- 1Rice Research Institute, Anhui Academy of Agricultural Sciences, Hefei, China
- 2College of Agriculture, Anhui Science and Technology University, Fengyang, China
- 3Key Laboratory of Rice Genetics and Breeding in Anhui Province, Hefei, China
Rice drought resistance is a complicated quantitative feature involving a range of biological and agronomic variables, but little is known about the underlying genetics and regulatory mechanisms that regulate drought tolerance. This study used 120 recombinant inbred lines (RILs), derived from a cross between drought tolerant Lvhan 1 and susceptible Aixian 1. The RILs were subjected to drought stress at the first ear stage, and phenotypic data of 16 agronomic and physiological traits under varying conditions were investigated. Genome-wide association study (GWAS) on the drought resistance index of traits was carried out. A total of 9 quantitative trait loci (QTLs) associated with drought-related traits were identified on chromosomes 2, 6, 7, 8, 9, and 10, which includes QTLs for plant height (PH) qPH10.1, effective panicles number (EPN) qEPN6.1, panicle length (PL) qPL9.1, thousand-grain weight (TGW) qTGW2.1, qTGW6.1, qTGW8.1, leaf length (LL) qLL7.1, leaf width (LW) qLW7.1, and leaf area (LA) qLA7.1. The fraction of phenotypic variation explained by individual QTL varied from 10.6% to 13.9%. Except for days to flowering (DTF), the mean values of all traits under normal water management conditions were considerably higher than those under drought conditions. Except for the DTF, the drought resistance index of all rice traits was less than 1, indicating that drought treatment reduced the EPN, FGPP, SSR, PH, and LA, which affected the growth and development of rice. The drought resistance index of DTF was 1.02, indicating that drought prolonged the heading time of rice and diminish the yield parameters. Along with identifying QTLs, the results also predicted ten candidate genes, which are directly or indirectly involved in various metabolic functioning related to drought stress. The identification of these genomic sites or QTLs that effectively respond to water scarcity will aid in the quest of understanding the drought tolerance mechanisms. This study will facilitate the marker-assisted rice breeding and handy in the breeding of drought-tolerant rice varieties.
1 Introduction
Rice (Oryza sativa L.) is an important grain crop, and water is crucial for maintaining optimal growth and development. It has been estimated that about 3000 liters of irrigation is needed to produce 1 kg of rice grains (Oladosu et al., 2019). However, in recent years, global warming, water scarcity, and frequent seasonal drought spells seriously restrict the development of China’s agriculture (Hassan et al., 2021). Rice’s development, growth, and physiological processes were severely hampered by drought (Kumar et al., 2017; Ikmal et al., 2019; Yang et al., 2021). According to Dixit et al. (2017) it is imperative to investigate the potential to improve rice productivity in facing the challenge of limited water resources. It is the need of the hour to develop rice cultivars that can withstand drought stress without compromising their yield potential (Wang & Qin, 2017). Multiple genes control rice drought tolerance. Combining rice drought tolerance gene mining with molecular marker-assisted breeding technology is beneficial for developing rice varieties resistant to drought, increasing rice yield, conserving water, and preserving national food security.
The ability of rice to withstand drought is a complicated quantitative feature regulated by numerous quantitative trait loci (QTLs). Although researchers have discovered thousands of QTLS, few have been successfully combined with breeding (Liang et al., 2021; Wang et al., 2021). Traditional QTL mapping techniques are incapable of locating genes properly and efficiently. Genome-wide association study (GWAS) has become a popular tool for breeding rice with the advancement of biotechnology. The basis of GWAS is the population’s linkage disequilibrium (LD) and single nucleotide polymorphisms (SNPs) in the genome. An analytical method that combines population structure, genome-wide LD level, and phenotypic data to identify the relationship between target traits and genetic markers/candidate genes within a population. Its advantages include high accuracy, rapid processing, and no construction of population-based mapping. GWAS provides an efficient method and approach for studying the genetic mechanism of rice drought resistance and mining potential drought resistance genes (Li et al., 2017; Bhandari et al., 2020). Wang et al. (2020) performed GWAS investigation on 272 indica materials, analyzed source-sink relationships and yield-related variables, and identified 70 QTLs influencing 11 related traits. Guo et al. (2018) used 507 diverse rice varieties to conduct a genome-wide association analysis on 51 image qualities and traditional parameters like green holding and yield, and they discovered 470 loci related to drought resistance. Additionally, employing RIL populations for GWAS and linkage analysis, 69 image trait association loci were also found. It has also been proved that some image traits and related genes can be used for drought resistance improvement in the field. Ma et al. (2016) used GWAS to find 29 QTLS related to plant height, yield, and drought resistance index in 270 cultivars. He also found a candidate gene for drought resistance, OsRLK5, vital in increasing rice productivity under drought stress. The GWAS based on deep sequencing is useful for detecting genetic variation in rice drought resistance enhancement. Several genes for rice drought resistance have been cloned and examined recently, including OsMYB6 (Tang et al., 2019), DROT1 (Sun et al., 2022), and OsRINGzf1 (Chen et al., 2022), which have demonstrated positive benefits in controlling rice drought tolerance. However, they have not been utilized in developing new, drought-resistant rice cultivars.
In brief, GWAS has been employed to research rice attributes linked to drought resistance and to examine the genetic basis of rice drought resistance. This study constructed a recombinant inbred line population of indica rice line Lvhan 1 and drought-sensitive japonica strain Aixian 1, which had been verified in production. The population’s agronomic, physiological, and other relevant traits were examined under various water stress conditions, and GWAS was used to identify the main effect of QTLs associated with drought resistance. The results of this study provided a foundation for breeding and enhancing high-yielding and high-quality rice cultivars that are drought-resistant.
2 Material and methods
2.1 Test materials and location
The experiment was conducted in 2021 at the Lujiang Base of the Rice Research Institute of Anhui Academy of Agricultural Sciences, using the recombinant inbred line population of drought-tolerant rice Lvhan 1 and drought-sensitive rice Aixian 1, including 120 lines with significant differences in drought resistance of F10.
2.2 Test methods
2.2.1 Experiment design
Conventional and drought water treatments were designed for the experiment and planted in a mobile greenhouse and an open-air field. The experiment was conducted using a randomized complete block design (RCBD). The rice seeds were sown on May 30, 2021, and transplanted on June 22, 2021. Each plot had three rows with ten plants each, with a plant and row spacing of 20 cm and 26 cm, respectively. Each water treatment was replicated three times. The open-air field is managed according to daily field management; the drought management field is 1 m higher than the ground and covered with portable greenhouses, which are managed in the open air on sunny and rainy days. The soil water potential is maintained at -15 kPa ~ 0 kPa. When the soil water potential is lower than -15 kPa, immediately supplementary irrigation is applied, and irrigation is stopped at the first ear stage of rice and subjected to drought stress. When the leaf wilting reached 50%, or the soil water potential dropped below -50 kPa for more than five days, irrigation was resumed until harvesting maturity. Table 1 shows the weather conditions during the course of the experiment.
