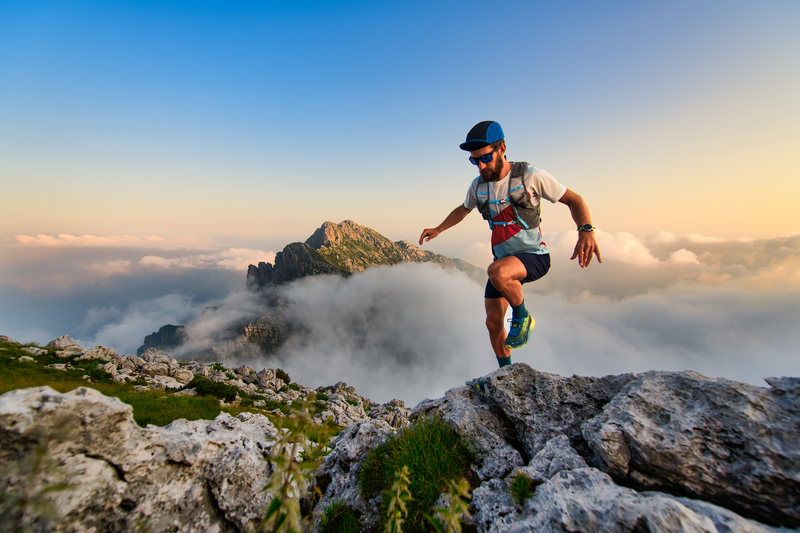
95% of researchers rate our articles as excellent or good
Learn more about the work of our research integrity team to safeguard the quality of each article we publish.
Find out more
REVIEW article
Front. Plant Sci. , 15 November 2023
Sec. Crop and Product Physiology
Volume 14 - 2023 | https://doi.org/10.3389/fpls.2023.1195210
This article is part of the Research Topic Molecular Regulation of Seed Development and Storage Reserve Metabolism in Crops View all 8 articles
Soybean (Glycine max L. Merr.) is a crucial oilseed cash crop grown worldwide and consumed as oil, protein, and food by humans and feed by animals. Comparatively, soybean seed yield is lower than cereal crops, such as maize, rice, and wheat, and the demand for soybean production does not keep up with the increasing consumption level. Therefore, increasing soybean yield per unit area is the most crucial breeding objective and is challenging for the scientific community. Moreover, yield and associated traits are extensively researched in cereal crops, but little is known about soybeans’ genetics, genomics, and molecular regulation of yield traits. Soybean seed yield is a complex quantitative trait governed by multiple genes. Understanding the genetic and molecular processes governing closely related attributes to seed yield is crucial to increasing soybean yield. Advances in sequencing technologies have made it possible to conduct functional genomic research to understand yield traits’ genetic and molecular underpinnings. Here, we provide an overview of recent progress in the genetic regulation of seed size in soybean, molecular, genetics, and genomic bases of yield, and related key seed yield traits. In addition, phytohormones, such as auxin, gibberellins, cytokinins, and abscisic acid, regulate seed size and yield. Hence, we also highlight the implications of these factors, challenges in soybean yield, and seed trait improvement. The information reviewed in this study will help expand the knowledge base and may provide the way forward for developing high-yielding soybean cultivars for future food demands.
Many civilizations around the world consider seeds to be a staple diet. Seed grains, such as wheat (Triticum aestivum), rice (Oryza sativa), maize (Zea mays), and soybean (Glycine max L. Merr.), are regularly consumed by humans as a source of energy and nutrients. Global food security is continuously worsened by numerous drivers, such as climate change, declining cropland, rising human population, and, more recently, the COVID-19 pandemic and the Russia-Ukraine war (Ben Hassen and El Bilali, 2022; Lin et al., 2023). Furthermore, the yield of most staple food crops, including maize, wheat, rice, and soybean, is stagnating globally and may further decline with the increasing global temperatures (Zhao et al., 2017). Thus, increasing crop yield is a big challenge for the coming decades. Seed development is a complex process and involves diverse events, such as cell division, differentiation, and developmental regulatory processes. The seed developmental process influences the final seed size (SS) and morphology. Typically, SS is determined based on the seed’s thickness, length, and width and their ratios (Figure 1) (Cui et al., 2020). Seed width, height, and length impact the morphological integrity and quality of the seed. Given the importance of SS, many plant species established and demonstrated the molecular and genetic mechanisms involved in regulating SS and shape. Mainly, studies on model plant species like Arabidopsis (Arabidopsis thaliana) and barrel medic indicate that the molecular and genetic basis of SS depends on the early stages of seed development and involves different cellular events. These cellular events are initiated by zygotic division followed by the formation of nutritive tissues and the maturation stage, which subsequently accumulates storage products (carbohydrates, oil/lipids, proteins, vitamins, minerals, and nutrients) (Sreenivasulu and Wobus, 2013; Han et al., 2016).
Soybean is a top-rank economically important oilseed crop cultivated and consumed worldwide. Soybean seeds are excellent sources of energy, oil, protein, carbohydrates, and fat (Hudson, 2022). They are a rich, inexpensive source of protein for humans and an essential source of fodder/feed for domesticated livestock. Consequently, soybean is regarded as one of the most commercially significant oilseed crops. The cultivated soybean has a long history of domestication and breeding from wild soybeans (Glycine soja Sieb. and Zucc.) (Hymowitz, 1970; Caldwell and Howell, 1973). Domestication and selection have undoubtedly resulted in modern soybean cultivars with superior seed characteristics, such as seed color, size, shape, and weight, compared to their wild progenitors (Liu et al., 2020d; Bohra et al., 2022). Most seed domestication and improvement traits are directly related to crop yield. In addition, seed-based products, such as soya tofu, sprout, edamame, and natto, vary with SS characteristics. Thus, SS and seed weight (SW) are essential traits in soybean breeding for crop yield improvement. The yield is a complex trait governed by multiple genes/loci or minor quantitative trait loci (QTLs) and influenced by environmental factors. The genetic makeup and molecular mechanisms underlying the seed traits are crucial for identifying gene regulatory networks of SS and yield. In cereals like rice and maize, genetic and molecular controls of seed development and yield are well established. The research on cereal seed traits leads to significant yield enhancement compared to soybean. However, basic research on the seed and embryo development of legumes, including soybean, medicago (Medicago truncatula), common bean (Phaseolus vulgaris), pea (Pisum sativum), and broad bean (Vicia faba) was reported (Le et al., 2007).
Recent research has employed genomic technologies, including genomics, proteomics, and transcriptomics, to uncover QTLs, genes, and specific underlying pathways that are critical for leguminous seed development (Gao et al., 2018; Afzal et al., 2020; Liu et al., 2022). More than 500 QTLs specific to soybean and associated with yield-related traits (SW, 100-seed weight (HSW), number of branches on main stem, plant height, total seed number per plant, number of nodes, total pod number per plant (NPP), seed yield per plant, and diameter of main stem) have been revealed on the Soybase Genome Database (SoyBase, 2023). In addition, previous studies in soybean reported crucial genes (GmFAD3, GA20OX, GmLEC2, GmPDAT, GmKIX8-1, GmSSS1, ST1, and GmGA3ox1) that alter SS and yield by regulating lipid accumulation, cell expansion, and cell proliferation (Manan et al., 2017; Liu et al., 2020a; Nguyen et al., 2021; Hu et al., 2022; Li et al., 2022b; Zhu et al., 2022). Furthermore, researchers identified and reported genetic factors that influence SS and SW in soybean (Zhou et al., 2016; Jing et al., 2018). Similarly, (Gao et al., 2018) discovered several genes involved in soybean seed development, accumulation of storage proteins and oils, and seed coat development. However, although genetic and genomic resources are available for soybean, information on yield and underlying genes for most reported QTLs has not been extracted. Thus, broadening our knowledge of genetics and genomics can provide insight into the molecular, genetic, and genomic bases of seed yield in soybean and identify genes and pathways essential for seed development. This knowledge can then be used to breed soybean cultivars with improved seed yields through traditional or advanced molecular breeding approaches.
Overall, molecular, genetic, and genomic studies of soybean seed development as well as related traits are essential for understanding the underlying biological processes and developing strategies to increase seed yield in this important crop. Although the genetics and genomics of seed development and yield in soybean have been the subject of many studies in recent years, there is no complete compiled information on the genetic and genomic basis of the key seed yield traits. Thus, this review aims to provide an overview of recent progress in molecular, genetic, and genomic levels in the context of yield and related key seed yield traits. In addition, several phytohormones, such as auxin, gibberellins (GA), cytokinins (CK), and abscisic acid (ABA), regulate SS and yield in soybeans; therefore, we also highlight the implications of these factors in our review. Finally, challenges in soybean yield and seed trait improvement and avenues to expand the knowledge base and its significance in soybean crop genetic improvement are addressed.
Seed yield is an essential trait in crops because it determines the number of seeds a plant produces, which can be used for food, feed, or propagation. Improving seed yield can help increase crop production and enhance food security. Several factors can affect seed yield in crops, including genetics, environment, and management practices.
Soybean seed yield is also a complex quantitative trait governed by multiple genes, broadly influenced by the growing conditions and latitudes. Although soybean is cultivated globally, it is a short-day, photoperiod-sensitive crop with different geographical latitude ranges (Chen et al., 2020a; Toleikiene et al., 2021). Soybean seeds vary in size, shape, length, and color, as displayed in Figure 1. Seed yield is a complex trait determined by various components. Similarly, a complex features that are influenced by both genetic and environmental factors during seed growth and maturation include seed size, oil content, and protein content. Given their significance in soybean breeding, researchers have used numerous bi-parental derived populations, including the F2 population, recombinant inbred lines (RILs), chromosome segment substitution lines (CSSLs), and near-isogenic lines (NILs), to perform extensive linkage analysis to QTL associated with these three seed traits (Qi et al., 2014; Warrington et al., 2015; Yang et al., 2019; Yang et al., 2022). In the SoyBase Genome Database, hundreds of QTLs associated with SS (including SW), oil accumulation, and protein content have so far been identified. For instance, 396 QTLs are associated with SW and SS, 333 with seed oil content, and 234 with seed protein content. Some of these QTLs, including those relating to protein content, oil accumulation, and seed size, had overlapping areas, indicating the presence of pleiotropic regulating genes in these QTLs (Wang et al., 2015a; Cui et al., 2020; Kumawat and Xu, 2021; Kumar et al., 2022; Luo et al., 2022). Additionally, characteristics like SS, SW, NPP, number of seeds per pod, and HSW are key component traits directly influencing crop yield. In some studies, SS is considered as SW and vice versa (Xu et al., 2022). The same study revealed how the size of seeds affects the yield. It was found that bigger seeds didn’t make the yield better; in fact, they sometimes made the yield lower compared to smaller seeds, especially in good growing conditions. Similarly, another study reported a negative correlation of HSW (r= -0.05) with yield while a significant positive correlation of pod number (r= 0.44), and total grain number (r = 0.44) was noticed with the yield output (Attipoe et al., 2023) while contrastingly, some studies have revealed different results. A study conducted to check the effect of SS on crop yield, including others, has revealed a positive impact, where larger seeds produced an increase of 5.4% on average from the small seeds genotypes in 10 experiments (Smith and Camper, 1975), furthermore, a separated study also revealed high crop yield for the accessions having larger SS comparatively to the smaller genotypes (Adebisi et al., 2013), adding more, another study conducted revealed NPP, total number of seeds, and HSW showed a positive correlation of (r = 0.92), (r = 0.93), and (r = 0.063) with yield, respectively (Vu et al., 2019). Analyzing the mentioned studies, it can be concluded that the most important and defining traits contributing to crop yield directly are the pods number and number of seeds, revealing the biggest correlation with the yield in every study conducted (Adebisi et al., 2013; Attipoe et al., 2023). However, relying solely on simple correlation to establish the causal relationship between yield components and yield might not accurately capture the intricate cause-and-effect dynamics, thus leading to potential inefficiencies in the selection strategy. Although the SS is one of the factors that influence the crop’s overall yield directly, it also has a wide indirect influence on the crop output such as larger seeds are noted to be heavier (Alonso-Blanco et al., 1999) which also means more stored energy reserves, larger cotyledons, and greater initial vigor, ultimately resulting in good overall crop production and yield output (Ambika et al., 2014). On the other hand, traits like plant height, number of nodes, branches, and growth also indirectly influence crop yield. Adding to that, research reported SS as a key yield component, where it stated; it influences seed thickness, length, and width (Alonso-Blanco et al., 1999). In addition, it was reported that contrary to stress-associated traits, the domestication and development of soybeans through both natural and artificial selection resulted in an increase in genetic variation for traits related to seed protein, oil content, flowering, SW, and total yield (Hwang et al., 2014; Lu et al., 2020; Miao et al., 2020). Arabidopsis and cereal crops are well studied and documented for yield traits compared to soybean. For example, in rice, several seed-size regulatory genes have been cloned and characterized (Li and Li, 2016; Duan and Li, 2021; Chen et al., 2022). However, the quantitative nature of the trait impedes the discovery and functional characterization of the underlying regions/loci and genes in soybean. In this regard, the most recent studies on the genetic basis, genomic regions/loci, QTLs, and genes associated with soybean yield attributes may help us better understand the molecular underpinnings determining soybean seed yield. This knowledge could lead to the best exploration of traits and associated genes through molecular breeding strategies for greater yield enhancement in soybean.
