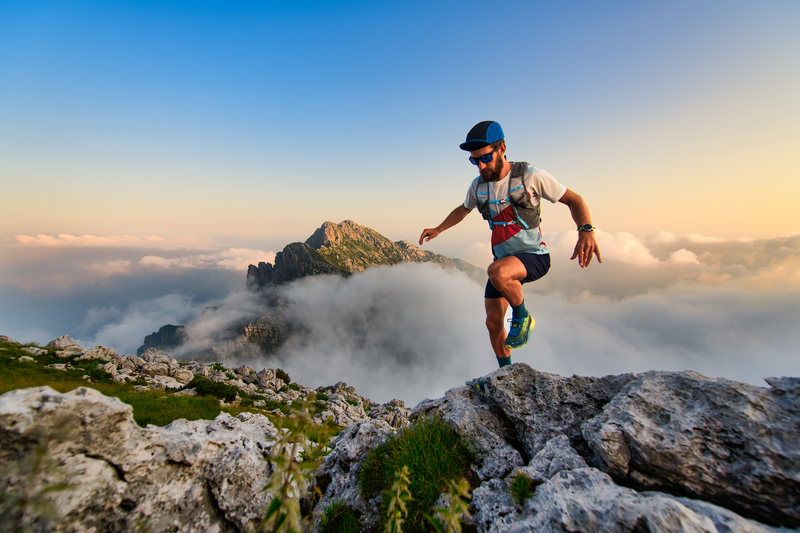
95% of researchers rate our articles as excellent or good
Learn more about the work of our research integrity team to safeguard the quality of each article we publish.
Find out more
ORIGINAL RESEARCH article
Front. Plant Sci. , 06 June 2023
Sec. Functional and Applied Plant Genomics
Volume 14 - 2023 | https://doi.org/10.3389/fpls.2023.1182771
Soybean seed protein content (PC) and oil content (OC) have important economic value. Detecting the loci/gene related to PC and OC is important for the marker-assisted selection (MAS) breeding of soybean. To detect the stable and new loci for PC and OC, a total of 320 soybean accessions collected from the major soybean-growing countries were used to conduct a genome-wide association study (GWAS) by resequencing. The PC ranged from 37.8% to 46.5% with an average of 41.1% and the OC ranged from 16.7% to 22.6% with an average of 21.0%. In total, 23 and 29 loci were identified, explaining 3.4%–15.4% and 5.1%–16.3% of the phenotypic variations for PC and OC, respectively. Of these, eight and five loci for PC and OC, respectively, overlapped previously reported loci and the other 15 and 24 loci were newly identified. In addition, nine candidate genes were identified, which are known to be involved in protein and oil biosynthesis/metabolism, including lipid transport and metabolism, signal transduction, and plant development pathway. These results uncover the genetic basis of soybean protein and oil biosynthesis and could be used to accelerate the progress in enhancing soybean PC and OC.
Soybean (Glycine max L.) is an important economic crop in the world and is a major source of vegetable oil and feed protein (Liu et al., 2008). Dry soybean seeds are composed of approximately 40% protein content (PC) and 20% oil content (OC). Increasing seeds’ PC (Li et al., 2021) and OC (Zhang et al., 2019) is an important breeding objective for soybean. The improvement of PC and OC is challenging due to their polygenic inheritance (Li et al., 2020). China, Russia, the United States, and Canada are the major soybean-producing countries (Xue et al., 2022). Although soybean production has been improved largely by traditional breeding, it is still not enough to meet the demand (Li et al., 2019; Li et al., 2020).
PC and OC are typical quantitative traits and are controlled by two major loci on chromosomes 15 and 20 and other genes with minor effects (Wang et al., 2020), and they are influenced by both environmental and genetic factors (Clemente and Cahoon, 2009; Pathan et al., 2013). Marker-assisted selection (MAS) is an effective method for the genetic improvement of PC and OC (Jiang et al., 2018; Karikari et al., 2019). The reliability and efficacy of MAS depend on the number and phenotypic effects of the genes/quantitative trait loci (QTL) on the corresponding trait (Zhang et al., 2018; Karikari et al., 2019; Wang et al., 2020). Thus, QTL mapping for soybean seed PC and OC plays a vital role in soybean MAS breeding (Zafar et al., 2019; Wang et al., 2020). Over 200 and 300 QTLs for PC and OC have been deposited in SoyBase (http://www.soybase.org) (Brown et al., 2021), and over 30 genes and functional markers for PC and OC have been cloned and developed, such as GmbZIP123, GmMYB73, GmDOF4, GmDOF11, and GmNFYA (Li et al., 2019; Wang et al., 2020). Overexpression of GmSDP1-4 and GmPDAT increased seed oil (Liu et al., 2020; Liu et al., 2020b). GA20 oxidase (GA20OX) and nuclear transcription factor Y subunit alpha (NFYA) are two key drivers of seed traits and enhanced seed size and weight and OC, respectively. GmOLEO1 and GmWRI1a also significantly increased total OC and fatty acid content (Zhang et al., 2019; Zhang et al., 2022). Furthermore, several transcription factors were also found to be associated with soybean seed oil. Overexpression of GmbZIP123, GmMYB73, GmZF351, GmZF392, and GmWRI1b enhanced lipid content (Guo et al., 2020). Compared with genes associated with OC, fewer genes controlling PC or amino acids have been identified. These include MGL (a putative methionine γ-lyase), OASS (the cytosolic isoform of O-acetylserine sulfhydrylase), Rab5a (a small GTPase-encoding gene), and SWEETs (sugar will eventually be exported transporters) (GmSWEET15, GmSWEET10a, and GmSWEET10b), which played important roles in soybean seed quality through their effects on both OC and PC (Wang and Tian, 2015; Zhang et al., 2022).
Most of these QTLs were mapped by linkage mapping based on bi-parental populations, including the recombinant inbred line (RIL), F2, and backcross populations. However, linkage mapping was limited by the small phenotypic variation of bi-parental populations. Furthermore, traditional molecular markers used in these studies reduced the accuracy of QTL mapping due to their low densities (Rasheed et al., 2016; Liu et al., 2017; Klepadlo et al., 2019; Samanfar et al., 2019; Wang et al., 2020; He et al., 2021; Ullah et al., 2021).
