- 1Rice Breeding Innovations Platform, International Rice Research Institute, Los Baños, Laguna, Philippines
- 2College of Agriculture and Food Science, University of the Philippines, Los Baños, Laguna, Philippines
- 3PhilRice Negros Branch Station, Philippine Rice Research Institute, Murcia, Negros Occidental, Philippines
- 4Cant Ho University, Cant Ho, Vietnam
- 5College of Agriculture, University of Southern Mindanao, Kabacan, North Cotabato, Philippines
Breeding staple crops with increased micronutrient concentration is a sustainable approach to address micronutrient malnutrition. We carried out Multi-Cross QTL analysis and Inclusive Composite Interval Mapping for 11 agronomic, yield and biofortification traits using four connected RILs populations of rice. Overall, MC-156 QTLs were detected for agronomic (115) and biofortification (41) traits, which were higher in number but smaller in effects compared to single population analysis. The MC-QTL analysis was able to detect important QTLs viz: qZn5.2, qFe7.1, qGY10.1, qDF7.1, qPH1.1, qNT4.1, qPT4.1, qPL1.2, qTGW5.1, qGL3.1, and qGW6.1, which can be used in rice genomics assisted breeding. A major QTL (qZn5.2) for grain Zn concentration has been detected on chromosome 5 that accounted for 13% of R2. In all, 26 QTL clusters were identified on different chromosomes. qPH6.1 epistatically interacted with qZn5.1 and qGY6.2. Most of QTLs were co-located with functionally related candidate genes indicating the accuracy of QTL mapping. The genomic region of qZn5.2 was co-located with putative genes such as OsZIP5, OsZIP9, and LOC_OS05G40490 that are involved in Zn uptake. These genes included polymorphic functional SNPs, and their promoter regions were enriched with cis-regulatory elements involved in plant growth and development, and biotic and abiotic stress tolerance. Major effect QTL identified for biofortification and agronomic traits can be utilized in breeding for Zn biofortified rice varieties.
Introduction
Globally, more than 3 billion people depend on rice for their daily caloric intake and nutritional needs (Yadaw et al., 2006; Elert, 2014; Bouis and Saltzman, 2017). However, milled rice is a poor source of micronutrients; hence, majority of the resource-poor rice consumers without access to adequate nutrition suffer mineral deficiencies (Dipti et al., 2012; Goudia and Hash, 2015; Garcia-Oliveira et al., 2018; Goloran et al., 2019; Ludwig and Slamet-Loedin, 2019). Iron (Fe) and Zinc (Zn) malnutrition is common across all age groups, especially among children and women in the developing world (Bouis et al., 2002; Ahmed et al., 2016; Palanog et al., 2019; Gupta et al., 2020; Calayugan et al., 2021). Increasing the mineral density in the edible portion of the staple crops is a food-based approach to tackle mineral deficiencies. It is reported to be the most economical and sustainable solution to address malnutrition (Dipti et al., 2012; Bouis and Saltzman, 2017; Goloran et al., 2019; Ludwig and Slamet-Loedin, 2019). However, for the successful adoption and consumption of biofortified rice varieties, they should be high-yielding, agronomically-superior, and desirable in terms of grain quality (Swamy et al., 2016; Swamy et al., 2021b).
Rice has a vast amount of genetic diversity available in cultivars, landraces, and wild relatives (Khush, 1997; Jackson and Lettington, 2003; Huggins et al., 2019). In particular, aus is a genetically-distinct group of rice accessions mainly originated from Bangladesh and India (Garis et al., 2005; Norton et al., 2018; Swamy et al., 2018). They are well-known for their wider adaptability, tolerance to biotic and abiotic stresses, and nutritional value (Xu et al., 2006; Ali et al., 2011; Henry et al., 2011; Gamuyao et al., 2012; Zhu et al., 2016). Currently, aus accessions are being explored for Zn biofortification due to their high grain Zn concentration (Swamy et al., 2018; Rakotondramana et al., 2022).
Quantitative trait loci (QTL) mapping using bi-parental populations is widely popular in rice (Al-Shugeairy et al., 2014; Travis et al., 2015). However, it has limited recombination and lesser allelic diversity, which results in larger confidence intervals of the QTLs, and captures only a portion of the total genetic variation (Xu et al., 2016; Verdeprado et al., 2018). Further, QTLs have to be fine-mapped and validated in multiple genetic backgrounds for their use in Marker Assisted Breeding (MAB). An alternative approach is QTL mapping in multi-parent populations that can increase the power of detecting major QTLs, and helps to understand QTL interactions and QTL by genetic background effects (Wang et al., 2014; Descalsota et al., 2018). Multi-parent advanced generation intercross (MAGIC) and nested-association mapping (NAM) populations increase the probability of identifying precise QTL (Bandillo et al., 2013).
In this study, we developed four connected recombinant inbred lines (RILs) populations derived from Kaliboro (IRGC 77201-1) - a high grain Zn germplasm crossed with four elite Zn breeding lines. These populations were analyzed using multi-cross QTL (MC-QTL) method to identify QTLs for agronomic, yield and biofortification traits, candidate genes were shortlisted for major effect QTLs, and detailed analyses of major effect Zn QTLs was conducted to characterize candidate genes and cis-regulatory elements.
Materials and methods
Materials
All the four recombinant inbred lines (RILs) populations were developed using high Zn aus donor parent Kaliboro::IRGC77201-1 (P2), while promising Zn breeding lines developed at International Rice Research Institute (IRRI) were used as recipient parents viz; IR14M141 (P1), IR14M110 (P3), IR14M125 (P4), and IR95044:8-B-5-22-19-GBS (P5) (Table S1).
Field establishment and phenotyping
All the mapping populations were grown in an alpha lattice design with two replications at Zeigler Experimental Station at IRRI during the dry and wet seasons of 2017 (DS2017 and WS2017) and in the dry season of 2018 (DS2018). Seeds were sown in the seedbed, and 21-day old seedlings were transplanted in the field at 20 cm x 20 cm planting distance. Each plot consisted of 2 rows with 20 hills per plot. Inorganic nitrogen (N), phosphorus (P), and potassium (K) fertilizers were applied at the rate of 120:30:30 NPK kg ha-1 during the DS and 90:30:30 NPK during the WS. Standard crop management practices were employed to ensure good crop growth.
We collected data on days to 50% flowering (DF), plant height (PH), number of tillers (NT), number of productive tillers (PT), panicle length (PL), thousand-grain weight (TGW), grain length (GL), grain width (GW) and grain yield per hectare (GY). We followed the standard evaluation system to gather data (IRRI, 2014). The Fe and Zn concentrations in the rice grains was measured using 3 grams of milled rice per sample by using XRF-Bruker S2 Ranger. Samples were analyzed twice, and mean values were considered for statistical analysis.
Statistical analysis of phenotypic traits
Basic statistical analyses were performed using STAR v.2.0.1 (http://bbi.irri.org), PBTools v1.4 (http://bbi.irri.org), and R v3.5.2 in R Studio (RStudio Team, 2015). Best linear unbiased prediction (BLUP) values for each of the traits across three seasons were computed using PBTools v1.4, and were used for further analyses. Pearson’s correlation, histograms, analysis of variance (ANOVA), and descriptive statistics of traits were performed using R v3.5.2 in R Studio v1.0.153 (RStudio Team, 2015). Broad-sense heritability (H2) of all the traits was estimated using following formula:
Where σ2g is the genotypic variance, σ2p is the phenotypic variance,
DNA extraction and genotyping
Fresh leaf samples were collected from RILs and parental lines during the early seedling stage. Samples were ground after freezing in liquid nitrogen and genomic DNA was extracted from each sample using the modified CTAB method (Murray and Thompson, 1980), and the quality of DNA was estimated by using 1% agarose gel electrophoresis. High-quality DNA samples with desirable concentration (~50 ng) were submitted to IRRI-Genotyping Services Laboratory (GSL) for SNP genotyping using the 7K Infinium Chip (Morales et al., 2020).
SNP genotypic data analysis
A set of 7,086 high-quality SNP markers were generated after thorough filtering using Illumina’s protocol based on >80% call rate, homozygosity, and polymorphism between respective parents. SNP markers were further filtered based on homozygosity of the parents and with high heterozygosity on the segregating families. There were 495 high quality SNPs selected based on the distribution across the genome with < 20% missing SNPs among the parental lines. The selected SNPs were used to generate individual genetic maps and merged to construct a consensus physical map using BioMercator v4.2.1 considering a conversion of 250 kb = 1 cM. The scoring of alleles for the combined populations was performed by comparing parental alleles for each SNP. Four recipient parents were scored as “A”, donor allele was scored as “B”, and ambiguous/missing data were scored as “X”.
Multi-cross QTL (MC-QTL) analysis
Combined phenotypic (BLUP) and genotypic data of four populations were used to perform MC-QTL analysis using MC-QTL v1.0 (Jourjon et al., 2005; De Oliviera et al., 2016). A linear regression model and iterative QTL mapping method (iQTLm) was used to detect QTL (Haley and Knott, 1992; Charcosset et al., 2000). A logarithm of odds (LOD) threshold of ≥5 was used to declare putative QTLs (Churchill and Doerge, 1994). The Phenotypic variance (R2) for each QTL and global R2 for each trait were generated. Epistatic analysis was also performed.
QTL detection using inclusive composite interval mapping (ICIM)
QTL detection for each individual bi-parental mapping population was conducted using inclusive interval mapping (ICIM) with the aid of IciMapping v.4.1 (Wang, 2009). Critical threshold value for QTL detection was calculated by 1000 random permutations of the phenotypic data to establish an experiment-wise significance value at 0.05 (Churchill and Doerge, 1994). Estimated phenotypic variance explained by QTL for each trait and corresponding additive effect was also generated.
Candidate gene analysis
Consistent major effect QTLs with overlapping regions and with consensus boundaries of ≤500 Kb, found across different types of QTL analysis were subjected to candidate gene analysis. Predicted candidate genes were searched within or near ( ± 500 Kb) the QTL for each trait using its flanking markers. Physical locations of the annotated genes were determined with the aid of the RAP-DB (http://rapdb.dna.affrc.go.jp/viewer/gbrowse/irgsp1) (accessed on March 20, 2019). Functions, ontology, and gene networks for each of the candidate genes were determined using the KNetminer (http://knetminer.rothamsted.ac.uk/Oryzasativa/) (accessed on March 20, 2019) and the Rice Genome Annotation Project (http://rice.plantbiology.msu.edu/cgi-bin/gbrowse/rice/) (accessed on March 20, 2019). The patterns of gene expression in various organs and stages were displayed using the eFP browser (http://bar.utoronto.ca/efprice/cgi-bin/efpWeb.cgi) (accessed on March 21, 2019), and the CoNekT (http://conekt.mpimp-golm.mpg.de/pub/) (accessed on March 21, 2019). Further searches on the previously reported QTL that co-localize with the QTL identified in our study were made using the Gramene (http://archive.gramene.org/qtl/) (accessed on March 22, 2019).
