- 1Misión Biológica de Galicia (CSIC), Pontevedra, Spain
- 2Agrobiología Ambiental, Calidad de Suelos y Plantas (UVIGO), Unidad Asociada a la MBG (CSIC), Pontevedra, Spain
Fusarium verticillioides poses a threat to worldwide maize production due to its ability to infect maize kernel and synthesize fumonisins that can be accumulated above safety levels for humans and animals. Maize breeding has been proposed as key tool to decrease kernel contamination with fumonisins, but metabolic studies complementary to genomic approaches are necessary to disclose the complexity of maize resistance. An untargeted metabolomic study was proposed using inbreds genetically related but with contrasting levels of resistance in order to uncover pathways implicated in resistance to Fusarium ear rot (FER) and fumonisin contamination in the maize kernel and to look for possible biomarkers. Metabolite determinations were performed in kernels collected at 3 and 10 days after inoculation with F. verticillioides (dat). Discriminant metabolites between resistant and susceptible RILs were rather found at 10 than 3 dat, although metabolite differences at later stages of colonization could be driven by subtle variations at earlier stages of infection. Within this context, differences for membrane lipid homeostasis, methionine metabolism, and indolacetic acid conjugation seemed highly relevant to distinguish between resistant and susceptible inbreds, confirming the polygenic nature of resistance to FER and fumonisin contamination in the maize kernels. Nevertheless, some specific metabolites such as the polyamine spermidine and/or the alkaloid isoquinoline seemed to be promising indirect selection traits to improve resistance to FER and reduce fumonisin accumulation. Therefore, in vitro and in vivo experiments will be necessary to validate the inhibitory effects of these compounds on fumonisins biosynthesis.
Introduction
Fusarium verticillioides poses a threat to worldwide maize production because maize kernels infected with this fungus can accumulate fumonisins above safety levels for animal and human health. Fumonisin accumulation is mainly determined by environmental conditions, but there is wide genetic variability in maize for resistance to fumonisin accumulation that can be used to generate maize varieties with reduced fumonisin content (Eller et al, 2008; Santiago et al, 2015). However, breeding gains are hindered by the complex inheritance of kernel resistance to fumonisin accumulation due to the highly polygenic nature of the trait and the large effect of the genotype x environment interaction (Santiago et al, 2020). In such scenario, the predictive power of genetic data can be significantly improved when combined with metabolic measurements, because metabolites are the result of both genetic and environmental factors and, as such, provide great potential to bridge knowledge between genotype and phenotype (Gartner et al, 2009; Schrimpe-Rutledge et al, 2016). In this sense, untargeted metabolomics offers a discovery and hypothesis-generating approach to provide new biomarkers to be used in plant breeding, as well as valuable insights into the pathways used by maize to limit kernel contamination with fumonisins (Gauthier et al, 2015; Ribbenstedt et al, 2018). In addition, these metabolic studies could complement and/or corroborate results previously obtained by using transcriptomic and proteomic approaches (Lanubile et al., 2010; Lanubile et al., 2012; Campos-Bermudez et al., 2013; Yuan et al., 2013; Lanubile et al., 2014; Wang et al., 2016; Lanubile et al., 2017; Cao et al., 2022).
Campos-Bermudez et al. (2013) studied the transcriptomic and metabolomic changes associated to kernel infection by F. verticillioides in mature kernels of two unrelated inbreds with different performance against infection by F. verticillioides. These authors found no significant changes in transcriptional and metabolomic profiles between resistant and susceptible inbreds, and they suggested that a constitutive defense mechanism may confer the resistant inbred an advantage against F. verticillioides infection. Righetti et al. (2019) investigated differences in mature kernel metabolic profiles among three maize commercial hybrids under natural inoculation at open-field conditions and concluded that the maize lipid signature was strongly involved in the maize-F. verticillioides interaction and in the modulation of fumonisin accumulation; maize lipidome signature being genotype dependent. However, as metabolic differences among unrelated genotypes with contrasting values for resistance to fumonisin contamination could be attributed to genes related to resistance but also to many other genes these genotypes differ for, metabolomic studies aimed at identifying relevant biomarkers of genotype resistance should be performed with genotypes genetically related to avoid the possible biased caused by the genetic background. Ciasca et al. (2020) studied the metabolomics changes of two maize recombinant inbred lines with contrasting phenotypes obtained from the same cross, but they sampled germinated kernels instead of intact kernels. In the current study, we propose to compare the metabolomes of recombinant inbred lines (RILs) derived from the same cross but differing for resistance to kernel contamination with fumonisins to eliminate as much as possible the influence of genetic background on metabolic differences. Four different RILs were included in each category, resistant and susceptible, and would be identical in one or more genomic regions implicated in resistance but arbitrary in all unlinked regions (Michelmore et al, 1991). Specifically, this study was focused on maize metabolomics of immature kernels since genotype-driven specific resistance factors could act at the beginning of maize colonization by the fungus and would be no longer detectable at the harvest stage (Righetti et al, 2021). In this sense, authors have reported that fumonisin production can be initiated during late-milk stage (approximately 18 days after pollination), but plant resistance mechanisms appear to became relevant for reducing fumonisin accumulation between 22 and 29 days after pollination (Picot et al, 2011; Maschietto et al, 2015). Therefore, in order to find compounds in developing maize kernels that contribute most to the resistance to fumonisin accumulation, kernels should be harvested at the milk-dough stage (Atanasova-Penichon et al, 2014). Taking all this into account the main objectives of this study were (i) to uncover metabolic pathways implicated in resistance to FER and fumonisin contamination in the maize kernel and (ii) to identify possible biomarkers to be used in future breeding programs.
Materials and methods
Experiment setup and sample collection
Eight RILs of maize were selected from a set of 144 RILs derived from the cross between the European flint inbred line EP42 (susceptible to FER and kernel contamination with fumonisins) and the American dent inbred line A637 (resistant), and previously genotyped and phenotyped for FER and kernel fumonisin content under inoculation with F. verticillioides (Santiago et al, 2013; Samayoa et al, 2014; Cao et al, 2022). The four RILs with the lowest values (resistant) for fumonisin content (10-15 µg/g) and FER (~ 2 in a visual scale from 1 to 7) along with the four RILs with the highest values (susceptible) for fumonisin content (55-75 µg/g) and FER (~ 4) were selected based on previous evaluations (Supplementary Table 1) (Cao et al., 2022). In 2018, 15 seeds from each RIL were sown in a single row of 3.5 m; distance between adjacent rows being 0.8 m. RILs were arranged in four pairs, each pair formed with one resistant and one susceptible RIL. RILs within each pair were planted in adjacent rows in order to minimize the contribution of field heterogeneity to differential metabolite content between resistant and susceptible inbreds. We self-crossed at least six plants per RIL, and 15 days later the main ear of each plant was inoculated, using a kernel inoculation technique (Cao et al., 2014), with a spore suspension of F. verticillioides as previously described (Cao et al, 2022). Ears were individually collected at 3 or 10 days after inoculation treatment (dat) (18 and 25 days after pollination, respectively). Immediately, undamaged immature kernels around the inoculation point were carefully collected into closed cap tubes kept in liquid nitrogen and stored at – 80 °C until lyophilization. Three biological replicates (ears) were obtained for each RIL and sampling date, except for one susceptible RIL at the 3-day sampling date for which no ears were obtained.
Metabolite extraction
Lyophilized kernels were ground in a mortar and 20 mg per sample were extracted twice with 1 ml of 75% methanol in acetate buffer, mixed in a vortex, sonicated (ultrasonic bath 30 Hz for 5 min) and centrifuged (20,000 g for 10 min). The supernatants were combined and filtered through a 0.22 µm PTFE membrane filter to an Eppendorf tube and an aliquot was transferred to a certified vial. Samples were stored at 4° C until analysis. For MS/MS analysis sample replicates pools were prepared in separate vials.
Untargeted liquid chromatography–mass spectrometry
The untargeted metabolomic analysis was conducted by ultra-high-performance liquid chromatography (UHPLC) (Ultimate 3000 LC; Thermo Scientific) coupled to a quadrupole-time-of-flight mass spectrometer (QTOF-MS) equipped with an electrospray ionization source (ESI) (Bruker Compact; Bruker Daltonics). Samples (5 µl injection volume) were separated in an Intensity Solo 2 C18 column (1.7 µm, 2.1× 100 mm; Bruker Daltonics) at 35°C. A binary solvent system consisted of 0.1% of formic acid on water (solvent A) and acetonitrile (solvent B) with a 0.4 ml/min flow rate was used with the following gradient conditions: 0 min, 3% B; 4 min, 3% B; 16 min, 25% B; 25min, 80% B; 30 min, 100% B; 32 min, 100% B; return to initial conditions at 33 min (3% B) and maintain until 36 min.
The MS acquisition was performed in both negative and positive ionization modes for full scan and auto MS/MS, in a mass scan range of m/z 100-1200. Specific conditions used were: gas flow 9 L/min, nebulizer pressure 38 psi, dry gas 9 L/min, and dry temperature 220°C; capillary and end plate offset were set to 4500 and 500 V, respectively. MS/MS analysis was performed by using different collision energy ramps to cover a range from 15 to 50 eV. The instrument was calibrated externally with a solution of 1mM sodium formate/acetate in 2-propanol:water 50:50 with 0.2% formic acid directly infused to the source. The calibration solution was injected at the beginning of each run and all the spectra were calibrated prior to statistical analysis.
