- 1Rice Department, Thailand Rice Science Institute, Ministry of Agriculture and Cooperatives (MOAC), Suphan Buri, Thailand
- 2Ubon Ratchathani Rice Research Center, Rice Department, Ministry of Agriculture and Cooperatives (MOAC), Ubon Ratchathani, Thailand
Introduction: The anatomy of rice leaves is closely related to photosynthesis and grain yield. Therefore, exploring insight into the quantitative trait loci (QTLs) and alleles related to rice flag leaf anatomical and vein traits is vital for rice improvement.
Methods: Here, we aimed to explore the genetic architecture of eight flag leaf traits using one single-locus model; mixed-linear model (MLM), and two multi-locus models; fixed and random model circulating probability unification (FarmCPU) and Bayesian information and linkage disequilibrium iteratively nested keyway (BLINK). We performed multi-model GWAS using 329 rice accessions of RDP1 with 700K single-nucleotide polymorphisms (SNPs) markers.
Results: The phenotypic correlation results indicated that rice flag leaf thickness was strongly correlated with leaf mesophyll cells layer (ML) and thickness of both major and minor veins. All three models were able to identify several significant loci associated with the traits. MLM identified three non-synonymous SNPs near NARROW LEAF 1 (NAL1) in association with ML and the distance between minor veins (IVD) traits.
Discussion: Several numbers of significant SNPs associated with known gene function in leaf development and yield traits were detected by multi-model GWAS performed in this study. Our findings indicate that flag leaf traits could be improved via molecular breeding and can be one of the targets in high-yield rice development.
Introduction
Genome-wide association studies (GWAS) have been developed into a powerful tool in underpinning the genetic architecture of complex and agriculturally important traits in many major crop species, including rice (Oryza sativa L.). Leaf shape, size, and thickness determine the leaf’s photosynthetic capability and have a great impact on yield, disease resistance, and stress responses in crops (Tsukaya, 2005; Pérez-Pérez et al., 2010; Wang et al., 2011; Liu et al., 2014; Fan et al., 2015). Therefore, improving leaf traits is a very important target in rice breeding.
Plants establish their leaf function via the precise spatial specification of specialized cell types. In rice, the outer leaf comprises epidermal pavement cells, stomatal pores, bulliform cells located at the adaxial epidermis, and trichomes, whereas the inner leaf consists of photosynthetic mesophyll cells and a network of vasculature enclosed by bundle sheaths of parenchyma cells. Rice leaf originates as lateral outgrowths from the leaf primordial cells in the shoot apical meristem (SAM), requiring a synchronized developmental process. Leaf shape is determined through cell division and expansion changes during the leaf proximodistal axis formation, tissue differentiation, and specification (Itoh et al., 2005).
The rice flag leaf has been indicated as one of the top two leaves of a rice plant that contributes over 80% of the total assimilates of rice grains (Tomoshiro et al., 1983; Gladun and Karpov, 1993; Fujii and Saka, 2001; San-oh et al., 2004). In modern rice breeding, a narrow and short leaf rather than a wide and long leaf is preferable considering the efficiency in light perception, as a short and narrow leaf has been indicated to associate more with the small leaf angle thus erect leaf type (Vangahun, 2012). The erect flag leaf can enhance light capture, boosting photosynthetic activity and yield (Yoshida, 1972; Sinclair and Sheehy, 1999). However, longer and lower stomatal density leaves tend to be more desirable and perform better under drought conditions (Kumar et al., 2021).
Leaf thickness is one of the anatomical traits that has been considered a key index in high-yield potential rice breeding (Yoshida, 1972; Peng et al., 1994; Peng, 2000). Thick leaves usually have high chlorophyll and Rubisco content together with high mesophyll conductance affecting CO2 diffusion in the leaves (Evans and Loreto, 2000; Terashima et al., 2001; Murchie et al., 2002; Li et al., 2011; Narawatthana, 2013). Consequently, flag leaf thickness influences the net photosynthetic rate and is positively correlated with rice grain yield (Cook and Evans, 1983; Peng, 2000; Wu et al., 2009; Takai et al., 2010; Liu et al., 2014). Compared with leaf length and width, rice leaf thickness was scarcely studied since direct measurement of leaf thickness is extremely challenging and laborious. Some indirect measurements; e.g., leaf dry matter percentage, specific leaf weight (mass per area), and specific leaf area (area per mass) had been commonly applied as a proxy in determining the leaf thickness in rice (Kanbe et al., 2008; Takai et al., 2010; Liu et al., 2014; Hoang et al., 2019).
Recently, leaf venation architecture in rice has been focused on as one of the important leaf anatomical characteristics influencing leaf gas exchange and hydraulic conductance. Vein density and size were positively correlated with leaf hydraulic conductance thus improving leaf CO2 assimilation efficiency which is vital for improving photosynthesis (Tabassum et al., 2016; Pitaloka et al., 2021; Ye et al., 2021). Rice leaf veins are different in size and functions and, apart from the midrib, are divided into two categories (major and minor veins) based on their size. Leaf venation architecture: i.e., vein arrangement, density, size, and geometry of xylem and phloem vessels, has enormous variation and contributes to different leaf performances (Sack and Scoffoni, 2013). Although leaf venation and leaf thickness can greatly affect photosynthetic efficiency, these traits have not been set as a major target in rice breeding programs due to lacking their genetic background.
Genes and quantitative trait loci (QTLs) related to leaf morphogenesis in rice regulate leaf shape through cell division and differentiation as well as the signaling pathways of phytohormone and transcription factors. Many genes and QTLs involved in the developmental processes of leaf morphogenesis have been identified and characterized by mutant analysis. The rice dwarf mutant named narrow leaf 1 (nal1) exhibits reduced polar auxin transport capacity leading to decreased leaf width and a defective vascular system (Qi et al., 2008). Consequently, qFW4-2, a QTL for flag leaf width identified in a chromosomal segment substitution line (CSSL) population, was also mapped to the location corresponding to NAL1 (Tang et al., 2018). Tang et al. (2018) confirmed the function of the gene GRAIN NUMBER, PLANT HEIGHT, AND HEADING DATE 7.1 (GHD7.1) in increasing flag leaf size and photosynthetic capacity thus improving yield potential. Furthermore, a genome-wide association study (GWAS) conducted using a panel of 529 rice accessions indicated that GHD7 and NAL were the major loci controlling the natural variation of chlorophyll content in rice leaves (Wang et al., 2015). Recently, GWAS was carried out for 29 rice leaf traits related to leaf size, shape, and color and unraveled several QTLs (Yang et al., 2015).
To date, gene mapping and GWAS can provide the most comprehensive investigation for identifying genes in plants for almost all traits which are complex and regulated by many genes and influenced by the environment. However, several false positives could be generated from GWAS due to population structure and family relatedness (Kaler et al., 2020). In the mixed linear models (MLM), controlling the false positives is often performed by incorporating population structure and a kinship matrix as covariates into this type of single locus model (Price et al., 2006). Nonetheless, false negatives can also be introduced by overfitting the model that might exclude some important loci. Recently, multi-locus GWAS analysis methods such as fixed and random model circulating probability unification (FarmCPU) and Bayesian-information and linkage-disequilibrium iteratively nested keyway (BLINK) have been developed to overcome the false-negative problem (Zhang et al., 2019). In this study, three different statistical models both single locus and multi-locus models: MLM, BLINK, and FarmCPU, were compared for GWAS of rice leaf thickness and venation architecture, in a genetically diverse worldwide collection called Rice Diversity Panel 1 (RDP1). The multi-model GWAS was performed to detect an association between SNP and the flag leaf traits.
Materials and methods
Plant material and genotypic data
The Rice Diversity Panel 1 (Reg. No. MP-6, NSL500357 MAP) (RDP1) consists of 421 rice germplasm including both Asian landraces and worldwide elite cultivars obtained from 79 countries representing the major rice growing regions (Eizenga et al., 2014). In this study, we used 329 accessions of the RDP1 containing 141 accessions belonging to the Indica varietal group including the indica (78 accessions), aus (52 accessions), and admixture of Indica (11 accessions) and 188 accessions comprising the Japonica varietal group including the tropical japonica (64 accessions), temperate japonica (87 accessions), an admixture of Japonica (24 accessions), and aromatic (13 accessions) were analyzed. Supplemental Table S1 contains information about the accession name, accession number, original providing country, and sub-population group based on 36 SSRs and 700,000 SNPs (Ali et al., 2011; McCouch et al., 2016).
Leaf anatomical traits and vein architecture measurements
A total of 329 rice accessions of RDP1 were grown in irrigated paddy fields at Thailand Rice Science Institute, Suphan Buri, Thailand (14° 28’ 16.79” N and 100° 07’ 3.60” E) in the wet season from 2017 to 2018. Individual rice seedlings at 20 days old were manually transplanted in 3 rows per accession at a spacing of 25 cm between rows and 25 cm between plants. The N:P: K fertilizer was applied at the rate of 37.5:37.5:37.5 kg ha-1 before transplanting and at the rate of 37.5:0:0 kg ha-1 during the heading stage. Fully expanded flag leaves were collected, and the widest part of the individual leaf was excised in 1 cm long for free-hand sectioning. Leaf sections were cleared with an 85% (w/v) lactic acid in saturated chloral hydrate at 70°C for 1 hour before staining with 0.01% (w/v) toluidine blue in 15% boric acid for 10 seconds. Stained leaf sections were examined under a microscope (IX71, Olympus, Japan) and documented using a digital camera DP73 (Olympus, Japan) for image analysis using Figi (Schindelin et al., 2012). Leaf thickness (LT), major vein thickness (MJVT), minor vein thickness (MNVT), major vein width (MJVW), minor vein width (MNVW), and the number of mesophyll cell layer (ML) were measured as illustrated in Figure 1.
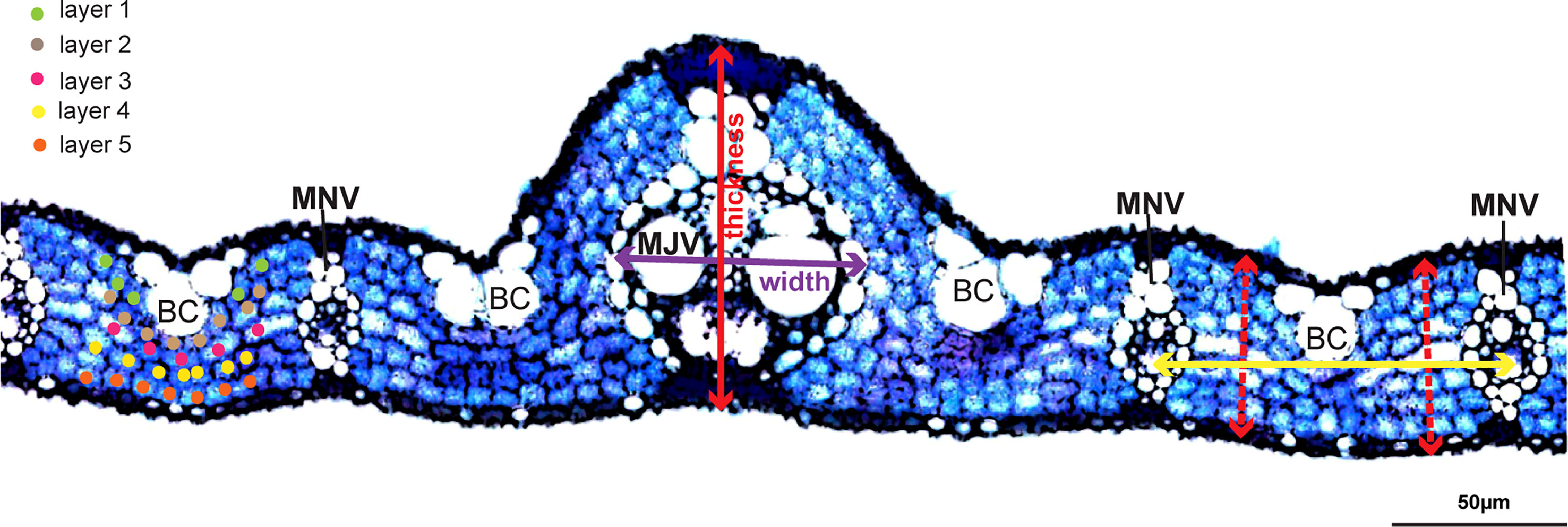
Figure 1 Measurement of rice leaf anatomical and vein traits. Leaf thickness, minor vein (MNV), and major vein (MJV) thickness were measured by drawing a straight line from the top of the upper epidermis to the bottom of the lower epidermis as illustrated using the red lines. Width at minor and major veins was measured along the axis as indicated with the purple line. Different color dots represent different mesophyll cell layers in the counting of mesophyll cell layer numbers. The yellow line shows the inter-veinal distance measurement between 2 adjacent minor veins. BC, Bulliform cells. Scale bar = 50 µm.
Leaf width and length were measured in vivo, and the stained leaf sections were used for major veins and minor veins counting. Vein density or vein length per leaf area (VLA) was calculated as follows (Ye et al., 2021).
Statistical analysis
Phenotypic data were analyzed using R software version 4.1.3 to estimate the means, standard deviations (sd), coefficients of variation (CV), variances, and broad-sense heritability for each trait. The broad-sense heritability (H2) was estimated by using the genotypic and phenotypic variances as follows: H2 = (F-1)/F, where F is the F-value from ANOVA for the genotype factor. Phenotypic correlations among the traits were computed by Pearson’s method, using the ‘psych’ package in R (Revelle and Condon, 2019). Multiple pairwise comparisons were performed using the Kruskal-Wallis test to examine the significant difference among sub-population groups. A pairwise Wilcoxon signed-rank test with Bonferroni correction was conducted to detect a significant difference in between two sub-population groups. All statistical graphs were created using R version 4.1.3 (R Core Team, 2022).
Genome-wide association analysis
The association analysis was conducted using MLM (Yu et al., 2006), BLINK (Huang M. et al., 2019), and FarmCPU (Liu et al., 2016) model using the R software package Genome Association and Prediction Integrated Tool (GAPIT) version 3 (Lipka et al., 2012). The mean of each trait collected over two years was used as input data for GWAS. MLM analysis was applied using the P3D (population parameters previously determined) method without compression. A total of 700,000 SNPs were used for GWAS. The genome-wide significant P value threshold was adjusted based on Bonferroni correction. The SNP-trait associations were declared significant when the P value<1e-07 and the corrected P value< 0.05. Gene annotation was performed on 100 kb of the flanking regions of the associated loci. The Manhattan plots and Q-Q plots were generated using the GAPIT package in R.
Identification of candidate genes
To explore candidate genes underlying leaf anatomical traits and vein architecture identified in this study, the SNPs with p< 0.0001 were considered as genomic regions carrying candidate genes. All gene loci within 100kb of each SNP were extracted from the annotation of the Oryza sativa reference sequence (OsNipponbare-Reference-IRGSP-1.03; http://rapdb.dna.affrc.go.jp/download/irgsp1.html) using the Bioconductor packages in R (Huber et al., 2015). Gene Ontology (GO) terms were retrieved from the BMRF tool (https://www.ab.wur.nl/bmrf). To validate the GWAS results, all annotated gene loci included in the genomic regions were compared with genes known to be related to the phenotypic traits analyzed available in the Oryzabase database (https://shigen.nig.ac.jp/rice/oryzabase/) or QTLs/genes present in literature. LDBlockshow (Dong et al., 2021) was used to estimate the local linkage disequilibrium (LD) block on 100 kb of the genomic region containing significant SNPs. The haplotype analysis was conducted on selected candidate genes using all SNPs within the gene coding region using Haploview 4.1 software (Barrett et al., 2005). The haplotype contains at least 10 accessions was considered a major haplotype. Kruskal-Wallis test and pairwise Wilcoxon signed-rank test with Bonferroni correction were performed to examine the significant difference in phenotypic variation among haplotypes.