In silico gene prediction and cis-regulatory elements analysis of grain Zn QTL
SNPs within the candidate genes related to Zn homeostasis were downloaded from the rice SNP-Seek 18 (http://snp-seek.irri.org) (accessed on March 22, 2019). Coordinates of the genomic regions of interest were identified using the reference genome (Nipponbare) by extracting flanking sequences and aligning these to the target reference genome assemblies of IR64 and Kaliboro 600 (representing the genomes of the parents). A region in the target genome with best flank hits was then extracted and characterized. Alternative SNPs between indica-IR64 (representing the recipient parents) and aus-Kaliboro 600 (representing the donor parent) were identified using Rice SNP-Seek 18 (Mansueto et al., 2017). SNP genotyping data were sourced from 3K Rice Genome Project (The 3,000 rice genome project, 2014; Wang et al., 2018). The 1 Kb upstream region of each gene was used to shortlist the SNPs in their promoter regions. All polymorphic SNPs in the promoter and coding regions were listed.
Predicted genes showing SNP polymorphisms between the query genomes were further subjected to cis-regulatory analysis. The 1Kb region from the coding sequences in the 5’ upstream strand of the Zn homeostasis gene was scanned for putative cis-regulatory elements using PlantPAN (http://PlantPAN.mbc.nctu.edu.tw) (accessed on March 23, 2019).
Results
Phenotypic analysis of connected populations
The data of the various traits from four populations evaluated over three seasons are presented in Table 1. All the traits had large phenotypic variations and showed typical normal distribution across seasons and populations (Table 1 and Figures S1, S2). The highest mean values were recorded for GY, TGW, and GW during the DS; while DF, PH, and PL were higher during the WS. Grain Zn and Fe concentration were high during the DS. A low coefficient of variation (CV, <10%) was observed for DF and GL, whereas a moderate to high CV (10-45%) was observed for other traits. High broad sense heritability (H2) was observed for Zn, DF, PH, and TGW (>70%) while it was low to moderate (10 to 60%) for all other traits (Table 1). Zn exhibited moderate to strong negative correlation with GY across populations (Figures 1A–D). Likewise, it has moderate negative correlation with TGW and GL in Pop2 (Figure 1B), and strong negative correlation with DF in Pop3 (Figure 1C). Similarly, Fe was negatively correlated with several agronomic traits: PH, PL, DF, TGW, and GL in Pop1, Pop2 and Pop3 (Figures 1A–C), while showed positive correlation with GW, NT, PT and GY in Pop4 (Figure 1D). Meanwhile, a strong positive correlation between NT, PT, TGW, and GL; with PH and PL were observed consistently across populations (Figures 1A–D). Notable positive correlations were observed among agronomic traits such as TGW, GW, PH, DF, and GL. The grain Fe and Zn concentration had consistent positive correlation across populations (Figures 1A–D).
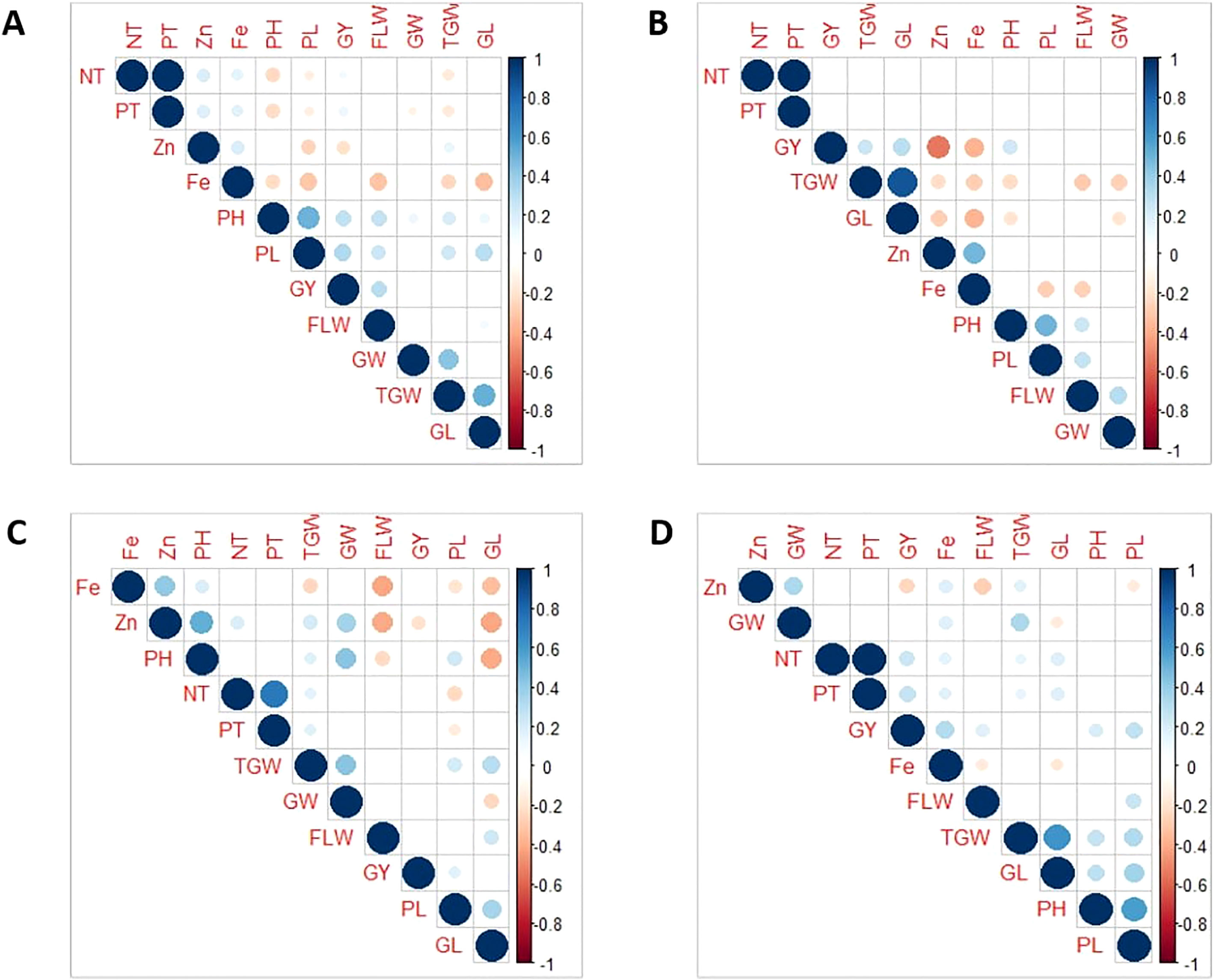
Figure 1 Pearson’s correlation coefficients of 11 traits in four connected populations: Pop1 (A), Pop2 (B), Pop3 (C), and Pop4 (D). GY, Grain Yield; DF/FLW, Days to flowering; PH, Plant Height; NT, Number of Tillers; PT, Productive Tillers; PL, Panicle Length; TGW, Total Grain Weight; GL, grain length; GW, Grain width.
Multi-cross QTL analyses of connected populations
Overall, 495 SNPs across four populations were used to construct a consensus genetic map and used for MC-QTL analyses. The number of SNPs per chromosome ranged from 11 to 75 with lowest and highest number of SNPs on chromosomes 9 and 1, respectively (Figure S5). The total map length was 1627 cM with an average distance of 6.0 cM between SNPs. MC-QTL analyses identified MC-156 QTLs for 11 traits (Table 2). The global R2 captured by the QTLs varied from 12 to 58% for various traits, while the R2 explained by individual QTL ranged from 0.1 to 22%. The two major QTLs with highest R2 (22% and 13%, respectively) were qPH1.1 and qZn5.2. The tall parent (P2) allele increased PH by 7.19 cm while the other parents reduced PH by 1.77 to 1.91 cm. Some of the major QTLs for agronomic and yield traits were: qDF7.1, qNT4.1, qPT4.1, qPL1.2, qTGW5.1, qGL3.1, qGW6.1 and qGY10.1 (Table 2). The qDF7.1located between id7005418 and c7p27670416 on chromosome 7 explained 7.3% R2, P1 allele increased maturity by 1.70 days while P5 allele reduced maturity by 0.80 days. Whereas, qNT4.1 and qPT4.1 were detected between 3647133 and 3653113 on chromosome 4, each explained by a R2 >3%. Meanwhile, qPL1.2detected on chromosome 1 explained 7% R2, and qTGW5.1 identified between 5020568 and 5056493 increased TGW by 0.52 g. Among the 21 QTLs identified for GL, qGL3.1 explained 8% R2, P1 allele increased GL by 0.09 mm. For grain width, qGW6.1 contributed 4.2% R2 and P3 allele increased grain width 0.03 mm. The qGY10.1 explained 4% R2 with highest positive allelic contribution from P2 (85.5 kg/ha).

Table 2 QTL detected for Zn, Fe, GY, and GY-related traits of four connected populations using MC-QTL.
A total of 27 QTL was detected for Zn that explained R2 of 0.3 to 13.0% with a global R2 of 58%. As expected, the donor parent (P2) had the highest combined additive effect (2.6 ppm). Interestingly, the recipient parent (P5) total allele contribution was 2.5 ppm (Table 2). A major QTL (qZn5.2) with an R2 of 13% was detected on chromosome 5 at 22.03 Mb flanked by markers 5711540 and 5726844. It has a narrow genomic region of 600 Kb among the Zn QTLs. There were three QTLs, qZn3.2, qZn11.1aand qZn12.1b each contributed >3% R2. Similarly for Fe, 14 QTLs were detected with R2 ranging 0.7-3.2% and a global R2 of 25%. These QTL were located on all the chromosomes except chromosomes 5 and 10. The qFe7.2, located on chromosome 7 at 21 Mb, accounted for the highest R2 (3.2%). However, the highest positive allele contribution with 0.16 ppm increase in Fe concentration observed for qFe2.1.
Co-location of QTL identified
QTLs that shared common SNPs were considered co-located. In all, 26 QTL clusters were identified on different chromosomes (Figure 2). Four QTLs, qGY5.1, qTGW5.1, qGL5.1, and qGW5.1 were co-located at 2.5 Mb on chromosome 5, while QTLs qZn8.1, qFe8.1, qNT8.1, and qPT8.1 were at the same genomic region on the short arm of chromosome 8. Similarly, qGY9.1, qZn9.1, qFe9.1, and qGL9.1 co-localized between markers id9000710 and 9569595 on chromosome 9. We observed three QTLs clusters at four genomic regions on chromosomes 3, 4, 8, and 9, while 20 genomic regions had two QTLs co-located (Figure 2).
