 soybean accessions for plant height (A), number of nodes (B), pods per plant (C), seeds per plant (D), seed weight per plant (E), hundred grain weight (F), and total yield (G).
Genes located in 500-kbp genomic regions of each significant SNP were identified as candidate genes. For 51 QTNs, 2,356 genes were identified closer to significant SNPs. Gene Ontology web software ShinyGO was used to clarify the putative activities of these genes and classified them on the basis of distinct functional groups (Figure 9). Of these genes, 17 were found to be functionally annotated genes, while the remaining genes were hypothetical proteins with no functional annotation (Table 3; Supplementary Table 4). To confirm the function of these genes, the soybean data base SoyBase (https://www.soybase.org/) was used. Among these functionally annotated genes, Glyma.09G171300 (GO:0017004), cytochrome b6-f complex subunit 8 is located on chromosome 9 near significant SNP ss715603826 for HGW. Candidate genes located near peak SNPs for PH were Glyma.01G199200, Glyma.01G201600 (GO:0005515), Glyma.11G143900 (GO:0033063), Glyma.11G145300 (GO:0008146), Glyma.12G017300 (GO:0003676), and Glyma.12G014600 (GO:0003677) encoding lsd one like 1, Tetratricopeptide repeat (TPR)-like superfamily protein, DNA repair protein RAD51 homolog 4, Protein-tyrosine sulfotransferase, binding partner of acd11 1, and Origin recognition complex subunit 3. A total of five candidate genes that were identified closer to most significant SNPs for PPP were Glyma.02G065600 (GO:0016779), Glyma.06G153500 (GO:0005524), Glyma.06G153400 (GO:0016655), Glyma.08G018500 (GO:0016787), and Glyma.10G065700 (GO:0050613), which encode DNA polymerase lambda (POLL), ABC transporter family protein, NAD(P)H-quinone oxidoreductase subunit O, Serine/threonine-protein phosphatase, and Delta (14)-sterol reductase, respectively. For SWPP, GWAS identified seven significant SNPs; however, no annotated gene was located near four of these SNPs, while the other three SNPs have the functionally annotated genes Glyma.01G007800 (GO:0003682), Glyma.06G321300 (GO:0030366), and Glyma.12G083700 (GO:0016538) on chromosomes 1, 6, and 12, respectively. These genes have the functional annotation of DNA (cytosine-5)-methyltransferase CMT3, Molybdopterin synthase sulfur carrier subunit, and Cyclin-dependent kinases regulatory subunit 2, respectively. Out of six significantly associated SNPs for SWPP, only the single SNP ss715632502 on chromosome 18 has candidate gene Glyma.18G297900 (GO:0005488), which functions as HEAT repeat-containing protein. Similar to the SWPP, TY has only one significant SNP, ss715620131, on chromosome 14 that has the functionally annotated candidate gene Glyma.14G009900 (GO:0061077) encoding Chaperonin-like RbcX protein. Of these 17 genes, four candidate genes, Glyma.01g199200, Glyma.10g065700 (GO:0050613), Glyma.18g297900 (GO:0005488), and Glyma.14g009900 (GO:0061077), were located in the vicinity of the novel QTNs ss715580365, ss715608427, ss715632502, and ss715620131 (Tables 2, 3), respectively.
Figure 9 Gene ontology enrichment analysis of 17 genes for their functional categories. (A) Molecular function. (B) Biology process. (C) Cellular components identified using Shiny GO. Fo enrichment analysis false discovery rate (FDR) was calculated based on a p value of 0.05.
Table 3 Functional annotation of potential candidate genes along with their expression tissues with respect to Arabidopsis thaliana homologs.
Numerous studies on QTL mapping in soybean have revealed details about the genetic regions that underlie the genetic control of important agronomic traits. However, these results have very low mapping resolution. Despite being an essential source of plant protein and vegetable oil, soybean production is lower than other key crops. The precision of QTNs and the genetic diversity in the selected association panel dictate the usefulness and efficacy of MAS in a crop. More phenotypic and genotypic variation in the association panel would increase the chances of discovering QTNs and valuable alleles that might be employed as molecular markers for marker-assisted breeding (Zhao et al., 2019). Because of its significant photoperiod response, soybean was challenging to grow in unfavorable environmental conditions and grow to full maturity (Zhang et al., 2016). Breeders will always continue to focus on yield-related traits and other qualitative traits as they are directly related to the productivity and quality of crops (Bruce et al., 2019; Luo et al., 2023). When direct selection for yield is difficult, they also serve as selection goals in plant breeding programs. To promote crop development, crop germplasm collections are characterized for yield-related traits (Adeboye et al., 2021). There are reports about a complicated inheritance pattern for soybean yield and its sensitivity to the environment (Bhat et al., 2022). Therefore, improving soybean production through the manipulation of traits associated with yield has been the long-term objective of breeders. A key component of the soybean improvement method for creating varieties with greater yield potential is identifying the genetic basis of yield-related features.
Genome-wide association studies are now viewed as an important method for identifying genomic regions linked to complex traits in a variety of crops (Tibbs Cortes et al., 2021; Priyanatha et al., 2022). In the current study, GWAS was used for the identification of QTNs associated with PH, NN, PPP, SDPP, SWPP, HGW, and TY. A panel of 155 soybeans accessions and 35,100 SNPs after imputation were used for marker-trait association. LD block helps in determining the distance between the marker and candidate gene that will not undergo a crossing over event during meiosis. However, LD varies between species and populations (Li et al., 2018b). In our study, for 155 soybean accessions, the overall LD decay distance across the entire genome was 479,078 bp (r2 = 0.1), which was higher than the previously reported distance of 119.07 kb in cultivated soybean but within the reported range (90–574 kb) (Jiang et al., 2019). Moreover, 54,175 functionally annotated genes are present in the 975 Mb genome of cultivated soybean (Wang et al., 2016). Average SNP spacing reported in our study was 27.78 kb (Supplementary Table 3), with large gaps, which was theoretically enough for effective GWAS analysis; however, a high-resolution map with SNP markers can be helpful in future to find more trait-QTN relationships. In a previous study, Priyanatha et al. (2022) also reported low SNP coverage that can be improved in future GWAS studies by increasing SNP coverage with few chromosomal gaps. Improvements in GWAS can be made for lower level polymorphisms and shorter LD decay block, as proposed by He et al. (2017). In addition, some other strategies, such as mapping of LD blocks (Bandillo et al., 2015), SNPLDBs (He et al., 2017), and haplotype blocks (Greenspan and Geiger, 2004; Contreras-Soto et al., 2017), are also being used. In GWAS, panel RILs can be employed to maximize the heritability of QTNs (Viana et al., 2017; Luo et al., 2023). All of these factors may strengthen marker-trait relationships and boost the detection rate. Furthermore, Mohammadi et al. (2020) describe further techniques to improve GWAS detection of real marker-trait relationships and QTL validation.
A total of 51 QTNs were identified in this study of which 33 are colocalized with the previously reported QTLs and 18 were putatively novel QTNs (Table 2). Of these novel QTNs, two were identified for HGW and NN each, while 6, 4, 3, and 1 QTNs were associated with PH, SDPP, PPP, and TY respectively. After confirming the SNP validation, the information obtained from this study could be used in future breeding programs for trait introgression. These QTNs were further used to find the candidate genes in a 500 kb region.
In current study, GWAS revealed 2,356 genes for six traits based on the gene expression data and annotations. We only included 17 potential candidate genes, the activities of which were involved in controlling soybean plant height, node number, pods per plant, seeds per plant, seed weight per plant, hundred grain weight, and total yield (Table 3). Among these genes, Glyma.09g171300 is proposed as a candidate gene for HGW and is located in the vicinity of ss715603826, which was previously reported by Li et al. (2018a) when identifying the role of amino acids in soybean seed. A pleiotropic cluster of six QTLs was colocated at ss715603826 on chromosome 9. This QTN showed a positive allelic effect on the HGW (Figure 7) and is present in a similar region with a previously reported QTL seed weight QTL viz., Seed weight 27-3 (Vieira et al., 2006). This gene encodes Cytochrome b6-f complex subunit 8, which mediates electron transfer during photosynthesis. Yamori et al. (2016) confirmed in rice that increasing photosynthesis through the manipulation of cyt b6f results in an increase or decrease in plant yield.
