- 1College of Artificial Intelligence, Nanjing Agricultural University, Nanjing, China
- 2School of Engineering, College of Science, University of Lincoln, Lincoln, United Kingdom
- 3National Engineering and Technology Center for Information Agriculture (NETCIA), Nanjing Agricultural University, Nanjing, China
- 4College of Horticulture, Nanjing Agricultural University, Nanjing, Jiangsu, China
- 5Lincoln Institute for Agri-food Technology, University of Lincoln, Lincoln, United Kingdom
- 6College of Engineering, Nanjing Agricultural University, Nanjing, Jiangsu, China
1 Introduction
Chrysanthemums, which originated from China, have an economic value in flower as well as a high value in both edibility and health care in the form of food and tea (Li et al., 2019). With the improved living standards of people, chrysanthemum tea has become a popular drinking target, due to its inherent advantages: 1) it improves the body’s anti-ageing, anti-hypertensive, anti-bacterial, and anti-viral abilities; 2) it regulate the body’s immunity by anti-inflammatory, antipyretic, sedative, and anti-arthritic abilities (Han et al., 2019; Yuan et al., 2019). Hence, the planting area of tea chrysanthemums in China is increasing every year. According to the recent survey (Yang et al., 2018), the planting area in Hangbaiju in Tongxiang City, Zhejiang Province, increased to nearly 4000 hm2 with an output of about 12000 kg/hm2. Due to its best medicinal properties associated with a specific flowering stage, the harvesting period is very narrow and usually lasts 25 days (Yang et al., 2018). In this regard, there is an urgent need for many farmers to harvest a good quality and quantity of tea chrysanthemums. However, it is difficult to recruit many well-trained farmers in a short time.
During tea chrysanthemums harvesting, the harvesters judge whether to pick or not after observing the state of the tea chrysanthemums flowers. Similarly, the harvestable flowers can be detected by image processing technology, then the picking operation can be completed by the corresponding machines, such as a fruit-picking robot. However, there are many challenges to accurately identifying chrysanthemums in the field due to complex external environmental factors (light, shade, wind, and photo distance, etc.) as well as differences in maturity, colour, and the direction of chrysanthemums’ flower heads. Many researchers have identified chrysanthemums by overcoming some of the above (Yang et al., 2018; Yuan et al., 2018; Liu et al., 2019; Yang et al., 2019; Liu et al., 2020; Qi et al., 2021; Qi et al., 2022a; Qi et al., 2022b), and these studies indicate that:
1) Chrysanthemum flower detection can be realized by machine learning models, which are highly dependent on the quantity and quality of image datasets;
2) The accuracy of flower detection based on RGB images is high, and most of the detection is tested in an ideal environment.