Results
Phenotypic variation and heritability of the leaf anatomical traits and vein architecture
We measured 8 leaf and vein traits in the 329 accessions of RDP1 over two years. These traits can be categorized into 2 major groups: leaf anatomical traits (leaf thickness, number of mesophyll cell layer, and inter-veinal distance), and vein traits (major vein thickness, minor vein thickness, major vein width, minor vein width, and vein density). All the traits exhibited high values of broad-sense heritability (H2), ranging from 0.743 to 0.996 (Table 1). Heritability for mesophyll cell layer and vein density was very high in the RDP1 sub-population (0.994 and 0.996). The results revealed the extensive variation in leaf anatomical and vein traits under strong genetic control among the rice accessions. The coefficient of variance (CV) of these traits varied from 9.7% to 51.53% and VLA showed the strongest variation.
The phenotypic correlations among the leaf anatomical and vein traits were analyzed (Figure 2). LT, ML, and MNVT were strongly positively correlated, with correlation coefficients over 0.85 (P< 0.001), among themselves. These results indicate that rice leaf thickness is enormously influenced by the number of mesophyll cell layers and minor vein size. Here, we demonstrate a very strong positive correlation in thickness and width among major and minor veins of the RDP1 sub-population. MJVT, MJVW, and MNVW showed a relatively strong positive relationship with LT and ML, albeit less correlated than observed for MNVT. The previous report has found that thick rice leaves are supported by wider minor veins and thin rice leaves are supported by narrow minor veins (Chatterjee et al., 2016). Contrary to the previous report (Chatterjee et al., 2016), we found no significant relationship between IVD and VLA in this population. However, VLA was negatively correlated with MJVW and MNVW, while a strong positive relationship between IVD and both minor and major veins was detected. Notably, the vein density of the RDP1 sub-population tends to be negatively related to vein size, number of the mesophyll cell layer, and leaf thickness. Given our finding that high vein density positively relates to thin leaf and narrow veins which also correlate to a smaller veins space, it can be hypothesized that narrow or smaller leaf vein is a prerequisite for high vein density in rice leaves. An increase in vein density in rice leaves due to alteration in vein diameter and a change in mesophyll cell size or number were reported (Smillie et al., 2012; Feldman et al., 2014; Chatterjee et al., 2016).
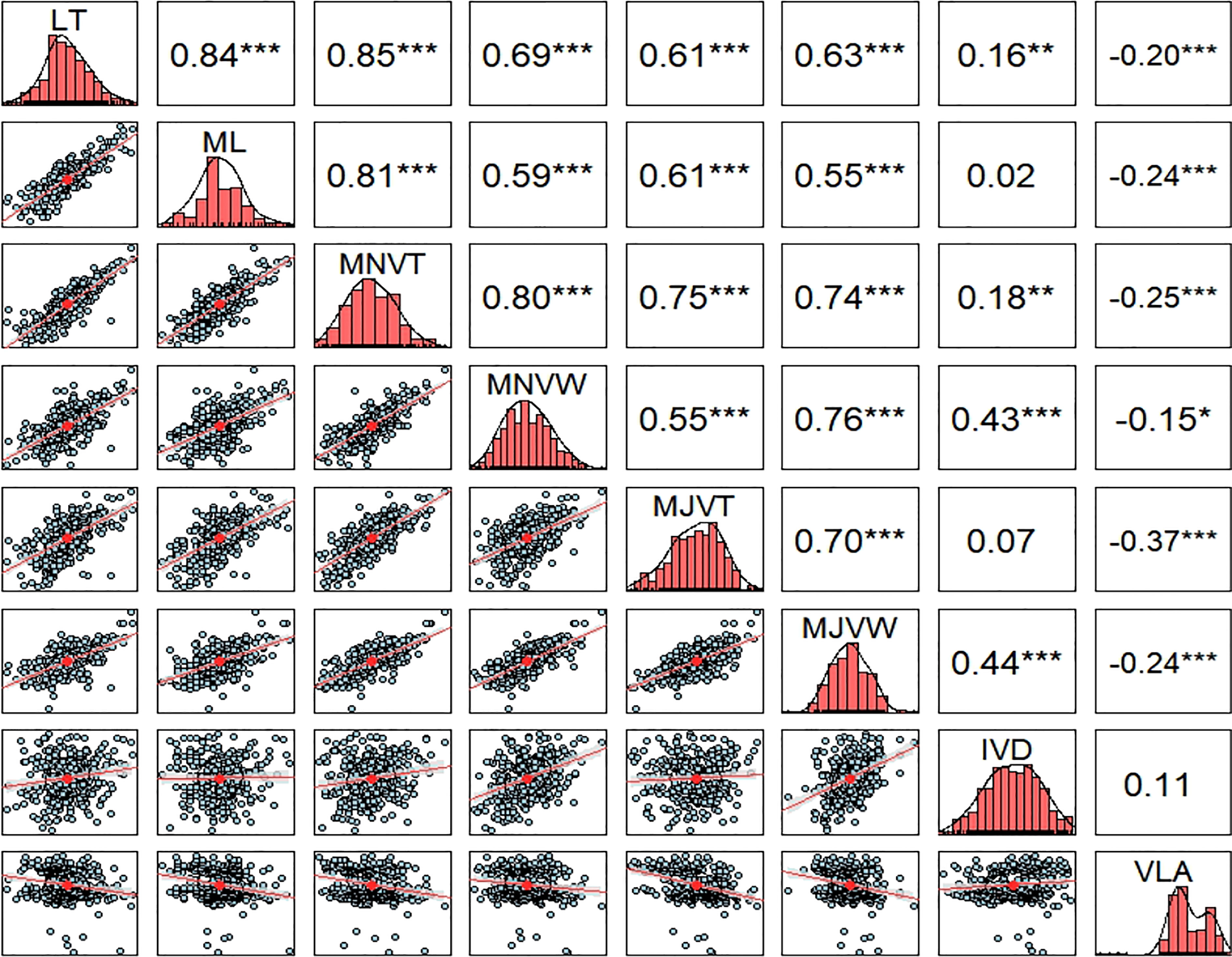
Figure 2 Pearson correlation coefficients between leaf and vein traits across all the rice accessions of the RDP1 subpopulation. The diagonal represents the density histogram of the trait. Asterisks indicate a significant level of Pearson correlations (*< 0.01, **< 0.005, ***< 0.001), n = 329 varieties. LT, leaf thickness; ML, number of mesophyll layers; MJVT, major vein thickness; MJVW, major vein width; MNVT, minor vein thickness; MNVW, minor vein width; IVD, inter-veinal distance; VLA, vein length per leaf area.
The RDP1 has five major sub-populations: i.e., indica (IND), aus (AUS), tropical japonica (TRJ), temperate japonica (TEJ), and aromatic (ARO) and admixture (ADMIX). Classification of LT according to sub-populations indicated significant differences between TRJ and TEJ while there was no significant difference in LT between TEJ and IND (Figure 3A). ADMIX, ARO, and TRJ had no significant differences in LT. For ML which showed an extremely positive relationship to LT, we observed a similar pattern of ML variation among the RDP1 sub-populations as in LT (Figure 3B). We found that the distances in between 2 adjacent minor veins of IND and TRJ were significantly lower than the other sub-population groups (Figure 3C). The variation in major vein; both MJVT and MJVW, of RDP1 observed in our work are well depicted in Figures 3D, E. The MJVT of TEJ was the lowest and significantly different from the others (Figure 3D). For the thickness of minor veins, TEJ together with IND were also lower than the other sub-population groups (Figure 3F), which is corresponded with the LT and ML results. TEJ and IND were also exhibited the significantly lower MNVW than the other groups as shown in (Figure 3G). When vein density was classified according to the sub-population groups, we found that the VLA of TEJ was extensively high and significantly different from all the other groups (Figure 3H). We observed that there was no statistically significant difference between TRJ, ARO, and ADMIX in all the traits. Here, the leaf shape of TRJ, ADMIX, and ARO sub-population tend to be thick leaves with thick and wide major and minor veins and low vein density.
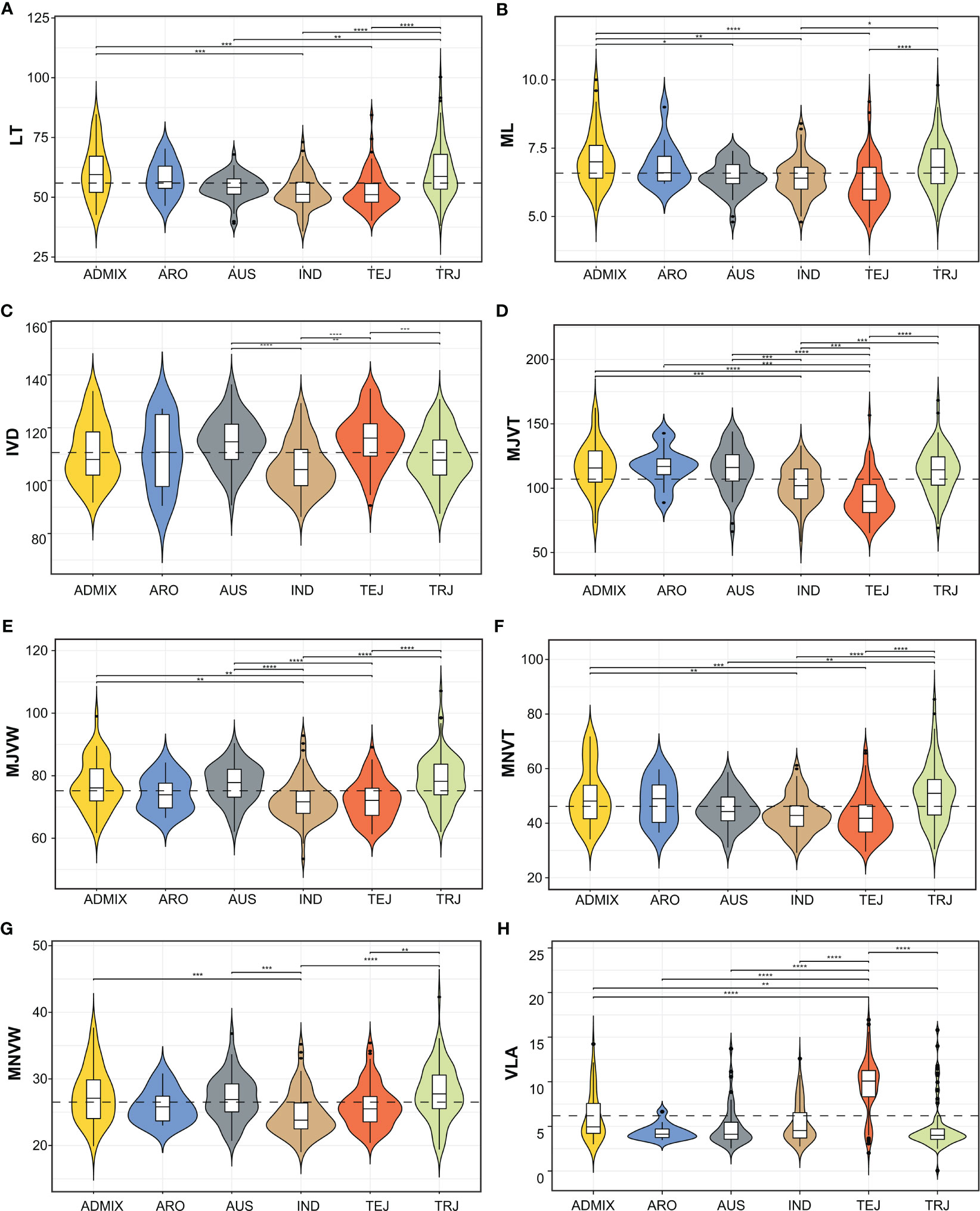
Figure 3 Violin plots of the distribution of leaf anatomical and vein traits. The dotted line represents the mean of each trait. (A) leaf thickness (LT), (B) number of mesophyll layers (ML), (C) inter-veinal distance (IVD), (D) major vein thickness (MJVT), (E) major vein width (MJVW), (F) minor vein thickness (MNVT), (G) minor vein width (MNVW), (H) vein length per leaf area (VLA). Asterisks indicate significant differences between sub-populations groups according to pairwise comparisons using Wilcoxon signed-rank test with Bonferroni correction (**¾ 0.001, ***< 0.0001, ****< 0.00001). ADMIX, admixture; ARO, aromatic; AUS, aus; IND, indica; TEJ, temperate japonica; TRJ, tropical japonica.
Multi-model GWAS analysis of leaf anatomical and vein traits
GWAS analysis for leaf anatomical and vein traits in RDP1 using three GWAS models: BLINK, FarmCPU, and MLM, was performed by the R-based GAPIT program. In our study, a threshold of the genome-wide significance at 7 x 10-8 and a false discovery rate (FDR) cut-off with an alpha of 0.05 were determined. In almost all the traits, MLM, the only single locus method used here, predicted the highest number of significant single nucleotide polymorphisms (SNPs), especially in ML traits (Figure 4). The multi-locus models i.e., BLINK and FarmCPU, which are usually employed to reduce false positives in GWAS analysis implemented the highest number of significant SNPs in MJVT and LT, respectively. It is noted that there were no significant SNPs implemented by MLM in either LT or MJVT traits. Moreover, BLINK and MLM were the only models that identified the significant SNPs associated with MJVT and VLA, respectively.
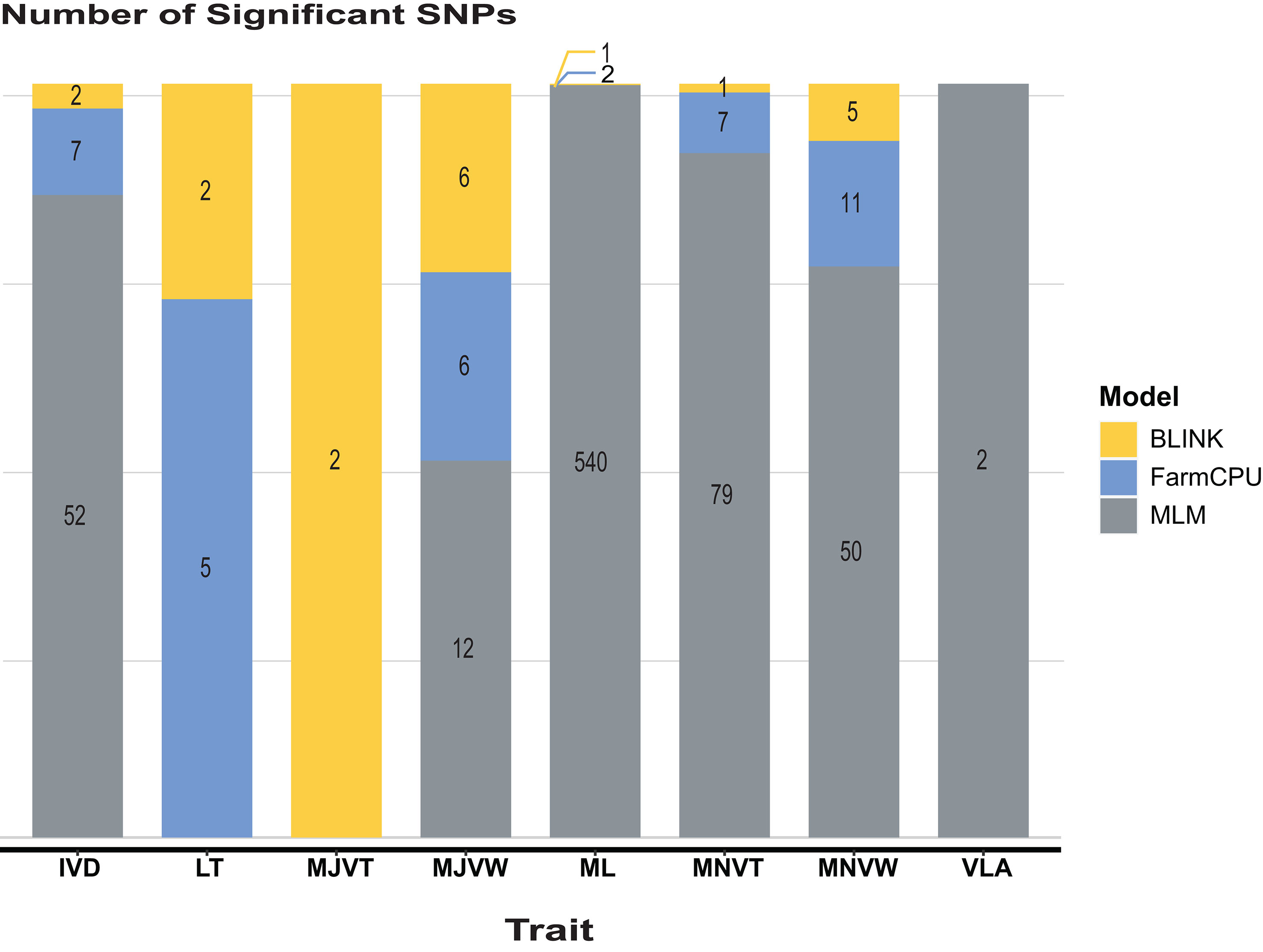
Figure 4 The number of significant SNPs identified within the RDP1. Models compared are Bayesian information and linkage-disequilibrium iteratively nested keyway (BLINK), fixed and random model circulating probability unification (FarmCPU), and mixed-linear model (MLM). IVD, inter-veinal distance; LT, leaf thickness; MJVT, major vein thickness; MJVW, major vein width; ML, number of mesophyll layers; MNVT, minor vein thickness; MNVW, minor vein width; VLA, vein length per leaf area.
