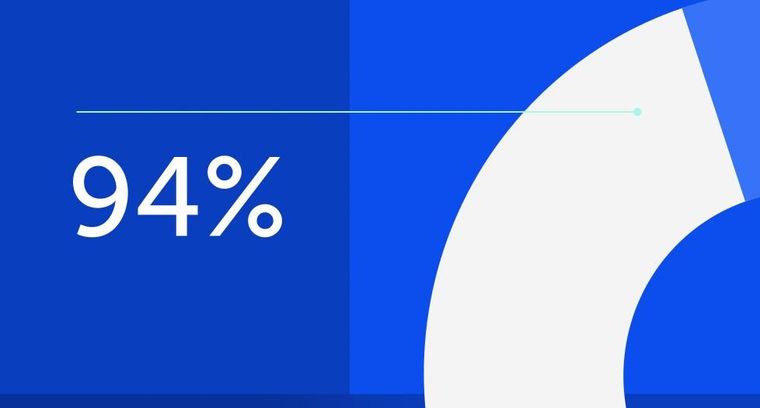
94% of researchers rate our articles as excellent or good
Learn more about the work of our research integrity team to safeguard the quality of each article we publish.
Find out more
ORIGINAL RESEARCH article
Front. Plant Sci., 10 February 2023
Sec. Plant Breeding
Volume 14 - 2023 | https://doi.org/10.3389/fpls.2023.1072233
This article is part of the Research TopicGenetics and Molecular Breeding in Cereal CropsView all 29 articles
Genetic dissection of yield component traits including kernel characteristics is essential for the continuous improvement in wheat yield. In the present study, one recombinant inbred line (RIL) F6 population derived from a cross between Avocet and Chilero was used to evaluate the phenotypes of kernel traits of thousand-kernel weight (TKW), kernel length (KL), and kernel width (KW) in four environments at three experimental stations during the 2018–2020 wheat growing seasons. The high-density genetic linkage map was constructed with the diversity arrays technology (DArT) markers and the inclusive composite interval mapping (ICIM) method to identify the quantitative trait loci (QTLs) for TKW, KL, and KW. A total of 48 QTLs for three traits were identified in the RIL population on the 21 chromosomes besides 2A, 4D, and 5B, accounting for 3.00%–33.85% of the phenotypic variances. Based on the physical positions of each QTL, nine stable QTL clusters were identified in the RILs, and among these QTL clusters, TaTKW-1A was tightly linked to the DArT marker interval 3950546–1213099, explaining 10.31%–33.85% of the phenotypic variances. A total of 347 high-confidence genes were identified in a 34.74-Mb physical interval. TraesCS1A02G045300 and TraesCS1A02G058400 were among the putative candidate genes associated with kernel traits, and they were expressed during grain development. Moreover, we also developed high-throughput kompetitive allele-specific PCR (KASP) markers of TaTKW-1A, validated in a natural population of 114 wheat varieties. The study provides a basis for cloning the functional genes underlying the QTL for kernel traits and a practical and accurate marker for molecular breeding.
Wheat (Triticum aestivum L.) is one of the most important cereal crops and is a major contributor to the diet of 4.5 billion people worldwide, providing approximately 20% of the daily protein and calorie requirements. Consequently, high yield has long been the primary aim in wheat breeding (Guo et al., 2016; Tao et al., 2018; Michel et al., 2019). Kernel traits are important indicators of wheat yield (Li et al., 2018; Ma et al., 2019); understanding the genes that control these traits can provide a theoretical basis and useful information for wheat breeding (Fatiukha et al., 2020; Xin et al., 2020).
With the development of high-throughput molecular biotechnology and functional genomics, research in yield-related traits is becoming more and more convenient (Saini et al., 2022). DNA sequencing technology and single-nucleotide polymorphism (SNP) markers have been widely used in constructing genetic linkage maps (Qu et al., 2022). In recent years, the successful development of wheat diversity arrays technology (DArT) has dramatically accelerated the research on wheat genetic diversity, gene mapping, and cloning (Ahmed et al., 2021). Grain yield, thousand-kernel weight (TKW), kernel length (KL), and kernel width (KW) are widely known complex quantitative traits, which are controlled by a large number of quantitative trait loci (QTLs)/genes (Simmonds et al., 2016; Wang et al., 2018; Guan et al., 2019; Xu et al., 2019) and environmental influences (Kumar et al., 2016; Kumari et al., 2018). Among such traits, TKW has a high and relatively stable heritability (Kuchel et al., 2007; Sharma et al., 2018); meanwhile, relevant research shows that TKW is influenced by KL and KW (Dholakia et al., 2008; Su et al., 2016). Currently, many genes contributing to grain yield have been identified and cloned in crops, such as TGW2 (Ruan et al., 2020), GS3 (Mao et al., 2010), GW7 (Wang et al., 2015), TaTPP-6AL1 (Zhang et al., 2017), TaTGW6 (Hanif et al., 2015), TaGS-D1 (Zhang et al., 2014), and TaGS1a (Guo et al., 2013). A high-yielding gene (OsDREB1C), which was detected in rice, is important to improve photosynthetic efficiency and nitrogen use efficiency, increasing more than 30% of the crop yield (Wei et al., 2022). A kernel length gene (VRT-A2), which was identified on chromosome 7AS between markers XP85 and XP87 with a physical interval of 128.79–128.92 Mb, is a positive regulator of brassinosteroid responses; it encodes an MIKC-type MADS-box protein and significantly increases the kernel length of wheat (Chai et al., 2022).
During the continuous discovery of novel genes, a significant amount of work has been done on gene mining, including QTL mapping, QTL clusters, and pleiotropic QTLs. Many QTLs of kernel traits have been identified on all chromosomes, explaining 0.38%–46.2% of the phenotypic variances (Okamoto et al., 2013; Tyagi et al., 2014; Cheng et al., 2017; Hu et al., 2020; Saini et al., 2022). In addition, some pleiotropic QTLs controlling kernel shape and TKW were discovered on chromosomes 2A, 2B, 2D, 4B, 5B, 5D, and 6A, contributing 3.3%–26.4% of the phenotypic variances (Dholakia et al., 2008; Sun et al., 2008; Ramya et al., 2010; Schierenbeck et al., 2021). Three QTL clusters associated with kernel size were located on chromosomes 1B, 2D, and 6D, accounting for 3.92%–27.78% of the phenotypic variances; the physical position of the QTL clusters is 566.6–583.6 Mb, 481.5–512.8 Mb, and 45.9–73.3 Mb, respectively (Qu et al., 2021). Those gene functions that were associated with kernel traits or kernel weight were mainly affected by three pathways; these pathways are involved in the regulation of cell division and expansion, including phytohormones, G-protein signaling, ubiquitination-mediated proteasomal degradation, and other unknown pathways (Ma et al., 2016; Zhang et al., 2017; Li et al., 2018).
In recent years, with the release of the wheat and closely related species genome sequence, and numerous transcriptome datasets (Duan et al., 2012; Kumar et al., 2015; Yang et al., 2022), all of these might lead to greater convenience for gene mapping, discovery of candidate genes, gene cloning, and development of markers, especially in the area of marker development, such as simple sequence repeat (SSR) markers, cleaved amplified polymorphic sequence (CAPS) markers, kompetitive allele-specific PCR (KASP) markers, and semi-thermal asymmetric reverse PCR (STARP) markers (Wu et al., 2020). The rapid evolution of molecular technology has provided powerful tools to dissect complex traits (Li et al., 2015); many molecular markers for kernel traits have been developed, especially in KASP markers, for example, KASP-AX-111112626 (tightly linked to kernel length QTL QKL.sicau-AM-3B), KASP-AX-108974756 (tightly linked to kernel width QTL QKW.sicau-AM-4B) (Zhou et al., 2021), and KASP-AX-109379070 (tightly linked to kernel length QTL QKL.sicau-2SY-1B) (Qu et al., 2021). The development of these markers has accelerated the rapid development of wheat molecular breeding.