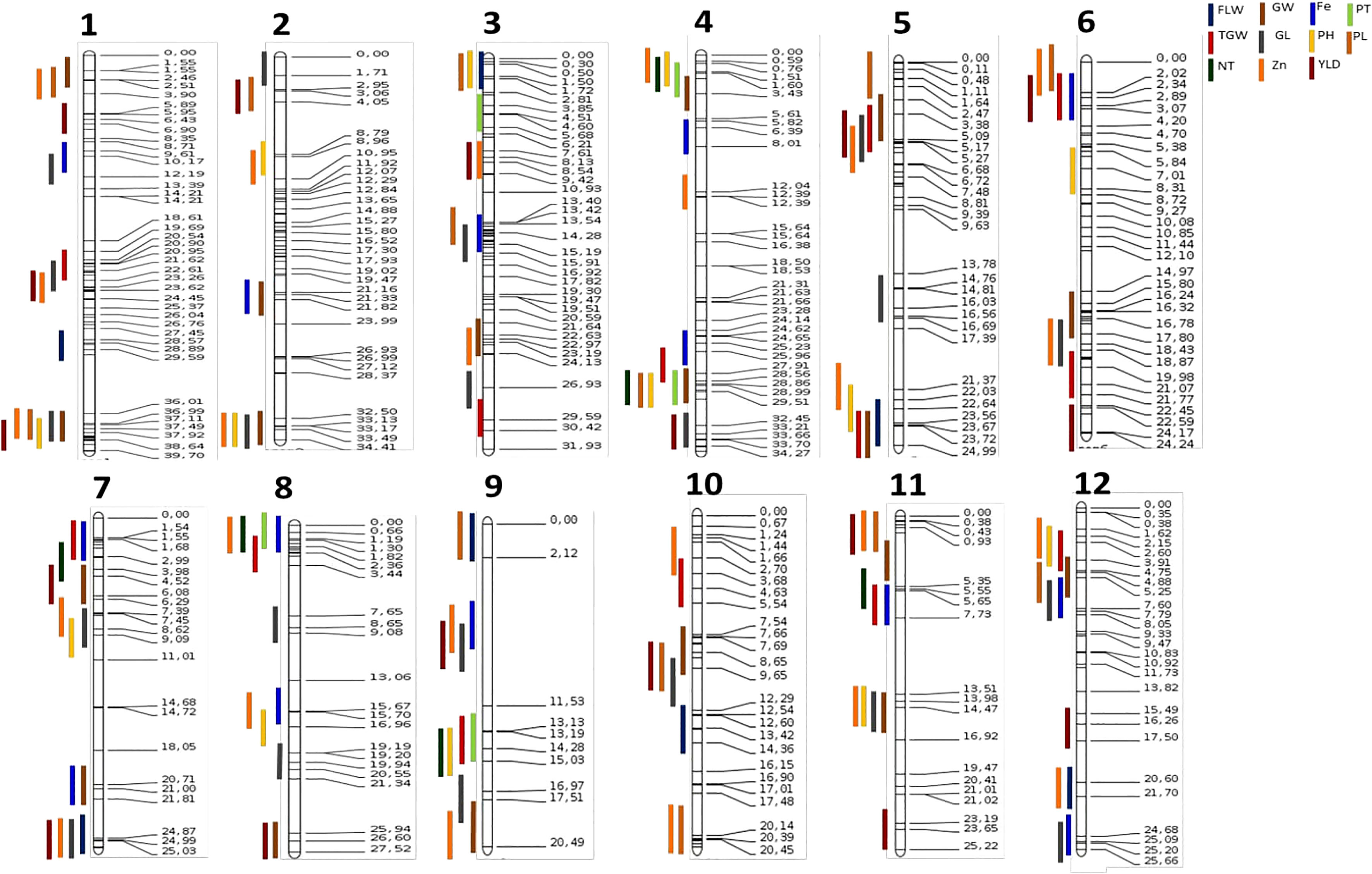
Figure 2 Consensus map of QTL detected for various traits. Vertical colored lines on the left represent different QTL while values on the horizontal lines at the right side show the position of the markers on the chromosomes; numbers below the figure indicate the corresponding chromosome.
Epistasis
Epistatic interactions were detected between qZn5.1 and qPH6.1, and qPH6.1 and qGY6.2; each explained a R2 of 5% (Table S2). qPH6.1 is a minor QTL flanked by id6007220 and 6228191 with genetic interval of 8.08 Mb. The positive allele (P3) increased PH by 1.25 cm while P4 allele reduced PH by 1.70 cm. On the other hand, qZn5.1 is also a minor allele associated with Zn flanked by 514403 and SNP-5.9394949 with positive allele contributed by P2 (0.60 ppm). Minor QTL, qPH6.1 is also interacted with qGY6.2 which was flanked by markers 6907224 and id6016547 with a narrow interval of 0.07 Mb. The positive alleles were contributed by P3 and P4 with an additive effect of 100 kg/ha (Table 2).
Candidate genes for agronomic, yield and biofortification traits
Largest-effect QTL for grain yield, agronomic traits, Fe, and all the QTLs detected for grain Zn were subjected to candidate gene analysis (Table 2 and Figure S3). Genes conferring biological functions related to the trait were shortlisted (Table 3). The OsMADS18 is co-located with qFe7.1, it helps in Fe transport, cellular and inter-cellular responses under Fe deficiency, it is also known to regulate seed maturation and days to maturity. Similarly, HUA2 was linked to flowering locus qDF7.1 and regulates flowering time and reproductive development. A pyruvate dehydrogenase kinase gene (OsPdk1) is found nearest to qPL1.2 which is involved in root hair length and panicle threshability. Meanwhile, Glycogen Synthase Kinase (GSK) is found to influence days to maturity, seed maturation, brown rice shape, grain width and grain weight. GSK2 was co-located with major effect QTL, qTGW5.1, while GSK4 was co-located with qGW6.1. Similarly, GS3 was within the confidence interval of qGL3.1. Candidate genes OS01G0899425, OS04G0350700, and OS10G0326900 were co-located with qPH1.1, qPT4.1, and qGY10.1, respectively (Table 3).
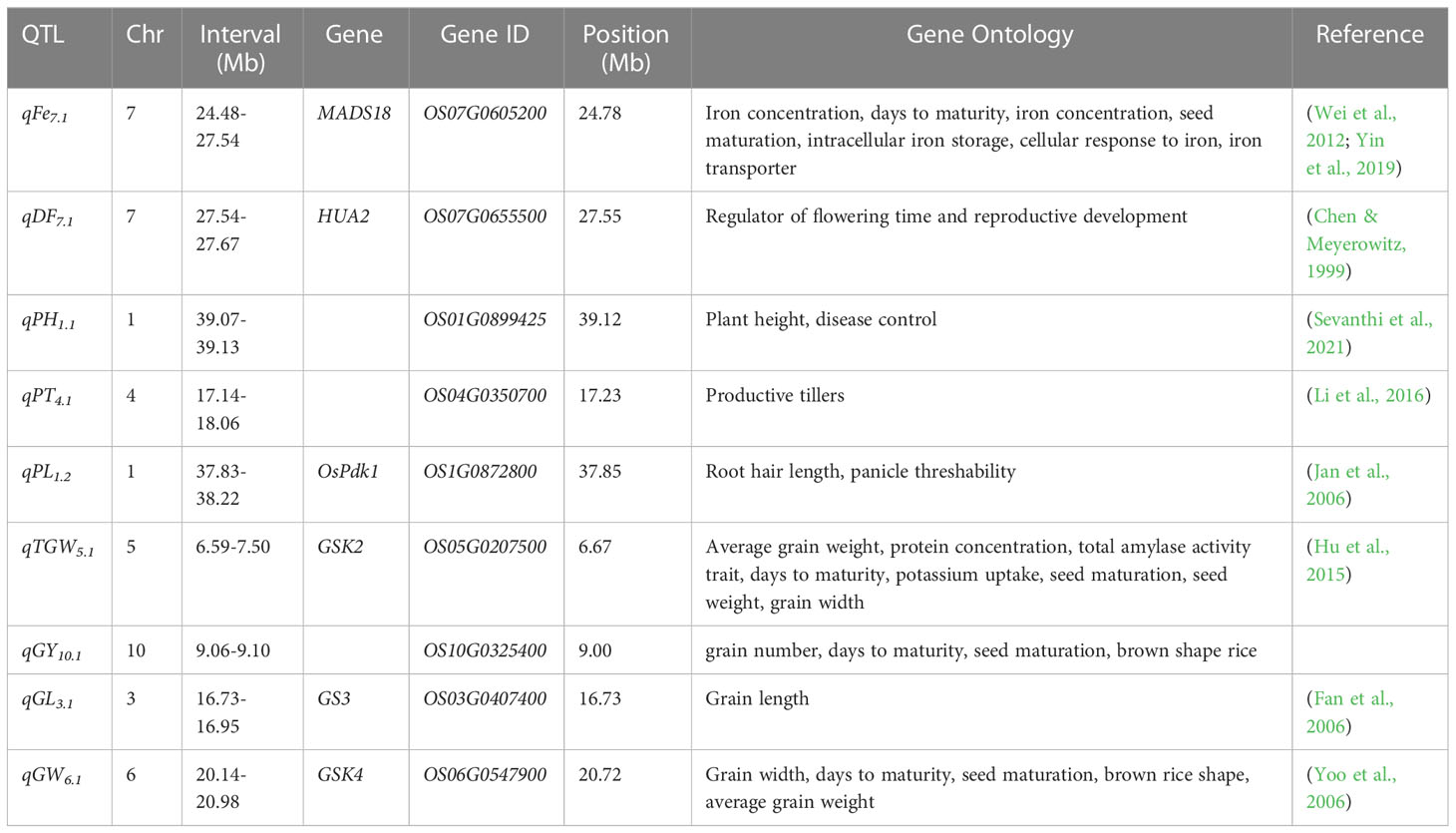
Table 3 Identified putative genes associated with major QTL for Zn, Fe, GY and grain-related traits of connected populations.