The Glyma.14g009900 gene that was identified in the flanking region of TY QTN is homologous to Arabidopsis gene AT5G19855.1. This gene encodes an RbcX protein that has a chaperon-like function; therefore, it plays a significant role in the correct assembly of RbcL and RbcS subunits during RuBisCO biogenesis and is also essential for the protein to attain its maximum activity (Rudi et al., 1998; Kolesiński et al., 2011). Rubisco catalyzes the first step in two opposing chemical pathways: photorespiration (using O2 as a substrate) and photosynthetic carbon fixation (using CO2 as a substrate) (Andrews and Lorimer, 1985; Erb and Zarzycki, 2018). The photosynthetic uptake of CO2 results in the production of functioning sugars (Gutteridge and Gatenby, 1995; Choquet and Wollman, 2023), which are responsible for plant development and yield (Saschenbrecker et al., 2007). The Glyma.18g297900 gene that was identified in the flanking region of ss715632502, a QTN for seed weight per plant, is homologous to the Arabidopsis gene AT1G67140.3 (SWEETIE) and encodes HEAT repeat-containing protein. In Arabidopsis, this gene affects carbon utilization and has major role in the growth and development stages of the plant (Veyres et al., 2008).
Three candidate genes, Glyma.01g007800, Glyma.06g321300, and Glyma.12g083700, were found in the flanking region of QTNs for seeds per plant. Glyma.01g007800, which encodes DNA (cytosine-5)-methyltransferase CMT3, is homologous to the Arabidopsis gene AT1G69770.1. DNA methylation is an epigenetic variation that regulates a variety of functions, including stress responses, expression of transposable elements (TEs), and gene expression (Gallego-Bartolomé, 2020). The methods for maintaining DNA methylation (MDM) are dependent on the context of the cytosine sequence (CG, CHG, or CHH, H=T, C, A), and they are catalyzed by several DNA methyltransferases (Zhang et al., 2018). Methyltransferase 1 (MET1) maintains CG cytosine methylation. Chromomethylase 3 (CMT3) and CMT2 sustain CHG cytosine methylation (Stroud et al., 2014). Numerous studies have shown that altering DNA methylation offers an alternate strategy for crop improvement, making it a significant target for such manipulation (King, 2015; Feng et al., 2022). In previous studies, different activations of DNA C5-MTase genes were reported during the developmental stages of embryos and seeds in Arabidopsis, cereals, and legumes (Sharma et al., 2009; Garg et al., 2014; Qian et al., 2014; Feng et al., 2022). Another gene, Glyma.06g321300, which is homologous to the Arabidopsis gene AT4G10100.1, encodes Molybdopterin synthase sulfur carrier subunit, a ubiquitin-like protein that is similar to a molybdopterin synthase small subunit called MoaD, which contains a C-terminal thiocarboxylated glycine residue that acts as a sulfur donor for molybdopterin production. In soybean, the use of Mo as a fertilizer increases total yield (Rana et al., 2020). Additionally, Glyma.12g083700 is the gene identified for seed per plant that encodes Cyclin-dependent kinases regulatory subunit 2. The Arabidopsis homolog of this gene is AT2G27970.1, which is also known as CKS2. In a previous study, it was reported that CcKS2 regulates the function of different genes by entering the nucleus and plays an important role in the developmental stages of plants (Tamirisa et al., 2017).
For pods per plant, five genes were identified in overlapping regions or near regions of four significant QTNs. Two genes, Glyma.06g153500 and Glyma.06g153400, at chromosome six, overlap one another. Glyma.06g153500 encodes ABC transporter family protein, which is homologous to the Arabidopsis gene AT4G33460.1. In Arabidopsis, 22 functionally analyzed ABC transporters have been identified that are involved in plant development, plant nutrition, organ growth, and responses to many biotic and abiotic stresses (Kang et al., 2011; Lü et al., 2018). Many essential cellular activities that use ATP hydrolysis to energize the transport of solutes across membranes are mediated by the ATP-binding cassette (ABC) protein family, particularly the intrinsic membrane subfamilies. The ABC transport family has been widely identified in many crops, including 130 in maize (Pang et al., 2013), 121 in rice (Moon and Jung, 2014), 179 in Brassica (Yan et al., 2017a), and 154 in tomato (Ofori et al., 2018). Previously, Mishra et al. (2019), through in silico analysis, identified 261 ABC genes in soybean that are present in nine different plant tissues and are involved in seven developmental stages and stress conditions. Therefore, Glyma.06g153500 is considered as a strong candidate gene that plays an important role in soybean pods. Another candidate gene, Glyma.06g153400, was homologous to the Arabidopsis gene AT1G74880.1. This gene encodes NAD(P)H-quinone oxidoreductase subunit O, which is important for prenylquinone metabolism and vitamin K1 accumulation and is located in chloroplasts (Eugeni Piller et al., 2011; Vidal et al., 2018). Candidate gene Glyma.02g065600, which encodes DNA polymerase lambda (POLL), is homologous to the Arabidopsis gene AT1G10520.1. This gene is still novel in plants and is the only member of the X family as it is homologous to a mammalian gene. Maintenance of genome integrity is a key process in all organisms. DNA polymerases (Pols) are central players in this process as they are in charge of the faithful reproduction of the genetic information, as well as DNA repair (Pedroza-Garcia et al., 2019). The fact that the POLL promoter is activated by UV and that both overexpressing and silenced plants exhibit altered growth phenotypes support the hypothesis that DNA pol plays a significant role in plant growth (Roy, 2014). Candidate gene Glyma.08g018500 is homologous to the Arabidopsis gene AT4G11240. This gene encodes Serine/threonine-protein phosphatase, which acts as a negative regulator of the plant defense response (País et al., 2009; Máthé et al., 2019). In soybean cotyledons, the inhibitor triggers anti-fungal defense responses even in the absence of infection or elicitors (Mackintosh et al., 1994). Another candidate gene identified for pods was Glyma.10g065700, which encodes Ergosterol biosynthesis ERG4/ERG24 family and is homologous to the Arabidopsis gene AT3G52940.1, which encodes sterol C-14 reductase and plays a major role in plant cell division, embryogenesis, and development (He et al., 2003).
For plant height in soybean, six genes were identified in the genomic region of three significant QTNs. Two candidate genes, Glyma.11g143900 and Glyma.11g145300, were located in the CDS region of ss715608806, which has a positive additive effect of 3.27 on plant height. Glyma.11g145300, which encodes Protein-tyrosine sulfotransferase (TPST), is homologous to the Arabidopsis gene AT1G08030.1. TPST has been linked to a variety of significant biological processes in eukaryotic species (Zhong et al., 2020). This protein is a 500-aa type I transmembrane protein that expresses throughout the plant body. To control root development and gene expression in biological processes in Arabidopsis, including auxin production and accumulation, TPST is involved in fructose signaling (Zhong et al., 2020). TPST responds to the plant hormone auxin, which plays an important role in stem elongation (Zhou et al., 2010). Glyma.11g143900 encodes the DNA repair protein RAD51 homolog 4, which is involved in the pathway of homologous recombination, which is considered as a precise DNA damage repair process (Markmann-Mulisch et al., 2007; Angelis et al., 2023). This gene was identified as homologous to the Arabidopsis gene AT1G07745.1, which plays a role in somatic homologous recombination and pathogen-related gene transcription (Durrant et al., 2007; Angelis et al., 2023). Although the precise physiological roles of the RAD51 paralogs are still not entirely understood, they operate to promote break repair and transduce the DNA damage signal to effector kinases (Bonilla et al., 2020). Glyma.01g199200 and Glyma.01g201600, at chromosome 1, are proposed candidate genes for PH. QTN ss715580365 has been located in the CDS region of these two genes. Glyma.01g199200 encodes lsd one like 1 protein. The homolog of this gene in Arabidopsis is AT1G32540.2, which is symbolized as LOL1 and encodes plant-specific zinc finger protein and is expressed in almost all parts of plants and functions in controlled cell death (Epple et al., 2003; Borovsky et al., 2019). The rice homolog of this gene negatively regulates programmed cell death, but when it is overexpressed, it increases chlorophyll in shoots (Wang et al., 2005). In Solanaceae, this gene is involved in fruit development (Borovsky et al., 2019). Another candidate gene, Glyma.01g201600, which is homologous to the Arabidopsis gene ATCG00360.1, encodes Tetratricopeptide repeat (TPR)-like superfamily protein. In nature, tetratricopeptide repeat (TPR) and TPR-like domains are common. They participate in a variety of biological processes and are known for binding to short linear peptide motifs (Perez-Riba and Itzhaki, 2019). TPR proteins function in auxin, cytokinin, and gibberellin responses and ethylene production (Greenboim-Wainberg et al., 2005; Yoshida et al., 2005; Wei and Han, 2017). Auxin is an important plant hormone that promotes cell growth through stem elongation (Dilworth et al., 2017). Therefore, Glyma.01g201600 can be considered a strong candidate gene that plays an important role in plant height. At chromosome 12, two candidate genes, Glyma.12g017300 and Glyma.12g014600, were predicted for plant height. Glyma.12g017300 encodes a binding partner of acd11 1 and is homologous to AT4G17720.1 in Arabidopsis. This gene is uniformly present in land plants, which raises the possibility that this immunological regulatory module emerged in the early developmental stages of land plants and assisted in their colonization (Zhang et al., 2020). Glyma.12g014600 encodes Origin recognition complex subunit 3, which is an important component element in plants and plays a significant role in many biological processes, including DNA replication, checkpoint regulation, heterochromatin formation, and chromosome assembly (Chen et al., 2013; Popova et al., 2018). Glyma.12g014600 is homologous to the Arabidopsis gene AT5G16690.1, which is also known as AtORC3. All the members of the ORC gene family are expressed in all three stages of flowering, except AtORC3, which is only expressed after fertilization (Collinge et al., 2004).