Currently, with the completion of whole-genome sequencing and a fully annotated reference genome of Chinese Spring, and the rapid growth of transcriptomic technologies, the candidate genes can be more conveniently identified and characterized with the help of multiple technologies. The present study is yet another effort to identify the new QTLs of kernel traits and the following related aspects: (1) finding the QTLs for kernel traits, (2) exploring the stable and novel QTL clusters, (3) identifying candidate genes by multiple sequence alignments and gene annotation, and (4) developing KASP markers of the major loci for breeders in breeding programs. We believe that these results should provide useful information not only for molecular breeding but also for basic research on fine mapping and cloning of QTLs in wheat or in other cereals.
The recombinant inbred line (RIL) population of 163 F6 lines was used for QTL analysis of kernel traits in this study, derived from the cross Avocet × Chilero using the single seed descent approach (Basnet et al., 2014). Chilero had significantly higher values (p < 0.05) for all investigated kernel traits than those of Avocet. The International Maize and Wheat Improvement Center (CIMMYT) developed the RIL population.
A natural population with 114 cultivars was utilized for validation of the KASP markers, including 53 wheat accessions collected within the country and 61 cultivars from other countries, such as those in Europe, USA, Mexico, and Australia. Materials were provided by the wheat germplasm innovation and molecular breeding project of Henan University of Science and Technology, China.
For phenotyping, the RIL population and parents were grown under four environments at three experimental stations in three seasons: (1) a test field at the farm of Henan University of Science and Technology (34°C60N″, 112°C42E″), during the autumn–winter cycle of 2018–2019 (2018XN) and 2020–2021 (2020XN) cropping seasons; (2) an open field at Luoning county (34°C42″N, 111°C67″E) in the 2019–2020 (2019LN) cropping season; and (3) a test field at Mengjin county (34°C83″N, 112°C58″E) during the 2020–2021 (2020MJ) cropping season. The natural population of 114 varieties or lines was planted in the three experimental stations at Mengjin county and the farm of Henan University of Science and Technology during 2019–2021.
Each trial of both populations was arranged following a randomized block design with three replicates; the lines of the RIL population and the natural population were grown in a 6.0 m2 plot at each location, with 10 rows, 20 cm apart and 3.0 m in length for each plot. Field management followed the local agronomic practices.
In each experiment, plants were chosen to be harvested after they were completely matured, in order to avoid other factors that may affect the phenotypic analyses. Meanwhile, seeds were dried before analysis. Data parameters of kernel traits were evaluated when all the kernels had approximately 11% moisture content.
The phenotypic values of two populations were determined using the same method. Three kernel traits (TKW, KL, and KW) were measured by using Wanshen SC-A automatic testing equipment, which was developed by Wanshen Science and Technology Ltd. (Hangzhou, Zhejiang, China; www.wseen.com). At least 300 kernels from each line were measured with three replicates, and the average of the three replicates was taken as the evaluation result of each line.
For each phenotypic trait, SPSS version 22.0 software (SPSS, Chicago, USA) was used to conduct statistical analysis of phenotypic data, including the means, standard deviation (SD), range, kurtosis, skewness, and coefficient of variation. The QTL IciMapping v4.1 software was used to compute the broad-sense heritability (H2) and to calculate the best linear unbiased estimate (BLUE) of each kernel trait. The OriginPro 22b software was used to draw the histograms and correlation plot, and the linkage map was drawn using Mapchart.
A total of 23,526 DArTSeq markers were genotyped for both parents and the RIL population, and the QTL IciMapping v4.1 software was used to construct the genetic linkage map and identify significant QTLs (http://www.isbreeding.net) (Zeng et al., 2020). The Kosambi mapping function was used to calculate centiMorgan units (cM), and the inclusive composite interval mapping (ICIM) method was performed for QTL analysis. For all significant QTLs, the critical LOD values were set at 3.0 to increase the reliability and accuracy of QTL detection, and the walking speed parameter of each step for the genome-wide scan was set at 1.0 cM; the significance thresholds were calculated using 1,000 permutations, with genome-wide error rates of 0.10 and a type I error of 0.01. The naming of QTL followed the rule “QTL + trait + research department + chromosome”. The QTLs detected in two or more environments are considered as stable QTLs (Ruan et al., 2021).
In this study, we performed the candidate gene analysis for a highly significant and stable region on wheat chromosome 1AS (http://plants.ensembl.org/index.html). The high-confidence genes were extracted from the IWGSC reference genome and identified using IWGSC RefSeq v1.1 (https://urgi.versailles.inra.fr/) annotation for the identification of likely candidates.
Based on the mapping results, the sequences flanking the QTL TaTKW-1A were used for designing KASP primers (composed of the two forward primers and the reverse primer) (PolyMarker, http://polymarker.tgac.ac.uk/). The primers were synthesized by Sangon Biotech (Shanghai) Co., Ltd. (China).
All KASP reactions were performed in a 4-μl reaction volume, which included 2 μl of diluted DNA, 2 μl of KASP master mix, and 0.045 μl of primer mix. A total of 114 wheat varieties were genotyped on an CFX 384 Real-Time System (BIO-RAD). The fluorescence signals of each reaction well were collected and genotyping was performed using the BioRad CFX Manager Software.
In the four field trials conducted, the means, standard deviation (SD), range, kurtosis, skewness, and coefficient of variation for each of the phenotypes were calculated in the RIL population. The parental genotype Chilero of the mapping population consistently had significantly higher mean values (p < 0.05) for all investigated kernel traits than those of Avocet (Table 1). According to the phenotypic distribution, TKW was larger than KL and KW on the range of variation, and the scores of skewness and kurtosis were mostly less than 1.0 for all kernel traits in the four field trials, indicating that they were quantitative traits controlled by multiple genes. All kernel traits had broad-sense heritability higher than 90% (Table 1).
Table 1 Descriptive statistics of the parental genotypes and RILs for different kernel traits in four environments.
Continuous variations and strong transgressive segregations have been shown for all three traits in the RIL population, suggesting that favorable alleles of these traits are distributed in both parents, and indicating segregation patterns of quantitative traits (Figures 1, 2). Correlations among kernel traits are significant (Figure 3).
Figure 1 Histograms of distributions for wheat kernel traits in the Avocet/Chilero RIL population. Note: 2018XN: The farm of Henan University of Science and Technology 2018–2019, 2019LN: Luoning 2019–2020, 2020XN: The farm of Henan University of Science and Technology 2020–2021, 2020MJ: Mengjin 2020–2021. Green represents 2018XN, red represents 2019LN, blue represents 2020XN, magenta represents 2020MJ, cyan represents mean values, and orange represents BLUE.