Single nucleotide polymorphisms (SNPs) are more abundant with higher coverage and are markers of choice for gene discovery and MAS (Yuan et al., 2014; Rasheed et al., 2016). The development of SNP chips and next-generation sequencing (NGS) made the use of SNP quite affordable and feasible in molecular breeding. Linkage mapping and association analysis identifying genes/QTLs for complex traits are most widely used for gene discovery (Kim et al., 2010; Chan et al., 2012; Liu et al., 2016; Liu et al., 2017; Song et al., 2017; Wang et al., 2020). Currently, soybean SNP arrays are widely used in genetic analysis for yield, processing quality, and disease resistance-related traits (Akond et al., 2014; Chaudhary et al., 2015; Zhang et al., 2018; Beyer et al., 2019; Li et al., 2019; Zhang et al., 2019; Tian et al., 2020; Wang et al., 2020). In contrast to traditional bi-parental mapping, genome-wide association studies (GWASs) use natural diversity (such as wild types, landraces, and cultivars) and offer an effective and reliable way to uncover the genetic basis of complex traits (Zhu et al., 2008; Sela et al., 2014; Liu et al., 2017; Schläppi et al., 2017; Shi et al., 2017; Wang et al., 2019; Alqudah et al., 2020). GWAS has been widely used in the genetic analysis of yield, disease resistance, and quality-related traits in soybean (Vuong et al., 2015; Li et al., 2019; Wang et al., 2020; Zahid et al., 2022).
In this study, 320 soybean accessions collected mainly from the major soybean-growing countries were used to (1) identify loci underpinning PC and OC in soybean and (2) identify candidate genes for further study.
A total of 320 soybean accessions from the main soybean-growing countries (including the United States, Algeria, Canada, China, Czechia, France, Germany, Hungary, Japan, Moldova, Romania, Russia, Serbia, Sweden, and Ukraine) were collected and used for the evaluation of PC and OC (Table S1). All 320 soybean accessions were planted at the Heilongjiang Academy of Agricultural Sciences experimental station in Harbin, China, with three replications in 2017, 2018, 2019, and 2021; Mudanjiang in 2018 and Qingan in 2018. A completely randomized block design with three replicates was used, with each line 3.0 m in length and 0.65 m apart, and with 6 cm spacing between two plants. Field management was in accordance with local field cultivation conditions.
Soybean seeds were harvested from 10 plants from each genotype and subsequently used for the PC and OC determination. The Infratec 1241 NIR Grain Analyzer (FOSS, Sweden) was used to analyze three seed samples from each genotype in each replicate (20–25 g). The phenotypic values given for each accession used in this study were all the mean values of the three replicates. The best linear unbiased estimation (BLUE) for PC and OC among all environments was calculated using the R package “sommer”. The maximum, minimum, and standard deviation of the PC and OC were calculated. Analysis of variance (ANOVA) was used to compare the phenotypic values of PC and OC in each environment or jointly in multiple environments. The heritability was estimated using the entry-mean basis formula , where , , and is the genetic effect, environmental effect, and residual, respectively, and and is the number of environments and the number of replicates, respectively.
For QTL-seq, the genomic DNA from the seedling leaf was isolated. The DNA was genotyped by re-sequencing using the Illumina HiSeq 2500 platform (Illumina, Inc., San Diego, CA, United States) by Biomarker Biotechnology Co., Ltd. The paired-end read data (PE150) with a sequencing depth of approximately 10× of the soybean genome were generated. In total, 3814.10 giga base pairs (Gbp) of clean data, with a base call accuracy of about 93.10%, were obtained. The average comparison rate between the sample and reference genome was 98.47%, with an average coverage depth of 10× and genome coverage of 97.18%. The SNPs were filtered by minor allele frequency (MAF)< 0.05 and missing rate > 10%. Population structure was analyzed using Structure v2.3.4 (Pritchard et al., 2000). Principal component analysis (PCA) was performed and mega trees were created using Tassel v5.0 (Breseghello and Sorrells, 2006). Linkage disequilibrium (LD) decay was calculated using the full matrix and sliding window options in Tassel v5.0.
A mixed linear model (MLM, PCA (fixed-effect factor) + K (random-effect factor)) in Tassel v5.0 (Breseghello and Sorrells, 2006) was used to avoid spurious marker–trait associations (MTAs) as follows: y=μ+xβ+u+e (y: phenotype; µ: mean value; x: genotype; β: effect of the SNP; u: the random effects). In this study, the Bonferroni–Holm correction for multiple testing (alpha = 0.05) was too conservative, and no significant MTAs were detected. Thus, markers with the threshold for the significant associations is –log10 (p-value) ≥ 6.0. Manhattan and quantile–quantile (Q–Q) plots were drawn using CMplot. Candidate genes for PC and OC consistently identified in two or more populations were identified in this study. The following steps were conducted to identify the candidate genes. Firstly, all genes located in the LD block region around the peak SNP ( ± 480 kb based on previous LD decay analysis) of each important QTL were retrieved. Then, all available SNPs located inside these genes were searched against GenBank using the flanking sequences of the SNPs (including the LD decay interval) significantly associated with PC and OC. The genes (except the hypothetical protein, transposon protein, and retrotransposon protein) that were identified with MTAs of non-synonymous SNPs in the coding region that could further lead to sense mutations were considered. Thus, genes involved in protein and oil biosynthesis/metabolism were regarded as high-confidence candidate genes for PC and OC.
Quantitative real-time PCR (qRT-PCR) was performed to test the expression of selected candidate genes in the accessions with extreme PC and OC. All seeds were sampled for RNA extraction after maturity. cDNA was synthesized using the HiScript II 1st Strand cDNA synthesis kit and the primers were designed using Primer 5.0 software. PCR was conducted in a volume of 20 µL (2 µL cDNA, 10 µL ChamQ Universal SYBR qPCR Master Mix, and 0.4 µL of each primer (µM)) (Table S2). All assays were conducted in two independent experiments with three repetitions.
OC and PC showed continuous and significant variations in the 320 soybean accessions. The BLUE values of OC and PC were 21.0% (from 16.7% to 22.6%) and 41.1% (from 37.8% to 46.5%), respectively (Figure S1; Table S1). The standard deviation and coefficient of variation of OC and PC were 0.86% (0.041) and 1.38% (0.034) across all environments, respectively. The OC was negatively correlated with PC (–0.532, p<0.001). ANOVA indicated highly significant effects (p<0.01) of genotypes, environments, and genotype × environment interactions on PC and OC (Table 1). The SNP-based heritability for OC and PC was 0.77 and 0.78, respectively.