2.2.2 Investigation of agronomic traits
The following agronomic traits were investigated:
(1) Tiller number (TN): Five plants were chosen as observation points in every treatment plot. The tiller dynamics were investigated, and the effective tiller number was recorded at the heading stage.
(2) Days to flowering (DTF): The number of days from sowing to flowering was counted.
(3) Agronomic traits of leaves: After one week of drought treatment, each plot’s middle row was chosen to determine chlorophyll content (CC). The CC values in the upper, middle, and base parts of the uppermost three main stem leaves were measured by handheld chlorophyll SPAD apparatus, and the average value was calculated. Leaf length (LL), leaf width (LW), leaf area (LA), and leaf aspect ratio (LAR) were measured by the YMJ-A leaf area measuring instrument.
(4) Yield traits: Five plants were selected from each plot in each treatment at harvesting maturity to examine traits associated with yield, such as plant height (PH), effective panicles number (EPN), panicle length (PL), total grains per panicle (GPP), filled grains per panicle (FGPP), seed setting rate (SSR), thousand-grain weight (TGW), grain yield plant (GYP), and aboveground biomass (AB) which was measured by drying individual plants at 80°C till constant weight. Supplementary File 1 shows the raw data of all above mentioned phenotypic traits.
2.2.3 Calculation of drought resistance index
The drought-resistance index (DRI) was calculated using the following formula.
DRI = [measured value under drought stress/measured value under normal irrigation] ×100%.
The drought-resistance index of all traits was calculated for association analysis.
2.2.4 Genotype identification
The population genotype identification was carried out by Huazhi biotechnology limited company. For this, a random leaf sample from one plant was collected for each RIL to extract DNA. Repeated PCR amplifications were performed using the Huazhi 1K rice SNP chip. The amplified fragments were obtained and constructed with a sequencing kit from Beijing Genomics Institution (BGI) and sequenced in the BGI MGISEQ-T7 sequencing machine. All experiments were conducted following the standard procedures of the sequencing kit. Supplementary File 2 shows the raw data of genotypes in each RIL.
2.3 Data analysis
Excel 2018 and SPSS 26 were used for trait description and correlation analysis. The sequencing data were compared with the reference genome of Nipponbare to screen SNPs with polymorphism among parents. The correlation analysis combined the SNP marker obtained and the drought resistance index of drought resistance-related traits. The genotype data was filtered by TASSEL (v5.2.24) software first, removing the heterozygote sites, the deletion rate was less than 10%, and the genotype frequency was set to be 0.05-1.0. A total of 3550 high-quality SNP markers were selected from 5429 SNPS, and association analysis was performed using a mixed linear model(MLM)combined with Scaled IBS Kinship, genotype, and phenotypic data. The corresponding value of the observed log10p is used as the P value to plot the Q-Q scatter plot and the Manhattan plot. For the interval with a p-value less than 0.001, QTL was considered to exist in the region. The phenotypic contribution rate (R2) was used to evaluate the overall contribution rate of associated QTL to the drought resistance index of phenotypic traits. Refer to the reference for QTL naming principles (Yonemaru et al., 2010).
3 Results and analysis
3.1 Genetic analysis of population traits
The drought-resistance index of each agronomic character of Lvhan 1 and Aixian 1 was significantly different. The drought resistance index of each character of Aixian 1 was mostly lower than that of Lvhan 1 (Figures 1, 2). Sixteen drought-resistant agronomic traits, including EPN, FGPP, SSR, PH, and LA of the RIL population, showed normal distribution, and most of the traits showed right skew and peak state. The PL is left skew, low peak state. The SSR, TGW, and CC are left skewed and in a peak state, and the data are relatively centralized. The characters with high coefficient of variation were GYP (55.18), FGPP (51.18), LA (44.17), TN (38.4), LL (37.32) and EPN (36.76). The phenotypic values of the traits showed a large range and bidirectional transgressive segregation (Table 2), indicating that these drought-related traits were quantitative traits controlled by multiple genes and suitable for QTL mapping. Except for the DTF, the drought resistance index of all rice traits was less than 1, indicating that drought treatment reduced the EPN, FGPP, SSR, PH, and LA, which affected the growth and development of rice. The drought resistance index of DTF was 1.02, indicating that drought prolonged the heading time of rice.
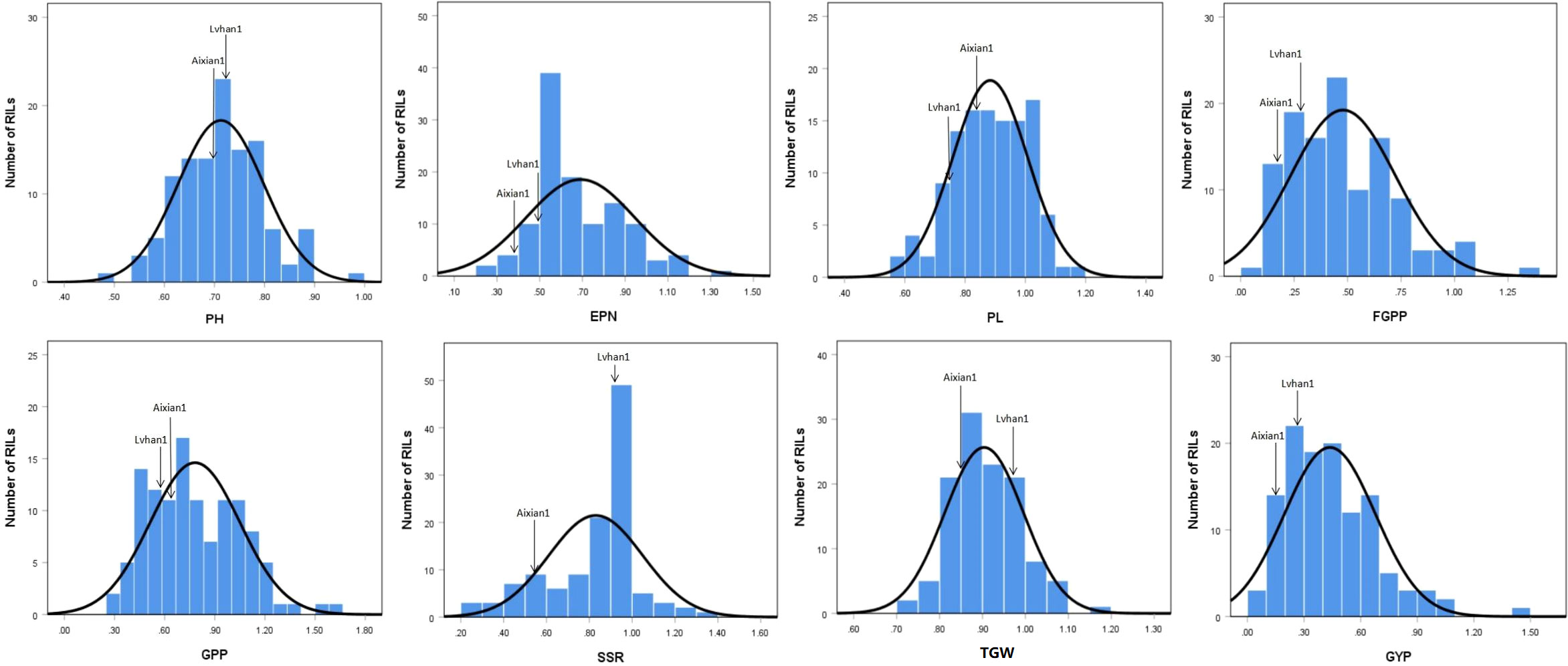
Figure 1 Histogram of the drought-resistance index of drought-related traits in the RIL population. PH=plant height, EPN=effective panicles number, PL=panicle length, FGPP=filled grains per panicle, GPP=grains per panicle, SSR=seed setting rate, TGW= thousand-grain weight, GYP=grain yield plant.