In this study, we obtained the results using the ICIM method; a total of 48 QTLs for TKW, KL, and KW were detected and mapped on chromosomes 1A, 1B, 1D, 2B, 2D, 3A, 3B, 3D, 4A, 4B, 5A, 5D, 6A, 6B, 6D, 7A, 7B, and 7D (Tables S1, S2, S3; Figures S1, S2, S3), including 12 QTLs for TKW, 18 QTLs for KL, and 18 QTLs for KW, individually explaining 3.00%–33.85% of the phenotypic variance. Among these QTLs, 9 QTLs for TKW, 12 QTLs for KL, and 13 QTLs for KW explained >10% of the phenotypic variance and were considered the major QTLs.
The sequences of the flanking markers of the QTLs were employed to perform BLASTN against the Chinese Spring reference genome sequence v1.1. According to the physical location of the QTLs, we identified the stable QTL clusters in the Avocet/Chilero RIL population; the detailed information is described in Table 2 and Figure 4.
The results showed that there were eight stable QTL clusters in the population, and they were renamed TaTKW-1A, TaKW-3A, TaTKW-3B, TaKW-3B, TaTKW-4A, TaKW-4B, TaTKW-5A, and TaKL-6A. Among the eight stable QTL clusters, four QTL clusters have the favorable allele from Avocet, TaTKW-1A, TaKW-3A, TaTKW-4A, and TaKL-6A, and four QTL clusters have the favorable allele from Chilero, TaTKW-3B, TaKW-3B, TaKW-4B, and TaTKW-5A. TaTKW-1A was closely linked to DArT markers SNP1158610, SNP1090977, 1010408, SNP100461256, 3950546, and 1213099 in the physical interval of 14.56–49.30 Mb, explaining 10.31%–33.85% of the phenotypic variance. TaKW-3A was tightly linked to marker intervals SNP1093344–100008048 with a physical interval of 69.50–69.51 Mb, accounting for 7.76%–20.42% of the phenotypic variance.
To clarify the physical position of TaTKW-1A, the DArT marker sequence was subjected to alignment with the whole-genome database of Chinese Spring (https://wheat-urgi.versailles.inra.fr/) by using the BLAST tool. Sequence comparison revealed that TaTKW-1A was in a physical interval from 14557761 to 49301348 bp on chromosome 1A (Figure 5). A total of 347 high-confidence genes with a physical length of 34.74 Mb were identified in the DarT marker interval 3950546–1213099 (https://www.wheatgmap.org/).
For the effective utilization of the major QTL in plant breeding, KASP markers closely linked to TaTKW-1A were developed and used to genotype 114 lines (Table 3, Figure 6).
Figure 6 Genotype calling screenshots of the KASP markers. Blue indicates the G allele of Chilero, orange indicates the A allele of Avocet, and black indicates the blank control, **significant at p < 0.01. The same below.
Of the 114 accessions, there were 26 GG (22.8%) genotypes and 88 AA (77.2%) genotypes (Figure 6), and TKW was significantly different (p < 0.01) between the two genotypes; in addition, AA (Avocet) genotypes were higher than GG (Chilero) genotypes. Furthermore, 15 wheat varieties have genotype GG and 38 have genotype AA in the 53 domestic wheat accessions, and 11 have genotype GG and 50 have genotype AA in the 61 foreign varieties (Figure S4).
Wheat has a very huge and complex genome; QTL mapping can provide important information regarding the molecular basis of determination of kernel-related traits. In past decades, more than 400 QTLs for TKW and approximately 200 QTLs for KL and KW have been reported across all 21 chromosomes, and some stable and robust QTLs were detected (Saini et al., 2022). As a whole, these QTLs were mostly distributed across the A and B genomes as compared to the D genome (Yang et al., 2020). A similar trend was observed in this study, with more QTLs on the A (24) and B (14) genomes than on the D (10) genome. Although many QTLs associated with kernel traits have been identified, its application is rarely reported in molecular marker-assisted breeding (MAS), due to the fact that many QTL locations were based on genetic distances rather than the physical distances.
TKW was a complex quantitative trait that was affected by polygenes. Studies indicated that TKW increased gradually when KL and KW increased, and TKW had a significant positive correlation with KL and KW (Gao et al., 2015; Cui et al., 2016; Chai et al., 2022). In this study, the significant correlation is found between TKW and KL, between TKW and KW, and between KL and KW, with the Pearson correlation coefficients of 0.51, 0.90, and 0.20, respectively, which was consistent with the conclusions of other studies (Liu et al., 2014; Michel et al., 2019; Qu et al., 2022).
TKW was a complex polygenic trait with high broad-sense heritability and was less affected by the environment (Cuthbert et al., 2008; Mcintyre et al., 2010; Gao et al., 2015), and QTLs for TKW have been reported on all 21 wheat chromosomes (Huang et al., 2006; Li et al., 2007; Sun et al., 2008; Ramya et al., 2010; Zhang et al., 2014; Shukla et al., 2015; Yu et al., 2018; Chen et al., 2020). In this study, we detected four QTL clusters for TKW, designated TaTKW-1A, TaTKW-3B, TaTKW-4A, and TaTKW-5A. Then, based on the sequence information of the markers flanking these QTLs, we found some genes within each of these QTL intervals by using the BLAST tool.
In earlier studies, many QTLs for TKW were reported on 1A (Pinto et al., 2010; Ramya et al., 2010; Williams and Sorrells, 2014; Wu et al., 2015; Cui et al., 2016; Assanga et al., 2017), 3B (Russo et al., 2014; Xu et al., 2014; Shirdelmoghanloo et al., 2016; Li et al., 2018), 4A (Zou et al., 2017; Li et al., 2020), and 5A (Liu et al., 2014; Fowler et al., 2016; Kumar et al., 2016; Assanga et al., 2017; Brinton et al., 2017). Among the four loci, TaTKW-1A colocalized with QTKW.haust-1A.1, QKW.haust-1A.2, QTKW.haust-1A.2, and QKL.haust-1A.2, with a corresponding physical position of 14557761–49301348 bp on the short arm of chromosome 1A. It overlapped with few published QTLs, such as QGw.ccsu-1A.1 (physical position: 27.27 Mb), QTkw.ncl-1A.1 (physical position: 27.27 Mb), and qTgw.nwipb-1AS (physical interval: 20.02–25.31 Mb), which was detected by Kumar et al. (2006); Ramya et al. (2010), and Liu et al. (2020a), respectively. However, these QTLs were only detected in one environment, and further studies of the locus have not been reported in the literature. TaTKW-4A colocalized with QTKW.haust-4A and QKL.haust-4A.2. The physical interval (584.41–606.37 Mb) of the loci was not identical to that previously identified (Maphosa et al., 2014; Gao et al., 2015; Chen et al., 2020; Li et al., 2020); these loci may be novel loci for TKW. Another colocated QTL, TaTKW-5A (QTKW.haust-5A.1, QTKW.haust-5A.2, and QKW.haust-5A) at 436.63–481.93 Mb, does not coincide with the physical position of other reported QTLs (Wang et al., 2009; Yuan et al., 2017; Guan et al., 2018). TaTKW-3B colocalized with QKW.haust-3B.1 and QTKW.haust-3B.1, and it was localized to the physical interval 7.29–31.66 Mb. We have undertaken a detailed analysis of the loci identified on chromosome 3B in previous studies (Huang et al., 2004; Maphosa et al., 2014; Echeverry-Solarte et al., 2015; Shi et al., 2017; Duan et al., 2020); TaTKW-3B was different from those QTLs reported on 3B, and was likely a new locus.
