- Irrigation Department, Centro de Edafología y Biología Aplicada del Segura (CEBAS-CSIC), Campus de Espinardo, Murcia, Spain
The objective of this work was to validate the trunk water potential (Ψtrunk), using emerged microtensiometer devices, as a potential biosensor to ascertain plant water status in field-grown nectarine trees. During the summer of 2022, trees were subjected to different irrigation protocols based on maximum allowed depletion (MAD), automatically managed by real-time soil water content values measured by capacitance probes. Three percentages of depletion of available soil water (α) were imposed: (i) α=10% (MAD=27.5%); (ii) α=50% (MAD=21.5%); and (iii) α=100%, no-irrigation until Ψstem reached -2.0 MPa. Thereafter, irrigation was recovered to the maximum water requirement of the crop. Seasonal and diurnal patterns of indicators of water status in the soil-plant-atmosphere continuum (SPAC) were characterised, including air and soil water potentials, pressure chamber-derived stem (Ψstem) and leaf (Ψleaf) water potentials, and leaf gas exchange, together with Ψtrunk. Continuous measurements of Ψtrunk served as a promising indicator to determine plant water status. There was a strong linear relationship between Ψtrunk vs. Ψstem (R2 = 0.86, p<0.001), while it was not significant between Ψtrunk vs. Ψleaf (R2 = 0.37, p>0.05). A mean gradient of 0.3 and 1.8 MPa was observed between Ψtrunk vs.Ψstem and Ψleaf, respectively. In addition, Ψtrunk was the best matched to the soil matric potential. The main finding of this work points to the potential use of trunk microtensiometer as a valuable biosensor for monitoring the water status of nectarine trees. Also, trunk water potential agreed with the automated soil-based irrigation protocols implemented.
1. Introduction
World production of peaches and nectarines (Prunus persica L. Batsch) has increased steadily over the last decade, ranging from 20.53 to 24.56 million metric tons (Mt) in 2010, and 2020, respectively. China alone accounts for over 45% of world peach and nectarine production, also leading in harvested area. Meanwhile, Spain leads the commercial production of peach and nectarine in the Mediterranean basin (followed by Italy), with an average of 11.58 Mt year-1 in the period 2015–2020 (FAOSTAST, 2022).
Water availability set the upper limit of yield productivity which is the main economic concern for growers worldwide (Fereres and Soriano, 2007). Irrigated crops are exposed to different environmental stresses during their growth and development, with drought being the most severe stress that negatively affects plant productivity (Katerji et al., 2008). The effects of drought are aggravated in arid and semi-arid areas, such as the Mediterranean region, due to the alarming depletion of water resources and the increasing demand for food due to population growth (Varela-Ortega et al., 2016; Fernández-García et al., 2020). In addition, the COVID-19 pandemic put a strain on food supply chains worldwide, so urgent and ambitious actions are needed to build more resilient agricultural systems to maximise irrigation water productivity (FAO, 2021).
Drip irrigation is probably the most important and widespread irrigation technique for improving water use efficiency, as it allows optimal use of both water and fertiliser, since they are applied directly to the root system through low-flow emitters (Burt and Styles, 2007). Another advance has been the incorporation of drip irrigation into precise irrigation agriculture, using irrigation scheduling techniques based on monitoring soil and plant water status (Vera et al., 2017; Vera et al., 2019).
Automated irrigation scheduling, based on soil water sensors that provide real-time information, has become a major challenge for precise sustainable irrigation (Vories and Sudduth, 2021). Soil water content (Ɵv) is a state variable often proposed as a key input for irrigation management in decision support systems. Most of the available literature on fruit crops reported automatic irrigation controllers, using Ɵv values with on/off strategies based on real-time feedback protocols, which establish an upper and lower limit of each system state (Casadesús et al., 2012; Romero et al., 2012; Osroosh et al., 2016; Millán et al., 2019; Vories and Sudduth, 2021). In drip-irrigated nectarine trees, threshold Ɵv values converted to management allowed depletion (MAD) values were proposed to trigger/stop irrigation, thus allowing a more accurate soil-based irrigation scheduling (Vera et al., 2019). In this sense, Conesa et al. (2021) demonstrated that the automated MAD-based irrigation method, combined with regulated deficit irrigation criteria (Ruiz-Sánchez et al., 2010) proved to be a promising method for irrigation scheduling in Mediterranean agrosystems. In fact, precise deficit irrigation based on MAD threshold values used 40% less irrigation volume compared to irrigation based on conventional crop evapotranspiration (ETc) calculations (as the product of crop reference ET by local crop coefficients), maintaining yield and quality of nectarine fruits, and even increasing water use efficiency (Conesa et al., 2019).
Plant-based sensors for water status purposes address the concept of using plants as ‘biosensors’, where soil-water, atmospheric conditions and plant response are integrated (Jones, 2004). Midday stem water potential (Ψstem) has been accepted worldwide as the most reliable indicator of plant water status (Abrisqueta et al., 2015). Conesa et al. (2019) proposed the long-established Ψstem as the best reference indicator of the discontinuous plant water status for drip-irrigated nectarine trees. However, Ψstem is a very labour-demanding and destructive measurement that cannot be automated.
Nowadays, IoT in agriculture has led to the development of many detection methods as plant indicators to measure water status and to assess plant responses to environmental stresses. Indicators of plant water status on a continuous basis include those based on sap flow and stem heat balance (Smith and Allen, 1996; Navarro et al., 2020; Dix and Aubrey, 2021), trunk diameter fluctuations (Fernández and Cuevas, 2010; Ortuño et al., 2010), and leaf turgor (Martínez-Gimeno et al., 2017; Padilla-Díaz et al., 2018). However, although the latter two are non-invasive techniques (Fernández, 2014), the equipment used requires a significant labour input to properly monitor plant water status, as well as specialised staff for data processing.
The emerging sensors identified as microtensiometers (MTs) are embedded in the tree trunk and directly measure the trunk water potential (Ψtrunk) on a continuous basis, which is a major advantage over discrete Ψstem determinations. This sensor is a microelectromechanical system-based microtensiometer that measures plant water status with a high degree of accuracy. It can be automated and provides easy-to-interpret continuous data, in pressure units comparable to those of the Ψleaf or Ψstem acquired with traditional pressure chamber methods (Pagay et al., 2014; Lakso et al., 2022).
To our knowledge, only a few studies have addressed the performance of these MTs sensors in field conditions and under different water availability scenarios (e.g. Blanco and Kalcsits (2021) in apple and Pagay (2022) in gravepines). Our hypothesis is that MTs can provide stable continuous Ψtrunk data, and we seek to know if they can be used to validate automated MAD-based irrigation protocols, as we have already done from discrete Ψstem determinations in previous experiences (Conesa et al., 2019; Vera et al., 2019, Conesa et al., 2021; Mira-García et al., 2021).
This study aims to validate the use of Ψtrunk as a continuous plant-based water status indicator in drip-irrigated nectarine trees grown under Mediterranean conditions threatened by water scarcity. Irrigation scheduling was automatically managed by real-time Ɵv values at different levels of MAD corresponding to well-irrigated, moderate deficit and drought conditions. The performance of MAD-based irrigation method was also analysed in the soil-plant-atmosphere continuum
2. Material and methods
2.1. Field conditions
The experiment was carried out from June to September in 2022, in a 0.5 ha orchard of twelve-year-old early-maturing nectarine trees (Prunus persica (L.) Batsch, cv. Flariba, on GxN-15 rootstock), at the CEBAS-CSIC experimental station, Murcia (Spain, 38° 06’ 31’’ N, 1° 02’ 14’’ W). The trees were spaced 6.5 m x 3.5 m and trained to an open-centre canopy. The soil in the 0-0.5 m layer was stony and shallow with a clay-loam texture and low organic matter content 1.3%. The average bulk density was 1.43 g cm-3. Soil water content (Ɵv) at field capacity and at permanent wilting point was 0.29 and 0.14 m3 m-3, respectively. The drip-irrigation system consisted of one dripline per row of trees with four pressure-compensated emitters (4 l h-1) per tree located 0.5 and 1.3 m from the tree trunk. The amount of water applied was measured with a pulse flowmeter (Sensus, 120 HRI-A, Barcelona, Spain).
Seasonal fertiliser applications were 83, 56, and 109 kg ha−1 of N, P2O5 and K2O, respectively, applied by fertigation system (Vera and de la Peña, 1994). Other usual cultural practices (e.g. weed control, fertilization, pruning, fruit thinning) were carried out following the recommendations of commercial fruit tree orchards.
The experiment consisted of an automated soil-based irrigation treatment, managed according to different irrigation criteria (see 2.4 section), which were randomly distributed in four replicates, each consisting of six nectarine trees (n= 24). Measurements of soil and plant water relations were taken on a representative tree from each replicate.
2.2. Agrometeorological status
During the experimental period, agrometeorological data (air temperature, Ta; relative humidity, RH; and rainfall) were recorded every 15 min by an automatic weather station located in the CEBAS-CSIC experimental field, next to the nectarine tree orchard (http://www.cebas.csic.es/general_spain/est_meteo.html). Hourly reference crop evapotranspiration (ET0, mm) was calculated following the Penman-Monteith equation (Allen et al., 1998). Vapour pressure deficit (VPD, kPa) was calculated from daily maximum Taand minimum RH.
The hourly air water potential (Ψair,MPa) was calculated with the equation (Nobel, 1983):
where, R is the gas constant (R=0.082 atm L K-1 mol-1), T is the absolute temperature (273+Ta, °C), Vw the partial molar volume of water in the atmosphere (18 cm3 at 20 °C), and RH is the air relative humidity (%).
2.3. Soil water status
Soil water status was continuously monitored by measurements of soil water content (Ɵv) and soil matric potential (Ψm), as follows:
2.3.1. Soil water content
Volumetric soil water content (Ɵv, %) was monitored with multi-depth EnviroScan® capacitance probes (Sentek Sensor Technologies, Sidney, Australia). Four PVC access tubes were installed 10 cm from the emitter located close (0.5 m) to the tree trunk in four representative trees (one in each replicate). Each capacitance probe had sensors at 0.1, 0.3, 0.5, and 0.7 m depth, and was connected to a radio transmission unit. Values were read every 5 min and the average was recorded every 15 min. The probes were normalised and calibrated following the procedure proposed by Starr and Paltineanu (2002). Drip gauges (Pronamic, Ringkoebing, Denmark) were installed below the emitter near the capacitance probe to monitor real-time irrigation amounts and to detect any flow rate failures during the irrigation events. The radio-transmission units sent the data to a gateway that is connected to the addVANTAGE cloud server (ADCON Telemetry, Vienna, Austria) for data acquisition, processing, and visualisation.
2.3.2. Soil matric potential
Soil matric potential (Ψm, kPa) was measured with digital tensiometers (WEENAT, Nantes, France) consisting of granular matrix sensors, which were installed in the wet bulb of two nectarine trees, at 0.3 and 0.6 m soil depth. Data were recorded and visualised on the cloud platform www.weenat.com.
2.4. MAD-based irrigation protocol
Average Ɵv values of the 0-0.5 m soil profile, representing the active water uptake of the roots (Abrisqueta et al., 2017), were used to act on electro-valves by means of the telemetry network (see 2.3.1 section). The maximum allowable depletion (MAD) values were established as irrigation threshold Ɵv, as derived from the concept proposed by Merriam (1966), as:
where, FC is the field capacity, WP is the wilting point, α is the percentage depletion of available water in the soil.
In the experiment, the following α criteria were applied:
α = 10%: well-irrigated, from 3 to 29 June 2022.
α = 50%: moderate soil water deficit, from 30 June to 29 July 2022.
α = 100%: severe soil water deficit. No irrigation was applied from 30 July to 1 September 2022.
Recovery: Irrigation recovered at full crop water requirements, when Ψstem reached -2.0 MPa, from 2 September to 30 September 2022.
2.5. Plant water status
During the experimental period, plant water status was estimated by weekly measurements of discrete plant-based water indicators: leaf and stem water potentials and leaf gas exchange. In addition, daily time-courses were made on representative days of the well-irrigated period (23 June 2022, DOY 174), at the end of the moderate water deficit (α=50%) period (29 July 2022, DOY 210), and at the end of drought (α=100%) period (1 September 2022, DOY 244). All measurements were always performed on one leaf of the same trees in each replicate (n=4). In addition, trunk water potential was measured continuously in two of the four replicates (n=2).
2.5.1. Leaf and stem water potentials
Leaf (Ψleaf, MPa) and stem (Ψstem, MPa) water potentials were measured on four leaves (one leaf per replication) at midday (13:00-14:00 h, GMT+2), and hourly during daily courses on fully expanded healthy leaves, using a pressure chamber (Soil Moisture Equipment Corp. Model 3000, Santa Bárbara, CA, USA) as recommended by Turner (1988). Measurements of Ψleaf were made in sunny, freely transpiring leaves, while for Ψstem, leaves were located on the shaded side of the tree, close to the tree trunk, and covered with aluminium foil for at least 2 h before the determinations (McCutchan and Shackel, 1992). Both measurements were carried out weekly during the experiment, as well as hourly in the daily time-courses.