In total, 3,290,923 polymorphic SNPs after filtration (MAF< 0.05, missing rate > 0.1) were used for GWAS. Chromosome 18 had the highest number of SNPs (233,764), whereas chromosome 11 had the lowest number of SNPs (74,209). The average marker density was 304.0 marker/kb on the genome-wide scale. The population structure divided the 320 soybean accessions into three subgroups, namely subgroup I, II, and III. Of these, subgroup I consisted of 156 accessions from China, Russia, and Ukraine, subgroup II had 102 accessions from the United States and Canada, and subgroup III comprised 62 accessions from Germany, France, and Czechia (Figure 1). The neighbor-joining (NJ) tree results also suggested that the 320 soybean accessions could be divided into three subgroups. PCA analysis indicated that the top three PCAs explained 22.1%, 18.3%, and 12.5% of the total variances. In addition, PCA results indicated that all 320 soybean accessions could belong to the three subgroups. The NJ tree and PCA analysis validated the results of the population structure analysis. The average LD decay of the genome was about 480 kb according to the locally estimated scatterplot smoothing (LOESS) curve (Figure 1).
Figure 1 Population structure analysis for the 320 soybean accessions. (A) principal component analysis (PCA) plots; (B) population structure analysis from k=2 to 12; (C) neighbor-joining (NJ) tree; (D) LD decay.
In total, 29 loci for OC were identified on all chromosomes except for chromosome 9, and each explained 5.1%–16.3% of the total phenotypic variances. For OC, chromosome 20 contained three loci, and chromosomes 2, 6, 7, 11, 12, and 19 contained two loci, whereas chromosomes 3, 4, 5, 8, and 10 each had only one locus. For PC, chromosome 11 contained four loci, and chromosomes 2, 5, and 20 contained two loci, whereas chromosomes 1, 3, 4, 6, 7, 8, 9, 13, 14, 15, 16, and 18 each had only one locus. Twenty-three loci for PC were identified on all chromosomes except for chromosomes 12, 17, and 19, and explained 3.4%–15.4% of the phenotypic variances. Of these, six pleiotropic loci including qOC2.2 (44.7–49.2 Mb) and qPC2.2 (45.0–50.8 Mb); qOC4.1 (41.3–45.3 Mb) and qPC4.1 (44.6–5.0.7 Mb); qPC5.2 (44.1–48.7 Mb) and qOC5.1 (46.8–47.7 Mb); qOC13.1 (19.9–25.0 Mb) and qPC13.1 (24.3–28.6 Mb); qOC14.1 (43.5–49.1 Mb) and qPC14.1 (47.2–49.8 Mb); and qOC20.3 (43.9–49.0 Mb) and qPC20.2 (45.0–48.0 Mb) were significantly associated with both PC and OC.
For PC, qPC5.2, qPC9.1, qPC10.1, qPC11.1, qPC11.2, qPC11.3, qPC11.4, qPC14.1, and qPC16.1 were identified across all six environments, qPC1.1, qPC3.1, qPC4.1, qPC18.1, qPC20.1, and qPC20.2 were identified in five environments, and the other loci were identified in three or four environments. For OC, qOC1.2, qOC6.2, qOC11.1, qOC12.1, qOC13.1, qOC15.1, qOC16.1, and qOC20.1 were identified in five environments, whereas qOC1.1, qOC2.2, and qOC3.1 were identified in only one environment. The other loci were identified in two or three environments (Tables 2, 3, S2; Figures 2, S2).
Figure 2 The distribution of protein content and oil content across all environments. PC: protein content; OC: oil content; E1, E2, E3 E4, E5, and E6 indicate 2017 Harbin, 2018 Harbin, 2019 Harbin, 2021 Harbin, 2018 Mudanjiang, and 2018 Qingan, respectively.
In total, nine candidate genes for OC and PC were identified and showed significant differential expression between the accessions with contrasting phenotypes (Table S2). For Glyma.03G261000 and Glyma.07G137400, the gene expression was 1.3–5.9-fold higher in accessions with higher PC and OC than in accessions with lower PC and OC. In contrast, the gene expressions were 1.2- to 5.4-fold lower in accessions with higher PC and OC than in accessions with lower PC and OC for the gene Glyma.06G263800, Glyma.08G107800, Glyma.10G065000, Glyma.12G014800, Glyma.13G119800, Glyma.15G049200, and Glyma.20G189300 (Tables 4, S3; Figure S3).
Glyma.03G261000 (chromosome 3: 45465440–45469548 bp) for qPC3.1 (47.3–50.4 Mb) regulated pyruvate dehydrogenase (acetyl-transferring) kinase activity; Glyma.10G065000 (chromosome 10: 6209769–6215999 bp) for qPC10.1 (6.0–10.8 Mb) encoded pyruvate kinase; Glyma.06G263800 (chromosome 6: 45124651–45126548 bp) for qPC6.1 (45.1–47.4 Mb) encoded acetyl-CoA carboxylase 1; Glyma.07G137400 (chromosome 7: 16298029–16299140 bp) for qPC7.1 (3.9–8.4) encoded acetyl-CoA carboxylase 2; Glyma.12G014800 (chromosome 12: 1071368–1079413 bp) for qOC12.1 (2.1–7.4 Mb) is an abscisic acid G-protein-coupled receptor; Glyma.13G119800 (chromosome 13: 23237418–23241011 bp) for qOC13.1 (19.9–25.0 Mb) encoded pyruvate dehydrogenase (acetyl-transferring) kinase activity; Glyma.20G189300 (chromosome 20: 42789586–42797140 bp) for qPC20.1 (0.5–3.2 Mb) is a Glycine max pyruvate kinase, cytosolic isozyme; Glyma.08G107800 (chromosome 8: 8296214–8307239 bp) for qOC8.1 (8.3–8.4 Mb) encoded a bifunctional aspartate kinase/homoserine dehydrogenase (AK-HSDH); and Glyma.15G049200 (chromosome 15: 3861401–3863151 bp) for qPC15.1 (1.0–4.9 Mb) encoded a SWEET family.
The characterization of the subgroups for all 320 soybean accessions was largely consistent with geographical origins (Wang et al., 2018; Jeong et al., 2019; Kim et al., 2021; Torkamaneh et al., 2021). Most of the cultivars from China, Russia, and Ukraine belonged to subgroup I, the accessions mainly from the United States and Canada belonged to subgroup II, and subgroup III mainly comprised 62 varieties from Germany, France, and Czechia (Figure 1). To avoid spurious MTAs, an MLM model with PCA and kinship matrix was applied in this study (Zhu et al., 2008). LD decay influenced the precision of GWAS and was affected by allele frequency, population structure, and recombination rate (Liu et al., 2017). The LD decay of the whole genome was 450 kb, indicating that the marker density is sufficient for subsequent GWAS.