With respect to KL and KW, many QTLs for KL and KW were previously reported on chromosomes 3A, 3B, 4B, and 6A (Breseghello and Sorrells, 2006; Gegas et al., 2010; Cui et al., 2016; Cheng et al., 2017; Li et al., 2018; Zhai et al., 2018). In our study, we detected one QTL cluster for KL on chromosome 6A (TaKL-6A, physical interval: 61.21–65.66 Mb) and three QTLs for KW on chromosomes 3A (TaKW-3A, physical interval: 69.5–69.51 Mb), 3B (TaKW-3B, physical interval: 490.89–614.57 Mb), and 4B (TaKW-4B, physical interval: 629.7–630.13 Mb). To confirm whether we identified four loci, a comparative analysis of the physical positions of previously reported QTLs with those identified in this study was conducted. According to the physical intervals of TaKL-6A, TaKW-3A, TaKW-3B, and TaKW-4B, we speculated that the four loci were new QTLs (Cui et al., 2011; Cui et al., 2014; Li et al., 2018; Su et al., 2018; Chen et al., 2020; Duan et al., 2020; Xin et al., 2020).
In recent years, with the rapid development of sequencing technology and bioinformatics, a fully annotated reference genome of Chinese Spring was released (IWGSC RefSeq v1.1, https://urgi.versailles.inra.fr/blast_iwgsc/blast.php), providing a better approach in searching for candidate genes. Meanwhile, due to the co-linearity with grasses and the conservation of gene function among different species, many functional markers in wheat have been developed for many cloned genes of kernel traits.
In TaTKW-1A, blasting results showed a physical interval of 14.56–49.30 Mb, and a total of 347 high-confidence genes were found (Figure 5). Among these genes (Figure 7), TraesCS1A02G045300 and TraesCS1A02G058400 were the most promising candidate genes associated with kernel weight, and their orthologs were Os05g0115800 and Os05g0121600 in rice, respectively. Os05g0115800 was involved in the mitogen-activated protein kinase signaling pathway and was a mitogen-activated protein kinase phosphatase, affecting grain yield by regulating the grain number and grain size (Jiang et al., 2019). Os05g0121600 was involved in the regulation of transcription, flower development, seed development, and endosperm development, and acted as a negative regulator in starch synthesis (Seetharam et al., 2021). In this study, we speculated candidate genes for TKW on chromosomes 4A and 5A. The physical interval of TaTKW-4A was 584.41–606.37 Mb, and 406 annotated genes were presumed. TraesCS4A02G293900, TraesCS4A02G294000, and TraesCS4A02G303500 were the candidate genes for TKW, and the orthologs were Os03g0669100, At4g34460, and At3g21510, respectively. Among these genes, Os03g0669100 and At4g34460 encoded a regulator of G-protein signaling (RGB1 and AGB1) (Oliveira et al., 2022), and At3g21510 (AHPs) and its encoded protein are related to the regulation of endosperm growth (Tran et al., 2021). RGB1, AGB1, and AHPs were involved in the regulation of grain traits (Li et al., 2019; Li et al., 2021). In TaTKW-5A, the physical interval was 436.63–481.93 Mb, and there were 512 high-confidence genes. Os09g0448500 of the ortholog of TraesCS5A02G233400 was a transcriptional regulator in rice and was associated with kernel traits (Li et al., 2019; Zhou and Xue, 2020). Consequently, TraesCS4A02G293900, TraesCS4A02G294000, TraesCS4A02G303500, and TraesCS5A02G233400 were the candidate genes on 4A and 5A chromosomes in wheat (Figure 7). In TaTKW-3B, the physical interval was 7.29–31.66 Mb; a total of 418 annotated genes were found in the physical intervals, but we did not find orthologs related to TKW.
Figure 7 Cloned genes affecting kernel traits in candidate intervals. (A) Cloned gene of TaTKW-1A. (B) Cloned gene of TaTKW-4A. (C) Cloned gene of TaTKW-5A.
Previous studies have shown that the growth of maternal tissues is able to control seed size through several signaling pathways, including the ubiquitin–proteasome pathway (Huang et al., 2017; Xie et al., 2018), G-protein signaling (Liu et al., 2018; Sun et al., 2018), mitogen-activated protein kinase signaling (Guo et al., 2018; Xu et al., 2018), phytohormones (Xu et al., 2015; Zhou et al., 2017), and transcriptional regulators (Wang et al., 2015; Segami et al., 2017). In our study, we found TaTKW-1A, which has two major candidate genes, TraesCS1A02G045300 and TraesCS1A02G058400, with grain-related traits (Figure 7). We identified them in the transcriptome of wheat grain through the website https://www.ebi.ac.uk/gxa/home (Gillies et al., 2012; Li et al., 2013; Takafuji et al., 2021; Yu et al., 2021) (Figure S5). Results showed that both genes were expressed during grain development, although the expression profiles of these two genes clearly differ during grain development; both of them were expressed in the pericarp, endosperm, and seed coat (Li et al., 2013; Yu et al., 2021). Specifically, TraesCS1A02G045300 is important because it was expressed consistently from anthesis to maturity (Pfeifer et al., 2014; Pearce et al., 2015; Yu et al., 2021).
With the rapid development of marker-assisted selection in wheat breeding, the molecular marker technology has received increasing attention in recent years in crops (Song et al., 2022), and numerous markers have been developed (Kang et al., 2020; Rambla et al., 2022; Shin et al., 2022). A CAPS marker, TaTPP-6AL1-CAPS, was developed to differentiate TaTPP-6AL1a and TaTPP-6AL1b, which was associated with TKW (Zhang et al., 2017). A KASP marker of the candidate gene TaFT-D1, which was associated with TKW and KW, was developed and verified in a natural population (Liu et al., 2020b). Ten SSR markers for grain weight were developed and tested in 60 genotypes; all SSR primers had a high polymorphism (Sallam et al., 2019). In particular, numerous KASP markers for kernel traits have been developed in the last 2 years: Kasp_5B_Tgw for QTgw.caas-5B was developed and validated in wheat (Zhao et al., 2021), a KASP functional marker of TaTAP46-5A associated with kernel weight in wheat was developed and identified (Zhang et al., 2021), the KASP markers for QTKW.caas-5DL (Song et al., 2022), and the KASP markers for QGl.cib-4A (Li et al., 2022). These KASP markers provide a robust tool for genetic mapping and molecular breeding in crops. In this study, in order to use the advantage haplotypes, we developed the KASP markers of TaTKW-1A, which have been validated in the natural population. The results showed that the KASP markers could be used in wheat.
In this study, 48 QTLs were found in the RIL population, explaining 3.00%–33.85% of the phenotypic variances. Nine QTL clusters for kernel traits were identified in the RILs, and among these QTL clusters, we developed and validated the KASP markers of TaTKW-1A, and two candidate genes were predicted. The KASP markers and predicted candidate genes will be valuable for fine mapping and cloning the functional genes in wheat breeding.
The original contributions presented in the study are included in the article/Supplementary Material. Further inquiries can be directed to the corresponding author.
CW (Chunping Wang) provided the test materials, performed the experiment, and revised the manuscript; ZZ participated in the trials, constructed the linkage maps, and wrote the paper. ZD participated in the development of the KASP markers. All authors read the final version of the manuscript and approved it for publication.
This work was supported by the National Key Research and Development Program of China (2018YFD0100904), the Natural Science Foundation of Henan Province (162300410077), and the International Cooperation Project of Henan Province (172102410052).
We are grateful to Profs. Xianchun Xia and Akshay Kumar Biswal for the critical review of this manuscript. We thank the referees for critically reading and revising this manuscript.