2.5.2. Trunk water potential
Trunk water potential (Ψtrunk, MPa) was determined using microtensiometers (MTs; FloraPulse, Davis, CA, USA, www-florapulse.com) embedded directly into the trunk on the shaded side of two nectarine trees, at 0.4 m from soil surface (Illustration 1A). Installation of the MTs was carried out according to the recommendations of the manufacturer. The technical details given by Pagay et al. (2014); Black et al. (2020), and Lakso et al. (2022) were also considered. The sensors were allowed to equilibrate with the tree (through the mating compound) within 2 days of installation (Pagay, 2022). Trunk water potential (Ψtrunk) data were obtained every 15 min, and transmitted using the same telemetry network (ADCON Telemetry, Vienna, Austria) (Illustration 1B).
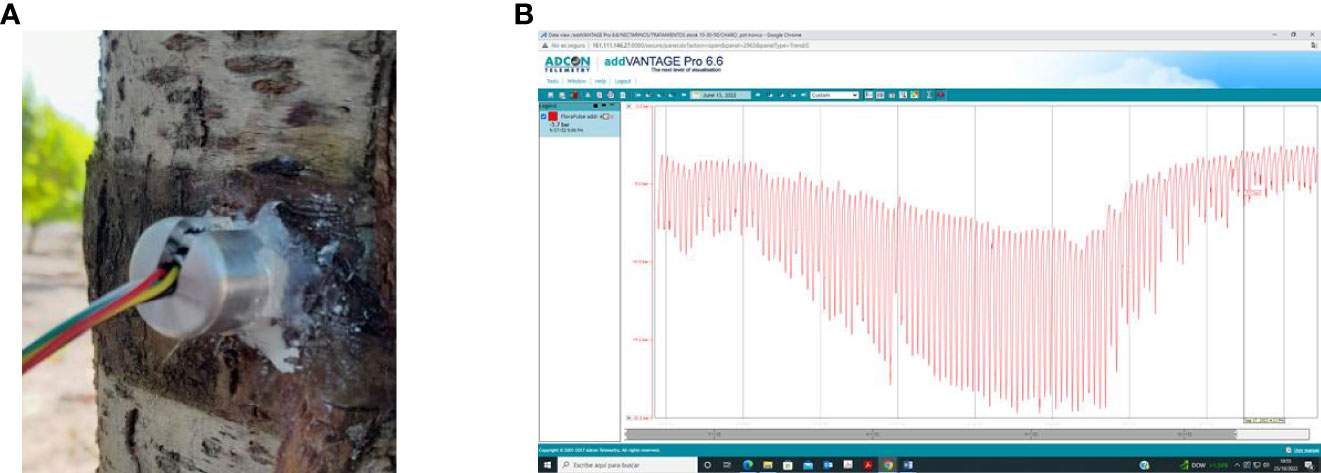
Illustration 1 (A) MT sensor installed in the nectarine tree trunk, and (B) data visualisation of Ψtrunk on addVANTAGE web server.
2.5.3. Leaf osmotic potentials
Leaf osmotic potentials (Ψπ, MPa) were determined at predawn, midday and afternoon on the same leaves used for Ψleaf determinations, coinciding with daily time-courses. Leaves were frozen in liquid nitrogen and the osmotic potential was measured after thawing the samples and expressing sap by using a vapour pressure osmometer (model WESCOR-5520; Wescor Inc., Logan, UT, USA) following the recommendations of Gucci et al. (1991). Leaf turgor potentials (Ψt, MPa) at predawn, midday and afternoon were calculated as the difference between osmotic and leaf water potentials. Leaf osmotic potential at full turgor (Ψπ100, MPa) was measured on leaves adjacent to those used for Ψleaf at predawn. The leaves were excised and placed by their petioles in distilled water overnight to reach full saturation, after which they were frozen in liquid nitrogen (-196 °C) and stored at -30 °C, following the same methodology as for Ψπ. The osmotic adjustment was estimated by comparing Ψπ100 values at α=10% (well-irrigated), and α=100% (non-irrigated).
2.5.4. Leaf gas exchange
Net photosynthesis (Pn, μmol m−2 s−1), stomatal conductance (gs mmol m−2 s-1), and transpiration rate (E, mmol m−2 s−1) were measured on one mature sunny leaf per replication (n=4) in the early morning (9:00-10:00 h, GMT+2), using a portable gas exchange system (LI-COR, LI-6400) at photon flux density (PPFD) ≈ 1500 μmol m−2 s−1 and CO2 concentration ≈ 400 μmol mol−1. During daily time-courses, hourly leaf gas exchange measurements were taken under ambient PPFD conditions at the time of measurements. Transpiration efficiency (WUET, μmol mmol-1) was calculated as the Pn/E ratio.
2.6. Sensitivity analysis
For the plant-based status indicators, the signal intensity (SI) was calculated as the ratio between all data registered at α=100% (drought conditions) and α=10% (well-irrigated conditions) periods. To determine noise, the coefficient of variation (CV) of the measurements was calculated for each indicator.
Sensitivity was determined using two algorithms:
- Traditional method (S), as proposed by Goldhamer and Fereres (2001):
S is always greater than 0, and the higher the value, the greater the sensitivity.
- Corrected sensitivity (S*), as proposed by De la Rosa et al. (2014).
The interpretation of the values obtained with this algorithm is as follows:
(a) S* > 1: indicates sensitivity to water deficit.
(b) 1 > S* > 0: The noise is greater than the increase in signal intensity.
(c) S* = 0: not sensitive to water deficit.
(d) S*< 0: anomalous behavior.
2.7. Statistical analysis
Data were depicted using the SigmaPlot v. 14.5 software (Inpixon, PA, USA). Statistical comparisons were considered significant at p<0.05, using Pearson’s correlation coefficient. Relationships between indicators of plant and soil water status were explored by linear regression analyses. The coefficient of determination (R2) and mean squared error (MSE) were used to assess the goodness of fit. All analyses were performed with SPSS v. 9.1 (IBM, Armonk, NY, USA).
3. Results
3.1. Automated control of irrigation and climatology
The climatic conditions during the experiment, comprising the postharvest period of the early-maturing nectarine trees (June to October), corresponded to a typical Mediterranean semi-arid summer environment, high values of ET0 (472.1 mm) and low rainfall (10.2 mm concentrated during the recovery period). Daily VPD values varied in a range of 0.2 and 3.3 kPa, representing the greatest day-to-day variability of the agrometeorological variables studied (Figure 1A).
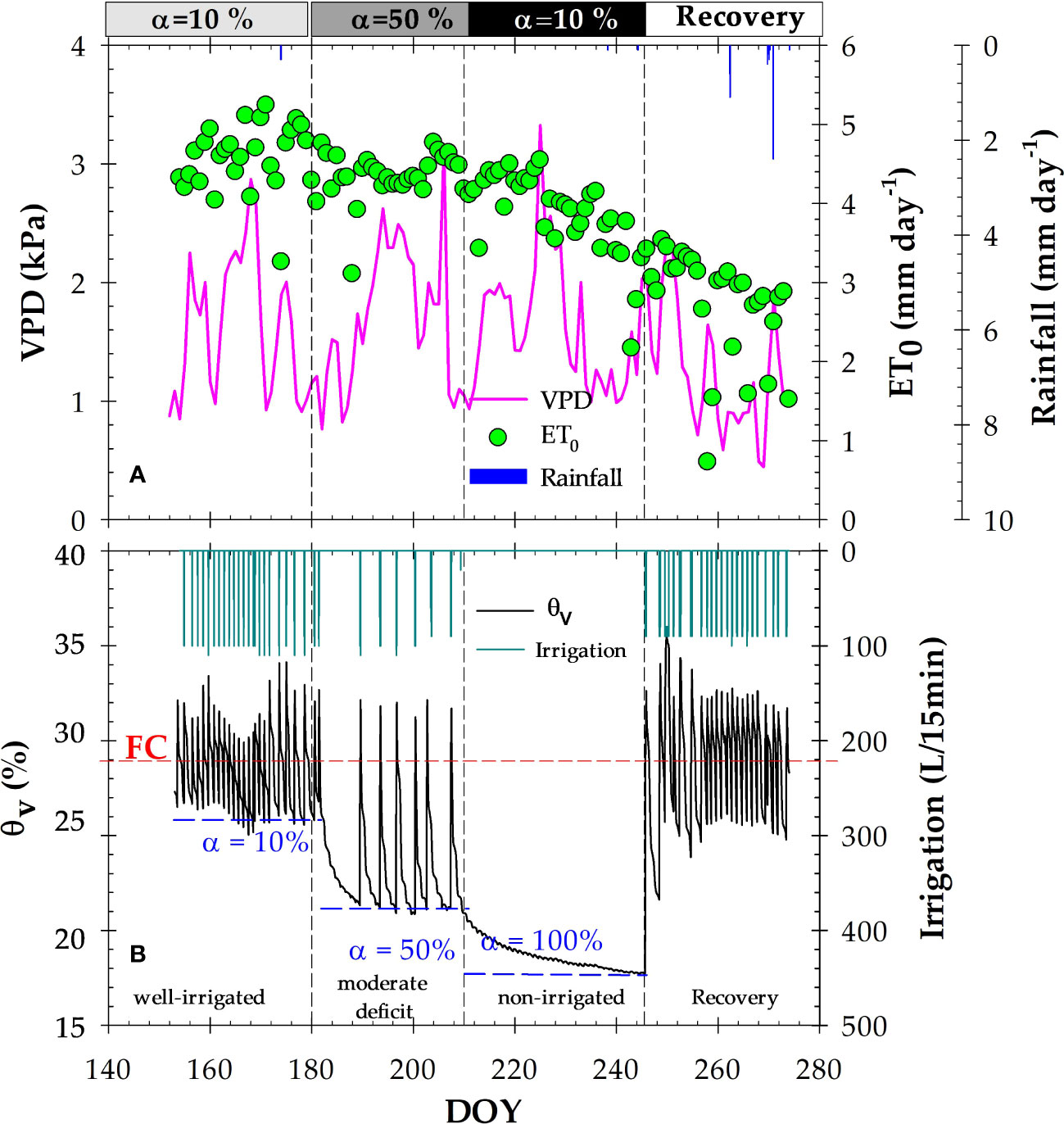
Figure 1 (A) Daily vapour pressure deficit (VPD, kPa), reference crop evapotranspiration (ET0, mm), and rainfall (mm); (B) Soil water content (θv, %) in the 0–0.5 m soil profile, and irrigation events (mm), during the experimental period. The dashed horizontal red line corresponds to the field capacity (FC), and the dashed blue lines indicate the soil water deficit (α) criteria: 10% (well-irrigated), 50% (moderate deficit) and 100% (severe deficit, non-irrigated), respectively. The dashed vertical lines delimit each irrigation criterion. DOY: Day of the year.
Volumetric soil water content (Ɵv) fluctuated in response to irrigation, root water uptake and rainfall events. Furthermore, Ɵv in the active root zone (0-0.5 m depth) was clearly influenced by the different imposed MAD-based protocols (Figure 1B). At α=10%, MAD=27.5% (well-irrigated conditions) induced by daily irrigation frequency, Ɵv values varied around field capacity (FC), increasing slightly above this value at the end of each irrigation event. At α=50%, MAD=21.5% (moderate soil water deficit) induced an irrigation frequency of 2 or 3 day. When irrigation water was withheld (α=100%), Ɵv decreased until the minimum value of Ɵv ≈ 17%, close to the wilting point value. Subsequently, during the recovery period, Ɵv reached variable FC values in response to irrigation and, to a lesser extent, rainfall events. The total amount of irrigation applied during the experiment (including the recovery phase) was 109.5 mm (Figure 1B).
3.2. Seasonal soil-plant-atmosphere water indicators
The data in Figure 2 show the seasonal course of water status in the soil-plant-atmosphere continuum (SPAC). The seasonal trend of air water potential (Ψair) was highly variable from day-to-day during the study, with a maximum value of -81.5 MPa (DOY 210, α=50%) and minimum of -224 MPa (DOY 168, α=10%) (Figure 2A).