Data processing and statistical analyses
The UHPLC-MS and MS/MS raw data were processed using the MetaboScape 4.0 software (Bruker Daltonics) and the algorithm T–Rex 3D for peak detection and alignment in a retention time (Rt) range from 0.5 to 30 min. Data obtained from positive and negative ionization modes were combined and system contaminants were manually removed (Supplemental Tables 2, 3). The web server MetaboAnalyst 5.0 (Chong et al, 2019) was used for further data filtering and statistical analyses. Variables with more than 50% missing values were removed and all missing values were replaced with a low value (1/5 of the minimum positive value of each variable). Then, the interquantile range filter was used to remove uninformative variables with a near-constant values throughout the dataset and a Pareto scaling was applied for adjusting for the disparities in fold differences between the metabolites. Within each sampling date, Orthogonal Projections to Latent Structures Discriminant Analysis (OPLS‐DA) was carried out to investigate and visualize the pattern of metabolite changes between the resistant and susceptible RILs. OPLS-DA disentangle group-predictive and group-unrelated variation in the measured data and provides a model in which the variables with the largest discriminatory power between groups are determined (Bylesjo et al, 2006). The OPLS‐DA model was evaluated through cross‐validation and the R2Y (estimation of the goodness of fit of the model) and Q2 (qualitative measure of the predictive ability of the model) statistics were used for quality assessment. We performed 1000-run permutations to test the possibility of obtaining those values for the goodness of fit and predictability by chance. In addition, the fold change ratio (FC) and p-value of the t-test for each peak between resistant and susceptible inbreds were calculated. For each sampling date, features with a variable importance in projection (VIP) score >1 in the OPLS‐DA model, |Log2FC| > 0.6 and p value < 0.05 were considered as significant and those with FDR < 0.10, VIP score >1, and |Log2FC| > 0.6 as highly significant.
Metabolite annotation
Significant metabolite features were annotated based on the accurate mass, molecular formula and fragmentation spectrum when available. MetaboScape 4.0 software and the bioinformatic tool SIRIUS 4 (version 4.9.12) (Duhrkop et al, 2019) were used for molecular formulas calculations, and the CSi : FingerID tool (Duhrkop et al, 2015) in SIRIUS 4 and the spectral library MS-DIAL (Tsugawa et al, 2020) for molecular structures and MS/MS experimental spectra comparisons in metabolomic databases. Putative annotations were performed using publicly available databases as Pubchem (https://pubchem.ncbi.nlm.nih.gov ), Lipid Maps (https://www.lipidmaps.org), KEGG (https://www.genome.jp), ChEBI, (https://www.ebi.ac.uk/chebi), MoNA (https://mona.fiehnlab.ucdavis.edu), PlantCyc (https://plantcyc.org) (all accesed between February and October 2022), and consulting literature references.
Pathway analyses
For addressing the metabolic pathways and network-level changes in the resistant vs susceptible RILs, we performed a joint analysis with mummichog and gene set enrichment analysis (GSEA) using the Functional Analysis module of MetaboAnalyst (Pang et al, 2021). These computational algorithms predict functional activity from mass spectrometry data without a priori identification of metabolites by leveraging the collective power of metabolic pathways and networks. The mummichog algorithm infers pathway activities using an over-representation analysis method to evaluate pathway-level enrichment based on significant features (with p-values above a p-value cutoff) from a ranked list of MS peaks identified by untargeted metabolomics (Li et al. (2013). The GSEA method (Subramanian et al, 2005) can extract biological meaning from a ranked feature list (based on t scores) without using a significance cutoff. The setup parameters used to perform functional analysis were: the library selected was the KEGG pathway library for Oryza sativa japonica; a mass tolerance of 5 ppm was stablished for putative annotation; and mummichog default cutoffs for p-values were used (0.1 and 0.01 for metabolite data at 3 and 10 dat, respectively).
Finally, we used the Pathview platform (Luo and Brouwer, 2013; Luo et al, 2017) to integrate and visualize previous transcriptomic data (Cao et al, 2022) and the current untargeted metabolomic data. Target pathways for visualization were: phenylpropanoid biosynthesis, and glutathione and glycerophospholipid metabolisms.
Results
738 and 609 features were identified in kernel samples taken three and 10 days, respectively, after inoculation with Fusarium verticillioides (Supplementary Tables 2, 3). In order to identify the metabolomic differences between resistant and susceptible maize RILs, we first performed an OPLS-DA of the metabolomic data. The OPLS-DA model for kernel samples harvested at 3 dat explained 63% of metabolite variability (R2X); 57.3% of variability was structured in three orthogonal components meanwhile only the 5.8% of metabolite variation was predictive and assisted in differentiating resistant from susceptible inbreds (Figure 1 and Supplemental Figure 1). The model had an overall goodness of fit (R2Y) of 0.961 and an overall cross-validation coefficient (Q2) of 0.504; the probabilities of obtaining those values by chance being 0.083 for R2Y and 0.007 for Q2, respectively.
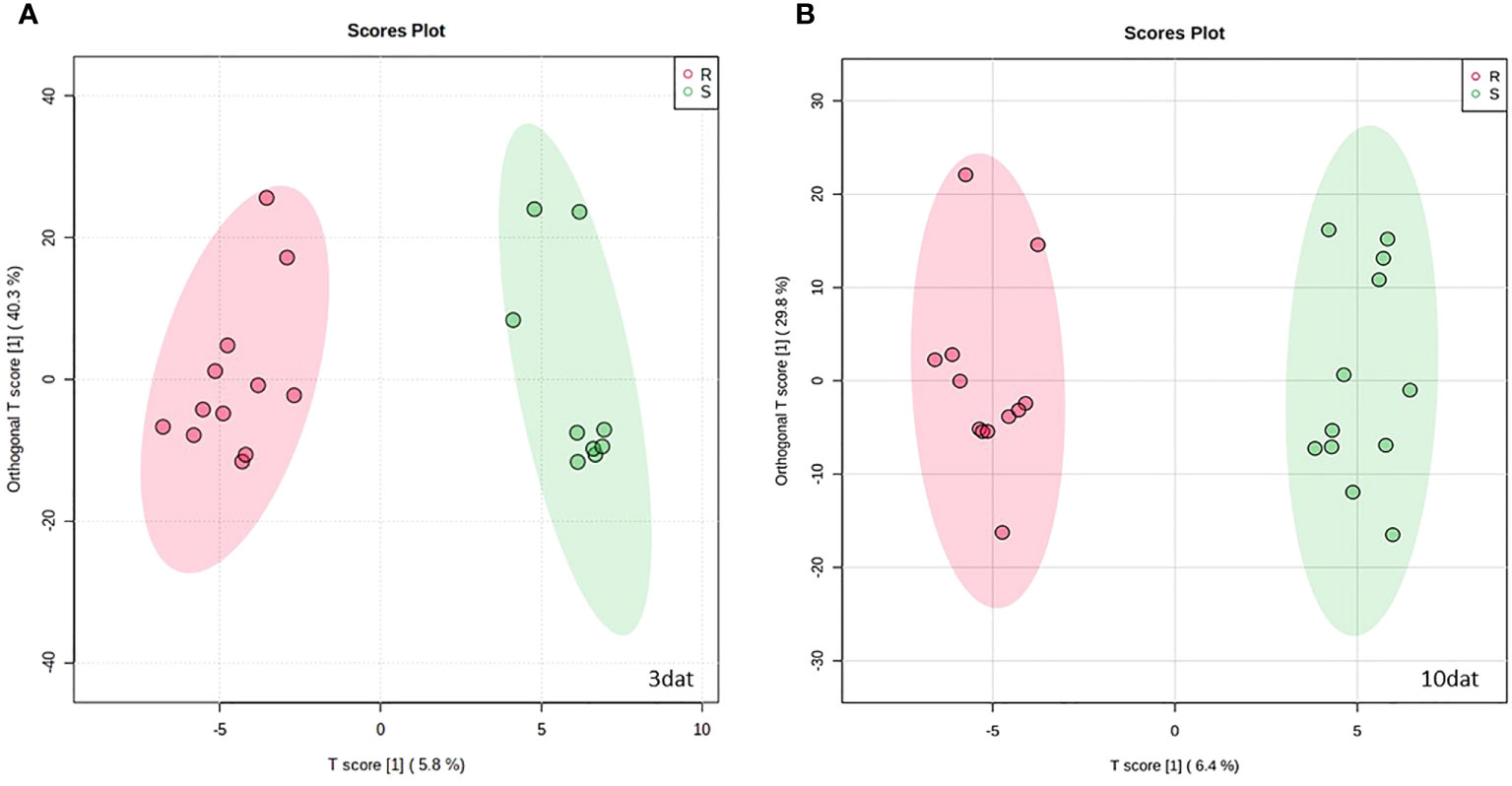
Figure 1 Biplot representation of the scores of resistant (R), in red, and susceptible (S), in green, recombinant inbred lines of maize for predictive and first orthogonal components of supervised least square and orthogonal projections to latent structures discriminant analysis (OPLS-DA) for metabolomic data acquired at (A) 3 and (B) 10 days after inoculation (dat) with F. verticillioides.
The OPLS-DA model for kernel samples collected at 10 dat comprised one predictive and three orthogonal components; predictive metabolite variability being the 6.4% of total metabolite variability (Figure 1 and Supplemental Figure 1). R2Y was 0.975 (p < 0.001) and Q2 0.714 (p < 0.001). The overall metabolite variability explained by the model was 55.5%, most variability being structured but uncorrelated to the differentiation between resistant and susceptible RILs.