In the recent decade, dissecting the genetic basis of soybean yield traits has drawn growing interest. The development of advanced technologies such as Next Generation Sequencing (NGS); enables researchers to obtain comprehensive genomic information by rapibly sequencing large amounts of DNA. This has been instrumental in the development of high-resolution genetic linkage maps, allowing for detailed investigations into desired agronomic traits (Singh et al., 2015) and, Clustered Regularly Interspaced Short Palindromic Repeats (CRISPR) technology has provided a powerful tool for precise genome editing, enabling researchers to elucidate gene functions and dissect the genetic basis of complex traits. By utilizing CRISPR-Cas9, scientists have been able to investigate the role of candidate genes identified through transcriptome and proteome analyses, as well as study mutants and transgenic plants to gain insights into the mechanisms underlying soybean yield traits (Ran et al., 2013). CRISPR/Cas9 has been used in 20 crop species, improving traits like yield and resistance to biotic and abiotic stresses (Chaudhary et al., 2022). As mentioned earlier, yield is a quantitative trait governed by multiple genes and influenced by small-effect QTLs and environmental conditions. Thus, investigating the yield traits and identifying the undermining genetic and molecular basis of these traits is very challenging. However, ongoing advances in genomics and molecular biology and the availability of pangenome of wild and cultivated soybeans have facilitated the identification of major and minor QTLs, genomic regions, and candidate genes associated with yield traits in soybean (Tayade et al., 2019; Liu et al., 2020e; Li et al., 2022d). Researchers have developed high-resolution genetic linkage maps based on single nucleotide polymorphism (SNP) markers to study the desired agronomic traits (Song et al., 2016; Zhang et al., 2018; Knizia et al., 2021). By keeping in mind the complex nature of traits, several studies developed biparental mapping populations to dissect the genetic bases of yield and related traits, which led to the identification of several essential QTLs for regulatory genetic variation associated with seed yield in soybean (Li et al., 2020; Li et al., 2022c).
In comparison to the method of gene identification related to specific traits using biparental QTL mapping, a more precise location of QTLs can be obtained from genome-wide association studies (GWAS) (Zeng et al., 2017). Researchers used the GWAS approach to identify genomic regions associated with soybean yield traits. Additionally, candidate genes have been identified through transcriptome and proteome analyses and functional studies of mutants and transgenic plants. While it is not our objective to fully explain all the QTLs or candidate genes identified through transcriptome and proteome, we highlight some of the key characteristics and QTLs of yield traits in the following part.
Several traits are considered essential and directly impact the overall yield of the crop. As previously discussed, SS, SW, shape, and HSW are important yield component traits. Although these are the main elements determining crop yield, most earlier investigations focused on main-effect QTLs for SS, morphology, and HSW in soybean. Globally, numerous studies have been conducted on the QTL localization of soybean seed yield traits, especially for SS and SW. Compared to studies on SW, studies on QTL localization for SS were fewer. So far, 304 QTLs have been reported for SW, whereas, <100 QTLs have been reported for SS (seed shape, length, width, and volume/thickness) on the Soybase. However, some researchers recently reported additional multi-environment QTLs using the different genetic backgrounds for SS and weight, which may not be listed in the soybean database. Mian et al. (1996) reported 16 QTLs for SS and shape on 12 soybean chromosomes in this context. However, they used low throughput outdated restriction fragment length polymorphisim (RFLP) markers. Similarly, 27 QTLs for SS and 3 QTLs for seed length were identified using the low-density simple sequence repeats (SSR) markers in different mapping populations of soybean (Hoeck et al., 2003; Li et al., 2008). Further, Lu et al (2017) identified 19 main-effect QTLs and three epistatic-effect QTLs for seed length. Sun et al (2022) identified 10 QTLs using the RIL population for SS in four environments with phenotypic variations (PVE) ranging from 3.6% to 9.4%. Similarly, 53 and 27 QTLs were identified for SS and seed shape, respectively, in three growing seasons using the RIL population created by crossing K099 (small SS) × Fendou 16 (large SS) parents. Among them, six QTLs (qSW8.1, qSW16.1, qSLW2.1, qSLT2.1, qSWT1.2, and qSWT4.3) displayed a significant range of logarithm of odd (LOD) of 3.80–14.0 and PVE of 2.36%–39.49% in at least two growing seasons (Kumawat and Xu, 2021). Luo et al. (2023) recently identified 60 QTLs for SS and 25 QTLs for SW using the RIL population in five natural environments and their combined environment. For the seed yield, 365 QTL loci have been reported in the SoyBase. QTLs for SS and SW are represented in Figure 2 from recently published articles and the soybean database, and major QTLs for SS and SW with a PVE >10 are reviewed in Table 1. Another crucial yield-related attribute is HSW. During domestication, HSW was one of the fitness traits and selection parameters for soybean (Duan et al., 2022; Goettel et al., 2022). Previously, researchers found wide variation in the HSW in the American and Chinese germplasms ranging from 5.64 to 34.80 g (Zhang et al., 2016a; Zhao et al., 2019). To determine the genetic factor for the variation of HSW, linkage mapping was conducted with multiple backgrounds, and associated QTLs were identified. For example, 19 QTLs were identified for HSW in individual and combined environments, among which seven novel minor (R2 < 10%), 2 (qSW-17-1 and qSW-17-4) major (R2 > 10%), and eight stable QTLs (Karikari et al., 2019). Recently, 3 major QTLs (q100SW-4-1, q100SW-11-1, and q100SW-17-1) with PVEs of 11.41%, 14.67%, and 14.37%, respectively, were identified (Kumar et al., 2023).
Figure 2 Chromosomal distribution of important QTLs associated with the seed yield traits throughout the soybean genome. QTLs reported on SoyBase are mapped on chromosomes. Individual QTLs explaining >10% of phenotypic variation for the respective yield traits are highlighted in pink. They include seed volume (SV), seed length (SL, SLYZ), seed width (SWa), seed height (SH), seed length-to-height ratio (SLH), seed length-to-width ratio (SLW), seed width-to-height ratio (SWH), seed hilum width (SHW), seed hilum length (SHL), seed hilum area (SHA/PSHA), seed thickness (ST/STHN), seed weight (sw, SWb, SWTb, swHCB, swHMK, and swHBD), and hundred-seed weight (100SW).
Table 1 Major quantitative trait loci (QTLs) discovered contributing to > 10% phenotypic variation for the yield traits in soybean.
Regarding the NPP, several QTLs have been identified using diverse genetic backgrounds and environments, indicating that the variation in pod number is widespread in soybean. Researchers demonstrated that increased pod setting per plant and NPP highly influence the soybean yield; however, it is sensitive to the environment controlled by multiple genes, most of them with small effects (Xavier and Rainey, 2020). In order to improve the effectiveness of breeding for higher yields while taking the significance of the total NPP into mind, several studies identified QTLs linked to the total number of pods (Zhang et al., 2010; Rodrigues et al., 2016; Liu et al., 2017b). To date, 51 QTLs were reported in the SoyBase for the NPP. Figure 2 displays the identified QTLs, excluding QTLs whose position was not determined. Recently, Sun et al. (2022) identified the 6 and 5 QTLs for total flower pod numbers (TFPN) and NPP, respectively. Furthermore, among the 11 QTLs, they identified QTLs (qFPN4) with PVEs of 9.2% and 9.6% for TFPN and NPP, respectively, on chromosome 4.
Previous research primarily concentrated on discovering significant QTLs for SS/SW under various genetic backgrounds. However, soybean linkage mapping, particularly for yield attributes, still needs to be researched, as several studies have been unable to discover stable QTLs and lack a thorough examination of epistasis and environmental impacts.
GWAS have become a common approach to finding out marker-trait associations (MTAs) for complex traits in the genetically diverse population of plants and detecting the relationship between the genetic variance and these traits (Visscher et al., 2017; Kaler et al., 2020). In addition to perform QTL mapping to analyze soybean yield traits such as SS/SW and HSW, GWAS was undertaken to discover QTLs, quantitative trait nucleotides (QTNs), and the genetic loci associated with these traits. In association analysis, mostly natural populations or germplasm resources are taken as the research object, based on linkage disequilibrium, and millions of SNP markers apply for correlation analysis of genetic factors of desired traits. Compared with linkage analysis, association analysis is fast, high-throughput, and displays a high-resolution advantage. With the recent advancements in powerful statistical genetics models, the adjustment of false discovery rates, and the use of improved population structure, kinship matrices, and computational tools, GWAS became an efficient method for QTN detection for desired traits. GWAS, QTLs related to 140 different soybean traits, such as root, shoot, yield, nutrient constituents, and biotic and abiotic stresses, are listed in the SoyBase (https://www.soybase.org/GWAS/list.php). Thus, it is clear that GWAS QTL research in soybean is increasing.
In soybean, this approach is used for MTAs and the identification of QTLs or QTNs For example, Li et al (2019b) identified 139 QTNs linked with four yield-related traits using 82,187 SNPs by phenotyping 133 soybean landraces. Among them, 35 QTNs were repeated across evaluated environments. Similarly, Qi et al. (2020) identified 118 QTNs associated with the HWS of soybean, using 109,676 SNP markers from 144 RILs. Ayalew et al. (2022) used the largest number of diverse germplasm resources (541) and 50K SNPs, which led to identifying 19 QTNs significantly associated with seed yield. Their study found two stable seed yield QTNs on chromosomes 9 and 17 detected consistently in three environments. In addition, using 6K SNP markers and 470 soybean accessions, 14 QTNs were identified for seed yield-related traits on 6 different chromosomes (Jo et al., 2022). Similar studies with significant effects on seed yield traits in a selected population of soybean germplasm were reported (Zhang et al., 2016a; Yan et al., 2017; Copley et al., 2018; Li et al., 2019a; Li et al., 2019c). In Table 2, we have provided the major GWAS QTNs reported in the last decade, displaying significant LOD and contributing to higher PVE for yield traits, all tested in two or more environments.
Table 2 Reported genome-wide quantitative trait nucleotides (QTNs) for major seed yield traits in soybean.
Genes governing particular traits are always a topic of interest for plant breeding programs since most crop yield-related traits are governed by numerous genes (Zhang et al., 2020). Although QTL mapping and GWAS for yield traits are conducted in soybean, it remains challenging to effectively boost yield or quality by genetic modification due to the lack of stable QTLs and functional genes. Discovering QTLs and genes is the first step toward understanding the molecular basis of yield and a necessary step for establishing efficient marker-assisted breeding technology and conducting gene identification and editing.
