- 1Department of Horticulture, University of Arkansas, Fayetteville, AR, United States
- 2Sugarcane Research Unit, Untied State Department of Agriculture – Agriculture Research Service (USDA-ARS), Houma, LA, United States
- 3Henan Academy of Crops Molecular Breeding, National Centre for Plant Breeding, Zhengzhou, China
Soybean brown rust (SBR), caused by Phakopsora pachyrhizi, is a devastating fungal disease that threatens global soybean production. This study conducted a genome-wide association study (GWAS) with seven models on a panel of 3,082 soybean accessions to identify the markers associated with SBR resistance by 30,314 high quality single nucleotide polymorphism (SNPs). Then five genomic selection (GS) models, including Ridge regression best linear unbiased predictor (rrBLUP), Genomic best linear unbiased predictor (gBLUP), Bayesian least absolute shrinkage and selection operator (Bayesian LASSO), Random Forest (RF), and Support vector machines (SVM), were used to predict breeding values of SBR resistance using whole genome SNP sets and GWAS-based marker sets. Four SNPs, namely Gm18_57,223,391 (LOD = 2.69), Gm16_29,491,946 (LOD = 3.86), Gm06_45,035,185 (LOD = 4.74), and Gm18_51,994,200 (LOD = 3.60), were located near the reported P. pachyrhizi R genes, Rpp1, Rpp2, Rpp3, and Rpp4, respectively. Other significant SNPs, including Gm02_7,235,181 (LOD = 7.91), Gm02_7234594 (LOD = 7.61), Gm03_38,913,029 (LOD = 6.85), Gm04_46,003,059 (LOD = 6.03), Gm09_1,951,644 (LOD = 10.07), Gm10_39,142,024 (LOD = 7.12), Gm12_28,136,735 (LOD = 7.03), Gm13_16,350,701(LOD = 5.63), Gm14_6,185,611 (LOD = 5.51), and Gm19_44,734,953 (LOD = 6.02), were associated with abundant disease resistance genes, such as Glyma.02G084100, Glyma.03G175300, Glyma.04g189500, Glyma.09G023800, Glyma.12G160400, Glyma.13G064500, Glyma.14g073300, and Glyma.19G190200. The annotations of these genes included but not limited to: LRR class gene, cytochrome 450, cell wall structure, RCC1, NAC, ABC transporter, F-box domain, etc. The GWAS based markers showed more accuracies in genomic prediction than the whole genome SNPs, and Bayesian LASSO model was the ideal model in SBR resistance prediction with 44.5% ~ 60.4% accuracies. This study aids breeders in predicting selection accuracy of complex traits such as disease resistance and can shorten the soybean breeding cycle by the identified markers
Introduction
Soybean brown rust (SBR) is one of the most devastating fungal diseases of soybean (Glycine max) (Hartman et al., 2005). It first emerged around 1900 as a threat to soybean production in China and Japan and has since spread globally, in part due to human activities and meteorological phenomena (Hartman et al., 1991). The disease arrived in Africa and the Pacific Islands in the 1980s and 1990s and later reached the American continents in the 2000s (Miles et al., 2004). The risk of SBR attracted more attention with the disease outbreak in China in 1975 and in Brazil in 2001, that caused 10 billion US dollar losses in each country (Yorinori et al., 2005; Godoy et al., 2016). Comparing to the native American rust pathogen (Phakopsora meibomiae), the exotic one (Phakopsora pachyrhizi) was much more aggressive and caused an epidemic on soybean in South America and spread to North America (Pivonia and Yang, 2004).
Soybean plants are susceptible to SBR at any stage of growth and development and Phakopsora pachyrhizi can quickly spread over a long-range through wind-borne urediniospores (Isard et al., 2005). Therefore, it is important to develop control strategies for controlling SBR. Currently, the SBR can be managed by applying fungicides and employing specific cultivation practices (Levy, 2005). However, considering the high cost and the harm to non-target beneficial fungi, a more economic, safer, and environmental friendly solution is to raise varieties’ own resistance by developing new resistance lines through breeding or engineering (Bromfield and Hartwig, 1980). In the past 30 years, the well-known Rpp 1–7 genes were mapped to chromosome 3, 6, 16, 18, and 19 (Garcia et al., 2008; Pandey et al., 2011; Li et al., 2012; Kashiwa et al., 2020). However, Rpp genes were race-specific and provided resistance exclusively to specific P. pachyrhizi isolates. Currently, there is no resistant soybean genotype that can ward off all known P. pachyrhizi isolates (Childs et al., 2018a). In addition, Rpp gene-mediated resistance can be overcome swiftly in the field due to pathogen’s adaptation and evolution to resistant host (Godoy and Meyer, 2020). Pyramiding three or more Rpp genes into one genotype to obtain broader and/or more durable resistance has been reported on other crops like wheat or barley, but traditional breeding is still time-consuming and may introduce unwanted traits (Childs et al., 2018a). Another promising strategy for sustainable and effective SBR resistance is to utilize alternative R gene combinations and dynamic turnover in the field (Childs et al., 2018a). However, the identity of these Rpp genes needs to be revealed (Gebremedhn et al., 2020). Under the current conditions, it is also impractical to rely only on several major genes or combinations of these genes to control the SBR disease in field production.
In addition to major genes, many recent molecular studies have revealed more disease-resistant pathways in soybeans (Childs et al., 2018b). The resistance usually occurs in the form of signals, transcription factors, NB-LRR, or secondary metabolites (Gebremedhn et al., 2020; Waheed et al., 2021). They usually improve not only the resistance to a particular pathogen but the overall resistance of the plant as well. In addition, many minor resistance/tolerance genes are widely distributed throughout the whole soybean genome and exhibit partial defense response (PDR) to SBR (Langenbach et al., 2016). PDR is characterized by low infection frequency, long-lasting latency, small lesions, and reduced spore production per uredinium (Langenbach et al., 2016). At the molecular level, their specific functions are sometimes very similar or overlapping to the context components; however, they are more complex and obscure (Langenbach et al., 2016). Screening for or silencing susceptibility is another strategy that can provide durable disease resistance in breeding, because of susceptible (S) gene function either as susceptibility factors or suppressors of plant defense, thus potential targets of fungal effectors (De Wit, 1992). For example, absence of the S gene Mlo in barley results in an incompatibility interaction with Blumeria graminis hordei that resembles nuclear hormone receptors (Büschges et al., 1997; Lucas, 2020). However, the identification and mapping of S gene are more difficult than those of major R genes by linkage mapping, and only one [Cys(2)His(2) zinc finger TF palmate-like pentafoliata1, PALM1] would classify as a S gene so far (Uppalapati et al., 2012).
Molecular marker-assisted selection (MAS) has been applied in soybean breeding to accelerate the development of disease-resistant varieties, and the GWAS is of vital help to MAS (He et al., 2014). Comparing with linkage mapping, GWAS can not only find the major genes, but also has the incomparable ability to map and identify the minor and S genes. Moreover, since the mapping populations such as natural population and multi-parent advanced generation inter-cross, contain more diversity, the markers developed have more universal applicability (Visscher et al., 2012). So far, only one SBR-related GWAS has been reported by Chang et al. (2016), who used GWAS to discover five SBR-related loci from USDA germplasm. Genomic selection (GS) has gained popularity in recent years in modern and large-scale crop breeding programs. GS can predict the breeding value of an individual plant based on its genotype to estimate the field performance of the plant, whereas MAS relies on the detection of a few QTLs using a simple linear model. Therefore, molecular breeding would shift from marker-assisted selection to genomic selection, as the genetic architecture of resistance changes from a single major R gene to multiple minor diffusion gene architectures (Poland and Rutkoski, 2016). Additionally, GS has been reported to be a useful tool in soybean breeding to predict a wide range of traits, including both agronomic and quality traits (Lorenz et al., 2011). However, no research has been done with respect to investigating GS accuracy for SBR resistance/tolerance.
The objectives of this study were to identify SBR resistance-associated SNP markers and to characterize the ability of genomic prediction in order to use SNP markers in selecting soybean breeding lines highly resistant to SBR.
Materials and methods
Plant materials and phenotyping
SBR disease scores and phenotyping data of 3,082 soybean accessions (Table S1) were downloaded from the USDA GRIN website (https://npgsweb.ars-grin.gov/gringlobal/method?id=492634) (Miles et al., 2006). Based on the website, a greenhouse study was initiated. Soybean plants of 3,082 accessions were spray-inoculated between the first and second trifoliate stage with a mixture of urediniospores (60,000 spores per ml) from four Phakopsora pachyrhizi isolates, incubated overnight in a dew chamber at 22–25°C, and placed in a greenhouse at 20–25°C for 14 days. Disease severity was evaluated on the first trifoliate leaves for most accessions; however, the unifoliate leaves were evaluated for a few accessions due to slow germination (Miles et al., 2006). Based on the symptom and lesion development, a disease severity scale of 1 to 5 was used, where 1 = no visible symptom, 2 = light infection: only a few small (less than 1 cm) rust lesion present on the leaves, 3 = light to moderate infection: moderately sized (1–2 cm) rust lesion present on a limited number of leaves, 4 = moderate to severe infection: large (greater than 2 cm) rust lesion present on a significant number of leaves, and 5 = severe infection: nearly all leaves are covered in large rust lesion, and the disease is causing a significant damage to the plant growth (Walker et al., 2011).
Genotyping
The Soy50K SNP Infinium Chips (Song et al., 2013) and a total of 42,292 SNPs across 3,082 soybean accessions were downloaded from the Soybase at https://www.soybase.org/snps/download.php. SNPs with >10% missing data, >8% heterozygous genotypes, and <10% minor allele frequencies (MAF) were removed, and 30,314 SNPs were included in the GWAS study.