The authors declare that the research was conducted in the absence of any commercial or financial relationships that could be construed as a potential conflict of interest.
All claims expressed in this article are solely those of the authors and do not necessarily represent those of their affiliated organizations, or those of the publisher, the editors and the reviewers. Any product that may be evaluated in this article, or claim that may be made by its manufacturer, is not guaranteed or endorsed by the publisher.
Ahmed, A. A. M., Mohamed, E. A., Hussein, M. Y., Sallam, A. (2021). Genomic regions associated with leaf wilting traits under drought stress in spring wheat at the seedling stage revealed by GWAS. Environ. Exp. Bot. 184, 104393. doi: 10.1016/j.envexpbot.2021.104393
Assanga, S. O., Fuentealba, M., Zhang, G., Tan, C., Dhakal, S., Rudd, J. C., et al. (2017). Mapping of quantitative trait loci for grain yield and its components in a US popular winter wheat TAM 111 using 90K SNPs. PloS One 12, e0189669. doi: 10.1371/journal.pone.0189669
Basnet, B. R., Singh, G. P., Ibrahim, A. M. H., Herrera-Foessel, S. A., Huerta-Espino, J., Lan, C., et al. (2014). Characterization of Yr54 and other genes associated with adult plant resistance to yellow rust and leaf rust in common wheat quaiu 3. Mol. Breed. 33, 385–399. doi: 10.1007/s11032-013-9957-2
Breseghello, F., Sorrells, M. E. (2006). Association mapping of kernel size and milling quality in wheat (Triticum aestivum l.) cultivars. Genet 172, 1165–1177. doi: 10.1534/genetics.105.044586
Brinton, J., Simmonds, J., Minter, F., Leverington-Waite, M., Snape, J., Uauy, C. (2017). Increased pericarp cell length underlies a major quantitative trait locus for grain weight in hexaploid wheat. New Phytol. 215, 1026–1038. doi: 10.1111/nph.14624
Chai, S., Yao, Q., Liu, R., Xiang, W., Xiao, X., Fan, X., et al. (2022). Identification and validation of a major gene for kernel length at the P1 locus in Triticum polonicum. Crop J. 10, 387–396. doi: 10.1016/j.cj.2021.07.006
Chen, Z., Cheng, X., Chai, L., Wang, Z., Bian, R., Li, J., et al. (2020). Dissection of genetic factors underlying grain size and fine mapping of QTgw.cau-7D in common wheat (Triticum aestivum l.). Theor. Appl. Genet. 133, 149–162. doi: 10.1007/s00122-019-03447-5
Cheng, R., Kong, Z., Zhang, L., Xie, Q., Jia, H., Yu, D., et al. (2017). Mapping QTLs controlling kernel dimensions in a wheat inter-varietal RIL mapping population. Theor. Appl. Genet. 130, 1405–1414. doi: 10.1007/s00122-017-2896-2
Cui, F. A., Ding, A., Li, J., Zhao, C., Li, X., Feng, D., et al. (2011). Wheat kernel dimensions: how do they contribute to kernel weight at an individual QTL level? J. Genet. 90, 409–425. doi: 10.1007/s12041-011-0103-9
Cui, F., Fan, X., Chen, M., Zhang, N., Zhao, C., Zhang, W., et al. (2016). QTL detection for wheat kernel size and quality and the responses of these traits to low nitrogen stress. Theor. Appl. Genet. 129, 469–484. doi: 10.1007/s00122-015-2641-7
Cui, F., Zhao, C., Ding, A., Li, J., Wang, L., Li, X., et al. (2014). Construction of an integrative linkage map and QTL mapping of grain yield-related traits using three related wheat RIL populations. Theor. Appl. Genet. 127, 659–675. doi: 10.1007/s00122-013-2249-8
Cuthbert, J. L., Somers, D. J., Brule-Babel, A. L., Brown, P. D., Crow, G. H. (2008). Molecular mapping of quantitative trait loci for yield and yield components in spring wheat (Triticum aestivum l.). Theor. Appl. Genet. 117, 595–608. doi: 10.1007/s00122-008-0804-5
Dholakia, B. B., Ammiraju, J. S. S., Singh, H., Lagu, M. D., Röder, M. S., Rao, V. S., et al. (2008). Molecular marker analysis of kernel size and shape in bread wheat. Plant Breed. 122, 392–395. doi: 10.1046/j.1439-0523.2003.00896.x
Duan, J., Xian, C., Zhao, G., Jia, J., Kong, X. (2012). Optimizing de novo common wheat transcriptome assembly using short-read RNA-seq data. BMC Genomics 13, 392. doi: 10.1186/1471-2164-13-392
Duan, X., Yu, H., Ma, W., Sun, J., Zhao, Y., Yang, R., et al. (2020). A major and stable QTL controlling wheat thousand grain weight: identification, characterization, and CAPS marker development. Mol. Breed. 40, 68. doi: 10.1007/s11032-020-01147-3
Echeverry-Solarte, M., Kumar, A., Kianian, S., Simsek, S., Alamri, M. S., Mantovani, E. E., et al. (2015). New QTL alleles for quality-related traits in spring wheat revealed by RIL population derived from supernumerary x non-supernumerary spikelet genotypes. Theor. Appl. Genet. 128, 893–912. doi: 10.1007/s00122-015-2478-0
Fatiukha, A., Filler, N., Lupo, I., Lidzbarsky, G., Klymiuk, V., Korol, A. B., et al. (2020). Grain protein content and thousand kernel weight QTLs identified in a durum x wild emmer wheat mapping population tested in five environments. Theor. Appl. Genet. 133, 119–131. doi: 10.1007/s00122-019-03444-8
Fowler, D. B., N'diaye, A., Laudencia-Chingcuanco, D., Pozniak, C. J. (2016). Quantitative trait loci associated with phenological development, low-temperature tolerance, grain quality, and agronomic characters in wheat (Triticum aestivum l.). PloS One 11, e0152185. doi: 10.1371/journal.pone.0152185
Gao, F., Wen, W., Liu, J., Rasheed, A., Yin, G., Xia, X., et al. (2015). Genome-wide linkage mapping of QTL for yield components, plant height and yield-related physiological traits in the Chinese wheat cross zhou 8425B/Chinese spring. Front. Plant Sci. 6. doi: 10.3389/fpls.2015.01099
Gegas, V. C., Nazari, A., Griffiths, S., Simmonds, J., Fish, L., Orford, S., et al. (2010). A genetic framework for grain size and shape variation in wheat. Plant Cell 22, 1046–1056. doi: 10.1105/tpc.110.074153
Gillies, S. A., Futardo, A., Henry, R. J. (2012). Gene expression in the developing aleurone and starchyendosperm of wheat. Plant Biotechnol. J. 10, 668–679. doi: 10.1111/j.1467-76
Guan, P., Di, N., Mu, Q., Shen, X., Wang, Y., Wang, X., et al. (2019). Use of near-isogenic lines to precisely map and validate a major QTL for grain weight on chromosome 4AL in bread wheat (Triticum aestivum l.). Theor. Appl. Genet. 132, 2367–2379. doi: 10.1007/s00122-019-03359-4