With the recent advancement in genomic tools and DNA sequencing, crop production, yield-related traits, and their respective genomic location, can be identified more precisely and faster (Ravelombola et al., 2021). Several genes governing the soybean’s productivity or seed yield have been identified to date. For example, the BIG SEEDS1 (BS1) gene involved in controlling SS and SW has been reported and described in Medicago truncatula and soybean (Ge et al., 2016), where it is also involved in monitoring the size of pods and leaves. Similarly, GmCYP78A10b regulates the SS, width, and thickness in soybean with 7.2% of the variation in the SW (Wang et al., 2015b). GmCYP78A72 is involved in the increase in SS (Zhao et al., 2016), GmCYP78A5 regulates SS and weight (Du et al., 2017b), and the expression of GmPSKγ1 increases SS and yield (Yu et al., 2019). GmSWEET10a (Glyma.15G049200) is related to SS increase and has a positive relation with the oil content but a negative relation with the protein content of the seeds (Wang et al., 2020). Genes located in the ST1 locus, mainly Glyma.08g109100, affect the seed thickness and increase the oil content of the seeds through the pectin biosynthesis pathway (Li et al., 2022a). GmSSS1, located on chromosome 19, is related to increased SS (Zhu et al., 2022), and GmGA3ox1, encoding the gibberellin synthesis pathway enhanced the photosynthesis, resulting in increased SW (Huang et al., 2022). Similarly, Lu et al. (2017) reported that GmBZR1 in transgenic soybean, associated with the PP2C-1 allele, increases SW and SS by facilitating the accumulation of dephosphorylated GmBZR1(Lu et al., 2017). Recently, a novel SS gene, Glyma.08G309000—named Novel Seed Size (NSS)—was found to control seed development in soybean (Zhang et al., 2023). The major genes related to the soybean seed yield and associated traits are listed in Table 3.
Soybean SS is an important trait in crop yield. Increasing soybean yield per unit area is a primary breeding objective and a challenging task for the scientific community. Soybean SS, SW, and its protein and oil content are always been a priority of plant breeders to improve where significant improvements have been noted throughout time, and still going on, resulting in better seeds; phenotypically and nutritionally. Typically, plant seeds are composed of three essential parts; seed coat, diploid embryo, and triploid endosperm, which regulate seed development and subsequently determine SS (Garcia et al., 2005; Haughn and Chaudhury, 2005). The endosperm and embryo of dicots like Arabidopsis and soybean proliferate during seed development, and this pattern continues until the seed matures and desiccates (Figure 3). The maternal ovule simultaneously experiences controlled development to provide room for the developing embryo and endosperm, and its integuments eventually become the mature seed’s coat at a later developmental stage (Figure 3). The seed size directly correlates with the endosperm’s cell division (Garcia et al., 2005). Plant signaling mechanisms that control SS include the ubiquitin-protease route, mitogen-activated protein kinase (MAPK) signaling, transcriptional control, sugar, G protein signaling, HAIKU (IKU) pathways, and plant hormone signaling (Ruan et al., 2012; Li et al., 2019b; Pandey, 2019). Based on previous studies, we have briefly illustrated the major regulatory networks involved during seed development, ultimately determining the soybean SS.
Figure 3 Illustrations of different stages and processes of soybean seed development from the globular to the mature seed stage. Globular stage to early maturation stage images adapted from http://seedgenenetwork.net/soybean (Images of globular to early maturation stage were hand-drawn by Sharon Lee Belkin (Morphographics).
Besides its ultimate importance, the molecular processes underpinning soybean SS, shape, and weight are poorly understood. Nevertheless, several soybean genes have been identified and functionally characterized to be involved in regulating SS and SW (Table 3). Among them, some genes are identified for embryo and endosperm development, seed coat, and plant hormones, and others are related to different seed developmental stages, all of which are essential in the regulation of soybean SS (Figure 4).
Figure 4 Overview of a regulatory network that determines soybean seed size (SS). The soybean SS determination mainly involves the ubiquitin-protease factors, mitogen-activated protein kinase (MAPK) signaling, G protein, transcriptional, carbohydrate, or sugar regulation, HAIKU (IKU) pathways, and plant hormone regulation. Based on the literature, key genes involved in SS regulation are explained in section 3. The green lines represent the activities in seed coat development; the black lines indicate the development of embryo/endosperm; the red represents the hormonal regulations; and the gray line demonstrates the regulation of an unknown location.
Although the exact mechanism and pathways regulating seed size are poorly studied and widely unexplored, though some transcriptional regulators that can control SS by regulating embryo development have been reported. For example, BIG SEEDS1 (BS1)belongs to a group II member of the TIFY TF family and encodes the plant-specific transcriptional regulator TIFY, which plays a vital role in controlling the size of seeds, pods, and leaves via a regulatory module that targets cell proliferation (Ge et al., 2016). Ge et al. (2016) reported increased SS when the soybean BS1 homolog is suppressed or downregulated. The study also indicated that in Medicago truncatula big seeds1-1 (mtbs1-1) mutants, the expression of GIF1, GROWTH REGULATING FACTOR 5 (GRF5), and core cell cycle genes were upregulated, and the embryo size was significantly increased. These results indicate that BS1 is a negative regulator related to embryonic cell proliferation. Similarly, the IKU pathway mainly regulates early endosperm development and includes the IKU1, IKU2, MINISEED3 (MINI3), and SHORT HYPOCOTYL UNDER BLUE1 (SHB1) genes which reduced endosperm growth and small seeds. As IKU1 encodes a protein containing the plant-specific VQ motif and is expressed in early endosperm and in the central cell, which thereby it was consider an essential regulatory element for seed size regulation, where the IKU2 encoded a leucine-rich repeat (LRR) kinase, and its overexpression led to an increase in seed size, weight, and oil content (Fatihi et al., 2013). Additionally, loss of function of OsNAC129 was found to significantly increase grain length, weight, apparent amylose content (AAC), and plant height, while overexpression of OsNAC129 had the opposite effect. The expression of OsNAC129 was linked to induced by ABA, and overexpression of OsNAC129 in plants reduced sensitivity to exogenous BR, thereby indicating that overexpression of OsNAC129 negatively regulates seed development and plant growth and participates in the BR signaling pathway (Jin et al., 2022). In addition, studies demonstrated that the DREB-type transcription factor gene GmDREBL is essential for seed oil accumulation (Zhang et al., 2016b). GmDREBL is located in the nucleus and can activate transcription. It can bind to the promoter region of WR1 to activate its expression. An overexpression study of GmDREBL in Arabidopsis indicated a specificity in stamens, embryos, and cotyledon organs and a significant increase in SS. The average expression of DREBL in cultivated soybean was higher than in wild soybean, suggesting that the trait might have been artificially selected to increase yield and oil content during soybean domestication (Zhang et al., 2016b). In addition to the myeloblastosis (MYB) family in this context, recent reviews and references describe the transcriptional factors regulating SS in Arabidopsis and other crops (Dwivedi et al., 2021; Alam et al., 2022).
Some genes affect seed development by regulating the synthesis and metabolism of plant hormones. For example, the Arabidopsis AtGA20OX and its soybean homolog GmGA20OX encode the gibberellin oxidase GA-20. GmGA20OX, located in the soybean SW locus, was significantly correlated with HSW (Lu et al., 2016). As a multifunctional enzyme, GA20OX regulates gibberellin (GA) synthesis and metabolism, which are crucial in reproductive and physiological processes (Lu et al., 2016). Similarly, (Shi et al., 2020) Shi et al. (2020), successfully cloned GW6 (GRAIN WIDTH 6), a QTL affecting grain size, which positively influenced grain size and weight, and was encoded as GA-induced GAST family protein. Additionally, the GA content of young panicles was decreased by GW6 knockout. GW6’s transcript abundance, grain breadth, and grain weight are all influenced by a natural variation in the CAAT-box of the promoter. Transgenic plants overexpressing Arabidopsis GA2-oxidase gene (AtGA2ox8) in Brassica napus L. were shown to exhibit a significant increase in seed yield by 9.6–12.4% (Zhou et al., 2012). Gibberellic acid-stimulated Arabidopsis 4 (GASA4) is one of the 14 members of the small polypeptide family in Arabidopsis, regulating flowering and seed development and affecting seed size, weight, and yield. Furthermore, auxin is a crucial traditional phytohormone that plays crucial roles in a variety of processes related to plant growth and development, including grain size. Indole-3-acetic acid (IAA)-glucose hydrolase is encoded by qTGW6, which is a major QTL regulating rice grain weight. This enzyme produces free IAA. According to (Ishimaru et al., 2013), a loss-of-function TGW6 allele improved grain length and weight. TGW6 may be crucial in controlling pollen development, according to recent research that found it to be primarily expressed in pre-emergent inflorescences (Akabane et al., 2021; Kabir and Nonhebel, 2021). Auxin transport is regulated by a protein that is encoded by the auxin major response gene BG1. By encouraging cell division and elongation, it influences grain size (Liu et al., 2015) (Liu et al., 2015a). According to Hu et al. (2018) qTGW3/GL3.3, which codes for the SHAGGY-like kinase 41 (OsSK41), is a significant QTL for grain weight. A transcription repressor in the auxin pathway called OsARF4 is directly interacted with by OsSK41 and phosphorylated by it. ABA regulates various aspects of plant growth and development as well as responses to abiotic stress (Shi et al., 2021). LOS5/ABA3 is involved in ABA biosynthesis by encoding molybdenum co-factor sulfurase, which is required by aldehyde oxidase (AO) in the last step of ABA biosynthesis in plants. Transgenic plants overexpressing LOS5/ABA3 have been reported to show at least a 21% increase in seed yield compared to the wild type (WT) under drought stress conditions (Li et al., 2013b). OsAO3 is essential for the regulation of grain yield in rice, since osao3 mutant increases grain yield, while overexpression of OsAO3 reduces grain yield by affecting panicle number per plant, spikelet number per panicle, and spikelet fertility (Shi et al., 2021). Cytosolic ABA receptors PYRABACTIN RESISTANCE 1 IKE/REGULATORY COMPONENTS OF ABA RECEPTORS (PYL/RCARs) can regulate ABA-dependent gene expression in rice (Kim et al., 2014). Manan and Zhao (2020) reported that Glycine max ABSCISIC ACID INSENSETIVE 3 (GmABI3) is involved in lipid biosynthesis. The expression of GmABI3 under environmental stress (heat and cold) and hormal stress (ABA and methyl jasmonate) was also studied. This research also identified a 34.9% increase in triacylglycerol (TAG) levels in transgenic GmABI3/wildtype seeds when compared to regular wildtype seeds. Furthermore, it revealed that this specific gene was accountable for producing long-chain fatty acids and generating TAG in a seed-specific manner. Constitutive expression of OsPYL/RCAR5 slightly reduces plant height and severely decreases seed yield under paddy field conditions, although abiotic stress tolerance is improved. Similarly, soybean Glyma.02G115900 regulates multiple action pathways of plant hormones by affecting 14-3-3. Such pathways are complex and need further exploration and research. Recently, GmGA30x1, which encodes a gibberellin 3β-hydroxylase, displayed a positive SW and length regulation. The mutant gmga30x1 displayed a reduced bioactive GA production and an enhanced net photosynthesis rate and Rubisco activity, leading to a reduced SW and an increased seed yield—through an increased seed number (Hu et al., 2022). The polyhydroxylated steroidal hormones known as BRs, which are specific to plants, regulate a variety of growth and developmental processes, including grain size (Li et al., 2018). As GSK2, which is a key negative regulator of the BR pathway, and a homolog of BIN2 in the GSK3/SHAGGY kinases family. As a result, increasing grain size and leaf angles were achieved by decreasing GSK2 expression (Tong et al., 2012). The main QTL for grain width, GW5, is composed of three haplotypes (Zhou et al., 2017a). The transcription factors OsBZR1 and DLT are released into the active state by GW5, which participates in the BR pathway by inhibiting GSK2 kinase activity and regulates grain width and weight (Liu et al., 2017a). Similarly, (Tang et al., 2017) reported that two invertase inhibitors, GmCIF1 and GmC/VIF2, had inhibitory activity in vitro through heterologous expression. Transcript analysis revealed that they were predominantly expressed in developing seeds and the ABA response. The silencing of GmCIF1 significantly increased the CWI activity of mature seeds through processes that fine-tuned sucrose metabolism and pool strength and increased SW, hexose, starch, and protein accumulation (Tang et al., 2017), indicating a role for GmCIF1 in the negative regulation of soybean SW. Other genes in soybean can affect SS by regulating other metabolites. For example, GmFAD3, an omega-3 fatty acid desaturase (FAD3), is involved in the biosynthesis of fatty acid and jasmonic acid (JA). A study indicated that gmfad3-RNAi silenced plants accumulate higher levels of JA, thereby increasing the susceptibility of soybean to bean pod mottle virus and producing larger and heavier seeds (Singh et al., 2010)
Cultivated soybeans were successively domesticated from wild soybeans and generally displayed larger SS. Understanding the differences between cultivated and wild soybean traits, especially at the genomic level, can lead to genetic and cultivation improvement. In addition to the abovementioned regulatory pathways and genes, other genes are involved in regulating SS in soybean. For example, the expression level of SoyWRKY15a in wild soybean populations was positively correlated with SS (Gu et al., 2017). The coding sequences of GmWRKY15a (cultivated) and GsWRKY15a (wild) are identical, but the number of CT repeats in the 5’ untranslated region (5’UTR) is different, causing haplotype and, ultimately, SS variation (Gu et al., 2017). Previously, the regulatory role of WRKY transcription factors TRANSPARENT TESTA GLABRA 2 (TTG2) and MINISEED 3 (MINI3) in SS was demonstrated in Arabidopsis (Garcia et al., 2005; Li et al., 2013a). The loss of TTG2 function in ttg2 mutant plants causes impaired elongation of epidermal cells, impacting the endosperm and seed development (Garcia et al., 2005). Nonetheless, MINI3 can bind to the cytokinin oxidase 2 (CKX2) promoter, activate CKX2 expression, and control endosperm growth (Li et al., 2013a). However, further research is needed to fully understand the role of SoyWRKY15 in SS and development.