Due to a large number of varieties of tea chrysanthemums, high planting density, and different field conditions, it is difficult to meet the photo quality requirements using the same collection parameters in a complex outdoor environment. In addition, the quality of the images collected is significantly affected by outdoor conditions; hence, the following four factors need to be considered simultaneously when taking photos of tea chrysanthemums (as illustrated in Figure 1, the serial number of the images in the dataset is provided),
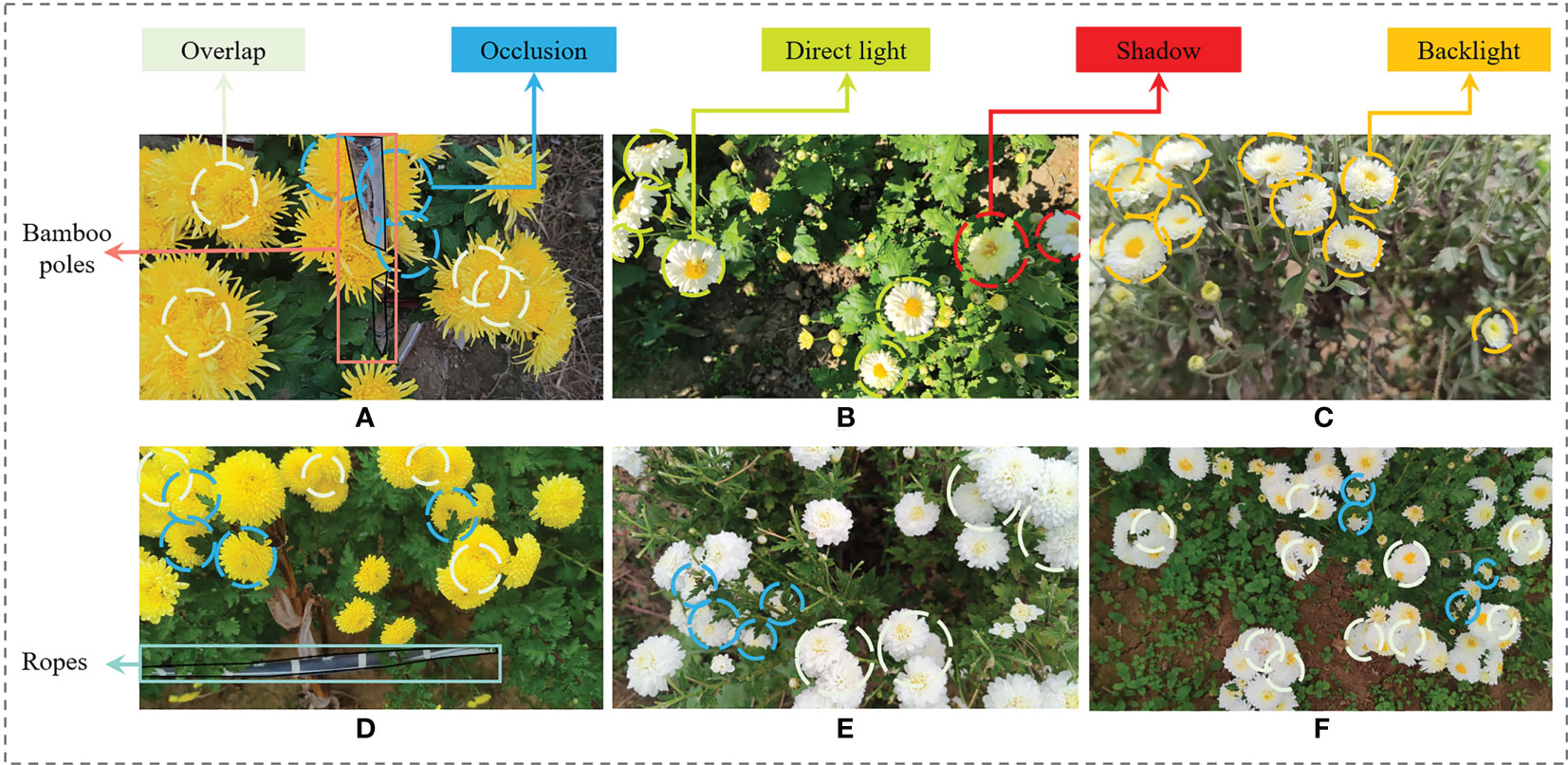
Figure 1 The category to which the sample images belong and their number in the related chrysanthemum dataset: (A) Jinsihuangju-01314, (B) Hangbaiju-00063, (C) Bo-chrysanthemum-11093, (D) Wuyuanhuangju-03285, (E) Gongju-00002, and (F) Chuju-00110.
(1) Variety: Different varieties of tea chrysanthemums varies greatly in size, especially the size difference between the Jinsihuangju (Figure 1A) and Hangbaiju (Figure 1B). If the images are collected by the camera at the same distance, the number of flowers contained in one image will exhibit considerable diversity, further leading to unbalanced data which will affect the precision of the training models. Therefore, the distance to the flower should be adjusted between 30cm to 50cm according to the variety.
(2) Planting density: As the planting density of tea chrysanthemums is relatively high, which generally results in significant overlap (The cyan circle in Figure 1) and occlusion between flowers (The blue circle in Figure 1), can occur when the images are collected in the fixed angle, which can reduce the precision of detection models. To avoid these problems, three camera views, including ∼0°, ∼45°, and ∼90° , are chosen for image collection in the field.
(3) Field conditions: Tea chrysanthemums are planted in different regions under diverse field conditions. For Jinsihuangju, Wuyuanhuangju, and Gongju, a set of ropes and bamboo poles are used on both sides of tea chrysanthemums plants to avoid lodging. However, both bamboo poles (Figure 1A) and ropes (Figure 1D) in the images will influence the detection precision, which can also be solved by taking photos in the above three views.
(4) Photographing conditions: A large number of occlusions, overlap, direct light (The green circle in Figure 1), shadow (the red circle in Figure 1), and backlight (The orange circle in Figure 1) images are collected under the photographing conditions, such as wind and the light intensity changes. To reduce these external factor influences on image quality, a large number of images are necessary to detection models (Qi et al., 2022b).
There is currently no publicly available tea chrysanthemum dataset to the authors’ knowledge. Consequently, we provide an image dataset for six varieties of tea chrysanthemums in three camera view angles obtained under complex outdoor scenes, and this open-source image dataset can greatly promote the development of tea chrysanthemums detection methodology.