Figure 2 shows the metabolite features from kernel samples harvested 3 and 10 dat with FC higher than 1.5 or lower than 0.7 (|Log2FC | > 0.6) and p values less than 0.05 for t-test between resistant and susceptible RILs. Discriminant features with a VIP score > 1 in the OPLS-DA model and that fulfilled the above mentioned FC and t-test criteria were considered as differentially accumulated metabolites in the resistant vs susceptible RILs (Tables 1, 2). However, only 10 metabolites out of them were high-significant differentially (FDR < 0.10, p value < 0.002) accumulated at 10 dat and no metabolite at 3 dat in contrast with most metabolomic studies in which differentially accumulated features between treatments on a single genotype are studied. In the current study, the genetically heterogeneous composition of each bulk could be behind the reduced number of features differentially accumulated between resistant and susceptible RILs. However, detected features are expected to play significant roles in resistance as they would be regulated by genomic regions shared by all resistant or susceptible RILs.
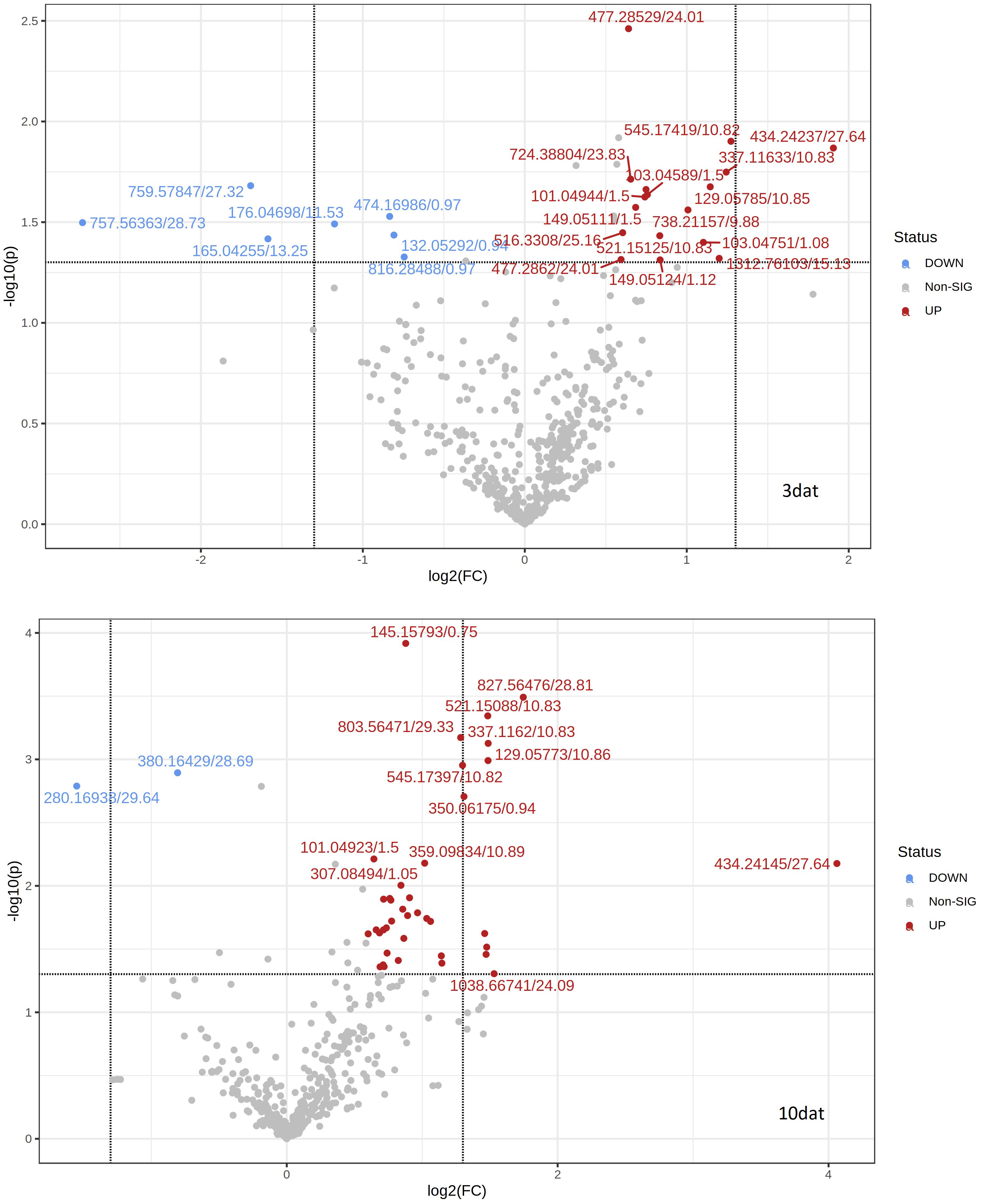
Figure 2 Volcano plot of differentially accumulated features in kernels collected 3 (3dat) and 10 (10dat) days after inoculation with F. verticillioides in resistant and susceptible RILs. Up-regulated (UP), marked in red, and down-regulated (DOWN), marked in blue, features were more and less, respectively, accumulated in resistant versus susceptible RILs. Grey dots corresponded to features not differentially accumulated (Non-SIG). Labels of features were composed of the neutral mass/retention time.
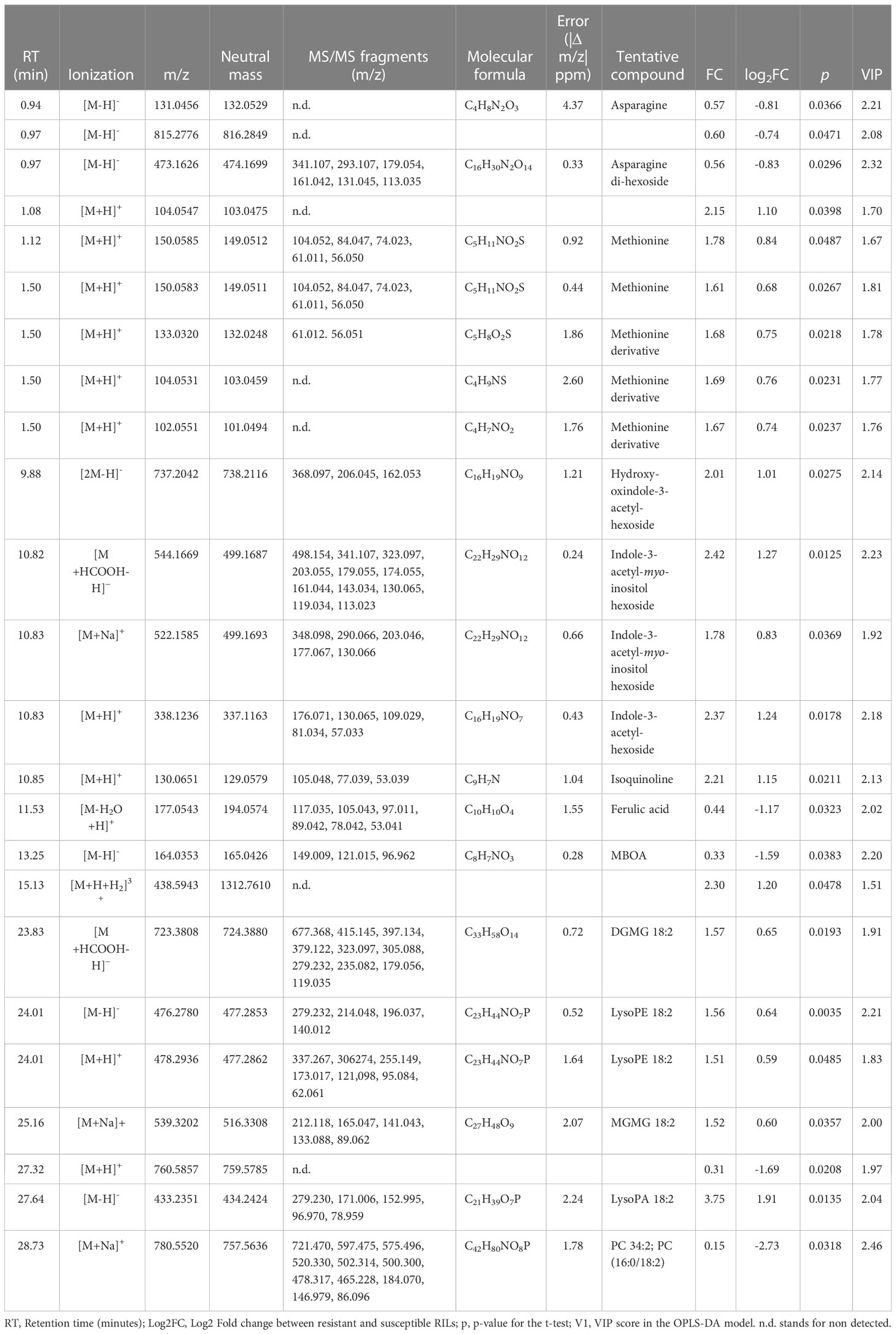
Table 1 LC-MS/MS data, tentative annotation, fold changes, p values and VIP scores for differentially accumulated metabolites between resistant and susceptible maize RILs at 3 days after inoculation with Fusarium verticillioides.