According to the Q-Q plots, FarmCPU and BLINK models had a straight line with a sharp upward deviated tail which implied that both false positives and false negatives were efficiently controlled in almost all the traits, especially in LT and MNVW traits (Figure 5). However, for ML traits, the Q-Q plots for both models deflated downward and most of the SNPs were very close to the identity line reflecting that FarmCPU and BLINK may have been reported false negatives (Figure 5B). Notably, the FarmCPU exhibited strongly inflated P values in both ML and MNVT while the BLINK model inflated the P values in ML and IVD. Regarding ML, MNVT, and VLA which were the highest heritability traits here, the MLM model rather than FarmCPU and BLINK could effectively control both false positives and false negatives as indicated by the straight line with a slightly deviated tail of the Q-Q plots (Figures 5B, H). Q-Q plots of the FarmCPU model for LT, MJVW, MNVW, and IVD followed a straight line of the identity line with a sharp deviated tale implying that FarmCPU was the most powerful model in controlling both false positives and false negatives for the leaf anatomical and vein traits (Figures 5A, C–G). Interestingly, it was only the Q-Q plots of the BLINK model for MJVT which had a straight line close to the identity line with a sharp deviated tail compared to other models.
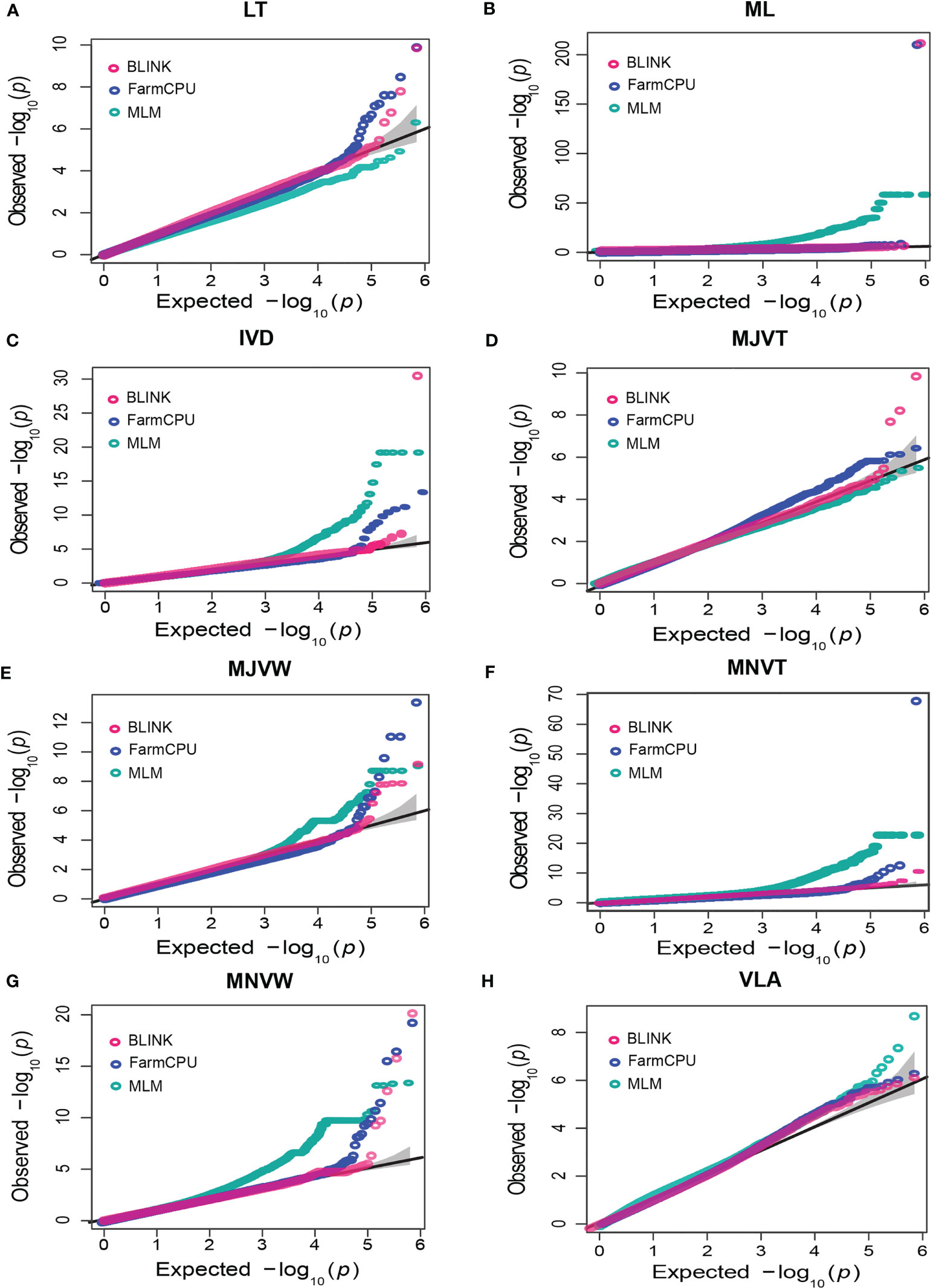
Figure 5 Quantile-quantile (QQ) plots of Bayesian information and linkage-disequilibrium iteratively nested keyway (BLINK), fixed and random model circulating probability unification (FarmCPU), and mixed-linear model (MLM) for leaf anatomical and vein traits. (A) leaf thickness (LT), (B) number of mesophyll layers (ML), (C) inter-veinal distance (IVD), (D) major vein thickness (MJVT), (E) major vein width (MJVW), (F) minor vein thickness (MNVT), (G) minor vein width (MNVW), (H) vein length per leaf area (VLA). The solid black line is the identity line showing the expected null distribution of the P value assuming no associations. The grey area represents the 95% concentration band.
Regarding the FarmCPU model, five SNPs were significantly associated with the leaf thickness trait in rice (Figure 6). Among them, there were two SNPs i.e, LOC_Os07g28280 and LOC_Os02g30730 located on chromosome 7 and chromosome 2 respectively, which were similar to the position of the only two significant SNPs associated with LT identified by the BLINK model. However, the FarmCPU and BLINK models identified the most significant SNPs associated with LT at different positions located on chromosome 2 and chromosome 7, respectively. As we observed the efficiency of the FarmCPU model and the fact that the FarmCPU and the BLINK models identified similar significant loci in many traits (Table 2), a comparison of the FarmCPU model with MLM for MJVW, MNVW, MNVT, and IVD traits were performed (Figure 7). For these traits, the FarmCPU model identified single significant SNPs with higher P values and more precise chromosome positions. MLM showed the lower significant SNPs P values with broader peaks which were containing multiple significant SNPs or SNP clusters in Manhattan plots. Therefore, the number of the significant SNPs identified with the MLM model was larger than with the FarmCPU model. Moreover, the identified significant SNPs from the MLM were different from the position of the significant SNPs identified by the FarmCPU model which strongly inflated the P value. The inflation of the P values implemented by the FarmCPU resulted in a lower number of identified SNPs with the P values smaller than the threshold. We noticed that some of the significant SNPs identified by the MLM model were among the non-significant SNPs implemented by the FarmCPU model.
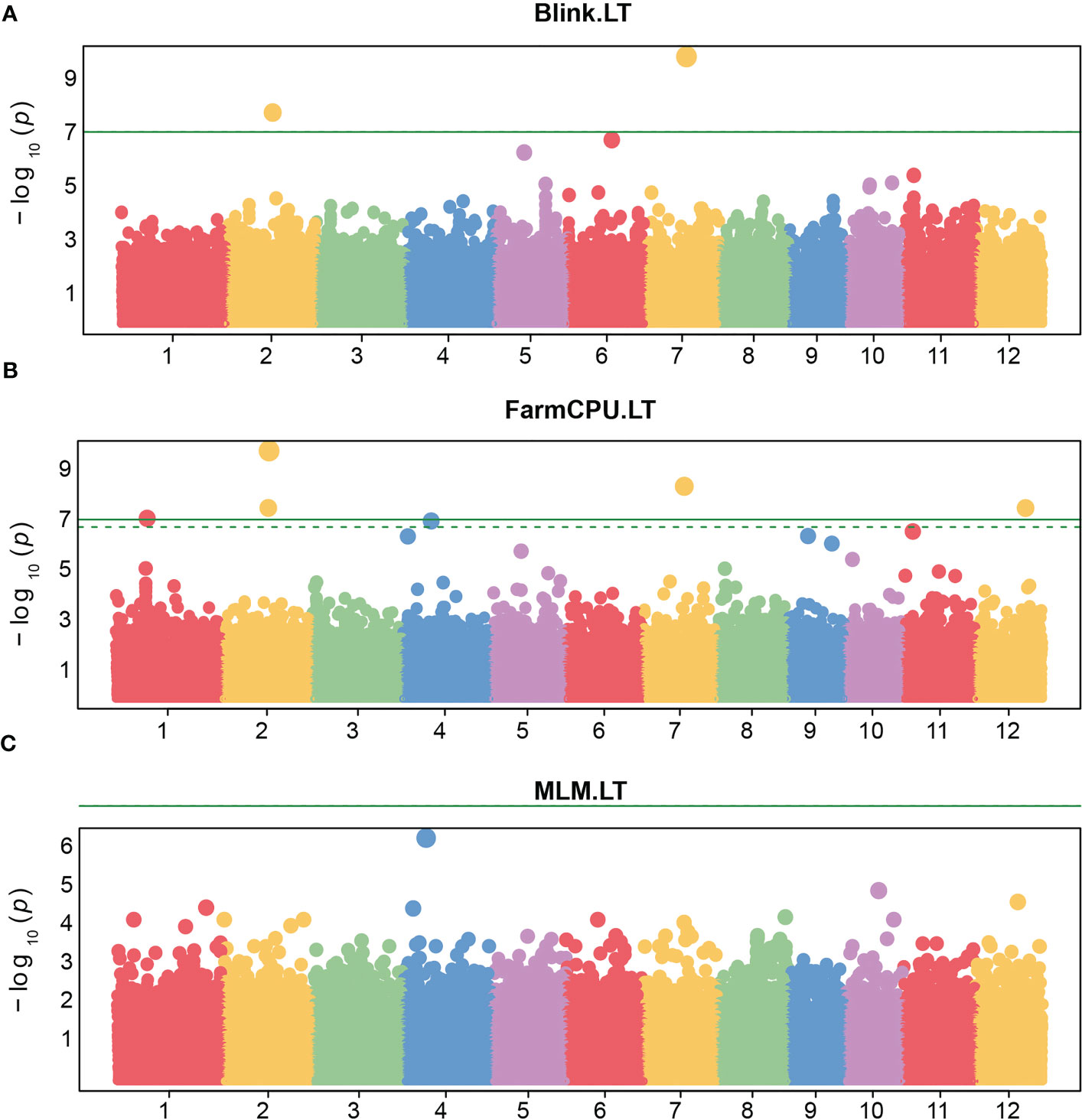
Figure 6 Manhattan plots of -log10 (P value) versus the physical location of SNPs across the 12 chromosomes associated with rice leaf thickness (LT) from the three models including Bayesian information and linkage-disequilibrium iteratively nested keyway (BLINK). (A), fixed and random model circulating probability unification (FarmCPU) (B), and mixed-linear model (MLM) (C). The green horizontal line represents the genome-wide significance threshold of Bonferroni adjusted p-value = 7 × 10−8. The dotted line represents the threshold of false discovery rate adjusted p-value.
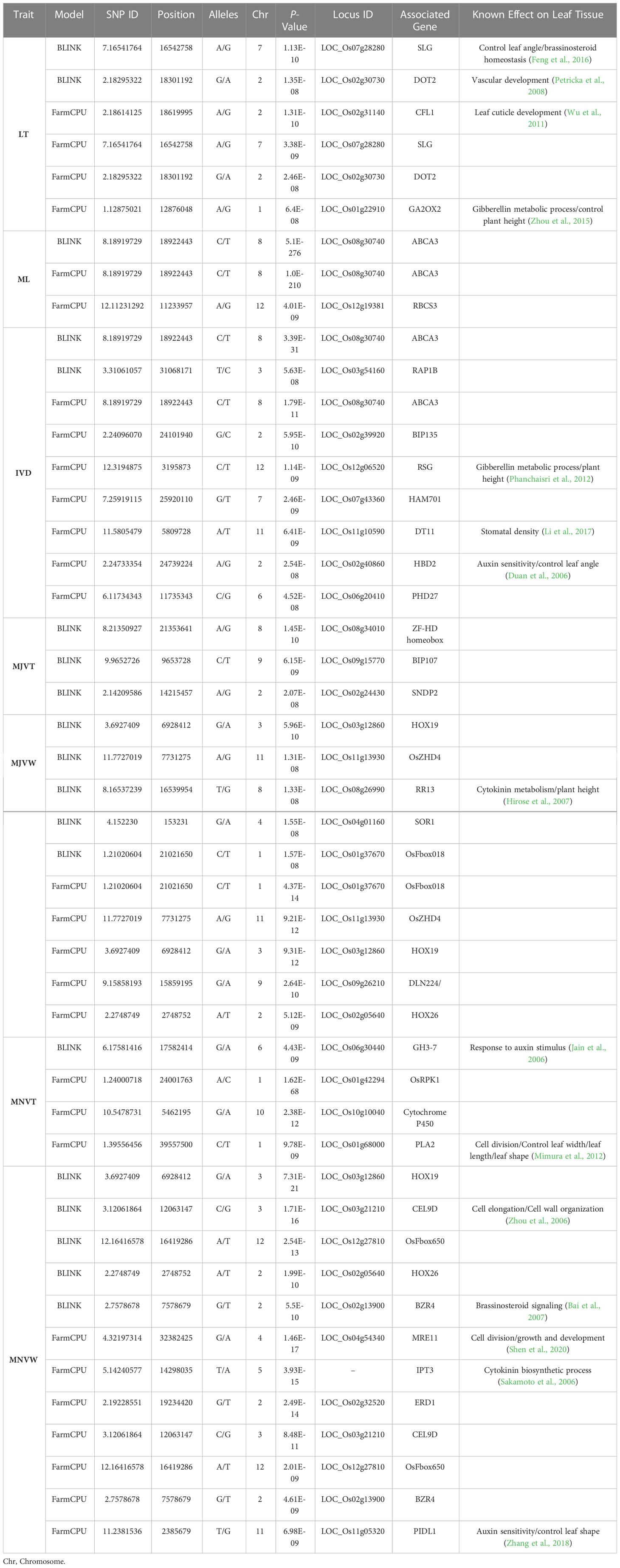
Table 2 Candidate genes for each significant SNP associated with leaf anatomical and vein traits implemented by BLINK and FarmCPU models.