The expression levels of the 17 genes described above varied significantly between extreme materials in the current investigation during the growth and developmental stages of soybean seeds. Four candidate genes, Glyma.01g199200, Glyma.10g065700, Glyma.18g297900, and Glyma.14g009900, were identified in the vicinity of the novel QTNs. Although further experimental validation of these candidate genes is required, many are involved in developmental processes controlling the expression of the respective traits, as determined through comparison with their homologs in Arabidopsis. Thus, we hypothesized that these 17 genes are potential candidates for PH, PPP, SDPP, SWPP, HGW, and TY. Consequently, the discovery of these fresh putative QTNs and candidate genes opens up a potential new supply of desired genetics for research and analysis. Therefore, these genes could be chosen for further investigation and potential functional confirmation to advance our understanding of how important agronomic traits in soybean are regulated.
To the best of our knowledge, this study is the first to look into a genetic panel of soybean lines in Pakistan using a GWAS design to identify QTLs for soybean plant height, node number, pods per plant, seeds per plant, seed weight per plant, hundred grain weight, and total yield. This study confirmed 33 QTNs that were colocalized with previously reported QTLs for yield and its components. Additionally, 19 putative novel QTNs were identified for yield and its components using a panel of 155 diverse soybean accessions. There were 17 candidate genes within a ±250 kb region of significant SNPs. Results obtained from Gene Ontology analysis of these genes showed that most of are involved in the growth and developmental stages of soybean and hence play an important role in the final yield. By adding to the growing body of research, this work increases our understanding of the true strength of genetics underlying agronomic features in soybean. The findings of the current GWAS study, along with those from the previous reports, support the idea that exotic germplasm can serve as a source of unique genetic diversity for ongoing agricultural improvement. The current study’s limitations might be overcome in future by the addition of better SNP coverage or alternative strategies, such as high-density mapping.
The original contributions presented in the study are included in the article/Supplementary Material. Further inquiries can be directed to the corresponding authors.
MA, GR, and RR conceived and designed the project. RR and GR conducted the experiments. RR, HA, AD, MW, and MKR analyzed the data. MR and HS provided technical inputs in executing experiments and data analysis. RR wrote the manuscript with input from HA and MR and feedback from all the authors. MR and HS proofread the manuscript. The final manuscript was read and approved by all the authors.
We want to convey our sincere gratitude to the United States Department of Agriculture Agricultural Research Service (USDA-ARS) for providing germplasm.
Tha author declare that the research was conducted in the absence of any commercial or financial relationships that could be construed as a potential conflict of interest.
All claims expressed in this article are solely those of the authors and do not necessarily represent those of their affiliated organizations, or those of the publisher, the editors and the reviewers. Any product that may be evaluated in this article, or claim that may be made by its manufacturer, is not guaranteed or endorsed by the publisher.
The Supplementary Material for this article can be found online at: https://www.frontiersin.org/articles/10.3389/fpls.2023.1229495/full#supplementary-material
GWAS, genome-wide association study; MCMC, Monte Carlo Markov chain; GLM, general linear model; MLMM, multiple locus linear mixed model; FDR, false discovery rate; ANOVA, analysis of variance; LD, linkage disequilibrium; SNP, single nucleotide polymorphism; QTL, quantitative trait loci; QTN, quantitative trait nucleotide; GO, gene ontology; PH, plant height; NN, nodes number; PPP, pods per plant; SDPP, seeds per plant; SWPP, seed weight per plant; HGW, hundred grain weight; TY, total yield.
Adeboye, K. A., Oduwaye, O. A., Daniel, I. O., Fofana, M., Semon, M. (2021). Characterization of flowering time response among recombinant inbred lines of WAB638-1/PRIMAVERA rice under reproductive stage drought stress. Plant Genet. Resour. 19, 1–8. doi: 10.1017/S1479262121000010
Andrews, T. J., Lorimer, G. (1985). Catalytic properties of a hybrid between cyanobacterial large subunits and higher plant small subunits of ribulose bisphosphate carboxylase-oxygenase. J. Biol. Chem. 260, 4632–4636. doi: 10.1016/S0021-9258(18)89117-7
Angelis, K. J., Záveská Drábková, L., Vágnerová, R., Holá, M. (2023). RAD51 and RAD51B play diverse roles in the repair of DNA double strand breaks in physcomitrium patens. Genes 14, 305. doi: 10.3390/genes14020305
Angelovici, R., Batushansky, A., Deason, N., Gonzalez-Jorge, S., Gore, M. A., Fait, A., et al. (2017). Network-guided GWAS improves identification of genes affecting free amino acids. Plant Physiol. 173, 872–886. doi: 10.1104/pp.16.01287
Asins, M. (2002). Present and future of quantitative trait locus analysis in plant breeding. Plant Breed. 121, 281–291. doi: 10.1046/j.1439-0523.2002.730285.x
Assefa, T., Otyama, P. I., Brown, A. V., Kalberer, S. R., Kulkarni, R. S., Cannon, S. B. (2019). Genome-wide associations and epistatic interactions for internode number, plant height, seed weight and seed yield in soybean. BMC Genom. 20, 1–12. doi: 10.1186/s12864-019-5907-7
Bandillo, N., Jarquin, D., Song, Q., Nelson, R., Cregan, P., Specht, J., et al. (2015). A population structure and genome-wide association analysis on the USDA soybean germplasm collection. Plant Genome 8, 2004.0024. doi: 10.3835/plantgenome2015.04.0024
Bhat, J. A., Adeboye, K. A., Ganie, S. A., Barmukh, R., Hu, D., Varshney, R. K., et al. (2022). Genome-wide association study, haplotype analysis, and genomic prediction reveal the genetic basis of yield-related traits in soybean (Glycine max L.). Front. Genet. 13. doi: 10.3389/fgene.2022.953833
Bonilla, B., Hengel, S. R., Grundy, M. K., Bernstein, K. A. (2020). RAD51 gene family structure and function. Annu. Rev. Genet. 54, 25–46. doi: 10.1146/annurev-genet-021920-092410
Borovsky, Y., Monsonego, N., Mohan, V., Shabtai, S., Kamara, I., Faigenboim, A., et al. (2019). The zinc-finger transcription factor Cc LOL 1 controls chloroplast development and immature pepper fruit color in Capsicum chinense and its function is conserved in tomato. Plant J. 99, 41–55. doi: 10.1111/tpj.14305
Bruce, R. W., Grainger, C. M., Ficht, A., Eskandari, M., Rajcan, I. (2019). Trends in soybean trait improvement over generations of selective breeding. Crop Sci. 59, 1870–1879. doi: 10.2135/cropsci2018.11.0664
Brzyski, D., Peterson, C. B., Sobczyk, P., Candès, E. J., Bogdan, M., Sabatti, C. (2017). Controlling the rate of GWAS false discoveries. Genetics 205, 61–75. doi: 10.1534/genetics.116.193987
Chaudhary, J., Patil, G. B., Sonah, H., Deshmukh, R. K., Vuong, T. D., Valliyodan, B., et al. (2015). Expanding omics resources for improvement of soybean seed composition traits. Front. Plant Sci. 6. doi: 10.3389/fpls.2015.01021
Chen, X., Shi, J., Hao, X., Liu, H., Shi, J., Wu, Y., et al. (2013). Os ORC 3 is required for lateral root development in rice. Plant J. 74, 339–350. doi: 10.1111/tpj.12126
Chen, Q. S., Zhang, Z. C., Liu, C. Y., Xin, D. W., Qiu, H. M., Shan, D. P., et al. (2007). QTL analysis of major agronomic traits in soybean. Agric. Sci. China 6, 399–405. doi: 10.1016/S1671-2927(07)60062-5
Choquet, Y., Wollman, F.-A. (2023). “The assembly of photosynthetic proteins,” in The Chlamydomonas Sourcebook (Massachusetts: Academic Press), 615–646.