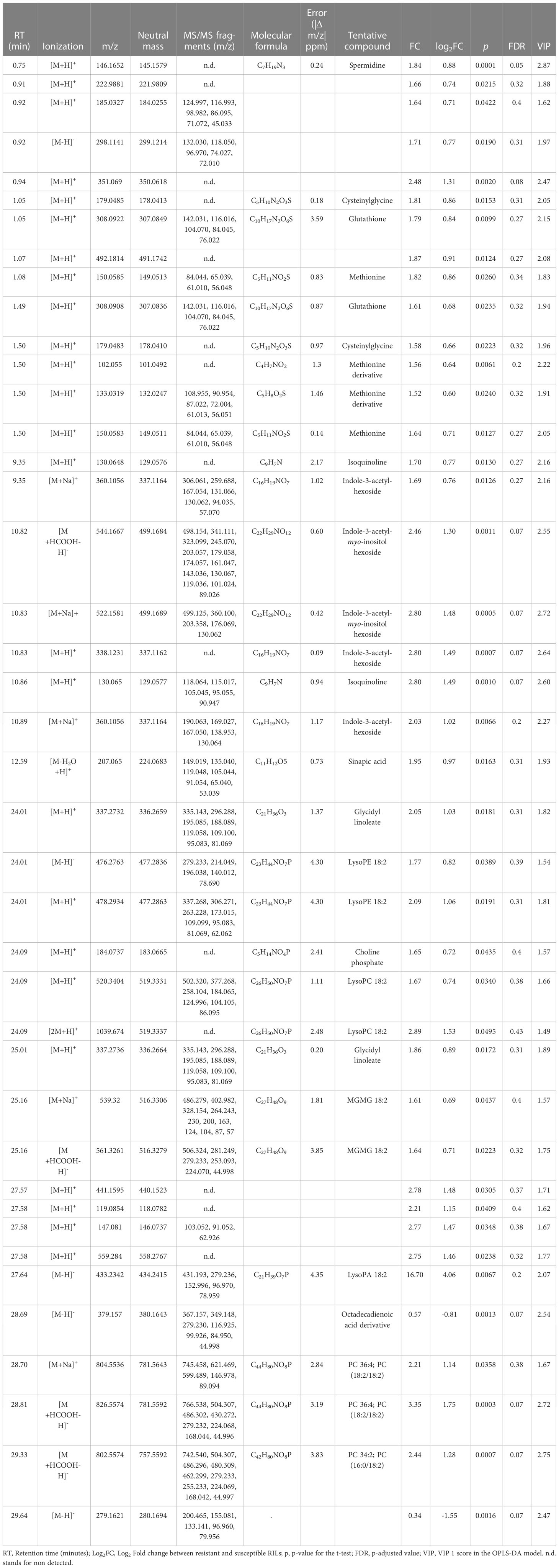
Table 2 LC-MS/MS data, tentative annotation, fold changes, p values and VIP scores for differentially accumulated metabolites between resistant and susceptible maize RILs at 10 days after inoculation with Fusarium verticillioides.
When possible, the differentially accumulated metabolite features in kernels were putatively annotated based on public databases and literature references, and about 75% of them could be related to known compounds (Tables 1, 2). A total of 30% were lipid or lipid-related compounds, 21% were related to methionine and glutathione, 20% were indole-3-acetic acid (IAA) derivative compounds, and only 3% were phenolic compounds. Compounds displayed a deprotonated ion at m/z 337 were annotated as IIA-hexose based on the exact mass and the characteristic fragmentation ions at m/z 176 (IAA) and at m/z 130 (isoquinoline). The fragment at m/z 176 (IAA) was generated by a neutral loss of 162 Da of one hexose that probably correspond to myo-inositol, since the forms of indole-3-acetyl-myo-inositol are the most abundant IAA conjugates in maize kernels (Bandurski, 1979; Ostrowski et al, 2020). Thus, ions [M+Na]+ at m/z 522 and [M+HCOOH-H]- at m/z 544 were assigned as sodium and formic acid adducts of IAA-myo-inositol-hexose (499.1693 Da). Compounds eluted at 1.5 min with [M+H]+ ions at m/z 133, 104 and 102 were assigned as dissociation products of methionine (m/z 150) eluted at the same retention time (Kotiaho et al, 2000). Compound with an [M-H]- ion at m/z 164 in 3 dat samples was annotated as the benzoxazinoid 6-methoxy-2-benzoxazolinone (MBOA) based on its fragmentation pattern at m/z 164, 149, 121 according to Bruijn et al. (2016). Metabolite displayed a [M-H]- ion at m/z 379 at 28.6 min in 10 dat samples was left as unidentified. However, the fragment ion at m/z 279.23 and the detection of a octadecadienoic acid form ([M-H]- at m/z 279.23) at the same retention time, suggested that it could be a fatty acid related compound.
The Functional Analysis results showed several pathways such as the cysteine and methionine metabolism; aminoacyl-tRNA biosynthesis; arginine biosynthesis; alanine, aspartate and glutamate metabolism; and porphyrin and chlorophyll metabolism that were significantly (combined p-values < 0.01) enriched among metabolites differentially accumulated in 3 dat kernel samples of resistant and susceptible RILs (Figure 3). Meanwhile, the pathway enrichment analysis for metabolites in kernel samples collected at 10 dat showed that the pathways glutathione metabolism; arginine and proline metabolism; and cysteine and methionine metabolism were significantly enriched (Figure 3).
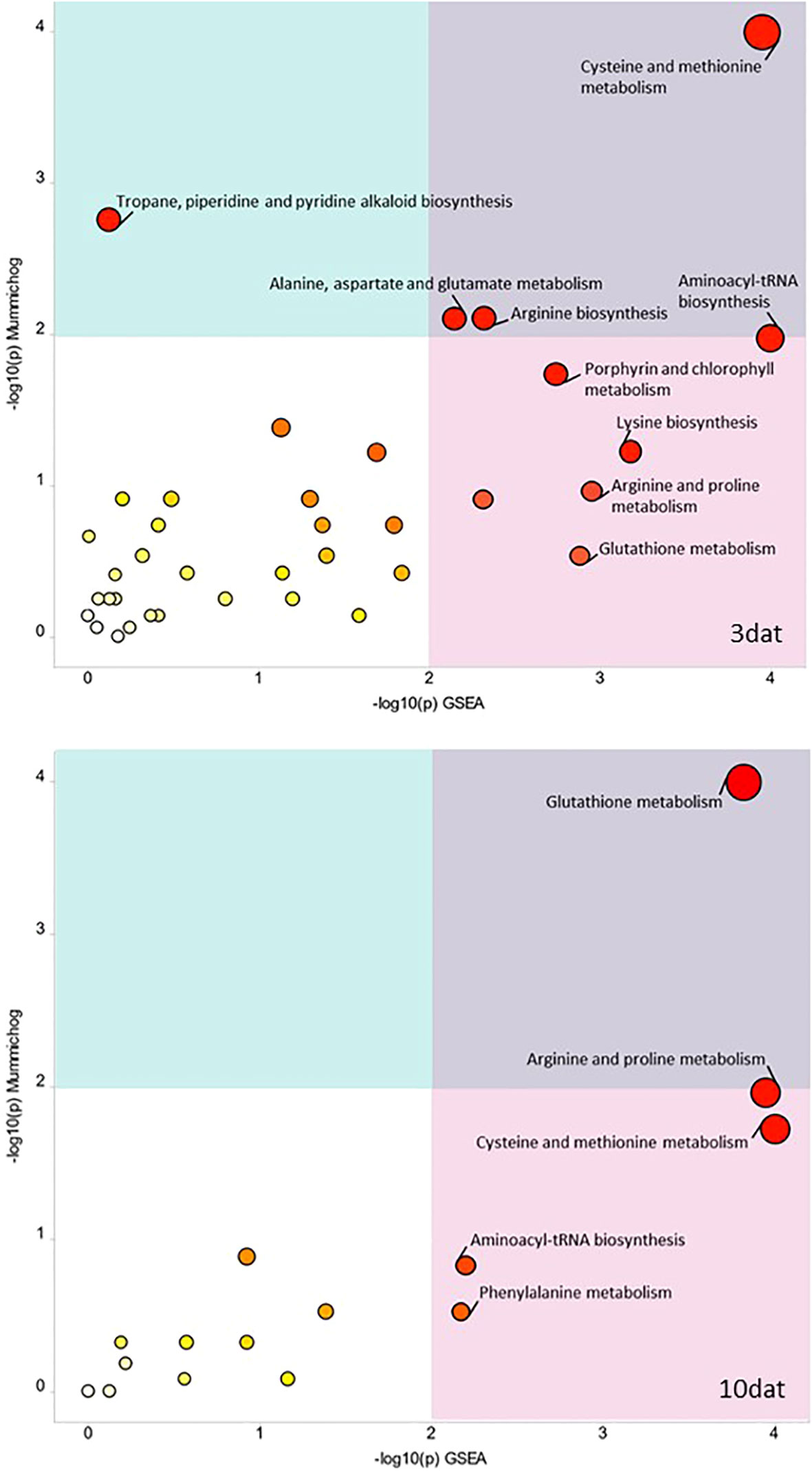
Figure 3 Functional analysis using the Oryza database of metabolomic features detected in maize kernels collected 3 (3dat) and 10 (10dat) days after inoculation (dat) with F. verticillioides in resistant and susceptible RILs. This analysis uses the “mummichog” algorithm to predict pathway activities based on a list of peaks ranked based on t-tests (Pang et al., 2021). Larger and more reddish circles (smaller combined p-value) represent more reliably perturbed pathways.