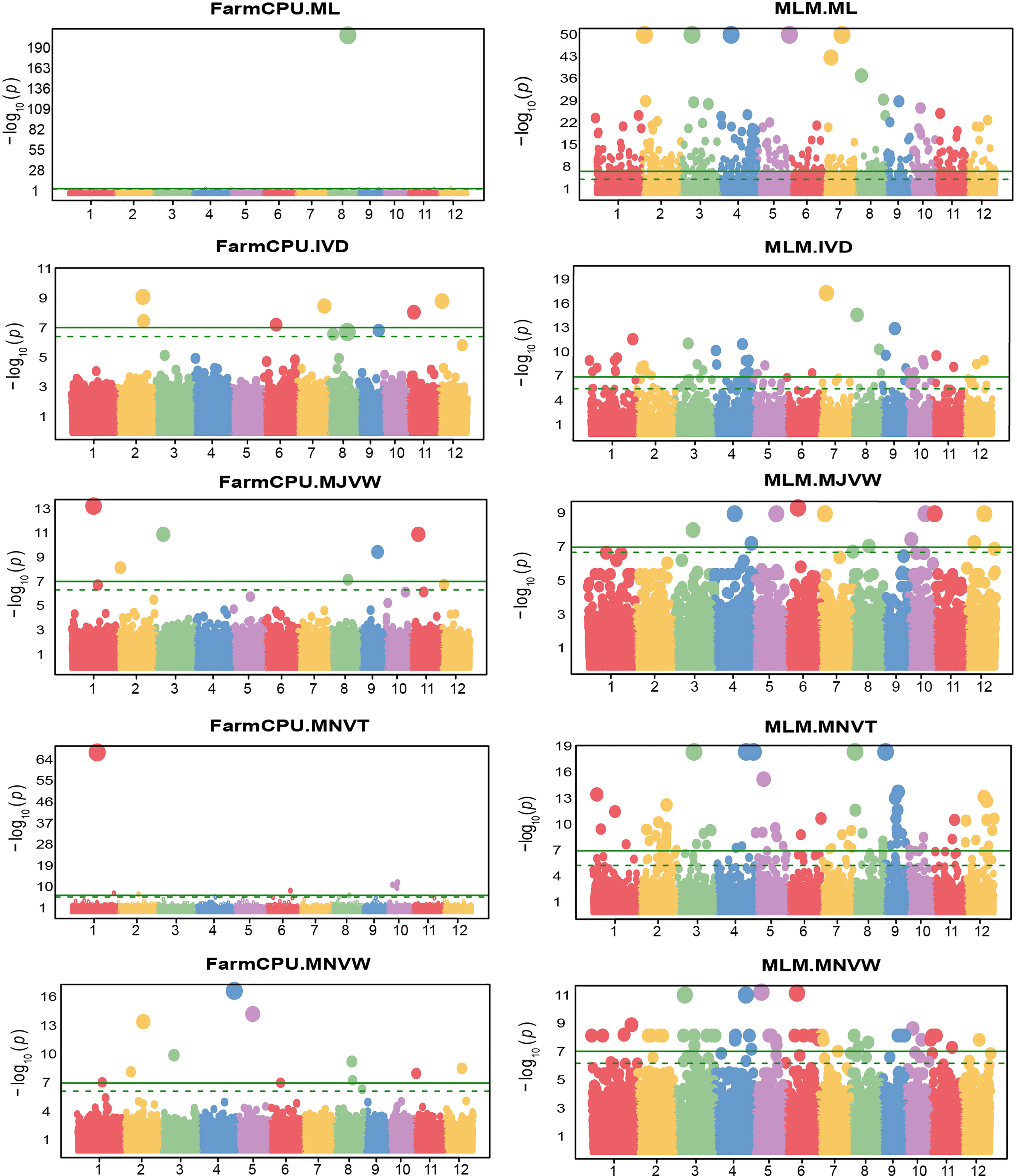
Figure 7 Manhattan plots of -log10 (P value) versus the physical location of SNPs across the 12 chromosomes associated with the number of mesophyll layer (ML), inter-veinal distance (IVD), major vein width (MJVW), minor vein thickness (MNVT), and minor vein width (MNVW) in rice from fixed and random model circulating probability unification (FarmCPU) and mixed-linear model (MLM). The green horizontal line represents the genome-wide significance threshold of Bonferroni adjusted p-value = 7 × 10−8. The dotted line represents the threshold of false discovery rate adjusted p-value.
Identification of candidate genes for the leaf anatomical traits
Candidate genes containing SNPs significantly associated with rice leaf anatomical traits were identified by the gene annotation network RGAP (http://rice.uga.edu/, accessed on 15 January 2022) and the Oryzabase database (Kurata and Yamazaki, 2006). Association analysis using the FarmCPU model identified five significant SNPs associated with LT traits. Among these, the most significant SNP was located on chromosome 2 near CURLY FLAG LEAF 1 (CFL1) which encodes a WW domain protein regulating the cuticle development of leaf epidermal cells (Wu et al., 2011). The second most significant SNP detected by the FarmCPU, which was repeatedly identified by BLINK as the most significant SNP associated with LT, was located near SLENDER GRAIN (SLG) (Table 2). SLG regulates leaf angle and plant height in rice through cell division and expansion which is mediated by brassinosteroid signaling (Feng et al., 2016). A significant SNP located near DEFECTIVELY ORGANIZED TRIBUTARIES 2 (DOT2) was identified by both the FarmCPU and BLINK models. In Arabidopsis thaliana, DOT2 is encoded by At5g16780, which corresponds to an 820-aa leucine zipper protein. DOT2 mutation leads to disruption of vein patterning and an increase in leaf cell number together with a decrease in cell size (Petricka et al., 2008). An SNP on chromosome 1 positioned near GIBBERELLIN 2-OXIDASE 2 (GA2OX2), which is a member of rice gibberellic acid (GA) 2-oxidase family genes, was identified by the FarmCPU model.
For the ML trait, FarmCPU and BLINK implemented 2 and 1 significant SNPs respectively. The significant SNP near ABC TRANSPORTER A FAMILY MEMBER 3 (ABCA3) gene was repeatedly identified by both multi-locus models. In contrast, MLM identified 540 significant SNPs associated with ML that most of the SNP loci associated with known genes functioning in rice leaf development (Table 3). Intriguingly, three non-synonymous SNPs were located within the region of NARROW LEAF 1 (NAL1) which regulates rice leaf shape, leaf width, and vein patterning via cell division and expansion (Zhang et al., 2014; Jiang et al., 2015; Taguchi et al., 2015; Lin et al., 2019). Moreover, another gene that regulates rice leaf width i.e., NARROW LEAF 7 (NAL7) was associated with the significant SNP located on chromosome 3. NAL7 encodes a flavin monooxygenase protein which plays an important role in regulating leaf width, bulliform cell, and vascular bundle development through the auxin signaling pathway (Fujino et al., 2008). We also found a significant SNP in WUSCHEL-RELATED HOMEOBOX4 (WOX4) associated with the ML trait. WOX4 is a transcription factor promoting cell division thus controlling vascular bundle development and leaf width (Ohmori et al., 2013; Yasui et al., 2018). One of the most important candidate genes identified to be associated with this mesophyll cells trait was located on chromosome 4 near ENT-KAURENE SYNTHASE 2 (KS2) gene which encodes a GA metabolic enzyme regulating mesophyll cells development in rice leaf (Ji et al., 2014). The significant locus on chromosome 7 is located within the region of Ghd7.1 or HD2 (Heading date2) which is recently reported as a key gene in the regulation of flag leaf size (Tang et al., 2018). Some of the significant SNPs associated with the number of mesophyll layer trait were in the candidate regions near the reported genes regulating cell division and cell expansion during leaf morphogenesis such as VIRESCENT-ALBINO LEAF1 (VAL1) (Zhang T. et al., 2018), Loose Plant Architecture1 (LPA1) (Wu et al., 2012), RICE MINUTE-LIKE1 (RML1) (Zheng et al., 2016), and CYSTEINE ENDOPEPTIDASE REP-2 or VPE3 (Lu et al., 2016).
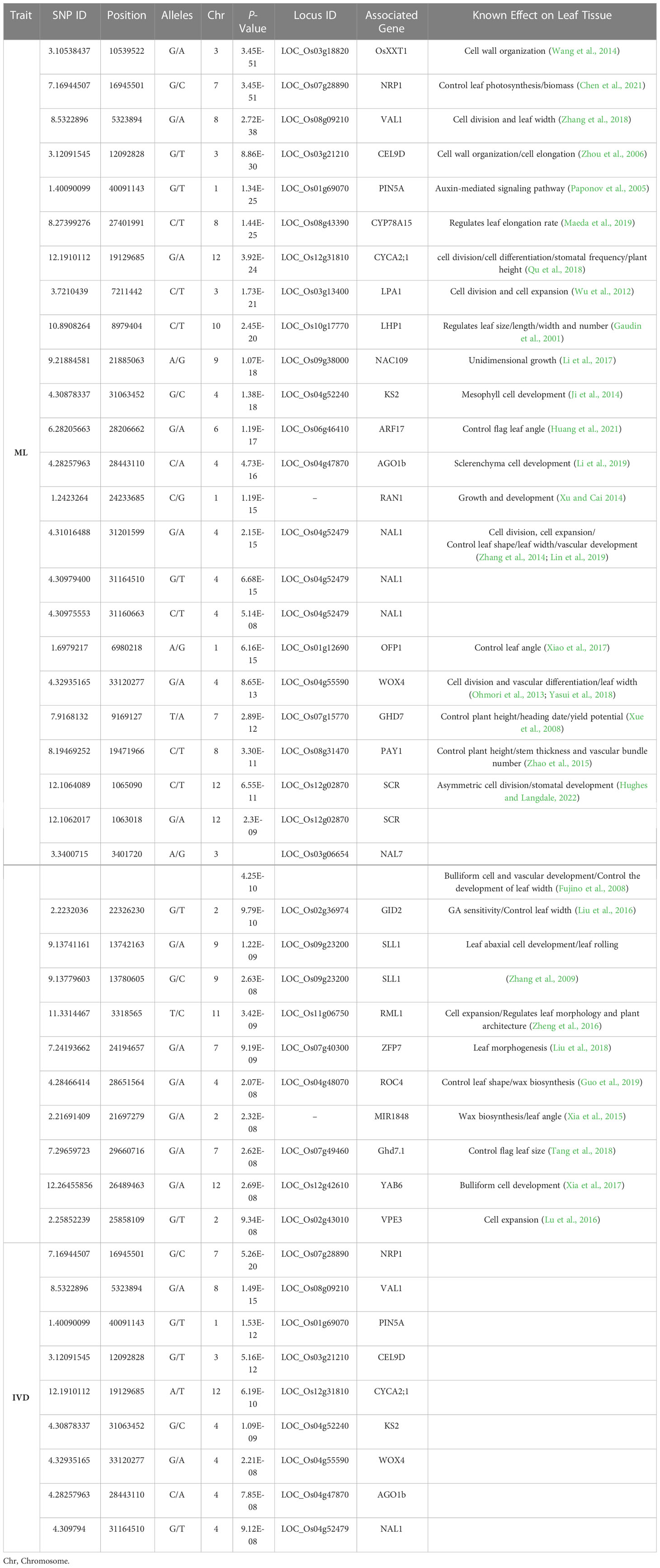
Table 3 Selected candidate genes with known effects on the leaf for each significant SNP associated with the number of mesophyll cells layer (ML) and interveinal distance (IVD) traits implemented by the MLM model.
Regarding the significant SNPs associated with the space in between minor veins or IVD trait, we observed some of the significant SNPs which were repeatedly detected in the ML trait by all the models (Table 3). For instance, the ABCA3 gene was identified as the most significant SNP associated with IVD by FarmCPU and BLINK. NAL1, WOX4, and VAL1, which regulate rice leaf width and vascular bundle development, were also repeatedly identified by MLM as the candidate genes for the IVD trait. We noticed that CELLULASE 9D (CEL9D) was repeatedly detected as a candidate locus associated with ML, IVD, MNVT, and MNVW by FarmCPU, BLINK, and MLM models.
Identification of candidate genes for the vein traits
Candidate genes containing SNPs associated with the five vein traits including MJVT, MJVW, MNVT, MNVW, and VLA, were identified. Regarding MJVT, BLINK was the only model here that could identify some significant SNPs for this vein trait (Table 2). The most significant SNP associated with the thickness of the major vein trait was LOC_Os08g34010 which encodes for a zinc finger homeodomain protein. The second most significant locus for MJVT was LOC_Os09g15770 encoding for a brassinosteroid receptor kinase (BRI1)-interacting protein 107. For the width of the major vein trait, MLM identified the most significant SNP near DWARF53 (D53) on chromosome 11 (Table 4). The D53 gene encodes for a class I Clp ATPase protein which is a repressor for the strigolactones hormone signaling pathway. Mutation of D53 leads to alteration in both small and large vascular bundle numbers in rice internode as well as a reduction in leaf length (Zhou et al., 2013). The SNP locus on chromosome 4, LOC_Os04g56620, which was detected by MLM as a candidate gene for MJVW, was located within the interval of gene CO-FACTOR FOR NITRATE REDUCTASE AND XANTHINE DEHYDROGENASE 1 (CNX1) whose functional annotation is nitrate reductase and xanthine dehydrogenase co-factor. Slender and twisted leaves were observed in CNX1 mutant rice plants (Liu et al., 2019). The peak on chromosome 8 near MEDIATOR 14_1 (MED14_1) was one of the significant loci of MJVW. The MED14_1 is an RNA polymerase II transcription cofactor that plays an important role in vascular bundle development. This gene functions in cell division and differentiation and thus regulates a number of both minor and major veins and rice leaf width (Malik et al., 2020). The Arabidopsis Mediator gene STRUWWELPETER (SWP) encodes subunits of the Mediator transcriptional regulatory complex of RNA polymerase II activity. The swp mutant reduced cell numbers of leaf primordium which leads to small leaves with aberrant morphology (Autran et al., 2002).