In the present study, qPC2.2, qPC-7-1, qPC13.1, qPC15.1, and qPC20.1 related to the PC overlapped with previously reported loci, indicating the reliability of the results (Mao et al., 2013; Warrington et al., 2015; Zhou et al., 2015; Phansak et al., 2016; Zhang et al., 2021). Meta-QTLs were detected consistent with qPC2.2, qPC-7-1, and qPC20.1 in various populations (Pathan et al., 2013; Warrington et al., 2015; Zhou et al., 2015; Kim et al., 2016; Phansak et al., 2016; Van and McHale, 2017). qPC13.1 (24.3–28.6 Mb, explaining 5.2%–9.6% of phenotypic variation) on chromosome 13 was also reported (Mao et al., 2013; Zhang et al., 2021). Previous studies also identified the locus qPC15.1 as an important QTL for PC in soybean (Warrington et al., 2015; Zhou et al., 2015; Phansak et al., 2016; Zhang et al., 2021).
QTL for OC was distributed on all chromosomes except chromosome 9. The loci qOC2.2, qOC6.1, qOC6.2, qOC7.1, qOC8.1, qOC12.2, qOC19.2, and qOC20.2 overlapped with QTL related to oil and its compositional content in previous studies (Chung et al., 2003; Liang et al., 2010; Li et al., 2019; Zhang et al., 2019; Zhang et al., 2021). qOC12.2 (9.7–10.1 Mb) overlapped with the loci for qOC-12-1 (9.1–11.5 Mb), which was identified by Zhang et al. (2021) using 211 diverse soybean accessions genotyped with 355 K SoySNP array. qOC8.1 (8.3–8.4 Mb) overlapped with GqOil-8 (7.9–8.3 Mb), which was detected in several previous studies (Zhang et al., 2019; Zhang et al., 2021). qOC6.1 (9.6–10.4 Mb) was also reported by Zhang et al. (2019) (8.3–9.6 Mb, explaining 7.3% of phenotypic variation) and (9.0–11.2 Mb, explaining 11.2% of phenotypic variation). qOC2.2 (44.7–49.2 Mb) overlapped with the loci for qOil2-1 (42.5–45.4 Mb), identified by Zhang et al. (2019) using 200 soybean accessions. qOC6.2 on chromosome 6 (9.6–10.4 Mb) overlapped with the loci qOC-6-1 (8.5–12.3 Mb), which has been reported by a series of studies with 6.3%–15.6% phenotypic variations (Diers et al., 1992; Hyten et al., 2004; Zhang et al., 2021). qOC7.1 (11.7–15.6 Mb) overlapped with the loci (associated SNP marker rs15774585) from 185 soybean cultivars identified by Li et al. (2019) (10.2–13.0 Mb). qOC8.1 (8.3–8.4 Mb) overlapped with previously reported QTL related to the PC with 5.2%–11.5% phenotypic variations (chromosome 8: 7.9–11.2 Mb) (Lu et al., 2013; Pathan et al., 2013). qOC19.2 on chromosome 19 (43.9–46.8 Mb) was reported by Chapman et al. (2003) (44.4–47.2 Mb, explaining 7.9% of phenotypic variations) and Li et al. (2019) (41.5–44.6 Mb, explaining 6.9% of phenotypic variations), whereas qOC20.2 (31.5–32.9 Mb, explaining 5.2%–11.5% of phenotypic variations) was reported previously by Bachlava et al. (2009) and Zhang et al. (2021). The remaining three QTLs related to the OC were novel. Among the loci identified for PC and OC, 13 loci mentioned above are probably the same as the QTL reported in previous studies, whereas the remaining loci are likely to be new. The stable loci validated by our studies and previous studies indicated that they are less affected by environmental factors.
A total of nine candidate genes were identified for soybean PC and OC. Glyma.15G049200 was identified in the LD block of qPC15.1 and belonged to the SWEET family, which is involved in the transportation of carbohydrates and plays a vital role in transporting sucrose molecules across a membrane. The accumulation of storage substances promotes the development of seeds. Sucrose in seeds could be metabolized to produce protein precursors (Smith et al., 1989). Glyma.08G107800 was located with the LD decay of qPC8.1. Glyma.08G107800 encoded an AK-HSDH. AK-HSDH plays a vital role in the synthesis of amino acids for Lys, Ile, and Met (Kang et al., 2018). The aspartic acid family is the main component in the biosynthesis of other amino acids (Zhang et al., 2018; Zhang et al., 2021).
The oil and protein involved in carbohydrate transport in soybean seeds are complex, mainly including the carbon source competition and distribution process. The candidate gene of loci qPC3.1 (Glyma.03G261000), qPC10.1 (Glyma.10G065000), qOC13.1 (Glyma.13G119800), and qPC20.1 (Glyma.20G189300) encoded pyruvate dehydrogenase (acetyl-transferring) kinase, pyruvate kinase, pyruvate dehydrogenase (acetyl-transferring) kinase, and glycine max pyruvate kinase, respectively. The energy produced by photosynthesis is stored mainly in the form of proteins and lipids (Zhang et al., 2021). Pyruvate dehydrogenase is very important in carbon metabolism, the tricarboxylic acid (TCA) cycle, and glycolysis/gluconeogenesis (Zhang et al., 2019; Yao et al., 2020). Pyruvate dehydrogenase catalyzes the formation of pyruvate, which is the substrate of the Calvin cycle (Yao et al., 2020).
The candidate gene for qPC6.1 (Glyma.06G263800) and qPC7.1 (Glyma.07G137400) encoded acetyl-CoA carboxylase 1 and acetyl-CoA carboxylase 2, respectively. Acetyl-CoA carboxylase provides a carbon skeleton for the synthesis of fatty acids and plays a vital role in the glycolysis pathway (Alfonso, 2020). The metabolites are required for the formation of fatty acids by acetyl coenzyme (Alfonso, 2020; Megha et al., 2022). In addition, starch and sucrose produced by glycolysis can accelerate mitochondrial respiration and the TCA cycle, which is the most critical metabolic pathway for carbohydrate, protein, and fat oxidation (Allen et al., 2009; Liu et al., 2020). Glyma.12G014800 is the candidate gene for qOC12.1 and encoded an abscisic acid G-protein-coupled receptor. Abscisic acid affects the accumulation of assimilates (Manan and Zhao, 2020). G-protein promotes oil increase by regulating the abscisic acid signal transduction (Manan and Zhao, 2020).