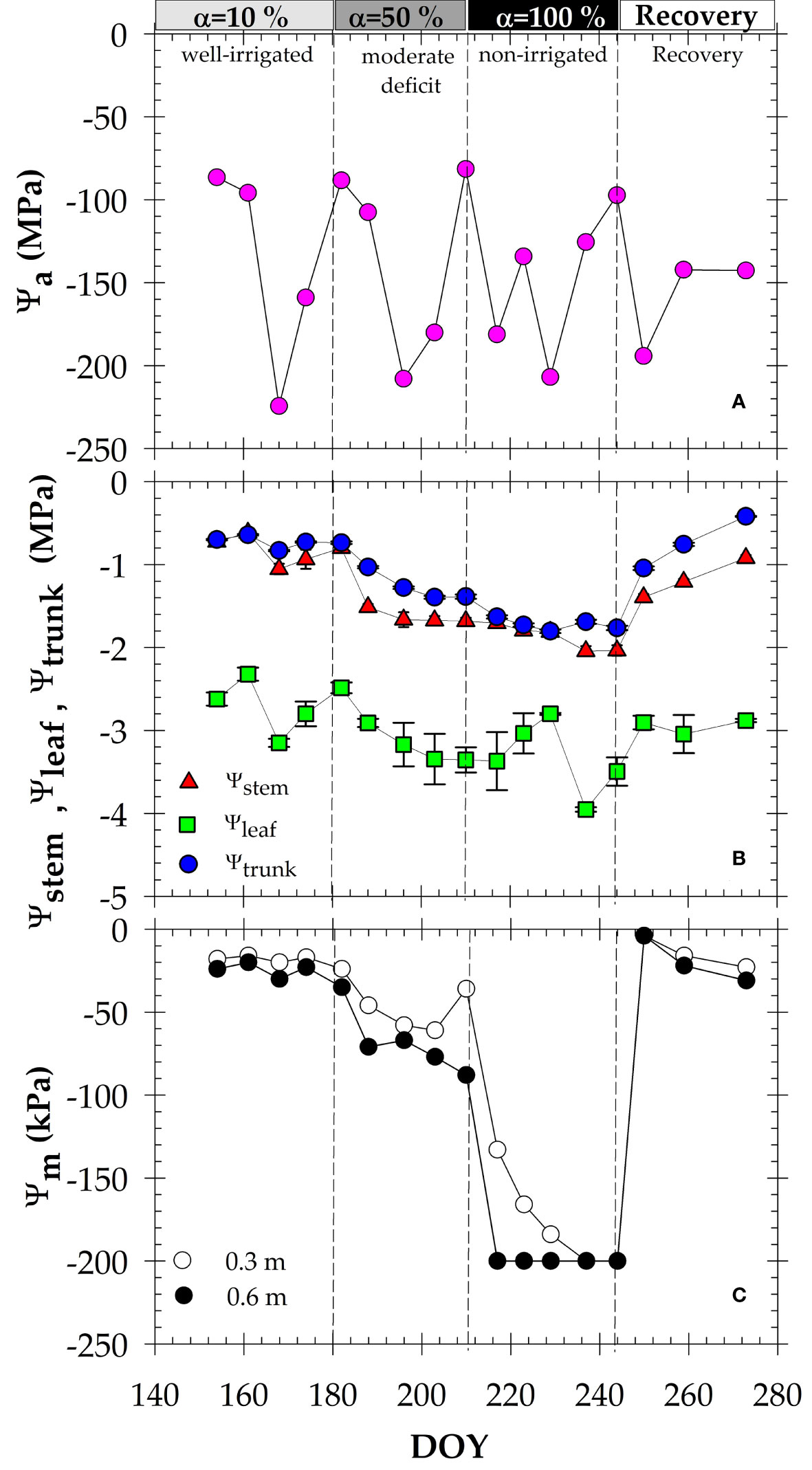
Figure 2 Seasonal course of: (A) daily mean air water potential (Ψair); (B) midday stem (Ψstem), leaf (Ψleaf) and trunk (Ψtrunk) water potentials, and (C) soil matric potential (Ψm) at 0.3 and 0.6 m of the soil profile. Each point is the average of four leaves, two MTs, and two granular matrix sensors. Vertical bars at data points are ± SE (not shown when smaller than the symbols). Dashed vertical lines delimit each irrigation criterion. DOY: Day of the year.
Soil water potential from the granular matrix sensors (Ψm), assuming osmotic and gravitational components to be negligible, ranged from -4 ± 0.85 to -26 ± 1.26 kPa at both depths explored (0.3 and 0.6 m) under well-irrigated conditions (α=10%). Under moderate deficit conditions (α=50%), Ψm decreased, showing slightly lower values at 0.6 than at 0.3 m, and reaching minimum values of -61 ± 3.45 and -77 ± 4.48 kPa (MPa) at 0.3 and 0.6 m, respectively. When irrigation was suspended (α=100%), Ψm continued to decrease, reaching its minimum allowable reading (-200 kPa) only one week later at 0.6 m depth, and after 13 days of withholding irrigation at 0.3 m (Figure 2C).
Plant water potentials evaluated at three canopy levels (leaf, stem and trunk) reflected the different MAD applied during the experiment (Figures 2A, B). Both Ψstem and Ψtrunk exhibited a constant pattern during α=10%, averaging -0.83 ± 0.09 and -0.73 ± 0.06 MPa, respectively, during this well-irrigated period. In accordance with the imposed soil water deficit, the trend of both plant indicators decreased, reaching the minimum values of Ψstem = -2.04 ± 0.06 MPa and Ψtrunk = -1.81 ± 0.29 MPa, at the end of α=100%. A more irregular trend was observed for Ψleaf during the experiment, showing lower values than those of Ψstem and Ψtrunk, and minimum values of -3.95 ± 0.26 MPa at the end of the irrigation withholding phase (DOY 237, α=100%).
Correlation analysis between soil and plant water potentials showed a close linear relationship with the highest dependence found between Ψm and Ψtrunk (R2 = 0.79), and the lowest (not significant) between Ψm and Ψleaf (R2 = 0.26) (Figure 3). However, there was no significant correlation between Ψair and plant water potentials (data not shown).
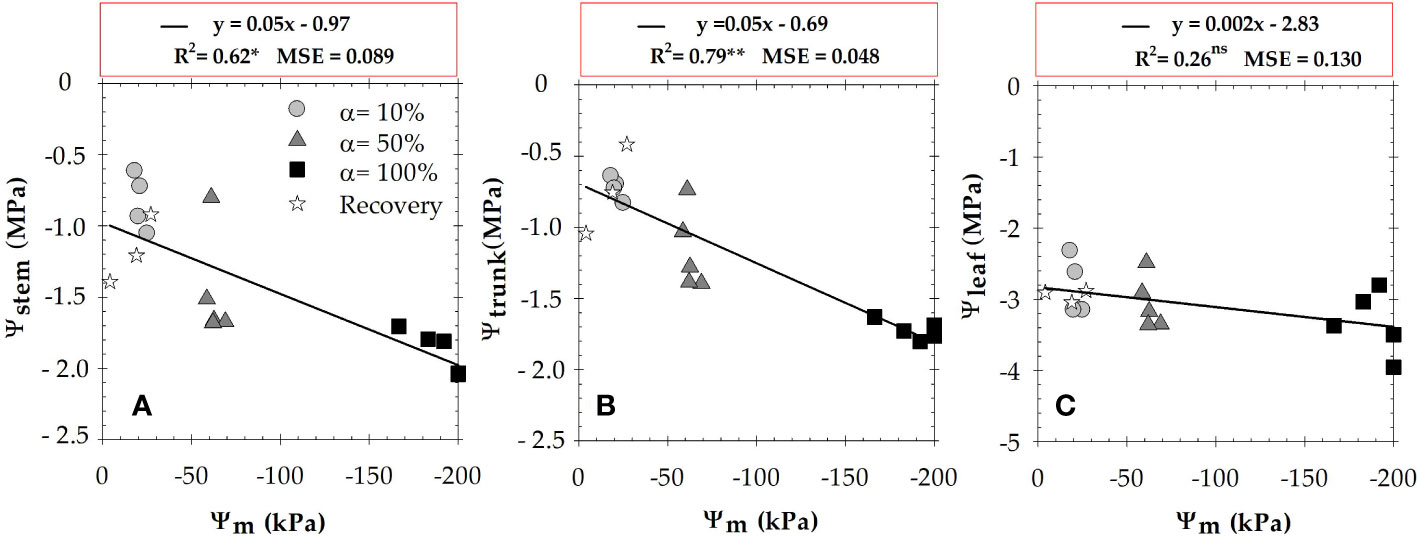
Figure 3 Relationship between the midday values of soil matric potential (Ψm) (average of 0.3 and 0.6 m), and (A) stem water potential (Ψstem); (B) trunk water potential (Ψtrunk); and (C) leaf water potential (Ψleaf), during the experimental period. The different symbols correspond to the four irrigation criteria. Each point is the mean of four leaves and two matrix sensors. R2 is the coefficient of determination. *: p ≤ 0.05 **: p ≤ 0.01, ns: not significant. MSE: mean squared error.
During the experiment, the gradient between midday values of Ψstem and Ψtrunk varied over a range of 0.02 to 0.5 MPa, while this gradient was higher for Ψleaf and Ψtrunk (1.0 to 2.5 MPa) (Figure 2B). In this regard, Ψtrunk data obtained with microtensiometers (MTs) were correlated with the plant-based indicators measured with a pressure chamber: Ψstem and Ψleaf (Figure 4). The results indicated a robust significant correlation between Ψtrunk to Ψstem (R2 = 0.86), and, again, to a lesser extent between Ψtrunk to Ψleaf (R2 = 0.37).
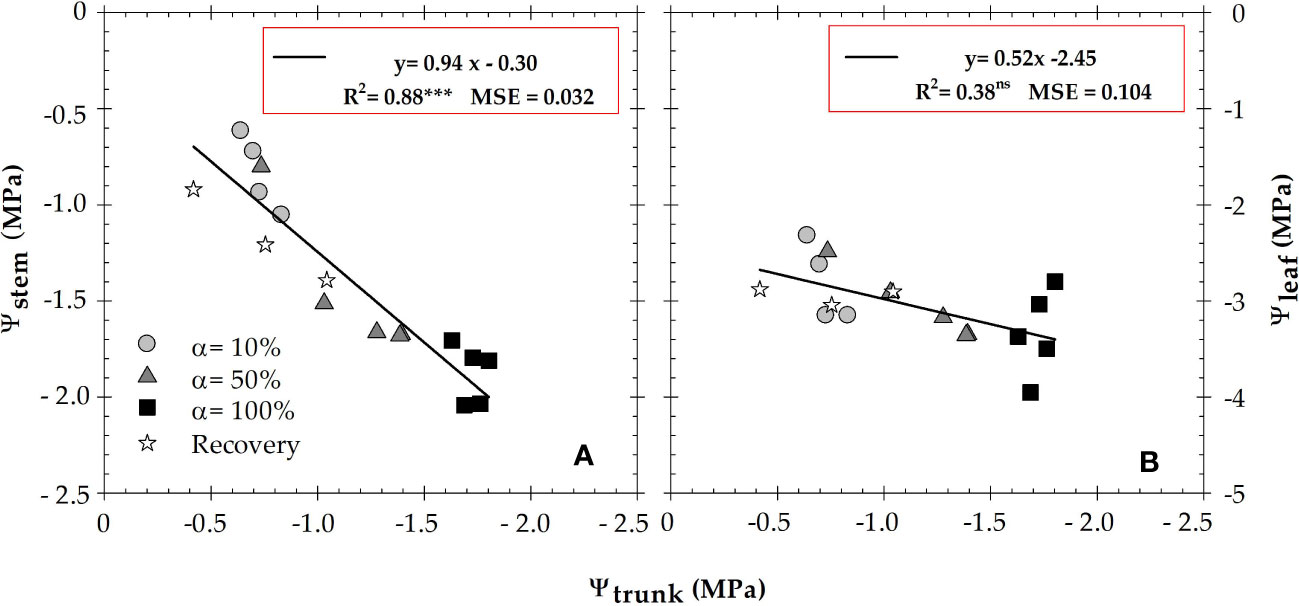
Figure 4 Relationship between midday values of trunk water potential (Ψtrunk) and (A) stem water potential (Ψstem), and (B) leaf water potential (Ψleaf), during the experimental period. The different symbols correspond to the different irrigation criteria. Each point is the mean of four leaves and two matrix sensors. R2 is the coefficient of determination. ***: p ≤ 0.001, ns: not significant. MSE: mean squared error.
Leaf gas exchange (Pn and gs), measured simultaneously with stem and leaf water potentials, showed a seasonal trend that mirrored the soil deficit imposed by the MAD-irrigation protocols (Figures 5A, B). At α=10%, both Pn and gs reached their maximum values of about 22 ± 0.34 µmol m-2 s-1 and 320 ± 30.5 mmol m-2 s-1, respectively. The lowest values of Pn (8.6 ± 0.51 µmol m-2 s-1) and gs (63.5 ± 9.05 mmol m-2 s-1) were obtained at the end of the α=100% period (severe water deficit). Pn and gs also varied in response to plant water potentials under quite contrasting environmental conditions (Figure 2B). Values of WUET increased with the imposed soil water deficit (Figure 5C), reaching a maximum value of 5.5 ± 0.10 µmol mmol-1. (Figures 5C). It is also important to note that despite irrigation being re-established during the recovery phase, the mean values of Pn and gs were lower than those obtained under well-irrigated conditions (α=10%).