Visualization of genes and metabolites differentially regulated in resistant compared to susceptible RILs within three different pathways, phenylpropanoid biosynthesis and glutathione and glycerophospholipid metabolisms can be made through Supplemental Figures 2–4, respectively.
Discussion
Higher metabolic differences at later stage of Fusarium infection
The metabolic profiles in maize kernels from RILs with contrasting resistance-susceptibility levels to F. verticillioides infection and fumonisin accumulation were explored at 3 and 10 days after infection with the fungus. Results of the supervised OPLS-DA show that differences between resistant and susceptible RILs were better explained by metabolite abundances at 10 dat than at 3 dat. In addition, high-significant differentially accumulated metabolites were only found at 10 dat. This suggests a larger response occurs at later stages of F. verticillioides infection of maize kernels. These results agree with those obtained by Wang et al. (2016) comparing gene expressions at 1 and 10 dat; these authors suggested that genes involved in biosynthesis of secondary metabolites with antifungal effects would be preferentially induced at the late stage of F. verticillioides colonization. Conversely, functional analyses showed that aminoacyl-tRNA biosynthesis and cysteine, methionine, arginine, proline and glutathione metabolic pathways were enriched among metabolites differentially accumulated in resistant and susceptible RILs in both sampling dates. Therefore, we could hypothesize that some final changes could be driven by subtle differences at earlier stages of Fusarium infection between resistant and susceptible inbreds. That is the case for isoquinoline, a type of alkaloid with a clear fungicidal effect and proven inhibitory effects on the synthesis of fumonisins by Fusarium oxysporum (Tims and Batista, 2007). However, at 10 dat, fewer features differentially accumulated were detected in comparison to differentially transcribed genes (364) between resistant and susceptible RILs that could suggest that a longer period of exposition to the fungus is necessary to reveal all relevant metabolome differences between resistant and susceptible inbreds (Cao et al., 2022). Therefore, metabolome and transcriptome studies would bring complementary results although some pathways detected by both approaches will be emphasized.
Importance of the membrane lipid homeostasis in resistance
Three probable lysophospholipids were differentially accumulated in resistant vs susceptible RILs at 10 dat, but those changes co-occurred with a more significant difference for phosphatidylcholines content between resistant and susceptible RILs. Similarly, an untargeted approach to look for metabolites involved in kernel resistance to fumonisin contamination showed that kernel lipid signature at harvest was strongly involved in the plant−pathogen interaction and in the modulation of fumonisin accumulation; the phosphatidylcholine PC(O-16:0/18:2) being significantly more accumulated in the resistant than in the susceptible hybrid at harvest (Righetti et al, 2019; Righetti et al, 2021). The gene Zm00001d010840, that encodes the enzyme triacylglycerol lipase-like 1, has been proposed as QTL candidate gene for fumonisin content since this gene was significantly upregulated in the resistant RILs compared to the susceptible ones, and was within the confidence interval of one QTL for fumonisin content detected in the RIL population from which the eight RILs used in the current study were selected (Cao et al, 2022). This gene could be involved in degradation of oil bodies in seeds by hydrolysis of ester linkages of triglycerides to diacylglycerol which can be re-directed to the synthesis of lysophospholipids and, specially, phosphatydilcholine in the resistant RILs (Dubots et al, 2012; Wang et al, 2012; Lu et al, 2020; Bates, 2022). The higher accumulation of phosphatidylcholines, key building blocks of membrane bilayers, in resistant RILs could contribute to ROS scavenging as it has been shown that phosphatidylcholine inhibits lipid oxidation synergistically with primary antioxidants, especially tocopherols (Cui and Decker, 2016). In addition, exogenous application of phosphatidylcholine had a positive effect on maintaining cell integrity and cell-membrane structure possibly through keeping cell membrane phospholipid homeostasis (Sun et al, 2022). On the other hand, head-group acylation of mono- and digalactosyldiacylglycerols (MGDC and DGDG, respectively), main lipids in plastid membranes (amyloblasts, seed plastids in which starch is synthesized and stored, membranes contain MGDG and DGDG in high abundance), is a common stress response in plants. In this sense, the galactolipid monogalactosylmonoacylglycerol (18:2) (MGMG) derived from the acylation of MGDG could contribute to the maintenance of galactolipid homeostasis (Myers et al, 2011; Shimojima and Ohta, 2022). Therefore, lipid differences found between resistant and susceptible RILs could be a key factor in order to increase the stability of cell membranes during F. verticillioides infection.
The multifunctional role of methionine metabolism in resistance
The amino acid methionine is the immediate precursor of S-adenosylmethionine (SAM), the major methyl-group donor in transmethylation reactions and intermediate in the biosynthesis of compounds related to plant defense against pathogens such as glutathione, polyamines and the phytohormone ethylene (Ravanel et al, 1998; Nikiforova et al, 2002; Makinen and De, 2019). Methyl groups of choline, phosphatidylcholine, and phosphorylcholine being major end products of transmethylation by SAM (Giovanelli et al, 1985). In kernels of the resistant RILs collected at 10 dat, Cao et al. (2022) already showed that genes involved in ethylene signaling were upregulated compared with the susceptible ones. In the current study, glutathione and spermidine, a polyamine that at a concentration of 1 ng/mL has proven to inhibit in vitro fumonisin production in an 80% with no effect on F. proliferatum growth, were more accumulated in the kernels of resistant RILs. These results suggested that increased levels of methionine at earlier stages of fungal infection could lead to increased accumulation of metabolites involved in detoxification and inhibition of fumonisins (Maschietto et al, 2016; Perincherry et al, 2021). Higher contents of spermidine and/or spermine have been also associated to higher resistance to Aspegillus flavus and aflatoxin contamination (Majumdar et al, 2019). Kovács et al. (2023) showed that, after inoculation with Fusarium verticillioides, seedlings of a tolerant inbred line presented higher levels of spermidine in the radicle than those of a susceptible inbred although differences were not significant. The visual integration of transcriptomic and metabolomic data showed that genes involved in glutathione catabolism were inhibited meanwhile spermidine and reduced glutathione increased in resistant versus susceptible RILs (Supplemental Figure 1). Therefore, we hypothesize that interplay between spermidine and ROS homeostasis could have and important role in controlling fumonisin accumulation in a similar mechanism to that showed by the plant to control Na+ toxicity (Saha et al, 2015; Chen et al, 2017). Chen et al. (2017) showed that spermidine was a key molecule for inducing plant salt tolerance through accumulation of glutathione to reduce damage by reactive oxygen species and regulation of the salt overly sensitive pathway that led to detoxification of Na+. Saha et al. (2015) suggested that polyamines modulate ROS homeostasis by the shift between polyamine anabolism and catabolism resulting in a lower polyamine concentration which, in turn, may favor programmed cell death (Saha et al, 2015). According with this hypothesis, Cao et al. (2022) found that genes involved in programmed cell death were downregulated in the resistant RILs compared to the susceptible ones and the candidate gene proposed (probably encoding an NF-κB inhibitor-like protein) for the most relevant QTL for fumonisin content could participate in preventing polyamide catabolism (Liu et al, 2020).
Modulation of IAA conjugates in relation to resistance
IAA-myo-inositol and its glycosidic forms with galactose and arabinose are the major IAA ester conjugates in maize seed endosperm; IAA ester conjugates comprising the 97-99% of endogenous IAA (Ostrowski et al, 2020). Cao et al. (2022) found that genes involved in auxin signaling repression were upregulated in the resistant compared to susceptible RILs using the same kernel samples, agreeing with the idea that induced auxin biosynthesis or modulated auxin signaling is associated with increased host susceptibility (Brauer et al, 2019). The net level of free IAA in the cell is determined by hormone IAA synthesis, conjugation, degradation and transport, but Fusarium species appear much more likely to manipulate plant auxin homeostasis by hydrolysis of IAA-amino acid and sugar conjugates or perhaps, regulating enzymes participating in synthesis, than by de novo synthesis (Vrabka et al, 2019). Therefore, the higher accumulation of IAA-conjugates, particularly IAA-myo-inositol conjugates (immature maize seeds do not produce amide linkages of IAA), in resistant compared to susceptible RILs could be related to reduced susceptibility by controlling auxin homeostasis (Ostrowski et al, 2020).
Other metabolites likely related to resistance
Therefore, subtle changes observed at 3 dat for isoquinoline and metabolites involved in methionine metabolism, lipid remodeling, IAA signaling are kept at 10 dat and, in some cases, amplified as it is the case for phosphatidylcholines, isoquinoline and IAA-conjugates. However, there were other metabolites that were high-differentially accumulated in kernel collected at 10 dat but they did not appear as differentially accumulated at 3 dat: the polyamine spermidine, already discussed, and an octadecadienoic acid derivative were over and under-accumulated, respectively, in resistant compared to susceptible RILs. Octadecadienoic acid derivatives such as the Lox3 derived oxylipins haves been already associated to increased fumonisin content (Dall’Asta et al, 2012; Battilani et al, 2018; Righetti et al, 2019). Sinapic acid also increased in resistant versus susceptible RILs at 10 dat and deserves to be discussed as it has been found along with two other compounds as the only discriminants between phenolic extracts from different parts of the same mushroom species (Lentinula edodes) with very contrasting effects on fumonisin biosynthesis and no effect on F. verticillioides biomass (Merel et al, 2020).