Population structure and genetic diversity
LEA is an R package for population structure and genomic signature analysis of local adaptation. The inference algorithms used by R are based on a fast version of structure available from the R package LEA (Frichot and François, 2015). The structure analysis identifies K clusters by measuring an optimum ΔK based on the SNP data provided. A preliminary analysis was performed in multiple runs by inputting successive values of K from 2 to 20. After an optimum K was determined, each soybean accession was assigned to a cluster (Q) based on the probability that the accession belonged to that cluster. The cut-off probability for the assignment to a cluster was 0.5. Based on the optimum K, a bar plot with “Sort by Q” was obtained to visualize the population structure among the 3,082 accessions. Phylogenetic relationships among the accessions was generated by TASSEL 5.2.13 and phylogenetic tree was drawn using R package: Phytologist and Phytools (Revell, 2012). During the drawing of the phylogeny trees, the population structure and the cluster information were imported for the combined analysis of genetic diversity. For sub-tree of each Q (cluster), the shape of “Node/Subtree Marker” and the “Branch Line” was drawn using the same color scheme of the STRUCTURE analysis.
Linkage disequilibrium analysis and SNP based haplotype blocks
TASSEL 5.0 (Bradbury et al., 2007) was used to calculate the linkage disequilibrium (LD) for all pairwise loci. Only SNPs with a minor allele frequency (MAF) greater than 0.10 and less than 10% missing data were included in the LD estimation process. Haplotype blocks (HAP) were estimated by Plink 2.0 (Purcell et al., 2007) within 200kb (r2 ≈ 0.4), and a minimum threshold value 0.05 for MAF.
Genome-wide association study
GWAS was performed using the Generalized Linear Model (GLM), Mixed Linear Model (MLM) (Jiang and Nguyen, 2021), Compressed Mixed Linear Model (CMLM), Multiple Loci Mixed Model (MLMM) (Wen et al., 2018), Settlement of MLM Under Progressively Exclusive Relationship (SUPER) (Wang et al., 2014), Fixed and Random Model Circulating Probability Unification (FarmCPU) (Liu et al., 2016), and Bayesian-information and Linkage-disequilibrium Iteratively Nested Keyway (BLINK) (Wang et al., 2014) in R software GAPIT 3 (Genomic Association and Prediction Integrated Tool version 3) (Wang and Zhang, 2021; Lipka et al., 2012; https://zzlab.net/GAPIT/index.html; https://github.com/jiabowang/GAPIT3) by setting PCA = 6, with the Kinship for MLM, CMLM, MLMM, SUPER; and Pseudo QTNs for FarmCPU and BLINK.
SNP selection accuracy and selection efficiency
The accuracy and efficiency of SNP selection were computed to evaluate the significant SNP by the allele proportion in the population.
Selection accuracy (SA) = 100*[(Number of S or R genotypes with the favorable SNP allele)/(Number of R genotypes with the favorable SNP allele + Number of S genotypes with the favorable SNP allele)]/ΔE, where ΔE = E1/E2, E1 = Observed number of S or R genotypes/(Number of R genotypes + Number of S genotypes); E2= Expected number of S or R genotypes/(Number of R genotypes + Number of S genotypes).
Selection efficiency (SE) =100*[(Number of S or R genotypes with the favorable SNP allele)/(Total number of S or R genotypes)]/ΔF, where ΔF = F1/F2, F1 = Observed allele frequency of SNP, and F2 = Expected allele frequency of SNP. In this study we set the E2 and F2 as an ideal equilibrium value (50%).
Candidate gene prediction
Candidate genes were selected based on the peak significant SNP in each LD region located within 50 kb on either side of significant SNPs (Zhang et al., 2016), and furtherly by 0 kb (on the gene), 1 kb, 5 kb, 10 kb, 20 kb, 30 kb, and 50 kb, respectively. Candidate genes were retrieved from the reference annotation of the soybean reference genome Wm82.a2.v1 from the SoyBase (http://www.soybase.org) and the Phytozome database (https://phytozome.jgi.doe.gov).
Genomic prediction
GP was conducted using seven SNP sets: All SNP set (30,314 SNPs) and six GWAS-derived SNP marker sets. The six GWAS-derived SNP marker sets consisted of those significant SNPs from highest LOD [–log(P-value)] to low LOD value (2.0) to make each set as 28, 100, 500, 1,000, 2,000, and 5,000 SNPs, respectively. Genomic estimated breeding value (GEBV) was computed using five statistical models: Ridge regression best linear unbiased predictor (rrBLUP) (Endelman, 2011), Genomic best linear unbiased predictor (gBLUP) (Zhang et al., 2007), Bayesian least absolute shrinkage and selection operator (Bayesian LASSO) (Heslot et al., 2012), Random Forest (RF) (Poland et al., 2012), and Support vector machines (SVM) (Ogutu et al., 2011) (Table S2).
A five-fold cross-validation was performed for each GP. The association panel was randomly divided into 5 disjoint subsets, 4 subsets were used as training set, and the remaining set was considered testing set. A total of 100 replications were conducted at each fold. Mean and standard errors corresponding to each fold were computed. Genomic prediction accuracy was obtained by computing the Pearson’s correlation coefficient (r) between GEBV and the observed phenotypic value for the testing set (Shikha et al., 2017).
Results
Germplasm evaluation of Phakopsora pachyrhizi
Out of 3,082 soybean accessions evaluated for TAN lesion type, 71 (2.3%) were rated 1~2, 1,009 (32.7%) were rated 2.3~3, 1,746 (56.7%) were rated 3.1~4; and 256 (8.3%) were rated 4.2~5 in a rating scale of 1 to 5. Accessions with a mean severity of 2.7 or less (299, 9.5%) were considered resistant, while those with a mean severity of 4.0 or more (791, 25.6%) were considered susceptible. Accessions between the two categories were considered moderate. There was a large range in the distribution of each category (Figure 1). Majority of accessions displayed a disease severity rating of 3 or 4 being susceptible to SBR.
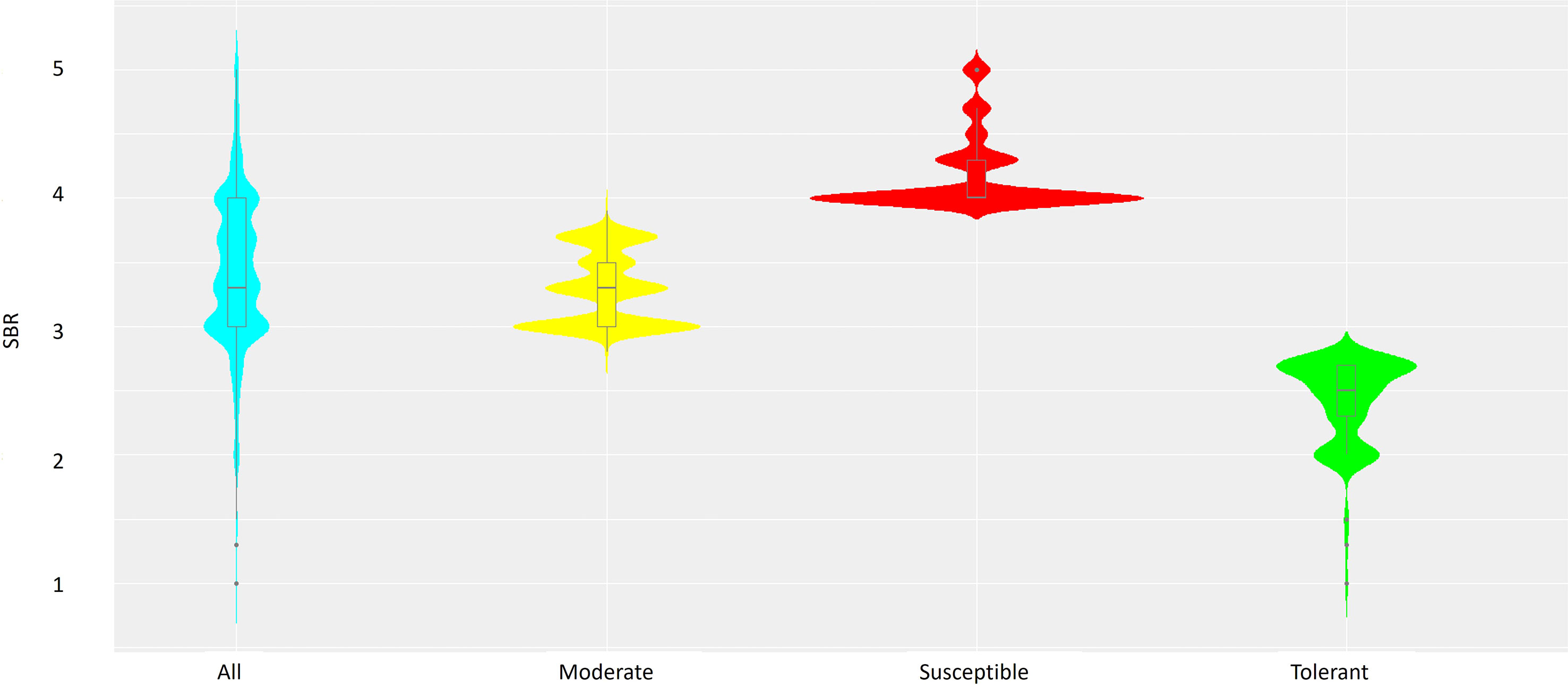
Figure 1 Combined violin-boxplots based on SBR ranking of the 3,082 soybean accessions, including Susceptible (red), Moderate (yellow) and Tolerant (green) groups.
SNP profile
A total of 30,314 high quality SNPs were used to perform GWAS in the soybean accessions. Number of SNPs per chromosome ranged from 1,027 on chr20 to 1,898 on Chr16, with an average of 1,515.7 SNPs (Figure 2). The average distance between two SNPs per chromosome varied from 23.6 kb to 46.6 kb, with an average of 33.1 kb. The shortest average distance between SNPs was found on Chr18, whereas the longest one was on Chr20. Average MAF per chromosome ranged between 25.8% and 30.1%, with an average of 28.7% (Table S3). Percentage of heterozygous SNPs across all chromosomes were below 0.7%, and the percentage of missing SNPs per chromosome varied from 0.3% to 0.7%.