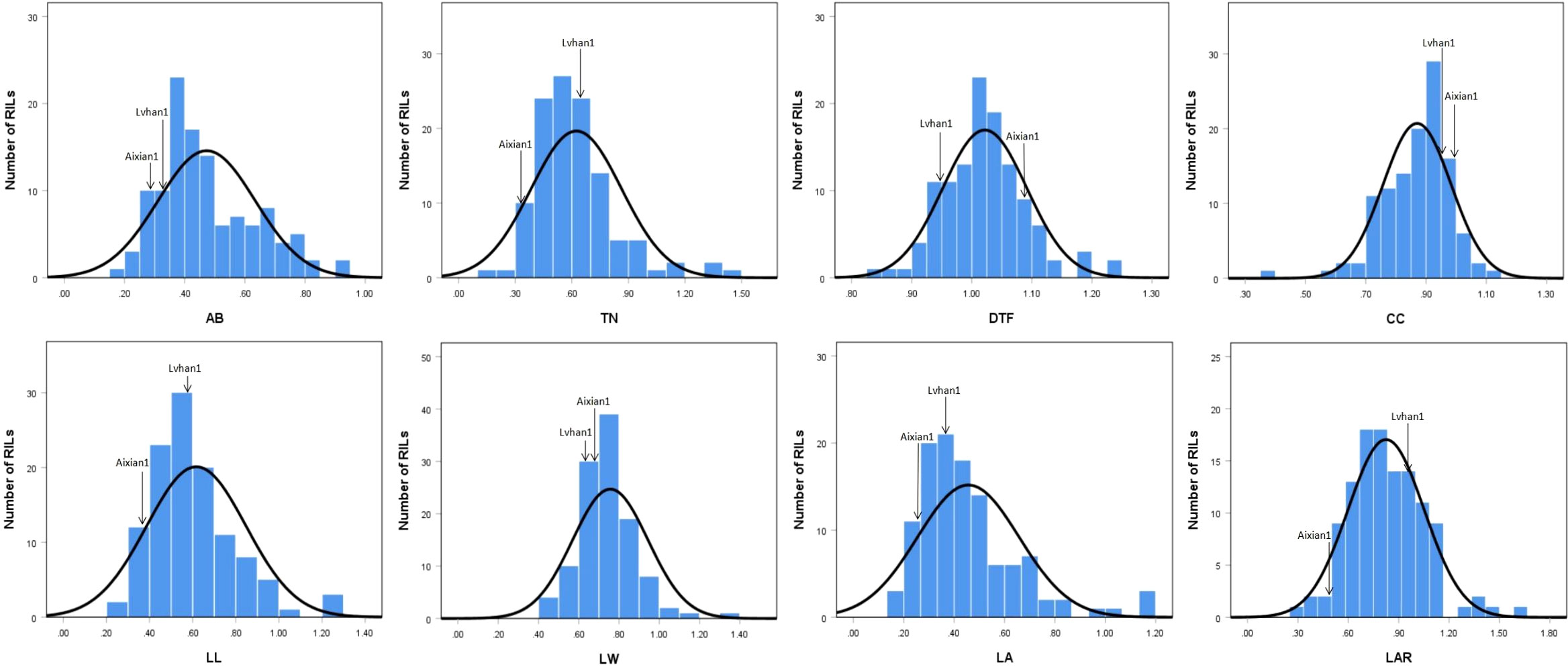
Figure 2 Histogram of the drought-resistance index of drought-related traits in the RIL population AB, aboveground biomass; TN, tiller number; DTF, days to flowering; CC, chlorophyll content; LL, leaf length; LW, leaf width; LA, leaf area; LAR, leaf aspect ratio.
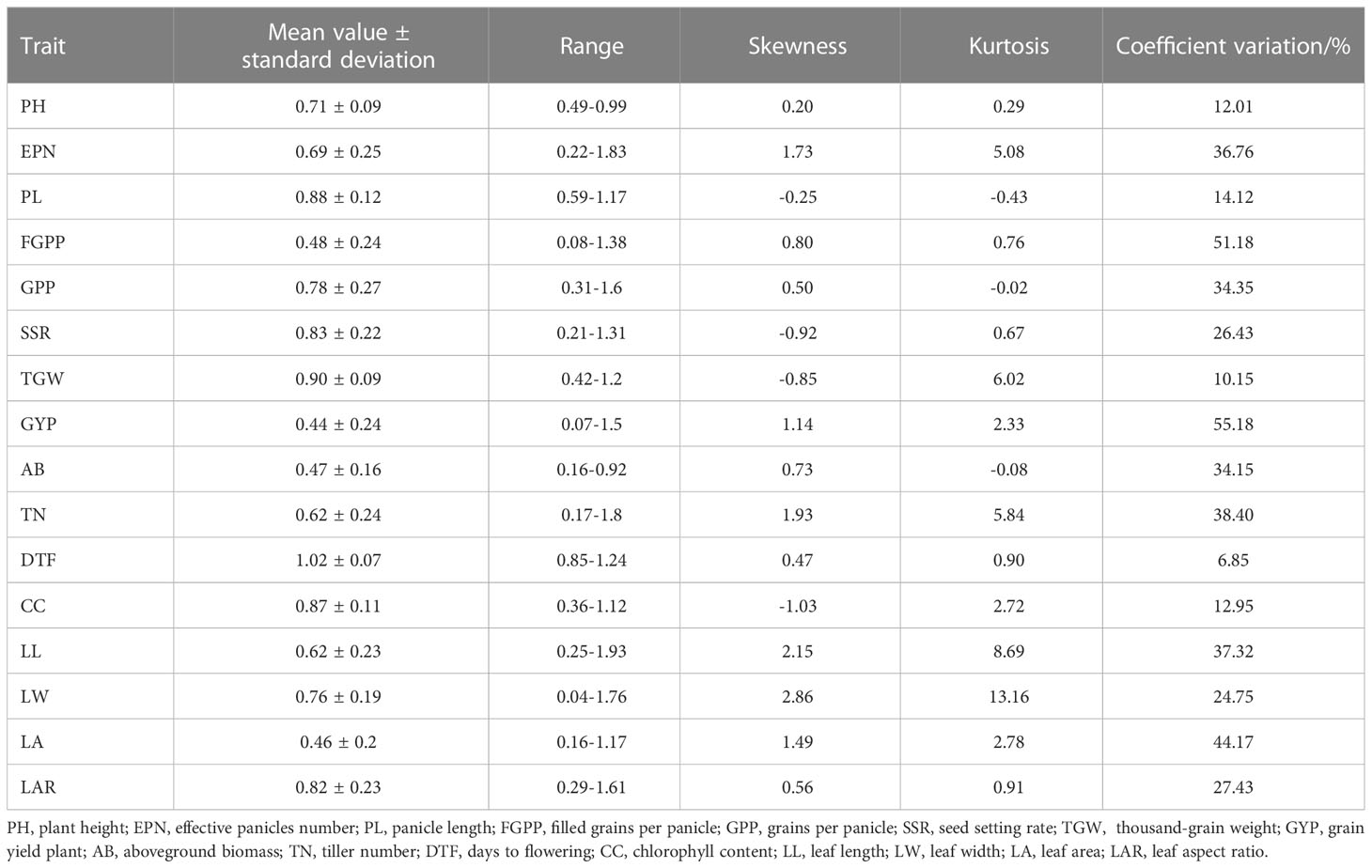
Table 2 Statistical analysis of the drought-resistance index of measured traits in the RIL population.