2 Value of the data
(1) RGB-based images of tea chrysanthemums in three view angles can provide sufficient flower features for detection models. This will further increase the detection precision while facing the overlap, occlusion, and shadow in complex outdoor scenes.
(2) Collecting large quantities of tea chrysanthemums images according to five different outdoor conditions, including occlusion, overlap, direct light, backlight, and shadow in complex outdoor scenes, are of great benefit for extending the applicability and enabling better precision of detection models.
3 Materials and methods
3.1 Collection and construction of the dataset
3.1.1 Image acquisition
With the continuous development of imaging technology, smartphones have become important media equipment for common image and video acquisition and their usage has higher flexibility. In this work, as illustrated in Figure 2B, the Mi 10 phone (Manufacturer: Xiaomi Corporation) is used for acquiring both images and videos, and more images can be extracted from the videos to enrich the image dataset. The videos are also included in the image dataset.
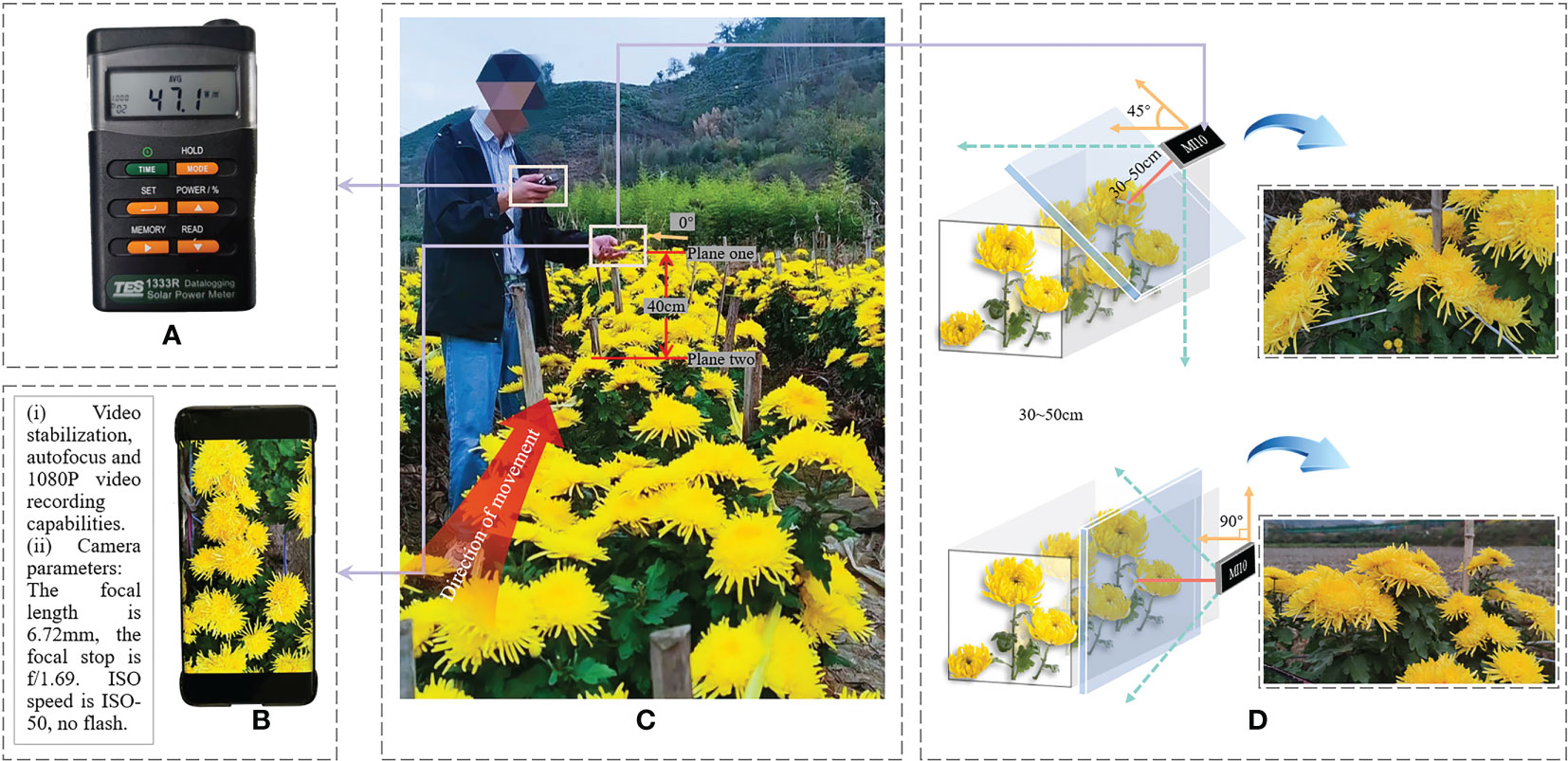
Figure 2 (A) TES-1333R Solar Power Meter, (B) Mi 10 phone, and (C) Image acquisition scene, (D) Schematic diagram of the camera angles.