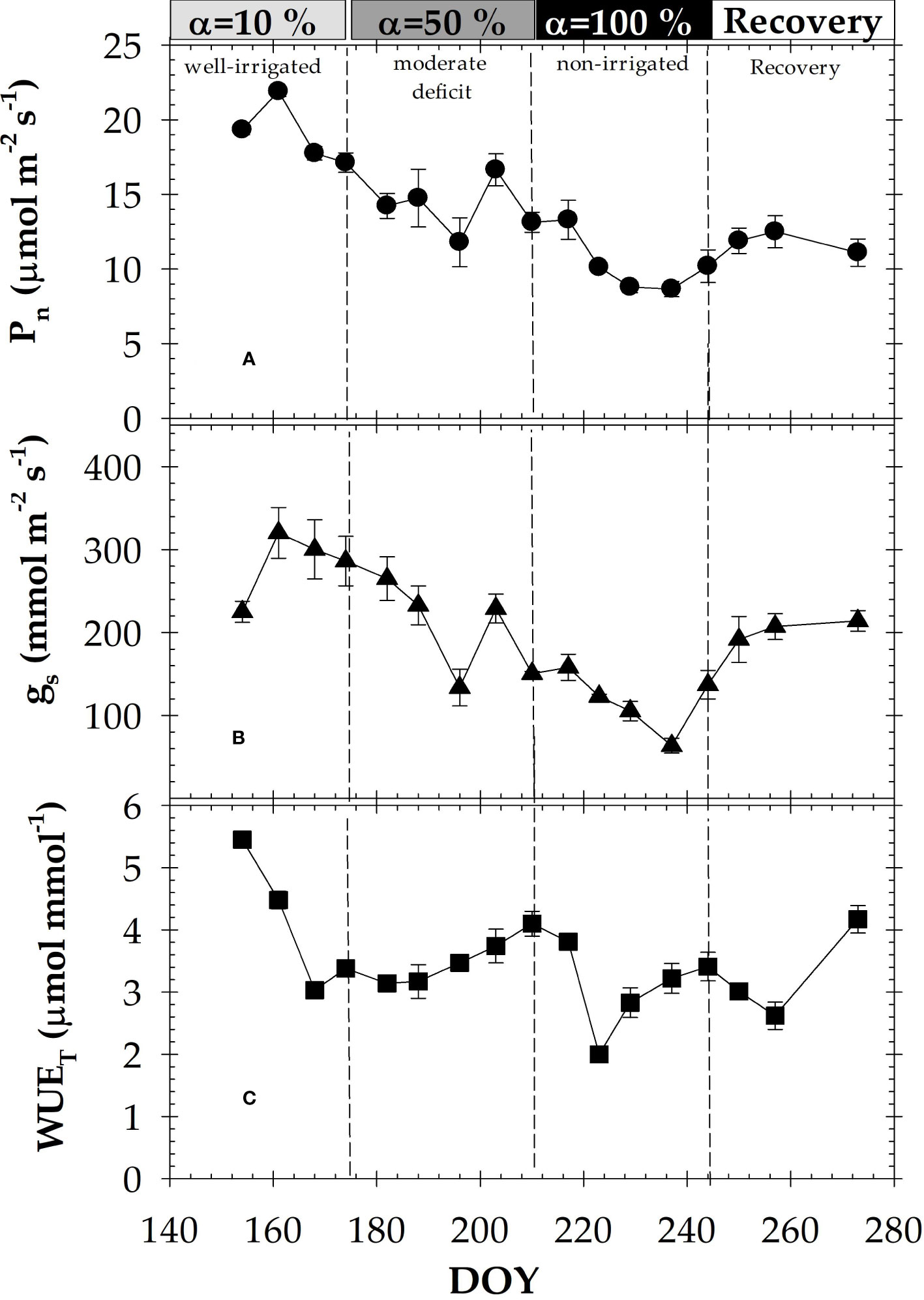
Figure 5 Seasonal time-course of: (A) net photosynthesis (Pn); (B) stomatal conductance (gs); and (C) transpiration efficiency (WUET) during the experimental period. Each point is the mean of four leaves. Vertical bars in data points are ± SE (not shown when smaller than the symbols). Dashed vertical lines delimit each irrigation criterion. DOY: Day of the year.
3.3. Diurnal indicators of soil-plant-atmosphere water status
The daily time-course of soil-plant-atmosphere water status indicators were evaluated on representative days of the well irrigated period (23 June 2022, DOY=174), at the end of moderate water deficit (α=50%) period (29 July 2022, DOY=210), and at the end of drought (α=100%) period (1 September 2022, DOY=244) covering the whole daily light period (06:00 to 21:00 h). The values of soil water content during well irrigated period were 27.82 ± 0.49; 39.54 ± 0.35; 26.63 ± 0.28 and 33.50± 0.19% at 0.1, 0.3, 0.5, and 0.7 m of soil depth, respectively. Meanwhile, at α=50%, and α=100%, θv decreased up to 35% below the FC values, mainly affecting the upper soil depth (> 0.5 m) with little variation observed at the deeper layer (data not shown). In addition, Ψm remained constant during each daily course, decreasing as water deficit increased (Figures 6G–I).
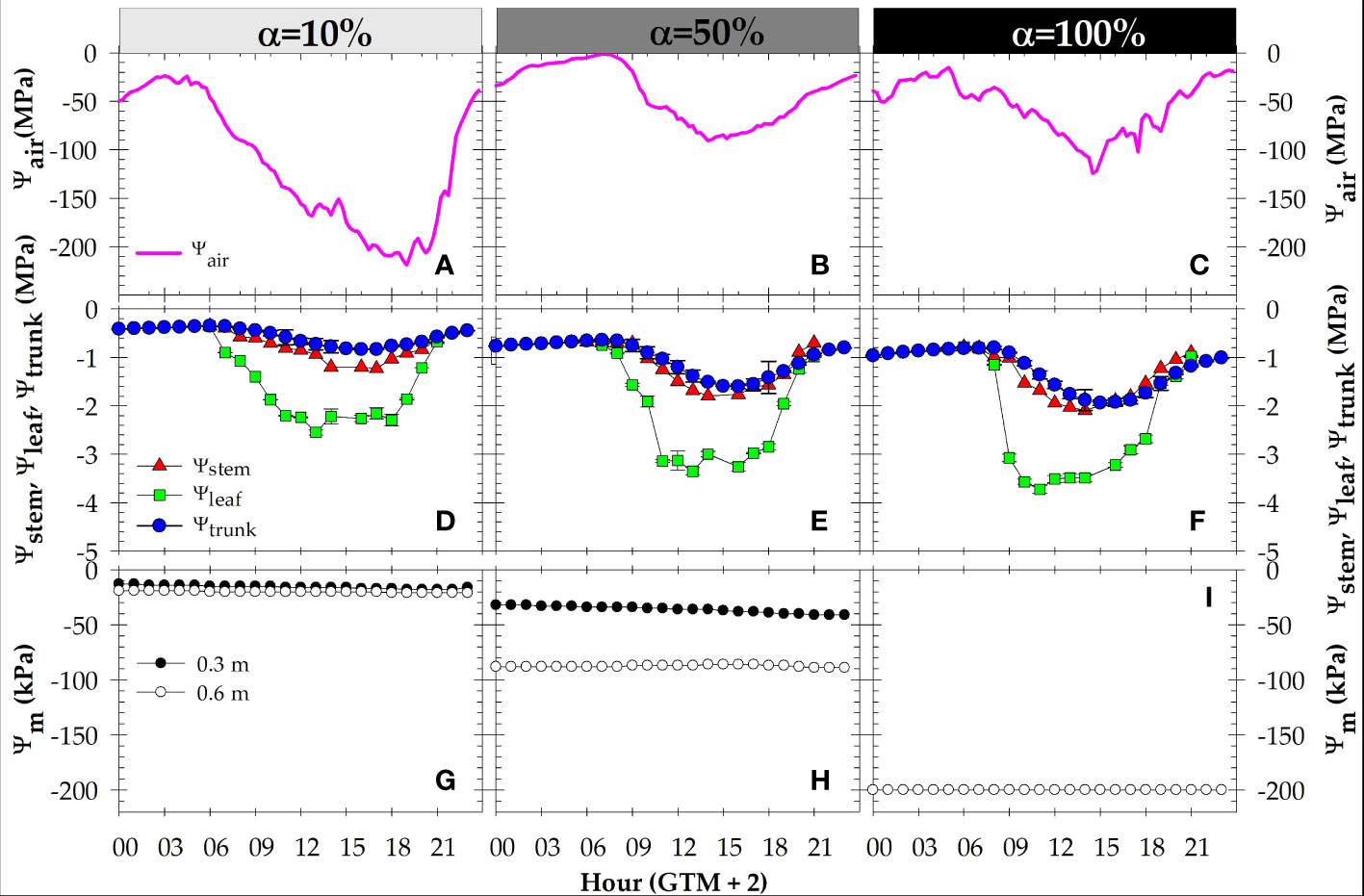
Figure 6 Daily time-courses of: (A-C) air water potential (Ψair); (D-F) leaf (Ψleaf), stem (Ψstem), and trunk (Ψtrunk) water potentials; and (G-I) soil matric potential (Ψm) at 0.3 and 0.6 m in the soil profile, during different irrigation criteria: α=10% (DOY 174), α=50% (DOY 210) and α=100% (DOY 244). Each point is the mean of four leaves, two MTs, and two granular matrix sensors. Vertical bars in the data points are ± SE (not shown when smaller than the symbols). GMT: Greenwich mean time.
Agrometeorological conditions changed greatly during the days selected for punctual measurements (Figures 6A–C). A very demanding day coincided with the well-irrigated period (α=10%), being the warmest of the three diurnal courses studied, with minimum Ψair values of -218 MPa registered in the early afternoon. Sunny mild-demanding days corresponded to the end of α=50% and α=100% periods, when minimum Ψair values of -84 and -124 MPa were recorded at midday, respectively.
The diurnal patterns of plant water potentials mirrored the imposed soil water deficit based on MAD-threshold values (Figures 6D–F), despite the different climatic conditions observed. At α=10%, minimum values of -0.87 ± 0.08, -1.30 ± 0.06 and -2.1 ± 0.31 MPa were measured in the early afternoon (16:00 h GMT+2) for Ψtrunk, Ψstem, and Ψleaf, respectively. At α=50%, plant water potentials recorded their minimum values at different times of the day. In this sense, Ψleaf and Ψstem obtained their minimum values at midday: -3.3 ± 0.26 MPa (Ψleaf) and -1.8 ± 0.12 MPa (Ψstem), whereas the minimum value of Ψtrunk (-1.6 ± 0.19 MPa) was obtained in the afternoon (17:00 GMT+2). The severe water deficit situation recorded at α=100% induced a decrease in plant water potentials from predawn onwards. In this period, Ψleaf and Ψstem reached again their minimum values at midday (-3.5 ± 0.26, and -2.1 ± 0.12 MPa, respectively); and those for Ψtrunk (-1.9 ± 0.21 MPa) in the afternoon (17:00 GMT+2) (Figures 6D–F). It must be emphasized that the values of water potentials at predawn decreased from -0.35 ± 0.08, -0.68 ± 0.03 to -0.80 ± 0.11 MPa, at α=10, 50, and 100% periods, respectively.
To represent the SPAC resistances to water flow along the soil-plant-atmosphere continuum, the experimental values of water potentials at midday were drawn (Figure 7). It can be observed that the highest gradient was found from leaf to air, which is tuned by stomatal aperture, regulating the change of water state from liquid to gas, while the lowest gradient (0.3 MPa) was between Ψtrunk and Ψstem. Under well irrigated conditions, the next important gradient was between Ψstem and Ψleaf (1.7 MPa), followed by Ψm to Ψtrunk gradient (0.7). As the water deficit progresses, these gradients increase, especially in the case of root to trunk water potential differences (1.5 MPa at the end of the non-irrigation period), and remained almost constant for Ψstem to Ψleaf gradient, and even decreased for leaf to air water potentials.
