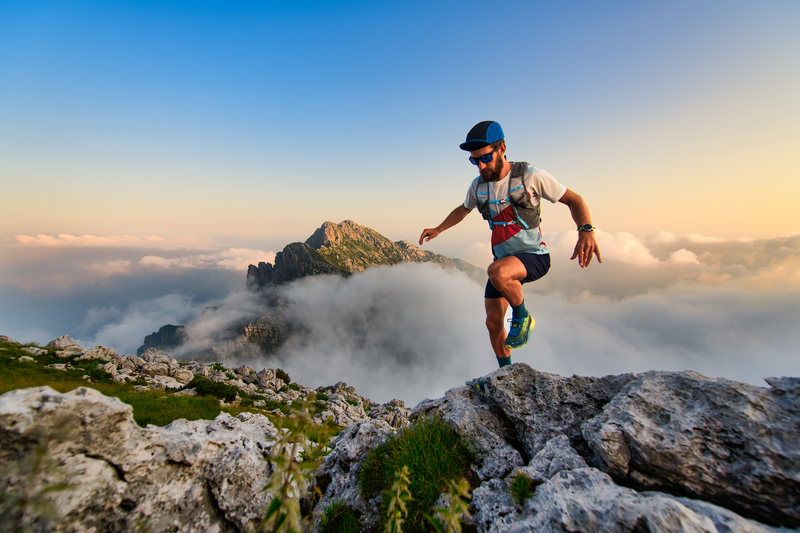
94% of researchers rate our articles as excellent or good
Learn more about the work of our research integrity team to safeguard the quality of each article we publish.
Find out more
ORIGINAL RESEARCH article
Front. Plant Sci. , 16 October 2023
Sec. Plant Bioinformatics
Volume 14 - 2023 | https://doi.org/10.3389/fpls.2023.1224394
Plants produce a wide range of bioactive peptides as part of their innate defense mechanisms. With the explosive growth of plant-derived peptides, verifying the therapeutic function using traditional experimental methods are resources and time consuming. Therefore, it is necessary to predict the therapeutic function of plant-derived peptides more effectively and accurately with reduced waste of resources and thus expedite the development of plant peptides. We herein developed a repository of plant peptides predicted to have multiple therapeutic functions, named as MFPPDB (multi-functional plant peptide database). MFPPDB including 1,482,409 single or multiple functional plant origin therapeutic peptides derived from 121 fundamental plant species. The functional categories of these therapeutic peptides include 41 different features such as anti-bacterial, anti-fungal, anti-HIV, anti-viral, and anti-cancer. The detailed physicochemical information of these peptides was presented in functional search and physicochemical property search module, which can help users easily access the peptide information by the plant peptide species, ID, and functions, or by their peptide ID, isoelectric point, peptide sequence, and molecular weight through web-friendly interface. We further matched the predicted peptides to nine state-of-the-art curated functional peptide databases and found that at least 293,408 of the peptides possess functional potentials. Overall, MFPPDB integrated a massive number of plant peptides have single or multiple therapeutic functions, which will facilitate the comprehensive research in plant peptidomics. MFPPDB can be freely accessed through http://124.223.195.214:9188/mfppdb/index.
Since the introduction of insulin therapy in the last century, peptide therapies have also been used in medical treatment in recent years, and dozens of peptide drugs have been approved to enter the market (Muttenthaler et al., 2021). For example, the peptides for oral use (Kong et al., 2020), a long-acting glucagon-like peptide-1 in healthy male subjects (Elbrønd et al., 2002), an anti-cancer peptide targeting the transcription factor FOXM1 (Cheng et al., 2023), the cyclic peptide drugs (Zhang and Chen, 2021), peptide Etelcalcetide indicated for secondary hyperparathyroidism (Hamano et al., 2017). Therapeutic peptides have been used to treat various diseases such as cancer, diabetes, HIV, and cardiovascular diseases (Wang et al., 2022). Historically, therapeutic peptides were defined as peptides with 2 to 50 amino acids, which play a essential therapeutic role in the human body (Henninot et al., 2018). However, it was also noted that peptide length might not be a serious limitation for peptide drug development (Lau and Dunn, 2018).
Plant proteins contain an average of 350 to 400 amino acids (Ramírez-Sánchez et al., 2016), while longer peptides are universally toxic and less stable (Kim et al., 2014). Plants possess many kinds of defense mechanisms to combat the physical, chemical, and biological coercion they face in nature (Das et al., 2020). Plant-derived bioactive peptides have potentially wide applicability in the agrochemical and pharmaceutical industries and are a new and unexplored area in the field of proteomics and peptidomics (Sarethy, 2017). An enormous amount of therapeutic peptides with potential functions for the treatment of human diseases have been identified from plant sources, making plant peptides may become a new beginnings for therapeutic peptides (Chai et al., 2021).