Population structure and LD haplotype
The structure analysis helped identify the most promising genetic variations to better understand the genetic basis of the trait. The population structure of the soybean accessions was analyzed using the R packages “LEA” and the peak of ΔK was observed at K = 6, indicating of the presence of six subpopulations or clusters (Figure 3A). A total of 337 (10.9%) accessions were assigned to subpopulation Q1; 306 (9.9%) assigned to Q2; 543 (17.6%) assigned to Q3; 534 (17.3%) assigned to Q4; 358 assigned to Q5; and 1,004 (32.5%) assigned to Q6 (Figure 3B). Phylogenetic analysis and population admixture map using R packages “Phytool” and “LEA” also revealed that the clustering of accessions was consistent with that inferred by structure K = 6 (Figure 3C). Additionally, there was a clear tendency of clustering by geographical areas. The controlling for population structure by taking geography into account is crucial for accurate GWAS results and for identifying true genetic associations with the trait of interest. As Q6 was dominant in South and Central China and Southeast Asia, Q3 and Q4 were main populations in Northeast and Northwest Asia, and the population in Europe was dominated by Q2 and Q5 (Figure 3D and Table S1). Kinship matrix, based on 30,314 polymorphic SNPs for the studied genotypes, indicated that there was no clear clustering among the 3082 genotypes.
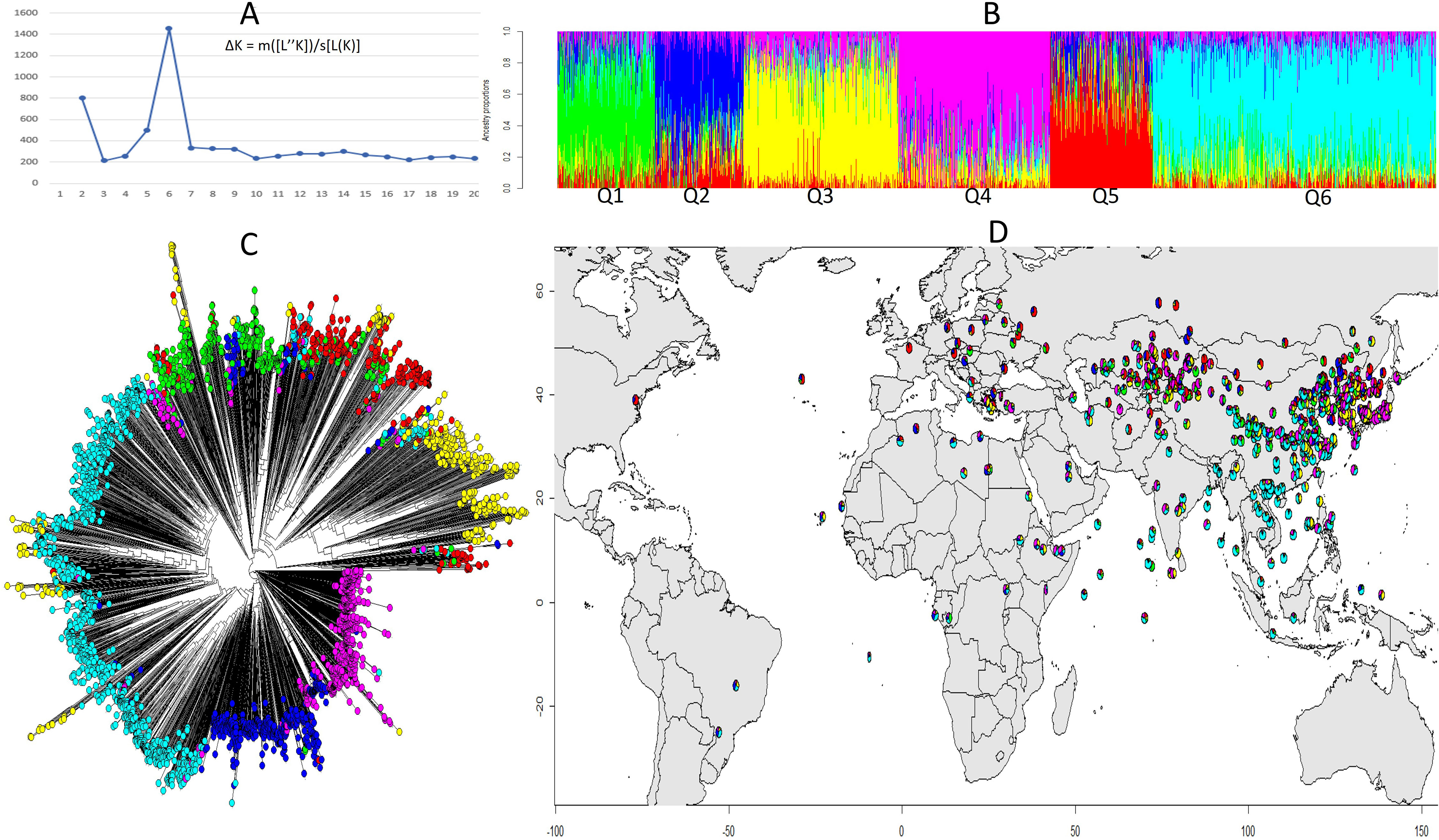
Figure 3 Structural and phylogenetic analysis of 3,082 soybean accessions based on 30,314 SNPs. (A) Delta K values for different numbers of populations assumed (K=20) in the STRUCTURE analysis. (B) Classification of soybean accessions in six groups (K=6) using STRUCTURE. The distribution of accessions to different populations is color coded, Q1 (green), Q2 (blue), Q3 (yellow), Q4 (pink), Q5 (red), Q6 (cyan). The x-axis shown the accessions of each subgroup, and the number on the y-axis shows the Q likelihood of accessions. (C) Phylogenetic analysis of the 3,082 soybean accessions with the corresponded labels used in (B). (D) Geographical distribution of the soybean accessions by colored pie chart corresponding with the group proportion (B).
We examined the linkage disequilibrium (LD) decay patterns by 30,314 genome-wide SNPs. To visualize the LD decay patterns across distances, we plotted the LD decay curves by GAPIT 3 (Figure 4). The LD decay curves showed a clear distance-dependent pattern, with steeper decay curves at longer distances. Specifically, at a distance of 103 kb, the LD decayed with an R2 value of 0.6, indicating a relatively strong LD correlation between nearby variants. At 216 kb, the LD decayed with an R2 value of 0.4, indicating a moderate level of LD correlation between nearby variants. Finally, at 296 kb, the LD decayed with an R2 value of 0.2, indicating a weak level of LD correlation between nearby variants (Figure S1). A total of 4,940 haplotype blocks were identified based on 30,314 SNPs. Number of blocks per chromosome varied from 170 on Chr11 to 357 on Chr18. Number of SNPs within each block varied from 2 to 67. Many haplotype blocks contained more than two significant SNP markers, for example, Gm01_47,462,126, Gm01_47,476,910, Gm01_47,481,216, Gm01_47,495,955, Gm01_47,503,665, Gm01_47,516,500, and Gm01_47,548,257 were in the same haplotype block on Chr1 (Table S4).
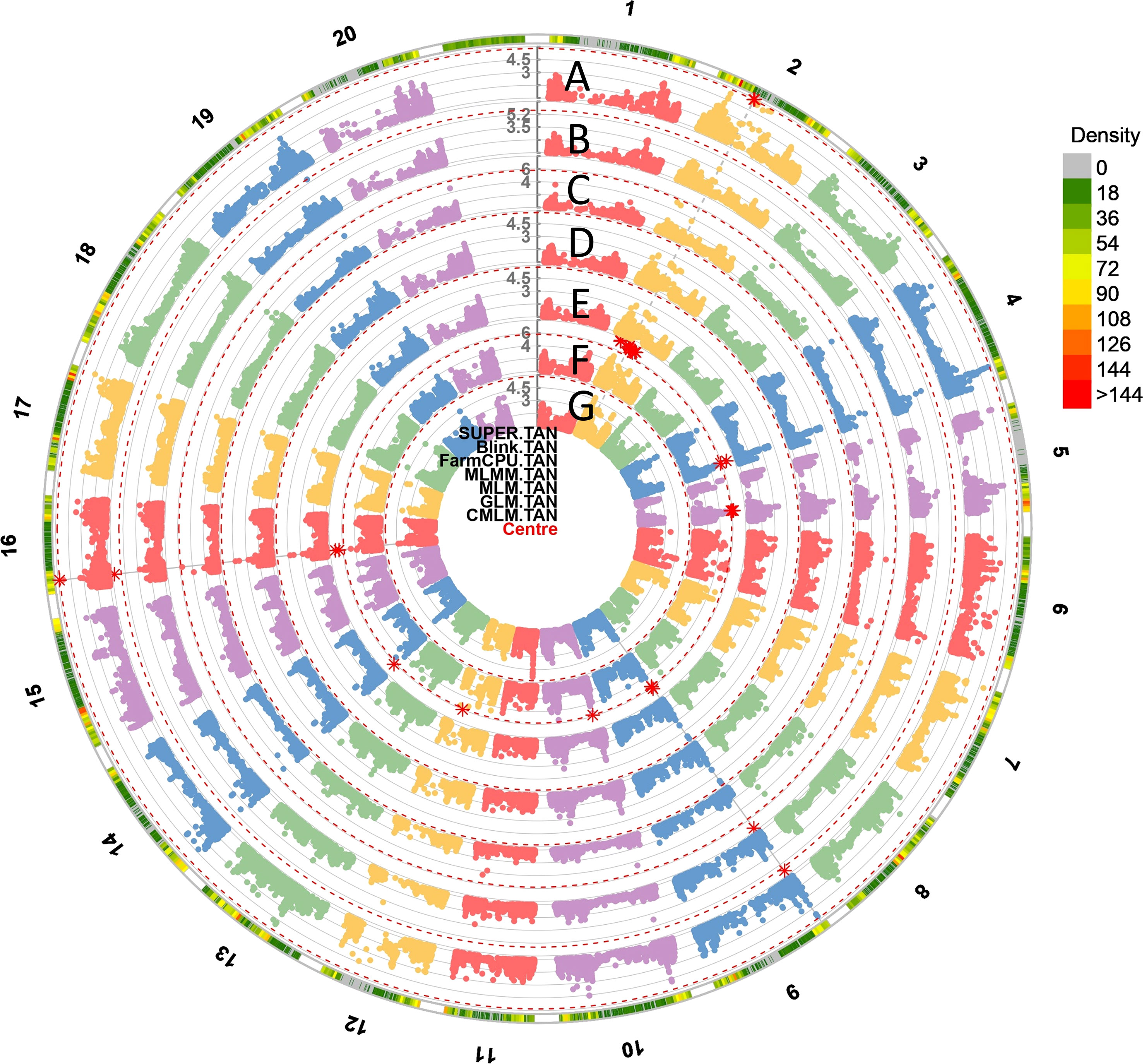
Figure 4 The circular Manhattan plots of seven GWAS models: (A) Settlement of MLM Under Progressively Exclusive Relationship (SUPER), (B) Bayesian-information and Linkage-disequilibrium Iteratively Nested Keyway (BLINK), (C) Fixed and Random Model Circulating Probability Unification (FarmCPU), (D) Multiple Loci Mixed Model (MLMM), (E) Mixed Linear Model (MLM), (F) Generalized Linear Models (GLM) and (G) Compressed Mixed Linear Model (CMLM) for SBR. The red asterisk points to the significant spots associated with SBR on 20 chromosomes. The outmost circle indicates the hotspots associated with SBR response among seven models.