A few transgenic studies have also provided evidence about genes influencing the SS. For example, Wang et al. (2007) studied soybean Dof-type transcription factor genes and found that transgenic Arabidopsis plants expressing GmDof4 and GmDof11 had slightly larger SS and SW and increased levels of lipid content compared to Col-0 (wild-type) plants, indicating that GmDof4 and GmDof11 affect soybean SW (Wang et al., 2007), and have a potential application value. Liu et al. (2012) introduced an inositol polyphosphate kinase gene from halophilic bacteria (ThIPK2) to soybean through Agrobacterium-mediated transformation, and the transformed soybean plants significantly improved their SS and stress resistance.
In summary, soybean seed size is regulated by multiple pathways and involves several molecular networks during seed development. An in-depth research is required to identify molecular mechanisms of SS development. However, based on recent advances at the molecular level, we illustrated a regulatory network that plays a role in the development of soybean SS. This information may be helpful in describing soybean SS and may open new opportunities for developing new soybean varieties with higher yield potential.
Soybean is one of the most important crops globally, and its yield has increased over the years but has become stagnant in the past few years. Challenges remain to be addressed to sustainably improve soybean yield and related traits. Moreover, the molecular mechanisms driving the fundamental biological processes involved in yield traits remain mostly unknown. Several studies using bi-parental mapping and association mapping identified the number of QTLs and QTNs for the soybean seed yield and related traits, and some of it has already been integrated into the SoyBase. However, a significant approach is lacking to successfully utilize identified QTLs by marker-assisted selection or breeding, because of the quantitative nature of the trait where a huge number of minor QTLs contribute to it which is difficult comparatively to the traits where one or few putative genes are involved, increasing predicament in molecular breeding. Previous studies comparatively used the low-density marker (SSR), confining low resolution and higher confidence interval for discovered SS/SW; other seed yield traits QTLs have not mined the causing candidate genes (Panthee et al., 2005; Gai et al., 2007; Kato et al., 2014; Kulkarni et al., 2016). Considering the complexity of traits and low inheritance of associated traits, it is challenging to detect stable QTLs. Moreover, few studies reported stable QTLs across the tested environment for soybean seed yield traits (Cui et al., 2020; Hina et al., 2020; Kumawat and Xu, 2021). Furthermore, validation of these identified and stable or previously reported QTLs in different genetic backgrounds is challenging, and very limited efforts have been made toward it. Several stable yield QTLs and genes have been identified and cloned in other crop species, such as rice (Xing and Zhang, 2010; He et al., 2022). However, progress toward functional characterization of seed yield-related QTLs or genes is lagging in soybean. Functional characterization of QTLs or genes reported for yield traits to develop high-yielding crops will be simpler if we can comprehend the genetic basis of each attribute and the regulatory network of seed yield traits (Tian et al., 2021; Zhang et al., 2022). Thus, perspectives to improve soybean seed yield should include the following steps. (1) In the soybean breeding program, effort must be given for the precise detection of phenotypic variation, selection or creation of genetically diverse resources, identification of stable QTLs or QTNs, and validation into diverse backgrounds. (2) Researchers should focus on understanding and identifying the genetics of soybean yield and related component traits using the high density and high-throughput markers (SNP) to further discover the candidate genes for traits. (3) These markers could then be used to develop molecular breeding tools that enable breeders to select plants and even perform the prediction breeding for desirable yield-related traits. (4) Studies should be conducted on soybean seed yield epigenetic changes, which may lead to finding ways to manipulate these changes to increase yield. Moreover, epigenetic changes can be influenced by environmental factors and profoundly impact plant growth and development. (5) In addition to genomics studies, transcriptomic and proteomic studies are needed for soybean seeds to identify genes and proteins that play key roles in seed development and yield. This information can be used to develop targeted breeding strategies or to engineer soybean plants to express specific genes or proteins that enhance seed yield. (6) Efforts need to be put in for the functional characterization of more genes associated with seed yield-related traits. (7) In addition to QTLs or genes responsible for key yield traits (SS, SW, and HSW), other traits like plant architecture (which is not discussed in this review), plant height, number of branching, number of internodes, NPP, number of seeds per pod, petiole angle, petiole length, and leaf size must be isolated and characterized using functional genomic investigations. The use of CRISPR/Cas9 technology for gene characterization for mentioned traits, where help can be taken from specific databases like SoyBase, could be a way forward to speed up the process. The CRISPR/Cas9 method has become a powerful tool with numerous uses in the areas of reverse genetics and crop enhancement. This pioneering technique has the potential to greatly improve the economic value and sustainability of crops in the face of biotic and abiotic stresses by precisely targeting specific genetic characteristics. At present, the CRISPR/Cas9 gene editing system has been successfully used in around 20 crop species, allowing for the enhancement of various desirable traits such as yield and resistance to biotic and abiotic stresses. In the last five years, major progress in genome editing research has mainly been made on important crops such as rice, wheat, maize, and soybean (Chaudhary et al., 2022) where focusing on the important yield related traits such as pod number, number of seeds, SS, SW, can bring enormous improvements in the crop yield. (8) Timely selection, introgression of superior yield traits, and pyramiding of high-yielding genes and other plant attributes may aid in developing a soybean cultivar with a significant yield increase. Besides genetic and genomic approaches, to combat the effects of climate change, disease, and pests on yield, new approaches are required, such as creating climate- and disease-resistant soybean cultivars, enhancing soil health, and implementing artificial intelligence for sustainable agriculture methods. Future soybean breeding efforts should prioritize the development of high-yielding varieties with enhanced agronomic traits to maximize soybean productivity. Breeding programs should aim to improve traits such as increased seed yield per plant, optimized seed size and weight, enhanced branching and podding characteristics, and improved stress tolerance. These advancements would contribute to the overall goal of achieving higher soybean yields and ensuring economic viability for soybean growers. Additionally, it is essential to emphasize the development of soybean varieties with improved resistance to biotic and abiotic stresses, including pests, diseases, drought, and temperature fluctuations. By incorporating traits associated with resistance and stress tolerance into breeding programs, breeders can mitigate yield losses caused by these challenges and improve overall crop performance. Furthermore, future breeding efforts should focus on harnessing the potential of emerging technologies, such as CRISPR/cas9 and marker-assisted breeding, to accelerate the breeding process and enhance precision in selecting desirable yield-related traits. This integration of cutting-edge tools and techniques can expedite the development of high-yielding soybean varieties with superior agronomic performance. By prioritizing the breeding objectives related to increasing soybean yield and yield-related traits, breeders can contribute to meeting the growing global demand for soybean products, ensuring food security, and promoting the economic prosperity of soybean production.
RT, MI, wrote the manuscript. WK, AG, and RS prepared illustrations, figures, tables, and references. YK contributed critical comments to the draft. RT and YK conceptualized. YK critically edited, and approved the manuscript. All authors contributed to the article and approved the submitted version.
This research was supported by biological materials Specialized Graduate Program through the Korea Environmental Industry & Technology Institute (KEITI) funded by the Ministry of Environment (MOE). This research was supported by the Korea Basic Science Institute (National Research Facilities and Equipment Center) grant funded by the Ministry of Education(2021R1A6C101A416) and Basic Science Research Program through the National Research Foundation of Korea (NRF) funded by the Ministry of Education (2021R1I1A3040280). Whole genome analysis was done at KNU NGS center (Daegu, South Korea).
The authors declare that the research was conducted in the absence of any commercial or financial relationships that could be construed as a potential conflict of interest.
All claims expressed in this article are solely those of the authors and do not necessarily represent those of their affiliated organizations, or those of the publisher, the editors and the reviewers. Any product that may be evaluated in this article, or claim that may be made by its manufacturer, is not guaranteed or endorsed by the publisher.