Guan, P., Lu, L., Jia, L., Kabir, M. R., Zhang, J., Lan, T., et al. (2018). Global QTL analysis identifies genomic regions on chromosomes 4A and 4B harboring stable loci for yield-related traits across different environments in wheat (Triticum aestivum l.). Front. Plant Sci. 9. doi: 10.3389/fpls.2018.00529
Guo, L., Wang, Z., Wang, S., Liu, Z., Liu, X., Hu, S., et al. (2016). QTL mapping of wheat grain quality traits based on SRAP and SSR marker. J. Triticeae Crops 36, 1275–1282. doi: 10.7606/j.issn.1009-1041.2016.10.02
Guo, T., Chen, K., Dong, N., Shi, C., Ye, W., Gao, J., et al. (2018). GRAIN SIZE AND NUMBER1 negatively regulates the OsMKKK10-OsMKK4-OsMPK6 cascade to coordinate the trade-off between grain number per panicle and grain size in rice. Plant Cell 30, 871–888. doi: 10.1105/tpc.17.00959
Guo, Y., Sun, J., Zhang, G., Wang, Y., Kong, F., Zhao, Y., et al. (2013). Haplotype, molecular marker and phenotype effects associated with mineral nutrient and grain size traits of TaGS1a in wheat. Field Crops Res. 154, 119–125. doi: 10.1016/j.fcr.2013.07.012
Hanif, M., Gao, F., Liu, J., Wen, W., Zhang, Y., Rasheed, A., et al. (2015). TaTGW6-A1, an ortholog of rice TGW6, is associated with grain weight and yield in bread wheat. Mol. Breed. 36, 1. doi: 10.1007/s11032-015-0425-z
Hu, J., Wang, X., Zhang, G., Jiang, P., Chen, W., Hao, Y., et al. (2020). QTL mapping for yield-related traits in wheat based on four RIL populations. Theor. Appl. Genet. 133, 917–933. doi: 10.1007/s00122-019-03515-w
Huang, K., Wang, D., Duan, P., Zhang, B., Xu, R., Li, N., et al. (2017). WIDE AND THICK GRAIN 1, which encodes an otubain-likeprotease with deubiquitination activity, influences grain sizeand shape in rice. Plant J. 91, 849–860. doi: 10.1111/tpj.13613
Huang, X. Q., Cloutier, S., Lycar, L., Radovanovic, N., Humphreys, D. G., Noll, J. S., et al. (2006). Molecular detection of QTLs for agronomic and quality traits in a doubled haploid population derived from two Canadian wheats (Triticum aestivum l.). Theor. Appl. Genet. 113, 753–766. doi: 10.1007/s00122-006-0346-7
Huang, X. Q., Kempf, H., Ganal, M. W., Roder, M. S. (2004). Advanced backcross QTL analysis in progenies derived from a cross between a German elite winter wheat variety and a synthetic wheat (Triticum aestivum l.). Theor. Appl. Genet. 109, 933–943. doi: 10.1007/s00122-004-1708-7
Jiang, L., Li, G., Chern, M., Jain, R., Pham, N. T., Martin, J. A., et al. (2019). Whole-genome sequencing identifies a rice grain shape mutant, gs9-1. Rice 12, 52. doi: 10.1186/s12284-019-0308-8
Kang, J. W., Kabange, N. R., Phyo, Z., Park, S. Y., Lee, S. M., Lee, J. Y., et al. (2020). Combined linkage mapping and genome-wide association study identified QTLs associated with grain shape and weight in rice (Oryza sativa l.). Agronomy 10, 1532. doi: 10.3390/agronomy10101532
Kuchel, H., Williams, K., Langridge, P., Eagles, H. A., Jefferies, S. P. (2007). Genetic dissection of grain yield in bread wheat. II. QTL-by-environment interaction. Theor. Appl. Genet. 115, 1015–1027. doi: 10.1007/s00122-007-0628-8
Kumar, A., Mantovani, E. E., Seetan, R., Soltani, A., Echeverry-Solarte, M., Jain, S., et al. (2016). Dissection of genetic factors underlying wheat kernel shape and size in an elite x nonadapted cross using a high density SNP linkage map. Plant Genome 9, 1–22. doi: 10.3835/plantgenome2015.09.0081
Kumar, N., Kulwal, P. L., Gaur, A., Tyagi, A. K., Khurana, J. P., Khurana, P., et al. (2006). QTL analysis for grain weight in common wheat. Euphytica 151, 135–144. doi: 10.1007/s10681-006-9133-4
Kumar, R. R., Goswami, S., Sharma, S. K., Kala, Y. K., Rai, G. K., Mishra, D. C., et al. (2015). Harnessing next generation sequencing in climate change: RNA-seq analysis of heat stress-responsive genes in wheat (Triticum aestivum l.). OMICS 19, 632–647. doi: 10.1089/omi.2015.0097
Kumari, S., Jaiswal, V., Mishra, V. K., Paliwal, R., Balyan, H. S., Gupta, P. K. (2018). QTL mapping for some grain traits in bread wheat (Triticum aestivum l.). Physiol. Mol. Biol. Plants 24, 909–920. doi: 10.1007/s12298-018-0552-1
Li, F., Wen, W., He, Z., Liu, J., Jin, H., Cao, S., et al. (2018). Genome-wide linkage mapping of yield-related traits in three Chinese bread wheat populations using high-density SNP markers. Theor. Appl. Genet. 131, 1903–1924. doi: 10.1007/s00122-018-3122-6
Li, H. Z., Gao, X., Li, X. Y., Chen, Q. J., Dong, J., Zhao, W. C. (2013). Evaluation of assembly strategies using RNA-seq data associated with grain development of wheat (Triticum aestivum l.). PloS One 8, e83530. doi: 10.1371/journal.pone.0083530
Li, N., Xu, R., Li, Y. (2019). Molecular networks of seed size control in plants. Annu. Rev. Plant Biol. 70, 435–463. doi: 10.1146/annurev-arplant-050718-095851
Li, S., Jia, J., Wei, X., Zhang, X., Li, L., Chen, H., et al. (2007). A intervarietal genetic map and QTL analysis for yield traits in wheat. Mol. Breed. 20, 167–178. doi: 10.1007/s11032-007-9080-3
Li, S., Wang, J., Zhang, L. (2015). Inclusive composite interval mapping of QTL by environment interactions in biparental populations. PloS One 10, e0132414. doi: 10.1371/journal.pone.0132414
Li, T., Deng, G., Su, Y., Yang, Z., Tang, Y., Wang, J., et al. (2022). Genetic dissection of quantitative trait loci for grain size and weight by high−resolution genetic mapping in bread wheat (Triticum aestivum l.). Theor. Appl. Genet. 135, 257–271. doi: 10.1007/s00122-021-03964-2
Li, Y., Xiong, H., Guo, H., Zhou, C., Xie, Y., Zhao, L., et al. (2020). Identification of the vernalization gene VRN-B1 responsible for heading date variation by QTL mapping using a RIL population in wheat. BMC Plant Biol. 20, 331. doi: 10.1186/s12870-020-02539-5
Li, Y. J., Yu, Y., Liu, X., Zhang, X., Su, Y. (2021). The arabidopsis MATERNAL EFFECT EMBRYO ARREST45 protein modulates maternal auxin biosynthesis and controls seed size by inducing AINTEGUMENTA. Plant J. 33, 1907–1926. doi: 10.1093/plcell/koab084
Liu, G., Jia, L., Lu, L., Qin, D., Zhang, J., Guan, P., et al. (2014). Mapping QTLs of yield-related traits using RIL population derived from common wheat and Tibetan semi-wild wheat. Theor. Appl. Genet. 127, 2415–2432. doi: 10.1007/s00122-014-2387-7