Genome-wide association study
The high convergence and consistency of the GWAS were observed among seven models. For example, the top six significant SNPs from the FarmCPU model including: Gm09_1,951,644 (10.06), Gm20_36,724,867 (6.54), Gm03_38,913,029 (6.10), Gm19_44,734,953 (5.7), Gm02_7,235,181 (5.18), and Gm04_47,132,429 (5.06) also had the high LOD values, which were at least 5.20, 2.67, 3.77, 3.59, 3.69, and 4.00 in other models. SNPs Gm04_45,884,688, Gm10_39,142,024, Gm14_2,492,139, Gm16_4,935,328, etc. were significant among all seven models (Figures 4, S2). A total of 100 SNPs were collected in this study by considering both model consistency and significance (Table S5). These SNPs were positioned at 47 haplotype blocks (Table S4). Then the top 28 SNPs with LOD > 5.50 were listed in Table 1 for future discussion. These 28 SNPs were located on 13 chromosomes (Chr. 2, 3, 4, 6, 8, 9, 10, 12, 13, 14, 16, 19, and 20), indicating their wide distribution and presence of genes that confer SBR resistance across the genome. Several SNPs were found in the same blocks, such as Gm02_7,235,181 and Gm02_7,234,594 in block 436; Gm09_1,944,730, Gm09_1,943,831, and Gm09_1,951,644 in block 1902; Gm10_5,573,877, Gm10_5,573,007, Gm10_5,559,592, Gm10_5,541,691, and Gm10_5,578,693 in block 2331; and Gm10_39,142,024 and Gm10_3,9147,121 in block 2215, which might be due to the gene clustering or pleiotropy.
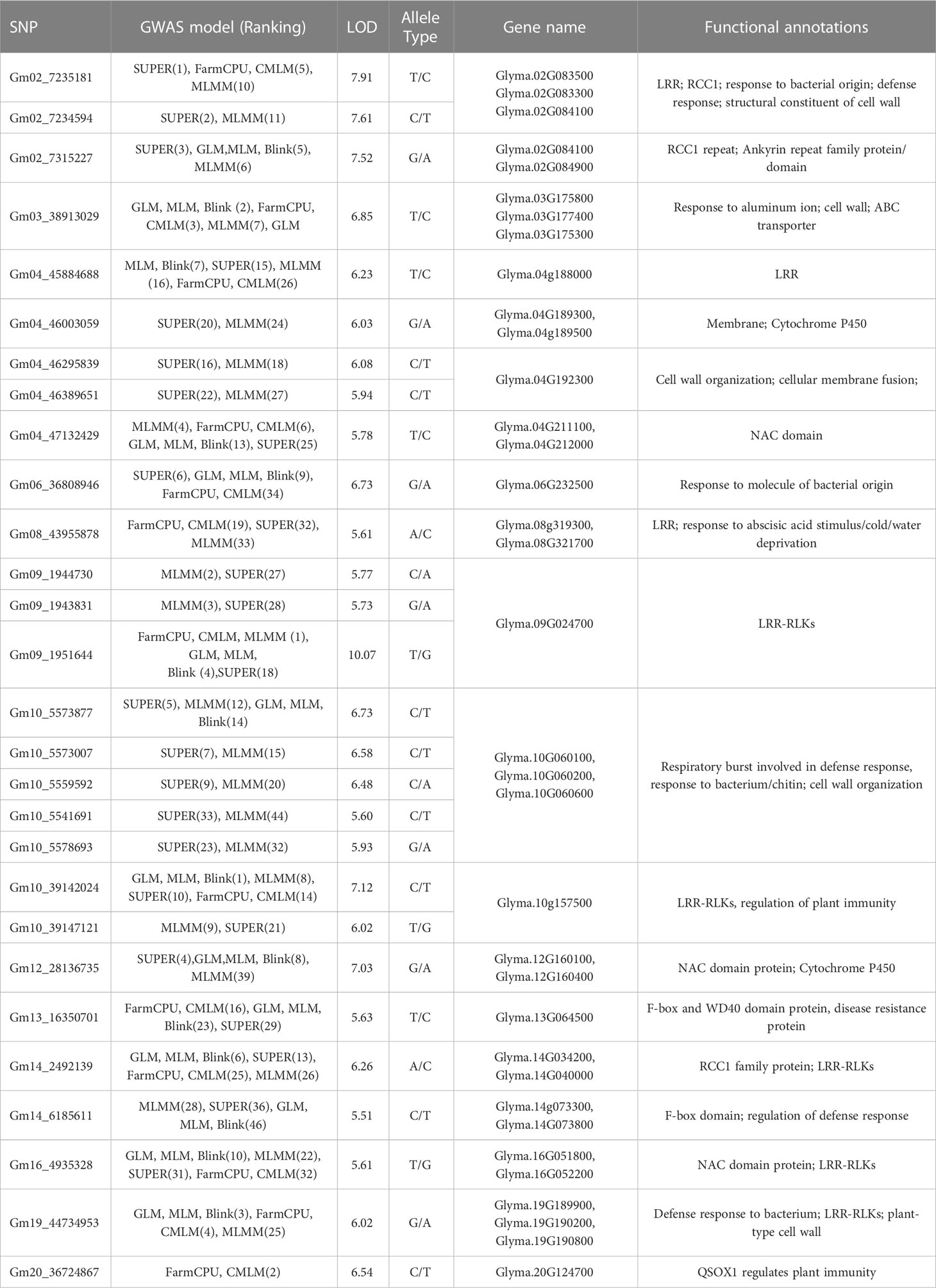
Table 1 The genes within 50 kb genomic region of the top 28 significant SBR-associated SNPs with functional annotations.
Candidate genes of significant SNPs
Due to variations in LD decay across different regions, a conservative distance of 50 kb was set to select candidate genes as the region of the significant SNPs. There are four SNPs (loci) out of the top 100 associated markers, including Gm18_57,223,391, Gm16_29,491,946, Gm06_45,035,185, and Gm18_51,994,200, were identified to locate in close proximity to four main P. pachyrhizi R genes Rpp1, 2, 3, and 4, respectively, which were verified and reported in last decades.
Thirty-five candidate genes that might be associated with SBR disease resistance were found in the regions of the top 28 significant SNP markers (Table 1). Disease related annotations of these candidate genes were included but not limited to: LRR (Leucine Rich Repeat class protein), cytochrome 450, cell wall structure, RCC1 (regulator of chromosome condensation 1), AKR (ankyrin repeat-containing protein), F-box domain, NAC (NAM, ATAF and CUC family). Furthermore, most of the top 28 significant SNP regions were harboring more than one candidate gene, for example, the region of Gm02_7,235,181 and Gm02_7,234,594 contained three candidate genes, Glyma.02G083500, Glyma.02G083300, and Glyma.02G084100, coding for cell wall constituent, LRR-RLK, and RCC1, respectively.
Selection accuracy and selection efficiency
Selection accuracy (SA) and Selection efficiency (SE) reflect the contributions of selected alleles from the top 100 significant SNP to the resistance or susceptibility to Phakopsora. For the resistance alleles, SE varied from 50.0% to 84.2%, with an average of 57.5%; and the SA varied from 50.0% to 82.2%, with an average of 58.2%. SNP Gm09_1,951,644 had the highest values in both SA and SE in resistance effect. For susceptible alleles, the SE varied from 50.0% to 69.8%, with an average of 55.1%; and the SA varied from 50.3% to 56.9%, with an average of 52.7%. SNP Gm04_46,295,839 (52.7%) had the highest values in both SA and SE in susceptible effect (Table S6). This result identified the specific nucleotide of SBR-related alleles.
Genomic prediction
The 100 significant SNPs not only had the highest LOD value but were most repeatable across all GWAS methods as well. Following the same approach, six additional GWAS-based SNP sets were created, each consisting of 28, 100, 500, 1,000, 2,000, and 5,000 SNPs, respectively. In this study, we applied seven datasets, namely, All_SNPs (30,314), GWAS_5000SNPs, GWAS_2000SNPs, GWAS_1000SNPs, GWAS_500SNPs, GWAS_100SNPs and GWAS_28SNPs for GP analysis by five different GS models (Figure 5). The average GS accuracies of the All_SNPs set were at a medium level that was similar to those, ranging from 28.0% (RF) to 32.4% (gBLUP), among all the models.