Adebisi, M., Kehinde, T., Salau, A., Okesola, L. A., Porbeni, J. B. O., Esuruoso, A. O., et al. (2013). Influence of different seed size fractions on seed germination, seedling emergence and seed yield characters in tropical soybean (Glycine max L. Merrill). Int. J. Agric. Res. 8, 26–33. doi: 10.3923/ijar.2013.26.33
Afzal, M., Alghamdi, S. S., Migdadi, H. H., Khan, M. A., Nurmansyah, Mirza, S. B., et al. (2020). Legume genomics and transcriptomics: From classic breeding to modern technologies. Saudi. J. Biol. Sci. 27, 543–555. doi: 10.1016/j.sjbs.2019.11.018
Akabane, T., Suzuki, N., Tsuchiya, W., Yoshizawa, T., Matsumura, H., Hirotsu, N., et al. (2021). Expression, purification and crystallization of TGW6, which limits grain weight in rice. Protein. Expr. Purif 188, 105975. doi: 10.1016/j.pep.2021.105975
Alam, I., Batool, K., Huang, Y., Liu, J., Ge, L. (2022). Developing Genetic Engineering Techniques for Control of Seed Size and Yield. Int. J. Mol. Sci. 23, 13256. doi: 10.3390/ijms232113256
Alonso-Blanco, C., Blankestijn-De Vries, H., Hanhart, C. J., Koornneef, M. (1999). Natural allelic variation at seed size loci in relation to other life history traits of Arabidopsis thaliana. Proc. Natl. Acad. Sci. U S. A 96, 4710–4717. doi: 10.1073/pnas.96.8.4710
Ambika, S., Velusamy, M., Somasundar, G. (2014). ). Review on effect of seed size on seedling vigour and seed yield. Res. J. Seed Sci. 7, 31–38. doi: 10.3923/rjss.2014.31.38
Attipoe, J. Q., Khan, W., Tayade, R., Steven, S., Islam, M. S., Lay, L., et al. (2023). Evaluating the effectiveness of calcium silicate in enhancing soybean growth and yield. Plants (Basel) 12, 2190. doi: 10.3390/plants12112190
Ayalew, H., Schapaugh, W., Vuong, T., Nguyen, H. T. (2022). Genome-wide association analysis identified consistent QTL for seed yield in a soybean diversity panel tested across multiple environments. Plant Genome 15, e20268. doi: 10.1002/tpg2.20268
Ben Hassen, T., El Bilali, H. (2022). Impacts of the Russia-Ukraine war on global food security: towards more sustainable and resilient food systems? Foods. 11, 2301. doi: 10.3390/foods11152301
Bian, X. H., Li, W., Niu, C. F., Wei, W., Hu, Y., Han, J. Q., et al. (2020). A class B heat shock factor selected for during soybean domestication contributes to salt tolerance by promoting flavonoid biosynthesis. New Phytol. 225, 268–283. doi: 10.1111/nph.16104
Bohra, A., Tiwari, A., Kaur, P., Ganie, S. A., Raza, A., Roorkiwal, M., et al. (2022). The key to the future lies in the past: insights from grain legume domestication and improvement should inform future breeding strategies. Plant Cell Physiol. 63, 1554–1572. doi: 10.1093/pcp/pcac086
Caldwell, B. E., Howell, R. W. (1973)Soybeans: Improvement, production, and uses. Am. Soc. Agron. Madison.
Campbell, B. W., Hofstad, A. N., Sreekanta, S., Fu, F., Kono, T. J., O'rourke, J. A., et al. (2016). Fast neutron-induced structural rearrangements at a soybean NAP1 locus result in gnarled trichomes. Theor. Appl. Genet. 129, 1725–1738. doi: 10.1007/s00122-016-2735-x
Chaudhary, M., Mukherjee, T. K., Singh, R., Gupta, M., Goyal, S., Singhal, P., et al. (2022). CRISPR/Cas technology for improving nutritional values in the agricultural sector: an update. Mol. Biol. Rep. 49, 7101–7110. doi: 10.1007/s11033-022-07523-w
Chen, L., Cai, Y. P., Liu, X. J., Yao, W. W., Guo, C., Sun, S., et al. (2018). Improvement of soybean Agrobacterium-mediated transformation efficiency by adding glutamine and asparagine into the culture media. Int. J. Mol. Sci. 19, 3039. doi: 10.3390/ijms19103039
Chen, L., Cai, Y., Qu, M., Wang, L., Sun, H., Jiang, B., et al. (2020a). ). Soybean adaption to high-latitude regions is associated with natural variations of GmFT2b, an ortholog of FLOWERING LOCUS T. Plant Cell Environ. 43, 934–944. doi: 10.1111/pce.13695
Chen, R., Deng, Y., Ding, Y., Guo, J., Qiu, J., Wang, B., et al. (2022). Rice functional genomics: decades' efforts and roads ahead. Sci. China Life Sci. 65, 33–92. doi: 10.1007/s11427-021-2024-0
Chen, L., Nan, H., Kong, L., Yue, L., Yang, H., Zhao, Q., et al. (2020b). Soybean AP1 homologs control flowering time and plant height. J. Integr. Plant Biol. 62, 1868–1879. doi: 10.1111/jipb.12988
Contreras-Soto, R. I., Mora, F., de Oliveira, M. A. R, Higashi, W., Scapim, C. A., Schuster, I. (2017). A genome-wide association study for agronomic traits in soybean using SNP markers and SNP-based haplotype analysis. PloS One 12, e0171105. doi: 10.1371/journal.pone.0171105
Copley, T. R., Duceppe, M.-O., O’donoughue, L. S. (2018). Identification of novel loci associated with maturity and yield traits in early maturity soybean plant introduction lines. BMC Genomics 19, 167. doi: 10.1186/s12864-018-4558-4
Cui, B., Chen, L., Yang, Y., Liao, H. (2020). Genetic analysis and map-based delimitation of a major locus qSS3 for seed size in soybean. Plant Breed. 139, 1145–1157. doi: 10.1111/pbr.12853
Du, J., Wang, S., He, C., Zhou, B., Ruan, Y. L., Shou, H. (2017b). Identification of regulatory networks and hub genes controlling soybean seed set and size using RNA sequencing analysis. J. Exp. Bot. 68, 1955–1972. doi: 10.1093/jxb/erw460
Duan, P., Li, Y. (2021). Size matters: G protein signaling is crucial for grain size control in rice. Mol. Plant 14, 1618–1620. doi: 10.1016/j.molp.2021.08.010
Duan, Z., Zhang, M., Zhang, Z., Liang, S., Fan, L., Yang, X., et al. (2022). Natural allelic variation of GmST05 controlling seed size and quality in soybean. Plant Biotechnol. J. 20, 1807–1818. doi: 10.1111/pbi.13865
Dwivedi, S. L., Spillane, C., Lopez, F., Ayele, B. T., Ortiz, R. (2021). First the seed: Genomic advances in seed science for improved crop productivity and food security. Crop Sci. 61, 1501–1526. doi: 10.1002/csc2.20402
Fang, C., Li, W., Li, G., Wang, Z., Zhou, Z., Ma, Y., et al. (2013). Cloning of Ln gene through combined approach of map-based cloning and association study in soybean. J. Genet. Genomics 40, 93–96. doi: 10.1016/j.jgg.2013.01.002
Fang, C., Li, C., Li, W., Wang, Z., Zhou, Z., Shen, Y., et al. (2014). Concerted evolution of D1 and D2 to regulate chlorophyll degradation in soybean. Plant J. 77, 700–712. doi: 10.1111/tpj.12419
Fang, C., Ma, Y., Wu, S., Liu, Z., Wang, Z., Yang, R., et al. (2017). Genome-wide association studies dissect the genetic networks underlying agronomical traits in soybean. Genome Biol. 18, 161. doi: 10.1186/s13059-017-1289-9
Fatihi, A., Zbierzak, A. M., Dörmann, P. (2013). Alterations in seed development gene expression affect size and oil content of Arabidopsis seeds. Plant Physiol. 163, 973–985. doi: 10.1104/pp.113.226761
Gai, J., Wang, Y., Wu, X., Chen, S. (2007). A comparative study on segregation analysis and QTL mapping of quantitative traits in plants—with a case in soybean. Front. Agric. China 1, 1–7. doi: 10.1007/s11703-007-0001-3
Gao, H., Wang, Y., Li, W., Gu, Y., Lai, Y., Bi, Y., et al. (2018). Transcriptomic comparison reveals genetic variation potentially underlying seed developmental evolution of soybeans. J. Exp. Bot. 69, 5089–5104. doi: 10.1093/jxb/ery291
Garcia, D., Fitz Gerald, J. N., Berger, F. D. R. (2005). Maternal control of integument cell elongation and zygotic control of endosperm growth are coordinated to determine seed size in Arabidopsis. Plant Cell. 17, 52–60. doi: 10.1105/tpc.104.027136
Ge, L., Yu, J., Wang, H., Luth, D., Bai, G., Wang, K., et al. (2016). Increasing seed size and quality by manipulating BIG SEEDS1 in legume species. Proc. Natl. Acad. Sci. 113, 12414–12419. doi: 10.1073/pnas.1611763113
Goettel, W., Zhang, H., Li, Y., Qiao, Z., Jiang, H., Hou, D., et al. (2022). POWR1 is a domestication gene pleiotropically regulating seed quality and yield in soybean. Nat. Commun. 13, 3051. doi: 10.1038/s41467-022-30314-7
Gu, Y., Li, W., Jiang, H., Wang, Y., Gao, H., Liu, M., et al. (2017). Differential expression of a WRKY gene between wild and cultivated soybeans correlates to seed size. J. Exp. Bot. 68, 2717–2729. doi: 10.1093/jxb/erx147
Guo, W., Chen, L., Chen, H., Yang, H., You, Q., Bao, A., et al. (2020). Overexpression of GmWRI1b in soybean stably improves plant architecture and associated yield parameters, and increases total seed oil production under field conditions. Plant Biotechnol. J. 18, 1639–1641. doi: 10.1111/pbi.13324
Han, Y., Li, D., Zhu, D., Li, H., Li, X., Teng, W., et al. (2012). QTL analysis of soybean seed weight across multi-genetic backgrounds and environments. Theor. Appl. Genet. 125, 671–683. doi: 10.1007/s00122-012-1859-x
Han, Y., Zhao, X., Liu, D., Li, Y., Lightfoot, D. A., Yang, Z., et al. (2016). Domestication footprints anchor genomic regions of agronomic importance in soybeans. New Phytol. 209, 871–884. doi: 10.1111/nph.13626
Haughn, G., Chaudhury, A. (2005). Genetic analysis of seed coat development in Arabidopsis. Trends Plant Sci. 10, 472–477. doi: 10.1016/j.tplants.2005.08.005
He, N., Zhan, G., Huang, F., Abou-Elwafa, S. F., Yang, D. (2022). Fine mapping and cloning of a major qtl qph12, which simultaneously affects the plant height, panicle length, spikelet number and yield in rice (Oryza sativa L.). Front. Plant Sci. 13. doi: 10.3389/fpls.2022.878558
Hina, A., Cao, Y., Song, S., Li, S., Sharmin, R. A., Elattar, M. A., et al. (2020). High-resolution mapping in two ril populations refines major “QTL Hotspot” regions for seed size and shape in soybean (Glycine max L.). Int. J. Mol. Sci. 21, 1040. doi: 10.3390/ijms21031040