In order to facilitate the comprehensive application of therapeutic peptides, various databases of therapeutic peptides have been established over the decades, such as APD3 that includes the antimicrobial peptides (Wang et al., 2016); PhytAMP, dedicated to plant antimicrobial peptides (Hammami et al., 2009); PlantPepDB, which include the manually curated plant peptides (Das et al., 2020); ACovPepDB, which collected anti-coronavirus peptides (Zhang et al., 2022a); PlantAFP, which collected curated plant-origin antifungal peptides (Tyagi et al., 2019); CancerPPD, a database of anticancer peptides and proteins (Tyagi et al., 2015); AHTPDB for analysis and presentation of antihypertensive peptides (Kumar et al., 2015); BIOPEP, database of sensory peptides and amino acids (Iwaniak et al., 2016); DAMPD, a manually curated antimicrobial peptide database (Seshadri Sundararajan et al., 2012); THPdb, which collectes FDA-approved peptide and protein therapeutics (Usmani et al., 2017); and YADAMP, another database of antimicrobial peptides (Piotto et al., 2012). While, PlantPepDB, PhytAMP, and PlantAFP are all databases of plant-derived therapeutic peptides; PhytAMP only contains antimicrobial peptides; PlantAFP just contains plant-derived antifungal peptides. Although PlantPepDB is a plant-specific database with a variety of therapeutic and bioactive functions, it currently only includes 3,848 peptides, of which only 1,465 are therapeutic peptides.
Identifying the therapeutic functions of plant peptides through traditional experimental methods can be both expensive and time-consuming. To address this issue, we propose the Multi-Functional Plant Peptides Database (MFPPDB), a database of plant peptides predicted to contain multiple therapeutic functions. With the MFPPDB, researchers can more efficiently identify plant peptides with potential therapeutic functions, reducing the scope and cost of identification. MFPPDB provides comprehensive information on multifunctional plant peptides, including information on their functional characteristics, detailed physicochemical properties, and a universal multifunctional prediction server. It contains 1,482,409 plant peptides with single or multiple therapeutic functions derived from represented plant databases, i.e. TPIA (Xia et al., 2019), TAIR (Lamesch et al., 2012), RGAP (Kawahara et al., 2013), SGN (Zhou et al., 2022), SpudDB (Pham et al., 2020), IWGSC (Consortium et al., 2014) and Phytozome (Goodstein et al., 2012). The establishment of MFPPDB will not only facilitate the identification of multifunctional plant peptides, but also accelerate the research and development of therapeutic peptide drugs, making them more accessible to the market. Furthermore, it will also attract researchers from a wide range of fields involved in the research and development of peptide drugs.
The MFPPDB database applied a modifying PrMFTP (Multi-functional therapeutic peptides) algorithm based on multi-head self-attention mechanism and class weight optimization to identify the peptides from 121 plant species (Yan et al., 2022). A total of 1,482,409 peptide sequences with therapeutic functions were finally obtained, with average 12,251 in each species (Figure 1). The therapeutic function category includes 21 unique functional characteristics, such as anti-angiogenic peptide (AAP) (Zhang and Zou, 2020), anti-bacterial peptide (ABP) (Xiao et al., 2021), anti-cancer peptide (ACP) (Agrawal et al., 2021), anti-coronavirus peptide (ACVP) (Pang et al., 2021), and anti-diabetic peptide (ADP) (Roy and Teron, 2019). These peptides were classified into 41 therapeutic peptides categories with different functional characteristics (Table 1). Each species has one or more peptide sequences with distinct functions. Users can retrieve functional peptides specific to the species they are interested in. The length of peptides ranges from 5 to 200 amino acids. Each peptide in MFPPDB contains a maximum of three different therapeutic functions, but the number of peptides with three functions simultaneously is relatively small. Since, anti-bacterial peptides are extremely widespread in nature, as well as a large number of anti-bacterial peptides in the training samples, which resulted in a high number of anti-bacterial peptides in the predicted results (Figure 2). A total of 999,471 entries (67.4% of total) belongs to anti-bacterial peptides followed by anti-fungal peptide with 315,564 entries (21.3% of total identified peptides) (Figure 2). The number of peptides with dual functions, i.e., anti-bacterial and anti-fungal, is 73,373 entries (4.9% of total peptides identified). The database also includes 59,283 entries of anti-viral peptides, and 1.2% of peptides with anti-HIV and anti-viral functions. Additionally, we have also matched the predicted peptides identified in MFPPDB to the functional curated or structurally annotated therapeutic peptide datasets from nine state-of-the-art peptide databases currently accessible, including THPdb (Usmani et al., 2017), AHTPDB (Kumar et al., 2015), CPPsite 2.0 (Agrawal et al., 2016), APD3 (Wang et al., 2016), ACovPepDB (Zhang et al., 2022a), AntiTbPdb (Usmani et al., 2018), PlantPepDB (Das et al., 2020), YADAMP (Piotto et al., 2012) and SATPdb (Singh et al., 2016) (Supplementary Table 1). These databases totally comprise 46,319 peptides distributed in categories such as FDA-approved therapeutic peptides, anti-hypertensive peptides, cell-penetrating peptides, anti-microbial peptides, anti-coronavirus peptides, anti-tubercular peptides, plant-derived peptides (Supplementary Table 1). After matching roughly based on BLASTP (Altschul et al., 1990), we identified a total of 293,408 hits with 75,718 unique predicted plant peptides in MFPPDB against the peptides in the nine databases, indicating that those plant peptides possess the potential functions with the targeted terms in the database (Supplementary Table 2).