Collinge, M. A., Spillane, C., Kohler, C., Gheyselinck, J., Grossniklaus, U. (2004). Genetic interaction of an origin recognition complex subunit and the Polycomb group gene MEDEA during seed development. Plant Cell 16, 1035–1046. doi: 10.1105/tpc.019059
Contreras-Soto, R. I., Mora, F., De Oliveira, M., Higashi, W., Scapim, C. A., Schuster, I. (2017). A genome-wide association study for agronomic traits in soybean using SNP markers and SNP-based haplotype analysis. PloS One 12, e0171105. doi: 10.1371/journal.pone.0171105
Copley, T. R., Duceppe, M.-O., O’donoughue, L. S. (2018). Identification of novel loci associated with maturity and yield traits in early maturity soybean plant introduction lines. BMC Genom. 19, 1–12. doi: 10.1186/s12864-018-4558-4
Cui, Z., James, A., Miyazaki, S., Wilson, R., Carter, T. (2004). Breeding specialty soybeans for traditional and new soyfoods. In: Liu, K. Ed., Soybeans as a Functional Food (Champaign, Illinois: AOCS Press), 264–322. doi: 10.1201/9781439822203.ch14
Das, S., Meher, P. K., Rai, A., Bhar, L. M., Mandal, B. N. (2017). Statistical approaches for gene selection, hub gene identification and module interaction in gene co-expression network analysis: an application to aluminum stress in soybean (Glycine max L.). PloS One 12, e0169605. doi: 10.1371/journal.pone.0169605
Dilworth, L., Riley, C., Stennett, D. (2017). “Plant constituents: Carbohydrates, oils, resins, balsams, and plant hormones,” in Pharmacognosy (Massachusetts: Academic Press), 61–80. doi: 10.1016/B978-0-12-802104-0.00005-6
Dita, M. A., Rispail, N., Prats, E., Rubiales, D., Singh, K. B. (2006). Biotechnology approaches to overcome biotic and abiotic stress constraints in legumes. Euphytica 147, 1–24. doi: 10.1007/s10681-006-6156-9
Durrant, W. E., Wang, S., Dong, X. (2007). Arabidopsis SNI1 and RAD51D regulate both gene transcription and DNA recombination during the defense response. Proc. Natl. Acad. Sci. 104, 4223–4227. doi: 10.1073/pnas.0609357104
Eltaher, S., Baenziger, P. S., Belamkar, V., Emara, H. A., Nower, A. A., Salem, K. F., et al. (2021). GWAS revealed effect of genotype× environment interactions for grain yield of Nebraska winter wheat. BMC Genom. 22, 1–14. doi: 10.1186/s12864-020-07308-0
Epple, P., Mack, A. A., Morris, V. R., Dangl, J. L. (2003). Antagonistic control of oxidative stress-induced cell death in Arabidopsis by two related, plant-specific zinc finger proteins. Proc. Natl. Acad. Sci. 100, 6831–6836. doi: 10.1073/pnas.1130421100
Erb, T. J., Zarzycki, J. (2018). A short history of RubisCO: the rise and fall (?) of Nature's predominant CO2 fixing enzyme. Curr. Opin. Biotechnol. 49, 100–107. doi: 10.1016/j.copbio.2017.07.017
Eugeni Piller, L., Besagni, C., Ksas, B., Rumeau, D., Bréhélin, C., Glauser, G., et al. (2011). Chloroplast lipid droplet type II NAD (P) H quinone oxidoreductase is essential for prenylquinone metabolism and vitamin K1 accumulation. Proc. Natl. Acad. Sci. 108, 14354–14359. doi: 10.1073/pnas.1104790108
Fang, C., Ma, Y., Wu, S., Liu, Z., Wang, Z., Yang, R., et al. (2017). Genome-wide association studies dissect the genetic networks underlying agronomical traits in soybean. Genome Biol. 18, 1–14. doi: 10.1186/s13059-017-1289-9
Feng, A., Kang, Z., Ling-Kui, Z., Xing, L., Shu-Min, C., Hua-Sen, W., et al. (2022). Genome-wide identification, evolutionary selection, and genetic variation of DNA methylation-related genes in Brassica rapa and Brassica oleracea. J. Integr. Agric. 21, 1620–1632. doi: 10.1016/S2095-3119(21)63827-3
Fox, C. M., Cary, T. R., Nelson, R. L., Diers, B. W. (2015). Confirmation of a seed yield QTL in soybean. Crop Sci. 55, 992–998. doi: 10.2135/cropsci2014.10.0688
Funatsuki, H., Kawaguchi, K., Matsuba, S., Sato, Y., Ishimoto, M. (2005). Mapping of QTL associated with chilling tolerance during reproductive growth in soybean. Theor. Appl. Genet. 111, 851–861. doi: 10.1007/s00122-005-0007-2
Gai, J., Wang, Y., Wu, X., Chen, S. (2007). A comparative study on segregation analysis and QTL mapping of quantitative traits in plants with a case in soybean. Front. Agric. China 1, 1–7. doi: 10.1007/s11703-007-0001-3
Gallego-Bartolomé, J. (2020). DNA methylation in plants: mechanisms and tools for targeted manipulation. New Phytol. 227, 38–44. doi: 10.1111/nph.16529
Garg, R., Kumari, R., Tiwari, S., Goyal, S. (2014). Genomic survey, gene expression analysis and structural modeling suggest diverse roles of DNA methyltransferases in legumes. PloS One 9, e88947. doi: 10.1371/journal.pone.0088947
Ge, S. X., Jung, D., Yao, R. (2020). ShinyGO: a graphical gene-set enrichment tool for animals and plants. Bioinformatics 36, 2628–2629. doi: 10.1093/bioinformatics/btz931
Greenboim-Wainberg, Y., Maymon, I., Borochov, R., Alvarez, J., Olszewski, N., Ori, N., et al. (2005). Cross talk between gibberellin and cytokinin: the Arabidopsis GA response inhibitor SPINDLY plays a positive role in cytokinin signaling. Plant Cell 17, 92–102. doi: 10.1105/tpc.104.028472
Greenspan, G., Geiger, D. (2004). Model-based inference of haplotype block variation. J. Comput. Biol. 11, 493–504. doi: 10.1089/1066527041410300
Gupta, P. K., Rustgi, S., Kulwal, P. L. (2005). Linkage disequilibrium and association studies in higher plants: present status and future prospects. Plant Mol. Biol. 57, 461–485. doi: 10.1007/s11103-005-0257-z
Gutteridge, S., Gatenby, A. A. (1995). Rubisco synthesis, assembly, mechanism, and regulation. Plant Cell 7, 809. doi: 10.1105/tpc.7.7.809
Guzman, P., Diers, B. W., Neece, D., St. Martin, S., Leroy, A., Grau, C., et al. (2007). QTL associated with yield in three backcross-derived populations of soybean. Crop Sci. 47, 111–122. doi: 10.2135/cropsci2006.01.0003
Han, K., Lee, H. Y., Ro, N. Y., Hur, O. S., Lee, J. H., Kwon, J. K., et al. (2018). QTL mapping and GWAS reveal candidate genes controlling capsaicinoid content in Capsicum. Plant Biotechnol. J. 16, 1546–1558. doi: 10.1111/pbi.12894
Han, Y., Li, D., Zhu, D., Li, H., Li, X., Teng, W., et al. (2012). QTL analysis of soybean seed weight across multi-genetic backgrounds and environments. Theor. Appl. Genet. 125, 671–683. doi: 10.1007/s00122-012-1859-x