3.2 Correlation analysis
By analyzing the correlation of the drought resistance index of various agronomic and yield traits of rice, it was found that PL, FGPP, GPP, SSR, TGW, AB, CC, and PH showed a significant positive correlation (Table 3), indicating that various traits of rice plant affected drought resistance. A highly significant correlation existed between the number of FGPP and PH, EPN, PL, GPP, SSR, GYP, AB, and CC. The correlation coefficient between FGPP and GYP was the highest (0.981), followed by AB (0.795). The correlation between GPP and TGW achieved significant levels; the correlation coefficient was 0.211. There were highly significant correlations between GYP and yield-related traits. CC was significantly correlated with PH, PL, FGPP, GPP and SSR, and AB and DTF. There was a negative correlation between the DTF and EPN. LL was positively correlated with LW, leaf area, and LAR.
3.3 Statistical analysis of SNP markers
The 5429 SNP loci were obtained by sequencing, and 3550 SNP loci were obtained by screening according to the deletion rate of less than 10% for GWAS analysis. SNP markers were evenly distributed on rice chromosomes, and the total length of the genome was 364.86 Mb, among which chromosome 1 was the longest (42.3 Mb) and chromosome 9 was the shortest (21.82 Mb). The distribution information of SNP markers on chromosomes of the whole genome is shown in Table 4.
3.4 Genome-wide association study
The TASSEL software was used to conduct a genome-wide association study on the drought-resistance index of 16 traits in the RIL population and the selected marker loci. As can be seen from the Q-Q diagram in Figure 3, when the abscissa was greater than 1.5, there was a significant difference between the P value of the GWAS result and the theoretical P value, indicating that there was indeed a significant correlation between phenotype and genotype. The Q-Q diagram illustrates that the model observations are close to the anticipated values.
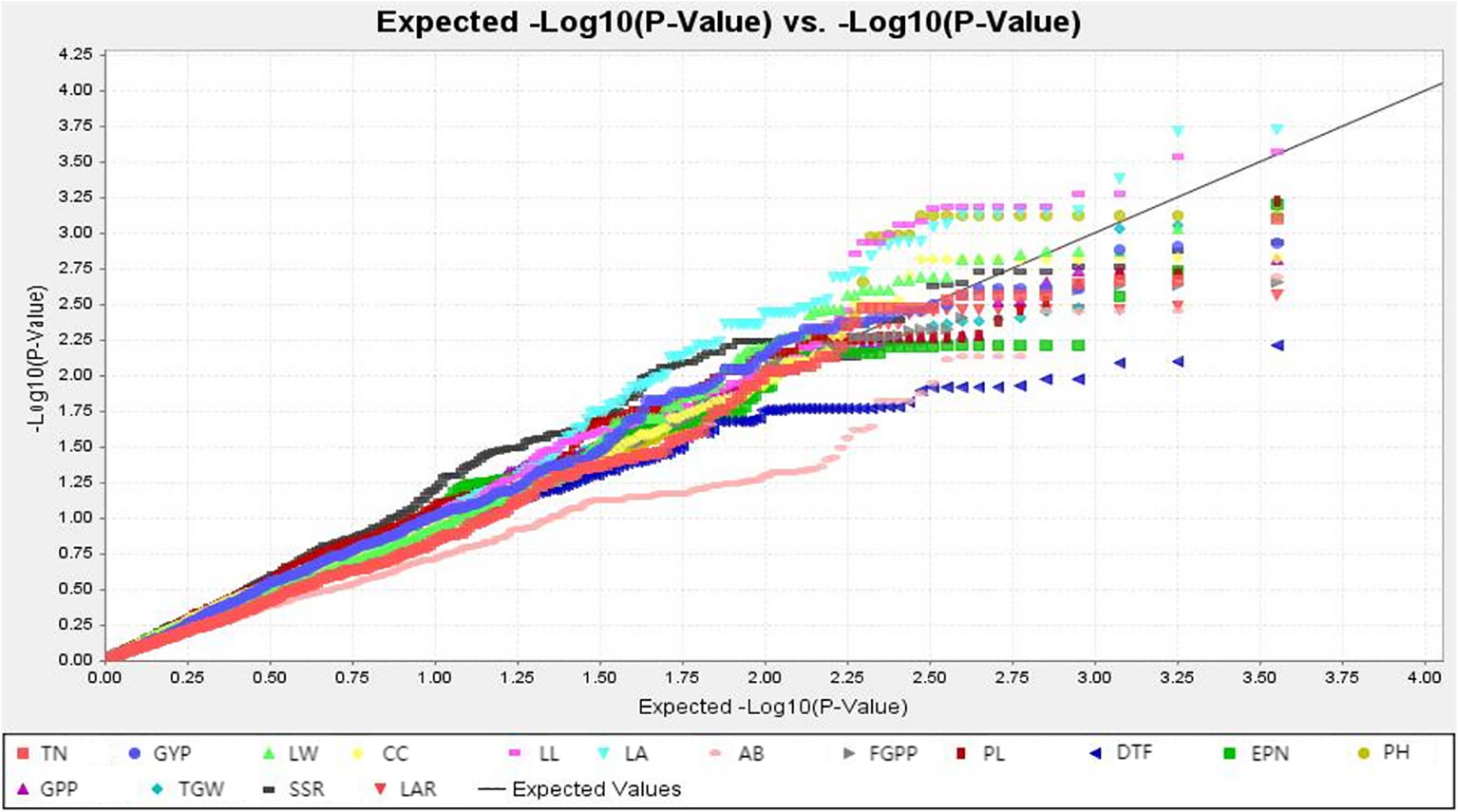
Figure 3 Q-Q plots illustration of drought tolerance index of measured trait. The abscissa of the Manhattan plot is 12 chromosomes of rice, the ordinate is -log10 (p) of SNPs, and the dashed horizontal line is the threshold of genome-wide significance.