Figure 1 The phylogenetic relationship and the number of functional therapeutic peptides categories of plant species in MFPPDB database. The heatmap indicates the number of functional peptides contained in each species.
Table 1 Functional classification of the identified peptides and the dataset source used to train the model in MFPPDB.
Figure 2 All the functional peptide types and corresponding quantities contained in MFPPDB database. We classified the peptide types into four group according to the quantity. (A) The five functional peptides with quantity more than 10,000, (B) The three functional peptides with quantity between 1000 and 10,000, (C) The eight functional peptides with quantity between 100 and 1000, (D) 25 functional peptides with quantity below 100.
We then carried out statistical analyses of the therapeutic peptide data stored in MFPPDB. We found that the top five plant species with most abundant therapeutic functions are Triticum aestivum (99,401 entries), Hordeum vulgare (58,941 entries), Thinopyrum intermedium (47,090 entries), Zea mays (33,732 entries), and Camellia sinensis (30,059 entries). There are more than 20 functional types of peptides were observed in 10 plant species, and only Citrus clementina contains 9 types of therapeutic functional peptides. About 68.5% of the plant species (83 out of total 121 species) contain 12 -16 types of therapeutic peptides. Among them, Vitis vinifera has the highest variety of therapeutic peptides with 26 therapeutic peptides. Both Carica papaya and Triticum aestivum have 24 types of therapeutic plant peptides. While, Brachypodium mexicanum, Musa acuminata, and Oropetium thomaeum have 21 types of functional peptides. Cucumis sativus, Spinacia oleracea, and Trifolium pratense contain 20 functional peptides. The statistics can be found in Supplementary Figure 1. MFPPDB provides researchers with a large variety of therapeutic plant peptides. If researchers want to find therapeutic peptides with multiple functional categories, they can focus on species such as Vitis vinifera and Carica papaya. If users want to obtain a large number of functional peptides in certain species, they can refer to Triticum aestivum and Hordeum vulgare. Depending on specific requirements, the development of functional peptides and peptide drugs can be supported in a targeted manner, thereby contributing to the application of peptide therapy in the medical field.
We developed the MFPPDB database using MySQL, Apache Tomcat web server, Java Spring Boot, and Python Flask tools. The environment required for the development and deployment of the application is integrated into the Tencent Cloud Linux server. Spring Boot is the main development tool for the web backend, while Python Flask is used to quickly deploy a multifunctional plant peptide predictor on the web server. All data constituting the MFPPDB database are stored on the relational database management system MySQL server (version 8.0.31). HTML, CSS, and JavaScript are used for developing web front-end pages, with Bootstrap as the front-end framework. Apache Echarts is used for data visualization, which presents rich multifunctional plant peptide data to users. The architecture of MFPPDB is shown in Figure 3.
Figure 3 The overall architecture of the MFPPDB database. (A) The data resources of MFPPDB database, including public databases such as TPIA, TAIR, RGAP, SGN, IWGSC, and Phytozome. The formed data set is injected with plant peptides with single or multiple functions, such as anti-bacterial peptide, anti-fungal peptide, etc., into the database species through function prediction. (B) The tools used to build the website. The visualized MFPPDB database uses HTML, CSS, JavaScript, Apache ECharts and Bootstrap. The deployment environment and back-end implementation tools are Linux, Apache, MySQL, Spring Boot, and Flask. (C) The main interfaces (such as Browse, Download, Predictor, etc.) and retrieval tools (Function Search and Physicochemical Properties Search) contained in the MFPPDB database.
The search module in MFPPDB provides users with two search modes: functional search and physicochemical property search (Figure 4). With the ‘Functional Search’ module, users can search against each individual plant species for peptides with specific class of functions, e.g., ‘ABP’ or ‘ABP, AHIVP’ in the original predicted datasets or the functional matched datasets. By selecting the species name, MFPPDB ID or the functions in function search, users can easily retrieve the detail information of target functional peptides. On the other hand, the ‘Physicochemical Properties Search’ module allows users to perform complex queries and use conditional operators on different fields, as well as submit queries using Boolean expressions (such as “AND” or “OR”). The fields in this module contain essential functional and physicochemical properties of therapeutic peptides, such as function, isoelectric point, sequence length, instability index, and so on. By utilizing these conditions simultaneously, users can easily find the therapeutic peptides of their interest. To help users obtain the possible functions of the total predicted plant peptides, we also integrated the information of the matched peptides in MFPPDB to other nine databases into the search module, in which users can easily search and retrieve the matched peptides. The output is an additional column in the search page that shows the functional entries in the selected databases.