Liu, H., Zhang, X., Xu, Y., Ma, F., Zhang, J., Cao, Y., et al. (2020b). Identification and validation of quantitative trait loci for kernel traits in common wheat (Triticum aestivum l.). BMC Plant Biol. 20, 529. doi: 10.1186/s12870-020-02661-4
Liu, Q., Han, R., Wu, K., Zhang, J., Ye, Y., Wang, S., et al. (2018). G-Protein βγ subunits determine grain size through interaction with MADS-domain transcription factors in rice. Nat. Commun. 9, 852. doi: 10.1038/s41467-018-03047-9
Liu, T., Wu, L., Gan, X., Chen, W., Liu, B., Fedak, G., et al. (2020a). Mapping quantitative trait loci for 1000-grain weight in a double haploid population of common wheat. Int. J. Mol. Sci. 21, 3960. doi: 10.3390/ijms21113960
Ma, J., Zhang, H., Li, S., Zou, Y., Li, T., Liu, J., et al. (2019). Identification of quantitative trait loci for kernel traits in a wheat cultivar Chuannong16. BMC Genet. 20, 77. doi: 10.1186/s12863-019-0782-4
Ma, L., Li, T., Hao, C., Wang, Y., Chen, X., Zhang, X. (2016). TaGS5-3A, a grain size gene selected during wheat improvement for larger kernel and yield. Plant Biotechnol. J. 14, 1269–1280. doi: 10.1111/pbi.12492
Mao, H., Sun, S., Yao, J., Wang, C., Yu, S., Xu, C., et al. (2010). Linking differential domain functions of the GS3 protein to natural variation of grain size in rice. PANS 107, 19579–19584. doi: 10.1073/pnas.101441910
Maphosa, L., Langridge, P., Taylor, H., Parent, B., Emebiri, L. C., Kuchel, H., et al. (2014). Genetic control of grain yield and grain physical characteristics in a bread wheat population grown under a range of environmental conditions. Theor. Appl. Genet. 127, 1607–1624. doi: 10.1007/s00122-014-2322-y
Mcintyre, C. L., Mathews, K. L., Rattey, A., Chapman, S. C., Drenth, J., Ghaderi, M., et al. (2010). Molecular detection of genomic regions associated with grain yield and yield-related components in an elite bread wheat cross evaluated under irrigated and rainfed conditions. Theor. Appl. Genet. 120, 527–541. doi: 10.1007/s00122-009-1173-4
Michel, S., Loschenberger, F., Ametz, C., Pachler, B., Sparry, E., Burstmayr, H. (2019). Combining grain yield, protein content and protein quality by multi-trait genomic selection in bread wheat. Theor. Appl. Genet. 132, 2767–2780. doi: 10.1007/s00122-019-03386-1
Okamoto, Y., Nguyen, A. T., Yoshioka, M., Iehisa, J. C., Takumi, S. (2013). Identification of quantitative trait loci controlling grain size and shape in the d genome of synthetic hexaploid wheat lines. Breed. Sci. 63, 423–429. doi: 10.1270/jsbbs.63.423
Oliveira, C. C., Jones, A. M., Fontes, E. P. B., dos Reis, P. A. B. (2022). G-Protein phosphorylation: Aspects of binding specificity and function in the plant kingdom. Int. J. Mol. Sci. 23, 6544. doi: 10.3390/ijms23126544
Pearce, S., Huttly, A. K., Prosser, I. M., Li, Y., Vaughan, S. P., Gallova, B., et al. (2015). Heterologous expression and transcript analysis of gibberellin biosynthetic genes of grasses reveals novel functionality in the GA3ox family. BMC Plant Biol. 15, 130. doi: 10.1186/s12870-015-0520-7
Pfeifer, M., Kugler, K. G., Sandve, S. R., Zhan, B., Rudi, H., Hvidsten, T. R. (2014). Genome interplay in the grain transcriptome of hexaploid bread wheat. Science 345, 1250091. doi: 10.1126/science.1250091
Pinto, R. S., Reynolds, M. P., Mathews, K. L., Mcintyre, C. L., Olivares-Villegas, J. J., Chapman, S. C. (2010). Heat and drought adaptive QTL in a wheat population designed to minimize confounding agronomic effects. Theor. Appl. Genet. 121, 1001–1021. doi: 10.1007/s00122-010-1351-4
Qu, X., Li, C., Liu, H., Liu, J., Luo, W., Xu, Q., et al. (2022). Quick mapping and characterization of a co−located kernel length and thousand−kernel weight−related QTL in wheat. Theor. Appl. Genet. 135, 2849–2860. doi: 10.1007/s00122-022-04154-4
Qu, X., Liu, J., Xie, X., Xu, Q., Tang, H., Mu, Y., et al. (2021). Genetic mapping and validation of loci for kernel-related traits in wheat (Triticum aestivum l.). Front. Plant Sci. 12. doi: 10.3389/fpls.2021.66749
Rambla, C., Meer, S. V. D., P.Voss-Fels, K., Makhoul, M., Obermeier, C., Snowdon, R., et al. (2022). A toolkit to rapidly modify root systems through single plant selection. Plang Methods 18, 2. doi: 10.1186/s13007-021-00834-2
Ramya, P., Chaubal, A., Kulkarni, K., Gupta, L., Kadoo, N., Dhaliwal, H. S., et al. (2010). QTL mapping of 1000-kernel weight, kernel length, and kernel width in bread wheat (Triticum aestivum l.). J. Appl. Genet. 51, 421–429. doi: 10.1007/BF03208872
Ruan, B., Shang, L., Zhang, B., Hu, J., Wang, Y., Lin, H., et al. (2020). Natural variation in the promoter of TGW2 determines grain width and weight in rice. New Phytol. 227, 629–640. doi: 10.1111/nph.16540
Ruan, Y., Yu, B., Knox, R. E., Zhang, W., Singh, A. K., Cuthber, R., et al. (2021). Conditional mapping identified quantitative trait loci for grain protein concentration expressing independently of grain yield in Canadian durum wheat. Front. Plant Sci. 12. doi: 10.3389/fpls.2021.64295
Russo, M. A., Ficco, D. B. M., Laido`, G., Marone, D., Papa, R., Blanco, A., et al. (2014). A dense durum wheat × T. dicoccum linkage map based on SNP markers for the study of seed morphology. Mol. Breed. 34, 1579–1597. doi: 10.1007/s11032-014-0181-5
Saini, D. K., Srivastava, P., Pal, N., Gupta, P. K. (2022). Meta-QTLs, ortho-metaQTLs and candidate genes for grain yield and associated traits in wheat (Triticum aestivum l.). Theor. Appl. Genet. 135, 1049–1081. doi: 10.1007/s00122-021-04018-3
Sallam, A., Amro, A., Elakhdar, A., Dawood, M. F. A., Moursi, Y. S., Baenziger, P. S. (2019). Marker-trait association for grain weight of spring barley in well-watered and drought environments. Mol. Biol. Rep. 46, 2907–2918. doi: 10.1007/s11033-019-04750-6
Schierenbeck, M., Alqudah, A. M., Lohwasser, U., Tarawneh, R. A., Simón, M. R., Börner, A. (2021). Genetic dissection of grain architecture-related traits in a winter wheat population. BMC Plant Biol. 21, 440. doi: 10.1186/s12870-021-03216-x