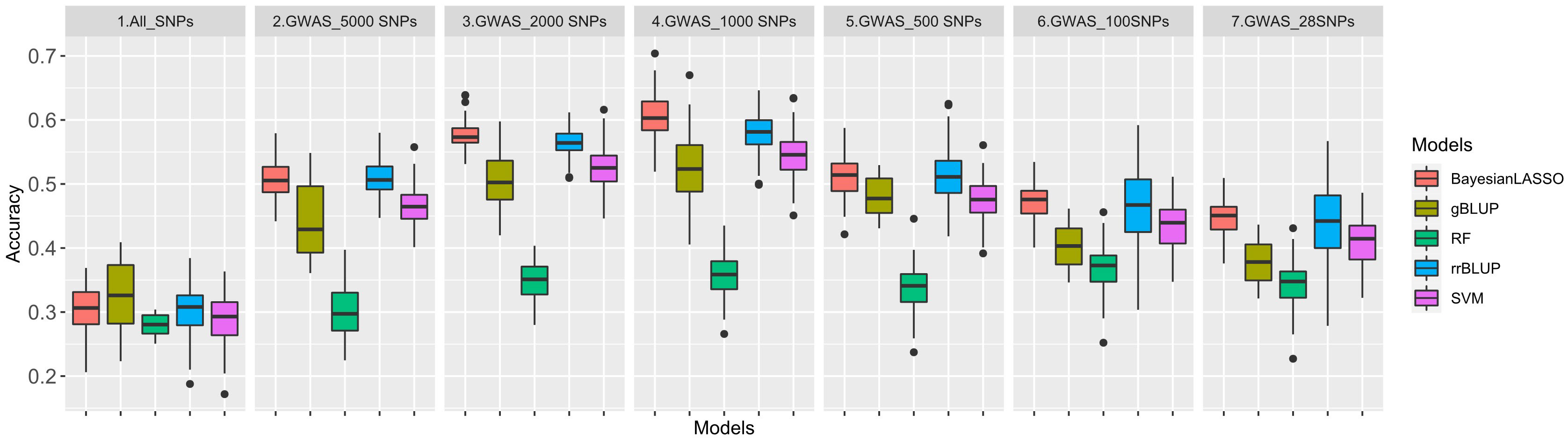
Figure 5 Genomic prediction (GP) accuracy for rust using five GP models: Ridge regression best linear unbiased predictor (rrBLUP) = blue, Genomic best linear unbiased predictor (gBLUP) = dark yellow, Bayesian least absolute shrinkage and selection operator(Bayesian LASSO) = red, Random Forest (RF) = green, Support vector machines (SVM) = purple based on seven datasets: All_SNPs (30314), and six GWAS based SNP sets with top28, 100, 500, 1,000, 2,000 and 5,000 SNPs.
Although the number of SNPs fluctuated by GWAS datasets, all the accuracy curves showed a similar pattern among the five models. The trend depicted by the left side of the curves indicated that as the number of SNPs decreases from 5,000 to 1,000, the accuracy of the prediction increases, too. The highest accuracies were observed when using the 1,000 SNP set, which were varying from 35.7% (RF) to 60.4% (Bayesian LASSO). And, as the number of SNPs continued to decrease from 1,000 to 100, the accuracy of GP also decreased. In all six GWAS based SNP sets, the Bayesian LASSO achieved the highest average GS accuracy of 53.0%, followed by rrBLUP with an average accuracy of 51.9%. On the other hand, the lowest accuracy of 36.2% was recorded when using the RF model. The GS accuracies of gBLUP and SVM models were at almost the same level but were relatively lower using the SVM model (Table S7).
Discussion
Phenotype
Resistance to P. pachyrhizi is commonly evaluated based on three types of SBR lesions: “TAN”, “RB”, and “Mixed”. The “TAN” lesion type is characterized by heavy fungal sporulation typically develop on susceptible soybean leaves, while the RB or “reddish-brown” lesion type has been linked to resistance in known single gene resistance. The “Mixed” reaction is recorded when both RB and TAN lesions were observed on the same leaf (Miles et al., 2006). The simple classification of TAN and RB lesions had been widely used decades ago; however, it had been noted as oversimplified to the symptom observation. Nowadays, the appropriate practice is to separately divide TAN and RB into multiple classes to provide more accurate descriptions of disease symptoms while taking into account variations in fungal sporulation. Considering data consistency and method popularity, we took the TAN lesion as the phenotype of the association analysis for this study, which had sufficient observations and good distribution of SBR resistance. In the present study, the resistance resources were primarily sourced from China, Japan, and Korea, comprising 40%, 16%, and 21% of the total resources, respectively. These figures closely align with the respective proportions of 43%, 13%, and 18% observed in the overall population. In addition, according to the ANOVA between groups, it is obvious that the variability (99%) within groups is greater than the variability (1%) between groups (Table S8).
GWAS and candidate genes
Specific resistance to P. pachyrhizi is controlled by seven single dominant genes, namely, Rpp1 (Chr 18), Rpp2 (Chr16), Rpp3 (Chr6), Rpp4 (Chr7), Rpp5 (Chr3), Rpp6 (Chr18), and Rpp7 (Chr19) (Calvo et al., 2008; Meyer et al., 2009; Lemos et al., 2011; Childs et al., 2018b). The single genes played an important role in SBR resistance, but this kind of resistance is not durable, and the usefulness of the sources loses its effectiveness once it is identified and applied in breeding (Chander et al., 2019). GWAS was performed in efforts to discover loci contributing SBR resistance, thus helping find all genes for SBR control (Chang et al., 2016). Multiple models were developed for GWAS based on linkage disequilibrium, including GLM, MLM, CMLM, MLMM, SUPER, FarmCPU, and BLINK (Wang and Zhang, 2021). Previous studies demonstrated that the differences of the models were caused by the interactions between the methods and other factors, including populations, sample size, mapping resolution, trait complexity, and quality of the data. Typically, all GWAS methods perform well when the aforementioned factors are favorable; however, each model may have varying numbers of false positives depends on the strengths and weaknesses of the model in different circumstance. Therefore, it is important to carefully consider the advantages and limitations of each GWAS method and choose the most appropriate one for the specific study and data. Additionally, multiple methods and independent replication studies are often used to confirm the validity of the results and minimize the risk of false positive findings. However, GWAS studies on SBR resistance were scarce, with the exception of a few studies that used a single model to discover loci contributing to general disease resistance in soybean (Kang et al., 2012; Chang et al., 2016). In this study, we applied all seven models and also considered both significance and consistency of each model for candidate SNPs of SBR resistance to hedge the false positives.
A total of four significant SNPs were located on or nearby the reported R genes. SNP Gm06_45,035,185 in chromosome 6 was located at gene Rpp3; Gm18_51,994,200 and Gm18_57,223,391 in chromosome 18 were nearby the genes Rpp4/Rpp4-b and Rpp1/Rpp1-b, respectively; and Gm16_29,491,946 in chromosome 16 was located at Rpp2, which showed the promise of GWAS on SBR resistance (Sharma and Gupta, 2006). However, we only observed moderate significance for these four SNPs in GWAS analysis, probably due to the following reasons: 1) different genetic variants contributing to the trait, rather than a single major gene; 2) major genes are often rare, the signal from a major gene may be diluted by underrepresented or even missing gene(s) in the samples.
Except for the major Rpps, some significant SNPs also associate with LRR class genes that were considered to be the majority of disease resistance genes in plants (Kang et al., 2012). Genes encoding cytochrome P450 have been shown to contribute to both plant development and defense under pathogen attack (Siminszky et al., 1999). The F-box family proteins have been demonstrated to be directly involved in plant defense against pathogens(Liu and Xue, 2011). The QSOX1 (quiescin sulfhydryl oxidase homolog) were reported to negatively regulate plant immunity against a pathogen (Chae et al., 2021); WD40 repeat-containing proteins which played an important effect on plant defense (Miller et al., 2016). The results were indicative of the robustness of the significant SNPs identified in this study. Other functional annotations pertaining to the candidate genes of cell wall structure/organization/construction and membrane fusion/proteins/structure/transporters have been demonstrated to play some roles in plant passive defense to pathogens (Mellersh and Heath, 2001; Hématy et al., 2009). The RCC1, NAC domain protein, ABC (ATP-binding cassette) transporters, etc. involve in many plant response-associated physiological activities to biotic or abiotic stresses and are widely annotated to the candidate genes (Table 1, S5) (Langenbach et al., 2016; Gautam et al., 2020; Oh et al., 2022). Furthermore, previous studies have reported the involvement of LRR (leucine-rich repeat), ABC transporters and F-box proteins in conferring resistance to rust fungi in other crop species belonging to the same order of Pucciniales, including wheat (Vikas et al., 2022), barley (Sallam et al., 2017), and maize (Juliana et al., 2018).
Selection accuracy and selection efficiency
SE and SA were computed for the significant SNPs associated SBR resistance or susceptibility (Ravelombola et al., 2017). The SA and SE of the marker were measured by relative proportion of an allele type (A/T/C/G) in resistant or/and susceptible accessions, as has been highlighted in other GWAS-related reports (Shi et al., 2016; Ravelombola et al., 2019). Specifically, the proportion of allele type for a completely un-associated SNP should be close to 50% in either resistant or susceptible group. Therefore, when the SA or SE value of the allele type is more than 50%, it contributes positively to the corresponding trait, or vice versa. In general, the two different nucleotides of any significant SNP must have opposite effects on disease resistance or susceptibility, which are defined as “R” or “S” alleles. We observed significant difference between “R” and “S” alleles in one SNP. For example, the “R” allele of SNP Gm04_46,295,839(C/T) has a “C” nucleotide with low SE and SA values (52.6% and 53.9%), but its “S” allele has a “T” nucleotide with high SE and SA values (67.8% and 57.%). This locus may relate to a S gene encoding a cellular membrane fusion protein as annotated in this study. On the contrary, the “A” allele of SNP Gm08_46,674,632(G/A) has high SE (84.2%) and SA (82.2%) values with resistance effect, whereas its “G” allele has low SE (51.5%) and SA (51.4%) values with susceptible effect. This locus is more likely to associate with a R gene coding for a LRR-containing protein in this study. In this study, all significant SNPs have higher than expected SA and SE values (>50%), suggesting that these SNPs can be used for further marker-assisted selection to enhance SBR resistance breeding in soybean.