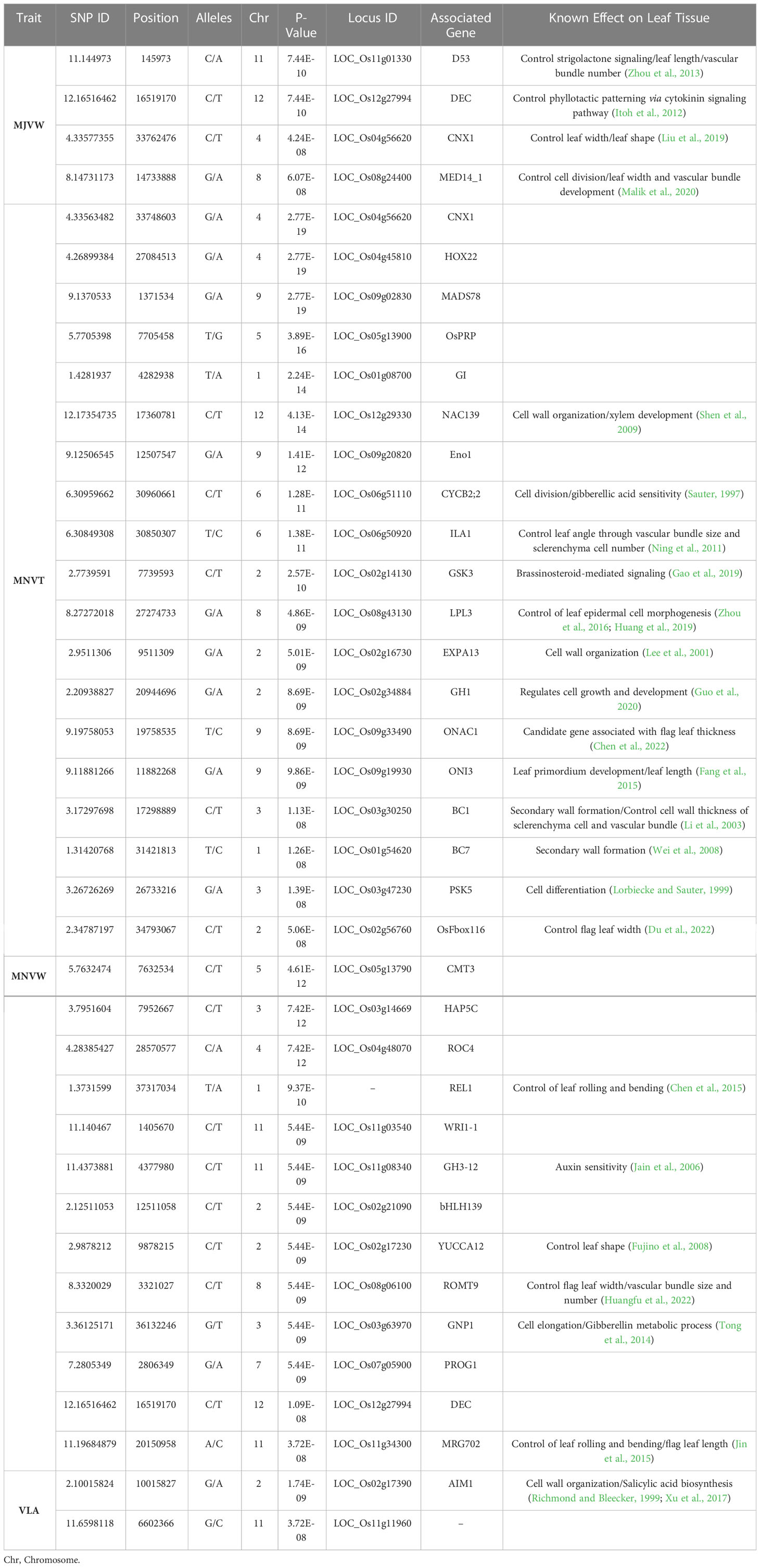
Table 4 Top candidate genes for each significant SNP associated with vein traits implemented by the MLM model.
The only significant locus of MNVT identified by BLINK was located near GH3-7 which is a member of the auxin-responsive GH3 gene family in rice (Jain et al., 2006). The FarmCPU detected significant SNPs on chromosome 1 near PLASTOCHRON 2 (PLA2) whose function in rice leaf development is well documented. The PLA2 controls the cell cycle and vegetative growth time during rice leaf morphogenesis and regulates rice leaf shape, width, and length (Mimura et al., 2012). Regarding the MLM model, a higher number of significant loci for MNVT were identified. Among these, a peak on chromosome 6 located in the interval of INCREASED LEAF ANGLE 1 (ILA1) was detected. ILA1 functions in secondary cell wall biogenesis and plays a major role in controlling leaf inclination through the regulation of vascular bundle size and sclerenchymatous cell number (Ning et al., 2011). A significant SNP on chromosome 9 was associated with ONION3 (ONI3) which is important for leaf primordium development and regulation of rice leaves (Fang et al., 2015). Interestingly, two significant loci related to known genes regulating secondary wall formation and cell wall thickness of vascular bundles, BRITTLE CULM 1 (BC1) (Li et al., 2003) and BRITTLE CULM 7 (BC7) (Wei et al., 2008), were identified for MNVT by MLM. Moreover, LOC_Os02g56760 encodes for F-box protein 116 which is reported as a candidate gene in controlling flag leaf width in rice (Du et al., 2022) was also identified as a significant SNP here. Intriguingly, MLM analysis for MNVT also reported a significant SNP on chromosome 9 located within the interval of the qFTL9 which is reported as a QTL associated with rice leaf thickness (Chen et al., 2022).
For MNVW, multiple significant SNPs identified by FarmCPU were similar to the significant loci identified by BLINK (Table 2). For example, the peak on chromosome 2 near BRASSINAZOLE RESISTANT 4 (BZR4) plays an important role in brassinosteroid signaling transduction (Bai et al., 2007). The distinct significant SNP, identified by only the FarnCPU model, was located in the interval of MEIOTIC RECOMBINATION 11 (MRE11) which is essential for the regulation of the cell cycle and required for normal vegetative growth and development in rice (Shen et al., 2020). The MLM model identified 50 significant SNPs in association with the MNVW trait. Among these, the most significant SNP was located near RICE OUTMOST CELL-SPECIFIC GENE 4 (ROC4). ROC4 is a GLABRA2-type homeobox gene that regulates leaf cuticular wax development (Wang et al., 2018). Up-regulation of the ROC4 gene in the rice Oschr4-5 mutant which produces narrow and rolled leaves with reduced vascular bundle number was reported (Guo et al., 2019). Moreover, the peak on chromosome 1 was located within the interval of ROLLED AND ERECT LEAF 1 (REL 1) which plays a crucial role in leaf rolling and bending. Leaves of the rel1 mutant are rolled and reduced in natural width due to an increase in the adaxial bulliform cell numbers and size (Chen et al., 2015). MLM also identified a significant locus related to another gene known in the regulation of leaf rolling and bending, MORF-RELATED GENE702 (MRG702) which encodes a reader protein for brassinosteroid (BR)-related genes (Jin et al., 2015). Strikingly, the significant SNP on chromosome 8 was located near ROMT9 (OsCOMT) which is recently reported as a key gene in the regulation of flag leaf width, vascular bundle size, and number (Huangfu et al., 2022).
Regarding the vein density trait VLA, the MLM was the only model that implemented two significant SNPs in this study. The most significant locus LOC_ Os02g17390 was located in the interval of ABNORMAL INFLORESCENCE MERISTEM 1 (AIM1) which functions in cell wall organization and salicylic acid biosynthesis (Richmond and Bleecker, 1999; Xu et al., 2017). The second most significant locus associated with VLA was near LOC_Os11g11960 which encodes for an NB-ARC domain-containing protein.
Haplotype analysis of the candidate genes
We selected 4 candidate genes at 4 loci and performed a haplotype analysis to detect significant differences in ML, IVD, MNVW, MJVW, and VLA traits between different haplotypes of each gene. For LOC_Os04g52479 (NAL1) on chromosome 4, the LD block region was started from 31.116 to 31.214 Mb (96.69 kb) and included 6 SNPs (Figure 8A). Five major haplotypes of LOC_Os04g52479 were detected in the region shared by at least 10 accessions of the RDP1 (Figure 8B). Hap1 is prevalent in the whole RDP1 population, especially in the TRJ sub-population. Hap3 had a significantly higher mean ML than Hap1 and Hap2 (Figure 8C). However, Hap1 is predominant in the accessions which showed a higher ML. LOC_Os04g52479 was also selected for haplotype analysis for IVD. In a comparison of IVD across the five haplotypes, Hap5 had a significantly higher IVD than the other haplotypes that mean IVD for Hap5 was 119.0 while the mean IVD for the predominant Hap1 was 111.2 (Figure 8D). Hap1 was the haplotype of LOC_Os04g52479 that contains the highest number the rice accessions of RDP1 (Figure 8E). According to the 700k SNPs dataset that we used for GWAS, Hap 3 is a synonymous variant that causes base substitution without changing in encoded amino acids. Unfortunately, the available data of the SNPs dataset does not cover all the genetic variants within the NAL1 region, we were unable to provide the variant of Hap5 here. Although the synonymous SNP is a type of non-sense mutation, there are reports of significant impacts of the synonymous SNPs on protein expression and function (Vihinen, 2022). This could be the reason reflecting the higher mean of ML of Hap 3 in our study.
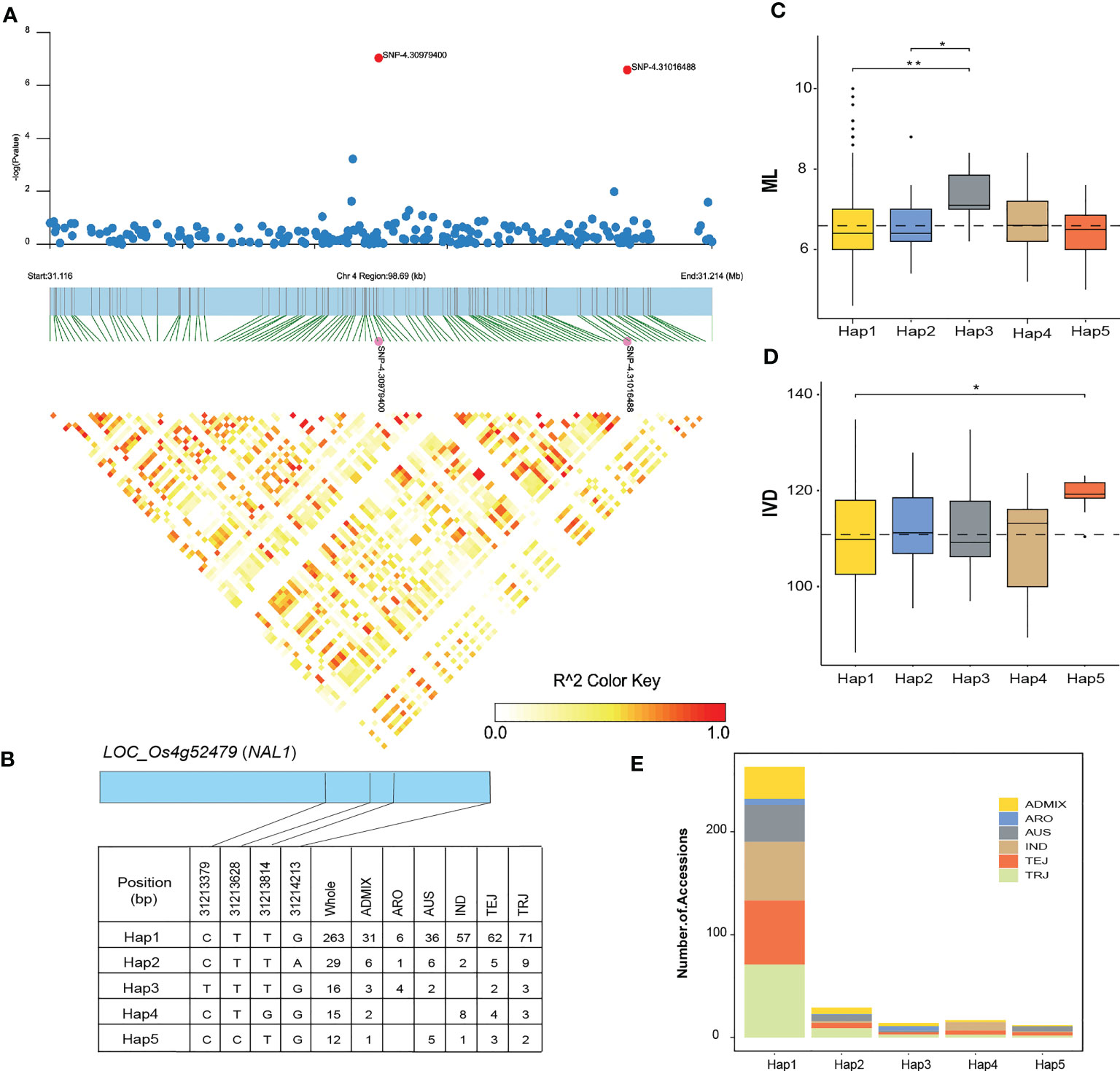
Figure 8 Haplotype analysis of LOC_Os04g52479 (NAL1). (A) Local Manhattan plot (top) and LD heatmap (bottom) of a haplotype block on chromosome 4 associated with the number of mesophyll layer (ML) and inter-veinal distance (IVD) in RDP1 sub-population. The red dots indicate the lead SNP 4.31016488 and SNP 4.30979400. (B) Haplotypes of LOC_Os04g52479. (C) The distribution of ML and (D) IVD in the RDP1 sub-population for the five haplotypes of LOC_Os04g52479. Asterisk indicates significant differences among haplotypes according to the Kruskal-Wallis test and the pairwise Wilcoxon test (P< 0.05). (E) Frequency of the five haplotypes of LOC_Os04g52479 in the RDP1 sub-population. The black dot indicates the outlier data.
The LD block region for haplotype analysis of the candidate gene LOC_Os04g48070 (ROC4) on chromosome 4 was predicted from 28.523 to 28.614 Mb (91.06 kb) and included 15 SNPs. LOC_Os04g48070 had a lead SNP 4.28385427 for MNVW (Supplementary Figure 1). Nine major haplotypes were detected in the coding region. Hap1 is prevalent in the whole RDP1. We detected that Hap1 is predominant in the TEJ sub-population while Hap2 is prevalent in the TRJ sub-population. Hap1 had a significantly higher MNVW of 27.3 than Hap3 which had a mean MNVW of 24.9. The haplotype analysis of the candidate gene LOC_Os08g24400 (MED14_1) was conducted using the LD block region estimated to be from 14.686 to 14.784 Mb (97.97 kb) on chromosome 8 and included 13 SNPs. The lead SNP 8.14731173, located in the candidate gene LOC_Os08g24400 (MED14_1) for MJVW in the RDP1 population. Using the SNPs within the region of LOC_Os08g24400, nine major haplotypes were identified (Supplementary Figure 2). Hap1 is predominantly in the whole population and TRJ while Hap2 and Hap3 are prevalently detected in IND and TEJ sub-population, respectively. Hap1 had a significantly higher mean MJVW than Hap 2 and Hap3 which is consequent with the high MJVW phenotype detected in TRJ (Figure 3A). Further, the candidate gene LOC_Os02g17390 (AIM1) for the VLA trait formed a haplotype block predicted to be from 9.966 to 10.08 Mb (99.32 kb) on chromosome 2 with 24 SNPs which consisted of eleven major alleles. Variation in these haplotypes led to significant phenotypic variation in VLA. Hap1 is prevalent in the whole RDP1 population. TEJ and TRJ are the most abundant sub-population with Hap 1 haplotype. In the whole population, Hap11 had the highest mean VLA of 10.1 which is significantly higher than the mean VLA of the RDP1 population (6.2). Therefore, we defined Hap11 as the favorable haplotype of the vein density trait (Supplementary Figure 3).