Candidate gene prediction and cis-regulatory analysis of qZn5.2
The details of 20 candidate genes shortlisted from qZn5.2 are presented in Table 4. Most of the candidate genes were either Zn finger or metal cation transporters. Four putative candidate genes identified within or nearest the QTL Viz: LOC_OS05G39540 (OsZIP9), LOC_OS05G39560 (OsZIP5), LOC_OS05G40490 and LOC_OS05G41790. These genes are differentially expressed in roots, leaves, stems, flowers, and meristems (Figure S4). OsZIP5 was highly expressed in stem, internode and seeds, and moderately expressed in roots; OsZIP9 highly expressed in roots differentiation zone, and in inflorescence. LOC_OS05G40490 had minimum expression in leaves during drought stress, but LOC_OS05G41790 has high expression in seeds and had moderate expression in leaves under well-watered and drought conditions (Figure S4). The in-silico gene prediction analyses of all these genes using the 3k genome identified 75 non-synonymous SNPs, 23 transversions, 34 transitions, and 18 deletions. Out of 20, 15 genes have non-synonymous SNPs found in their promoter and coding sequences (Table S3). The highest number of transitions (A/C, A/T, or G/C) was identified for OsZIP9 and LOC_OS05g40490, while OsZIP9 had the highest number of transversions (A/G or T/G). LOC_OS05g40490 had the highest number of deletions. The 15 predicted genes that show non-synonymous SNPs were further investigated for cis-regulatory elements in their promoter regions using PlantPAN. A large number of cis-regulatory elements were detected and they were mainly associated with important physiological processes involved in submergence tolerance, light-regulation, meristematic tissue activities, and disease resistance (Figure 3).
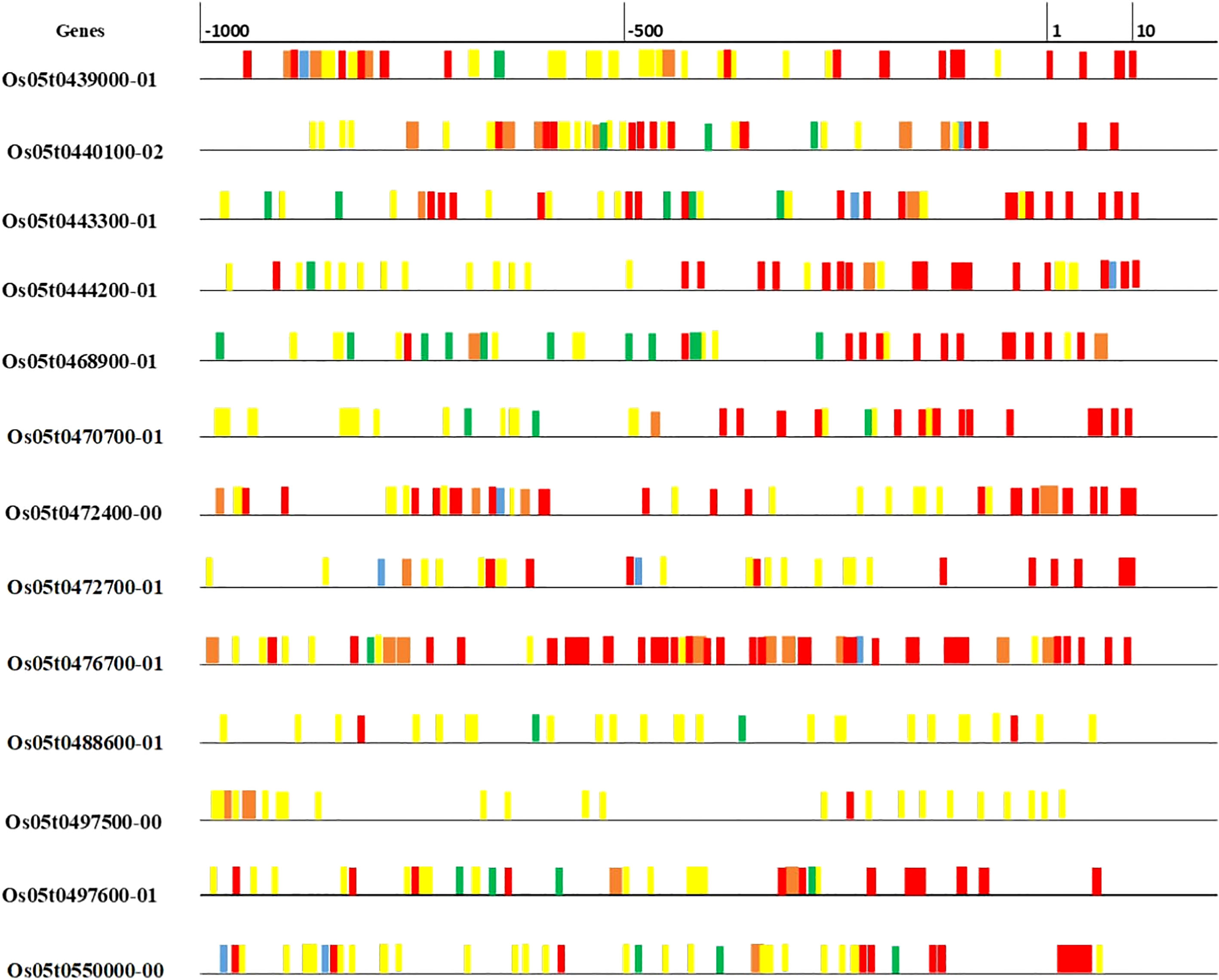
Figure 3 Cis-regulatory elements in the upstream region (500 Kb) of predicted genes for grain Zn. Various elements are associated with physiological functions such as submergence tolerance (blue), merismatic (orange), disease resistance (yellow), light regulation (green), amylase synthesis (red) were indicated using different colors.
Discussion
Breeding for improved nutrition has been prioritized in rice and other major staple food crops (Bouis and Saltzman, 2017; Gaikwad et al., 2020). There have been significant efforts over the last one decade to breed for high Fe, Zn and Vitamin A enriched crop varieties, and to deploy them on a large scale to create a health impact (Mwanga et al., 2021; Swamy et al., 2021a; Biswas et al., 2021). In rice, Zn biofortification breeding has been taken up on a large scale by IRRI and its partners leading to release of several high Zn rice varieties in Asia and Africa (Swamy et al., 2016; Tsakirpaloglou et al., 2019; Calayugan et al., 2021). Recently, Zn mainstreaming breeding has been initiated to incorporate Zn as a must trait in all the future rice varieties (Stangoulis and Knez, 2022). Identification of diverse donors, QTLs, genes, and a better understanding of molecular basis of grain Zn concentration, agronomic and yield traits are essential for the efficient Zn biofortification of rice (Swamy et al., 2016, Swamy et al., 2018; Calayugan et al., 2020; Rakotondramana et al., 2022)
We used high Zn aus accession Kaliboro (IRGC 77201-1) to develop four RILs mapping populations. It has acceptable yield potential (~4900 kg/ha) and twice the amount of grain Zn (~40 ppm) in comparison to popular rice varieties (14-16ppm). In addition to that aus accessions are also genetically diverse and adaptable to a wide range of environmental conditions (Redoña and Mackill, 1996; Xu et al., 2006; Jagadish et al., 2008; Norton et al., 2010; Henry et al., 2011; Gamuyao et al., 2012; Al-Shugeairy et al., 2014). All the four recipient parents were high Zn breeding lines (12-19 ppm) developed at IRRI.
A wide range of variation for GY, Zn, Fe, and other major agronomic traits was observed in all the populations. Most of the traits exhibited normal distribution indicating the complex genetic basis of these traits (Table 1 and Figures S1, S2). Broad-sense heritability (H2) was high for DF, PH, TGW, and Zn across populations (Table 1), which permits effective phenotypic selection for their improvement. These results are in consonance with earlier reports on traits distributions and heritability (Widodo et al., 2010; Du et al., 2013; Zhang et al., 2014; Swamy et al., 2016; Chang et al., 2018; Swamy et al., 2018). Consistent positive correlations were observed between Fe and Zn (Figure 1) indicating the possibility of simultaneous improvement (Swamy et al., 2016). However, concomitant breeding for high Zn and Fe with high GY will be challenging due to their strong negative correlations. Hence, appropriate breeding strategies would be essential for the successful Zn biofortification (Welch, 2004; Chang et al., 2018). One approach is to use high Zn donors with acceptable GY potential coupled with rapid generation advancement, speed breeding and genomic selection (Swamy et al., 2016; Calayugan et al., 2021; Swamy et al., 2021a; Yang et al., 2021). Moreover, breeding materials or populations that exhibit weak or no correlation between GY and Zn and transgressive seggregants that possess both high GY and Zn increases the chances of successful biofortification breeding (Figures 1, S1).
MC-QTL analysis detected major QTLs and epistasis
Genetic analyses using multi-parent derived populations or combined analysis of multiple biparental populations that share common parentage help to dissect major effect and stable QTLs, which can work across genetic backgrounds without any epistatic or genetic background effects (Specht et al., 2001; Jannink and Jansen, 2001; Blanc et al., 2006; Doust et al., 2014). We carried out MC-QTL analyses for 11 traits using a set of 495 SNPs distributed across 12 chromosomes (Figure S5). A high rate of SNPs polymorphism among parents was expected as Kaliboro (aus) and IR14M141, IR14M110, IR14M125, and IR95044:8-B-5-22-19-GBS (indica) are from genetically-distinct subgroups (Garis et al., 2005; Chen et al., 2014; Tang et al., 2016). The MC-QTL analysis detected more QTLs but effects were smaller compared to QTLs discovered by Inclusive Composite Interval Mapping (ICIM) (Tables 2 and S4). For instance, MC-QTL was able to detect 14 QTLs for Fe whereas only 2 QTLs were identified by ICIM. Some of the important QTLs detected using MC-QTL analysis viz: qZn5.2, qFe7.1, qGY10.1, qDF7.1, qPH1.1, qNT4.1, qPT4.1, qPL1.2, qTGW5.1, qGL3.1, and qGW6.1, which can be used in rice MAB and Genomic Selection (GS). Interestingly, most of the positive alleles for high GY and early DF were contributed by high yielding (IR14M110) and early maturing parents (IR95044:8-B-5-22-19-GBS) respectively. High-grain yield and early-maturity are two most desirable traits for rice varietal development (Zhao et al., 2011; MacKill and Khush, 2018; Li et al., 2019). Similarly, QTLs for PL and GW were contributed by Kaliboro, while TGW and GL were derived from IR14M141 (Table 2). Thus, it is evident that Kaliboro has contributed many positive alleles for yield and yield related traits and for improved grain Zn concentration. These favorable alleles can be pooled through genomics assisted breeding to develop rice varieties (Blanc et al., 2006; Grenier et al., 2015; Varshney et al., 2021; Xu et al., 2021; Reyes et al., 2022).