Genomic prediction
The study discovered 28 significant SNPs located in 20 loci with genes that are associated with plant disease response or resistance. However, before applying these findings in breeding, further verification work is needed (Jannink et al., 2010; Crossa et al., 2017). GS has gained popularity in recent years in large-scale crop breeding programs. Previous studies have shown that GS achieves a more robust prediction of genotypic values compared to QTLs for traits controlled by many genes with small effects. GS tends to have a better and more reliable prediction than the traditional QTL approach, because it uses more markers that are distributed throughout the genome and captures more genetic variation of a trait (Bhat et al., 2016). GS can make predictions about an individual’s performance even before it is phenotyped, which can save time and resources in the breeding process (Zhang et al., 2016; Ravelombola et al., 2019).
However, no research has investigated GS or GP for SBR resistance/tolerance. In this study, we performed GP with seven models on one All_SNP set and six GWAS-based SNP sets. The accuracies of All_SNP set (28.0%~32.4%) were similar to former studies on resistance/tolerance traits to abiotic and biotic stresses of several plant species, including wheat (Poland and Rutkoski, 2016), rice (Xu, 2013), maize (Technow et al., 2013), canola (Jan et al., 2016), alfalfa (Hawkins and Yu, 2018), cassava (Ly et al., 2013), oats (Asoro et al., 2011), miscanthus (Olatoye et al., 2020), grapevine (Brault et al., 2022), and intermediate wheatgrass (Crain et al., 2020). On the other hand, GWAS_SNPs-based GP accuracies were higher than those of All_SNP set-based, demonstrating the importance and contribution of significant SNPs in SBR resistance/tolerance. The accuracy using linear model gBLUP (45.5% in average) was close to those from machine learning (SVM), 47.5% in average, but lower than rrBLUP (51.2% in average) and Bayesian LASSO (52.0% in average) that had been considered to be the optimal approaches (Ravelombola et al., 2019).
Consistently with former reports (Bao et al., 2014; Li et al., 2018), we observed in this study that the accuracy of GP varied by the number of SNPs. For those GWAS-based SNP sets, a greater proportion of SNPs with more significance were retained for GS after further filtering of markers from 5,000 to 1,000, which led to increased accuracy. The accuracies of all models were improved until the number of SNPs reached 1,000, after which the accuracies began to decline until the number of markers dropped to 28. The apex of predictive accuracy was observed at a SNP count of 1,000, likely due to its ability to robustly capture LD and account for relatedness among soybean genotypes. An excess of SNPs beyond this threshold would introduce extraneous information to the models and elevate model complexity, while a SNP count lower than 1,000 would result in the loss of relevant information regarding LD and relatedness capture. Then again, the objective of this GWAS study was primarily to identify the associated loci and candidate genes related to SBR. The use of multiple SNP sets and GS models was employed to ensure the consistency of the GWAS results, rather than to quantitatively evaluate the superiority or variations between the models and data sets. However, the above results can still serve as a reference for future GS research in disease resistance.
Phenotypic selection has been successfully implemented for disease resistance, but without controlled experiments, it is difficult to determine whether the resistance is quantitative or qualitative. Therefore, it is difficult to determine whether the resistance will be durable in the long term. In this study, the SBR-related markers we identified can be used to select for both quantitative and qualitative disease resistance within the breeding lines to bypass the need for controlled experiments through the use of conventional MAS. Additionally, by utilizing GP, the breeders can select for the accumulation of QTL associated with resistance, thereby taking advantage of both quantitative and qualitative resistance genes, even those that have not yet been characterized. This allows them to select the most promising lines for further development and testing without multiple generations of phenotyping.
Data availability statement
The original contributions presented in the study are included in the article/Supplementary Material. Further inquiries can be directed to the corresponding authors.
Author contributions
HX, AS, JW, and YC organized and analysed the original data. HX and Y-BP drafted the manuscript. WL, Y-BP and AS critically revised the manuscript. All authors contributed to the article and approved the submitted version.
Funding
This study was supported by USDA NIFA HATCH project ARK0VG2018 and a USDA-ARS Non-Assistance Cooperative Agreement on Genetic Analysis and Trait-Specific Molecular Marker Development (Accession No. 440501).
Acknowledgments
The authors are thankful to Reid D. Frederick, Glen L. Hartman, and Monte R. Miles, the USDA-ARS, Foreign Disease-Weed Science Research Unit, Urbana, IL, USA for publicly accessible SBR disease scores and phenotyping data from the USDA GRIN website. The authors also thank Zhongqi He and Yulin Jia for review comments. USDA is an equal opportunity provider and employer.
Conflict of interest
The authors declare that the research was conducted in the absence of any commercial or financial relationships that could be construed as a potential conflict of interest.
Publisher’s note
All claims expressed in this article are solely those of the authors and do not necessarily represent those of their affiliated organizations, or those of the publisher, the editors and the reviewers. Any product that may be evaluated in this article, or claim that may be made by its manufacturer, is not guaranteed or endorsed by the publisher.
Supplementary material
The Supplementary Material for this article can be found online at: https://www.frontiersin.org/articles/10.3389/fpls.2023.1179357/full#supplementary-material
Supplementary Figure 1 | The linkage disequilibrium decay rate was estimated as squared correlation coefficient (r2) using all pairs of SNPs located within 4 Mb of physical distance in euchromatic. The red line is the moving average of the (r2) value of the ten adjacent markers.
Supplementary Figure 2 | The Manhattan plots for SBR by multi-GWAS models: (A) Blink, (B) GLM, (C) MLM, (D) CMLM, (E) FarmCPU, (F) SUPER, (G) MLMM. Additionally: (H) QQ-plots of the above seven models.
References
Asoro, F. G., Newell, M. A., Beavis, W. D., Scott, M. P., Jannink, J. L. (2011). Accuracy and training population design for genomic selection on quantitative traits in elite north American oats. Plant Genome 4, 132–144. doi: 10.3835/plantgenome2011.02.0007
Bao, Y., Vuong, T., Meinhardt, C., Tiffin, P., Denny, R., Chen, S., et al. (2014). Potential of association mapping and genomic selection to explore PI 88788 derived soybean cyst nematode resistance. Plant Genome 7, 2840–2854. doi: 10.3835/plantgenome2013.11.0039
Bhat, J. A., Ali, S., Salgotra, R. K., Mir, Z. A., Dutta, S., Jadon, V., et al. (2016). Genomic Selection in the Era of Next Generation Sequencing for Complex Traits in Plant Breeding. Front Genet. 7, 221–2854. doi: 10.3389/fgene.2016.00221
Bradbury, P. J., Zhang, Z., Kroon, D. E., Casstevens, T. M., Ramdoss, Y., Buckler, E. S. (2007). TASSEL: software for association mapping of complex traits in diverse samples. Bioinformatics 23, 2633–2635. doi: 10.1093/bioinformatics/btm308
Brault, C., Segura, V., This, P., Le Cunff, L., Flutre, T., François, P., et al. (2022). Across-population genomic prediction in grapevine opens up promising prospects for breeding. Horticult. Res. 9, uhac041. doi: 10.1093/hr/uhac041
Bromfield, K., Hartwig, E. (1980). Resistance to soybean rust and mode of inheritance. Crop Sci. 20, 254–255. doi: 10.2135/cropsci1980.0011183X002000020026x
Büschges, R., Hollricher, K., Panstruga, R., Simons, G., Wolter, M., Frijters, A., et al. (1997). The barley mlo gene: a novel control element of plant pathogen resistance. Cell 88, 695–705. doi: 10.1016/S0092-8674(00)81912-1
Calvo, É. S., Kiihl, R. A., Garcia, A., Harada, A., Hiromoto, D. M. (2008). Two major recessive soybean genes conferring soybean rust resistance. Crop Sci. 48, 1350–1354. doi: 10.2135/cropsci2007.10.0589
Chae, H. B., Kim, M. G., Kang, C. H., Park, J. H., Lee, E. S., Lee, S.-U., et al. (2021). Redox sensor QSOX1 regulates plant immunity by targeting GSNOR to modulate ROS generation. Mol. Plant 14, 1312–1327. doi: 10.1016/j.molp.2021.05.004
Chander, S., Ortega-Beltran, A., Bandyopadhyay, R., Sheoran, P., Ige, G. O., Vasconcelos, M. W., et al. (2019). Prospects for durable resistance against an old soybean enemy: a four-decade journey from Rpp1 (Resistance to phakopsora pachyrhizi) to Rpp7. Agronomy 9, 348. doi: 10.3390/agronomy9070348
Chang, H.-X., Lipka, A. E., Domier, L. L., Hartman, G. L. (2016). Characterization of disease resistance loci in the USDA soybean germplasm collection using genome-wide association studies. Phytopathology 106, 1139–1151. doi: 10.1094/PHYTO-01-16-0042-FI
Childs, S. P., Buck, J. W., Li, Z. (2018a). Breeding soybeans with resistance to soybean rust (Phakopsora pachyrhizi). Plant Breed. 137, 250–261. doi: 10.1111/pbr.12595
Childs, S. P., King, Z. R., Walker, D. R., Harris, D. K., Pedley, K. F., Buck, J. W., et al. (2018b). Discovery of a seventh rpp soybean rust resistance locus in soybean accession PI 605823. Theor. Appl. Genet. 131, 27–41. doi: 10.1007/s00122-017-2983-4
Crain, J., Bajgain, P., Anderson, J., Zhang, X., Dehaan, L., Poland, J. (2020). Enhancing crop domestication through genomic selection, a case study of intermediate wheatgrass. Front. Plant Sci. 11. doi: 10.3389/fpls.2020.00319
Crossa, J., Pérez-Rodríguez, P., Cuevas, J., Montesinos-López, O., Jarquín, D., De Los Campos, G., et al. (2017). Genomic selection in plant breeding: methods, models, and perspectives. Trends Plant Sci. 22, 961–975. doi: 10.1016/j.tplants.2017.08.011
De Wit, P. J. (1992). Molecular characterization of gene-for-gene systems in plant-fungus interactions and the application of avirulence genes in control of plant pathogens. Annu. Rev. Phytopathol. 30, 391–418. doi: 10.1146/annurev.py.30.090192.002135
Endelman, J. B. (2011). Ridge regression and other kernels for genomic selection with R package rrBLUP. Plant Genome 4, 250-255. doi: 10.3835/plantgenome2011.08.0024
Frichot, E., François, O. (2015). LEA: an R package for landscape and ecological association studies. Methods Ecol. Evol. 6, 925–929. doi: 10.1111/2041-210X.12382
Garcia, A., Calvo, É. S., De Souza Kiihl, R. A., Harada, A., Hiromoto, D. M., Vieira, L. G. E. (2008). Molecular mapping of soybean rust (Phakopsora pachyrhizi) resistance genes: discovery of a novel locus and alleles. Theor. Appl. Genet. 117, 545–553. doi: 10.1007/s00122-008-0798-z
Gautam, A., Pandey, A. K., Dubey, R. S. (2020). Unravelling molecular mechanisms for enhancing arsenic tolerance in plants: a review. Plant Gene 23, 100240. doi: 10.1016/j.plgene.2020.100240
Gebremedhn, H. M., Msiska, U. M., Weldekidan, M. B., Odong, T. L., Rubaihayo, P., Tukamuhabwa, P. (2020). Prediction of candidate genes associated with resistance to soybean rust (Phakopsora pachyrhizi) in line UG-5. Plant Breed. 139, 943–949. doi: 10.1111/pbr.12847
Godoy, C., Meyer, M., Braunschweig: Deutsche Phytomedizinische Gesellschaft (2020). “Overcoming the threat of Asian soybean rust in Brazil,” in Modern fungicides antifungal compounds, IX, ed. Eds. Fraaije, B., Deising, H. B., Mehl, A., Oerke, E. C., Sierotzki, H., Stammler, G. (Braunschweig: Deutsche Phytomedizinische Gesellschaft), 51–56.