Discussion
In this study, three different statistical models, ranging from single to multiple locus models, were compared for GWAS of eight leaf anatomical and vein traits which were different in heritability in 329 accessions of the RDP1. The phenotypic variation among the RDP1 sub-population was relatively high with the coefficients of variant ranging from 9.70% to 51.53% and the heritability ranging from 0.74 to 0.99. Recently, several statistical models for GWAS are available to identify associations of genotypes with numerous phenotypes. Generally, the power of SNP identification power is determined by the population size and structure as well as the heritability influencing the genetic architecture of the trait (Yu et al., 2006; Kaler et al., 2020; Uffelmann et al., 2021). The population structure and a kinship matrix as covariates are incorporated in MLM model that the computation of MLM is intensive. FarmCPU performs marker tests with associated markers as covariates and adopts REML optimization to replace the criterion that the variance explained by kinship is near zero. BLINK is the improved version of the FarmCPU model that Baysian Information criteria (BIC) and linkage disequilibrium approaches are used. In our study, several SNPs were identified by the three models, which implied that all these leaf traits were complex and controlled by an enormous number of genes. Q-Q plots can be used in determining if the models efficiently control false positives and false negatives (Stich et al., 2008; Stich and Melchinger, 2009; Riedelsheimer et al., 2012; Kristensen et al., 2018; Kaler et al., 2020). According to the Q-Q plots of FarmCPU and BLINK, we observed a straight line close to the identity line with a sharp deviated tail, which indicated that these multi-locus models controlled both false positives and false negatives better than MLM, a single locus model, in almost all the traits studied here. However, for ML, IVD, MNVT, and VLA traits, most of the significant markers from the FarmCPU and BLINK were present close to the identity line indicating the increased false negatives which could be generated due to the overfitting of these complex models. For the ML, IVD and MNVT, the FarmCPU and BLINK also exhibited strongly inflated P values which implied the contribution of the population structure and the cryptic relationships among individuals of RDP1 in these leaf and vein traits. From our results, Q-Q plots reflected the statistical power of the MLM model in identifying significant SNPs associated with almost all the leaf anatomical and veins traits of the RDP1 sub-population. MLM-based GWAS is an efficient tool that has been successfully used to analyze genetic variation in multiple leaf traits in rice (Hoang et al., 2019; Du et al., 2022).
Understanding leaf development in rice is important for rice yield improvement, as a good leaf shape can optimize rice plant canopy and capture more light thus increasing the photosynthetic efficiency resulting in boosting grain yield. The study of the natural variation of rice leaf thickness revealed a significant positive correlation between leaf thickness and leaf width (Liu et al., 2014). Similarly, the study of the natural variation of rice leaf anatomy showed that thick rice leaves were comprised of wide-diameter veins, while thin leaves were supported by narrow veins (Chatterjee et al., 2016). We found that the number of mesophyll cell layers (ML) in between 2 adjacent minor veins across the leaf was positively related to LT and MNVT. A recent study revealed that the reduction in the number of cell layers observed in bundle sheath cells of leaf veins would account for the thin leaf trait in rice (Chen et al., 2022). The bulked segregants analysis with whole-genome resequencing (BSA-seq) was used in the study to explore insight into the genetic mechanism and identify the quantitative trait loci (QTL) underlying variation of rice leaf thickness. Their results showed that flag leaf thickness is associated with the qFTL9 in chromosome 9 between 19.10 and 20.03 Mb. Our finding for the MNVT trait that the MLM model reported the significant SNP on chromosome 9 which is located exactly at the candidate region of the qFTL9 is intriguing. Moreover, for MJVT, BLINK and FarmCPU also reported a similar SNP near LOC_Os09g33690, the candidate gene located within the interval of the qFTL9. MLM also identified some correlated SNPs with both the vein’s thickness and width traits that were located in the interval of the qFTL9. However, according to our threshold of genome-wide significance, these SNPs were not statistically significant. These results support our finding that flag leaf thickness was strongly positively correlated with the thickness of either minor veins or major veins as well as the number of mesophyll cells layer.
From the numerous numbers of significant loci and candidate genes associated with eight leaf anatomical and vein traits, the three non-synonymous SNPs near NAL1 detected in ML traits are most intriguing, since the phenotype of NAL1 deletion mutant is narrow, thicker leaves with increased mesophyll cells layer number (Subudhi et al., 2020). The other remarkable phenotype of the NAL1 mutant is the reduction in the number of minor veins and the interveinal distance which is consistent with our GWAS results identifying a significant SNP located near NAL1 associated with the IVD trait. Additionally, a single nucleotide mutation of NAL1 accounted for variation in the distance between small vascular bundle, flag leaf width, and thickness in rice (Taguchi et al., 2015). Moreover, BZR4, the candidate gene for MNVW identified by both BLINK and FarmCPU is upregulated in the NAL1 deletion mutant which might be responsible for the reduction in the number of minor veins and triggering alteration in leaf width (Subudhi et al., 2020). NAL1 plays a crucial role in the cell cycle and cell division affecting vein patterning and leaf width since the early stage of leaf primordium initiation and involves in rice yield traits including chlorophyll content, photosynthetic rate, panicle length, and the number of spikelets per panicle (Takai et al., 2010; Fujita et al., 2013; Zhang et al., 2014; Jiang et al., 2015; Lin et al., 2019). In the present study, several significant loci located near the known genes which play important roles in both leaf and panicle development were detected. Among these, the gene GRAIN NUMBER, PLANT HEIGHT, AND HEADING DATE 7.1 (GHD7.1) which regulates flag leaf size and photosynthetic capacity thus improving yield potential in rice (Tang et al., 2018) were significantly identified as a candidate gene for ML trait. Taken all these together, our findings intensify the positive relationship between leaf morphology and yield thus shedding some light on rice molecular breeding which aims to improve yield potential via targeting leaf traits improvement. The haplotype analysis results from our study revealed that the haplotypes identified within the LD blocks regulated a diverse range of phenotypic variations in leaf anatomical and vein traits. Therefore, haplotype-based markers can provide more options to modify the desired leaf traits in rice. The incorporation of multiple favorable haplotypes in rice breeding programs can be an effective strategy that will assist in the selection of desirable leaf traits. However, we will need to perform functional validation of the identified candidate genes. Recent advances in molecular technology, such as CRISPR-Cas-based technology are a powerful tool in high-throughput gene editing and provide a rapid method to functionally validate genes and alleles for marker-assisted selection (MAS)-based rice improvement in the future.
Conclusion
In the present work, we performed GWAS of rice flag leaf traits by using three different statistical models ranging from single to multiple loci including, FarmCPU, BLINK, and MLM. FarmCPU and BLINK performed slightly better than MLM in terms of false-positive controlling. However, MLM was still a powerful model to identify associations of genotypes with flag leaf anatomical and veins traits of the RDP1 sub-population. Here, the MLM-based GWAS identified several significant loci which were associated with the known genes in rice leaf development. Intriguingly, significant SNPs were detected in the interval of NAL1, GHD7.1, SLL1, and some other genes that regulate leaf shape and yield traits. Our findings indicate that flag leaf traits could be improved via molecular breeding and can be one of the targets in high-yield rice development.
Data availability statement
The datasets presented in this study can be found in online repositories. The names of the repository/repositories and accession number(s) can be found in the article/Supplementary Material.
Author contributions
SN designed and performed the experiments in leaf anatomical and vein traits phenotyping. SN also analyzed corresponding data and write the manuscript. B-OT helped grow the rice population throughout the developmental stages and collect leaf samples. YP conducted the association analysis and analyzed GWAS results. PV conceived the project, helped in GWAS data analysis, and provided advice as well as experimental materials. PV also advised on the manuscript concept and prepared the manuscript.
Funding
This research was funded by grants from the National Research Council of Thailand (NRCT) NRCT-353804 and NRCT-705002.
Acknowledgments
We thank the International Rice Research Institute (IRRI) for providing the seeds of the RDP1 population.
Conflict of interest
The authors declare that the research was conducted in the absence of any commercial or financial relationships that could be construed as a potential conflict of interest.
Publisher’s note
All claims expressed in this article are solely those of the authors and do not necessarily represent those of their affiliated organizations, or those of the publisher, the editors and the reviewers. Any product that may be evaluated in this article, or claim that may be made by its manufacturer, is not guaranteed or endorsed by the publisher.
Supplementary material
The Supplementary Material for this article can be found online at: https://www.frontiersin.org/articles/10.3389/fpls.2023.1107718/full#supplementary-material
References
Ali, L. M., McClung, A. M., Jia, M. H., Kimball, J. A., McCouch, S. R., Eizenga., G. C. (2011) A rice diversity panel evaluated for genetic and agro-morphological diversity between subpopulations and its geographic distribution. Crop Sci. 51 (5), 2021–2355. doi: 10.2135/cropsci2010.11.0641
Autran, Daphné, Jonak, C., Belcram, K., Beemster, G. T.S., Kronenberger, J., Grandjean, O., et al (2002) Cell numbers and leaf development in arabidopsis: A functional analysis of the STRUWWELPETER gene EMBO J. 21 (22), 6036–6495. doi: 10.1093/emboj/cdf614
Bai, M.-Y., Zhang, L.-Y., Gampala, S. S., Zhu, S.-W., Song, W.-Y., Chong, K., et al (2007) Functions of OsBZR1 and 14-3-3 proteins in brassinosteroid signaling in rice. Proc. Natl. Acad. Sci. 104 (34), 13839–13445. doi: 10.1073/pnas.0706386104
Barrett, J. C., Fry, B., Maller, J., Daly, M. J. (2005) Haploview: Analysis and visualization of LD and haplotype maps. Bioinformatics 21 (2), 263–265. doi: 10.1093/bioinformatics/bth457
Chatterjee, J., Dionora, J., Elmido-Mabilangan, A., Wanchana, S., Thakur, V., Bandyopadhyay, A., et al. (2016). The evolutionary basis of naturally diverse rice leaves anatomy. edited by manoj prasad. PloS One 11 (10), e01645325. doi: 10.1371/journal.pone.0164532
Chen, K. E., Łyskowski, A., Jaremko, Łukasz, Jaremko., M. (2021) Genetic and molecular factors determining grain weight in rice. Front. Plant Sci. 12 (July). doi: 10.3389/fpls.2021.605799
Chen, Q., Xie, Q., Gao, J. U., Wang, W., Sun, B. O., Liu, B., et al (2015) Characterization of Rolled and erect leaf 1 in regulating leave morphology in rice. J. Exp. Bot. 66 (19), 6047–6058. doi: 10.1093/jxb/erv319
Chen, D.-g., Zhou, X.-q., Chen, K. E., Chen, P.-l., Guo, J., Liu, C.-g., et al (2022) Fine-mapping and candidate gene analysis of a major locus controlling leaf thickness in rice (Oryza sativa l.). Mol. Breed. 42 (2), 65. doi: 10.1007/s11032-022-01275-y
Cook, M. G., Evans, L. T. (1983). Some physiological aspects of the domestication and improvement of rice (Oryza spp.). Field Crops Res. 6 (January), 219–238. doi: 10.1016/0378-4290(83)90062-X
Dong, S.-S., He, W.-M., Ji, J.-J., Zhang, C., Guo, Y., Yang., T.-L. (2021). LDBlockShow: A fast and convenient tool for visualizing linkage disequilibrium and haplotype blocks based on variant call format files. Briefings Bioinf. 22 (4), bbaa227. doi: 10.1093/bib/bbaa227
Du, M., Xiong, M., Chang, Y., Liu, Z., Wang, R., Lin, X., et al. (2022). Mining candidate genes and favorable haplotypes for flag leaf shape in rice (Oryza sativa l.) based on a genome-wide association study. Agronomy 12 (8), 18145. doi: 10.3390/agronomy12081814
Duan, K. E., Li, L. I., Hu, P., Xu, S.-P., Xu, Z.-H., Xue., H.-W. (2006). A brassinolide-suppressed rice MADS-box transcription factor, OsMDP1, has a negative regulatory role in BR signaling. Plant J. 47 (4), 519–315. doi: 10.1111/j.1365-313X.2006.02804.x
Eizenga, G. C., Ali, Md. L., Bryant, R. J., Yeater, K. M., McClung, A. M., McCouch., S. R. (2014). Registration of the rice diversity panel 1 for genomewide association studies. J. Plant Registrations 8 (1), 109–165. doi: 10.3198/jpr2013.03.0013crmp
Evans, J. R., Loreto, F. (2000). Acquisition and diffusion of CO2 in higher plant leaves, Advances in photosynthesis and respiration, vol. 9 . Eds. Leegood, R. C., Sharkey, T. D., Caemmerer, S. v. (Dordrecht: Springer Netherlands), 321–351. doi: 10.1007/0-306-48137-5_14
Fan, X., Cui, F. A., Zhao, C., Zhang, W., Yang, L., Zhao, X., et al. (2015). QTLs for flag leaf size and their influence on yield-related traits in wheat (Triticum aestivum l.). Mol. Breed. 35 (1), 24. doi: 10.1007/s11032-015-0205-9
Fang, Y., Hu, J., Xu, J., Yu, H., Shi, Z., Xiong, G., et al. (2015). Identification and characterization of Mini1 , a gene regulating rice shoot development: Characterization of a mini rice mutant. J. Integr. Plant Biol. 57 (2), 151–161. doi: 10.1111/jipb.12230
Feldman, A. B., Murchie, E. H., Leung, H., Baraoidan, M., Coe, R., Yu, S.-M., et al. (2014). Increasing leaf vein density by mutagenesis: Laying the foundations for C4 rice. edited by keqiang Wu. PloS One 9 (4), e949475. doi: 10.1371/journal.pone.0094947
Feng, Z., Wu, C., Wang, C., Roh, J., Zhang, L., Chen, J., et al. (2016). SLG controls grain size and leaf angle by modulating brassinosteroid homeostasis in rice. J. Exp. Bot. 67 (14), 4241–4253. doi: 10.1093/jxb/erw204