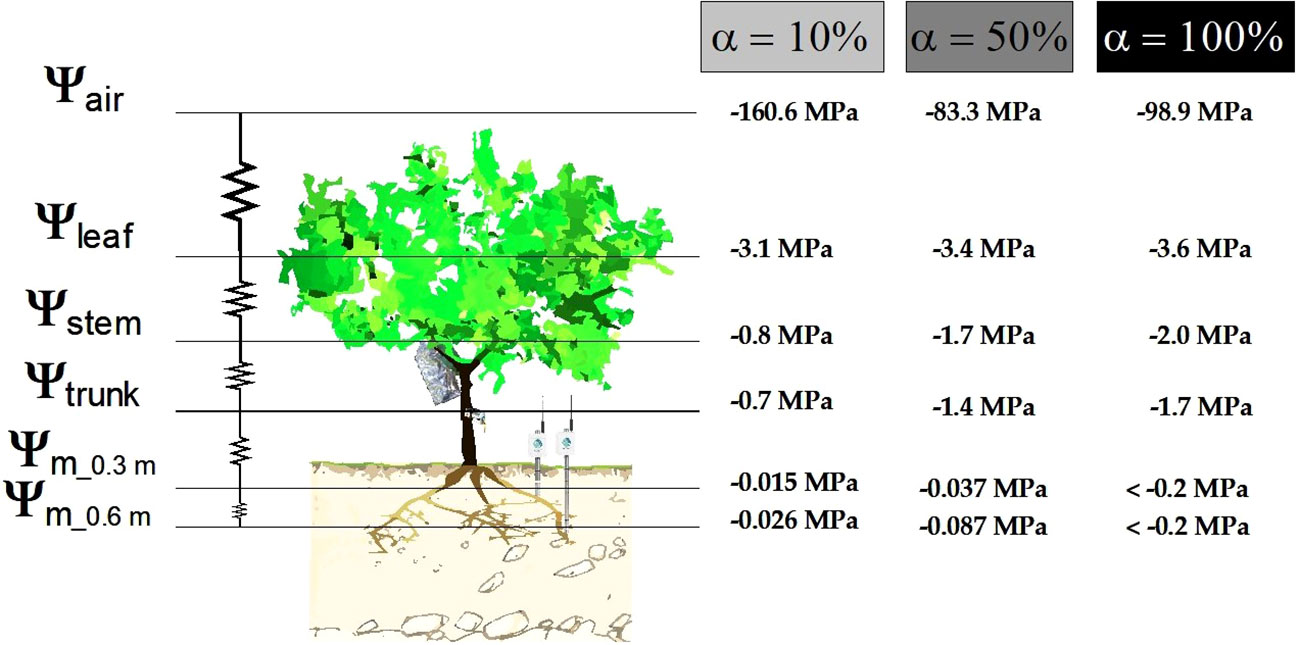
Figure 7 Mean values of water potential at midday in the SPAC during each irrigation criterion: α=10% (well-irrigated), α=50% (moderate water deficit), and α=100% (severe water deficit, non-irrigated).
The data in Figure 8 illustrates the diurnal variations of the relationship of Ψtrunk with Ψstem (A) and Ψleaf (B) at the different irrigation periods. Notably, values of Ψtrunk in the early afternoon recovered their morning values at higher Ψstem values (Figure 8A). This fact was more noticeable when considering Ψleaf values (Figure 8B). The significance of the coefficient of determinations was higher during the well irrigated period, and decreased at α=50%, not being significant at α=100% (Figures 8A, B).
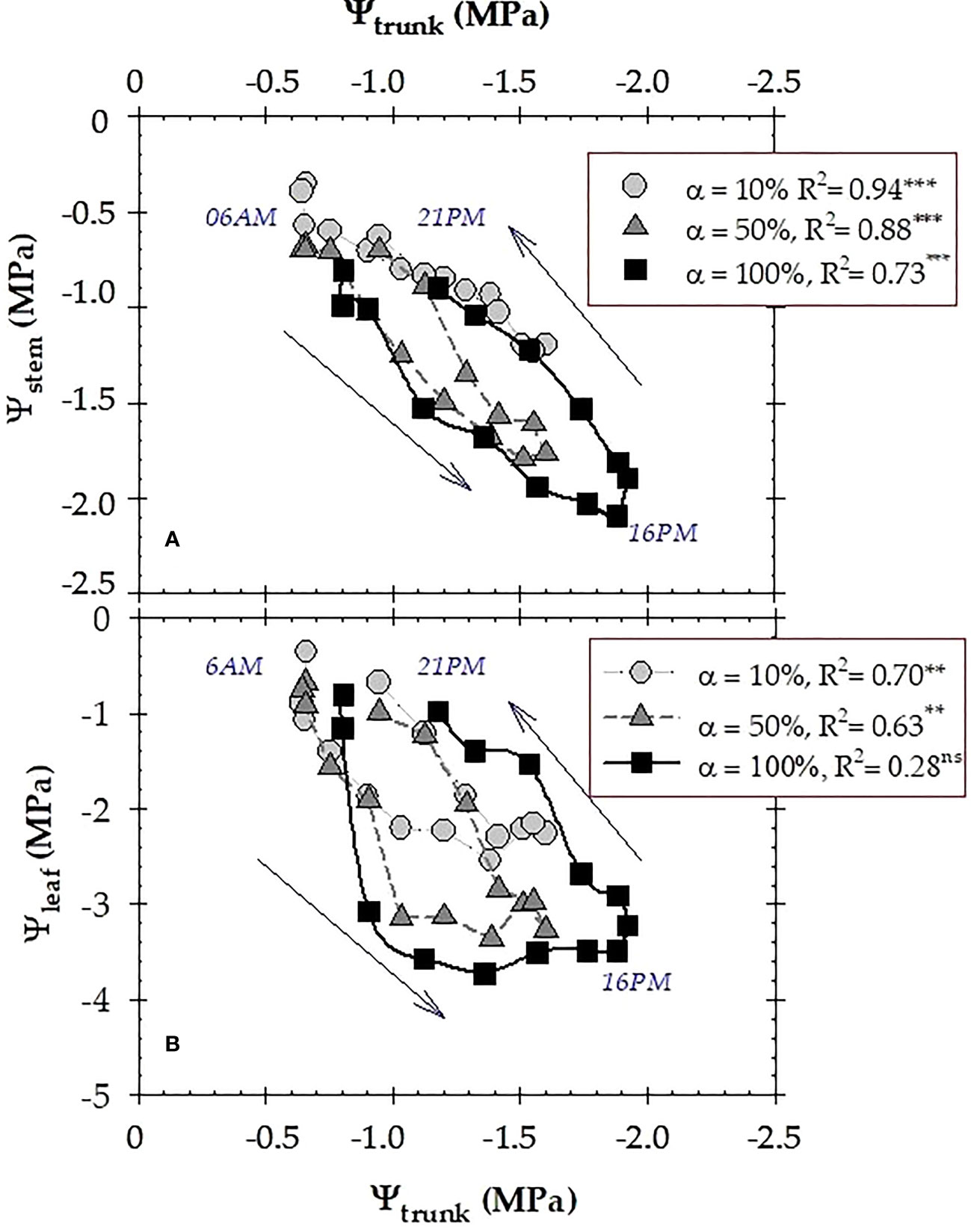
Figure 8 Daily time-course of the relationship between trunk water potential (Ψtrunk) and: (A) stem water potential (Ψstem) and (B) leaf water potential (Ψleaf) at each irrigation criterion: 10% (well-irrigated), 50% (moderate water deficit) and 100% (severe water deficit, non-irrigated), respectively. The data point is the mean of four leaves. R2 is the coefficient of determination of the linear regression. **: p ≤ 0.01; ***: p ≤ 0.001, ns: not significant.
Regarding daily leaf gas exchange courses, Pn and gs increased from sunrise at 08:00 h to 10:30 h GTM+2, which was the period of maximum photosynthetic efficiency in all irrigation conditions studied (Figure 9). During midday, leaf gas exchange exhibited a decrease in its values, although it corresponded with the peaks of solar radiation (Rs) (Figures 9A–C). In the afternoon from 16:00 h to 18:00 h GMT+2, leaf gas exchange parameters exhibited a slightly recovery, even under water deficit conditions (α=50 and 100%). From that moment on, the course of leaf gas exchange parameters tended to decrease, until the night hours when minimum values were recorded.
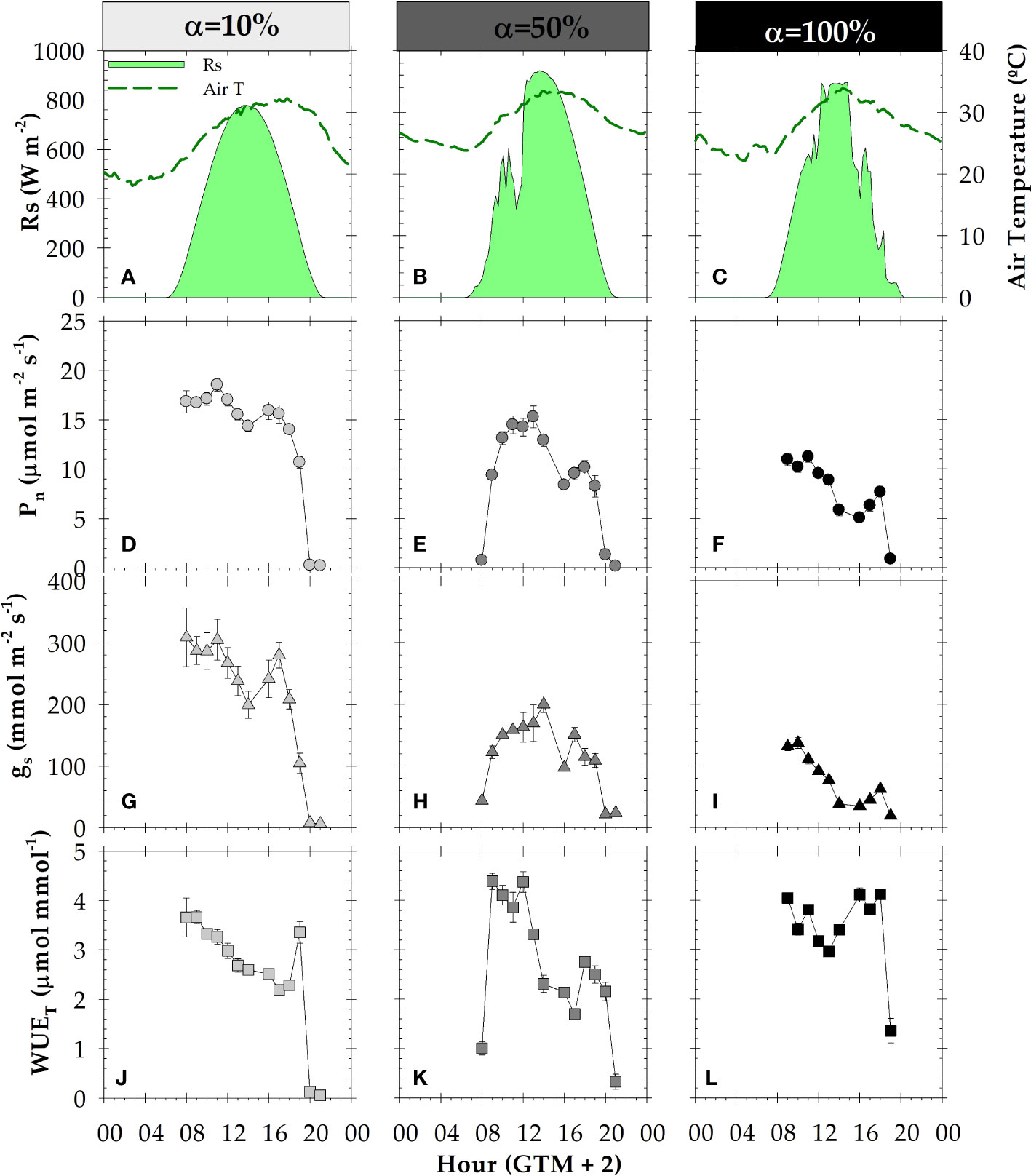
Figure 9 Daily courses of: (A–C) solar radiation (Rs) and air temperature; (D–F) net photosynthesis (Pn); (G–I) stomatal conductance (gs); (J–L) transpiration efficiency (WUET), during different irrigation criteria: α=10% (DOY 174), α=50% (DOY 210) and α=100% (DOY 244). Each point is the mean of four leaves. Vertical bars in data points are ± SE (not shown when smaller than the symbols). GMT: Greenwich mean time.
The diurnal patterns of leaf gas exchange followed the established MAD values (Figures 1B, 9). In this sense, at α=10%, the values corresponded to well-irrigated conditions, with maximum values of 17.12 ± 0.65 µmol m-2 s-1, 308 ± 32.9 µmol m-2 s-1 and 5.54 ± 0.35 mmol mmol-1, for Pn, gs and WUET, respectively. As expected, the lowest values were obtained under severe water deficit situation (α=100%), with maximum daily values of Pn = 10.94 ± 0.60 µmol m-2 s-1, gs = 137.1 ± 8.60 µmol m-2 s-1 and WUET = 4.04 ± 0.08 mmol mmol-1.