The SNPs whose Manhattan map peak value exceeded the horizontal threshold of 3 may be the gene loci significantly associated with the measured phenotypic traits. There were 9 loci associated with rice drought resistance index located on multiple chromosomes (Figures 4, 5). One leaf area-related QTL was located on chromosome 7, with a P value of 3.719, and the explanation rate of phenotypic variation was 13.9%. One QTL related to leaf length was located on chromosome 7, with a P value of 3.568, and the explanation rate of phenotypic variation was 13.2%. One QTL related to ear length was located on chromosome 9 with a P value of 3.217, and the explanation rate of phenotypic variation was 11.5%. A QTL related to effective panicle number was identified on chromosome 6, with a P value of 3.199, indicating an explanatory rate of 11.4% of phenotypic variation. One QTL related to leaf width was located on chromosome 7, with a P value of 3.124, and the explanation rate of phenotypic variation was 11.5%. A QTL related to plant height was identified on chromosome 10, with a P value of 3.121, and the explanation rate of phenotypic variation was 11.1%. Three QTLS related to centroid weight were located on chromosomes 2, 6, and 8, with P values of 3.028, 3.052, and 3.219, respectively, among which qTGW8.1 contributed the most to the phenotype (11.6%). Detailed data are shown in Table 5.
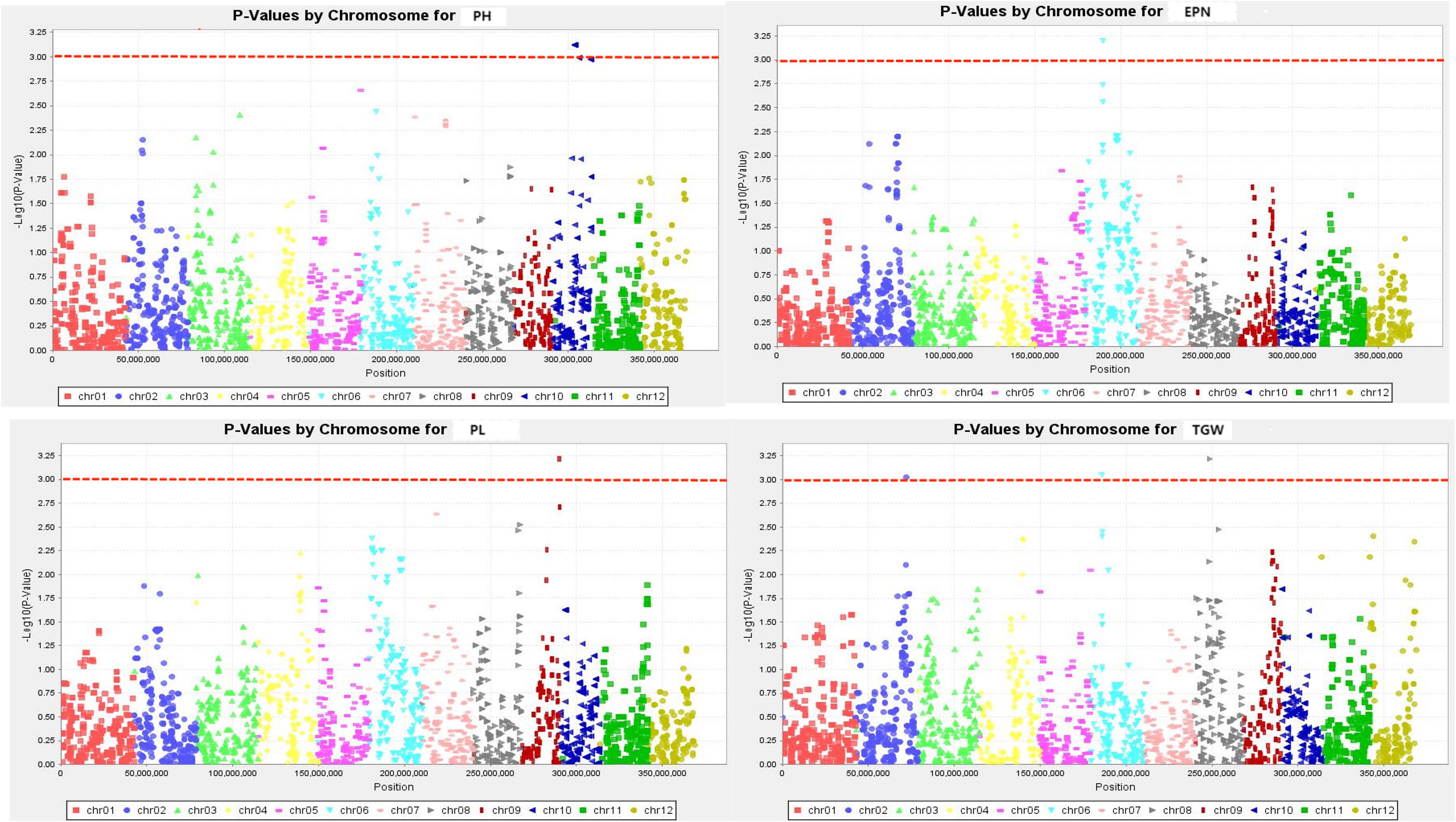
Figure 4 Manhattan plots exhibiting the drought tolerance index of measured traits. The abscissa of the Manhattan plot is 12 chromosomes of rice, the ordinate is -log10 (p) of SNPs, & the dashed horizontal line is the threshold of genome-wide significance.