Hoeck, J. A., Fehr, W. R., Shoemaker, R. C., Welke, G. A., Johnson, S. L., Cianzio, S. R. (2003). Molecular marker analysis of seed size in soybean. Crop Sci. 43, 68–74. doi: 10.2135/cropsci2003.6800
Hu, D., Li, X., Yang, Z., Liu, S., Hao, D., Chao, M., et al. (2022). Downregulation of a gibberellin 3β-hydroxylase enhances photosynthesis and increases seed yield in soybean. New Phytol. 235, 502–517. doi: 10.1111/nph.18153
Hu, Z., Lu, S.-J., Wang, M.-J., He, H., Sun, L. E., Wang, H., et al. (2018). A novel QTL qTGW3 encodes the GSK3/SHAGGY-like kinase OsGSK5/OsSK41 that interacts with OsARF4 to negatively regulate grain size and weight in rice. Mol. Plant 11 (5), 736–749. doi: 10.1016/j.molp.2018.03.005
Hu, D., Zhang, H., Du, Q., Hu, Z., Yang, Z., Li, X., et al. (2020). Genetic dissection of yield-related traits via genome-wide association analysis across multiple environments in wild soybean (Glycine soja Sieb. and Zucc.). Planta 251, 1–17. doi: 10.1007/s00425-019-03329-6
Hu, Z., Zhang, H., Kan, G., Ma, D., Zhang, D., Shi, G., et al. (2013). Determination of the genetic architecture of seed size and shape via linkage and association analysis in soybean (Glycine max L. Merr.). Genetica. 141, 247–254. doi: 10.1007/s10709-013-9723-8
Huang, F., Tian, Z., Yu, D. (2022). Downregulation of a gibberellin 3b-hydroxylase enhances photosynthesis and increases seed yield in soybean. New Phytol. 1, 502–517. doi: 10.1111/nph.18153
Hudson, K. (2022). Soybean protein and oil variants identified through a forward genetic screen for seed composition. Plants. 11, 2966. doi: 10.3390/plants11212966
Hwang, E.-Y., Song, Q., Jia, G., Specht, J. E., Hyten, D. L., Costa, J., et al. (2014). A genome-wide association study of seed protein and oil content in soybean. BMC Genom. 15, 1. doi: 10.1186/1471-2164-15-1
Hymowitz, T. (1970). On the domestication of the soybean. Econ. Bot. 24, 408–421. doi: 10.1007/BF02860745
Ishimaru, K., Hirotsu, N., Madoka, Y., Murakami, N., Hara, N., Onodera, H., et al. (2013). Loss of function of the IAA-glucose hydrolase gene TGW6 enhances rice grain weight and increases yield. Nat. Genet. 45, 707–711. doi: 10.1038/ng.2612
Jeong, N., Moon, J. K., Kim, H. S., Kim, C. G., Jeong, S. C. (2011). Fine genetic mapping of the genomic region controlling leaflet shape and number of seeds per pod in the soybean. Theor. Appl. Genet. 122, 865–874. doi: 10.1007/s00122-010-1492-5
Jeong, N., Suh, S. J., Kim, M. H., Lee, S., Moon, J. K., Kim, H. S., et al. (2012). Ln is a key regulator of leaflet shape and number of seeds per pod in soybean. Plant Cell. 24, 4807–4818. doi: 10.1105/tpc.112.104968
Jin, S. K., Zhang, M. Q., Leng, Y. J., Xu, L. N., Jia, S. W., Wang, S. L., et al. (2022). OsNAC129 regulates seed development and plant growth and participates in the brassinosteroid signaling pathway. Front. Plant Sci. 13, 905148. doi: 10.3389/fpls.2022.905148
Jing, Y., Zhao, X., Wang, J., Teng, W., Qiu, L., Han, Y., et al. (2018). Identification of the genomic region underlying seed weight per plant in soybean (Glycine max l. merr.) via high-throughput single-nucleotide polymorphisms and a genome-wide association study. Front. Plant Sci. 9. doi: 10.3389/fpls.2018.01392
Jo, H., Lee, J. Y., Lee, J.-D. (2022). Genome-wide association mapping for seed weight in soybean with black seed coats and green cotyledons. Agronomy 12, 250. doi: 10.3390/agronomy12020250
Kabir, M. R., Nonhebel, H. M. (2021). Reinvestigation of THOUSAND-GRAIN WEIGHT 6 grain weight genes in wheat and rice indicates a role in pollen development rather than regulation of auxin content in grains. Theor. Appl. Genet. 134, 2051–2062. doi: 10.1007/s00122-021-03804-3
Kaler, A. S., Gillman, J. D., Beissinger, T., Purcell, L. C. (2020). Comparing different statistical models and multiple testing corrections for association mapping in soybean and maize. Front. Plant Sci. 1794. doi: 10.3389/fpls.2019.01794
Kanai, M., Yamada, T., Hayashi, M., Mano, S., Nishimura, M. (2019). Soybean (Glycine max L.) triacylglycerol lipase GmSDP1 regulates the quality and quantity of seed oil. Sci. Rep. 9, 8924. doi: 10.1038/s41598-019-45331-8
Karikari, B., Chen, S., Xiao, Y., Chang, F., Zhou, Y., Kong, J., et al. (2019). Utilization of interspecific high-density genetic map of RIL population for the QTL detection and candidate gene mining for 100-seed weight in soybean. Front. Plant Sci. 10. doi: 10.3389/fpls.2019.01001
Kato, S., Sayama, T., Fujii, K., Yumoto, S., Kono, Y., Hwang, T.-Y., et al. (2014). A major and stable QTL associated with seed weight in soybean across multiple environments and genetic backgrounds. Theor. Appl. Genet. 127, 1365–1374. doi: 10.1007/s00122-014-2304-0
Kim, H., Lee, K., Hwang, H., Bhatnagar, N., Kim, D. Y., Yoon, I. S., et al. (2014). ). Overexpression of PYL5 in rice enhances drought tolerance, inhibits growth, and modulates gene expression. J. Exp. Bot. 65, 453–464. doi: 10.1093/jxb/ert397
Knizia, D., Yuan, J., Bellaloui, N., Vuong, T., Usovsky, M., Song, Q., et al. (2021). The soybean high density ‘Forrest’ by ‘Williams 82’ SNP-based genetic linkage map identifies QTL and candidate genes for seed isoflavone content. Plants. 10, 2029. doi: 10.3390/plants10102029
Kulkarni, K. P., Kim, M., Shannon, J. G., Lee, J.-D. (2016). Identification of quantitative trait loci controlling soybean seed weight in recombinant inbred lines derived from PI 483463 (Glycine soja) × ‘Hutcheson’ (G. max). Plant Breed. 135, 614–620. doi: 10.1111/pbr.12407
Kumar, R., Saini, M., Taku, M., Debbarma, P., Mahto, R. K., Ramlal, A., et al. (2022). Identification of quantitative trait loci (QTLs) and candidate genes for seed shape and 100-seed weight in soybean [Glycine max (L.) Merr.]. Front. Plant Sci. 13, 1074245. doi: 10.3389/fpls.2022.1074245
Kumar, R., Saini, M., Taku, M., Debbarma, P., Mahto, R. K., Ramlal, A., et al. (2023). Identification of quantitative trait loci (QTLs) and candidate genes for seed shape and 100-seed weight in soybean [Glycine max (L.) Merr.]. Front. Plant Sci. 13. doi: 10.3389/fpls.2022.1074245
Kumawat, G., Xu, D. (2021). A major and stable quantitative trait locus qss2 for seed size and shape traits in a soybean RIL population. Front. Genet. 12, 646102. doi: 10.3389/fgene.2021.646102
Le, B. H., Wagmaister, J. A., Kawashima, T., Bui, A. Q., Harada, J. J., Goldberg, R. B. (2007). Using genomics to study legume seed development. Plant Physiol. 144, 562–574. doi: 10.1104/pp.107.100362
Li, Q., Fang, C., Duan, Z., Liu, Y., Qin, H., Zhang, J., et al. (2016). Functional conservation and divergence of GmCHLI genes in polyploid soybean. Plant J. 88, 584–596. doi: 10.1111/tpj.13282
Li, M.-W., Jiang, B., Han, T., Zhang, G., Lam, H.-M. (2022c). “Chapter One - Genomic research on soybean and its impact on molecular breeding,” in Adv. Bot. Res (Academic Press), 1–42.
Li, N., Li, Y. (2016). Signaling pathways of seed size control in plants. Curr. Opin. Plant Biol. 33, 23–32. doi: 10.1016/j.pbi.2016.05.008
Li, W., Liu, J., Zhang, H., Liu, Z., Wang, Y., Xing, L., et al. (2022d). Plant pan-genomics: recent advances, new challenges, and roads ahead. J. Genet. Genom. 49, 833–846. doi: 10.1016/j.jgg.2022.06.004
Li, Q. F., Lu, J., Yu, J. W., Zhang, C. Q., He, J. X., Liu, Q. Q. (2018). The brassinosteroid-regulated transcription factors BZR1/BES1 function as a coordinator in multisignal-regulated plant growth. Biochim. Biophys. Acta Gene. Regul. Mech. 1861, 561–571. doi: 10.1016/j.bbagrm.2018.04.003
Li, J., Nie, X., Tan, J. L. H., Berger, F. (2013a). Integration of epigenetic and genetic controls of seed size by cytokinin in Arabidopsis. Proc. Natl. Acad. Sci. 110, 15479–15484. doi: 10.1073/pnas.1305175110
Li, M.-W., Wang, Z., Jiang, B., Kaga, A., Wong, F.-L., Zhang, G., et al. (2020). Impacts of genomic research on soybean improvement in East Asia. Theor. Appl. Genet. 133, 1655–1678. doi: 10.1007/s00122-019-03462-6
Li, N., Xu, R., Li, Y. (2019b). Molecular networks of seed size control in plants. Ann. Rev. Plant Biol. 70, 435–463. doi: 10.1146/annurev-arplant-050718-095851
Li, J., Zhang, Y., Ma, R., Huang, W., Hou, J., Fang, C., et al. (2022a). Identification of ST1 reveals a selection involving hitchhiking of seed morphology and oil content during soybean domestication. Plant Biotechnol. J. 20 (6), 1110–1121. doi: 10.1111/pbi.13791
Li, J., Zhang, Y., Ma, R., Huang, W., Hou, J., Fang, C., et al. (2022b). Identification of ST1 reveals a selection involving hitchhiking of seed morphology and oil content during soybean domestication. Plant Biotechnol. J. 20, 1110–1121. doi: 10.1111/pbi.13791
Li, Y., Zhang, J., Zhang, J., Hao, L., Hua, J., Duan, L., et al. (2013b). Expression of an Arabidopsis molybdenum cofactor sulphurase gene in soybean enhances drought tolerance and increases yield under field conditions. Plant Biotechnol. J. 11, 747–758. doi: 10.1111/pbi.12066
Li, X., Zhang, X., Zhu, L., Bu, Y., Wang, X., Zhang, X., et al. (2019c). Genome-wide association study of four yield-related traits at the R6 stage in soybean. BMC Genet. 20, 39. doi: 10.1186/s12863-019-0737-9
Li, J., Zhao, J., Li, Y., Gao, Y., Hua, S., Nadeem, M., et al. (2019a). Identification of a novel seed size associated locus SW9-1 in soybean. Crop J. 7, 548–559. doi: 10.1016/j.cj.2018.12.010
Li, W., Zheng, D.-H., Van, K.-J., Lee, S.-H. (2008). QTL mapping for major agronomic traits across two years in soybean (Glycine max L. Merr.). J. Crop Sci. Biotechnol. 11, 171–176.