Han, Y., Zhao, X., Liu, D., Li, Y., Lightfoot, D. A., Yang, Z., et al. (2016). Domestication footprints anchor genomic regions of agronomic importance in soybeans. New Phytol. 209, 871–884. doi: 10.1111/nph.13626
He, J. X., Fujioka, S., Li, T. C., Kang, S. G., Seto, H., Takatsuto, S., et al. (2003). Sterols regulate development and gene expression in Arabidopsis. Plant Physiol. 131, 1258–1269. doi: 10.1104/pp.014605
He, J., Meng, S., Zhao, T., Xing, G., Yang, S., Li, Y., et al. (2017). An innovative procedure of genome-wide association analysis fits studies on germplasm population and plant breeding. Theor.l Appl. Genet. 130, 2327–2343. doi: 10.1007/s00122-017-2962-9
Hickey, L. T., Hafeez, A. N., Robinson, H., Jackson, S. A., Leal-Bertioli, S., Tester, M., et al. (2019). Breeding crops to feed 10 billion. Nat. Biotechnol. 37, 744–754. doi: 10.1038/s41587-019-0152-9
Jiang, Z., Chen, J., Zhai, H., Zhou, F., Yan, X., Zhu, Y., et al. (2019). Kuroshio shape composition and distribution of filamentous diazotrophs in the East China Sea and southern Yellow Sea. J. Geophys. Res. Oceans 124, 7421–7436. doi: 10.1029/2019JC015413
Jun, T. H., Freewalt, K., Michel, A. P., Mian, R. (2014). Identification of novel QTL for leaf traits in soybean. Plant Breed. 133, 61–66. doi: 10.1111/pbr.12107
Kabelka, E., Diers, B., Fehr, W., Leroy, A., Baianu, I., You, T., et al. (2004). Putative alleles for increased yield from soybean plant introductions. Crop Sci. 44, 784–791. doi: 10.2135/cropsci2004.7840
Kang, S. T., Kwak, M., Kim, H. K., Choung, M. G., Han, W. Y., Baek, I. Y., et al. (2009). Population-specific QTLs and their different epistatic interactions for pod dehiscence in soybean [Glycine max (L.) Merr.]. Euphytica 166, 15–24. doi: 10.1007/s10681-008-9810-6
Kang, J., Park, J., Choi, H., Burla, B., Kretzschmar, T., Lee, Y., et al. (2011). “Plant ABC transporters,” in The Arabidopsis book (Rockville, USA: American Society of Plant Biologists). doi: 10.1199/tab.0153
Kang, H. M., Zaitlen, N. A., Wade, C. M., Kirby, A., Heckerman, D., Daly, M. J., et al. (2008). Efficient control of population structure in model organism association mapping. Genetics 178, 1709–1723. doi: 10.1534/genetics.107.080101
Karikari, B., Wang, Z., Zhou, Y., Yan, W., Feng, J., Zhao, T. (2020). Identification of quantitative trait nucleotides and candidate genes for soybean seed weight by multiple models of genome-wide association study. BMC Plant Biol. 20, 1–14. doi: 10.1186/s12870-020-02604-z
Kato, S., Sayama, T., Fujii, K., Yumoto, S., Kono, Y., Hwang, T.-Y., et al. (2014). A major and stable QTL associated with seed weight in soybean across multiple environments and genetic backgrounds. Theor. Appl. Genet. 127, 1365–1374. doi: 10.1007/s00122-014-2304-0
Kichaev, G., Bhatia, G., Loh, P.-R., Gazal, S., Burch, K., Freund, M. K., et al. (2019). Leveraging polygenic functional enrichment to improve GWAS power. Am. J. Hum. Genet. 104, 65–75. doi: 10.1016/j.ajhg.2018.11.008
Kim, K.-S., Diers, B., Hyten, D., Mian, R., Shannon, J., Nelson, R. (2012). Identification of positive yield QTL alleles from exotic soybean germplasm in two backcross populations. Theor. Appl. Genet. 125, 1353–1369. doi: 10.1007/s00122-012-1944-1
King, G. J. (2015). Crop epigenetics and the molecular hardware of genotype× environment interactions. Front. Plant Sci. 6. doi: 10.3389/fpls.2015.00968
Kolesiński, P., Piechota, J., Szczepaniak, A. (2011). Initial characteristics of RbcX proteins from Arabidopsis thaliana. Plant Mol. Biol. 77, 447–459. doi: 10.1007/s11103-011-9823-8
Korte, A., Farlow, A. (2013). The advantages and limitations of trait analysis with GWAS: a review. Plant Methods 9, 1–9. doi: 10.1186/1746-4811-9-29
Kuroda, Y., Kaga, A., Tomooka, N., Yano, H., Takada, Y., Kato, S., et al. (2013). QTL affecting fitness of hybrids between wild and cultivated soybeans in experimental fields. Ecol. Evol. 3, 2150–2168. doi: 10.1002/ece3.606
Lee, G. A., Crawford, G. W., Liu, L., Sasaki, Y., Chen, X. (2011). Archaeological soybean (Glycine max) in East Asia: does size matter? PloS One 6, e26720. doi: 10.1371/journal.pone.0026720
Lee, S., Jun, T., Michel, A. P., Mian, R. (2015). SNP markers linked to QTL conditioning plant height, lodging, and maturity in soybean. Euphytica 203, 521–532. doi: 10.1007/s10681-014-1252-8
Lee, S., Park, K., Lee, H., Park, E., Boerma, H. (2001). Genetic mapping of QTLs conditioning soybean sprout yield and quality. Theor. Appl. Genet. 103, 702–709. doi: 10.1007/s001220100595
Li, H., Peng, Z., Yang, X., Wang, W., Fu, J., Wang, J., et al. (2013). Genome-wide association study dissects the genetic architecture of oil biosynthesis in maize kernels. Nat. Genet. 45, 43–50. doi: 10.1038/ng.2484
Li, D., Pfeiffer, T., Cornelius, P. (2008a). Soybean QTL for yield and yield components associated with Glycine soja alleles. Crop Sci. 48, 571–581. doi: 10.2135/cropsci2007.06.0361
Li, Y. H., Reif, J. C., Hong, H. L., Li, H. H., Liu, Z. X., Ma, Y. S., et al. (2018b). Genome-wide association mapping of QTL underlying seed oil and protein contents of a diverse panel of soybean accessions. Plant Sci. 266, 95–101. doi: 10.1016/j.plantsci.2017.04.013
Li, D., Sun, M., Han, Y., Teng, W., Li, W. (2010). Identification of QTL underlying soluble pigment content in soybean stems related to resistance to soybean white mold (Sclerotinia sclerotiorum). Euphytica 172, 49–57. doi: 10.1007/s10681-009-0036-z
Li, X., Tian, R., Kamala, S., Du, H., Li, W., Kong, Y., et al. (2018a). Identification and verification of pleiotropic QTL controlling multiple amino acid contents in soybean seed. Euphytica 214, 1–14. doi: 10.1007/s10681-018-2170-y
Li, N., Xu, R., Li, Y. (2019). Molecular networks of seed size control in plants. Annu. Rev. Plant Biol. 70, 435–463. doi: 10.1146/annurev-arplant-050718-095851
Li, W., Zheng, D. H., Van, K. J., Lee, S. H. (2008b). QTL mapping for major agronomic traits across two years in soybean (Glycine max L. Merr.). J. Crop Sci. Biotechnol. 11, 171–176.