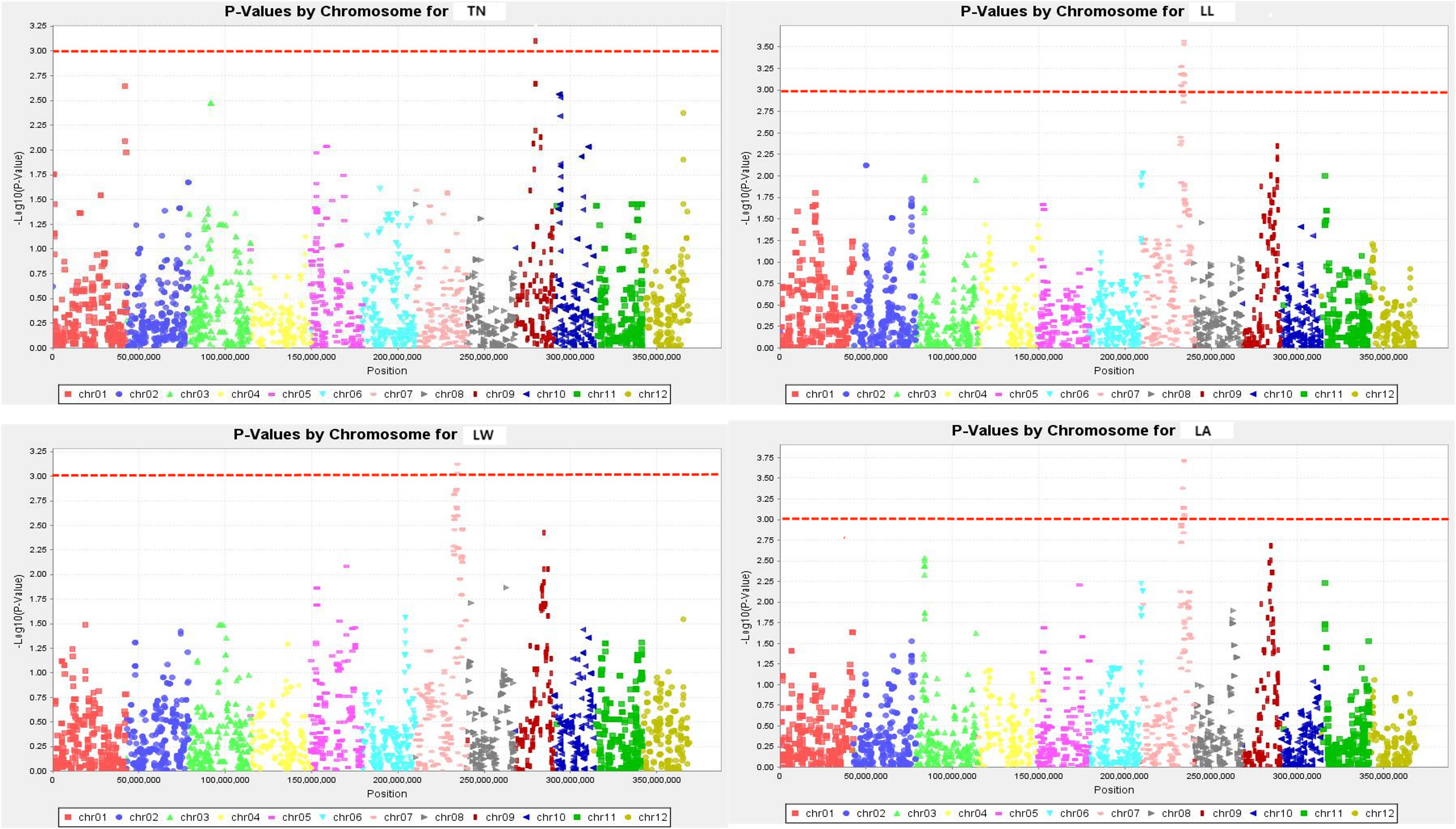
Figure 5 Manhattan plots exhibiting the drought tolerance index of measured traits. The abscissa of the Manhattan plot is 12 chromosomes of rice, the ordinate is -log10 (p) of SNPs, & the dashed horizontal line is the threshold of genome-wide significance.
3.5 Candidate gene association analysis
A total of 10 candidate gene loci were predicted on 12 rice chromosomes (Table 6). There were 23 candidate genes in the interval of 29.42 to 29.56Mb on chromosome 2. LOC_Os02g0712000 encoding a serine endopeptidase. There were 36 candidate genes between 6.7 and 10.4Mb on chromosome 6. LOC_Os06g0229800 was a cloned ALK, a key gene controlling rice’s gelatinization temperature and encoding soluble starch synthase II (Raza et al., 2020). LOC_Os06g0286500 encodes NBS-LRR disease resistance protein homologous; There were 261 candidate genes between 22.2-24.2MB on chromosome 7, among which LOC_Os07g05868 encoded a triacylglycerol lipase. LOC_Os07g0586700 encodes the transcriptional suppressant HOTR. LOC_Os07g0571800 is similar to the YABBY4 gene, which regulates plant growth and development by regulating the gibberellin pathway (Yang et al., 2016). LOC_Os07g0558500 encodes a fibro-alcohol tyrosine phosphatase antibody protein closely related to the NYC4 gene, which is involved in the degradation of chlorophyll-protein complexes during leaf aging (Yamatani et al., 2013). LOC_Os07g0558400 encodes chlorophyll a-b binding protein CP29.1, and LOC_Os07g0591100 encodes DUF620 family proteins. Seven candidate genes were in the 8.7-8.8MB region on chromosome 8, LOC_Os08g0243500 encoding NADPH oxidoreductases. LOC_Os09g0360400 encodes a holoenzyme synthase, and LOC_Os09g0555800 encodes a protein-containing AMP binding domain. There were 29 candidate genes in the 11.7-22.2MB range of chromosome 9. Five candidate genes encoding an oxidoreductase were between 13.6 and 13.7Mb of chromosome 10, LOC_Os09g0403400.
4 Discussion and conclusion
4.1 Heritability and correlation analysis of drought-resistant traits
Except for the DTF, the drought resistance index of all rice traits was less than 1. These results indicated that the phenotypic values of each trait under water stress were lower than those under normal water conditions. Drought stress had serious effects on rice morphology, yield, and physiology. Drought stress lengthened the heading time while decreased growth period and seed setting rate; this resulted in the significant reduction in the rice grain yield (Shamsudin et al., 2016; Sandhu & Kumar, 2017). The variation coefficients of FGPP and GYP were the highest under flood and drought conditions, indicating that drought had the most serious effect on grain number and yield. There was a significant positive correlation between yield-related and physiological traits in rice. The GYP was positively correlated with EPN, GPP, FGPP, and SSR, which was consistent with the analysis of Cu et al. (2021). There was a significant positive correlation among leaf traits.
4.2 GWAS location analysis
A total of 9 QTL loci related to drought resistance traits were identified by GWAS, which was distributed on chromosomes 2, 6, 7, 8, 9, and 10, which may provide important genetic resources for future breeding. In order of contribution rate value, they are qLA7.1, qLL7.1, qTGW8.1, qPL9.1, qEPN6.1, qLW7.1, qPH10.1, qTGW6.1, qTGW2.1. The site with the largest contribution rate is qLA7.1, which is related to leaf area. Studies have concluded that leaves are one of the most sensitive organs in plants to drought stress, and leaf area is negatively correlated with drought tolerance. Under mild drought stress, biomass accumulation was inhibited, plant area decreased, and leaf number decreased. Under severe drought stress, plant leaves will age, die and fall off rapidly (Chen et al., 2023).
The QTL related to plant height was located on chromosome 10. The QTL related to effective panicles number was located on chromosome 6. The physical location of the loci related to panicle length located by Zheng et al. (2022) on chromosome 9 was similar to the qPL9.1 loci region located in this study. QTLS related to 1000-grain weight were located on chromosomes 2, 6, and 8. The QTL related to the tiller number was located on chromosome 9. QTLS related to leaf length, leaf width, and leaf area were located on chromosome 7, and the loci of qLL7.1 and qLA7.1 overlap. The two loci are on the same chromosome, and there may be gene linkage. The phenotypic contribution rate of QTL loci ranged from 13.2% to 13.9%. Zhu and Xiong (2013) studied 1016 global rice core germplasm resources using GWAS analysis and identified 12 QTLS affecting the length of the rice blade, 7 QTLS affecting the width of the rice blade, and 6 QTLS affecting the area of the rice blade. The loci related to yield and leaf traits of each plant in this study were not similar to those of Zhu and Xiong (2013) so they may be new loci.