Godoy, C. V., Seixas, C. D. S., Soares, R. M., Marcelino-Guimarães, F. C., Meyer, M. C., Costamilan, L. M. (2016). Asian Soybean rust in Brazil: past, present, and future. Pesquisa Agropecuária Bras. 51, 407–421. doi: 10.1590/S0100-204X2016000500002
Hartman, G. L., Miles, M. R., Frederick, R. D. (2005). Breeding for resistance to soybean rust. Plant Dis. 89, 664–666. doi: 10.1094/PD-89-0664
Hartman, G., Wang, T., Tschanz, A. (1991). Soybean rust development and the quantitative relationship between rust severity and soybean yield. Plant Disease 75 (6), 596-600. doi: 10.1094/PD-75-0596
Hawkins, C., Yu, L.-X. (2018). Recent progress in alfalfa (Medicago sativa l.) genomics and genomic selection. Crop J. 6, 565–575. doi: 10.1016/j.cj.2018.01.006
He, J., Zhao, X., Laroche, A., Lu, Z.-X., Liu, H., Li, Z. (2014). Genotyping-by-sequencing (GBS), an ultimate marker-assisted selection (MAS) tool to accelerate plant breeding. Front. Plant Sci. 5. doi: 10.3389/fpls.2014.00484
Hématy, K., Cherk, C., Somerville, S. (2009). Host–pathogen warfare at the plant cell wall. Curr. Opin. Plant Biol. 12, 406–413. doi: 10.1016/j.pbi.2009.06.007
Heslot, N., Yang, H. P., Sorrells, M. E., Jannink, J. L. (2012). Genomic selection in plant breeding: a comparison of models. Crop Sci. 52, 146–160. doi: 10.2135/cropsci2011.06.0297
Isard, S. A., Gage, S. H., Comtois, P., Russo, J. M. (2005). Principles of the atmospheric pathway for invasive species applied to soybean rust. Bioscience 55, 851–861. doi: 10.1641/00063568(2005)055[0851:POTAPF]2.0.CO;2
Jan, H. U., Abbadi, A., Lücke, S., Nichols, R. A., Snowdon, R. J. (2016). Genomic prediction of testcross performance in canola (Brassica napus). PloS One 11, e0147769. doi: 10.1371/journal.pone.0147769
Jannink, J.-L., Lorenz, A. J., Iwata, H. (2010). Genomic selection in plant breeding: from theory to practice. Briefings Funct. Genomics 9, 166–177. doi: 10.1093/bfgp/elq001
Jiang, J., Nguyen, T. (2021). Linear and generalized linear mixed models and their applications (New York, NY: Springer Nature).
Juliana, P., Singh, R. P., Singh, P. K. (2018). Genome-wide association mapping for resistance to leaf rust, stripe rust and tan spot in wheat reveals potential candidate genes. Theor. Appl. Genet. 131, 1405–1422. doi: 10.1007/s00122-018-3086-6
Kang, Y. J., Kim, K. H., Shim, S., Yoon, M. Y., Sun, S., Kim, M. Y., et al. (2012). Genome-wide mapping of NBS-LRR genes and their association with disease resistance in soybean. BMC Plant Biol. 12, 1–13. doi: 10.1186/1471-2229-12-139
Kashiwa, T., Muraki, Y., Yamanaka, N. (2020). Near-isogenic soybean lines carrying Asian soybean rust resistance genes for practical pathogenicity validation. Sci. Rep. 10, 1–7. doi: 10.1038/s41598-020-70188-7
Langenbach, C., Campe, R., Beyer, S. F., Mueller, A. N., Conrath, U. (2016). Fighting Asian soybean rust. Front. Plant Sci. 7. doi: 10.3389/fpls.2016.00797
Lemos, N. G., Braccini, A. D. L. E., Abdelnoor, R. V., De Oliveira, M. C. N., Suenaga, K., Yamanaka, N. (2011). Characterization of genes Rpp2, Rpp4, and Rpp5 for resistance to soybean rust. Euphytica 182, 53–64. doi: 10.1007/s10681-011-0465-3
Levy, C. (2005). Epidemiology and chemical control of soybean rust in southern Africa. Plant Dis. 89, 669–674. doi: 10.1094/PD-89-0669
Li, Y., Ruperao, P., Batley, J., Edwards, D., Khan, T., Colmer, T. D., et al. (2018). Investigating drought tolerance in chickpea using genome-wide association mapping and genomic selection based on whole-genome resequencing data. Front. Plant Sci. 9. doi: 10.3389/fpls.2018.00190
Li, S., Smith, J. R., Ray, J. D., Frederick, R. D. (2012). Identification of a new soybean rust resistance gene in PI 567102B. Theor. Appl. Genet. 125, 133–142. doi: 10.1007/s00122-012-1821-y
Liu, X., Huang, M., Fan, B., Buckler, E. S., Zhang, Z. (2016). Iterative usage of fixed and random effect models for powerful and efficient genome-wide association studies. PloS Genet. 12, e1005767. doi: 10.1371/journal.pgen.1005767
Liu, T.-B., Xue, C. (2011). The ubiquitin-proteasome system and f-box proteins in pathogenic fungi. Mycobiology 39, 243–248. doi: 10.5941/MYCO.2011.39.4.243
Lipka, A. E., Tian, F., Wang, Q., Peiffer, J., Li, M., Bradbury, P. J., et al. (2012). GAPIT: genome association and prediction integrated tool. Bioinformatics 28 (18), 2397–2399. doi: 10.1093/bioinformatics/bts444
Lorenz, A. J., Chao, S., Asoro, F. G., Heffner, E. L., Hayashi, T., Iwata, H., et al. (2011). Genomic selection in plant breeding: knowledge and prospects. Adv. Agron. 110, 77–123. doi: 10.1016/B978-0-12-385531-2.00002-5
Ly, D., Hamblin, M., Rabbi, I., Melaku, G., Bakare, M., Gauch, H. G., Jr., et al. (2013). Relatedness and genotype× environment interaction affect prediction accuracies in genomic selection: a study in cassava. Crop Sci. 53, 1312–1325. doi: 10.2135/cropsci2012.11.0653
Mellersh, D. G., Heath, M. C. (2001). Plasma membrane–cell wall adhesion is required for expression of plant defense responses during fungal penetration. Plant Cell 13, 413–424. doi: 10.1105/tpc.13.2.413
Meyer, J. D., Silva, D. C., Yang, C., Pedley, K. F., Zhang, C., Van De Mortel, M., et al. (2009). Identification and analyses of candidate genes for Rpp4-mediated resistance to Asian soybean rust in soybean. Plant Physiol. 150, 295–307. doi: 10.1104/pp.108.134551
Miles, M. R., Frederick, R. D., Hartman, G. L. (2003). “Soybean rust: is the US soybean crop at risk,” in APS Net Plant Pathology Online. Available at: https://www.ars.usda.gov/research/publications/publication/?seqNo115=150029.