Conventional breeding has led to an increase in PC and OC (Li et al., 2020). However, selective breeding is time-consuming and costly (Qiu et al., 2013; Lin et al., 2022). The stable SNPs associated with PC and OC identified in this study, such as qPC3.1, qPC5.2, qPC9.1, qPC10.1, qPC16.1, qOC1.2, and qOC11.1 for PC, and qOC12.1, qOC15.1, qOC16.1, and qOC20.1 for OC, could be used for soybean MAS breeding, and pyramiding favorable alleles will improve PC and OC. Accessions with superior PC and OC alleles (such as R256, R188, R247, R200, R61, R238, R75, R31, R190, R156, R173, and R13 for OC, and R124, R70, R121, R74, R199, R127, R196, R144, R249, R248, R59, R54 and R134 for PC) could be used as parental lines for the molecular improvement of PC and OC in soybean.
In this study, we have identified 23 and 29 loci for PC and OC in 320 soybean accessions, respectively. Of these, 15 and 24 loci are likely to be new. In addition, nine candidate genes involved in protein and oil biosynthesis/metabolism were identified, including lipid transport and metabolism, signal transduction, and plant development pathway. These significantly associated SNPs and varieties with favorable alleles could be used to accelerate the progress of breeding soybean with higher PC and OC.
The datasets presented in this study can be found in online repositories. The names of the repository/repositories and accession number(s) can be found in the article/Supplementary Material.
We declare that these experiments complied with the ethical standards in China.
HJ performed the experiment and drafted the manuscript. HZ, XY, and XS analyzed the physiology data. YT, YW, and QG revised the manuscript. RZ and JZ designed the research. All authors contributed to the article and approved the submitted version.
This work was funded by the Natural Science Foundation of Heilongjiang (YQ2022C030), Heilongjiang Academy of Agricultural Sciences Project (2020FJZX011), the Heilongjiang Scientific Research Institution Foundation (CZKYF2021C001), Establishment of Joint Chinese-Bulgarian Laboratory for Molecular Biology of Crop Germplasm Resources (KY201901009), China Agriculture Research System (CARS-22) and Breeding, Popularization and Industrialization Development of New Soybean Varieties with Middle to Late Maturity, High Yield, High Quality, and Wide Adaptability.
The authors declare that the research was conducted in the absence of any commercial or financial relationships that could be construed as a potential conflict of interest.
All claims expressed in this article are solely those of the authors and do not necessarily represent those of their affiliated organizations, or those of the publisher, the editors and the reviewers. Any product that may be evaluated in this article, or claim that may be made by its manufacturer, is not guaranteed or endorsed by the publisher.
The Supplementary Material for this article can be found online at: https://www.frontiersin.org/articles/10.3389/fpls.2023.1182771/full#supplementary-material
Supplementary Figure 1 | Manhattan plot for the protein content and oil content across all environments. PC: protein content; OC: oil content; E1, E2, E3 E4, E5, and E6 indicate 2017 Harbin, 2018 Harbin, 2019 Harbin, 2021 Harbin, 2018 Mudanjiang, and 2018 Qingan, respectively.
Supplementary Figure 2 | Quantile–quantile (Q–Q) plot for protein content and oil content in all 320 soybean accessions analyzed by the mixed linear model (MLM) in Tassel v5.0. PC: protein content; OC: oil content; E1, E2, E3 E4, E5, and E6 indicate 2017 Harbin, 2018 Harbin, 2019 Harbin, 2021 Harbin, 2018 Mudanjiang, and 2018 Qingan, respectively.
Supplementary Figure 3 | The expression of the candidate gene by qRT-PCR results.
BLUE, Best linear unbiased estimation; PC, Protein content; OC, Oil content; GWAS, Genome-wide association study; hb2, Broad-sense heritability; KASP, Kompetitive allele-specific PCR; LD, Linkage disequilibrium; MAS, Marker-assisted selection; QTL, Quantitative trait locus; R2, Phenotypic variance explained; SNP, Single nucleotide polymorphism.
Akond, M., Liu, S., Boney, M., Kantartzi, S. K., Meksem, K., Bellaloui, N., et al. (2014). Identification of quantitative trait loci (QTL) underlying protein, oil, and five major fatty acids’ contents in soybean. Am. J. Plant Sci. 2014, 5, 158–167. doi: 10.4236/ajps.2014.51021
Alfonso, M. (2020). Improving soybean seed oil without poor agronomics. J. Exp. Bot. 71, 6857–6860. doi: 10.1093/jxb/eraa407
Allen, D. K., Ohlrogge, J. B., Shachar-Hill, Y. (2009). The role of light in soybean seed filling metabolism. Plant J. 58, 220–234. doi: 10.1111/j.1365-313X.2008.03771.x
Alqudah, A. M., Sallam, A., Baenziger, P. S., Börner, A. (2020). GWAS: fast-forwarding gene identification and characterization in temperate cereals: lessons from barley-a review. J. Adv. Res. 22, 119–135. doi: 10.1016/j.jare.2019.10.013
Bachlava, E., Dewey, R. E., Burton, J. W., Cardinal, A. J. (2009). Mapping and comparison of quantitative trait loci for oleic acid seed content in two segregating soybean populations. Crop Sci. 49, 433–442. doi: 10.2135/cropsci2008.06.0324
Beyer, S., Daba, S., Tyagi, P., Bockelman, H., Brown-Guedira, G., Mohammadi, M. (2019). Loci and candidate genes controlling root traits in soybean seedlings-a soybean root GWAS. Funct. Integr. Genomic. 19, 91–107. doi: 10.1007/s10142-018-0630-z
Breseghello, F., Sorrells, M. E. (2006). Association mapping of kernel size and milling quality in soybean (Triticum aestivum l.) cultivars. Genetics 172, 1165–1177. doi: 10.1534/genetics.105.044586