Finally, ferulic acid and the benzoxacinoid MBOA appeared as discriminant between resistant and susceptible RILs at 3 dat, and no longer, but they deserve special attention because these metabolites have been described as involved in resistance to fungal diseases but in the current study were more accumulated in the susceptible RILs. Benzoxazinoids are abundant indole-derived specialized metabolites in several monocot crop species that function as iron chelators, allelochemicals and in plant defense against herbivorous arthropods and fungal pathogens (Niemeyer, 2009; Poschenrieder et al, 2005). Benzoxazinoid hydroxamic acids are stored as inactive glucosides in the vacuoles, but they are enzymatically converted to the active aglycones by glucosidases upon plant cell disruption and the resulting aglycones are further degraded spontaneously to the corresponding benzoxazolinones, MBOA and its desmethoxy derivative (BOA) (Hashimoto and Shudo, 1996). Therefore, higher accumulation of MBOA could be associated to increased cell damage which promotes H2O2 and, consequently, ferulic acid accumulation, but would be only evident at early stages of infection because F. verticillioides can metabolize and detoxify active benzoxazinoids such as MBOA (Glenn et al, 2001; Jabeen et al, 2007; Li et al, 2020). According to the hypothesis of increased cell damage in susceptible inbreds, in a previous transcriptomic study with the same materials, genes related to cell death were downregulated in the resistant compared with the susceptible RILs (Cao et al, 2022).
Metabolomic differences between resistant and susceptible RILs would confirm some results from the previous transcriptomic study (Cao et al, 2022) such as the down-regulation of auxin signaling, up-regulation of the phenylpropanoid pathway, activation of electron transport chain toward amino acid synthesis and reduced oxidative stress in the resistant RILs compared with the susceptible ones. However, in the current study, new insights on the pathways involved in resistance have been uncovered because the specific increase in methionine metabolism toward polyamine and glutathione over accumulation in resistant versus susceptible RILs has been revealed. Similarly, Cao et al. (2022) suggested that mobilization of lipids from oil bodies to phytoalexin synthesis could have an important role in resistance, but the metabolic study have shown that lipid re-modelling would be rather re-directed toward phosphatidylcholine accumulation.
Conclusions
Discriminant metabolites between resistant and susceptible RILs were rather found at 10 than 3 dat, although differences for key metabolites were kept across kernel sampling times suggesting that longer exposition to fungal colonization is necessary to uncover biomarkers but those changes could be driven by subtle changes at earlier stages of infection. Within this context, differences for membrane lipid homeostasis, methionine metabolism and IAA-conjugation seemed highly relevant in order to distinguish between resistant and susceptible inbreds. In addition, some metabolites such as spermidine and isoquinoline seemed to be promising indirect traits to improve resistance to FER and reduce fumonisin accumulation. However, their role in resistance should be validated using in vitro and in vivo experiments to determine the real effects of these compounds on F. verticilllioides growth and fumonisins biosynthesis.
Data availability statement
Raw metabolic data are deposited in the online repository DIGITAL.CSIC and can be accessed using this link: https://doi.org/10.20350/digitalCSIC/15427.
Author contributions
AB conceived the study. AC took care of field experiments, data recording, and sample collection with the assistance of AB, RS, and RM. AC led extraction and acquisition and filtering of UHPLC-QTOF data with the assistance of NG. AC performed the identification of tentative compounds. AB performed statistical analyses of data. AB and NG drafted the initial manuscript and RS and NG edited the figures. All authors contributed to the article and approved the submitted version.
Funding
This research was funded by subsequent coordinated projects financed by MCIU/AEI/FEDER, UE (RTI2018-096776-B-C21, RTI2018-096776-B-C22, PID2021-122196OB-C21 and PID2021-122196OB-C22). We also used funds of the CSIC project 2021AEP132.
Acknowledgments
NG acknowledges an FPI contract (“Contrato predoctoral para la formación de doctores”) funded by project RTI2018-096776-B-C21 (financed by MCIU/AEI/FEDER, UE). AC acknowledges a PTA contract PTA2018-015873-I financed by MCIU/AEI/FSE+.
Conflict of interest
The authors declare that the research was conducted in the absence of any commercial or financial relationships that could be construed as a potential conflict of interest.
Publisher’s note
All claims expressed in this article are solely those of the authors and do not necessarily represent those of their affiliated organizations, or those of the publisher, the editors and the reviewers. Any product that may be evaluated in this article, or claim that may be made by its manufacturer, is not guaranteed or endorsed by the publisher.
Supplementary material
The Supplementary Material for this article can be found online at: https://www.frontiersin.org/articles/10.3389/fpls.2023.1160092/full#supplementary-material
Supplementary Figure 1 | Validation of supervised least square and orthogonal projections to latent structures discriminant analysis (OPLS-DA) for data acquired at 3 and 10 days after inoculation (dat) with Fusarium verticillioides in resistant and susceptible RILs. (A) Cross-validation for R2X, R2Y, and Q2 coefficients for the OPLS-DA model with one predictive (p1) and three orthogonal (o1, o2, and o3) components for samples collected at 3 dat, (B) Visualization of permutation test to validate the results of OPLS-DA analysis for samples collected at 3 dat, (C) Cross-validation for R2X, R2Y, and Q2 coefficients of the OPLS-DA model with one predictive (p1) and three orthogonal (o1, o2, and o3) components for samples collected at 10 dat, and (D) Visualization of permutation test to validate the results of OPLS-DA analysis for samples collected at 10 dat.
Supplementary Figure 2 | Integrative visualization by Pathview platform of genes (Cao et al, 2022) and metabolites of the phenylpropanoid biosynthesis pathway differentially expressed and accumulated, respectively, in immature maize kernels of resistant and susceptible RILs to FER and fumonisin contamination collected 10 days after inoculation with F. verticillioides.
Supplementary Figure 3 | Integrative visualization by Pathview platform of genes (Cao et al, 2022) and metabolites of the glutathione metabolism pathway differentially expressed and accumulated, respectively, in immature maize kernels of resistant and susceptible RILs to FER and fumonisin contamination collected 10 days after inoculation with Fusarium verticillioides.
Supplementary Figure 4 | Integrative visualization by Pathview platform of genes (Cao et al, 2022) and metabolites of the glycerophospholipid metabolism pathway differentially expressed and accumulated, respectively, in immature maize kernels of resistant and susceptible RILs to FER and fumonisin contamination collected 10 days after inoculation with Fusarium verticillioides.
References
Atanasova-Penichon, V., Bernillon, S., Marchegay, G., Lornac, A., Pinson-Gadais, L., Ponts, N., et al. (2014). Bioguided isolation, characterization, and biotransformation by Fusarium verticillioides of maize kernel compounds that inhibit fumonisin production. Mol. Plant-Microbe Interact. 27 (10), 1148–1158. doi: 10.1094/MPMI-04-14-0100-R
Bandurski, R. S. (1979). Chemistry and physiology of conjugates of indole-3-Acetic acid. Plant Growth Substances Mandava, N.B. (Washington, D.C.: American Chemical Society) 1979, 1–17. doi: 10.1021/bk-1979-0111.ch001
Bates, P. D. (2022). “Chapter six - the plant lipid metabolic network for assembly of diverse triacylglycerol molecular species,” in Advances in botanical research. Eds. Rébeillé, F., Maréchal, E. 101, 225–252. doi: 10.1016/bs.abr.2021.07.003
Battilani, P., Lanubile, A., Scala, V., Reverberi, M., Gregori, R., Falavigna, C., et al. (2018). Oxylipins from both pathogen and host antagonize jasmonic acid-mediated defence via the 9-lipoxygenase pathway in Fusarium verticillioides infection of maize. Mol. Plant Pathol. 19 (9), 2162–2176. doi: 10.1111/mpp.12690