3.1.2 Collection method
In Figure 2, the author took Jinsihuangju images at 4:00 p.m. in Xitou Village, She County, Huangshan City, Anhui Province, China. The TES-1333R Solar Power Meter (Figure 2A) in average mode was used to measure the average radiant illuminance before photography, describing the effect of lighting conditions on the photography, with the Solar Power Meter showing an average outdoor radiant illuminance of 47.1W/m2 at that time. Firstly, the Mi10 phone (Figure 2B) was held in the left hand and moved along the tea chrysanthemum (in the direction of the orange arrow in Figure 2C). Secondly, an angle of ∼0°, was selected for photography in Figure 2C, with a distance of 40cm between plane one, where the phone camera is located, and plane two, where the top canopy of the tea chrysanthemums is located. Since tea chrysanthemums heads are positioned differently, we used different angles for shooting that is better than a fixed angle. This will further acquire a large number of tea chrysanthemums images in different directions and reduce the noise from occlusion and overlap.
Therefore, to better display the complexity of the actual outdoor scenes, we used the following three angles, and the angles are not strictly fixed to fit the different orientations of photography.
•∼0°: The plane one, where the Mi 10 phone is located, is parallel to plane two, where the top canopy of the tea chrysanthemums is located, with an angle of ∼0° above the horizontal of the tea chrysanthemum.
•∼45°: The plane one, where the Mi 10 phone is located, is parallel to plane two, where the oblique side canopy of the tea chrysanthemums is located, with an angle of ∼45° above the horizontal of the tea chrysanthemum.
•∼90°: The plane one, where the Mi 10 phone is located, is parallel to plane two, where the lateral canopy of the tea chrysanthemums is located, with an angle of ∼90° above the horizontal of the tea chrysanthemum.
In addition, depending on whether the tea chrysanthemum planting density is high, it is necessary to choose another angle and shoot again for the same plants.
3.2 Image annotation and dataset production
As depicted in Table 1, the data on six varieties of tea chrysanthemums are collected in the form of images and videos, better displaying the complexity of the outdoor scenes. Taking the Bo-chrysanthemum as an example, the 221 images and videos with a total duration of 67 min were collected at Yaowang Village on 24 September 2022. Then FFmpeg (Get URL: https://ffmpeg.org/) was used to capture an image every 20 frames of the above video, and the resolution of all the captured images and the 221 images were adjusted into 1080x1920, all of which were stored in JPG format. Finally, the above-processed images were selected manually to delete the obscure images, leaving 11592 Bo-chrysanthemum images in the dataset, of which 221 images were reserved after the above processing step.
Qi et al. (2022b) showed that up to 3000 images are sufficient to train and achieve better detection precision in the TC-YOLO model. Thus, in the dataset, the number of labelled images for each type of tea chrysanthemum was limited to 3000. The labelling software used was LabelImg (Get URL: https://github.com/heartexlabs/labelImg), which was used to annotate six tea chrysanthemums, labelling 18,000 images in total and saving the result of each image as an XML file.
In summary, we present an image dataset of six types of tea chrysanthemums (Bo-chrysanthemum, Hangbaiju, Jinsihuangju, Wuyuanhuangju, Gongju, and Chuju), a total of 81,276 images (1080×1920 pixels), captured using Mi10 phone. The image dataset was collected under five difficult-to-identify complex outdoor conditions: (1) direct light, (2) backlight, (3) shadow, (4) occlusion, and (5) overlap. Besides, this dataset also provides 453 original images (5760×3240 pixels) and videos (1080P and 60FPS) of tea chrysanthemums, which enables other researchers to use these datasets for further image analyses.
4 Direct link to deposited data and information to users
Publicly available datasets were contributed in this study. This data can be found at: https://dx.doi.org/10.21227/vc18-rv06.
Data availability statement
The original contributions presented in the study are included in the article/supplementary material. Further inquiries can be directed to the corresponding author.
Author contributions
SZ, LS, RH, ZG, and XW designed the research. SZ conducted the experiment. SZ and KH analyzed the data. SZ, KH, LS, and RV wrote the paper and revised it. SZ, JB, YZ, and YC labeled the data based on the images. All authors contributed to the article and approved the submitted version.