3.4. Osmotic water potentials
Figure 10 shows the values of osmotic water potential (Ψπ) determined at different times (predawn, midday and afternoon) during the diurnal courses of the different irrigation criteria. At α=10% (well-irrigated), Ψπ significantly increased from -1.61 ± 0.01 MPa at predawn to -2.97 ± 0.01 MPa in the afternoon. Under water deficit conditions, the minimum Ψπ was found at midday, with values of -3.14 ± 0.05 MPa (at α=50%) and -3.10 ± 0.10 MPa (at α=100%) (Figure 10A). In contrast, the osmotic potential at full turgor (Ψπ100) measured at predawn was similar throughout the experimental period with a mean value of -1.76 ± 0.03 MPa (Figure 10A).
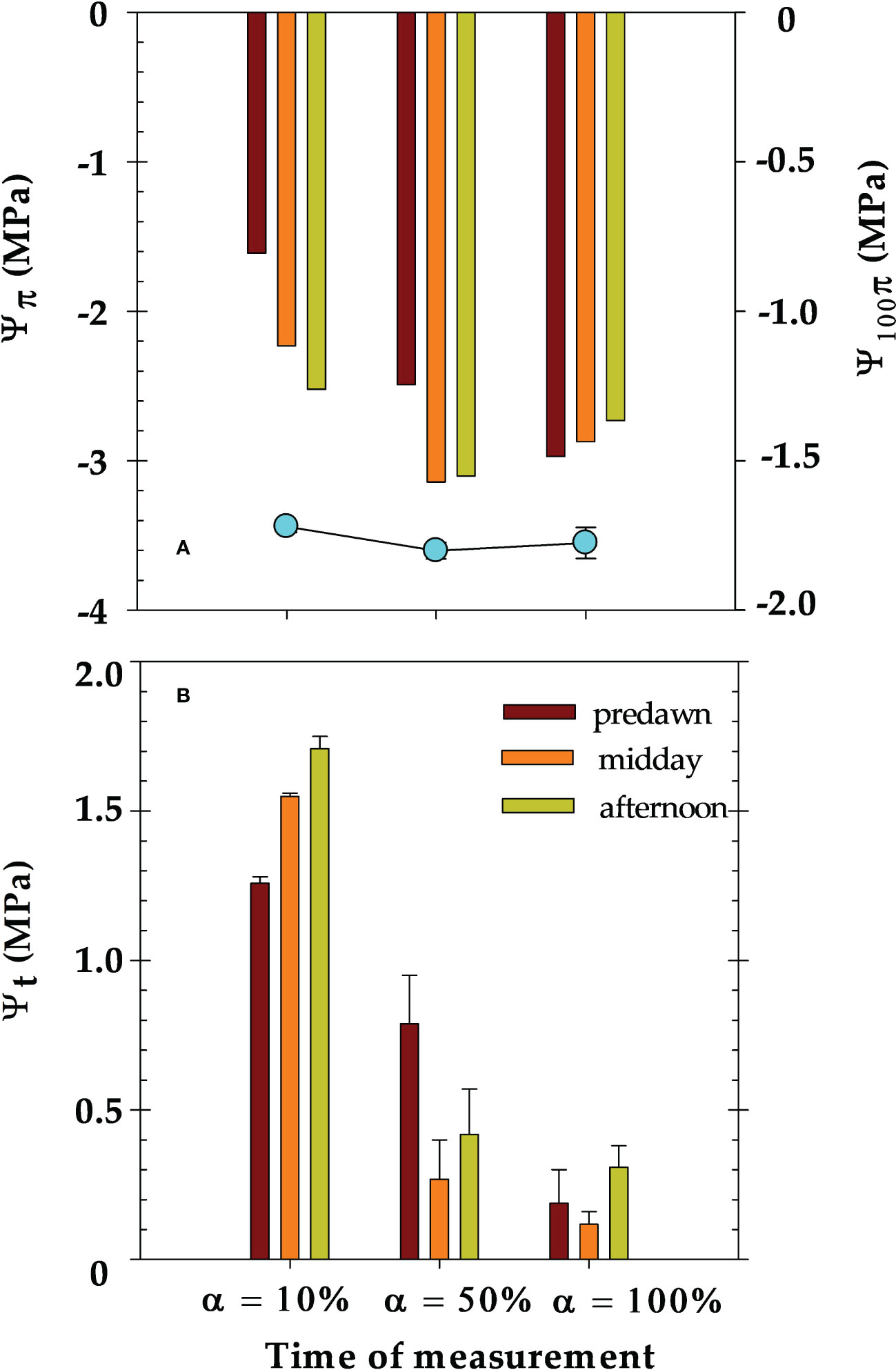
Figure 10 Values of: (A) actual osmotic water potential (Ψπ), and osmotic water potential at full turgor (Ψπ100), and (B) and leaf turgor potential (Ψt) at different times of the day (predawn, midday and afternoon) during the different irrigation criteria: α=10% (DOY 174), α=50% (DOY 210) and α=100% (DOY 244). The measurements were made on the same leaves used for leaf water potential. Each bar is the mean of four leaves ± ES.
The leaf turgor potential (Ψt) decreased close to zero as soil water deficit increased (Figure 10B). In this sense, at α= 100% lower Ψt values were computed at midday coinciding with the lower leaf water potential (Ψleaf) and higher evaporative demand values (Figure 6).
3.5. Sensitivity analysis
Comparative analysis of the sensitivity of the indicators of plant water status revealed that plant water potentials showed a higher sensitivity than those obtained for leaf gas exchange (Table 1). From the plant water potentials: Ψtrunk, Ψstem and Ψleaf, it was clear that Ψtrunk was clearly the plant-based water status indicator with the highest SI and sensitivity values by the two methods assessed (S and S*), followed by Ψstem and to a lesser extent by Ψleaf and leaf gas exchange parameters (Table 1). In particular, CV was slightly lower for Ψstem (2.15) than for Ψtrunk (2.47). The S was similar between Ψtrunk and Ψstem, even though S* indicated a higher sensitivity for Ψtrunk.
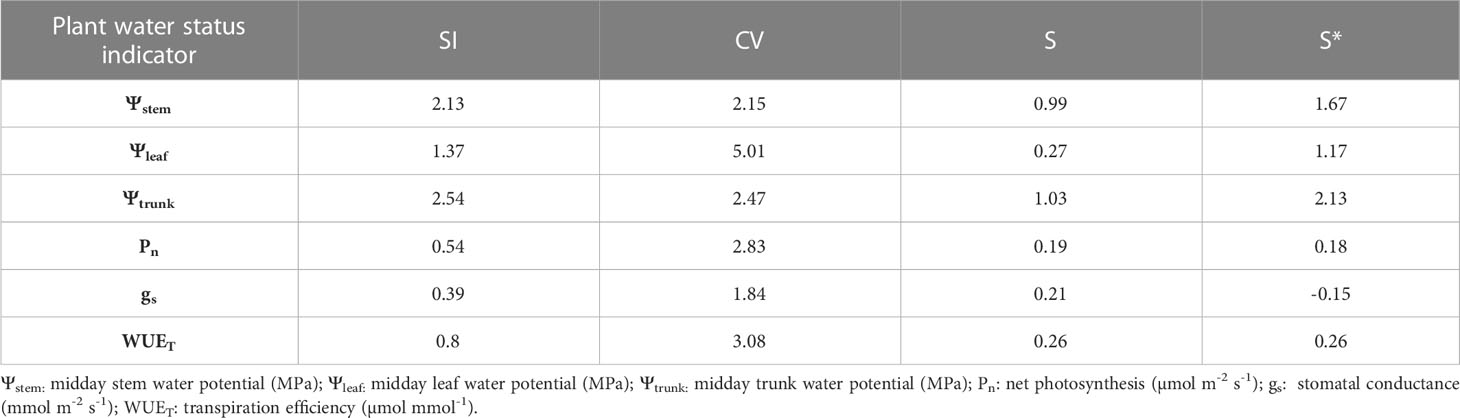
Table 1 Sensitivity analysis (SI: Signal intensity; CV: coefficient of variation; S: sensitivity (by Goldhamer and Fereres, 2001); and S*: corrected sensitivity (by De la Rosa et al., 2014) for the indicators of plant water status during the experimental period.
4. Discussion
Continuous recording of trunk water potential (Ψtrunk) obtained in situ with MTs has been a suitable measure of plant water status of drip-irrigated nectarine trees. Measurements of Ψtrunk have validated the established MAD-based irrigation protocols (Figure 1B), becoming useful alternative to discrete measurements of leaf or stem water potentials with traditional pressure chamber (Figures 2B, 6). Moreover, Ψtrunk has the advantage of being measured continuously and in real-time, which could lead to automation, whereas Ψleaf or Ψstem are destructive, labour-demanding and time-point measurements (Lakso et al., 2022). Nowadays, the information related to the use of Ψtrunk for irrigation management purposes is scarce, and the few available studies deal with irrigation scheduling based on farmer experience (Pagay, 2022) or ETc requirements (Blanco and Kalcsits, 2021).
In our experiment, automated irrigation, based on MAD threshold values fed by real-time θv measurements with capacitance probes, has been successfully implemented for drip-irrigated nectarine trees grown in a semi-arid Mediterranean environment. As confirmed in previous studies, significantly higher water, energy and labour savings were achieved using this MAD-based irrigation protocol compared to conventional irrigation scheduling based on calculated crop evapotranspiration (ETc), not only without penalising yield but also improving nectarine fruit quality (Conesa et al., 2019; Conesa et al., 2021; Vera et al., 2019; Vera et al., 2021). In this field experiment, a quite different postulate was applied, in which MAD were managed to reach different soil water deficit conditions, and thus θv in the active root zone (0-0.5 m) were remained close to FC values during the first period (α=10%), decreased to 21.5% during α=50% period, and barely reached 17% at the end of the withholding irrigation period (α=100%). These θv values were indicative of well-irrigated, mild and severe soil water deficit conditions, respectively (Figure 1B). Since θv sets the upper/lower interval of the available soil water, θv variations were due not only in response to irrigation or rainfall events, but also to root water uptake dynamics and, to a lesser extent, diurnal environmental changes. In fact, θv dynamics had been closely related to evapotranspiration demand, confirming the sensitivity of capacitance sensors to the nearby environment of soil and plant roots (Mira-García et al., 2021).
Water potentials in the soil-plant-atmosphere continuum (SPAC) provide a physical basis for a comparable quantification of water status. During the summer in the northern hemisphere, the agrometeorological measurements were typical of Mediterranean semi-arid climates (Lionello et al., 2023). Of these, air water potential (Ψa) was calculated as an environmental indicator (Figure 2A), behaving similarly to VPD (Figure 1A), showing a higher day-to-day variability. However, Ψa gives an indication of the water potential allowing water flow along the soil-plant path.
Soil water status, estimated by soil matric potential (Ψm), also correlated with the irrigation protocol applied (Figure 1B). However, under non-irrigated conditions (α=100%), the soil sensors reached their maximum allowed reading (-200 kPa), which mirrored a significant limitation of these soil water sensors under severe water stress conditions (Figure 2C). Thompson et al. (2006) reported the best performance of these granular matrix sensors when used in wet soil (-10 to -50 kPa). Also, the pattern of Ψm at both soil depths (0.3 and 0.6 m) remained almost constant during the daily courses studied (Figures 6G–I), highlighting the drawback of these soil water sensors in identifying diurnal changes because of root water uptake.
Plant water potentials understandably reflected the MAD-based irrigation criteria, evaporative demand and radiation changes that occurred throughout the day (Figures 2B, 6D–F). The values of Ψleaf measured at predawn during the diurnal courses, which decreased as stress accumulated (from -0.35 to -0.8 MPa) (Figures 6D–F), agreed with those obtained in deficit irrigated peach trees by Girona et al. (1993). This valued plant-based measurement, taken at night when there is little or no transpiration, gives an indication of the integrated water status of the soil around the roots (Schmidt and Gaudin et al., 2017), based on the idea that when the plant does not transpire, there is a balance between soil and plant water status. However, there can be erroneous values if there are large variations in soil water levels within the profile (Améglio et al., 1999).
The values of Ψleaf showed the highest variability of the plant water potentials studied (Figures 2B, 6D–F). This is because it is determined on non-cover sunlit leaves, highly dependent upon leaf conductance values and evaporative demand conditions existing at the time of the measurements (García-Tejara et al., 2021). In this sense, Ruiz-Sánchez et al. (2000) found a strong relationship between leaf insertion angle (LIA) and Ψleaf in apricot trees, so that the variability in Ψleaf caused by changes in leaf orientation allows a lower incidence of solar radiation, and a reduction in water loss and leaf heating (Sánchez-Blanco et al., 1994), which makes sunny leaves sensitive to the time of sun exposure. Consequently, Ψstem, measured on covered leaves, is considered the standard measure to determine tree water status in fruit trees (Shackel et al., 1997). Since leaf transpiration is prevented, the Ψstem roughly represents soil water status, and behaved more stable than Ψleaf (Figures 2B, 6D–F).