Brown, A. V., Conners, S. I., Huang, W., Wilkey, A. P., Grant, D., Weeks, N. T., et al. (2021). A new decade and new data at SoyBase, the USDA-ARS soybean genetics and genomics database. Nucleic Acids Res. 49, 1496–D1501. doi: 10.1093/nar/gkaa1107
Chan, C., Qi, X., Li, M. W., Wong, F. L., Lam, H. M. (2012). Recent developments of genomic research in soybean. J. Genet. Genomics 39, 317–324. doi: 10.1016/j.jgg.2012.02.002
Chapman, A., Pantalone, V. R., Ustun, A., Allen, F. L., Landau-Ellis, D., Trigiano, R. N., et al. (2003). Quantitative trait loci for agronomic and seed quality traits in an F2 and F4: 6 soybean population. Euphytica 129, 387–393. doi: 10.1023/A:1022282726117
Chaudhary, J., Patil, G. B., Sonah, H., Deshmukh, R. K., Vuong, T. D., Valliyodan, B., et al. (2015). Expanding omics resources for improvement of soybean seed composition traits. Front. Plant Sci. 1021 6. doi: 10.3389/fpls.2015.01021
Chung, J., Babka, H. L., Graef, G. L., Staswick, P. E., Lee, D. J., Cregan, P. B., et al. (2003). The seed protein, oil, and yield QTL on soybean linkage group I. Crop Sci. 43, 1053–1067. doi: 10.2135/cropsci2003.1053
Clemente, T. E., Cahoon, E. B. (2009). Soybean oil: genetic approaches for modification of functionality and total content. Plant Physiol. 151, 1030–1040. doi: 10.1104/pp.109.146282
Diers, B. W., Keim, P., Fehr, W., Shoemaker., R. (1992). RFLP analysis of soybean seed protein and oil content. Theor. Appl. Genet. 83, 608–612. doi: 10.1007/BF00226905
Guo, W., Chen, L., Chen, H., Yang, H., You, Q., Bao, A., et al. (2020). Overexpression of GmWRI1b in soybean stably improves plant architecture and associated yield parameters, and increases total seed oil production under field conditions. Plant Biotechnol. J. 18, 1639–1641. doi: 10.1007/s10681-021-02931-8
He, Q., Xiang, S., Yang, H., Wang, W., Shu, Y., Li, Z., et al. (2021). A genome-wide association study of seed size, protein content, and oil content using a natural population of sichuan and chongqing soybean. Euphytica 217, 1–14. doi: 10.1007/s10681-021-02931-8
Hyten, D. L., Pantalone, V. R., Sams, C. E., Saxton, A. M., Landau-Ellis, D., Stefaniak, T. R., et al. (2004). Seed quality QTL in a prominent soybean population. Theor. Appl. Genet. 109, 552–561. doi: 10.1007/s00122-004-1661-5
Jeong, N., Kim, K. S., Jeong, S., Kim, J. Y., Park, S. K., Lee, J. S., et al. (2019). Korean Soybean core collection: genotypic and phenotypic diversity population structure and genome-wide association study. PloS One 14, e0224074. doi: 10.1371/journal.pone.0224074
Jiang, G. L., Chen, P., Zhang, J., Florez-Palacios, L., Zeng, A., Wang, X., et al. (2018). Genetic analysis of sugar composition and its relationship with protein, oil, and fiber in soybean. Crop Sci. 58, 2413–2421. doi: 10.2135/cropsci2018.03.0173
Kang, W., Zhu, X., Wang, Y., Chen, L., Duan, Y. (2018). Transcriptomic and metabolomic analyses reveal that bacteria promote plant defense during infection of soybean cyst nematode in soybean. BMC Plant Biol. 18, 1–14. doi: 10.1186/s12870-018-1302-9
Karikari, B., Li, S., Bhat, J. A., Cao, Y., Kong, J., Yang, J., et al. (2019). Genome-wide detection of major and epistatic effect QTLs for seed protein and oil content in soybean under multiple environments using high-density bin map. Int. J. Mol. Sci. 20, 979. doi: 10.3390/ijms20040979
Kim, M. Y., Lee, S., Van, K., Kim, T. H., Jeong, S. C., Choi, I. Y., et al. (2010). Whole-genome sequencing and intensive analysis of the undomesticated soybean (Glycine soja sieb. and Zucc.) genome. P Natl. Acad. Sci. U.S.A. 107, 22032–22037. doi: 10.1073/pnas.1009526107
Kim, M. S., Lozano, R., Kim, J. H., Bae, D. N., Kim, S. T., Park, J. H., et al. (2021). The patterns of deleterious mutations during the domestication of soybean. Nat. Commun. 12, 1–14. doi: 10.1038/s41467-020-20337-3
Kim, M., Schultz, S., Nelson, R. L., Diers, B. W. (2016). Identification andfine mapping of a soybean seed protein QTL from PI 407788Aon chromosome 15. Crop Sci. 56, 219–225. doi: 10.2135/cropsci2015.06.0340
Klepadlo, M., Balk, C. S., Vuong, T. D., Dorrance, A. E., Nguyen, H. T. (2019). Molecular characterization of genomic regions for resistance to Pythium ultimum var. ultimum in the soybean cultivar Magellan. Theor. Appl. Genet. 132, 405–417. doi: 10.1007/s00122-018-3228-x
Li, M. W., Wang, Z., Jiang, B., Kaga, A., Wong, F. L., Zhang, G., et al. (2020). Impacts of genomic research on soybean improvement in East Asia. Theor. Appl. Genet. 133, 1655–1678. doi: 10.1007/s00122-019-03462-6
Li, X., Wang, P., Zhang, K., Liu, S., Qi, Z., Fang, Y., et al. (2021). Fine mapping QTL and mining genes for protein content in soybean by the combination of linkage and association analysis. Theor. Appl. Genet. 134, 1095–1122. doi: 10.1007/s00122-020-03756-0
Li, X., Xue, H., Zhang, K., Li, W., Fang, Y., Qi, Z., et al. (2019). Mapping QTLs for protein and oil content in soybean by removing the influence of related traits in a four-way recombinant inbred line population. J. Agric. Sci. 157, 659–675. doi: 10.1017/S0021859620000040
Liang, H. Z., Yu, Y. L., Wang, S. F., Lian, Y., Wang, T. F., Wei, Y. L., et al. (2010). QTL mapping of iso- flavone, oil and protein contents in soybean (Glycine max l. Merr.). Ag. Sci. China 9, 1108–1116. doi: 10.1016/S1671-2927(09)60197-8
Lin, F., Chhapekar, S. S., Vieira, C. C., Da Silva, M. P., Rojas, A., Lee, D., et al. (2022). Breeding for disease resistance in soybean: a global perspective. Theor. Appl. Genet. 135, 3773–3872. doi: 10.1007/s00122-022-04226-5
Liu, J., He, Z., Rasheed, A., Wen, W., Yan, J., Zhang, P., et al. (2017). Genome-wide association mapping of black point reaction in common wheat (Triticum aestivum l.). BMC Plant Biol. 17, 1–12. doi: 10.1186/s12870-017-1167-3