Fujii, S., Saka, H. (2001). Distribution of assimilates to each organ in rice plants exposed to a low temperature at the ripening stage, and the effect of brassinolide on the distribution. Plant Production Sci. 4 (2), 136–445. doi: 10.1626/pps.4.136
Fujino, K., Matsuda, Y., Ozawa, K., Nishimura, T., Koshiba, T., Fraaije, M. W., et al. (2008). NARROW LEAF 7 controls leaf shape mediated by auxin in rice. Mol. Genet. Genomics 279 (5), 499–5075. doi: 10.1007/s00438-008-0328-3
Fujita, D., Trijatmiko, K. R., Tagle, A. G., Sapasap, M. V., Koide, Y., Sasaki, K., et al. (2013). NAL1 allele from a rice landrace greatly increases yield in modern Indica cultivars. Proc. Natl. Acad. Sci. 110 (51), 20431–20436. doi: 10.1073/pnas.1310790110
Gao, X., Zhang, J.-Q., Zhang, X., Zhou, J., Jiang, Z., Huang, P., et al. (2019). Rice QGL3/OsPPKL1 functions with the GSK3/SHAGGY-like kinase OsGSK3 to modulate brassinosteroid signaling. Plant Cell 31 (5), 1077–1093. doi: 10.1105/tpc.18.00836
Gaudin, Valérie, Libault, M., Pouteau, S., Juul, T., Zhao, G., Lefebvre, D., et al (2001). Mutations in LIKE HETEROCHROMATIN PROTEIN 1 affect flowering time and plant architecture in Arabidopsis Development 128 (23), 4847–4585. doi: 10.1242/dev.128.23.4847
Gladun, I. V., Karpov, E. A. (1993). Distribution of assimilates from the flag leaf of rice during the reproductive period of development. Russian J. Plant Physiol. 40, 215–219. doi: 10.1626/pps.4.136
Guo, T., Chen, H.-C., Lu, Z.-Q., Diao, M., Chen, K. E., Dong, N.-Q., et al. (2020). A SAC phosphoinositide phosphatase controls rice development via hydrolyzing PI4P and PI(4,5)P 2. Plant Physiol. 182 (3), 1346–1585. doi: 10.1104/pp.19.01131
Guo, T., Wang, D., Fang, J., Zhao, J., Yuan, S., Xiao, L., et al. (2019). Mutations in the rice OsCHR4 gene, encoding a CHD3 family chromatin remodeler, induce narrow and rolled leaves with increased cuticular wax. Int. J. Mol. Sci. 20 (10), 25675. doi: 10.3390/ijms20102567
Hirose, N., Makita, N., Kojima, M., Kamada-Nobusada, T., Sakakibara, H. (2007). Overexpression of a type-a response regulator alters rice morphology and cytokinin metabolism. Plant Cell Physiol. 48 (3), 523–539. doi: 10.1093/pcp/pcm022
Hoang, G. T., Gantet, P., Nguyen, K. H., Phung, N. T. P., Ha, L. T., Nguyen, T. T., et al. (2019). Genome-wide association mapping of leaf mass traits in a Vietnamese rice landrace panel. edited by kandasamy ulaganathan. PloS One 14 (7), e02192745. doi: 10.1371/journal.pone.0219274
Huang, L., Chen, L., Wang, L., Yang, Y., Rao, Y., Ren, D., et al. (2019). A nck-associated protein 1-like protein affects drought sensitivity by its involvement in leaf epidermal development and stomatal closure in rice. Plant J. 98 (5), 884–897. doi: 10.1111/tpj.14288
Huang, G., Hu, H., de Meene, A. V., Zhang, J., Dong, L. E., Zheng, S., et al. (2021). AUXIN RESPONSE FACTORS 6 and 17 control the flag leaf angle in rice by regulating secondary cell wall biosynthesis of lamina joints. Plant Cell 33 (9), 3120–3133. doi: 10.1093/plcell/koab175
Huang, M., Liu, X., Zhou, Y., Summers, R. M., Zhang., Z. (2019). BLINK: A package for the next level of genome-wide association studies with both individuals and markers in the millions. GigaScience 8 (2), 1–12. doi: 10.1093/gigascience/giy154
Huangfu, L., Chen, R., Lu, Y., Zhang, E., Miao, J., Zuo, Z., et al. (2022). OsCOMT , encoding a caffeic acid O-methyltransferase in melatonin biosynthesis, increases rice grain yield through dual regulation of leaf senescence and vascular development. Plant Biotechnol. J. 20 (6), 1122–1139. doi: 10.1111/pbi.13794
Huber, W., Carey, V. J., Gentleman, R., Anders, S., Carlson, M., Carvalho, B. S., et al. (2015). Orchestrating high-throughput genomic analysis with bioconductor. Nat. Methods 12 (2), 115–121. doi: 10.1038/nmeth.3252
Hughes, T. E., Langdale, J. A. (2022). SCARECROW is deployed in distinct contexts during rice and maize leaf development. Development 149 (7), 1–11. doi: 10.1242/dev.200410
Itoh, J.-i., Hibara, K.-i., Kojima, M., Sakakibara, H., Nagato., Y. (2012). Rice DECUSSATE controls phyllotaxy by affecting the cytokinin signaling pathway. Plant J. 72 (6), 869–815. doi: 10.1111/j.1365-313x.2012.05123.x
Itoh, H., Shimada, A., Ueguchi-Tanaka, M., Kamiya, N., Hasegawa, Y., Ashikari, M., et al. (2005). Overexpression of a GRAS protein lacking the DELLA domain confers altered gibberellin responses in rice: Rice SLR1-like genes. Plant J. 44 (4), 669–795. doi: 10.1111/j.1365-313X.2005.02562.x
Jain, M., Kaur, N., Tyagi, A. K., Khurana., J. P. (2006). The auxin-responsive GH3 gene family in rice (Oryza sativa). Funct. Integr. Genomics 6 (1), 36–465. doi: 10.1007/s10142-005-0142-5
Ji, S. H., Gururani, M. A., Lee, J. W., Ahn, B.-O., Chun, S.-C. (2014). Isolation and characterisation of a dwarf rice mutant exhibiting defective gibberellins biosynthesis. Plant Biology 16 (2), 428–439. doi: 10.1111/plb.12069
Jiang, D., Fang, J., Lou, L., Zhao, J., Yuan, S., Yin, L., et al. (2015). Characterization of a null allelic mutant of the rice NAL1 gene reveals its role in regulating cell division. edited by keqiang Wu. PloS One 10 (2), e01181695. doi: 10.1371/journal.pone.0118169
Jin, J., Shi, J., Liu, B., Liu, Y., Huang, Y., Yu, Yu, et al. (2015). MORF-RELATED GENE702, a reader protein of trimethylated histone H3 lysine 4 and histone H3 lysine 36, is involved in brassinosteroid-regulated growth and flowering time control in rice. Plant Physiol. 168 (4), 1275–1855. doi: 10.1104/pp.114.255737
Kaler, A. S., Gillman, J. D., Beissinger, T., Purcell., L. C. (2020). Comparing different statistical models and multiple testing corrections for association mapping in soybean and maize. Front. Plant Sci. 10 (February). doi: 10.3389/fpls.2019.01794
Kanbe, T., Sasaki, H., Aoki, N., Yamagishi, T., Ebitani, T., Yano, M., et al. (2008). Identification of QTLs for improvement of plant type in rice (Oryza sativa l.) using koshihikari / kasalath chromosome segment substitution lines and backcross progeny F2 population. Plant Production Sci. 11 (4), 447–565. doi: 10.1626/pps.11.447
Kristensen, P. S., Jahoor, A., Andersen, J. R., Cericola, F., Orabi, J., Janss, L. L., et al. (2018). Genome-wide association studies and comparison of models and cross-validation strategies for genomic prediction of quality traits in advanced winter wheat breeding lines. Front. Plant Sci. 9 (February). doi: 10.3389/fpls.2018.00069
Kumar, S., Tripathi, S., Singh, S. P., Prasad, A., Akter, F., Syed, Md A., et al. (2021). Rice breeding for yield under drought has selected for longer flag leaves and lower stomatal density.J. Exp. Bot. 72 (13), 4981–4992. doi: 10.1093/jxb/erab160
Kurata, N., Yamazaki, Y. (2006). “Oryzabase. An Integrated Biological and Genome Information Database for Rice.” Plant Physiology 140 (1):12–17. doi: 10.1104/pp.105.063008
Lee, Y. I., Choi, D., Kende., H. (2001). Expansins: Ever-expanding numbers and functions. Curr. Opin. Plant Biol. 4 (6), 527–325. doi: 10.1016/S1369-5266(00)00211-9
Li, X., Han, H., Chen, M., Yang, W., Liu, L. I, Li, N., et al. (2017). Overexpression of OsDT11, which encodes a novel cysteine-rich peptide, enhances drought tolerance and increases ABA concentration in rice. Plant Mol. Biol. 93 (1–2), 21–34. doi: 10.1007/s11103-016-0544-x
Li, Y., Qian, Q., Zhou, Y., Yan, M., Sun, L., Zhang, M. U., et al. (2003). BRITTLE CULM1, which encodes a COBRA-like protein, affects the mechanical properties of rice plants. Plant Cell 15 (9), 2020–2031. doi: 10.1105/tpc.011775
Li, J., Yang, J., Li, D., Fei, P., Guo, T., Ge, C., et al. (2011). Chlorophyll meter’s estimate of weight-based nitrogen concentration in rice leaf is influenced by leaf thickness. Plant Production Sci. 14 (2), 177–835. doi: 10.1626/pps.14.177
Li, Y., Yang, Y., Liu, Ye, Li, D., Zhao, Y., Li, Z., et al. (2019). Overexpression of OsAGO1b induces adaxially rolled leaves by affecting leaf abaxial sclerenchymatous cell development in rice. Rice 12 (1), 60. doi: 10.1186/s12284-019-0323-9
Lin, L., Zhao, Y., Liu, F., Chen, Q., Qi., J. (2019). Narrow leaf 1 (NAL1) regulates leaf shape by affecting cell expansion in rice (Oryza sativa l.). Biochem. Biophys. Res. Commun. 516 (3), 957–625. doi: 10.1016/j.bbrc.2019.06.142
Lipka, A. E., Tian, F., Wang, Q., Peiffer, J., Li, M., Bradbury, P. J., et al. (2012). GAPIT: Genome association and prediction integrated tool. Bioinformatics 28 (18), 2397–2995. doi: 10.1093/bioinformatics/bts444
Liu, Q., Guo, X., Chen, G., Zhu, Z., Yin, W., Hu., Z. (2016). Silencing SlGID2, a putative f-box protein gene, generates a dwarf plant and dark-green leaves in tomato. Plant Physiol. Biochem. 109 (December), 491–501. doi: 10.1016/j.plaphy.2016.10.030
Liu, X., Huang, M., Fan, B., Buckler, E. S., Zhang., Z. (2016). Iterative usage of fixed and random effect models for powerful and efficient genome-wide association studies. edited by Jennifer listgarten. PloS Genet. 12 (2), e10057675. doi: 10.1371/journal.pgen.1005767
Liu, X., Li, D., Zhang, D., Yin, D., Zhao, Y. I., Ji, C., et al. (2018). A novel antisense long noncoding RNA, TWISTED LEAF , maintains leaf blade flattening by regulating its associated sense R2R3-MYB gene in rice. New Phytol. 218 (2), 774–788. doi: 10.1111/nph.15023
Liu, X., Wang, J., Yu, Y., Kong, L., Liu, Y., Liu, Z., et al. (2019). Identification and characterization of the rice pre-harvest sprouting mutants involved in molybdenum cofactor biosynthesis. New Phytol. 222 (1), 275–285. doi: 10.1111/nph.15607
Liu, C.-g., Zhou, X.-q., Chen, D.-g., Li, L.-j., Li, J.-c., Chen., Y.-d. (2014). Natural variation of leaf thickness and its association to yield traits in indica rice. J. Integr. Agric. 13 (2), 316–255. doi: 10.1016/S2095-3119(13)60498-0
Lorbiecke, René, Sauter, M. (1999). Adventitious root growth and cell-cycle induction in deepwater Rice1. Plant Physiol. 119 (1), 21–305. doi: 10.1104/pp.119.1.21
Lu, W., Deng, M., Guo, F. U., Wang, M., Zeng, Z., Han, N., et al. (2016). Suppression of OsVPE3 enhances salt tolerance by attenuating vacuole rupture during programmed cell death and affects stomata development in rice. Rice 9 (1), 655. doi: 10.1186/s12284-016-0138-x
Maeda, S., Dubouzet, J. G., Kondou, Y., Jikumaru, Y., Seo, S., Oda, K., et al. (2019). The rice CYP78A gene BSR2 confers resistance to rhizoctonia solani and affects seed size and growth in arabidopsis and rice. Sci. Rep. 9 (1), 5875. doi: 10.1038/s41598-018-37365-1
Malik, N., Ranjan, R., Parida, S. K., Agarwal, P., Tyagi., A. K. (2020). Mediator subunit OsMED14_1 plays an important role in rice development. Plant J. 101 (6), 1411–1295. doi: 10.1111/tpj.14605
McCouch, S. R., Wright, M. H., Tung, C.-W., Maron, L. G., McNally, K. L., Fitzgerald, M., et al. (2016). Open access resources for genome-wide association mapping in rice. Nat. Commun. 7 (1), 10532. doi: 10.1038/ncomms10532
Mimura, M., Nagato, Y., Itoh., J.-I. (2012). Rice PLASTOCHRON genes regulate leaf maturation downstream of the gibberellin signal transduction pathway. Planta 235 (5), 1081–1895. doi: 10.1007/s00425-012-1639-5
Murchie, E. H., Hubbart, S., Chen, Y., Peng, S., Horton., P. (2002). Acclimation of rice photosynthesis to irradiance under field conditions. Plant Physiol. 130 (4), 1999–20105. doi: 10.1104/pp.011098
Narawatthana, S. (2013). The regulation of leaf thickness in rice (Oryza sativa l.). (Sheffield, United Kingdom: The University of Sheffield).
Ning, J., Zhang, B., Wang, N., Zhou, Y., Xiong., L. (2011). Increased leaf Angle1, a raf-like MAPKKK that interacts with a nuclear protein family, regulates mechanical tissue formation in the lamina joint of rice. Plant Cell 23 (12), 4334–4475. doi: 10.1105/tpc.111.093419
Ohmori, Y., Tanaka, W., Kojima, M., Sakakibara, H., Hirano., H.-Y. (2013). WUSCHEL-RELATED HOMEOBOX4 is involved in meristem maintenance and is negatively regulated by the CLE gene. FCP1 Rice. Plant Cell 25 (1), 229–415. doi: 10.1105/tpc.112.103432
Paponov, I., Teale, W., Trebar, M., Blilou, I., Palme, K. (2005). The PIN auxin efflux facilitators: Evolutionary and functional perspectives. Trends Plant Sci. 10 (4), 170–177. doi: 10.1016/j.tplants.2005.02.009
Peng, S. (2000). Single-leaf and canopy photosynthesis of Rice11Citation, in Redesigning rice photosynthesis to increase yield. proceedings of the workshop on the quest to reduce hunger: Redesigning rice photosynthesis 7 . Eds. Sheehy, J. E., Mitchell, P. L., Hardy, B. (Los Baños. Philippines. Makati City (Philippines: International Rice Research Institute and Amsterdam (The Netherlands): Elsevier Science B.V), 213–228 . doi: 10.1016/S0928-3420(00)80017-8
Peng, S., Khush, G. S., Cassman, K. G. (1994). Evolution of the new plant ideotype for increased yield potential, in Breaking the yield barrier: Proceedings of a workshop on rice yield potential in favorable environments (Los Banos, Philippines: International Rice Research Institute), 5–20.
Pérez-Pérez, JoséM., Esteve-Bruna, D., Micol., JoséL. (2010). QTL analysis of leaf architecture. J. Plant Res. 123 (1), 15–235. doi: 10.1007/s10265-009-0267-z
Petricka, J. J., Clay, N. K., Nelson., T. M. (2008). Vein patterning screens and the defectively organized tributaries mutants in Arabidopsis thaliana: The dot Mutants of arabidopsis. Plant J. 56 (2), 251–635. doi: 10.1111/j.1365-313X.2008.03595.x
Phanchaisri, B., Samsang, N., Yu, L., Singkarat, S., Anuntalabhochai., S. (2012). Expression of OsSPY and 14-3-3 genes involved in plant height variations of ion-Beam-Induced KDML 105 rice mutants. Mutat. Research/Fundamental Mol. Mech. Mutagenesis 734 (1–2), 56–61. doi: 10.1016/j.mrfmmm.2012.03.002
Pitaloka, M., Harrison, E., Hepworth, C., Wanchana, S., Toojinda, T., Phetluan, W., et al. (2021). Rice stomatal mega-papillae restrict water loss and pathogen entry. Front. Plant Sci. 12 (June). doi: 10.3389/fpls.2021.677839
Price, A. L., Patterson, N. J., Plenge, R. M., Weinblatt, M. E., Shadick, N. A., Reich., D. (2006). Principal components analysis corrects for stratification in genome-wide association studies. Nat. Genet. 38 (8), 904–995. doi: 10.1038/ng1847
Qi, J., Qian, Q., Bu, Q., Li, S., Chen, Q., Sun, J., et al. (2008). Mutation of the rice Narrow Leaf1 gene, which encodes a novel protein, affects vein patterning and polar auxin transport. Plant Physiol. 147 (4), 1947–1959. doi: 10.1104/pp.108.118778
Qu, X., Yan, M., Zou, J., Jiang, M., Yang, K., Le., J. (2018). A2-type cyclin is required for the asymmetric entry division in rice stomatal development. J. Exp. Bot. 69 (15), 3587–3995. doi: 10.1093/jxb/ery158
R Core Team (2022). R: A language and environment for statistical computing, in R foundation for statistical computing (Vienna, Austria). Available at: https://www.R-project.org/.