Funding
This work was supported in part by High-end Foreign Experts Recruitment Plan of the Ministry of Science and Technology China under Grant G2021145009L, in part by Jiangsu Modern Agricultural Machinery Equipment and Technology Demonstration and Promotion Project under Grant NJ2021-11.
Conflict of interest
The authors declare that the research was conducted in the absence of any commercial or financial relationships that could be construed as a potential conflict of interest.
Publisher’s note
All claims expressed in this article are solely those of the authors and do not necessarily represent those of their affiliated organizations, or those of the publisher, the editors and the reviewers. Any product that may be evaluated in this article, or claim that may be made by its manufacturer, is not guaranteed or endorsed by the publisher.
References
Han, A.-R., Nam, B., Kim, B.-R., Lee, K.-C., Song, B.-S., Kim, S. H., et al. (2019). Phytochemical composition and antioxidant activities of two different color chrysanthemum flower teas. Molecules 24, 329. doi: 10.3390/molecules24020329
Li, Y., Yang, P., Luo, Y., Gao, B., Sun, J., Lu, W., et al. (2019). Chemical compositions of chrysanthemum teas and their anti-inflammatory and antioxidant properties. Food Chem. 286, 8–16. doi: 10.1016/j.foodchem.2019.02.013
Liu, C., Lu, W., Gao, B., Kimura, H., Li, Y., Wang, J. (2020). Rapid identification of chrysanthemum teas by computer vision and deep learning. Food Sci. Nutr. 8, 1968–1977. doi: 10.1002/fsn3.1484
Liu, Z., Wang, J., Tian, Y., Dai, S. (2019). Deep learning for image-based large-flowered chrysanthemum cultivar recognition. Plant Methods 15, 146. doi: 10.1186/s13007-019-0532-7
Qi, C., Gao, J., Chen, K., Shu, L., Pearson, S. (2022a). Tea chrysanthemum detection by leveraging generative adversarial networks and edge computing. Front. Plant Sci. 13. doi: 10.3389/fpls.2022.850606
Qi, C., Gao, J., Chen, K., Shu, L., Pearson, S. (2022b). Tea chrysanthemum detection under unstructured environments using the tc-yolo model. Expert Syst. Appl. 193, 116473. doi: 10.1016/j.eswa.2021.116473
Qi, C., Nyalala, I., Chen, K. (2021). Detecting the early flowering stage of tea chrysanthemum using the f-yolo model. Agronomy 11, 834. doi: 10.3390/agronomy11050834
Yang, Q., Chun, C., Bao, G., Fan, J., Xun, Y. (2018). Recognition and localization system of the robot for harvesting hangzhou white chrysanthemums. Int. J. Agric. Biol. Eng. 11, 88–95. doi: 10.25165/j.ijabe.20181101.3683
Yang, Q., Luo, S., Chang, C., Xun, Y., Bao, G. (2019). Segmentation algorithm for hangzhou white chrysanthemums based on least squares support vector machine. Int. J. Agric. Biol. Eng. 12, 127–134. doi: 10.25165/j.ijabe.20191204.4584
Yuan, Q., Fu, Y., Xiang, P., Zhao, L., Wang, S., Zhang, Q., et al. (2019). Structural characterization, antioxidant activity, and antiglycation activity of polysaccharides from different chrysanthemum teas. RSC Adv. 9, 35443–35451. doi: 10.1039/C9RA05820F
Keywords: tea chrysanthemum, outdoor scenes, image data, video data, unfixed angle
Citation: Zang S, Shu L, Huang K, Guan Z, Han R, Valluru R, Wang X, Bao J, Zheng Y and Chen Y (2023) Image dataset of tea chrysanthemums in complex outdoor scenes. Front. Plant Sci. 14:1134911. doi: 10.3389/fpls.2023.1134911
Received: 31 December 2022; Accepted: 29 March 2023;
Published: 14 April 2023.
Edited by:
Ning Yang, Jiangsu University, ChinaReviewed by:
Rui Xu, University of Georgia, United StatesZou Tengyue, Fujian Agriculture and Forestry University, China
Lei Zhou, Nanjing Forestry University, China
Copyright © 2023 Zang, Shu, Huang, Guan, Han, Valluru, Wang, Bao, Zheng and Chen. This is an open-access article distributed under the terms of the Creative Commons Attribution License (CC BY). The use, distribution or reproduction in other forums is permitted, provided the original author(s) and the copyright owner(s) are credited and that the original publication in this journal is cited, in accordance with accepted academic practice. No use, distribution or reproduction is permitted which does not comply with these terms.
*Correspondence: Lei Shu, lei.shu@njau.edu.cn