Liu, J., He, Z., Wu, L., Bai, B., Wen, W., Xie, C., et al. (2016). Genome-wide linkage mapping of QTL for black point reaction in bread soybean (Triticum aestivum l.). Theor. Appl. Genet. 129, 2179–2190. doi: 10.1007/s00122-016-2766-3
Liu, X., Jin, J., Wang, G., Herbert, S. J. (2008). Soybean yield physiology and development of high-yielding practices in northeast China. Field Crop Res. 105, 157–171. doi: 10.1016/j.fcr.2007.09.003
Liu, J. Y., Li, P., Zhang, Y. W., Zuo, J. F., Li, G., Han, X., et al. (2020). Three-dimensional genetic networks among seed oil-related traits, metabolites and genes reveal the genetic foundations of oil synthesis in soybean. Plant J. 103, 1103–1124. doi: 10.1111/tpj.14788
Lu, W., Wen, Z., Li, H., Yuan, D., Li, J., Zhang, H., et al. (2013). Identification of the quantitative trait loci (QTL) under-lying water soluble protein content in soybean. Theor. Appl. Genet. 126, 425–433. doi: 10.1007/s00122-012-1990-8
Manan, S., Zhao, J. (2020). Role of glycine max ABSCISIC ACID INSENSITIVE 3 (GmABI3) in lipid biosynthesis and stress tolerance in soybean. Funct. Plant Biol. 48, 171–179. doi: 10.1071/FP19260
Mao, T., Jiang, Z., Han, Y., Teng, W., Zhao., X., Li, W. (2013). Identification of quantitative trait loci underlying seed protein and oil contents of soybean across multi-genetic backgrounds and environments. Plant Breed. 132, 630–641. doi: 10.1111/pbr.12091
Megha, S., Wang, Z., Kav, N. N., Rahman, H. (2022). Genome-wide identification of biotin carboxyl carrier subunits of acetyl-CoA carboxylase in brassica and their role in stress tolerance in oilseed brassica napus. BMC Genomics 23, 1–22. doi: 10.1186/s12864-022-08920-y
Pathan, S. M., Vuong, T., Clark, K., Lee, J. D., Shannon, J. G., Roberts, C. A., et al. (2013). Genetic mapping and confirmation of quantitative trait loci for seed protein and oil contents and seed weight in soybean. Crop Sci. 53, 765–774. doi: 10.2135/cropsci2012.03.0153
Phansak, P., Soonsuwon, W., Hyten, D., Song, Q., Cregan, P., Graef, G., et al. (2016). Multi-population selective genotyping to identify soybean [Glycine max (L.) Merr.] seed protein and oil QTLs. G3 6, 1635–1648. doi: 10.1534/g3.116.027656
Pritchard, J. K., Stephens, M., Rosenberg, N. A., Donnelly, P. (2000). Association mapping in structured populations. Am. J. Hum. Genet. 67, 170–181. doi: 10.1086/302959
Qiu, L. J., Xing, L. L., Guo, Y., Wang, J., Jackson, S. A., Chang, R. Z. (2013). A platform for soybean molecular breeding: the utilization of core collections for food security. Plant Mol. Biol. 83, 41–50. doi: 10.1007/s11103-013-0076-6
Rasheed, A., Wen, W., Gao, F., Zhai, S., Jin, H., Liu, J., et al. (2016). Development and validation of KASP assays for genes underpinning key economic traits in bread soybean. Theor. Appl. Genet. 129, 1843–1860. doi: 10.1007/s00122-016-2743-x
Samanfar, B., Cober, E. R., Charette, M., Tan, L. H., Bekele, W. A., Morrison, M. J., et al. (2019). Genetic analysis of high protein content in ‘AC proteus’ related soybean populations using SSR, SNP, DArT and DArTseq markers. Sci-Rep 9, 1–10. doi: 10.1038/s41598-019-55862-9
Schläppi, M. R., Jackson, A. K., Eizenga, G. C., Wang, A., Chu, C., Shi, Y., et al. (2017). Assessment of five chilling tolerance traits and GWAS mapping in rice using the USDA mini-core collection. Front. Plant Sci. 8, 957. doi: 10.3389/fpls.2017.00957
Sela, H., Ezrati, S., Ben-Yehuda, P., Manisterski, J., Akhunov, E., Dvorak, J., et al. (2014). Linkage disequilibrium and association analysis of stripe rust resistance in wild emmer wheat (Triticum turgidum ssp. dicoccoides) population in Israel. Theor. Appl. Genet. 127, 2453–2463. doi: 10.1007/s00122-014-2389-5
Shi, W., Hao, C., Zhang, Y., Cheng, J., Zhang, Z., Liu, J., et al. (2017). A combined association mapping and linkage analysis of kernel number per spike in common wheat (Triticum aestivum l.). Front. Plant Sci. 8, 1412. doi: 10.3389/fpls.2017.01412
Smith, A. J., Rinne, R. W., Seif, R. D. (1989). Phosphoenolpyruvate carboxylase and pyruvate kinase involvement in protein and oil biosynthesis during soybean seed development. Crop Sci. 29, 349–353. doi: 10.2135/cropsci1989.0011183X002900020024x
Song, J., Li, Z., Liu, Z., Guo, Y., Qiu, L. J. (2017). Next-generation sequencing from bulked-segregant analysis accelerates the simultaneous identification of two qualitative genes in soybean. Front. Plant Sci. 8, 919. doi: 10.3389/fpls.2017.00919
Tian, X., Zhang, K., Liu, S., Sun, X., Li, X., Song, J., et al. (2020). Quantitative trait locus analysis of protein and oil content in response to planting density in soybean (Glycine max [L.] Merri.) seeds based on SNP linkage mapping. Front. Genet. 11, 563. doi: 10.3389/fgene.2020.00563
Torkamaneh, D., Laroche, J., Valliyodan, B., O’Donoughue, L., Cober, E., Rajcan, I., et al. (2021). Soybean (Glycine max) haplotype map (GmHapMap): a universal resource for soybean translational and functional genomics. Plant Biotechnol. J. 19, 324–334. doi: 10.1111/pbi.13466
Ullah, A., Akram, Z., Malik, S. I., Khan, K. S. U. (2021). Assessment of phenotypic and molecular diversity in soybean [Glycine max (L.) merr.] germplasm using morpho-biochemical attributes and SSR markers. Genet. Resour. Crop Ev. 68, 2827–2847. doi: 10.1007/s10722-021-01157-w
Van, K., McHale, L. K. (2017). Meta-analyses of QTLs associated with protein and oil contents and compositions in soybean [Glycine max (L.) merr.] seed. Int. J. Mol. Sci. 18, 1180. doi: 10.3390/ijms18061180