Revelle, W., Condon, D. M. (2019). Reliability from α to ω: A tutorial. psychol. Assess. 31 (12), 1395–14115. doi: 10.1037/pas0000754
Richmond, T. A., Bleecker, A. B. (1999). A defect in b-oxidation causes abnormal inflorescence development in arabidopsis. Plant Cell 11 (10), 19115. doi: 10.2307/3871086
Riedelsheimer, C., Lisec, J., Czedik-Eysenberg, A., Sulpice, R., Flis, A., Grieder, C., et al. (2012). Genome-wide association mapping of leaf metabolic profiles for dissecting complex traits in maize. Proc. Natl. Acad. Sci. 109 (23), 8872–8775. doi: 10.1073/pnas.1120813109
Sack, L., Scoffoni, C. (2013). Leaf venation: Structure, function, development, evolution, ecology and applications in the past, present and future. New Phytol. 198 (4), 983–10005. doi: 10.1111/nph.12253
Sakamoto, T., Sakakibara, H., Kojima, M., Yamamoto, Y., Nagasaki, H., Inukai, Y., et al. (2006). Ectopic expression of KNOTTED1-like homeobox protein induces expression of cytokinin biosynthesis genes in rice. Plant Physiol. 142 (1), 54–625. doi: 10.1104/pp.106.085811
San-oh, Y., Mano, Y., Ookawa, T., Hirasawa, T. (2004). Comparison of dry matter production and associated characteristics between direct-sown and transplanted rice plants in a submerged paddy field and relationships to planting patterns. Field Crops Res. 87 (1), 43–58. doi: 10.1016/j.fcr.2003.09.004
Sauter, M. (1997). Differential expression of a CAK (Cdc2-activating kinase)-like protein kinase, cyclins and Cdc2 genes from rice during the cell cycle and in response to gibberellin. Plant J. 11 (2), 181–190. doi: 10.1046/j.1365-313X.1997.11020181.x
Schindelin, J., Arganda-Carreras, I., Frise, E., Kaynig, V., Longair, M., Pietzsch, T., et al. (2012). Fiji: An open-source platform for biological-image analysis. Nat. Methods 9 (7), 676–682. doi: 10.1038/nmeth.2019
Shen, M., Nie, Y., Chen, Y., Zhang, X., Zhao., J. (2020). OsMre11 is required for mitosis during rice growth and development. Int. J. Mol. Sci. 22 (1), 1695. doi: 10.3390/ijms22010169
Shen, H., Yin, Y., Chen, F., Xu, Y., Dixon., R. A. (2009). A bioinformatic analysis of NAC genes for plant cell wall development in relation to lignocellulosic bioenergy production. Bioenergy Res. 2 (4), 217–325. doi: 10.1007/s12155-009-9047-9
Sinclair, T. R., Sheehy, J. E. (1999). Erect leaves and photosynthesis in rice. Science 283 (5407), 1455–1455. doi: 10.1126/science.283.5407.1455c
Smillie, I. R. A., Pyke, K. A., Murchie, E. H. (2012). Variation in vein density and mesophyll cell architecture in a rice deletion mutant population. J. Exp. Bot. 63 (12), 4563–4570. doi: 10.1093/jxb/ers142
Stich, B., Melchinger, A. E. (2009). Comparison of mixed-model approaches for association mapping in rapeseed, potato, sugar beet, maize, and arabidopsis. BMC Genomics 10 (1), 945. doi: 10.1186/1471-2164-10-94
Stich, B., Möhring, J., Piepho, H.-P., Heckenberger, M., Buckler, E. S., Melchinger., A. E. (2008). Comparison of mixed-model approaches for association mapping. Genetics 178 (3), 1745–1545. doi: 10.1534/genetics.107.079707
Subudhi, P. K., Garcia, R. S., Coronejo, S., De Leon., T. B. (2020). A novel mutation of the NARROW LEAF 1 gene adversely affects plant architecture in rice (Oryza sativa l.). Int. J. Mol. Sci. 21 (21), 81065. doi: 10.3390/ijms21218106
Tabassum, M. A., Zhu, G., Hafeez, A., Wahid, M. A., Shaban, M., Li., Y. (2016). Influence of leaf vein density and thickness on hydraulic conductance and photosynthesis in rice (Oryza sativa l.) during water stress. Sci. Rep. 6 (1), 368945. doi: 10.1038/srep36894
Taguchi, F., Ota, T., Ebana, K., Ookawa, T., Yamasaki, M., Tanabata, T., et al. (2015). Natural variation in the flag leaf morphology of rice due to a mutation of the NARROW LEAF 1 gene in Oryza sativa l. Genetics 201 (2), 795–808. doi: 10.1534/genetics.115.181040
Takai, T., Kondo, M., Yano, M., Yamamoto., T. (2010). A quantitative trait locus for chlorophyll content and its association with leaf photosynthesis in rice. Rice 3 (2–3), 172–180. doi: 10.1007/s12284-010-9047-6
Tang, X., Gong, R., Sun, W., Zhang, C., Yu., S. (2018). Genetic dissection and validation of candidate genes for flag leaf size in rice (Oryza sativa l.). Theor. Appl. Genet. 131 (4), 801–155. doi: 10.1007/s00122-017-3036-8
Terashima, I., Miyazawa, S.-I., Hanba., Y. T. (2001). Why are sun leaves thicker than shade leaves? — consideration based on analyses of CO2 diffusion in the leaf. J. Plant Res. 114 (1), 93–1055. doi: 10.1007/PL00013972
Tomoshiro, T., Mitsunori, O., Waichi, A. (1983). Comparison of the formation of dry substance by the old and new type of rice cultivars. Japan J. Crop Sci. 52, 299–305.
Tong, H., Xiao, Y., Liu, D., Gao, S., Liu, L., Yin, Y., et al. (2014). Brassinosteroid regulates cell elongation by modulating gibberellin metabolism in rice. Plant Cell 26 (11), 4376–4935. doi: 10.1105/tpc.114.132092
Tsukaya, H. (2005). Leaf shape: Genetic controls and environmental factors. Int. J. Dev. Biol. 49 (5–6), 547–555. doi: 10.1387/ijdb.041921ht
Uffelmann, E., Huang, Q. Q., Munung, N. S., de Vries, J., Okada, Y., Martin, A. R., et al. (2021). Genome-wide association studies. Nat. Rev. Methods Primers 1 (1), 595. doi: 10.1038/s43586-021-00056-9
Vangahun, J. M. (2012). Inheritance of flag leaf angle in two rice (Oryza sativa l) cultivars. (Kumasi, Ghana: Kwame Nkrumah University of Science and Technology).
Vihinen, M. (2022). When a synonymous variant is nonsynonymous. Genes 13 (8), 1485. doi: 10.3390/genes13081485
Wang, C., Li, S., Ng, S., Zhang, B., Zhou, Y., Whelan, J., et al. (2014). Mutation in xyloglucan 6-xylosytransferase results in abnormal root hair development in oryza sativa. J. Exp. Bot. 65 (15), 4149–4575. doi: 10.1093/jxb/eru189
Wang, Q., Xie, W., Xing, H., Yan, Ju, Meng, X., Li, X., et al. (2015). Genetic architecture of natural variation in rice chlorophyll content revealed by a genome-wide association study. Mol. Plant 8 (6), 946–957. doi: 10.1016/j.molp.2015.02.014
Wang, P., Zhou, G., Yu, H., Yu., S. (2011). Fine mapping a major QTL for flag leaf size and yield-related traits in rice. Theor. Appl. Genet. 123 (8), 1319–1305. doi: 10.1007/s00122-011-1669-6
Wang, Z., Tian, X., Zhao, Q., Liu, Z., Li, X., Ren, Y., et al. (2018). “The E3 Ligase DROUGHT HYPERSENSITIVE Negatively Regulates Cuticular Wax Biosynthesis by Promoting the Degradation of Transcription Factor ROC4 in Rice.” The Plant Cell 30 (1):228–44. doi: 10.1105/tpc.17.00823
Wei, C.-X., Xie, P.-S., Zhou, W.-D., Chen, Y.-F., Yan., C.-J. (2008). Anatomical structure and chemical features of leaf in brittle mutant of rice. Acta Agronomica Sin. 34 (8), 1417–1235. doi: 10.1016/S1875-2780(08)60048-6
Wu, R., Li, S., He, S., Waßmann, F., Yu, C., Qin, G., et al. (2011) CFL1, a WW domain protein, regulates cuticle development by modulating the function of HDG1, a class IV homeodomain transcription factor, in rice and Arabidopsis. Plant Cell 23 (9), 3392–3411. doi: 10.1105/tpc.111.088625
Wu, X., Tang, D., Li, M., Wang, K., Cheng., Z. (2012). Loose plant Architecture1, an INDETERMINATE DOMAIN protein involved in shoot gravitropism, regulates plant architecture in rice. Plant Physiol. 161 (1), 317–295. doi: 10.1104/pp.112.208496
Wu, Z., Zhou, G., Xu, K.-z., Ding, Y., Jiangkuan, N., Ling, F.-l., et al. (2009). Changes in photosynthetic indexes of rice varieties during forty-seven years of genetic improvement in jilin province, china. Chin. J. Rice Sci. 23, 165–171. doi: 10.1016/S1672-6308(08)60075-X
Xia, M. L., Tang, D. Y., Yang, Y. Z., Li, Yi X., Wang, W. W., Lu, H., et al. (2017). Preliminary study on the rice OsYABBY6 gene involving in the regulation of leaf development. Life Sci. Res. 21, 23–30.
Xia, K., Ou, X., Tang, H., Wang, R., Wu, P., Jia, X., et al. (2015). “Rice MicroRNA Osa‐miR1848 Targets the Obtusifoliol 14α-demethylase Gene Os CYP 51G3 and Mediates the Biosynthesis of Phytosterols and Brassinosteroids during Development and in Response to Stress.” New Phytologist 208 (3), 790–802. doi: 10.1111/nph.13513
Xiao, Y., Liu, D., Zhang, G., Tong, H., Chu., C. (2017). Brassinosteroids regulate OFP1, a DLT interacting protein, to modulate plant architecture and grain morphology in rice. Front. Plant Sci. 8 (September). doi: 10.3389/fpls.2017.01698
Xu, P., Cai., W. (2014). RAN1 is involved in plant cold resistance and development in rice (Oryza sativa). J. Exp. Bot. 65 (12), 3277–3875. doi: 10.1093/jxb/eru178
Xu, L., Zhao, H., Ruan, W., Deng, M., Wang, F., Peng, J., et al. (2017). ABNORMAL INFLORESCENCE MERISTEM1 functions in salicylic acid biosynthesis to maintain proper reactive oxygen species levels for root meristem activity in rice. Plant Cell 29 (3), 560–745. doi: 10.1105/tpc.16.00665
Xue, W., Xing, Y., Weng, X., Zhao, Yu, Tang, W., Wang, L., et al. (2008). Natural variation in Ghd7 is an important regulator of heading date and yield potential in rice. Nat. Genet. 40 (6), 761–767. doi: 10.1038/ng.143
Yang, W., Guo, Z., Huang, C., Wang, K. E., Jiang, N. I., Feng, H., et al. (2015). Genome-wide association study of rice ( oryza sativa l.) leaf traits with a high-throughput leaf scorer. J. Exp. Bot. 66 (18), 5605–5155. doi: 10.1093/jxb/erv100
Yasui, Y., Ohmori, Y., Takebayashi, Y., Sakakibara, H., Hirano., H.-Y. (2018). WUSCHEL-RELATED HOMEOBOX4 acts as a key regulator in early leaf development in rice. edited by Sarah hake. PloS Genet. 14 (4), e10073655. doi: 10.1371/journal.pgen.1007365
Ye, M., Wu, M., Zhang, H., Zhang, Z., Zhang., Z. (2021). High leaf vein density promotes leaf gas exchange by enhancing leaf hydraulic conductance in oryza sativa l. plants. Front. Plant Sci. 12 (October). doi: 10.3389/fpls.2021.693815
Yoshida, S. (1972). Physiological aspects of grain yield. Annu. Rev. Plant Physiol. 23 (1), 437–464. doi: 10.1146/annurev.pp.23.060172.002253
Yu, J., Pressoir, G., Briggs, W. H., Bi, I. V., Yamasaki, M., Doebley, J. F., et al. (2006). A unified mixed-model method for association mapping that accounts for multiple levels of relatedness. Nat. Genet. 38 (2), 203–208. doi: 10.1038/ng1702
Zhang, C., Chen, F., Zhao, Z., Hu, L., Liu, H., Cheng, Z., et al. (2018). Mutations in CsPID encoding a Ser/Thr protein kinase are responsible for round leaf shape in cucumber (Cucumis sativus l.). Theor. Appl. Genet. 131 (6), 1379–1895. doi: 10.1007/s00122-018-3084-8
Zhang, T., Feng, P., Li, Y., Yu, P., Yu, G., Sang, X., et al. (2018). VIRESCENT-ALBINO LEAF 1 regulates leaf colour development and cell division in rice. J. Exp. Bot. 69 (20), 4791–4804. doi: 10.1093/jxb/ery250
Zhang, Y.-M., Jia, Z., Dunwell., J. M. (2019). Editorial: The applications of new multi-locus GWAS methodologies in the genetic dissection of complex traits. Front. Plant Sci. 10 (February). doi: 10.3389/fpls.2019.00100
Zhang, G.-H., Li, S.-Y., Wang, L. I., Ye, W.-J., Zeng, D.-L., Rao, Y.-C., et al. (2014). LSCHL4 from japonica cultivar, which is allelic to NAL1 , increases yield of indica super rice 93-11. Mol. Plant 7 (8), 1350–1364. doi: 10.1093/mp/ssu055
Zhang, G.-H., Xu, Q., Zhu, X.-D., Qian, Q., Xue, H.-W. (2009). “SHALLOT-LIKE1 Is a KANADI Transcription Factor That Modulates Rice Leaf Rolling by Regulating Leaf Abaxial Cell Development.” The Plant Cell 21 (3):719–35.doi:10.1105/tpc.108.061457
Zhao, L., Tan, L., Zhu, Z., Xiao, L., Xie, D., Sun., C. (2015) PAY 1 improves plant architecture and enhances grain yield in rice Plant J. 83 (3), 528–365. doi: 10.1111/tpj.12905
Zheng, M., Wang, Y., Liu, X. I., Sun, J., Wang, Y., Xu, Y., et al (2016) The RICE MINUTE-LIKE1 ( RML1 ) gene, encoding a ribosomal Large subunit protein L3B, regulates leaf morphology and plant architecture in rice J. Exp. Bot 67 (11), 3457–3469. doi: 10.1093/jxb/erw167
Zhou, H.-L., He, S.-J., Cao, Y.-R., Chen, T., Du, B.-X., Chu, C.-C., et al (2006) OsGLU1, a putative membrane-bound endo-1,4-ß-D-Glucanase from rice, affects plant internode elongation Plant Mol. Biol 60 (1), 137–515. doi: 10.1007/s11103-005-2972-x
Zhou, F., Lin, Q., Zhu, L., Ren, Y., Zhou, K., Shabek, N., et al (2013) D14–SCFD3-Dependent degradation of D53 regulates strigolactone signalling Nature 504 (7480), 406–410. doi: 10.1038/nature12878
Zhou, W., Malabanan, P. B., Abrigo., E. (2015) OsHox4 regulates GA signaling by interacting with DELLA-like genes and GA oxidase genes in rice. Euphytica 201 (1), 97–1075. doi: 10.1007/s10681-014-1191-4
Keywords: rice, leaf thickness, vein size, vein density, GWAS
Citation: Narawatthana S, Phansenee Y, Thammasamisorn B-O and Vejchasarn P (2023) Multi-model genome-wide association studies of leaf anatomical traits and vein architecture in rice. Front. Plant Sci. 14:1107718. doi: 10.3389/fpls.2023.1107718
Received: 25 November 2022; Accepted: 20 March 2023;
Published: 12 April 2023.
Edited by:
Baltazar Antonio, Japan International Research Center for Agricultural Sciences (JIRCAS), JapanReviewed by:
Sibin Yu, Huazhong Agricultural University, ChinaXueyong Li, Institute of Crop Sciences (CAAS), China
Copyright © 2023 Narawatthana, Phansenee, Thammasamisorn and Vejchasarn. This is an open-access article distributed under the terms of the Creative Commons Attribution License (CC BY). The use, distribution or reproduction in other forums is permitted, provided the original author(s) and the copyright owner(s) are credited and that the original publication in this journal is cited, in accordance with accepted academic practice. No use, distribution or reproduction is permitted which does not comply with these terms.
*Correspondence: Supatthra Narawatthana, c3VwYXR0aHJhLm5AcmljZS5tYWlsLmdvLnRo