Figure 4 The MFPPDB database interfaces. (A) Search tools include “Function Search” and “Physicochemical Properties Search”. (B) “Predictor” interface and prediction result page. (C) The “Browse” interface. The figure shows the important information and Physicochemical information contained in the functional peptide taking ‘mfppdb_1’ as an example. (D) Download page for Functional peptide data.
The Browse page is the primary way for users to explore the MFPPDB database and discover peptides with various functional characteristics. Upon accessing this page, the users will be presented with a table containing peptide’s ID, scientific names, molecular weights, functions, and corresponding literature references (PMID and DOI). By clicking on a MFPPDB ID, users can access the detail information of the functional peptide, includes the therapeutic function, peptide sequence, sequence length, isoelectric point, and the description of the peptides matched to functional curated peptides in other nine peptide databases. Additionally, an interface is available for users to access more comprehensive physicochemical properties information for each peptide.
We have also incorporated a user-friendly prediction tool into the web server, which predicts the candidate function of plant peptides. The prediction server can effectively predict functional characteristics of plant peptides in both single and batch mode. Users can input the plant peptide sequence in FASTA format into the provided input box or submit the FASTA file containing the peptide sequence, and then click the submit button to initiate the prediction process. The prediction process only takes a few minutes, and the predicted results are presented to the user in the form of a table on the result page. Users can easily download the predicted results or receive them via email. The prediction algorithm used by the tool is based on the novel multi-label predictor, PrMFTP, which uses deep neural networks and a multi-head self-attention mechanism (Yan et al., 2022). The prediction tool in MFPPDB has been retrained using therapeutic peptides with sequence length between 5 and 200 amino acids following the original algorithm from PrMFTP, ensured the accuracy and reliability.
As a free public functional peptide database, the MFPPDB provides users with a page for downloading all the data used in the database. The Download page is displayed to the user in the form of a table, where they can observe the common name, number of peptides, and download interface corresponding to each species. MFPPDB provides data to users in the Excel format, which contains a lot of information about the current species, such as MFPPDBID, peptide sequence, sequence length, functional characteristics, scientific name, etc. We also provide an interface on this page that allows quick access to the functional peptide information of the current species. Users can simply click on the species to browse the peptide information for the species they interest.
The MFPPDB database is useful for the large number of plant peptides predicted to have multiple therapeutic functions. After the prediction, we also tried to match the possible functional descriptions of those predicted plant peptides to the functional verified or curated peptides in other nine state-of-the-art peptide databases. Indeed, we found at least 20% of the predicted plant peptides show the potential functions as with the hits in the nine databases. However, it should be noted that the matching is still based on peptide sequence alignments, even the matching targets were the experimentally validated or curated peptides. It owns to those excellent developed functional curated databases containing therapeutic peptides, such as THPdb, AHTPDB, CPPsite 2.0, APD3, ACovPepDB, AntiTbPdb, PlantPepDB, YADAMP and SATPdb. These results indicated that the plant peptides in MFPPDB have therapeutic functions in potential despite of the fact that the functional validation need to be experimentally conducted in the future. Among these databases, the PlantPepDB serves a similar function with MFPPDB, in which both databases focus on plant peptides having different functions and therapeutic activities. Compared to the abundant predicted or verified plant peptides in MFPPDB, although PlantPepDB only contains 3,848 peptide entries; however, the peptides in PlantPepDB are manually curated from 11 databases and 835 published research articles. The functions of these peptides might be more detail and accurate than those in MFPPDB. After matching and verifying the predicted peptides with those known manually curated plant peptides in PlantPepDB and other databases, we believe that these predicted and verified plant peptides in MFPPDB are also the important sources for therapeutic usage.
In the current version of MFPPDB, we have included a total of 1,482,409 peptides, in which 293,408 were potentially verified with therapeutic functions collected from the representative plant species in nature. In the further version of MFPPDB, we plan to expand our collection to include more categories of plants, such as bryophytes, ferns, gymnosperms, etc. We also aim to obtain more properties of the peptide sequences, such as their secondary and tertiary structures, and to add other effective computational tools to the MFPPDB. More importantly, we will also keep trying to verify or validate the functions of the predicted plant peptides either computationally or experimentally, thus providing more comprehensive and accurate information to assist biologists in their scientific research.
The multifunctional plant peptide database consists of plants from monocots and dicots of 121 species across 98 genera of angiosperms. The database includes peptide sequences from model plants such as Arabidopsis (from the TAIR database), rice (from the Rice Genome Annotation Project), Solanum lycopersicum (from the Solanaceae Genomics Network), Solanum tuberosum (from SpudDB), Triticum aestivum (from the International Wheat Genome Sequencing Consortium), and Camellia sinensis (from the TPIA database). The peptide sequences of the other 115 angiosperms were obtained from Phytozome (https://phytozome-next.jgi.doe.gov/).