Li, X., Zhou, Y., Bu, Y., Wang, X., Zhang, Y., Guo, N., et al. (2021). Genome-wide association analysis for yield-related traits at the R6 stage in a Chinese soybean mini core collection. Gene. Genom. 43, 897–912. doi: 10.1007/s13258-021-01109-9
Lin, F., Li, X., Jia, N., Feng, F., Huang, H., Huang, J., et al. (2023). The impact of Russia-Ukraine conflict on global food security. Glob. Food Secur. 36, 100661. doi: 10.1016/j.gfs.2022.100661
Liu, J., Chen, J., Zheng, X., Wu, F., Lin, Q., Heng, Y., et al. (2017a). GW5 acts in the brassinosteroid signalling pathway to regulate grain width and weight in rice. Nat. Plants. 3, 17043. doi: 10.1038/nplants.2017.43
Liu, Y., Du, H., Li, P., Shen, Y., Peng, H., Liu, S., et al. (2020e). Pan-genome of wild and cultivated soybeans. Cell. 182, 162–176.e113. doi: 10.1016/j.cell.2020.05.023
Liu, S., Fan, L., Liu, Z., Yang, X., Zhang, Z., Duan, Z., et al. (2020c). A pd1-ps-P1 feedback loop controls pubescence density in soybean. Mol. Plant 13, 1768–1783. doi: 10.1016/j.molp.2020.10.004
Liu, N., Li, M., Hu, X., Ma, Q., Mu, Y., Tan, Z., et al. (2017b). Construction of high-density genetic map and QTL mapping of yield-related and two quality traits in soybean RILs population by RAD-sequencing. BMC Genom. 18, 466. doi: 10.1186/s12864-017-3854-8
Liu, Y.-L., Li, Y.-H., Reif, J. C., Mette, M. F., Liu, Z.-X., Liu, B., et al. (2013). Identification of quantitative trait loci underlying plant height and seed weight in soybean. Plant Genom. 6, 841–856. doi: 10.3835/plantgenome2013.03.0006
Liu, M., Li, D., Wang, Z., Meng, F., Li, Y., Wu, X., et al. (2012). Transgenic expression of ThIPK2 gene in soybean improves stress tolerance, oleic acid content and seed size. Plant Cell Tissue Organ Cult. 111, 277–289. doi: 10.1007/s11240-012-0192-z
Liu, J.-Y., Li, P., Zhang, Y.-W., Zuo, J.-F., Li, G., Han, X., et al. (2020a). Three-dimensional genetic networks among seed oil-related traits, metabolites and genes reveal the genetic foundations of oil synthesis in soybean. Plant J. 103, 1103–1124. doi: 10.1111/tpj.14788
Liu, J., Lin, Y., Chen, J., Yan, Q., Xue, C., Wu, R., et al. (2022). Genome-wide association studies provide genetic insights into natural variation of seed-size-related traits in mungbean. Front. Plant Sci. 13, 997988. doi: 10.3389/fpls.2022.997988
Liu, L., Tong, H., Xiao, Y., Che, R., Xu, F., Hu, B., et al. (2015). Activation of Big Grain1 significantly improves grain size by regulating auxin transport in rice. Proc. Natl. Acad. Sci. U S A. 112, 11102–11107. doi: 10.1073/pnas.1512748112
Liu, D., Yan, Y., Fujita, Y., Xu, D. (2018). Identification and validation of QTLs for 100-seed weight using chromosome segment substitution lines in soybean. Breed. Sci. 68, 442–448. doi: 10.1270/jsbbs.17127
Liu, S., Zhang, M., Feng, F., Tian, Z. (2020d). Toward a “Green revolution” for soybean. Mol. Plant 13, 688–697. doi: 10.1016/j.molp.2020.03.002
Liu, J.-Y., Zhang, Y.-W., Han, X., Zuo, J.-F., Zhang, Z., Shang, H., et al. (2020b). An evolutionary population structure model reveals pleiotropic effects of GmPDAT for traits related to seed size and oil content in soybean. J. Exp. Bot. 71, 6988–7002. doi: 10.1093/jxb/eraa426
Lu, S., Dong, L., Fang, C., Liu, S., Kong, L., Cheng, Q., et al. (2020). Stepwise selection on homeologous PRR genes controlling flowering and maturity during soybean domestication. Nat. Genet. 52, 428–436. doi: 10.1038/s41588-020-0604-7
Lu, X., Li, Q. T., Xiong, Q., Li, W., Bi, Y. D., Lai, Y. C., et al. (2016). The transcriptomic signature of developing soybean seeds reveals the genetic basis of seed trait adaptation during domestication. Plant J. 86, 530–544. doi: 10.1111/tpj.13181
Lu, X., Xiong, Q., Cheng, T., Li, Q. T., Liu, X. L., Bi, Y. D., et al. (2017). A PP2C-1 allele underlying a quantitative trait locus enhances soybean 100-seed weight. Mol. Plant 10, 670–684. doi: 10.1016/j.molp.2017.03.006
Luo, S., Jia, J., Liu, R., Wei, R., Guo, Z., Cai, Z., et al. (2022). Identification of major QTLs for soybean seed size and seed weight traits using a RIL population in different environments. Front. Plant Sci. 13, 1094112. doi: 10.3389/fpls.2022.1094112
Luo, S., Jia, J., Liu, R., Wei, R., Guo, Z., Cai, Z., et al. (2023). Identification of major QTLs for soybean seed size and seed weight traits using a RIL population in different environments. Front. Plant Sci. 13. doi: 10.3389/fpls.2022.1094112
Lyu, X., Cheng, Q., Qin, C., Li, Y., Xu, X., Ji, R., et al. (2021). GmCRY1s modulate gibberellin metabolism to regulate soybean shade avoidance in response to reduced blue light. Mol. Plant 14, 298–314. doi: 10.1016/j.molp.2020.11.016
Manan, S., Ahmad, M. Z., Zhang, G., Chen, B., Haq, B. U., Yang, J., et al. (2017). Soybean LEC2 regulates subsets of genes involved in controlling the biosynthesis and catabolism of seed storage substances and seed development. Front. Plant Sci. 8. doi: 10.3389/fpls.2017.01604
Manan, S., Zhao, J. (2020). Role of Glycine max ABSCISIC ACID INSENSITIVE 3 (GmABI3) in lipid biosynthesis and stress tolerance in soybean. Funct. Plant Biol. 48 (2), 171–179. doi: 10.1071/FP19260
Meng, Y., Li, H., Wang, Q., Liu, B., Lin, C. (2013). Blue light-dependent interaction between cryptochrome2 and CIB1 regulates transcription and leaf senescence in soybean. Plant Cell. 25, 4405–4420. doi: 10.1105/tpc.113.116590
Mian, M. A., Bailey, M. A., Tamulonis, J. P., Shipe, E. R., Carter, T. E., Jr, Parrott, W. A., et al. (1996). Molecular markers associated with seed weight in two soybean populations. Theor. Appl. Genet. 93, 1011–1016. doi: 10.1007/BF00230118.
Miao, L., Yang, S., Zhang, K., He, J., Wu, C., Ren, Y., et al. (2020). Natural variation and selection in GmSWEET39 affect soybean seed oil content. New Phytol. 225, 1651–1666. doi: 10.1111/nph.16250
Moongkanna, J., Nakasathien, S., Novitzky, W., Kwanyuen, P., Sinchaisri, P., Srinives, P. (2011). SSR markers linking to seed traits and total oil content in soybean. Thai J. Agric. Sci. 44, 233–241.
Nguyen, C. X., Paddock, K. J., Zhang, Z., Stacey, M. G. (2021). GmKIX8-1 regulates organ size in soybean and is the causative gene for the major seed weight QTL qSw17-1. New Phytol. 229, 920–934. doi: 10.1111/nph.16928
Pandey, S. (2019). Heterotrimeric G-Protein signaling in plants: conserved and novel mechanisms. Annu. Rev. Plant Biol. 70, 213–238. doi: 10.1146/annurev-arplant-050718-100231
Panthee, D. R., Pantalone, V. R., West, D. R., Saxton, A. M., Sams, C. E. (2005). Quantitative trait loci for seed protein and oil concentration, and seed size in soybean. Crop Sci. 45, 2015–2022. doi: 10.2135/cropsci2004.0720
Qi, Z., Hou, M., Han, X., Liu, C., Jiang, H., Xin, D., et al. (2014). Identification of quantitative trait loci (QTLs) for seed protein concentration in soybean and analysis for additive effects and epistatic effects of QTLs under multiple environments. Plant Breed. 133, 499–507. doi: 10.1111/pbr.12179
Qi, Z., Song, J., Zhang, K., Liu, S., Tian, X., Wang, Y., et al. (2020). Identification of QTNs controlling 100-seed weight in soybean using multilocus genome-wide association studies. Front. Genet. 11. doi: 10.3389/fgene.2020.00689
Ran, F. A., Hsu, P. D., Wright, J., Agarwala, V., Scott, D. A., Zhang, F. (2013). Genome engineering using the CRISPR-Cas9 system. Nat. Protoc. 8, 2281–2308. doi: 10.1038/nprot.2013.143
Ravelombola, W., Qin, J., Shi, A., Song, Q., Yuan, J., Wang, F., et al. (2021). Genome-wide association study and genomic selection for yield and related traits in soybean. PloS One 16, e0255761. doi: 10.1371/journal.pone.0255761
Rodrigues, J. I. D. S., Miranda, F. D. D., Piovesan, N. D., Ferreira, A., Ferreira, M. F. D. S., Cruz, C. D., et al. (2016). QTL mapping for yield components and agronomic traits in a Brazilian soybean population. Crop Breed. Appl. Biotechnol. 16, 265–273. doi: 10.1590/1984-70332016v16n4a41
Ruan, Y.-L., Patrick, J. W., Bouzayen, M., Osorio, S., Fernie, A. R. (2012). Molecular regulation of seed and fruit set. Trends Plant Sci. 17, 656–665. doi: 10.1016/j.tplants.2012.06.005
Shi, C. L., Dong, N. Q., Guo, T., Ye, W. W., Shan, J. X., Lin, H. X. (2020). A quantitative trait locus GW6 controls rice grain size and yield through the gibberellin pathway. Plant J. 103, 1174–1188. doi: 10.1111/tpj.14793
Shi, X., Tian, Q., Deng, P., Zhang, W., Jing, W. (2021). The rice aldehyde oxidase OsAO3 gene regulates plant growth, grain yield, and drought tolerance by participating in ABA biosynthesis. Biochem. Biophys. Res. Commun. 548, 189–195. doi: 10.1016/j.bbrc.2021.02.047
Singh, A. K., Fu, D.-Q., El-Habbak, M., Navarre, D., Ghabrial, S., Kachroo, A. (2010). Silencing Genes Encoding Omega-3 Fatty Acid Desaturase Alters Seed Size and Accumulation of Bean pod mottle virus in Soybean. Mol. Plant Microbe Interact. 24, 506–515. doi: 10.1094/MPMI-09-10-0201
Singh, V. K., Singh, A. K., Singh, S., Singh, B. D. (2015). “Next-generation sequencing (NGS) tools and impact in plant breeding,” in Advances in plant breeding strategies: breeding, biotechnology and molecular tools. (Berlin/Heidelberg, Germany: Springer). 563–612.
Slattery, R. A., Grennan, A. K., Sivaguru, M., Sozzani, R., Ort, D. R. (2016). Light sheet microscopy reveals more gradual light attenuation in light-green versus dark-green soybean leaves. J. Exp. Bot. 67, 4697–4709. doi: 10.1093/jxb/erw246
Smith, T. J., Camper, J. H. M. (1975). Effects of seed size on soybean performance. Agron. J. 67, 681–684. doi: 10.2134/agronj1975.00021962006700050025x
Song, Q., Jenkins, J., Jia, G., Hyten, D. L., Pantalone, V., Jackson, S. A., et al. (2016). Construction of high resolution genetic linkage maps to improve the soybean genome sequence assembly Glyma1.01. BMC Genom. 17, 33. doi: 10.1186/s12864-015-2344-0
SoyBase (2023). Available at: https://www.soybase.org/ (Accessed February 2023).