In the present study, both Ψtrunk and Ψstem were strongly correlated (R2 = 0.86, p<0.001), as they provided similar data of plant water path (Figure 4A). Blanco and Kalcsits (2021) found similar correlations with a coefficient of determination up to 0.8 in pear trees. However, the relationship between Ψtrunk vs. Ψleaf was not significant, highlighting the higher Ψleaf variability and the weakness of this indicator of plant water status (Figure 4B).
Seasonal values of Ψstem and Ψtrunk averaged -0.83 and -0.73 MPa, respectively, during the period of α=10% (Figure 2B), coinciding with the postharvest period in nectarine trees. These values corresponded to non-limiting soil water conditions (Naor et al., 2005; Abrisqueta et al., 2015; De la Rosa et al., 2016; Conesa et al., 2019; Vera et al., 2019, Conesa et al., 2021). As expected, the minimum values of Ψstem (-2.04 MPa) and Ψtrunk (-1.74 MPa) were observed at the end of the non-irrigation period (α=100%) (Figure 2B). Blanco and Kalcsits (2021) reported that MTs can accurately assess plant water status within the range of -0.2 to -2.1 MPa of Ψtrunk values in pear trees. Our findings showed a mean gradient of 0.3 MPa between Ψstem and Ψtrunk (Figures 2B, 6D–F, 7), with slight differences during the experiment. Also, the gradient between Ψleaf and Ψtrunk was higher than that between Ψstem and Ψtrunk (mean values of ≈ 1.8 MPa), indicative of the high hydraulic resistance between trunk and leaves (Pagay, 2022).
It is noteworthy that when seasonal data of plant and soil water potentials were correlated, the most significant relationship was detected between Ψm vs. Ψtrunk (R2 = 0.79, p<0.01) followed by Ψm vs. Ψstem (R2 = 0.62, p<0.05) and it was not significant for Ψm vs. Ψleaf (R2 = 0.26, p>0.05) (Figure 3). Thus, it reveals that Ψtrunk is arguably the most stable indicator of the plant water status, integrating canopy leaves into a stable tissue relatively unaffected by external factors (Lakso et al., 2022; Pagay, 2022).
Leaf gas exchange was also sensitive to MAD-based irrigation criteria (Figure 5). As expected, Pn and gs decreased during the experiment as water deficit accumulated, suggesting a limitation in photosynthetic capacity under water stress condition (Wong et al., 1979). Meanwhile, transpiration efficiency (WUET) tended to increase (Figure 5C). Stomatal closure (Figure 5B) reduced the amount of H2O lost per CO2 assimilated, although, the response of this plant indicator to water stress was decreased by the effect of climatic demand (Figures 5C, 1A). It is also important to note that, despite irrigation recovery, mean values of Pn and gs at this period were lower than those obtained under early summer well irrigated conditions (α=10%). The absence of a full recovery of leaf gas exchange values was motivated by the initiation of leaf senescence typical of deciduous fruit trees (Andersen and Brodbeck, 1988). Furthermore, Conesa et al. (2022) explained the fact that leaf gas exchange levels of water stressed nectarine trees during late postharvest did not recover previous values, after irrigation was restored, by a decrease in the aspartate amino acid in leaves that affected chloroplasts formation.
The hysteresis phenomenon found in the relationships between the two plant water potentials: Ψtrunk vs. Ψstem (Figure 8A), which was more noticeable for Ψtrunk vs. Ψleaf (Figure 8B), was higher for the highest imposed soil water deficit (α=100%). This hysteretic behaviour revealed that the water status of the trunk assumes a dominant role in controlling canopy water status as water stress accumulates, which is related to plant hydraulic conductivity during the daily course (Assouline, 2021). In addition, stomata reopened in the afternoon, as indicated by the recovery values of the diurnal pattern of leaf gas exchange (Figures 9G–I).
No osmotic adjustment was observed in leaves of nectarine trees in response to the applied soil water deficit (Figure 10A). In this regard, Mellisho et al. (2011) in peach trees, and Torrecillas et al. (1999) in apricot trees reported the need to reach Ψleaf and Ψstem below -2.6 and -2.0 MPa, respectively, to activate this tolerance mechanism. Furthermore, it was observed that leaf turgor (Ψt) was maintained, even at α=100% (Figure 10A). In this sense, other drought tolerance characteristics could have taken place, such as high relative apoplastic water content, which would contribute to water retention at low leaf water potentials (Rodríguez et al., 2012).
It is important to note that Ψtrunk values showed the highest signal intensity and sensitivity values for the plant-based water status indicators studied, followed by Ψstem (Table 1). These results emphasise that although Ψtrunk had a higher variability (CV) than Ψstem, it can accurately assess plant water status. Indeed, the S* method (De la Rosa et al., 2014), which decreased the influence of CV in the analysis, showed an increased sensitivity of Ψtrunk. In the same cultivar, Ψstem and canopy to air temperature difference values recorded the highest signal intensity and the Normalised Difference Vegetation Index the highest sensitivity for detecting moderate water deficit situations by mid-July (Conesa et al., 2019).
5. Conclusions
Continuous measurements of trunk water potential (Ψtrunk) using microtensiometers, embedded in the tree trunk, agreed with the automated soil MAD-based irrigation protocols applied to a nectarine orchard. Changes in Ψtrunk explained 79% of the soil matric potential. In fact, Ψtrunk was strongly related to discrete determinations of Ψstem measured with a pressure chamber. A mean gradient of 0.3 MPa was observed between Ψtrunk vs.Ψstem, and 1.8 MPa between Ψtrunk vs.Ψleaf. The greatest variability was found in Ψleaf, due to its dependence on stomatal aperture and evaporative demand conditions. Regarding environmental variables, Ψair showed a high day-to-day variability and a similar dynamic to VPD. Therefore, Ψair could be used in water relations studies in the same terms of water potential as in soil and plant.
Considering that real-time Ψtrunk data allows for automation, further research is needed to determine Ψtrunk threshold values for a successful irrigation decision support system. In addition, the stability, and the long-term performance of trunk microtensiometers needs to be tested.
The promising results found in this work point to the potential use of trunk microtensiometers as novel biosensors to accurately real-time monitor plant water status, and eventually served for precise irrigation scheduling.
Data availability statement
The raw data supporting the conclusions of this article will be made available by the authors, without undue reservation.
Author contributions
Study conception and design were performed by MC, JV and MR-S. Formal analysis and data curation by WC and MC. Software and validation by JV and WC. Project administration and funding acquisition by MR-S. The first draft of the manuscript was written by MC. All authors contributed to the article and approved the submitted version.
Funding
This study was supported by the Spanish State Research Agency [PID2019–106226RB-C2 1/AEI/10.13039/501100011033].
Acknowledgments
MC thanks to the Spanish Juan de la Cierva postdoct programme (FJCI-2017-32045 and IJC2020-045450-I) funded by MCIN/AEI/10.13039/501100011033 and European Union NextGenerationEU/PRTR.
Conflict of interest
The authors declare that the research was conducted in the absence of any commercial or financial relationships that could be construed as a potential conflict of interest.
Publisher’s note
All claims expressed in this article are solely those of the authors and do not necessarily represent those of their affiliated organizations, or those of the publisher, the editors and the reviewers. Any product that may be evaluated in this article, or claim that may be made by its manufacturer, is not guaranteed or endorsed by the publisher.
Abbreviations
Ψair, air water potential; Ψleaf, leaf water potential; Ψπ, leaf osmotic potential; Ψ100π, leaf osmotic potential at full turgor; Ψt, leaf turgor potential; Ψtrunk, trunk water potential; Ψstem, stem water potential; Ψm, soil matric potential; MTs, microtensiometers; Ɵv, volumetric soil water content; ET0, reference crop evapotranspiration; ETc, crop evapotranspiration VPD, vapour pressure deficit; SPAC, soil-plant-atmosphere continuum; MAD, maximum allowable depletion; FC, field capacity; WP, wilting point; α, percent depletion of soil available water; Pn, net photosynthesis; gs, stomatal conductance; E, transpiration rate; WUET, transpiration efficiency; DOY, day of the year; GMT, Greenwich mean time.Ψstem: midday stem water potential (MPa); Ψleaf: midday leaf water potential (MPa); Ψtrunk: midday trunk water potential (MPa); Pn: net photosynthesis (µmol m-2 s-1); gs: stomatal conductance (mmol m-2 s-1); WUET: transpiration efficiency (µmol mmol-1).
References
Abrisqueta, I., Conejero, W., López-Martínez, L., Vera, J., Ruiz-Sánchez, M. C. (2017). Root and aerial growth in early-maturing peach trees under two crop load treatments. Span. J. Agric. Res. 15, e0803. doi: 10.5424/sjar/2017152-10714
Abrisqueta, I., Conejero, W., Valdés-Vela, M., Vera, J., Ortuño, M. F., Ruiz-Sánchez, M. C. (2015). Stem water potential estimation of drip-irrigated early-maturing peach trees under Mediterranean conditions. Comp. Electron. Agric. 114, 7–13. doi: 10.1016/j.compag.2015.03.004
Allen, R. G., Pereira, J. S., Raes, D., Smith, M. (1998). Crop evapotranspiration: guidelines for computing crop water requirements Vol. 56 (Rome (Italy: FAO), 300.
Améglio, T., Archerd, P., Cohen, M., Valancogne, C., Audet, F. A., Dayau, S., et al. (1999). Significance and limits in the use of predawn leaf water potential for tree irrigation. Plant Soil 207, 155–167. doi: 10.10123/A:1026415302759
Andersen, P. C., Brodbeck, B. V. (1988). Water relations and net CO2 assimilation of peach leaves of different ages. J. Am. Soc Hortic. Sci. 113, 242–248. doi: 10.21273/jashs.113.2.242
Assouline, S. (2021). What can we learn from the water retention characteristic of a soil regarding its hydrological and agricultural functions? review and analysis of actual knowledge. Water Res. Res. 57, e2021WR031026. doi: 10.1029/2021WR031026
Black, W. L., Santiago, M., Zhu, S. Y., Stroock, A. D. (2020). Ex situ and in situ measurement of water activity with a MEMS tensiometer. Anal. Chem. 92, 716–723. doi: 10.1021/acs.analchem.9b02647
Blanco, V., Kalcsits, L. (2021). Microtensiometers accurately measure stem water potential. Plants 10, 2780. doi: 10.3390/plants10122780
Casadesús, J., Mata, M., Marsal, J., Girona, J. (2012). A general algorithm for automated scheduling of drip irrigation in tree crops. Comp. Elect. Agric. 83, 11–20. doi: 10.1016/j.compag.2012.01.005
Conesa, M. R., Conejero, W., Vera, J., Agulló, V., García-Viguera, C., Ruiz-Sánchez, M. C. (2021). Irrigation management practices in nectarine fruit quality at harvest and after cold storage. Agric. Water Manage. 243, 106519. doi: 10.1016/j.agwat.2020.106519
Conesa, M. R., Conejero, W., Vera, J., Ramírez-Cuesta, J. M., Ruiz-Sánchez, M. C. (2019). Terrestrial and remote indexes to assess moderate deficit irrigation in early-maturing nectarine trees. Agronomy 9 (10), 630. doi: 10.3390/agronomy9100630
Conesa, M. R., Conejero, W., Vera, J., Ruiz-Sánchez, M. C. (2022). Root reserves ascertain postharvest sensitivity to water deficit of nectarine trees. Agronomy 12, 1805. doi: 10.3390/agronomy12081805
De la Rosa, J. M., Conesa, M. R., Domingo, R., Aguayo, E., Falagán, E., Pérez-Pastor, A. (2016). Combined effects of deficit irrigation and crop level on early nectarine trees. Agric. Water Manage. 170, 120–132. doi: 10.1016/j.agwat.2016.01.012
De la Rosa, J. M., Conesa, M. R., Domingo, R., Pérez-Pastor, A. (2014). A new approach to ascertain the sensitivity to water stress of different plant water indicators in extra-early nectarine trees. Sci. Hortic. 169, 147–153. doi: 10.1016/j.scienta.2014.02.021
Dix, M. J., Aubrey, D. P. (2021). Recalibrating best practices, challenges, and limitations of estimating tree transpiration via sap flow. Curr. Forestry Rep. 7, 31–37. doi: 10.1007/s40725-021-00134-x
FAO (2021). The state of food security and nutrition in the world (SOFI) (Rome (Italy: FAO), 240, ISBN: ISBN: 978-92-5-13425-8
FAOSTAST (2022) Food and agriculture organization statistical data. Available at: http://www.faco.org/faostat/en/#data/QC (Accessed 7 October 2022).