Leaf length, leaf width, and leaf area were controlled by LOC_Os07g0591100 closely linked gene, and leaf length and leaf area were controlled by LOC_Os07g0581300 closely linked gene, which suggested that there was a polytropic between the two genes. LOC_Os02g0712000, LOC_Os06g0229800, and LOC_Os08g0243500 tightly linked genes control the 1000-grain weight. LOC_Os02g0712000 encodes a serine endopeptidase. LOC_Os06g0229800 is an ALK gene. LOC_Os08g0243500 encodes a NADPH oxido-reductase. Loc_os08g0243500 is a crucial gene of rice gelatinization temperature, promoting starch and sucrose metabolism. The panicle length was mainly controlled by LOC_Os09g0555800 and LOC_Os09g0555850, closely linked genes. LOC_Os09g0555800 encodes a protein-containing AMP binding domain, and LOC_Os09g0555850 does not predict a known function. Effective panicle number was controlled by LOC_Os06g0286500 closely linked gene LOC_Os06g0286500 encoding NBS-LRR resistance protein homolog; Tiller number was mainly controlled by LOC_Os09g0360400 closely linked gene, encoding a holoenzyme synthase. Plant height was controlled by LOC_Os10g0403400 and LOC_Os10g0403700 closely linked genes. LOC_Os09g0403400 encodes an oxidoreductase, and LOC_Os10g0403700 has no predictive function.
4.3 Conclusion
Breeding for abiotic conditions like drought remains challenging due to the complex nature of the genetic system and the risk of unexpected developments (Debnath et al., 2023). To find the genes that control characteristics of drought tolerance, a modern molecular technique called QTL analysis was employed. In this study, nine QTL loci related to drought resistance were identified and located on chromosomes 2, 6, 7, 8, 9, and 10, which includes qPH10.1, qEPN6.1, qPL9.1, qTGW2.1, qTGW6.1, qTGW8.1 and qLL7.1, qLW7.1 and qLA7.1, respectively. The higher contribution rate sites were qLA7.1 related to leaf area, and qLL7.1, related to leaf length. Ten candidate genes were also predicted, that includes LOC_Os10g0403700 (oxido-reductase), LOC_Os06g0286500 (NBS-LRR disease-resistance protein homologs), LOC_Os09g0555800 (Amp-binding domains containing proteins), LOC_Os02g0712000 (Serine endopeptidase), LOC_Os06g0229800 (ALK gene), LOC_Os08g0243500 (NADPH oxido-reductase), LOC_Os07g0558400 (Chlorophyll a-b binding protein), LOC_Os07g0571800 (YABBY4 gene), LOC_Os07g0586700 (transcription repressor HOTR), and LOC_Os07g0591100 (DUF620 family protein). It is necessary to continue verifying and fine-locating these QTLs and candidate genes through the conduction of genetic transformation and functional verification of candidate genes. This research study laid a foundation for a better understanding of rice drought tolerance genetic basis and facilitated the rice breeding program with respect to drought tolerance.
Data availability statement
The original contributions presented in the study are included in the article/Supplementary Files, further inquiries can be directed to the corresponding author/s.
Author contributions
YY and MH designed the experiment and wrote the manuscript. XC, SW and QZ contributed to the data interpretation and writing. YL and HL designed the figures and formulated tables. WF, QZ, MH, and YY participated in the processing of the experimental material. All authors read and approved the final manuscript.
Funding
The work was supported by a grant from the Anhui Natural Science Foundation Project (2208085QC82; 1908085MC93), the Young Talent Project of Anhui Academy of Agricultural Sciences, Asian Cooperation Fund Project (125001012000210004), and Anhui Provincial Key Research and Development Program (202104f06020017).
Acknowledgments
We thank Changrong Ye from Higentec Co. for the data analysis, thank Jingde Wu, Yonggeng Chen, Cunli Gong, and Yanyan Ma who helped with data collection.
Conflict of interest
The authors declare that the research was conducted in the absence of any commercial or financial relationships that could be construed as a potential conflict of interest.
Publisher’s note
All claims expressed in this article are solely those of the authors and do not necessarily represent those of their affiliated organizations, or those of the publisher, the editors and the reviewers. Any product that may be evaluated in this article, or claim that may be made by its manufacturer, is not guaranteed or endorsed by the publisher.
Supplementary material
The Supplementary Material for this article can be found online at: https://www.frontiersin.org/articles/10.3389/fpls.2023.1223782/full#supplementary-material
References
Bhandari, A., Sandhu, N., Bartholome, J., Cao-Hamadoun, T. V., Ahmadi, N., Kumari, N., et al. (2020). Genome-Wide Association Study for Yield and Yield Related Traits under Reproductive Stage Drought in a Diverse indica-aus Rice Panel. Rice 13 (1), 1–22. doi: 10.1186/S12284-020-00406-3/FIGURES/9
Chen, L., Ma, J., Ma, X. D., Cui, D., Han, B., Sun, J. C., et al. (2023). QTL analysis of drought tolerance traits in rice during the vegetative growth period. Euphytica 219 (3), 1–16. doi: 10.1007/S10681-022-03151-4/METRICS
Chen, S., Xu, K., Kong, D., Wu, L., Chen, Q., Ma, X., et al. (2022). Ubiquitin ligase OsRINGzf1 regulates drought resistance by controlling the turnover of OsPIP2;1. Plant Biotechnol. J. 20 (9), 1743–1755. doi: 10.1111/PBI.13857
Cu, S. T., Warnock, N. I., Pasuquin, J., Dingkuhn, M., Stangoulis, J. (20212021). A high-resolution genome-wide association study of the grain ionome and agronomic traits in rice Oryza sativa subsp. indica. Sci. Rep. 11, 1. doi: 10.1038/s41598-021-98573-w
Debnath, S., Kant, A., Bhowmick, P., Malakar, A., Purkaystha, S., Jena, B. K., et al. (2023). The enhanced affinity of WRKY reinforces drought tolerance in Solanum lycopersicum L.: An innovative bioinformatics study Plants. Plants (Basel) 12, 4, 762. doi: 10.3390/plants12040762
Dixit, S., Singh, A., Sandhu, N., Bhandari, A., Vikram, P., Kumar, A. (2017). Combining drought and submergence tolerance in rice: marker-assisted breeding and QTL combination effects. Mol. Breeding : New Strategies Plant Improvement 37 (12), 1–12. doi: 10.1007/S11032-017-0737-2