After obtaining the original plant peptide sequences from multiple public databases, we performed preprocessing to eliminate non-compliant peptide sequences. Firstly, we discarded plant peptides containing non-standard amino acids in their sequences. Secondly, we removed plant peptide sequences containing repetitive amino acids. Lastly, we extracted plant peptides with sequence lengths between 5 and 200 amino acids. Subsequently, the peptide sequences were input into the function prediction model to identify plant peptides with multiple therapeutic functions, which were then included in the MFPPDB database as significant data. Matching of the predicted plant peptides from MFPPDB to other curated datasets from nine public databases were conducted using BLASTP (Altschul et al., 1990) with E-value less than 1E-3 and identity greater than 50%.
All the identified peptides in the MFPPDB library were carefully analyzed using computational tools to calculate the physicochemical properties of each functional peptide from the peptide sequence input. The atomic composition of peptides includes carbon, hydrogen, oxygen, nitrogen, and sulfur were calculated using the Proteomics Toolkit (http://db.systemsbiology.net/proteomicsToolkit/FragIonServlet.html). We obtained the source code on Github and ran it in the Java interpretation environment. The results of the operation output the atomic composition information of the peptide in the form of a table. We also used the “BioSeqUtils” module provided by Biopython (Cock et al., 2009) and imported the ProtParam program to analyze various attributes of plant peptide sequences with multiple therapeutic functions, such as the number of amino acids, amino acid percentages, isoelectric points, molecular weight, hydrophilicity (GRAVY) index, instability index, aromaticity, secondary structure fraction, and atomic composition. The number of positively and negatively charged residues was calculated based on the previously calculated amino acid counts.
In current report, we establishment the MFPPDB database, enables the extraction of therapeutic peptide information scattered across various plant species using a novel multi-functional therapeutic peptides prediction tool— PrMFTP. Based on those well predicted and pre-functional verified peptides, we integrate it into a comprehensive resource library and presenting the detail information or characteristics of the peptides to researchers in an intuitive way on current MFPPDB version. It greatly reduces the time and energy wasted in collecting data for bioinformatics researchers when exploring plant peptides or proteins of a certain species, allowing them to focus more on verifying the function of peptides from current database and by additional experimental methods. We believe that the development of MFPPDB will accelerate research on plant peptides with therapeutic functions.
The original contributions presented in the study are included in the article/Supplementary Material. Further inquiries can be directed to the corresponding authors.
KL and WT conceived the study and supervised all parts of the project. YY and HW collected samples and sequence data. YY, HW, and YG analyzed the data and constructed the database. YY, KL, and WT wrote and revised the manuscript. All authors contributed to the article and approved the submitted version.
This work was supported by the Open Fund of Information Materials and Intelligent Sensing Laboratory of Anhui Province (No. IMIS202009), the National Natural Science Foundation of China (Nos. 32002086 and 32172626), the Natural Science Research Project of University in Anhui Province (No. KJ2021A0182 and No. 2022AH050867), the Anhui Agricultural University Introduction and Stabilization of Talents Research Funding (No. yj2020-74), and The University Synergy Innovation Program of Anhui Province (No. GXXT-2022-041).
The authors declare that the research was conducted in the absence of any commercial or financial relationships that could be construed as a potential conflict of interest.
All claims expressed in this article are solely those of the authors and do not necessarily represent those of their affiliated organizations, or those of the publisher, the editors and the reviewers. Any product that may be evaluated in this article, or claim that may be made by its manufacturer, is not guaranteed or endorsed by the publisher.
The Supplementary Material for this article can be found online at: https://www.frontiersin.org/articles/10.3389/fpls.2023.1224394/full#supplementary-material
SUPPLEMENTARY FIGURE 1 | The number of functional peptides contained in each species.