The QTLs of correlated traits (positive or negative) tend to cluster together on a chromosomal region (Zhang et al., 2019; Yu et al., 2021). The GY, TGW, GL, and GW were highly correlated, and their respective QTLs co-located on chromosome 5. All the QTLs for PT and NT, and Fe and Zn were co-located (Figures 1, 2). However, these co-locations may be due to pleiotropy or linkage or shared regulatory genes (Collard and MacKill, 2007; Du et al., 2013; Swamy et al., 2016; Descalsota et al., 2018). Positively correlated traits and their co-located QTLs can be readily mobilized into the breeding programs, while negative linkages must be removed by pre breeding, phenotypic selections, and fine mapping for their deployment in the rice breeding (Du et al., 2013; Swamy et al., 2016; Descalsota et al., 2018; Descalsota-Empleo et al., 2019a; Pradhan et al., 2020).
A notable result is that a major QTL for grain Zn (qZn5.2) is consistently identified in all the populations and also in the combined population analysis. It had a R2 of 13% in MC-QTL analysis and went up to 19.75% in ICIM (Table S4), with an additive effect ranging from 0.44 to 2.10 ppm (Tables 2 and S4), and had a narrow genetic interval of 600Kb. It is also devoid of any epistatic interaction or background effects, making it one of the potential candidate loci for MAB or GS to improve grain Zn concentration. This locus has been frequently reported from aus derived populations. Several studies have also identified similar genomic regions (Lu et al., 2008; Garcia-Oliveira et al., 2009; Zhang et al., 2014; Descalsota-Empleo et al., 2019b; Islam et al., 2020).
We observed very minimal epistasis among the QTLs. The PH (qPH6.1) interacted with GY (qGY6.1) and Zn (qZn6.1), but their R2 (<5%) and additive effects (0.12-1.25 cm, 9-282 kg/ha, 0.13-1.0 ppm, respectively) were low to make any significant changes in their phenotypic expression (Table S2). There were reports on genetic interactions between Zn and PH that affected their phenotypic performance, and also genetic background altering the epistasis effects leading to variable trait expression (Jiang et al., 2008; Lekklar et al., 2019). Therefore, epistatic QTLs and the influence of genetic backgrounds on major QTLs should be verified before their use in the breeding programs (Xu et al., 2015; Islam et al., 2019; Lekklar et al., 2019).
Candidate genes co-located with major QTLs
A number of candidate genes that co-localized with QTLs were identified for different traits such as HUA2 (DF), OsPdk1 (PL), OS01G0899425 (PH), OS04G0350700 (PT), GSK2 (TGW), OS10G0325400 (YLD), GS3 (GL), and GSK4 (GW) (Table 3). These genes have proven biological functions directly or indirectly related to the traits but also involved in the important physiological processes related to stress tolerance (Chen and Meyerowitz, 1999; Fan et al., 2006; Jan and Komatsu, 2006; Yoo et al., 2006; Wei et al., 2012; Hu et al., 2015; Jan and Komatsu, 2006; Li et al., 2016; Yin et al., 2019; Sevanthi et al., 2021). Previously reported QTLs and genes for yield and yield related traits and grain micronutrients match with our results (Anuradha et al., 2012; Du et al., 2013; Descalsota et al., 2018; Zhang et al., 2019; Pradhan et al., 2020). Some of these genes are potential candidates for functional validation and for introgression through MAB.
The qZn5.2 was also enriched with Zn/Metal homeostasis genes, especially Zn-finger proteins (Table 3). They play an important role in Zn uptake, transport and loading into the grains, and their expression provides abiotic stress tolerance under diverse climatic conditions (Goel et al., 2011; Zhang et al., 2014; Hara et al., 2017; Papierniak et al., 2018; Lekklar et al., 2019). It is noteworthy that these genes were also enriched with cis-regulatory elements in their promoter regions, and actively regulates physiological processes involved in metal homeostasis, submergence tolerance, meristematic tissue activities, light-regulation and disease resistance (Pandey et al., 2015; Biłas et al., 2020) (Table 4 and Figure 3).
Functional polymorphisms within genes play an important role in phenotypic diversity (Srivastava et al., 2014). We report that 15 of the 20 genes shortlisted from qZn5.2 had functional polymorphic SNPs; notable ones are OsZIP9, OsZIP5, and LOC_OS05G40490. These transcription factors regulate expression of Zn homeostasis genes such as OsNAS1, OsNAS2, and OsNAS3, therefore play a role in metal transport, partitioning and loading into grains (Guerinot, 2000; Chandel et al., 2010). There are previous reports showing upregulation of OsZIP5 in roots and flag leaves, and OsZIP9 in roots of rice (Banerjee and Chandel, 2011; Chadha-Mohanty et al., 2015), but they are homologs and complement in phytosiderophore-mediated Zn uptake (Lee et al., 2010). Recent haplotype analysis of OsZIP5 and OsZIP9 using a 3K rice panel revealed that superior haplotypes for these two genes were in high frequency in aus and indica sub groups (Gaurav et al., in press). On the other hand, there is less information on LOC_OS05G40490, a Zn knuckle domain-containing protein gene involved in metal binding. Some of these genes can be pyramided using gene specific markers, explored for superior haplotypes, and can be used to develop high Zn transgenics or genome edited rice lines.
Conclusion
We carried out MC-QTL and ICIM analysis for agronomic, yield and grain micronutrient traits using connected rice populations. In all 156 MC-QTLs, 26 QTL co-locations and two epistatic interactions were detected. The donor parent, Kaliboro, contributed many positive alleles. A major QTL (qZn5.2) for grain Zn concentration has been detected on chromosome 5 that accounted for 13% of R2. There were 20 genes within qZn5.2 that are involved in metal binding, uptake, transport, partitioning and loading in different tissues. Three primary putative genes, OsZIP5, OsZIP9, and LOC_OS05G40490, along with 12 other genes showed functional SNP polymorphisms indicating their role in metal homeostasis. These genes were also enriched with cis-regulatory elements that regulate mineral uptake, growth and development, and tolerance to biotic and abiotic stresses etc. All the major effect QTLs can be readily mobilized into breeding programs through genomics assisted breeding. Promising candidate genes can be characterized to understand their functional roles. Over all our results provide insights into molecular bases of Zn homeostasis, and identified major QTLs/genes useful for Zn biofotification of rice.
Data availability statement
The phenotypic and genotypic data used in the analyses are provided as supplementary data in this article.
Author contributions
AP and BS designed the paper. AP led the conduct of the experiment and wrote the whole manuscript. CN, GD-E, MC, and ZS analyzed and gathered some genotypic and phenotypic data. AA and MI-A assisted in the establishment of field experiments and collection of phenotypic data. JH, PS, TB, AL, RM, KM and BS thoroughly edited and contributed significantly to the improvement of the manuscript. All authors contributed to the article and approved the submitted version.
Funding
The work was supported by HarvestPlus (5402), Bill and Melinda Gates Foundation (OPP1194925), Department of Science and Technology (DOST) - Philippines. The funding entities did not influence the design, data collection, and interpretation, and write-up of the paper.
Acknowledgments
The authors would like to thank the biofortification group of IRRI for its invaluable help in the conduct of the study. AP thanks PhilRice for the scholarship and Dr Jaime Manalo IV of PhilRice for serving as English critic.
Conflict of interest
The authors declare that the research was conducted in the absence of any commercial or financial relationships that could be construed as a potential conflict of interest.
Publisher’s note
All claims expressed in this article are solely those of the authors and do not necessarily represent those of their affiliated organizations, or those of the publisher, the editors and the reviewers. Any product that may be evaluated in this article, or claim that may be made by its manufacturer, is not guaranteed or endorsed by the publisher.
Supplementary material
The Supplementary Material for this article can be found online at: https://www.frontiersin.org/articles/10.3389/fpls.2023.1157507/full#supplementary-material
Abbreviations
MC-QTL, multi-cross quantitative trait loci analysis; Fe, Iron/grain Iron; Zn, Zinc/grain Zinc; GY, grain yield; PH, plant height; DF/FLW, days to flowering; NT, number of tillers; PT, productive tillers; TGW, total grain weight; PL, panicle length; GL, grain length; GW, grain width; Mb, mega base pairs; ICIM, inclusive composite interval mapping; GWAS, genome-wide association study; MAF, minor allele frequency
References
Ahmed, F., Prendiville, N., Narayan, A. (2016). Micronutrient deficiencies among children and women in Bangladesh: Progress and challenges. J. Nutr. Sci. 5, e46. doi: 10.1017/jns.2016.39
Ali, M. P., McClung, A. M., Jia, M. H., Kimball, K., McCouch, S. R., Eizenga, G. C. (2011). “A rice diversity panel evaluated for genetic and agro-morphological diversity between subpopulations and its geographic distribution. Crop Sci. 51, 2021–2035. doi: 10.2135/cropsci2010.11.0641
Al-Shugeairy, Z., Islam, M. S., Shrestha, R., Al-Ogaidi, F., Norton, G. J., Price, A. H. (2014). High throughput screening of rooting depth in rice using buried herbicide. Ann. Appl. Biol. 165, 96–107. doi: 10.1111/aab.12118
Anuradha, K., Agarwal, S., Rao, Y. V., Rao, K. V., Viraktamath, B. C., Sarla, N. (2012). Mapping QTLs and candidate genes for iron and zinc concentrations in unpolished rice madhukar x swarna RILs. Gene 508, 233–240. doi: 10.1016/j.gene.2012.07.054
Bandillo, N., Raghavan, C., Muyco, P. A., Sevilla, M. A. L., Lobina, I., Dilla-Ermita, C. J., et al. (2013). Multi-parent advanced generation inter-cross (MAGIC) population in rice. progress and potential for genetics research and breeding. Rice 6 (1), 1–15. doi: 10.1186/1939-8433-6-11
Banerjee, S., Chandel, G. (2011). Understanding the role of metal homeostasis related candidate genes in Fe/Zn uptake, transport and redistribution in rice using semi-quantitative RT-PCR. Plant Mol. Biol. Biotechnol. 2, 33–46.