Miles, M., Frederick, R., Hartman, G. (2006). Evaluation of soybean germplasm for resistance to phakopsora pachyrhizi. Plant Health Prog. 7, 33. doi: 10.1094/PHP-2006-0104-01-RS
Miller, J. C., Chezem, W. R., Clay, N. K. (2016). Ternary WD40 repeat-containing protein complexes: evolution, composition and roles in plant immunity. Front. Plant Sci. 6. doi: 10.3389/fpls.2015.01108
Ogutu, J. O., Piepho, H. P., Schulz-Streeck, T. (2011). A comparison of random forests, boosting and support vector machines for genomic selection. BMC Proc. 5 (Suppl 3), S11. doi: 10.1186/1753-6561-5-S3-S11
Oh, Y., Lee, S., Rioux, R., Singh, P., Jia, M. H., Jia, Y., et al. (2022). Analysis of differentially expressed rice genes reveals the ATP-binding cassette (ABC) transporters as a candidate gene against the sheath blight pathogen, rhizoctonia solani. Phytofrontiers 2, 105–115. doi: 10.1094/PHYTOFR-05-21-0035-R
Olatoye, M. O., Clark, L. V., Labonte, N. R., Dong, H., Dwiyanti, M. S., Anzoua, K. G., et al. (2020). Training population optimization for genomic selection in miscanthus. G3: Genes Genomes Genet. 10, 2465–2476. doi: 10.1534/g3.120.401402
Pandey, A. K., Yang, C., Zhang, C., Graham, M. A., Horstman, H. D., Lee, Y., et al. (2011). Functional analysis of the Asian soybean rust resistance pathway mediated by Rpp2. Mol. Plant-Microbe Interact. 24, 194–206. doi: 10.1094/MPMI-08-10-0187
Pivonia, S., Yang, X. (2004). Assessment of the potential year-round establishment of soybean rust throughout the world. Plant Dis. 88, 523–529. doi: 10.1094/PDIS.2004.88.5.523
Poland, J. A., Endelman, J., Dawson, J., Rutkoski, J., Wu, S., Manes, Y., et al. (2012). Genomic selection in wheat breeding using genotyping-by-sequencing. Plant Genome 5, 103–113. doi: 10.3835/plantgenome2012.06.0006
Poland, J., Rutkoski, J. (2016). Advances and challenges in genomic selection for disease resistance. Annu. Rev. Phytopathol. 54, 79–98. doi: 10.1146/annurev-phyto-080615-100056
Purcell, S., Neale, B., Todd-Brown, K., Thomas, L., Ferreira, M. A., Bender, D., et al. (2007). PLINK: a tool set for whole-genome association and population-based linkage analyses. Am. J. Hum. Genet. 81, 559-575. doi: 10.1086/519795
Ravelombola, W., Qin, J., Shi, A., Lu, W., Weng, Y., Xiong, H., et al. (2017). Association mapping revealed SNP markers for adaptation to low phosphorus conditions and rock phosphate response in USDA cowpea (Vigna unguiculata (L.) walp.) germplasm. Euphytica 213, 1–14. doi: 10.1007/s10681-017-1971-8
Ravelombola, W. S., Qin, J., Shi, A., Nice, L., Bao, Y., Lorenz, A., et al. (2019). Genome-wide association study and genomic selection for soybean chlorophyll content associated with soybean cyst nematode tolerance. BMC Genomics 20, 1–18. doi: 10.1186/s12864-019-6275-z
Revell, L. J. (2012). Phytools: an R package for phylogenetic comparative biology (and other things). Methods Ecol. Evol. 3, 217–223. doi: 10.1111/j.2041-210X.2011.00169.x
Sallam, A. H., Tyagi, P., Brown-Guedira, G., Muehlbauer, G. J., Hulse, A., Steffenson, B. J. (2017). Genome-wide association mapping of stem rust resistance in hordeum vulgare subsp. spontaneum. G3 Genes Genomes Genet. 7 (10), 3491–3507. doi: 10.1534/g3.117.300222
Sharma, S., Gupta, G. (2006). Current status of soybean rust (Phakopsora pachyrhizi)-a review. Agric. Rev. 27, 91–102. Available at: https://www.indianjournals.com/ijor.aspx?target=ijor:ar&volume=27&issue=2&article=002.
Shi, A., Buckley, B., Mou, B., Motes, D., Morris, J. B., Ma, J., et al. (2016). Association analysis of cowpea bacterial blight resistance in USDA cowpea germplasm. Euphytica 208, 143–155. doi: 10.1007/s10681-015-1610-1
Shikha, M., Kanika, A., Rao, A. R., Mallikarjuna, M. G., Gupta, H. S., Nepolean, T. (2017). Genomic selection for drought tolerance using genome-wide SNPs in maize. Front. Plant Sci. 8. doi: 10.3389/fpls.2017.00550
Siminszky, B., Corbin, F. T., Ward, E. R., Fleischmann, T. J., Dewey, R. E. (1999). Expression of a soybean cytochrome P450 monooxygenase cDNA in yeast and tobacco enhances the metabolism of phenylurea herbicides. Proc. Natl. Acad. Sci. 96, 1750–1755. doi: 10.1073/pnas.96.4.1750
Song, Q., Hyten, D. L., Jia, G., Quigley, C. V., Fickus, E. W., Nelson, R. L., et al. (2013). Development and evaluation of SoySNP50K, a high-density genotyping array for soybean. PloS One 8 (1), e54985. doi: 10.1371/journal.pone.0054985
Technow, F., Bürger, A., Melchinger, A. E. (2013). Genomic prediction of northern corn leaf blight resistance in maize with combined or separated training sets for heterotic groups. G3: Genes Genomes Genet. 3, 197–203. doi: 10.1534/g3.112.004630
Uppalapati, S. R., Ishiga, Y., Doraiswamy, V., Bedair, M., Mittal, S., Chen, J., et al. (2012). Loss of abaxial leaf epicuticular wax in medicago truncatula irg1/palm1 mutants results in reduced spore differentiation of anthracnose and nonhost rust pathogens. Plant Cell 24, 353–370. doi: 10.1105/tpc.111.093104
Vikas, V. K., Pradhan, A. K., Budhlakoti, N. (2022). Multi-locus genome-wide association studies (ML-GWAS) reveal novel genomic regions associated with seedling and adult plant stage leaf rust resistance in bread wheat (Triticum aestivum l.). Heredity 128, 434–449. doi: 10.1038/s41437-022-00525-1
Visscher, P. M., Brown, M. A., Mccarthy, M. I., Yang, J. (2012). Five years of GWAS discovery. Am. J. Hum. Genet. 90, 7–24. doi: 10.1016/j.ajhg.2011.11.029
Waheed, S., Anwar, M., Saleem, M. A., Wu, J., Tayyab, M., Hu, Z. (2021). The critical role of small RNAs in regulating plant innate immunity. Biomolecules 11, 184. doi: 10.3390/biom11020184
Walker, D., Boerma, H., Phillips, D., Schneider, R., Buckley, J., Shipe, E., et al. (2011). Evaluation of USDA soybean germplasm accessions for resistance to soybean rust in the southern united states. Crop Sci. 51, 678–693. doi: 10.2135/cropsci2010.06.0340
Wang, Q., Tian, F., Pan, Y., Buckler, E. S., Zhang, Z. (2014). A SUPER powerful method for genome wide association study. PloS One 9, e107684. doi: 10.1371/journal.pone.0107684
Wang, J., Zhang, Z. (2021). GAPIT version 3: boosting power and accuracy for genomic association and prediction. Genomics Proteomics Bioinf 19, 629-640. doi: 10.1016/j.gpb.2021.08.005
Wen, Y.-J., Zhang, H., Ni, Y.-L., Huang, B., Zhang, J., Feng, J.-Y., et al. (2018). Methodological implementation of mixed linear models in multi-locus genome-wide association studies. Briefings Bioinf. 19, 700–712. doi: 10.1093/bib/bbw145
Xu, S. (2013). Genetic mapping and genomic selection using recombination breakpoint data. Genetics 195, 1103–1115. doi: 10.1534/genetics.113.155309
Yorinori, J., Paiva, W., Frederick, R., Costamilan, L., Bertagnolli, P., Hartman, G., et al. (2005). Epidemics of soybean rust (Phakopsora pachyrhizi) in Brazil and Paraguay from 2001 to 2003. Plant Dis. 89, 675–677. doi: 10.1094/PD-89-0675
Zhang, J., Song, Q., Cregan, P. B., Jiang, G.-L. (2016). Genome-wide association study, genomic prediction and marker-assisted selection for seed weight in soybean (Glycinemax). Theor. Appl. Genet. 129, 117–130. doi: 10.1007/s00122-015-2614-x
Keywords: GWAS, soybean, disease resistance, genomic prediction, Phakopsora pachyrhizi
Citation: Xiong H, Chen Y, Pan Y-B, Wang J, Lu W and Shi A (2023) A genome-wide association study and genomic prediction for Phakopsora pachyrhizi resistance in soybean. Front. Plant Sci. 14:1179357. doi: 10.3389/fpls.2023.1179357
Received: 04 March 2023; Accepted: 25 April 2023;
Published: 29 May 2023.
Edited by:
Ting Peng, Henan Agricultural University, ChinaReviewed by:
Hengyou Zhang, Chinese Academy of Sciences (CAS), ChinaSang He, Chinese Academy of Agricultural Sciences, China
Copyright © 2023 Xiong, Chen, Pan, Wang, Lu and Shi. This is an open-access article distributed under the terms of the Creative Commons Attribution License (CC BY). The use, distribution or reproduction in other forums is permitted, provided the original author(s) and the copyright owner(s) are credited and that the original publication in this journal is cited, in accordance with accepted academic practice. No use, distribution or reproduction is permitted which does not comply with these terms.
*Correspondence: Haizheng Xiong, aHh4MDA3QHVhcmsuZWR1; Jinshe Wang, d2pzMzMzMTRAMTI2LmNvbQ==; Ainong Shi, YXNoaUB1YXJrLmVkdQ==