Agrawal, P., Bhagat, D., Mahalwal, M., Sharma, N., Raghava, G. P. (2021). AntiCP 2.0: an updated model for predicting anticancer peptides. Briefings Bioinf. 22 (3), bbaa153. doi: 10.1093/bib/bbaa153
Agrawal, P., Bhalla, S., Usmani, S. S., Singh, S., Chaudhary, K., Raghava, G. P., et al. (2016). CPPsite 2.0: a repository of experimentally validated cell-penetrating peptides. Nucleic Acids Res. 44 (D1), D1098–D1103. doi: 10.1093/nar/gkv1266
Altschul, S. F., Gish, W., Miller, W., Myers, E. W., Lipman, D. J. (1990). Basic local alignment search tool. J. Mol. Biol. 215 (3), 403–410. doi: 10.1016/S0022-2836(05)80360-2
Chai, T.-T., Ee, K.-Y., Kumar, D. T., Manan, F. A., Wong, F.-C. (2021). Plant bioactive peptides: Current status and prospects towards use on human health. Protein Pept. Lett. 28 (6), 623–642. doi: 10.2174/0929866527999201211195936
Charoenkwan, P., Kanthawong, S., Nantasenamat, C., Hasan, M. M., Shoombuatong, W. (2020). iDPPIV-SCM: a sequence-based predictor for identifying and analyzing dipeptidyl peptidase IV (DPP-IV) inhibitory peptides using a scoring card method. J. Proteome Res. 19 (10), 4125–4136. doi: 10.1021/acs.jproteome.0c00590
Cheng, H., Yuan, J., Pei, C., Ouyang, M., Bu, H., Chen, Y., et al. (2023). The development of an anti-cancer peptide M1-21 targeting transcription factor FOXM1. Cell Bioscience 13 (1), 1–16. doi: 10.1186/s13578-023-01059-7
Cock, P. J., Antao, T., Chang, J. T., Chapman, B. A., Cox, C. J., Dalke, A., et al. (2009). Biopython: freely available Python tools for computational molecular biology and bioinformatics. Bioinformatics 25 (11), 1422. doi: 10.1093/bioinformatics/btp163
Consortium, I. W. G. S., Mayer, K. F., Rogers, J., Doležel, J., Pozniak, C., Eversole, K., et al. (2014). A chromosome-based draft sequence of the hexaploid bread wheat (Triticum aestivum) genome. Science 345 (6194), 1251788. doi: 10.1126/science.1251788
Dai, R., Zhang, W., Tang, W., Wynendaele, E., Zhu, Q., Bin, Y., et al. (2021). BBPpred: sequence-based prediction of blood-brain barrier peptides with feature representation learning and logistic regression. J. Chem. Inf Model. 61 (1), 525–534. doi: 10.1021/acs.jcim.0c01115
Das, D., Jaiswal, M., Khan, F. N., Ahamad, S., Kumar, S. (2020). PlantPepDB: A manually curated plant peptide database. Sci. Rep. 10 (1), 2194. doi: 10.1038/s41598-020-59165-2
Elbrønd, B., Jakobsen, G., Larsen, S., Agersø, H., Jensen, L. B., Rolan, P., et al. (2002). Pharmacokinetics, pharmacodynamics, safety, and tolerability of a single-dose of NN2211, a long-acting glucagon-like peptide 1 derivative, in healthy male subjects. Diabetes Care 25 (8), 1398–1404. doi: 10.2337/diacare.25.8.1398
Fallah Atanaki, F., Behrouzi, S., Ariaeenejad, S., Boroomand, A., Kavousi, K. (2020). BIPEP: Sequence-based prediction of biofilm inhibitory peptides using a combination of nmr and physicochemical descriptors. ACS omega 5 (13), 7290–7297. doi: 10.1021/acsomega.9b04119
Fang, C., Moriwaki, Y., Li, C., Shimizu, K. (2019). Prediction of antifungal peptides by deep learning with character embedding. IPSJ Trans. Bioinf. 12, 21–29. doi: 10.2197/ipsjtbio.12.21
Goodstein, D. M., Shu, S., Howson, R., Neupane, R., Hayes, R. D., Fazo, J., et al. (2012). Phytozome: a comparative platform for green plant genomics. Nucleic Acids Res. 40 (D1), D1178–D1186. doi: 10.1093/nar/gkr944
Hamano, N., Komaba, H., Fukagawa, M. (2017). Etelcalcetide for the treatment of secondary hyperparathyroidism. Expert Opin Pharmacother. 18 (5), 529–534. doi: 10.1080/14656566.2017.1303482
Hammami, R., Ben Hamida, J., Vergoten, G., Fliss, I. (2009). PhytAMP: a database dedicated to antimicrobial plant peptides. Nucleic Acids Res. 37 (Database), D963–D968. doi: 10.1093/nar/gkn655
Henninot, A., Collins, J. C., Nuss, J. M. (2018). The current state of peptide drug discovery: back to the future? J. medicinal Chem. 61 (4), 1382–1414. doi: 10.1021/acs.jmedchem.7b00318
Iwaniak, A., Minkiewicz, P., Darewicz, M., Sieniawski, K., Starowicz, P. (2016). BIOPEP database of sensory peptides and amino acids. Food Res. Int. 85, 155–161. doi: 10.1016/j.foodres.2016.04.031
Jain, P., Tiwari, A. K., Som, T. (2021). Enhanced prediction of anti-tubercular peptides from sequence information using divergence measure-based intuitionistic fuzzy-rough feature selection. Soft Computing 25, 3065–3086. doi: 10.1007/s00500-020-05363-z