Guo, Z., Yang, W., Chang, Y., Ma, X., Tu, H., Xiong, F., et al. (2018). Genome-Wide Association Studies of Image Traits Reveal Genetic Architecture of Drought Resistance in Rice. Mol. Plant 11 (6), 789–805. doi: 10.1016/J.MOLP.2018.03.018
Hassan, M. A., Xiang, C., Farooq, M., Muhammad, N., Yan, Z., Hui, X., et al. (2021). Cold Stress in Wheat: Plant Acclimation Responses and Management Strategies. Front. Plant Sci. 12. doi: 10.3389/fpls.2021.676884
Ikmal, A. M., Nurasyikin, Z., Riana, T. A. T. N. A., Ellina, Z. P. D., Wickneswari, R., Noraziyah, A. A. S. (2019). Drought Yield QTL ( qDTY) with Consistent Effects on Morphological and Agronomical Traits of Two Populations of New Rice ( Oryza sativa) Lines. Plants (Basel Switzerland) 8 (6), 1–16. doi: 10.3390/PLANTS8060186
Kumar, A., Basu, S., Ramegowda, V., Pereira, A. (2017). “Mechanisms of drought tolerance in rice,” in Achieving sustainable cultivation of rice, Chapter 8. 1, 131–163. doi: 10.19103/AS.2106.0003.08
Li, X., Guo, Z., Lv, Y., Cen, X., Ding, X., Wu, H., et al. (2017). Genetic control of the root system in rice under normal and drought stress conditions by genome-wide association study. PLoS Genet. 13 (7), 1–24. doi: 10.1371/JOURNAL.PGEN.1006889
Liang, Y., Liu, H. J., Yan, J., Tian, F. (2021). Natural Variation in Crops: Realized Understanding, Continuing Promise. Annu. Rev. Plant Biol. 72, 357–385. doi: 10.1146/ANNUREV-ARPLANT-080720-090632
Ma, X., Feng, F., Wei, H., Mei, H., Xu, K., Chen, S., et al. (2016). Genome-Wide Association Study for Plant Height and Grain Yield in Rice under Contrasting Moisture Regimes. Front. Plant Sci. 7 (NOVEMBER2016). doi: 10.3389/FPLS.2016.01801
Oladosu, Y., Rafii, M. Y., Samuel, C., Fatai, A., Magaji, U., Kareem, I., et al. (2019). Drought Resistance in Rice from Conventional to Molecular Breeding: A Review. Int. J. Mol. Sci. 20 (14), 1–21. doi: 10.3390/IJMS20143519
Raza, Q., Riaz, A., Bashir, K., Sabar, M. (2020). Reproductive tissues-specific meta-QTLs and candidate genes for development of heat-tolerant rice cultivars. Plant Mol. Biol. 104 (1–2), 97–112. doi: 10.1007/S11103-020-01027-6
Sandhu, N., Kumar, A. (2017). Bridging the Rice Yield Gaps under Drought: QTLs, Genes, and their Use in Breeding Programs. Agronomy 7 (2), 27. doi: 10.3390/AGRONOMY7020027
Shamsudin, N. A. A., Swamy, B. P. M., Ratnam, W., Sta. Cruz, M. T., Sandhu, N., Raman, A. K., et al. (2016). Pyramiding of drought yield QTLs into a high quality Malaysian rice cultivar MRQ74 improves yield under reproductive stage drought. Rice (New York N.Y.) 9 (1), 21–21. doi: 10.1186/S12284-016-0093-6
Sun, X., Xiong, H., Jiang, C., Zhang, D., Yang, Z., Huang, Y., et al. (20222022). Natural variation of DROT1 confers drought adaptation in upland rice. Nat. Commun. 13, 1. doi: 10.1038/s41467-022-31844-w
Tang, Y., Bao, X., Zhi, Y., Wu, Q., Guo, Y., Yin, X., et al. (2019). Overexpression of a MYB Family Gene, OsMYB6, Increases Drought and Salinity Stress Tolerance in Transgenic Rice. Front. Plant Sci. 10. doi: 10.3389/FPLS.2019.00168
Wang, Y., Pang, Y., Chen, K., Zhai, L., Shen, C., Wang, S., et al. (2020). Genetic bases of source-, sink-, and yield-related traits revealed by genome-wide association study in Xian rice. Crop J. 8 (1), 119–131. doi: 10.1016/J.CJ.2019.05.001
Wang, H., Qin, F. (2017). Genome-Wide Association Study Reveals Natural Variations Contributing to Drought Resistance in Crops. Front. Plant Sci. 8. doi: 10.3389/FPLS.2017.01110
Wang, C., Song, B., Yu, S., Xiao, W., Huang, M. (2021). Construction of a genetic map of rice RILs based on whole genome sequencing. J. South China Agric. Univ 42 (2), 44–50. doi: 10.7671/J.ISSN.1001-411X.202006039
Yamatani, H., Sato, Y., Masuda, Y., Kato, Y., Morita, R., Fukunaga, K., et al. (2013). NYC4, the rice ortholog of Arabidopsis THF1, is involved in the degradation of chlorophyll - Protein complexes during leaf senescence. Plant J. 74 (4), 652–662. doi: 10.1111/TPJ.12154
Yang, X., Lu, M., Wang, Y., Wang, Y., Liu, Z., Chen, S. (2021). Response Mechanism of Plants to Drought Stress. Horticulturae 7 (3), 50. doi: 10.3390/HORTICULTURAE7030050
Yang, C., Ma, Y., Li, J. (2016). The rice YABBY4 gene regulates plant growth and development through modulating the gibberellin pathway. J. Exp. Bot. 67 (18), 5545–5556. doi: 10.1093/JXB/ERW319
Yonemaru, J., Yamamoto, T., Fukuoka, S., Uga, Y., Hori, K., Yano, M. (2010). Q-TARO: QTL annotation rice online database. Rice 3 (2–3), 194–203. doi: 10.1007/S12284-010-9041-Z/FIGURES/4
Zheng, H., Sun, S., Bai, L., Jiang, S., Ding, G., Wang, T., et al. (2022). Identification of candidate genes for panicle length in Oryza sativa L. ssp. japonica via genome-wide association study and linkage mapping. Euphytica 218 (2), 1–12. doi: 10.1007/S10681-022-02972-7/METRICS
Keywords: rice, drought tolerance, agronomic yield traits, genome-wide association studies, quantitative trait loci (QTL)
Citation: Yi Y, Hassan MA, Cheng X, Li Y, Liu H, Fang W, Zhu Q and Wang S (2023) QTL mapping and analysis for drought tolerance in rice by genome-wide association study. Front. Plant Sci. 14:1223782. doi: 10.3389/fpls.2023.1223782
Received: 16 May 2023; Accepted: 04 July 2023;
Published: 25 July 2023.
Edited by:
Muhammad Aamir Manzoor, School of Agriculture and Biology, Shanghai Jiao Tong University, ChinaReviewed by:
Fasih Ullah Haider, South China Botanical Garden (CAS), ChinaIrfan Ullah Khan, Jiangsu University, China
Zoia Arshad Awan, Teagasc Food Research Center, Ireland
Copyright © 2023 Yi, Hassan, Cheng, Li, Liu, Fang, Zhu and Wang. This is an open-access article distributed under the terms of the Creative Commons Attribution License (CC BY). The use, distribution or reproduction in other forums is permitted, provided the original author(s) and the copyright owner(s) are credited and that the original publication in this journal is cited, in accordance with accepted academic practice. No use, distribution or reproduction is permitted which does not comply with these terms.
*Correspondence: Shimei Wang, d2FuZ3NoaW1laTA1NTFAMTYzLmNvbQ==; Qian Zhu, emh1cWlhbndhbGtAMTYzLmNvbQ==
†These authors have contributed equally to this work