Biłas, R., Szafran, K., Hnatuszko-Konka, K. (2016). Cis -regulatory elements used to control gene expression in plants. Plant Cell, Tissue, and Organ Culture 127 (2), 269–287. doi: 10.1007/s11240-016-1057-7
Biswas, P.S., Swamy, B.P.M., Kader, M.A., Hossain, M.A., Boncodin, R., Samia, M., Hassan, M.L., et al (2021). Development and field evaluation of near-isogenic lines of GR2-EBRRI dhan29 golden rice. Front. Plant Sci 12: 619739. doi: 10.3389/fpls.2021.619739
Blanc, G., Charcosset, A., Mangin, B., Gallais, A., Moreau, L. (2006). Connected populations for detecting quantitative trait loci and testing for epistasis: An application in maize. Theor. Appl. Genet. 113 (2), 206–224. doi: 10.1007/s00122-006-0287-1
Bouis, H. E., Hortz, C., Claffery, M. B., Meenakshi, J. V., Wolfgang, P., Branca, F., et al. (2002). Community and international nutrition iron-biofortified rice improves the iron stores of nonanemic Filipino women 1,2. Ann. Nutr. Metab. 46 (2), 511–513. doi: 10.1177/15648265110321S105
Bouis, H. E., Saltzman, A. (2017). Improving nutrition through biofortification: A review of evidence from HarvestPlus 2003 through 2016.” Global Food Secur. 12, 49–58. doi: 10.1016/j.gfs.2017.01.009
Calayugan, M. I. C., Formantes, A. K., Amparado, A., Descalsota-Empleo, G. I., Nha, C. T., Inabangan-Asilo, M. A., et al. (2020). Genetic analysis of agronomic traits and grain iron and zinc concentrations in a doubled haploid population of rice (Oryza sativa l.). Sci. Rep. 10, 2283. doi: 10.1038/s41598-020-59184-z
Calayugan, M. I. C., Swamy, B. P. M., Nha, C. T., Palanog, A. D., Biswas, P. S., Descalsota-Empleo, G. I., et al. (2021). Zinc-Biofortified Rice: A Sustainable Food-Based Product for Fighting Zinc Malnutrition. In: Ali, J., Wani, S. H. (eds) Rice Improvement: Physiological, Molecular Breeding and Genetic Perspectives. Springer, Cham. doi: 10.1007/978-3-030-66530-2
Chadha-Mohanty, P., Rey, J., Francisco, P. B., Virk, P. S., Hossain, M. A., Swamy, B. P. M. (2015). Expression analysis of high zinc rice breeding lines using known homeostasis genes involved in iron and zinc acquisition and translocation. In P505, Plant and animal genome conference, pp. 10–14.
Chandel, G., Banerjee, S., Vasconcelos, M., Grusak, M. A. (2010). Characterization of the root transcriptome for iron and zinc homeostasis-related genes in indica rice (Oryza sativa l). J. Plant Biochem. Biotechnol. 19 (2), 1–8. doi: 10.1007/BF03263334
Chang, L. Y., Toghiani, S., Ling, A., Aggrey, S. E., Rekaya, R. (2018). High density marker panels, SNPs prioritizing and accuracy of genomic selection. BMC Genet. 19 (1), 4. doi: 10.1186/s12863-017-0595-2
Charcosset, A., Causse, M., Moreau, L., Gallais, A. (2000). Heterosis in maize investigated using connected RIL populations. In Quantitative Genet. Breed. Methods: Way Ahead, Proceedings of the Eleventh Meeting of the EUCARPIA Section Biometrics in Plant Breeding, Paris, France, pp.89–98.
Chen, X., Meyerowitz, E. M. (1999). HUA1 and HUA2 are two members of the floral homeotic AGAMOUS pathway. Mol. Cell. 3 (3), 349–360. doi: 10.1016/s1097-2765(00)80462-1
Chen, H., Xie, W., He, H., Yu, H., Chen, W., Li, J., et al. (2014). A high-density SNP genotyping array of rice biology and molecular breeding. Mol. Plant 7 (3), 541–553. doi: 10.1093/mp/ss135
Churchill, G. A., Doerge, R. W. (1994). “Empirical threshold values for quantitative trait mapping.” Genetics 138, 963–971. doi: 10.1093/genetics/138.3.963
Collard, B. C. Y., MacKill, D. J. (2007). Marker-assisted selection: An approach for precision breeding in the twentieth century. Philos. Trans. R. Soc B 363, 557–572. doi: 10.1098/rstb.2007.2170
De Oliviera, Y., Ait-Braham, L., Joets, J., Mabire, C., Negro, S., Nicolas, S., et al. (2016). BioMercator: A complete framework to integrate QTL, genome annotation and genome-wide association studies. 5ieme meeting annuel d’AMAIZING, Nov 2016, Paris, France.
Descalsota, G. I. L., Swamy, B. P. M., Zaw, H., Inabangan-Asilo, M. A. (2018). Genome-wide association mapping in a rice MAGIC plus population detects QTLs and genes useful for biofortification. Front. Plant Sci. 10. doi: 10.3389/fpls.2018.01347
Descalsota-Empleo, G. I., Amparado, A., Inabangan-Asilo, M. A., Tesoro, F., Stangoulis, J., Reinke, R., et al. (2019b). Genetic mapping of QTL for agronomic traits and grain mineral elements in rice. Crop J. 7 (4), 560–572. doi: 10.1016/j.cj.2019.03.002
Descalsota-Empleo, G. I., Noraziyah, A. A. S., Navea, I. P., Chung, C., Dwiyanti, M. S., Labios, R. J. D., et al. (2019a). Genetic disssection of grain nutritional traits and leaf blight resistance in rice. Genes 10, 30. doi: 10.3390/genes10010030
Dipti, S. S., Bergman, C., Indrasari, S. D., Herath, S., Hall, R. (2012). The potential of rice to offer solutions for malnutrition and chronic diseases. Rice 5 (1), 16. doi: 10.1186/1939-8433-5-16
Doust, A. N., Lukens, L., Olsen, K. M., Mauro-Herrera, M., Meyer, A., Rogers, K. (2014). Beyond single gene: How epistasis and gene-by-environment effects influence crop domestication. Proc. Natl. Acad. Sci. U.S.A. 111 (17), 6178–6183. doi: 10.1073/pnas.1308940110
Du, J., Zeng, D., Wang, B., Qian, Q., Zheng, S., Ling, H. Q. (2013). Environmental effects on mineral accumulation in rice grains and identification of ecological specific QTLs. Environ. Geochem Health 35, 161–170. doi: 10.1007/s10653-012-9473-z
Fan, C., Xing, Y., Mao, H., Lu, T., Han, B., Xu, C., et al. (2006). GS3, a major QTL for grain length and weight and minor QTL for grain width and thickness in rice, encodes a putative transmembrane protein. Theor. Appl. Genet. 112 (6), 1164–1171. doi: 10.1007/s00122-006-0218-1
Fedotova, A. A., Bonchuk, A. N., Mogila, V. A., Georgiev, P. G. (2017). C2H2 Zinc Finger Proteins: The Largest but Poorly Explored Family of Higher Eukaryotic Transcription Factors. Acta Naturae 9(2), 47–58.
Gaikwad, K. B., Rani, S., Kumar, M., Gupta, V., Babu, P. H., Bainsla, N. K., et al. (2020). Enhancing the nutritional quality of major food crops through conventional and genomics-assisted breeding. Front. Nutr. 7. doi: 10.3389/fnut.2020.533453
Gamuyao, R., Chin, J. H., Pariasca-Tanaka, J., Pesaresi, P., Catausan, S., Dalid, C., et al. (2012). The protein kinase Pstol1 from traditional rice confers tolerance of phosphorus deficiency. Nature 169, 1631–1638. doi: 10.1038/nature11346
Garcia-Oliveira, A. L., Chander, S., Ortiz, R., Menkir, A., Gedil, M. (2018). Genetic basis and breeding perspectives of grain iron and zinc enrichment in cereals. Front. Plant Sci. 9, 937. doi: 10.3389/fpls.2018.00937
Garcia-Oliveira, A. L., Tan, L., Fu, Y., Sun, C. (2009). Genetic identification of quantitative trait loci for contents of mineral nutrients in rice grain. J. Integr. Plant Biol. 51 (1), 84–92. doi: 10.1111/j.1744-7909.2008.00730.x
Garis, A. J., Tai, T. H., Coburn, J., Kresovich, S., McCouch, S. (2005). Genetic structure and diversity in Oryza sativa l. Genetics 169 (3), 1631–1638. doi: 10.1534/genetics.104.035642
Gaurav, J., Soe, Y. P., Palanog, A. D., Hore, T. K., Nha, C. T., Calayugan, M. I. C., et al. (in press). Meta-QTLs and haplotypes for efficient zinc biofortification of rice. Plant Genome.
Goel, A., Goha, T., Pandey, D., Gupta, S., Kumar, A. (2011). Genome-wide comparative in silico analysis of calcium transporters of rice and sorghum. Genomics Proteomics Bioinf. 9 (4-5), 138–150. doi: 10.1016/S1672-0229(11)60017-X
Goloran, J. B., Johnson-Beebout, S. E., Morete, M. J., Impa, S. M. P., Kirk, G. J. D., Wissuwa, M. (2019). Grain zn concentrations and yield of zn-biofortified versus zn-efficient rice genotypes under contrasting growth conditions. Field Crops Res. 234 (October 2018), 26–32. doi: 10.1016/j.fcr.2019.01.011
Goudia, B. D., Hash, T. C. (2015). Breeding for high grain fe and zn levels in cereals. Int. J. Innovation Appl. Stud. ISSN 12 (2), 2028–9324.
Grenier, C., Cao, T. V., Ospina, Y., Quintero, C., Chatel, M. H., Tohme, J., et al. (2015). Accuracy of genomic selection in a rice synthetic population developed for recurrent selection breeding. PloS One 10 (8), e0136594. doi: 10.1371/journal.pone.0136594
Guerinot, M. L. (2000). The ZIP family of metal transporters. Biochim. Biophys. Acta-Biomembranes 1465 (1–2), 190–198. doi: 10.1016/s0005-2736(00)00138-3
Gupta, S., Brazier, A. K. M., Lowe, M. N. (2020). Zinc deficiency in low- and middle-income countries: prevalence and approaches for mitigation. J. Hum. Nutr. Dietetics 33 (5), 624–643. doi: 10.1111/jhn.12791
Haley, C. S., Knott, S. A. (1992). A simple regression method for mapping quantitative trait loci in line crosses using flanking markers. Heredity 69, 315–324. doi: 10.1038/hdy.1992.131
Han, G., Lu, C., Guo, J., Qiao, Z., Sui, N., Qiu, N., et al. (2020). C2H2 Zinc finger proteins: Master regulators of abiotic stress responses in plants. Front. Plant Sci. 11, 115. doi: 10.3389/fpls.2020.00115
Hara, T., Takeda, T., Takagishi, T., Fukue, K., Kambe, T. (2017). Physiological roles of zinc transporters: Molecular and genetic importance of zinc homeostasis. J. Physiol. Sci. 67, 283–301. doi: 10.1007/s12576-017-0521-4
Henry, A., Gowda, V. R. P., Torres, R. O., McNally, K. L., Serraj, R. (2011). Variation in root system architecture and drought response in rice (Oryza sativa): Phenotyping of OryzaSNP panel in rainfed lowland fields. Field Crops Res. 120, 205–214. doi: 10.1016/j/fcr.21010.10.003
Hu, J., Wang, Y., Fang, Y., Zeng, L., Xu, J., Yu, H., et al. (2015). A rare allele of GS2 enhances grain size and grain yield in rice. Molecular Plant 8(10), 1455–1465. doi: 10.1016/j.molp.2015.07.002
Huggins, T. D., Chen, M. H., Fjellstrom, R. G., Jackson, A. K., McClung, A. M., Edwards, J. D. (2019). Association analysis of three diverse rice (Oryza sativa L.) germplasm collections for loci regulating grain quality traits. Plant Genome 12 (1). doi: 10.3835/plantgenome2017.09.0085
IRRI (International Rice Research Institute). (2014). Standard Evaluation System for rice (SES), 5th Edition. Los Banos (Philippines): International Rice Research Institute
Islam, M. Z., Arifuzzaman, M., Banik, S., Hossain, M. A., Ferdous, J., Khalequzzaman, M., et al. (2020). Mapping QTLs underpin nutrition components in aromatic rice germplasm. PloS One 15, 1–13. doi: 10.1371/journal.pone.0234395
Islam, M., Ontoy, J., Subudhi, P. K. (2019). Meta-analysis of quantitative trait loci associated with seedling-stage salt tolerance in rice (Oryza sative l.). Plants 8 (2), 33. doi: 10.3390/plants8020033
Jackson, M. T., Lettington, R. J. L. (2003). Conservation and use of rice germplasm: an evolving paradigm under the international treaty on plant genetic resources for food and agriculture. in Proceedings of the 20th session of the International Rice Commission, Bangkok, Thailand. 23–26.