Brauer, E. K., Rocheleau, H., Balcerzak, M., Pan, Y. L., Fauteux, F., Liu, Z. Y., et al. (2019). Transcriptional and hormonal profiling of Fusarium graminearum-infected wheat reveals an association between auxin and susceptibility. Physiol. Mol. Plant Pathol. 107, 33–39. doi: 10.1016/j.pmpp.2019.04.006
Bruijn, W. J. C., Gruppen, H., Vincken, J. P.. (2016). Structure and biosynthesis of benzoxazinoids: Plant defence metabolites with potential as antimicrobial scaffolds. Phytochemistry 155, 233. doi: 10.1016/j.phytochem.2018.07.005
Bylesjo, M., Rantalainen, M., Cloarec, O., Nicholson, J. K., Holmes, E., Trygg, J. (2006). OPLS discriminant analysis: combining the strengths of PLS-DA and SIMCA classification. J. Chemometrics 20 (8-10), 341–351. doi: 10.1002/cem.1006
Campos-Bermudez, V. A., Fauguel, C. M., Tronconi, M. A., Casati, P., Presello, D. A., Andreo, C. S. (2013). Transcriptional and metabolic changes associated to the infection by Fusarium verticillioides in maize inbreds with contrasting ear rot resistance. PloS One 8 (4), 10. doi: 10.1371/journal.pone.0061580
Cao, A., Butrón, A., Ramos, A. J., Marín, S., Souto, C., Santiago, R. (2014). Assessing white maize resistance to fumonisin contamination. Eur. J. Plant Pathol. 138, 283–292. doi: 10.1007/s10658-013-0328-y
Cao, A. A., de la Fuente, M., Gesteiro, N., Santiago, R., Malvar, R. A., Butron, A. (2022). Genomics and pathways involved in maize resistance to fusarium ear rot and kernel contamination with fumonisins. Front. Plant Sci. 13, 866478. doi: 10.3389/fpls.2022.866478
Chen, L., Liu, Y. P., Wu, G. W., Zhang, N., Shen, Q. R., Zhang, R. F. (2017). Beneficial rhizobacterium Bacillus amyloliquefaciens SQR9 induces plant salt tolerance through spermidine production. Mol. Plant-Microbe Interact. 30 (5), 423–432. doi: 10.1094/MPMI-02-17-0027-R
Chong, J., Wishart, D. S., Xia, J. (2019). Using MetaboAnalyst 4.0 for comprehensive and integrative metabolomics data analysis. Curr. Protoc. Bioinf. 68 (1), e86. doi: 10.1093/nar/gky310
Ciasca, B., Lanubile, A., Marocco, A., Pascale, M., Logrieco, A. F., Lattanzio, V. M. T. (2020). Application of an integrated and open source workflow for LC-HRMS plant metabolomics studies. case-control study: metabolic changes of maize in response to Fusarium verticillioides infection. Front. Plant Sci. 11, 15. doi: 10.3389/fpls.2020.00664
Cui, L. Q., Decker, E. A. (2016). Phospholipids in foods: prooxidants or antioxidants? J. Sci. Food Agric. 96 (1), 18–31. doi: 10.1002/jsfa.7320
Dall’Asta, C., Falavigna, C., Galaverna, G., Battilani, P. (2012). Role of maize hybrids and their chemical composition in Fusarium infection and fumonisin production. J. Agric. Food Chem. 60 (14), 3800–3808. doi: 10.1021/jf300250z
Dubots, E., Botte, C., Boudiere, L., Yamaryo-Botte, Y., Jouhet, J., Marechal, E., et al. (2012). Role of phosphatidic acid in plant galactolipid synthesis. Biochimie 94 (1), 86–93. doi: 10.1016/j.biochi.2011.03.012
Duhrkop, K., Fleischauer, M., Ludwig, M., Aksenov, A. A., Melnik, A. V., Meusel, M., et al. (2019). SIRIUS 4: a rapid tool for turning tandem mass spectra into metabolite structure information. Nat. Methods 16 (4), 299. doi: 10.1038/s41592-019-0344-8
Duhrkop, K., Shen, H. B., Meusel, M., Rousu, J., Bocker, S. (2015). Searching molecular structure databases with tandem mass spectra using CSI : FingerID. Proc. Natl. Acad. Sci. U. S. A. 112 (41), 12580–12585. doi: 10.1073/pnas.1509788112
Eller, M. S., Holland, J. B., Payne, G. A. (2008). Breeding for improved resistance to fumonisin contamination in maize. Toxin Rev. 27 (3-4), 371–389. doi: 10.1080/15569540802450326
Gartner, T., Steinfath, M., Andorf, S., Lisec, J., Meyer, R. C., Altmann, T., et al. (2009). Improved heterosis prediction by combining information on DNA- and metabolic markers. PloS One 4 (4), e5220. doi: 10.1371/journal.pone.0005220
Gauthier, L., Atanasova-Penichon, V., Chereau, S., Richard-Forget, F. (2015). Metabolomics to decipher the chemical defense of cereals against Fusarium graminearum and deoxynivalenol accumulation. Int. J. Mol. Sci. 16 (10), 24839–24872. doi: 10.3390/ijms161024839
Giovanelli, J., Mudd, S. H., Datko, A. H. (1985). Quantitative-analysis of pathways of methionine metabolism and their regulation in Lemna. Plant Physiol. 78 (3), 555–560. doi: 10.1104/pp.78.3.555
Glenn, A. E., Hinton, D. M., Yates, I. E., Bacon, C. W. (2001). Detoxification of corn antimicrobial compounds as the basis for isolating Fusarium verticillioides and some other Fusarium species from corn. Appl. Environ. Microbiol. 67 (7), 2973–2981. doi: 10.1128/AEM.67.7.2973-2981.2001
Hashimoto, Y., Shudo, K. (1996). Chemistry of biologically active benzoxazinoids. Phytochemistry 43 (3), 551–559. doi: 10.1016/0031-9422(96)00330-5
Jabeen, R., Yamada, K., Hasegawa, T., Minami, E., Shigemori, H., Hasegawa, K. (2007). Direct involvement of benzoxazinoids in the growth suppression induced by phototropic stimulation in maize coleoptiles. Heterocycles 71 (3), 523–529. doi: 10.3987/COM-06-10940
Kotiaho, T., Eberlin, M. N., Vainiotalo, P., Kostiainen, R. (2000). Electrospray mass and tandem mass spectrometry identification of ozone oxidation products of amino acids and small peptides. J. Am. Soc. Mass Spectrometry 11 (6), 526–535. doi: 10.1016/S1044-0305(00)00116-1
Kovács, B., Kovács, A., Pál, M., Spitkó, T., Marton, C. L., Szőke, C. (2023). Changes in polyamine contents during Fusarium graminearum and Fusarium verticillioides inoculation in maize seedlings with or without seed-priming. Biol. Futura. doi: 10.1007/s42977-023-00162-7
Lanubile, A., Bernardi, J., Battilani, P., Logrieco, A., Marocco, A. (2012). Resistant and susceptible maize genotypes activate different transcriptional responses against Fusarium verticillioides. Physiol. Mol. Plant Pathol. 77, 52–59. doi: 10.1016/j.pmpp.2011.12.002
Lanubile, A., Ferrarini, A., Maschietto, V., Delledonne, M., Marocco, A., Bellin, D. (2014). Functional genomic analysis of constitutive and inducible defense responses to Fusarium verticillioides infection in maize genotypes with contrasting ear rot resistance. BMC Genomics 15, 710. doi: 10.1186/1471-2164-15-710
Lanubile, A., Maschietto, V., Borrelli, V. M., Stagnati, L., Logrieco, A. F., Marocco, A. (2017). Molecular basis of resistance to fusarium ear rot in maize. Front. Plant Sci. 8, 1774. doi: 10.3389/fpls.2017.01774
Lanubile, A., Pasini, L., Marocco, A. (2010). Differential gene expression in kernels and silks of maize lines with contrasting levels of ear rot resistance after Fusarium verticillioides infection. J. Plant Physiol. 167, 1398–1406. doi: 10.1016/j.jplph.2010.05.015
Li, S. Z., Park, Y., Duraisingham, S., Strobel, F. H., Khan, N., Soltow, Q. A., et al. (2013). Predicting network activity from high throughput metabolomics. PloS Comput. Biol. 9 (7), e1003123. doi: 10.1371/journal.pcbi.1003123
Li, Q. Y., Xu, K. G., Wang, S. Y., Li, M. Y., Jiang, Y. M., Liang, X. L., et al. (2020). Enzymatic browning in wheat kernels produces symptom of black point caused by Bipolaris sorokiniana. Front. Microbiol. 11, 526266. doi: 10.3389/fmicb.2020.526266
Liu, J. H., Wang, T. W., Lin, Y. Y., Ho, W. C., Tsai, H. C., Chen, S. P., et al. (2020). Acrolein is involved in ischemic stroke-induced neurotoxicity through spermidine/spermine-N1-acetyltransferase activation. Exp. Neurol. 323, 113066. doi: 10.1016/j.expneurol.2019.113066
Lu, J. H., Xu, Y., Wang, J. L., Singer, S. D., Chen, G. Q. (2020). The role of triacylglycerol in plant stress response. Plants-Basel 9 (4), 472. doi: 10.3390/plants9040472
Luo, W. J., Brouwer, C. (2013). Pathview: an R/Bioconductor package for pathway-based data integration and visualization. Bioinformatics 29 (14), 1830–1831. doi: 10.1093/bioinformatics/btt285
Luo, W. J., Pant, G., Bhavnasi, Y. K., Blanchard, S. G., Brouwer, C. (2017). Pathview web: user friendly pathway visualization and data integration. Nucleic Acids Res. 45 (W1), W501–W508. doi: 10.1093/nar/gkx372
Majumdar, R., Minocha, R., Lebar, M. D., Rajasekaran, K., Long, S., Carter-Wientjes, C., et al. (2019). Contribution of maize polyamine and amino acid metabolism toward resistance against Aspergillus flavus infection and aflatoxin production. Front. Plant Sci. 10, 692. doi: 10.3389/fpls.2019.00692
Makinen, K., De, S. (2019). The significance of methionine cycle enzymes in plant virus infections. Curr. Opin. Plant Biol. 50, 67–75. doi: 10.1016/j.pbi.2019.03.002