Liu, X., Huang, M., Fan, B., Buckler, E. S., Zhang, Z. (2016). Iterative usage of fixed and random effect models for powerful and efficient genome-wide association studies. PloS Genet. 12, e1005767. doi: 10.1371/journal.pgen.1005767
Liu, W., Kim, M. Y., Van, K., Lee, Y. H., Li, H., Liu, X., et al. (2011). QTL identification of yield-related traits and their association with flowering and maturity in soybean. J. Crop Sci. Biotechnol. 14. doi: 10.3389/fpls.2018.00995
Lü, H., Yang, Y., Li, H., Liu, Q., Zhang, J., Yin, J., et al. (2018). Genome-wide association studies of photosynthetic traits related to phosphorus efficiency in soybean. Front. Plant Sci. 9, 1226. doi: 10.1371/journal.pgen.1005767
Luo, S., Jia, J., Liu, R., Wei, R., Guo, Z., Cai, Z., et al. (2023). Identification of major QTLs for soybean seed size and seed weight traits using a RIL population in different environments. Front. Plant Sci. 13. doi: 10.3389/fpls.2022.1094112
Mackintosh, C., Lyon, G. D., Mackintosh, R. W. (1994). Protein phosphatase inhibitors activate anti-fungal defence responses of soybean cotyledons and cell cultures. Plant J. 5, 137–147. doi: 10.1046/j.1365-313X.1994.5010137.x
Mansur, L., Orf, J., Chase, K., Jarvik, T., Cregan, P., Lark, K. (1996). Genetic mapping of agronomic traits using recombinant inbred lines of soybean. Crop Sci. 36, 1327–1336. doi: 10.2135/cropsci1996.0011183X003600050042x
Markmann-Mulisch, U., Wendeler, E., Zobell, O., Schween, G., Steinbiss, H. H., Reiss, B. (2007). Differential requirements for RAD51 in Physcomitrella patens and Arabidopsis thaliana development and DNA damage repair. Plant Cell 19, 3080–3089. doi: 10.1105/tpc.107.054049
Máthé, C., Garda, T., Freytag, C., M-Hamvas, M. (2019). The role of serine-threonine protein phosphatase PP2A in plant oxidative stress signaling facts and hypotheses. Int. J. Mol. Sci. 20, 3028. doi: 10.3390/ijms20123028
Messina, M. J. (1999). Legumes and soybeans: overview of their nutritional profiles and health effects. Am. J. Clin. Nutr. 70, 439s–450s. doi: 10.1093/ajcn/70.3.439s
Messina, M. (2016). Soy and health update: evaluation of the clinical and epidemiologic literature. Nutrients 8, 754. doi: 10.3390/nu8120754
Miao, L., Yang, S., Zhang, K., He, J., Wu, C., Ren, Y., et al. (2020). Natural variation and selection in GmSWEET39 affect soybean seed oil content. New Phytol. 225, 1651–1666. doi: 10.1111/nph.16250
Mishra, A. K., Choi, J., Rabbee, M. F., Baek, K.-H. (2019). In silico genome-wide analysis of the ATP-binding cassette transporter gene family in soybean (Glycine max L.) and their expression profiling. BioMed. Res. Int. 2019, 01–14. doi: 10.1155/2019/8150523
Mohammadi, M., Xavier, A., Beckett, T., Beyer, S., Chen, L., Chikssa, H., et al. (2020). Identification, deployment, and transferability of quantitative trait loci from genome-wide association studies in plants. Curr. Plant Biol. 24, 100145. doi: 10.1016/j.cpb.2020.100145
Moon, S., Jung, K.-H. (2014). Genome-wide expression analysis of rice ABC transporter family across spatio-temporal samples and in response to abiotic stresses. J. Plant Physiol. 171, 1276–1288. doi: 10.1016/j.jplph.2014.05.006
Morris, G. P., Ramu, P., Deshpande, S. P., Hash, C. T., Shah, T., Upadhyaya, H. D., et al. (2013). Population genomic and genome-wide association studies of agroclimatic traits in sorghum. Proc. Natl. Acad. Sci. 110, 453–458. doi: 10.1073/pnas.12159851
Neale, D. B., Savolainen, O. (2004). Association genetics of complex traits in conifers. Trends Plant Sci. 9, 325–330. doi: 10.1016/j.tplants.2004.05.006
Ofori, P. A., Mizuno, A., Suzuki, M., Martinoia, E., Reuscher, S., Aoki, K., et al. (2018). Genome-wide analysis of ATP binding cassette (ABC) transporters in tomato. PloS One 13, e0200854. doi: 10.1371/journal.pone.0200854
Orf, J., Chase, K., Jarvik, T., Mansur, L., Cregan, P., Adler, F., et al. (1999). Genetics of soybean agronomic traits: I. Comparison of three related recombinant inbred populations. Crop Sci. 39, 1642–1651. doi: 10.2135/cropsci1999.3961642x
País, S. M., Téllez-Iñón, M. T., Capiati, D. A. (2009). Serine/threonine protein phosphatases type 2A and their roles in stress signaling. Plant Signal. Behav. 4, 1013–1015. doi: 10.4161/psb.4.11.9783
Palomeque, L., Li-Jun, L., Li, W., Hedges, B., Cober, E. R., Rajcan, I. (2009). QTL in mega-environments: II. Agronomic trait QTL co-localized with seed yield QTL detected in a population derived from a cross of high-yielding adapted× high-yielding exotic soybean lines. Theor. Appl. Genet. 119, 429–436. doi: 10.1007/s00122-009-1048-8
Palumbo, M. C., Zenoni, S., Fasoli, M., Massonnet, M., Farina, L., Castiglione, F., et al. (2014). Integrated network analysis identifies fight-club nodes as a class of hubs encompassing key putative switch genes that induce major transcriptome reprogramming during grapevine development. Plant Cell 26, 4617–4635. doi: 10.1105/tpc.114.133710
Pandey, M. K., Roorkiwal, M., Singh, V. K., RaMalingam, A., Kudapa, H., Thudi, M., et al. (2016). Emerging genomic tools for legume breeding: current status and future prospects. Front. Plant Sci. 7. doi: 10.3389/fpls.2016.00455
Pang, K., Li, Y., Liu, M., Meng, Z., Yu, Y. (2013). Inventory and general analysis of the ATP-binding cassette (ABC) gene superfamily in maize (Zea mays L.). Gene 526, 411–428. doi: 10.1016/j.gene.2013.05.051
Panthee, D., Pantalone, V., West, D., Saxton, A., Sams, C. (2005). Quantitative trait loci for seed protein and oil concentration, and seed size in soybean. Crop Sci. 45, 2015–2022. doi: 10.2135/cropsci2004.0720
Pedroza-Garcia, J.-A., De Veylder, L., Raynaud, C. (2019). Plant DNA polymerases. Int. J. Mol. Sci. 20, 4814. doi: 10.3390/ijms20194814
Perez-Riba, A., Itzhaki, L. S. (2019). The tetratricopeptide-repeat motif is a versatile platform that enables diverse modes of molecular recognition. Curr. Opin. Struct. Biol. 54, 43–49. doi: 10.1016/j.sbi.2018.12.004
Popova, V. V., Brechalov, A. V., Georgieva, S. G., Kopytova, D. V. (2018). Nonreplicative functions of the origin recognition complex. Nucleus 9, 460–473. doi: 10.1080/19491034.2018.1516484
Priyanatha, C., Torkamaneh, D., Rajcan, I. (2022). Genome-Wide Association Study of soybean germplasm derived from Canadian× Chinese crosses to mine for novel alleles to improve seed yield and seed quality traits. Front. Plant Sci. 823. doi: 10.3389/fpls.2022.866300
Qi, Z., Song, J., Zhang, K., Liu, S., Tian, X., Wang, Y., et al. (2020). Identification of QTNs controlling 100-seed weight in soybean using multilocus genome-wide association studies. Front. Genet. 11. doi: 10.3389/fgene.2020.00689
Qian, Y., Xi, Y., Cheng, B., Zhu, S. (2014). Genome-wide identification and expression profiling of DNA methyltransferase gene family in maize. Plant Cell Rep. 33, 1661–1672. doi: 10.1007/s00299-014-1645-0