Kawahara, Y., de la Bastide, M., Hamilton, J. P., Kanamori, H., McCombie, W. R., Ouyang, S., et al. (2013). Improvement of the Oryza sativa Nipponbare reference genome using next generation sequence and optical map data. Rice 6, 1–10. doi: 10.1186/1939-8433-6-4
Khatun, M. S., Hasan, M. M., Shoombuatong, W., Kurata, H. (2020). ProIn-Fuse: improved and robust prediction of proinflammatory peptides by fusing of multiple feature representations. J. Computer-Aided Mol. Design 34, 1229–1236. doi: 10.1007/s10822-020-00343-9
Kim, H., Jang, J. H., Kim, S. C., Cho, J. H. (2014). De novo generation of short antimicrobial peptides with enhanced stability and cell specificity. J. Antimicrobial Chemotherapy 69 (1), 121–132. doi: 10.1093/jac/dkt322
Kong, X.-D., Moriya, J., Carle, V., Pojer, F., Abriata, L. A., Deyle, K., et al. (2020). De novo development of proteolytically resistant therapeutic peptides for oral administration. Nat. Biomed. Eng. 4 (5), 560–571. doi: 10.1038/s41551-020-0556-3
Kumar, R., Chaudhary, K., Sharma, M., Nagpal, G., Chauhan, J. S., Singh, S., et al. (2015). AHTPDB: a comprehensive platform for analysis and presentation of antihypertensive peptides. Nucleic Acids Res. 43 (D1), D956–D962. doi: 10.1093/nar/gku1141
Lamesch, P., Berardini, T. Z., Li, D., Swarbreck, D., Wilks, C., Sasidharan, R., et al. (2012). The Arabidopsis Information Resource (TAIR): improved gene annotation and new tools. Nucleic Acids Res. 40 (D1), D1202–D1210. doi: 10.1093/nar/gkr1090
Lau, J. L., Dunn, M. K. (2018). Therapeutic peptides: Historical perspectives, current development trends, and future directions. Bioorganic medicinal Chem. 26 (10), 2700–2707. doi: 10.1016/j.bmc.2017.06.052
Muttenthaler, M., King, G. F., Adams, D. J., Alewood, P. F. (2021). Trends in peptide drug discovery. Nat. Rev. Drug Discovery 20 (4), 309–325. doi: 10.1038/s41573-020-00135-8
Pang, Y., Wang, Z., Jhong, J.-H., Lee, T.-Y. (2021). Identifying anti-coronavirus peptides by incorporating different negative datasets and imbalanced learning strategies. Briefings Bioinf. 22 (2), 1085–1095. doi: 10.1093/bib/bbaa423
Pham, G. M., Hamilton, J. P., Wood, J. C., Burke, J. T., Zhao, H., Vaillancourt, B., et al. (2020). Construction of a chromosome-scale long-read reference genome assembly for potato. Gigascience 9 (9), giaa100. doi: 10.1093/gigascience/giaa100
Piotto, S. P., Sessa, L., Concilio, S., Iannelli, P. (2012). YADAMP: yet another database of antimicrobial peptides. Int. J. Antimicrobial Agents 39 (4), 346–351. doi: 10.1016/j.ijantimicag.2011.12.003
Ramírez-Sánchez, O., Pérez-Rodríguez, P., Delaye, L., Tiessen, A. (2016). Plant proteins are smaller because they are encoded by fewer exons than animal proteins. Genomics Proteomics Bioinf. 14 (6), 357–370. doi: 10.1016/j.gpb.2016.06.003
Roy, S., Teron, R. (2019). BioDADPep: A Bioinformatics database for anti diabetic peptides. Bioinformation 15 (11), 780–783. doi: 10.6026/97320630015780
Sarethy, I. P. (2017). Plant peptides: bioactivity, opportunities and challenges. Protein Pept. Lett. 24 (2), 102–108. doi: 10.2174/0929866523666161220113632
Seshadri Sundararajan, V., Gabere, M. N., Pretorius, A., Adam, S., Christoffels, A., Lehväslaiho, M., et al. (2012). DAMPD: a manually curated antimicrobial peptide database. Nucleic Acids Res. 40 (D1), D1108–D1112. doi: 10.1093/nar/gkr1063
Shoombuatong, W., SChaduangrat, N., Pratiwi, R., Nantasenamat, C. (2019). THPep: a machine learning-based approach for predicting tumor homing peptides. Comput. Biol. Chem. 80, 441–451. doi: 10.1016/j.compbiolchem.2019.05.008
Singh, S., Chaudhary, K., Dhanda, S. K., Bhalla, S., Usmani, S. S., Gautam, A., et al. (2016). SATPdb: a database of structurally annotated therapeutic peptides. Nucleic Acids Res. 44 (D1), D1119–D1126. doi: 10.1093/nar/gkv1114
Tyagi, A., Pankaj, V., Singh, S., Roy, S., Semwal, M., Shasany, A. K., et al. (2019). PlantAFP: a curated database of plant-origin antifungal peptides. Amino Acids 51 (10-12), 1561–1568. doi: 10.1007/s00726-019-02792-5
Tyagi, A., Tuknait, A., Anand, P., Gupta, S., Sharma, M., Mathur, D., et al. (2015). CancerPPD: a database of anticancer peptides and proteins. Nucleic Acids Res. 43 (D1), D837–D843. doi: 10.1093/nar/gku892