Jagadish, S. V. K., Craufurd, P. Q., Wheeler, T. R. (2008). Phenotyping parents of mapping populations of rice for heat tolerance during anthesis. Crop Sci. 48, 1140–1146. doi: 10.2135/cropsci2007.10.0559
Jan, A., Komatsu, S. (2006). Functional characterization of gibberellin-regulated genes in rice using microarray system. Genomics Proteomics Bioinf. 4 (3), 137–144. doi: 10.1016/S1672-0229(06)60026-0
Jannink, J. L., Jansen, R. (2001). Mapping epistatic quantitative trait loci with one-dimensional genome searches. Genetics 157, 445–454. doi: 10.1093/genetics/157.1.445
Jiang, S. L., Wu, J. G., Thang, N. B., Feng, Y., Yang, X. E., Shi, C. H. (2008). Genotypic variation of mineral elements contents in rice (Oryza sativa l.). Eur. Food Res. Technol. 228 (1), 115–122. doi: 10.1007/s00217-008-0914-y
Jourjon, M. F., Jasson, S., Marcel, J., Ngom, B., Mangin, B. (2005). MCQTL: Multi-allelic QTL mapping in multi-cross design. Bioinformatics 21, 128–130. doi: 10.1093/bioinformatics/bth481
Khush, G. S. (1997). Origin, dispersal, cultivation and variation of rice. Plant Mol. Biol. 35 (1-2), 25–34. doi: 10.1007/978-94-011-5794-0_3
Lee, S., Joong, H. J., Sun, A. K., Lee, J., Guerinot, M. L., An, G. (2010). OsZIP5 is a plasma membrane zinc transporter in rice. Plant Mol. Biol. 73 (4-5), 507–517. doi: 10.1007/s11103-010-9637-0
Lekklar, C., Pongpanich, M., Suriya-Arunroj, D., Chinpongpanich, A., Tsai, H., Comai, L., et al. (2019). Genome-wide association study for salinity tolerance at the flowering stage in a panel of rice accessions from Thailand. BMC Genomics 20, 76. doi: 10.1186/s12846-018-5317-2
Li, R., Li, M., Ashraf, U., Liu, S., Zhang, J. (2019). Exploring the relationship between yield and yield-related traits for rice varieties released in China from 1978 to 2017. Front. Plant Sci. 10, 543. doi: 10.1007/s001220000528
Li, M., Li, X., Zhou, Z., Wu, P., Fang, M., Pan, X., et al. (2016). Reassessment of the four yield-related genes Gn1a, DEP1, GS3, and IPA1 in rice using a CRISPR/Cas9 system. Front. Plant Sci. 7. doi: 10.3389/fpls.2016.00377
Lu, K., Li, L., Zheng, X., Zhang, Z., Mou, Y., Hu, Z. (2008). Quantitative trait loci controlling Cu Ca zn Mn, and fe content in rice grains. J. Genet. 87, 305–310. doi: 10.1007/s12041-008-0049-8
Ludwig, Y., Slamet-Loedin, I. H. (2019). Genetic biofortification to enrich rice and wheat grain iron: From genes to product. Front. Plant Sci. 10. doi: 10.3389/fpls.2019.00833
MacKill, D. J., Khush, G. S. (2018). IR64: A high-quality and high-yielding mega variety. Rice 11, 18. doi: 10.1186/s12284-18-0208-3
Malik, P., Huang, M., Neelam, K., Bhatia, D., Ramanjeet, K., Yadav, B., et al. (2022). Genotyping-by-sequencing based investigation of population structure and genome wide association studies for seven agronomically important traits in a set of 346 oryza rufipogon accessions. Rice 15, 37. doi: 10.1186/s12284-022-00582-4
Mansueto, L., Fuentes, R. R., Borja, F. K., Detras, J., Abriol-Santos, J. M., Chebotarov, D., et al. (2017). ). rice SNP-seek database update: New SNPs, indels, and queries. Nucleic Acids Res. 45, D1075–D1081. doi: 10.1093/nar/gkw1135
Morales, K. Y., Singh, N., Perez, F. A., Ignacio, J. C., Thapa, R., Arbalaez, J. D., et al. (2020). An improved 7K SNP array, the C7AIR, provides wealth of validated SNP markers for rice breeding and genetic studies. PloS One 15 (5), e0232479. doi: 10.1371/journal.pone.0232479
Murray, M. G., Thompson, W. F. (1980). Rapid isolation of high molecular weight plant DNA.” Nucleic Acids Res. 18 (12), 4321–4326. doi: 10.1093/nar/8.19.4321
Mwanga, R. O. M., Swanckaert, J., da Silva Pereira, G., ndrade, M. I., Makunde, G., Grüneberg, W. J., et al. (2021). Breeding progress for vitamin a, iron and zinc biofortification, drought tolerance, and sweetpotato virus disease resistance in sweetpotato. Front. Sustain. Food Syst. 5. doi: 10.3389/fsufs.2021.616674
Norton, G. J., Deacon, C. M., Xiong, L., Huang, S., Meharg, A. A., Price, A. H. (2010). Genetic mapping of the rice ionome in leaves and grain: Identification of QTLs for 17 elements including arsenic cadmium iron and selenium. Plant Soil 329, 139–153. doi: 10.1007/s11104-009-0141-8
Norton, G. J., Travis, A. J., Douglas, A., Fairley, S., Alves, E. P., Ruang-Areerate, P., et al. (2018). Genome wide association mapping of grain and straw biomass traits in the rice Bengal and Assam aus panel (BAAP) grown under alternate wetting and drying and permanently flooded irrigation. Front. Plant Sci. 9. doi: 10.3389/fpls.2018.01223
Palanog, A. D., Calayugan, M. I. C., Descalsota-Empleo, G. I., Amparado, A., Inabangan-Asilo, M. A., Arocena, E., et al. (2019). Zinc and iron status in the Philippines population and local soils. Front. Nutr. 6. doi: 10.3389/fnut.2019.00081
Pandey, S., Reddy, C. S., Yaqoob, U., Negi, Y. K., Arora, S., Kaul, T. (2015). Insilico analysis of cis acting regulatory elements CAREs in upstream regions of ascorbate glutathione pathway genes from oryza sativa. Biochem. Physiol. 4 (2), 159. doi: 10.4172/2168-9652.1000159
Papierniak, A., Kozak, K., Kendziorek, M., Barabasz, A., Palusinka, M., Tiuryn, et al. (2018). Contribution of NtZIP1-like to the regulation of zn homeostasis. Front. Plant Sci. 9. doi: 10.3389/fpls.2018.00185
Pradhan, S. K., Pandit, E., Pawar, S., Naveenkumar, R., Barik, S. R., Nayak, D. K., et al. (2020). Linkage disequilibrium mapping for grain fe and zn enhancing QTLs useful for nutrient dense rice breeding. BMC Plant Biol. 20, 57. doi: 10.1186/s12870-020-2262-4
Rakotondramana, M., Tanaka, R., Pariaska-Tanaka, J., Stangoulis, J., Grenier, C., Wissuwa, M. (2022). Genomic prediction of zinc-biofortification potential in rice gene bank accessions. Theor. Appl. Genet. 135, 2265–2278. doi: 10.1007/s00122-022-04110-2
Redoña, E. D., Mackill, D. J. (1996). Genetic variation for seedling vigor traits in rice. Crop Sci. 36 (2), 285–290. doi: 10.2135/cropsci1996.011183X00360002001x
Reyes, V. P., Kitony, J. K., Nishiuchi, S., Makihara, D., Doi, K. (2022). Utilization of genotyping-by-Sequencing (GBS) for rice pre-breeding and improvement: A review. Life 12, 1752. doi: 10.3390/life12111752
RStudio Team (2015). RStudio: Integrated Development Environment for R. Boston, MA. Availabl at: http://www.rstudio.com/
Sevanthi, A. M., Sinha, S. K., Sureshkumar, V., Rani, M., Saini, M. R., Kumari, S., et al. (2021). Integration of dual stress transcriptomes and major QTLs from a pair of genotypes contrasting for drought and chronic nitrogen starvation identifies key stress responsive genes in rice. Rice (N Y) 14, 49. doi: 10.1186/s12284-021-00487-8
Specht, J. E., Chase, K., Macrander, M., Graef, G. L., Chung, J., Maxwell, J. P., et al. (2001). Soybean response to water: A QTL analysis of drought tolerance. Crop Sci. 41, 493–509. doi: 10.1235/cropsci2001.412493x
Srivastava, S. K., Wolinski, P., Pereira, A. (2014). A strategy for genome-wide identification of gene based polymorphisms in rice reveals non-synonymous variation and functional genotypic markers. PloS One 9 (9), e105335. doi: 10.1371/journal.pone.0105335
Stangoulis, J. C. R., Knez, M. (2022). Biofortification of major crop plants with iron and zinc - achievements and future directions. Plant Soil 474, 57–76. doi: 10.1007/s11104-022-05330-7
Swamy, B. P. M., Descalsota, G. I. L., Nha, C. T., Amparado, A., Inabangan-Asilo, M. A., Manito, C., et al. (2018). Identification of genomic regions associated with agronomic and biofortification traits in DH populations of rice. PloS One 13 (8), 1–20. doi: 10.1371/journal.pone.0201756
Swamy, B. P. M., Marathi, B., Ribeiro-Barros, A. I. F., Ricachenevsky, F. P. (2021a). Editorial: Development of healthy and nutritious cereals: Recent insights on molecular advances in breeding. Front. Genet. 12. doi: 10.3389/fgene.2021.635006
Swamy, B. P. M., Marunadan, S., Jr., Samia, M., Ordonio, R. L., Ribong, D. B., Miranda, R., et al. (2021b). Development and characterization of GR2E golden rice introgression lines. Sci. Rep. 11, 2496. doi: 10.1038/s41598-021-82001-0