Rafalski, A. (2002). Applications of single nucleotide polymorphisms in crop genetics. Curr. Opin. Plant Biol. 5, 94–100. doi: 10.1016/s1369-5266(02)00240-6
Rana, M. S., Bhantana, P., Imran, M., Saleem, M. H., Moussa, M. G., Khan, Z., et al. (2020). Molybdenum potential vital role in plants metabolism for optimizing the growth and development. Ann. Environ. Sci. Toxicol. 4, 032–044. doi: 10.17352/aest
Rani, R., Raza, G., Ashfaq, H., Rizwan, M., Shimelis, H., Tung, M. H., et al. (2023a). Analysis of genotype× environment interactions for agronomic traits of soybean (Glycine max [L.] Merr.) using association mapping. Front. Genet. 13. doi: 10.3389/fgene.2022.1090994
Rani, R., Raza, G., Tung, M. H., Rizwan, M., Ashfaq, H., Shimelis, H., et al. (2023b). Genetic diversity and population structure analysis in cultivated soybean (Glycine max [L.] Merr.) using SSR and EST-SSR markers. PloS One 18, e0286099. doi: 10.1371/journal.pone.0286099
Razzaq, M. K., Rani, R., Xing, G., Xu, Y., Raza, G., Aleem, M., et al. (2023). Genome-wide identification and analysis of the hsp40/J-protein family reveals its role in soybean (Glycine max) growth and development. Genes 14, 1254. doi: 10.3390/genes14061254
Roy, S. (2014). DNA polymerase?-a novel DNA repair enzyme in higher plant genome. Plant Sci. Today 1, 140–146. doi: 10.14719/pst.2014.1.3.59
Rudi, K., Skulberg, O. M., Jakobsen, K. S. (1998). Evolution of cyanobacteria by exchange of genetic material among phyletically related strains. J. Bacteriol. Res. 180, 3453–3461. doi: 10.1128/JB.180.13.3453-3461.1998
Sarkar, N. K., Kim, Y.-K., Grover, A. (2014). Coexpression network analysis associated with call of rice seedlings for encountering heat stress. Plant Mol. Biol. 84, 125–143. doi: 10.1007/s11103-013-0123-3
Saschenbrecker, S., Bracher, A., Rao, K. V., Rao, B. V., Hartl, F. U., Hayer-Hartl, M. (2007). Structure and function of RbcX, an assembly chaperone for hexadecameric Rubisco. Cell 129, 1189–1200. doi: 10.1016/j.cell.2007.04.025
Schaefer, R. J., Michno, J.-M., Jeffers, J., Hoekenga, O., Dilkes, B., Baxter, I., et al. (2018). Integrating coexpression networks with GWAS to prioritize causal genes in maize. Plant Cell 30, 2922–2942. doi: 10.1105/tpc.18.00299
Sharma, R., Mohan Singh, R., Malik, G., Deveshwar, P., Tyagi, A. K., Kapoor, S., et al. (2009). Rice cytosine DNA methyltransferases–gene expression profiling during reproductive development and abiotic stress. FEBS J. 276, 6301–6311. doi: 10.1111/j.1742-4658.2009.07338.x
Shi, A., Buckley, B., Mou, B., Motes, D., Morris, J. B., Ma, J., et al. (2016). Association analysis of cowpea bacterial blight resistance in USDA cowpea germplasm. Euphytica 208, 143–155. doi: 10.1007/s10681-015-1610-1
Specht, J., Chase, K., Macrander, M., Graef, G., Chung, J., Markwell, J., et al. (2001). Soybean response to water: a QTL analysis of drought tolerance. Crop Sci. 41, 493–509. doi: 10.2135/cropsci2001.412493x
Stroud, H., Do, T., Du, J., Zhong, X., Feng, S., Johnson, L., et al. (2014). Non-CG methylation patterns shape the epigenetic landscape in Arabidopsis. Nat. Struct. Mol. 21, 64–72. doi: 10.1038/nsmb.2735
Sun, D., Li, W., Zhang, Z., Chen, Q., Ning, H., Qiu, L., et al. (2006). Quantitative trait loci analysis for the developmental behavior of soybean (Glycine max L. Merr.). Theor. Appl. Genet. 112, 665–673. doi: 10.1007/s00122-005-0169-y
Sun, Y. N., Pan, J. B., Shi, X. L., Du, X. Y., Wu, Q., Qi, Z. M., et al. (2012). Multi-environment mapping and meta-analysis of 100-seed weight in soybean. Mol. Biol. Rep. 39, 9435–9443. doi: 10.1007/s11033-012-1808-4
Takeuchi, Y., Nishimura, Y., Yoshikawa, T., Kuriyama, J., Kimura, Y., Saiga, T. (2013). Genome-Wide Association Studies and Genomic Prediction (New Jersey: Humana Press).
Tamirisa, S., Vudem, D. R., Khareedu, V. R. (2017). A cyclin dependent kinase regulatory subunit (CKS) gene of pigeonpea imparts abiotic stress tolerance and regulates plant growth and development in Arabidopsis. Front. Plant Sci. 8. doi: 10.3389/fpls.2017.00165
Tang, Y., Liu, X., Wang, J., Li, M., Wang, Q., Tian, F., et al. (2016). GAPIT version 2: an enhanced integrated tool for genomic association and prediction. Plant Genome 9, 2011.0120. doi: 10.3835/plantgenome2015.11.0120
Teng, W., Han, Y., Du, Y., Sun, D., Zhang, Z., Qiu, L., et al. (2009). QTL analyses of seed weight during the development of soybean (Glycine max L. Merr.). Heredity 102, 372–380. doi: 10.1038/hdy.2008.108
Tibbs Cortes, L., Zhang, Z., Yu, J. (2021). Status and prospects of genome-wide association studies in plants. Plant Genome 14, e20077. doi: 10.1002/tpg2.20077
Tischner, T., Allphin, L., Chase, K., Orf, J., Lark, K. (2003). Genetics of seed abortion and reproductive traits in soybean. Crop Sci. 43, 464–473. doi: 10.2135/cropsci2003.4640
Vanraden, P. M. (2008). Efficient methods to compute genomic predictions. J. Dairy Sci. 91, 4414–4423. doi: 10.3168/jds.2007-0980
Veyres, N., Danon, A., Aono, M., Galliot, S., Karibasappa, Y. B., Diet, A., et al. (2008). The Arabidopsis sweetie mutant is affected in carbohydrate metabolism and defective in the control of growth, development and senescence. Plant J. 55, 665–686. doi: 10.1111/j.1365-313X.2008.03541.x
Viana, J. M. S., Mundim, G. B., Pereira, H. D., Andrade, A. C. B. (2017). Efficiency of genome-wide association studies in random cross populations. Mol. Breed. 37, 1–13. doi: 10.1007/s11032-017-0703-z
Vidal, L. S., Kelly, C. L., Mordaka, P. M., Heap, J. T. (2018). Review of NAD (P) H-dependent oxidoreductases: Properties, engineering and application. Biochim. Biophys. Acta Bioenerg. 1866, 327–347. doi: 10.1016/j.bbapap.2017.11.005
Vieira, A. J. D., Oliveira, D., Soares, T. C. B., Schuster, I., Piovesan, N. D., Martínez, C. A., et al. (2006). Use of the QTL approach to the study of soybean trait relationships in two populations of recombinant inbred lines at the F7 and F8 generations. Braz. J. Plant Physiol. 18, 281–290. doi: 10.1590/S1677-04202006000200004
Wang, J., Chu, S., Zhang, H., Zhu, Y., Cheng, H., Yu, D. (2016). Development and application of a novel genome-wide SNP array reveals domestication history in soybean. Sci. Rep. 6, 1–10. doi: 10.1038/srep20728
Wang, X., Jiang, G. L., Green, M., Scott, R. A., Song, Q., Hyten, D. L., et al. (2014). Identification and validation of quantitative trait loci for seed yield, oil and protein contents in two recombinant inbred line populations of soybean. Mol. Genet. Genom. 289, 935–949. doi: 10.1007/s00438-014-0865-x
Wang, L., Pei, Z., Tian, Y., He, C. (2005). OsLSD1, a rice zinc finger protein, regulates programmed cell death and callus differentiation. Mol. Plant-Microbe Interact. 18, 375–384. doi: 10.1094/MPMI-18-0375
Wang, M., Yan, J., Zhao, J., Song, W., Zhang, X., Xiao, Y., et al. (2012). Genome-wide association study (GWAS) of resistance to head smut in maize. Plant Sci. 196, 125–131. doi: 10.1016/j.plantsci.2012.08.004