Maschietto, V., Lanubile, A., De Leonardis, S., Marocco, A., Paciolla, C. (2016). Constitutive expression of pathogenesis-related proteins and antioxydant enzyme activities triggers maize resistance towards Fusarium verticillioides. J. Plant Physiol. 200, 53–61. doi: 10.1016/j.jplph.2016.06.006
Maschietto, V., Marocco, A., Malachova, A., Lanubile, A. (2015). Resistance to Fusarium verticillioides and fumonisin accumulation in maize inbred lines involves an earlier and enhanced expression of lipoxygenase (LOX) genes. J. Plant Physiol. 188, 9–18. doi: 10.1016/j.jplph.2015.09.003
Merel, D., Savoie, J. M., Mata, G., Salmones, D., Ortega, C., Atanasova, V., et al. (2020). Methanolic extracts from cultivated mushrooms affect the production of fumonisins b and fusaric acid by Fusarium verticillioides. Toxins 12 (6), 366. doi: 10.3390/toxins12060366
Michelmore, R. W., Paran, I., Kesseli, R. V. (1991). Identification of markers linked to disease-resistance genes by bulked segregant analysis - a rapid method to detect markers in specific genomic regions by using segregating populations. Proc. Natl. Acad. Sci. United States America 88 (21), 9828–9832. doi: 10.1073/pnas.88.21.9828
Myers, A. M., James, M. G., Lin, Q. H., Yi, G., Stinard, P. S., Hennen-Bierwagen, T. A., et al. (2011). Maize opaque5 encodes monogalactosyldiacylglycerol synthase and specifically affects galactolipids necessary for amyloplast and chloroplast function. Plant Cell 23 (6), 2331–2347. doi: 10.1105/tpc.111.087205
Niemeyer, H. M. (2009). Hydroxamic acids derived from 2-hydroxy-2h-1,4-benzoxazin-3(4h)-one: key defense chemicals of cereals. J. Agric. Food Chem. 57 (5), 1677–1696. doi: 10.1021/jf8034034
Nikiforova, V., Kempa, S., Zeh, M., Maimann, S., Kreft, O., Casazza, A. P., et al. (2002). Engineering of cysteine and methionine biosynthesis in potato. Amino Acids 22 (3), 259–278. doi: 10.1007/s007260200013
Ostrowski, M., Ciarkowska, A., Dalka, A., Wilmowicz, E., Jakubowska, A. (2020). Biosynthesis pathway of indole-3-acetyl-myo-inositol during development of maize (Zea mays l.) seeds. J. Plant Physiol. 245, 153082. doi: 10.1016/j.jplph.2019.153082
Pang, Z. Q., Chong, J., Zhou, G. Y., Morais, D. A. D., Chang, L., Barrette, M., et al. (2021). MetaboAnalyst 5.0: narrowing the gap between raw spectra and functional insights. Nucleic Acids Res. 49 (W1), W388–W396. doi: 10.1093/nar/gkab382
Perincherry, L., Witaszak, N., Urbaniak, M., Waskiewicz, A., Stepien, L. (2021). Effects of secondary metabolites from pea on Fusarium growth and mycotoxin biosynthesis. J. Fungi 7 (12), 1004. doi: 10.3390/jof7121004
Picot, A., Barreau, C., Pinson-Gadais, L., Piraux, F., Caron, D., Lannou, C., et al. (2011). The dent stage of maize kernels is the most conducive for fumonisin biosynthesis under field conditions. Appl. Environ. Microbiol. 77 (23), 8382–8390. doi: 10.1128/AEM.05216-11
Poschenrieder, C., Tolra, R. P., Barcelo, J. (2005). A role for cyclic hydroxamates in aluminium resistance in maize? J. Inorganic Biochem. 99 (9), 1830–1836. doi: 10.1016/j.jinorgbio.2005.05.017
Ravanel, S., Gakiere, B., Job, D., Douce, R. (1998). The specific features of methionine biosynthesis and metabolism in plants. Proc. Natl. Acad. Sci. United States America 95 (13), 7805–7812. doi: 10.1073/pnas.95.13.7805
Ribbenstedt, A., Ziarrusta, H., Benskin, J. P. (2018). Development, characterization and comparisons of targeted and non-targeted metabolomics methods. PloS One 13 (11), e0207082. doi: 10.1371/journal.pone.0207082
Righetti, L., Dall’Asta, C., Lucini, L., Battilani, P. (2021). Lipid signaling modulates the response to fumonisin contamination and its source, Fusarium verticillioides, in maize. Front. Plant Sci. 12, 701680. doi: 10.3389/fpls.2021.701680
Righetti, L., Lucini, L., Giorni, P., Locatelli, S., Dall’Asta, C., Battilani, P. (2019). Lipids as key markers in maize response to fumonisin accumulation. J. Agric. Food Chem. 67 (14), 4064–4070. doi: 10.1021/acs.jafc.8b06316
Saha, J., Brauer, E. K., Sengupta, A., Popescuz, S. C., Gupta, K., Gupta, B. (2015). Polyamines as redox homeostasis regulators during salt stress in plants. Front. Environ. Sci. 3, 21. doi: 10.3389/fenvs.2015.00021
Samayoa, L. F., Butron, A., Malvar, R. A. (2014). QTL mapping for maize resistance and yield under infestation with sesamia nonagrioides. Mol. Breed. 34 (3), 1331–1344. doi: 10.1007/s11032-014-0119-y
Santiago, R., Cao, A., Butron, A. (2015). Genetic factors involved in fumonisin accumulation in maize kernels and their implications in maize agronomic management and breeding. Toxins 7 (8), 3267–3296. doi: 10.3390/toxins7083267
Santiago, R., Cao, A., Malvar, R. A., Butron, A. (2020). Genomics of maize resistance to fusarium ear rot and fumonisin contamination. Toxins 12 (7), 431. doi: 10.3390/toxins12070431
Santiago, R., Cao, A., Malvar, R. A., Reid, L. M., Butron, A. (2013). Assessment of corn resistance to fumonisin accumulation in a broad collection of inbred lines. Field Crops Res. 149, 193–202. doi: 10.1016/j.fcr.2013.04.011
Schrimpe-Rutledge, A. C., Codreanu, S. G., Sherrod, S. D., McLean, J. A. (2016). Untargeted metabolomics strategies-challenges and emerging directions. J. Am. Soc. Mass Spectrometry 27 (12), 1897–1905. doi: 10.1007/s13361-016-1469-y
Shimojima, M., Ohta, H. (2022). “Chapter two - plant and algal galactolipids: their function, biosynthesis and evolution,” in Advances in botanical research. Eds. Rébeillé, ,. F., Maréchal, E. 101, 59–89. doi: 10.1016/bs.abr.2021.09.007
Subramanian, A., Tamayo, P., Mootha, V. K., Mukherjee, S., Ebert, B. L., Gillette, M. A., et al. (2005). Gene set enrichment analysis: a knowledge-based approach for interpreting genome-wide expression profiles. Proc. Natl. Acad. Sci. U. S. A. 102 (43), 15545–15550. doi: 10.1073/pnas.0506580102
Sun, M. X., Liu, X. L., Gao, H. F., Zhang, B. B., Peng, F. T., Xiao, Y. S. (2022). Phosphatidylcholine enhances homeostasis in peach seedling cell membrane and increases its salt stress tolerance by phosphatidic acid. Int. J. Mol. Sci. 23 (5), 2585. doi: 10.3390/ijms23052585
Tims, M. C., Batista, C. (2007). Effects of root isoquinoline alkaloids from Hydrastis canadensis on Fusarium oxysporum isolated from Hydrastis root tissue. J. Chem. Ecol. 33, 1449–1455. doi: 10.1007/s10886-007-9319-9
Tsugawa, H., Ikeda, K., Takahashi, M., Satoh, A., Mori, Y., Uchino, H., et al. (2020). A lipidome atlas in MS-DIAL 4. Nat. Biotechnol. 38 (10), 1159. doi: 10.1038/s41587-020-0531-2
Vrabka, J., Niehaus, E. M., Munsterkotter, M., Proctor, R. H., Brown, D. W., Novak, O., et al. (2019). Production and role of hormones during interaction of Fusarium species with maize (Zea mays l.) seedlings. Front. Plant Sci. 9, 1936. doi: 10.3389/fpls.2018.01936
Wang, L. P., Shen, W. Y., Kazachkov, M., Chen, G. Q., Chen, Q. L., Carlsson, A. S., et al. (2012). Metabolic interactions between the lands cycle and the kennedy pathway of glycerolipid synthesis in arabidopsis developing seeds. Plant Cell 24 (11), 4652–4669. doi: 10.1105/tpc.112.104604
Wang, Y. P., Zhou, Z. J., Gao, J. Y., Wu, Y. B., Xia, Z. L., Zhang, H. Y., et al. (2016). The mechanisms of maize resistance to Fusarium verticillioides by comprehensive analysis of RNA-seq data. Front. Plant Sci. 7, 1654. doi: 10.3389/fpls.2016.01654
Keywords: Zea mays L., maize, Fusarium verticillioides, fumonisins, untarget metabolomics, resistance, fusarium ear rot
Citation: Cao A, Gesteiro N, Santiago R, Malvar RA and Butrón A (2023) Maize kernel metabolome involved in resistance to fusarium ear rot and fumonisin contamination. Front. Plant Sci. 14:1160092. doi: 10.3389/fpls.2023.1160092
Received: 06 February 2023; Accepted: 27 June 2023;
Published: 19 July 2023.
Edited by:
Olubukola Oluranti Babalola, North-West University, South AfricaReviewed by:
Magda Pál, Centre for Agricultural Research, HungaryJianyu Wu, Henan Agricultural University, China
Copyright © 2023 Cao, Gesteiro, Santiago, Malvar and Butrón. This is an open-access article distributed under the terms of the Creative Commons Attribution License (CC BY). The use, distribution or reproduction in other forums is permitted, provided the original author(s) and the copyright owner(s) are credited and that the original publication in this journal is cited, in accordance with accepted academic practice. No use, distribution or reproduction is permitted which does not comply with these terms.
*Correspondence: Ana Butrón, abutron@mbg.csic.es