Swamy, B. P. M., Rahman, M. A., Inabangan-Asilo, M. A., Amparado, A., Manito, C., Chadha-Mohanty, P., et al. (2016). Advances in breeding for high grain zinc in rice. Rice 9, 49. doi: 10.1186/s12284-016-0122-5
Tang, W., Wu, T., Ye, J., Sun, J., Jiang, Y., Yu, J., et al. (2016). SNP-based analysis of genetic diversity reveals important alleles associated with seed size in rice. BMC Plant Biol. 16, 93. doi: 10.1186/s12870-016-0779-3
The 3,000 rice genome project (2014). The 3,000 rice genome project. GigaSci 3, 7. doi: 10.1186/2047-217X-3-7
Travis, A. J., Norton, G. J., Datta, S., Sarma, R., Dasgupta, R., Savio, F. L., et al. (2015). assessing the genetic diversity of rice originating from bangladesh, assam and west bengal. Rice 8, 35. doi: 10.1186/s12284-015-0068-z
Tsakirpaloglou, N., Swamy, B. P. M., Acuin, C., Slamet-Loedin, I. H. (2019). “Biofortified zn and fe rice: potential contribution for dietary mineral and human health,” in Nutritional quality improvement in plants. concepts and strategies in plant sciences. Eds. Jaiwal, P., Chhilar, A., Chaudhary, D., Jaiwal, R. (Cham: Springer). doi: 10.1007/978-3-319-95354-0-1
Varshney, R. K., Bohra, A., Yu, J., Graner, A., Zhang, Q., Sorrells, M. (2021). Designing future crops; genomics-assisted breeding comes of age. Trends Plant Sci. 26 (6), 631–649. doi: 10.1016/j.tplants.2021.03.010
Verdeprado, H., Kretzschmar, T., Begum, H., Raghavan, C., Joyce, P., Lakshmanan, P., et al. (2018). Association mapping in rice: Basic concepts and perspectives for molecular breeding. Plant Production Sci. 21 (3), 159–176. doi: 10.1080/1343943X.2018.1483205
Wang, J. (2009). Inclusive composite interval mapping of quantitative trait genes. Acta Agronomica Sin. 35 (2), 239–245. doi: 10.3724/SP.J.1006.2009.00239
Wang, W., Mauleon, R., Hu, Z., Chebatarov, D., Tai, S., Wu, Z., et al. (2018). Genomic variation in 3,010 diverse accessions of Asian cultivated rice. Nat. Publishing Group 557, 43–49. doi: 10.1038/s41586-018-0063-9
Wang, X., Pang, Y., Zhang, J., Zhang, Q., Tao, Y., Feng, B., et al. (2014). Genetic background effects on QTL and QTL x environment interaction for yield and its component traits as revealed by reciprocal introgression lines in rice. Crop J. 2 (6), 345–357. doi: 10.1016/j.cj.2014.06.004
Wei, X., JunFeng, X., Ling, X., Lun, W. H., Ling, Z., Qu, Z., et al. (2012). Genetic analysis for the diversity of heading date of cultivated rice in China. Acta Agron. Sin. 38(1), 10–22.
Welch, R. M. (2004). Breeding strategies for biofortified staple plant food to reduce micronutrient malnutrition globally. J. Nutr. 132 (3), 495s–499s. doi: 10.1093/jn/132.3.495S
Widodo, B., Broadley, M. R., Rose, T., Frei, M., Pariasca-Tanaka, J., Yoshihashi, T., et al. (2010). Response to zinc deficiency of two rice lines with contrasting tolerance is determined by root growth maintenance and organic acid exudation rates, and not by zinc-transporter activity. New Phytol. 186 (2), 400–414. doi: 10.1111/j.1469-8137.2009.031777.x
Wu, W., Cheng, Z., Liu, M., Yang, X., Qiu, D. (2014). C3HC4-type RING finger protein NbZFP1 is involved in growth and fruit development in Nicotiana benthamiana. PLoS One 9(6), e99352. doi: 10.1371/journal.pone.0099352
Xiang, J., Lin, J., Tang, D., Zhou, B., Guo, M., He, R., et al. (2010). A DHHC-type zinc finger protein gene regulates shoot branching in Arabidopsis. Afr. J. Biotechnol. 9(45), 7759–7766. doi: 10.5897/AJB10.650
Xu, J., Xing, Y., Xu, Y., Wan, J. (2021). Breeding by design for future rice: Genes and genome technologies. Crop J. 9, 491–496. doi: 10.1016/j.cj.2021.05.001
Xu, Y., Li, P., Yang, Z., Xu, C. (2016). Genetic mapping of quantitative trait loci in crops. Crop J. 5 (2), 175–184. doi: 10.1016/j.cj.2016.06.003
Xu, K., Xu, X., Fukao, T., Canlas, P., Maghirang-Rodriguez, R., Heuer, S., et al. (2006). Sub1A is an ethylene-response-factor-like gene that confers submergence tolerance to rice. Nature 442, 705–708. doi: 10.1038/nature04920
Xu, Q., Zheng, T. Q., Hu, X., Cheng, L. R., Xu, J. L., Shi, Y. M., et al. (2015). Examining two sets of introgression lines in rice (Oryza sativa l) reveals favorable alleles that improve grain zn and fe concentrations. PloS One 10 (7), 1–20. doi: 10.1371/journal.pone.0131846
Yadaw, R. B., Khatiwada, S. P., Chaudhary, B., Adhikari, N. P., Baniya, B., Mudwari, A., et al. (2006). Participatory Varietal Selection (PVS) of rice varieties for rain fed conditions. International Rice Research Institute (IRRI).
Yang, M., Li, Y., Liu, Z., Tian, J., Liang, L., Qiu, Y., et al. (2020). A high activity zinc transporter OsZIP9 mediates zinc uptake in rice. Plant J. 103(5), 1695–1709. doi: 10.1111/tpj.14855
Yang, Y., Chou, H. L., Crofts, A. J., Zhang, L., Tian, L., Washida, H., et al. (2018). Selective sets of MRNAs localize to extracellular paramural bodies in a rice Glup6 mutant. J. Exp. Bot. 69 (21), 5045–5058. doi: 10.1093/jxb/ery297
Yang, X., Ma, K., Zhao, Y., Wang, X., Zhou, K., Yu, G., et al. (2021). Genomic selection: A breakthrough technology in rice breeding. Crop J. 9 (3), 669–677. doi: 10.1016/j.cj.2021.03.008
Yin, X., Liu, X., Xu, B., Liu, P., Dong, T., Yang, D., et al. (2019). OsMADS18, a membrane-bound MADS-box transcription factor, modulates plant architecture and the abscisic acid response in rice. J. Exp. Bot. 70 (15), 3895–3909. doi: 10.1093/jxb/erz198
Yoo, J., Cao, X., Zhou, Q., Ma, L. Q. (2006). Accumulation of Pb, Cu, and zn in native plants growing on a contaminated Florida site. Sci. Total Environ. 368 (2-3), 456–464. doi: 10.1016/j.scitoenv.2006.01.016
Yu, X., Yuan, S., Tao, X., Huang, J., Yang, G., Deng, Z., et al. (2021). Comparison between main and ratoon crops in resources use efficiencies, environmental impacts, economic profits of rice ratooning system in central China. Science of the Total Environment 799, 149246. doi: 10.1016/j.scitotenv.2021.149246
Zhang, H., Liu, Y., Wen, F., Yao, D., Wang, L., Guo, J., et al. (2019). A novel rice C2H2-type zinc finger protein, ZFP36, is a key player involved in abscisic acid-induced antioxidant defence and oxidative stress tolerance in rice.” J. Exp. Bot. 65 (20), 1–2. doi: 10.1093/jxb/eru313
Zhang, M., Shannon, R., Pinson, M., Tarpley, L., Huang, X. Y., Lahner, B., et al. (2014). Mapping and validation of quantitative trait loci associated with concentrations of 16 elements in unmilled rice grain. Theor. Appl. Genet. 127 (1), 137–655. doi: 10.1007/s00122-013-2207-5
Zhao, F. M., Zhang, G. Q., Zeng, R. Z., Yang, Z. L., Ling, Y. H., Sang, X. C., et al. (2011). Analysis of epistatic and additive effects of QTLs for grain shape using single segment substitution lines in rice (Oryza sativa l.). Acta Agron. Sin. 37, 469–476. doi: 10.3724/SP.J.1006.2011.00469
Keywords: rice, biofortification, micronutrients, Zn, QTL, candidate genes, connected populations
Citation: Palanog AD, Nha CT, Descalsota-Empleo GIL, Calayugan MI, Swe ZM, Amparado A, Inabangan-Asilo MA, Hernandez JE, Sta. Cruz PC, Borromeo TH, Lalusin AG, Mauleon R, McNally KL and Swamy BPM (2023) Molecular dissection of connected rice populations revealed important genomic regions for agronomic and biofortification traits. Front. Plant Sci. 14:1157507. doi: 10.3389/fpls.2023.1157507
Received: 02 February 2023; Accepted: 20 February 2023;
Published: 22 March 2023.
Edited by:
Shan Li, Nanjing Agricultural University, ChinaReviewed by:
Ye Yafeng, Hefei Institutes of Physical Science (CAS), ChinaHui Liu, Institute of Genetics and Developmental Biology (CAS), China
Copyright © 2023 Palanog, Nha, Descalsota-Empleo, Calayugan, Swe, Amparado, Inabangan-Asilo, Hernandez, Sta. Cruz, Borromeo, Lalusin, Mauleon, McNally and Swamy. This is an open-access article distributed under the terms of the Creative Commons Attribution License (CC BY). The use, distribution or reproduction in other forums is permitted, provided the original author(s) and the copyright owner(s) are credited and that the original publication in this journal is cited, in accordance with accepted academic practice. No use, distribution or reproduction is permitted which does not comply with these terms.
*Correspondence: B. P. Mallikarjuna Swamy, bS5zd2FteUBpcnJpLm9yZw==