Wei, K., Han, P. (2017). Comparative functional genomics of the TPR gene family in Arabidopsis, rice and maize. Mol. Breed. 37, 1–18. doi: 10.1007/s11032-017-0751-4
Weir, B. S. (2010). Statistical genetic issues for genome-wide association studies. Genome 53, 869–875. doi: 10.1139/G10-062
Wen, Y. J., Zhang, H., Ni, Y. L., Huang, B., Zhang, J., Feng, J. Y., et al. (2018). Methodological implementation of mixed linear models in multi-locus genome-wide association studies. Brief. Bioinform. 19, 700–712. doi: 10.1093/bib/bbw145
Wu, Z., Wang, M., Yang, S., Chen, S., Chen, X., Liu, C., et al. (2019). A global coexpression network of soybean genes gives insights into the evolution of nodulation in nonlegumes and legumes. New Phytol. 223, 2104–2119. doi: 10.1111/nph.15845
Yamori, W., Kondo, E., Sugiura, D., Terashima, I., Suzuki, Y., Makino, A. (2016). Enhanced leaf photosynthesis as a target to increase grain yield: insights from transgenic rice lines with variable Rieske FeS protein content in the cytochrome b6/f complex. Plant Cell Environ. 39, 80–87. doi: 10.1111/pce.12594
Yan, C., Duan, W., Lyu, S., Li, Y., Hou, X. (2017a). Genome-wide identification, evolution, and expression analysis of the ATP-binding cassette transporter gene family in Brassica rapa. Front. Plant Sci. 8. doi: 10.3389/fpls.2017.00349
Yan, L., Hofmann, N., Li, S., Fer reira, M. E., Song, B., Jiang, G., et al. (2017b). Identification of QTL with large effect on seed weight in a selective population of soybean with genome-wide association and fixation index analyses. BMC Genom. 18, 1–11. doi: 10.1186/s12864-017-3922-0
Yang, S., Miao, L., He, J., Zhang, K., Li, Y., Gai, J. (2019). Dynamic transcriptome changes related to oil accumulation in developing soybean seeds. Int. J. Mol. Sci. 20, 2202. doi: 10.3390/ijms20092202
Yang, Z., Xin, D., Liu, C., Jiang, H., Han, X., Sun, Y., et al. (2013). Identification of QTLs for seed and pod traits in soybean and analysis for additive effects and epistatic effects of QTLs among multiple environments. Mol. Genet. Genom. 288, 651–667. doi: 10.1007/s00438-013-0779-z
Yao, D., Liu, Z., Zhang, J., Liu, S., Qu, J., Guan, S., et al. (2015). Analysis of quantitative trait loci for main plant traits in soybean. Genet. Mol. Res. 14, 6101–6109. doi: 10.4238/2015.June.8.8
Yoshida, H., Nagata, M., Saito, K., Wang, K. L., Ecker, J. R. (2005). Arabidopsis ETO1 specifically interacts with and negatively regulates type 2 1-aminocyclopropane-1-carboxylate synthases. BMC Plant Biol. 5, 1–13. doi: 10.1186/1471-2229-5-14
Zhang, X., Ai, G., Wang, X., Peng, H., Yin, Z., Dou, D. (2020). Genome-wide identification and molecular evolution analysis of BPA genes in green plants. Phytopathol. Res. 2, 1–11. doi: 10.1186/s42483-020-0046-2
Zhang, D., Cheng, H., Wang, H., Zhang, H., Liu, C., Yu, D. (2010). Identification of genomic regions determining flower and pod numbers development in soybean (Glycine max L.). J. Genet. Genomics 37, 545–556. doi: 10.1016/S1673-8527(09)60074-6
Zhang, H., Hao, D., Sitoe, H. M., Yin, Z., Hu, Z., Zhang, G., et al. (2015a). Genetic dissection of the relationship between plant architecture and yield component traits in soybean (Glycine max) by association analysis across multiple environments. Plant Breed. 134, 564–572. doi: 10.1111/pbr.12305
Zhang, H., Lang, Z., Zhu, J.-K. (2018). Dynamics and function of DNA methylation in plants. Nat. Rev. Mol. Cell Biol. 19, 489–506. doi: 10.1038/s41580-018-0016-z
Zhang, J., Song, Q., Cregan, P. B., Jiang, G. L. (2016). Genome-wide association study, genomic prediction and marker-assisted selection for seed weight in soybean (Glycine max). Theor. Appl. Genet. 129, 117–130. doi: 10.1007/s00122-015-2614-x
Zhang, J., Song, Q., Cregan, P. B., Nelson, R. L., Wang, X., Wu, J., et al. (2015b). Genome-wide association study for flowering time, maturity dates and plant height in early maturing soybean (Glycine max) germplasm. BMC Genom. 16, 1–11. doi: 10.1186/s12864-015-1441-4
Zhang, H., Wang, M. L., Schaefer, R., Dang, P., Jiang, T., Chen, C. (2019). GWAS and coexpression network reveal Ionomic variation in cultivated Peanut. J. Agric. Food Chem. 67, 12026–12036. doi: 10.1021/acs.jafc.9b04939
Zhao, X., Dong, H., Chang, H., Zhao, J., Teng, W., Qiu, L., et al. (2019). Genome wide association mapping and candidate gene analysis for hundred seed weight in soybean [Glycine max (L.) Merrill]. BMC Genom. 20, 1–11. doi: 10.1186/s12864-019-6009-2
Zhong, Y., Xie, J., Wen, S., Wu, W., Tan, L., Lei, M., et al. (2020). TPST is involved in fructose regulation of primary root growth in Arabidopsis thaliana. Plant Mol. Bio. 103, 511–525. doi: 10.1007/s11103-020-01006-x
Zhou, Z., Jiang, Y., Wang, Z., Gou, Z., Lyu, J., Li, W., et al. (2015). Resequencing 302 wild and cultivated accessions identifies genes related to domestication and improvement in soybean. Nat. Biotech. 33, 408–414. doi: 10.1038/nbt.3096
Keywords: soybean, single nucleotide polymorphism, GWAS, gene ontology, candidate gene discovery
Citation: Rani R, Raza G, Ashfaq H, Rizwan M, Razzaq MK, Waheed MQ, Shimelis H, Babar AD and Arif M (2023) Genome-wide association study of soybean (Glycine max [L.] Merr.) germplasm for dissecting the quantitative trait nucleotides and candidate genes underlying yield-related traits. Front. Plant Sci. 14:1229495. doi: 10.3389/fpls.2023.1229495
Received: 30 May 2023; Accepted: 25 July 2023;
Published: 11 August 2023.
Edited by:
Babu Valliyodan, Lincoln University, United StatesReviewed by:
Milind Ratnaparkhe, ICAR Indian Institute of Soybean Research, IndiaCopyright © 2023 Rani, Raza, Ashfaq, Rizwan, Razzaq, Waheed, Shimelis, Babar and Arif. This is an open-access article distributed under the terms of the Creative Commons Attribution License (CC BY). The use, distribution or reproduction in other forums is permitted, provided the original author(s) and the copyright owner(s) are credited and that the original publication in this journal is cited, in accordance with accepted academic practice. No use, distribution or reproduction is permitted which does not comply with these terms.
*Correspondence: Muhammad Arif, bWFyaWZfbmliZ2VAeWFob28uY29t; Hussein Shimelis, c2hpbWVsaXNoQHVrem4uYWMuemE=
Disclaimer: All claims expressed in this article are solely those of the authors and do not necessarily represent those of their affiliated organizations, or those of the publisher, the editors and the reviewers. Any product that may be evaluated in this article or claim that may be made by its manufacturer is not guaranteed or endorsed by the publisher.
Research integrity at Frontiers
Learn more about the work of our research integrity team to safeguard the quality of each article we publish.