Vuong, T. D., Sonah, H., Meinhardt, C. G., Deshmukh, R., Kadam, S., Nelson, R. L., et al. (2015). Genetic architecture of cyst nematode resistance revealed by genome-wide association study in soybean. BMC Genomics 16, 1–13. doi: 10.1186/s12864-015-1811-y
Wang, Y., Hou, J., Liu, H., Li, T., Wang, K., Hao, C., et al. (2019). TaBT1, affecting starch synthesis and thousand kernel weight, underwent strong selection during soybean improvement. J. Exp. Bot. 70, 1497–1511. doi: 10.1093/jxb/erz032
Wang, Y. Y., Li, Y. Q., Wu, H. Y., Hu, B., Zheng, J. J., Zhai, H., et al. (2018). Genotyping of soybean cultivars with medium-density array reveals the population structure and QTNs underlying maturity and seed traits. Front. Plant Sci. 9, 610. doi: 10.3389/fpls.2018.00610
Wang, S., Liu, S., Wang, J., Yokosho, K., Zhou, B., Yu, Y. C., et al. (2020). Simultaneous changes in seed size, oil content and protein content driven by selection of SWEET homologues during soybean domestication. Nat. Sci. Rev. 7, 1776–1786. doi: 10.1093/nsr/nwaa110
Wang, Z., Tian, Z. (2015). Genomics progress will facilitate molecular breeding in soybean. Sci. China Life. Sci. 58, 813–815. doi: 10.1007/s11427-015-4908-2
Warrington, C. V., Abdel-Haleem, H., Hyten, D. L., Cregan, P. B., Orf, J. H., Killam, A. S., et al. (2015). QTL for seed protein and amino acids in the benning × danbaekkong soybean population. Theor. Appl. Genet. 128, 839–850. doi: 10.1007/s00122-015-2474-4
Xue, Y., Gao, H., Liu, X., Tang, X., Cao, D., Luan, X., et al. (2022). QTL mapping of palmitic acid content using specific-locus amplified fragment sequencing (SLAF-seq) genotyping in soybeans (Glycine max l.). Int. J. Mol. Sci. 23, 11273. doi: 10.3390/ijms231911273
Yao, Y., You, Q., Duan, G., Ren, J., Chu, S., Zhao, J., et al. (2020). Quantitative trait loci analysis of seed oil content and composition of wild and cultivated soybean. BMC Plant Biol. 20, 1–13. doi: 10.1186/s12870-019-2199-7
Yuan, J., Wen, Z., Gu, C., Wang, D. (2014). Introduction of high throughput and cost-effective SNP genotyping platforms in soybean. Plant Genetics Genomics Biotechnol. 2, 90–94. doi: 10.5147/pggb.v2i1.155
Zafar, S., Li, Y. L., Li, N. N., Zhu, K. M., Tan, X. L. (2019). Recent advances in enhancement of oil content in oilseed crops. J. Biotechnol. 301, 35–44. doi: 10.1016/j.jbiotec.2019.05.307
Zahid, G., Aka Kaçar, Y., Dönmez, D., Küden, A., Giordani, T. (2022). Perspectives and recent progress of genome-wide association studies (GWAS) in fruits. Mol. Biol. Rep. 521, 115381. doi: 10.1007/s11033-021-07055-9
Zhang, S., Hao, D., Zhang, S., Zhang, D., Wang, H., Du, H., et al. (2021). Genome-wide association mapping for protein, oil and water-soluble protein contents in soybean. Mol. Genet. Genomics 296, 91–102. doi: 10.1007/s00438-020-01704-7
Zhang, M., Liu, S., Wang, Z., Yuan, Y., Zhang, Z., Liang, Q., et al. (2022). Progress in soybean functional genomics over the past decade. Plant Biotechnol. J. 20, 256–282. doi: 10.1111/pbi.13682
Zhang, J., Wang, X., Lu, Y., Bhusal, S. J., Song, Q., Cregan, P. B., et al. (2018). Genome-wide scan for seed composition provides insights into soybean quality improvement and the impacts of domestication and breeding. Mol. Plant 11, 460–472. doi: 10.1016/j.molp.2017.12.016
Zhang, T., Wu, T., Wang, L., Jiang, B., Zhen, C., Yuan, S., et al. (2019). A combined linkage and GWAS analysis identified QTLs linked to soybean seed protein and oil content. Int. J. Mol. Sci. 20, 5915. doi: 10.3390/ijms20235915
Zhang, D., Zhang, H., Hu, Z., Chu, S., Yu, K., Lv, L., et al. (2019). Artificial selection on GmOLEO1 contributes to the increase in seed oil during soybean domestication. PLoS Genet. 15, e1008267. doi: 10.1371/journal.pgen.1008267
Zhou, Z., Jiang, Y., Wang, Z., Gou, Z., Lyu, J., Li, W., et al. (2015). Resequencing 302 wild and cultivated accessions identifies genes related to domestication and improvement in soybean. Nat. Biotechnol. 33, 408–414. doi: 10.1038/nbt.3096
Keywords: genome-wide association study (GWAS), marker-assisted selection (MAS), protein content, oil content, soybean, Glycine max L.
Citation: Jin H, Yang X, Zhao H, Song X, Tsvetkov YD, Wu Y, Gao Q, Zhang R and Zhang J (2023) Genetic analysis of protein content and oil content in soybean by genome-wide association study. Front. Plant Sci. 14:1182771. doi: 10.3389/fpls.2023.1182771
Received: 09 March 2023; Accepted: 09 May 2023;
Published: 06 June 2023.
Edited by:
Yi-Hong Wang, University of Louisiana at Lafayette, United StatesReviewed by:
Hengyou Zhang, Chinese Academy of Sciences (CAS), ChinaCopyright © 2023 Jin, Yang, Zhao, Song, Tsvetkov, Wu, Gao, Zhang and Zhang. This is an open-access article distributed under the terms of the Creative Commons Attribution License (CC BY). The use, distribution or reproduction in other forums is permitted, provided the original author(s) and the copyright owner(s) are credited and that the original publication in this journal is cited, in accordance with accepted academic practice. No use, distribution or reproduction is permitted which does not comply with these terms.
*Correspondence: Jumei Zhang, emptMzEyQGFsaXl1bi5jb20=; Rui Zhang, enIwNzA1QDEyNi5jb20=
†These authors have contributed equally to this work
Disclaimer: All claims expressed in this article are solely those of the authors and do not necessarily represent those of their affiliated organizations, or those of the publisher, the editors and the reviewers. Any product that may be evaluated in this article or claim that may be made by its manufacturer is not guaranteed or endorsed by the publisher.
Research integrity at Frontiers
Learn more about the work of our research integrity team to safeguard the quality of each article we publish.