Usmani, S. S., Bedi, G., Samuel, J. S., Singh, S., Kalra, S., Kumar, P., et al. (2017). THPdb: Database of FDA-approved peptide and protein therapeutics. PLoS One 12 (7), e0181748. doi: 10.1371/journal.pone.0181748
Usmani, S. S., Kumar, R., Kumar, V., Singh, S., Raghava, G. P. (2018). AntiTbPdb: a knowledgebase of anti-tubercular peptides. Database 2018, bay025. doi: 10.1093/database/bay025
Wang, G., Li, X., Wang, Z. (2016). APD3: the antimicrobial peptide database as a tool for research and education. Nucleic Acids Res. 44 (D1), D1087–D1093. doi: 10.1093/nar/gkv1278
Wang, L., Wang, N., Zhang, W., Cheng, X., Yan, Z., Shao, G., et al. (2022). Therapeutic peptides: Current applications and future directions. Signal Transduction Targeted Ther. 7 (1), 48. doi: 10.1038/s41392-022-00904-4
Xia, E. H., Li, F. D., Tong, W., Li, P. H., Wu, Q., Zhao, H. J., et al. (2019). Tea Plant Information Archive: a comprehensive genomics and bioinformatics platform for tea plant. Plant Biotechnol. J. 17 (10), 1938–1953. doi: 10.1111/pbi.13111
Xiao, X., Shao, Y.-T., Cheng, X., Stamatovic, B. (2021). iAMP-CA2L: a new CNN-BiLSTM-SVM classifier based on cellular automata image for identifying antimicrobial peptides and their functional types. Briefings Bioinf. 22 (6), bbab209. doi: 10.1093/bib/bbab209
Xu, D., Wu, Y., Cheng, Z., Yang, J., Ding, Y. (2021). ACHP: a web server for predicting anti-cancer peptide and anti-hypertensive peptide. Int. J. Pept. Res. Ther. 27 (3), 1933–1944. doi: 10.1007/s10989-021-10222-y
Yan, W., Tang, W., Wang, L., Bin, Y., Xia, J. (2022). PrMFTP: Multi-functional therapeutic peptides prediction based on multi-head self-attention mechanism and class weight optimization. PloS Comput. Biol. 18 (9), e1010511. doi: 10.1371/journal.pcbi.1010511
Zhang, H., Chen, S. (2021). Cyclic peptide drugs approved in the last two decades (2001-2021). RSC Chem. Biol. 3 (1), 18–31. doi: 10.1039/d1cb00154j
Zhang, Q., Chen, X., Li, B., Lu, C., Yang, S., Long, J., et al. (2022a). A database of anti-coronavirus peptides. Sci. Data 9 (1), 294. doi: 10.1038/s41597-022-01394-3
Zhang, W., Xia, E., Dai, R., Tang, W., Bin, Y., Xia, J. (2022b). PredAPP: predicting anti-parasitic peptides with undersampling and ensemble approaches. Interdiscip Sci. 14 (1), 258–268. doi: 10.1007/s12539-021-00484-x
Zhang, Y. P., Zou, Q. (2020). PPTPP: a novel therapeutic peptide prediction method using physicochemical property encoding and adaptive feature representation learning. Bioinformatics 36 (13), 3982–3987. doi: 10.1093/bioinformatics/btaa275
Keywords: multi-functional, therapeutic peptide, plant, database, peptidomes
Citation: Yang Y, Wu H, Gao Y, Tong W and Li K (2023) MFPPDB: a comprehensive multi-functional plant peptide database. Front. Plant Sci. 14:1224394. doi: 10.3389/fpls.2023.1224394
Received: 17 May 2023; Accepted: 29 September 2023;
Published: 16 October 2023.
Edited by:
Linchun Shi, Chinese Academy of Medical Sciences and Peking Union Medical College, ChinaReviewed by:
Salman Sadullah Usmani, Albert Einstein College of Medicine, United StatesCopyright © 2023 Yang, Wu, Gao, Tong and Li. This is an open-access article distributed under the terms of the Creative Commons Attribution License (CC BY). The use, distribution or reproduction in other forums is permitted, provided the original author(s) and the copyright owner(s) are credited and that the original publication in this journal is cited, in accordance with accepted academic practice. No use, distribution or reproduction is permitted which does not comply with these terms.
*Correspondence: Ke Li, bGlrZUBhaGF1LmVkdS5jbg==; Wei Tong, d3RvbmdAYWhhdS5lZHUuY24=
Disclaimer: All claims expressed in this article are solely those of the authors and do not necessarily represent those of their affiliated organizations, or those of the publisher, the editors and the reviewers. Any product that may be evaluated in this article or claim that may be made by its manufacturer is not guaranteed or endorsed by the publisher.
Research integrity at Frontiers
Learn more about the work of our research integrity team to safeguard the quality of each article we publish.