Sreenivasulu, N., Wobus, U. (2013). Seed-Development Programs: A Systems biology–based comparison between dicots and monocots. Annu. Rev. Plant Biol. 64, 189–217. doi: 10.1146/annurev-arplant-050312-120215
Sun, X., Sun, X., Pan, X., Zhang, H., Wang, Y., Ren, H., et al. (2022). Identification and fine mapping of a quantitative trait locus controlling the total flower and pod numbers in soybean. Agronomy 12, 790. doi: 10.3390/agronomy12040790
Tang, X., Su, T., Han, M., Wei, L., Wang, W., Yu, Z., et al. (2017). Suppression of extracellular invertase inhibitor gene expression improves seed weight in soybean (Glycine max). J. Exp. Bot. 68, 469–482. doi: 10.1093/jxb/erw425
Tang, K., Yang, S., Feng, X., Wu, T., Leng, J., Zhou, H., et al. (2020). GmNAP1 is essential for trichome and leaf epidermal cell development in soybean. Plant Mol. Biol. 103, 609–621. doi: 10.1007/s11103-020-01013-y
Tayade, R., Kulkarni, K. P., Jo, H., Song, J. T., Lee, J.-D. (2019). Insight into the prospects for the improvement of seed starch in legume—A Review. Front. Plant Sci. 10. doi: 10.3389/fpls.2019.01213
Tian, Z., Wang, J.-W., Li, J., Han, B. (2021). Designing future crops: challenges and strategies for sustainable agriculture. Plant J. 105, 1165–1178. doi: 10.1111/tpj.15107
Toleikiene, M., Slepetys, J., Sarunaite, L., Lazauskas, S., Deveikyte, I., Kadziuliene, Z. (2021). Soybean development and productivity in response to organic management above the northern boundary of soybean distribution in europe. Agronomy 11, 214. doi: 10.3390/agronomy11020214
Tong, H., Liu, L., Jin, Y., Du, L., Yin, Y., Qian, Q., et al. (2012). DWARF AND LOW-TILLERING Acts as a direct downstream target of a GSK3/SHAGGY-like kinase to mediate brassinosteroid responses in rice. Plant Cell 24, 2562–2577. doi: 10.1105/tpc.112.097394
Visscher, P. M., Wray, N. R., Zhang, Q., Sklar, P., Mccarthy, M. I., Brown, M. A., et al. (2017). 10 years of GWAS discovery: biology, function, and translation. Am. J. Hum. Genet. 101, 5–22. doi: 10.1016/j.ajhg.2017.06.005
Vu, H., Le, C., Vu Dinh, H., Tun, N., Ngoc, T. (2019). Correlations and path coefficients for yield related traits in soybean progenies. Asian J. Crop Sci. 11, 32–39. doi: 10.3923/ajcs.2019.32.39
Wang, X., Li, Y., Zhang, H., Sun, G., Zhang, W., Qiu, L. (2015a). Evolution and association analysis of GmCYP78A10 gene with seed size/weight and pod number in soybean. Mol. Biol. Rep. 42, 489–496. doi: 10.1007/s11033-014-3792-3
Wang, X., Li, Y., Zhang, H., Sun, G., Zhang, W., Qiu, L. (2015b). Evolution and association analysis of GmCYP78A10 gene with seed size/weight and pod number in soybean. Mol. Biol. Rep. 42, 489–496. doi: 10.1007/s11033-014-3792-3
Wang, S., Liu, S., Wang, J., Yokosho, K., Zhou, B., Yu, Y. C., et al. (2020). Simultaneous changes in seed size, oil content and protein content driven by selection of SWEET homologues during soybean domestication. Natl. Sci. Rev. 7, 1776–1786. doi: 10.1093/nsr/nwaa110
Wang, H.-W., Zhang, B., Hao, Y.-J., Huang, J., Tian, A.-G., Liao, Y., et al. (2007). The soybean Dof-type transcription factor genes, GmDof4 and GmDof11, enhance lipid content in the seeds of transgenic Arabidopsis plants. Plant J. 52, 716–729. doi: 10.1111/j.1365-313X.2007.03268.x
Warrington, C. V., Abdel-Haleem, H., Hyten, D. L., Cregan, P. B., Orf, J. H., Killam, A. S., et al. (2015). QTL for seed protein and amino acids in the Benning × Danbaekkong soybean population. Theor. Appl. Genet. 128, 839–850. doi: 10.1007/s00122-015-2474-4
Wu, D., Zhan, Y., Sun, Q., Xu, L., Lian, M., Zhao, X., et al. (2018). Identification of quantitative trait loci underlying soybean (Glycine max [L.] Merr.) seed weight including main, epistatic and QTL × environment effects in different regions of Northeast China. Plant Breed. 137, 194–202. doi: 10.1111/pbr.12574
Xavier, A., Rainey, K. M. (2020). Quantitative genomic dissection of soybean yield components. G3-Genes Genom. Genet. 10, 665–675. doi: 10.1534/g3.119.400896
Xing, Y., Zhang, Q. (2010). Genetic and molecular bases of rice yield. Annu. Rev. Plant Biol. 61, 421–442. doi: 10.1146/annurev-arplant-042809-112209
Xu, M., Kong, K., Miao, L., He, J., Liu, T., Zhang, K., et al. (2023). Identification of major quantitative trait loci and candidate genes for seed weight in soybean. Theor. Appl. Genet. 136, 22. doi: 10.1007/s00122-023-04299-w
Xu, C., Wu, T., Yuan, S., Sun, S., Han, T., Song, W., et al. (2022). Can soybean cultivars with larger seed size produce more protein, lipids, and seed yield? A Meta-Analysis. Foods 11, 4059. doi: 10.3390/foods11244059
Yan, L., Hofmann, N., Li, S., Ferreira, M. E., Song, B., Jiang, G., et al. (2017). Identification of QTL with large effect on seed weight in a selective population of soybean with genome-wide association and fixation index analyses. BMC Genom. 18, 529. doi: 10.1186/s12864-017-3922-0
Yan, L., Li, Y. H., Yang, C. Y., Ren, S. X., Chang, R. Z., Zhang, M. C., et al. (2014). Identification and validation of an over-dominant QTL controlling soybean seed weight using populations derived from Glycine max × Glycine soja. Plant Breed. 133, 632–637. doi: 10.1111/pbr.12197
Yang, Y., La, T. C., Gillman, J. D., Lyu, Z., Joshi, T., Usovsky, M., et al. (2022). Linkage analysis and residual heterozygotes derived near isogenic lines reveals a novel protein quantitative trait loci from a Glycine soja accession. Front. Plant Sci. 13, 938100. doi: 10.3389/fpls.2022.938100
Yang, H., Wang, W., He, Q., Xiang, S., Tian, D., Zhao, T., et al. (2019). Identifying a wild allele conferring small seed size, high protein content and low oil content using chromosome segment substitution lines in soybean. Theor. Appl. Genet. 132, 2793–2807. doi: 10.1007/s00122-019-03388-z
Yu, L., Liu, Y., Zeng, S., Yan, J., Wang, E., Luo, L. (2019). Expression of a novel PSK-encoding gene from soybean improves seed growth and yield in transgenic plants. Planta. 249, 1239–1250. doi: 10.1007/s00425-019-03101-w
Zeng, A., Chen, P., Korth, K., Hancock, F., Pereira, A., Brye, K., et al. (2017). Genome-wide association study (GWAS) of salt tolerance in worldwide soybean germplasm lines. Mol. Breed. 37, 1–14. doi: 10.1007/s11032-017-0634-8
Zhang, D., Cheng, H., Wang, H., Zhang, H., Liu, C., Yu, D. (2010). Identification of genomic regions determining flower and pod numbers development in soybean (Glycine max L.). J. Genet. Genom. 37, 545–556. doi: 10.1016/S1673-8527(09)60074-6
Zhang, M., Dong, R., Huang, P., Lu, M., Feng, X., Fu, Y., et al. (2023). Novel seed size: A novel seed-developing gene in glycine max. Int. J. Mol. Sci. 24, 4189. doi: 10.3390/ijms24044189
Zhang, H., Hao, D., Sitoe, H. M., Yin, Z., Hu, Z., Zhang, G., et al. (2015). Genetic dissection of the relationship between plant architecture and yield component traits in soybean (Glycine max) by association analysis across multiple environments. Plant Breed. 134, 564–572. doi: 10.1111/pbr.12305
Zhang, Q., Li, H., Li, R., Hu, R., Fan, C., Chen, F., et al. (2008). Association of the circadian rhythmic expression of GmCRY1a with a latitudinal cline in photoperiodic flowering of soybean. Proc. Natl. Acad. Sci. U.S.A. 105, 21028–21033. doi: 10.1073/pnas.0810585105
Zhang, Y., Li, W., Lin, Y., Zhang, L., Wang, C., Xu, R. (2018). Construction of a high-density genetic map and mapping of QTLs for soybean (Glycine max) agronomic and seed quality traits by specific length amplified fragment sequencing. BMC Genom. 19, 641. doi: 10.1186/s12864-018-5035-9
Zhang, M., Liu, S., Wang, Z., Yuan, Y., Zhang, Z., Liang, Q., et al. (2022). Progress in soybean functional genomics over the past decade. Plant Biotechnol. J. 20, 256–282. doi: 10.1111/pbi.13682
Zhang, M., Liu, Y.-H., Xu, W., Smith, C. W., Murray, S. C., Zhang, H.-B. (2020). Analysis of the genes controlling three quantitative traits in three diverse plant species reveals the molecular basis of quantitative traits. Sci. Rep. 10, 1–14. doi: 10.1038/s41598-020-66271-8
Zhang, Y.-Q., Lu, X., Zhao, F.-Y., Li, Q.-T., Niu, S.-L., Wei, W., et al. (2016b). Soybean GmDREBL increases lipid content in seeds of transgenic Arabidopsis. Sci. Rep. 6, 34307. doi: 10.1038/srep34307
Zhang, J., Song, Q., Cregan, P. B., Jiang, G.-L. (2016a). Genome-wide association study, genomic prediction and marker-assisted selection for seed weight in soybean (Glycine max). Theor. Appl. Genet. 129, 117–130. doi: 10.1007/s00122-015-2614-x
Zhang, D., Zhang, H., Hu, Z., Chu, S., Yu, K., Lv, L., et al. (2019). Artificial selection on GmOLEO1 contributes to the increase in seed oil during soybean domestication. PloS Genet. 15, e1008267. doi: 10.1371/journal.pgen.1008267
Zhao, J., Chen, L., Zhao, T., Gai, J. (2017). Chicken Toes-Like Leaf and Petalody Flower (CTP) is a novel regulator that controls leaf and flower development in soybean. J. Exp. Bot. 68, 5565–5581. doi: 10.1093/jxb/erx358
Zhao, B., Dai, A., Wei, H., Yang, S., Wang, B., Jiang, N., et al. (2016). Arabidopsis KLU homologue GmCYP78A72 regulates seed size in soybean. Plant Mol. Biol. 90, 33–47. doi: 10.1007/s11103-015-0392-0
Zhao, X., Dong, H., Chang, H., Zhao, J., Teng, W., Qiu, L., et al. (2019). Genome wide association mapping and candidate gene analysis for hundred seed weight in soybean [Glycine max (L.) Merrill]. BMC Genom. 20, 648. doi: 10.1186/s12864-019-6009-2
Zhao, Q., Shi, X., Yan, L., Yang, C., Liu, C., Feng, Y., et al. (2021). Characterization of the common genetic basis underlying seed hilum size, yield, and quality traits in soybean. Front. Plant Sci. 12, 610214. doi: 10.3389/fpls.2021.610214
Zhou, B., Lin, J., Peng, W., Peng, D., Zhuo, Y., Zhu, D., et al. (2012). Dwarfism in Brassica napus L. induced by the over-expression of a gibberellin 2-oxidase gene from Arabidopsis thaliana. Mol. Breed. 29, 1–17. doi: 10.1007/s11032-010-9530-1
Zhou, L., Luo, L., Zuo, J.-F., Yang, L., Zhang, L., Guang, X., et al. (2016). Identification and validation of candidate genes associated with domesticated and improved traits in soybean. Plant Genome. 9, plantgenome2015.2009.0090. doi: 10.3835/plantgenome2015.09.0090
Keywords: seed size, seed weight, yield, quantitative trait, soybean
Citation: Tayade R, Imran M, Ghimire A, Khan W, Nabi RBS and Kim Y (2023) Molecular, genetic, and genomic basis of seed size and yield characteristics in soybean. Front. Plant Sci. 14:1195210. doi: 10.3389/fpls.2023.1195210
Received: 28 March 2023; Accepted: 30 October 2023;
Published: 15 November 2023.
Edited by:
Sehrish Manan, Jiangsu University, ChinaReviewed by:
Zhi Gang Meng, Biotechnology Research institute of CAAS, ChinaCopyright © 2023 Tayade, Imran, Ghimire, Khan, Nabi and Kim. This is an open-access article distributed under the terms of the Creative Commons Attribution License (CC BY). The use, distribution or reproduction in other forums is permitted, provided the original author(s) and the copyright owner(s) are credited and that the original publication in this journal is cited, in accordance with accepted academic practice. No use, distribution or reproduction is permitted which does not comply with these terms.
*Correspondence: Yoonha Kim, a3loMTIyOUBrbnUuYWMua3I=
†These authors have contributed equally to this work
Disclaimer: All claims expressed in this article are solely those of the authors and do not necessarily represent those of their affiliated organizations, or those of the publisher, the editors and the reviewers. Any product that may be evaluated in this article or claim that may be made by its manufacturer is not guaranteed or endorsed by the publisher.
Research integrity at Frontiers
Learn more about the work of our research integrity team to safeguard the quality of each article we publish.