Fereres, E., Soriano, M. A. (2007). Deficit irrigation for reducing agricultural water use. J. Exp. Bot. 58, 147–159. doi: 10.1093/jxb/erl165
Fernández, J. E. (2014). Plant-based sensing to monitor water stress: Applicability to commercial orchards. Agric. Water Manage. 142, 99–109. doi: 10.1016/j.agwat.2014.04.017
Fernández, J. E., Cuevas, M. V. (2010). Irrigation scheduling from stem diameter variations: A review. Agric. For. Meteor. 150, 135–151. doi: 10.1016/j.agrformet.2009.11.006
Fernández-García, I., Lecina, S., Ruiz-Sánchez, M. C., Vera, J., Conejero, W., Conesa, M. R., et al. (2020). Trends and challenges in irrigation scheduling in the semi-arid area of Spain. Water 12, 785. doi: 10.3390/w12030785
García-Tejara, O., López-Bernal, A., Orgaz, F., Testi, L., Villalobos, F. J. (2021). The pitfalls of water potential for irrigation scheduling. Agric. Water Manage. 243, 106522. doi: 10.1016/j.agwat.2020.106522
Girona, J., Mata, M., Goldhamer, D. A., Johnson, R. S., DeJong, T. M. (1993). Patterns of soil and tree water status and leaf functioning during regulated deficit irrigation scheduling in peach. J. Am. Soc Hortic. Sci. 118, 580–586. doi: 10.21273/jashs.118.5.580
Goldhamer, D. A., Fereres, E. (2001). Irrigation scheduling protocols using continuously recorded trunk diameter measurements. Irrig. Sci. 20, 115–125. doi: 10.1007/s002710000034
Gucci, R., Xiloyannis, C., Flores, J. A. (1991). Gas exchange parameters: water relations and carbohydrate partitioning in leaves of field-grown Prunus domestica following fruit removal. Physiol. Plant 83, 497–505. doi: 10.1111/j.1399-3054.1991.tb00126.x
Jones, H. G. (2004). Irrigation scheduling: advantages and pitfalls of plant-based methods. J. Exp. Bot. 55, 2427–2436. doi: 10.1093/jxb/erh213
Katerji, N., Mastrorilli, M., Rana, G. (2008). Water use efficiency of crops cultivated in the Mediterranean regions: Review and analysis. Europ. J. Agron. 28 (4), 493–507. doi: 10.1016/j.eja.2007.12.003
Lakso, A. M., Zhu, A., Santiago, M., Shackel, V., Volkov, A., Stroock., A. D. (2022). “A microtensiometer sensor to continuously monitor stem water potentials in woody plants-design and field testing,” in Proc. IX int. symp on irrigation of horticultural crops, vol. 1335 . Ed. Xiloyannis, C., et al (Acta Hortic), 317–324. doi: 10.17660/ActaHortic.2022.133.39
Lionello, P., Giorgi, F., Rohling, E., Seager, R. (2023). “Chapter 3. Mediterranean climate: past, present and future,” in Oceanography of the Mediterranean Sea. Eds. Schroeder, K., Chiggiato, J. (Elsevier), 41–91, ISBN: ISBN 9780128236925. doi: 10.1016/B978-0-12-823692-5.00011-X
Martínez-Gimeno, M. A., Castiella, M., Rüger, S., Intrigliolo, D. S., Ballester, C. (2017). Evaluating the usefulness of continuous leaf turgor pressure measurements for the assessment of persimmon tree water status. Irrig. Sci. 35, 159–167. doi: 10.1007/s00271-016-0527-3
McCutchan, H., Shackel, K. A. (1992). Stem water potential as a sensitive indicator of water stress in prune trees (Prunus domestica l. cv. French). J. Am. Soc Hortic. Sci. 177), 607–611. doi: 10.21273/jashs.117.4.607
Mellisho, C. D., Cruz, N. Z., Conejero, W., Ortuño, M. F., Rodriguez, P. (2011). Mechanisms for drought resistance in early maturing cv. flordastar peach trees. J. Agric. Sci. 149, 609–616. doi: 10.1017/S0021859611000141
Merriam, J. L. (1966). A management control concept for determining the economical depth and frequency of irrigation. Trans. ASAE 9, 492–498. doi: 10.13031/2013.40014
Millán, S., Casadesús, J., Campillo, C., Moñino, M. J., Prieto, M. H. (2019). Using soil moisture sensors for automated irrigation scheduling in a plum crop. Water 11, e2061. doi: 10.3390/w11102061
Mira-García, A. B., Vera, J., Conejero, W., Conesa, M. R., Ruiz-Sanchez., M. C. (2021). Evapotranspiration in young lime trees with automated irrigation. Sci. Hortic. 288, 110396. doi: 10.1016/j.scienta.2021.110396
Naor, A., Stern, R., Peres, M., Greenblat, Y., Gal, Y., Flaishman, M. A. (2005). Timing and severity of postharvest water stress affect following year productivity and fruit quality of field-grown ‘Snow queen’ nectarine. J. Amer. Soc Hortic. Sci. 130, 806–812. doi: 10.21273/jashs.130.6.806
Navarro, A., Portillo-Estrada, M., Ceulemans, R. (2020). Identifying the best plant water status indicator for bio-energy poplar genotypes. GCB Bioenergy. 12, 426–444. doi: 10.1111/gcbb.12687
Nobel, P. (1983). Biophysical plant physiology and ecology (New York, USA: W.H. Freeman and Company), 608.
Ortuño, M. F., Conejero, W., Moreno, F., Moriana, A., Intrigliolo, D. S., Biel, C., et al. (2010). Could trunk diameter sensors be used in woody crops for irrigation scheduling? a review of current knowledge and future perspectives. Agric. Water Manage. 97, 1–11. doi: 10.1016/j.agwat.2009.09.008
Osroosh, Y., Peters, R. T., Campbell, C. S., Zhang, Q. (2016). Comparison of irrigation automation algorithms for drip-irrigated apple trees. Comp. Elect. Agric. 128, 87–99. doi: 10.1016/j.compag.2016.08.013
Padilla-Díaz, C. M., Rodríguez-Domínguez, C. M., Pérez-Martín, A., Montero, A., García, J. M., Fernández, J. E. (2018). Scheduling a deficit irrigation strategy from leaf turgor measurements: Impact on water status, gas exchange and oil yield. Acta Hortic. 1199, 267–272. doi: 10.17660/ActaHortic.2018.1199.41
Pagay, V. (2022). Evaluating a novel microtensiometer for continuous trunk water potential measurements in field-grown irrigated grapevines. Irrig. Sci. 40, 45–54. doi: 10.1007/s00271-021-00758-8
Pagay, V., Santiago, M., Sessoms, D. A., Huber, W. J., Vincent, O., Pharkya, A., et al. (2014). A microtensiometer capable of measuring water potential below -10 MPa. Lab. Chip. 14, 2806–2817. doi: 10.1039/c4lc00342j
Rodríguez, P., Mellisho, C. D., Conejero, W., Cruz, Z. N., Ortuño, M. F., Galindo, A., et al. (2012). Plant water relations of leaves of pomegranate trees under different irrigation conditions. Environ. Exp. Botany. 77, 19–24. doi: 10.1016/j.envexpbot.2011.08.018
Romero, R., Muriel, J. L., García, I., Muñoz-de la Peña, D. (2012). Research on automatic irrigation control: State of the art and recent results. Agric. Water Manage. 114, 59–66. doi: 10.1016/j.agwat.2012.06.026
Ruiz-Sánchez, M. C., Domingo, R., Castel, J. R. (2010). Review. deficit irrigation in fruit trees and vines in Spain. Span. J. Agric. Res. 8 (S2), 5–20. doi: 10.5424/sjar/201008s2-1343
Ruiz-Sánchez, M. C., Domingo, R., Torrecillas, A., Pérez-Pastor, A. (2000). Water stress preconditioning to improve drought resistance in young apricot plants. Plant Sci. 156, 245–225. doi: 10.1111/j.1744-7348.1999.tb00883.x
Sánchez-Blanco, M. J., Alarcón, J. J., Planes, A., Torrecillas, A. (1994). Differential flood stress resistance of two almond cultivars based on survival, growth and water relations as stress indicators. J. Hortic. Sci. 69, 947–953. doi: 10.1080/14620316.1994.11516531
Schmidt, J. E., Gaudin, A. M. C. (2017). Toward an integrated root ideotype for irrigated systems. Trends in Plant Science 22 (5), 433–443. doi: 10.1016/j.tplants.2017.02.001
Shackel, K. A., Ahmadi, H., Biasi, W., Buchner, R., Goldhamer, D., Gurusinghe, S., et al (1997). Plant water status as an index of irrigation need in deciduous fruit trees. HortTechnology 7 (1), 23–29. doi: 10.21273/HORTTECH.7.1.23
Smith, D. H., Allen, S. J. (1996). Measurement of sap flow in plant stems. J. Exp. Bot. 47 (12), 1833–1844. doi: 10.1093/jxb/47.12.1833
Starr, J. L., Paltineanu, I. C. (2002). “Capacitance devices,” in Methods of soil analysis. part 4 physical methods. Eds. Dane, J. H., Topp, G. C.(Madison, Wisconsin, USA), 463–474.
Thompson, R. B., Gallardo, M., Aguilera, T., Valdez, L. C., Fernández, M. D. (2006). Evaluation of watermark sensor for use with drip irrigated vegetable crops. Irrig. Sci. 24, 185–2002. doi: 10.1007/s00271-005-0009-5
Torrecillas, A., Galego, R., Pérez-Pastor, A., Ruiz-Sánchez, M. C. (1999). Gas exchange and water relations of young apricot plants under drought conditions. J. Agric. Sci. 132, 445–452. doi: 10.1017/s0021859699006577
Turner, N. C. (1988). Measurement of plant water status by the pressure technique. Irrig. Sci. 9, 289–308. doi: 10.1007/BF00296704
Varela-Ortega, C., Blanco-Gutiérrez, I., Esteve, P., Bharwani, S., Fronzek, S., Downing, T. E. (2016). How can irrigated agriculture adapt to climate change? insights from the guadiana basin in Spain. Reg. Environ. Change 16, 59–70. doi: 10.1007/s10113-014-0720-y
Vera, J., Abrisqueta, I., Conejero, W., Ruiz-Sánchez, M. C. (2017). Precise sustainable irrigation: A review of soil-plant-atmosphere monitoring. Acta Hortic. 1150, 195–200. doi: 10.17660/ActaHortic.2017.1150.28
Vera, J., Conejero, W., Conesa, M. R., Ruiz-Sánchez, M. C. (2019). Irrigation factor approach based on soil water content: A nectarine orchard case study. Water 11, 589. doi: 10.3390/w11030589
Vera, J., Conejero, W., Mira-García, A. B., Conesa, M. R., Ruiz-Sánchez, M. C. (2021). Towards irrigation automation based on dielectric soil sensors. J. Hortic. Sci. Biotech. 96 (6), 696–707. doi: 10.1080/14620316.2021.1906761
Vera, J., de la Peña, J. M. (1994). FERTIGA: Programa de fertirrigación de frutales (Murcia, Spain: CEBAS-CSIC), 69.
Vories, E., Sudduth, K. (2021). Determining sensor-based field capacity for irrigation scheduling. Agric. Water Manage. 250, 106860. doi: 10.1016/j.agwat.2021.106860
Keywords: automated irrigation, SPAC, stem water potential, trunk water potential, Prunus persica (L)
Citation: Conesa MR, Conejero W, Vera J and Ruiz-Sánchez MC (2023) Assessment of trunk microtensiometer as a novel biosensor to continuously monitor plant water status in nectarine trees. Front. Plant Sci. 14:1123045. doi: 10.3389/fpls.2023.1123045
Received: 13 December 2022; Accepted: 25 January 2023;
Published: 15 February 2023.
Edited by:
Jaime Lloret, Universitat Politècnica de València, SpainReviewed by:
Alejandra Navarro, Council for Agricultural and Economics Research (CREA), ItalyJoaquim Bellvert, Institute of Agrifood Research and Technology (IRTA), Spain
Copyright © 2023 Conesa, Conejero, Vera and Ruiz-Sánchez. This is an open-access article distributed under the terms of the Creative Commons Attribution License (CC BY). The use, distribution or reproduction in other forums is permitted, provided the original author(s) and the copyright owner(s) are credited and that the original publication in this journal is cited, in accordance with accepted academic practice. No use, distribution or reproduction is permitted which does not comply with these terms.
*Correspondence: María R. Conesa, mrconesa@cebas.csic.es