Seetharam, A. S., Yu, Y., Bélanger, S., Clark, L. G., Meyers, B. C., Kellogg, E. A., et al. (2021). The streptochaeta genome and the evolution of the grasses. Front. Plant Sci. 12. doi: 10.3389/fpls.2021.710383
Segami, S., Takehara, K., Yamamoto, T., Kido, S., Kondo, S., Iwasaki, Y., et al. (2017). Overexpression of SRS5 improves grain size of brassinosteroid-related dwarf mutants in rice (Oryza sativa l.). Breed. Sci. 67, 393–397. doi: 10.1270/jsbbs.16198
Sharma, R., Draicchio, F., Bull, H., Herzig, P., Maurer, A., Pillen, K., et al. (2018). Genome-wide association of yield traits in a nested association mapping population of barley reveals new gene diversity for future breeding. J. Exp. Bot. 69, 3811–3822. doi: 10.1093/jxb/ery178
Shi, S., Azam, F. I., Li, H., Chang, X., Li, B., Jing, R. (2017). Mapping QTL for stay-green and agronomic traits in wheat under diverse water regimes. Euphytica 213, 246. doi: 10.1007/s10681-017-2002-5
Shin, Y., Won, Y. J., Lee, C., Cheon, K.-S., Oh, H., Lee, G.-S., et al. (2022). Identification of grain size-related QTLs in Korean japonica rice using genome resequencing and high-throughput image analysis. Agriculture 12, 51. doi: 10.3390/agriculture12010051
Shirdelmoghanloo, H., Taylor, J. D., Lohraseb, I., Rabie, H., Brien, C., Timmins, A., et al. (2016). A QTL on the short arm of wheat (Triticum aestivum l.) chromosome 3B affects the stability of grain weight in plants exposed to a brief heat shock early in grain filling. BMC Plant Biol. 16, 100. doi: 10.1186/s12870-016-0784-6
Shukla, S., Singh, K., Patil, R. V., Kadam, S., Bharti, S., Prasad, P., et al. (2015). Genomic regions associated with grain yield under drought stress in wheat (Triticum aestivum l.). Euphytica 203, 449–467. doi: 10.1007/s10681-014-1314-y
Simmonds, J., Scott, P., Brinton, J., Mestre, T. C., Bush, M., Del Blanco, A., et al. (2016). A splice acceptor site mutation in TaGW2-A1 increases thousand grain weight in tetraploid and hexaploid wheat through wider and longer grains. Theor. Appl. Genet. 129, 1099–1112. doi: 10.1007/s00122-016-2686-2
Song, J., Xu, D., Dong, Y., Li, F., Bian, Y., Li, L., et al. (2022). Fine mapping and characterization of a major QTL for grain weight on wheat chromosome arm 5DL. Theor. Appl. Genet. 135, 3237–3246. doi: 10.1007/s00122-022-04182-0
Su, Q., Zhang, X., Zhang, W., Zhang, N., Song, L., Liu, L., et al. (2018). QTL detection for kernel size and weight in bread wheat (Triticum aestivum l.) using a high-density SNP and SSR-based linkage map. Front. Plant Sci. 9. doi: 10.3389/fpls.2018.01484
Su, Z., Jin, S., Lu, Y., Zhang, G., Chao, S., Bai, G. (2016). Single nucleotide polymorphism tightly linked to a major QTL on chromosome 7A for both kernel length and kernel weight in wheat. Mol. Breed. 36, 15. doi: 10.1007/s11032-016-0436-4
Sun, S., Wang, L., Mao, H., Shao, L., Li, X., Xiao, J., et al. (2018). A G-protein pathway determines grain size in rice. Nat. Commun. 9, 851. doi: 10.1038/s41467-018-03141-y
Sun, X. Y., Wu, K., Zhao, Y., Kong, F. M., Han, G. Z., Jiang, H. M., et al. (2008). QTL analysis of kernel shape and weight using recombinant inbred lines in wheat. Euphytica 165, 615–624. doi: 10.1007/s10681-008-9794-2
Takafuji, T., Shimizu-Sato, S., Ta, K. N., Suzuki, T., Nosaka-Takahashi, M., Oiwa, T., et al. (2021). High-resolution spatiotemporal transcriptome analyses during cellularization of rice endosperm unveil the earliest gene regulation critical for aleurone and starchy endosperm cell fate specification. J. Plant Res. 134, 1061–1081. doi: 10.1007/s10265-021-01329-w
Tao, Z., Chang, X., Wang, D., Wang, Y., Ma, S., Yang, Y., et al. (2018). Effects of sulfur fertilization and short-term high temperature on wheat grain production and wheat flour proteins. Crop J. 6, 413–425. doi: 10.1016/j.cj.2018.01.007
Tran, L. H., Urbanowicz, A., Jasinski, M., Jasiński, M., Ruszkowski, M. (2021). 3D domain swapping dimerization of the receiver domain of cytokinin receptor CRE1 from Arabidopsis thaliana and Medicago truncatula. Front. Plant Sci. 12. doi: 10.3389/fpls.2021.756341
Tyagi, S., Mir, R. R., Balyan, H. S., Gupta, P. K. (2014). Interval mapping and meta-QTL analysis of grain traits in common wheat (Triticum aestivum l.). Euphytica 201, 367–380. doi: 10.1007/s10681-014-1217-y
Wang, R. X., Hai, L., Zhang, X. Y., You, G. X., Yan, C. S., Xiao, S. H. (2009). QTL mapping for grain filling rate and yield-related traits in RILs of the Chinese winter wheat population heshangmai x Yu8679. Theor. Appl. Genet. 118, 313–325. doi: 10.1007/s00122-008-0901-5
Wang, S., Li, S., Liu, Q., Wu, K., Zhang, J., Wang, S., et al. (2015). The OsSPL16-GW7 regulatory module determines grain shape and simultaneously improves rice yield and grain quality. Nat. Genet. 47, 949–954. doi: 10.1038/ng.3352
Wang, W., Simmonds, J., Pan, Q., Davidson, D., He, F., Battal, A., et al. (2018). Gene editing and mutagenesis reveal inter-cultivar differences and additivity in the contribution of TaGW2 homoeologues to grain size and weight in wheat. Theor. Appl. Genet. 131, 2463–2475. doi: 10.1007/s00122-018-3166-7
Wei, S., Li, X., Lu, Z., Zhang, H., Ye, X., Zhou, Y., et al. (2022). A transcriptional regulator that boosts grain yields and shortens the growth duration of rice. Science 377, 386. doi: 10.1126/science.abi8455
Williams, K., Sorrells, M. E. (2014). Three-dimensional seed size and shape QTL in hexaploid wheat (Triticum aestivum l.) populations. Crop Sci. 54, 98–110. doi: 10.2135/cropsci2012.10.0609
Wu, Q. H., Chen, Y. X., Zhou, S. H., Fu, L., Chen, J. J., Xiao, Y., et al. (2015). High-density genetic linkage map construction and QTL mapping of grain shape and size in the wheat population Yanda1817 x Beinong6. PloS One 10, e0118144. doi: 10.1371/journal.pone.0118144
Wu, Y., Li, M., He, Z., Dreisigacker, S., Wen, W., Jin, H., et al. (2020). Development and validation of high−throughput and low−cost STARP assays for genes underpinning economically important traits in wheat. Theor. Appl. Genet. 133, 2431–2450. doi: 10.1007/s00122-020-03609-w
Xie, G., Li, Z., Ran, Q., Wang, H., Zhang, J. (2018). Over-expression of mutated ZmDA1 or ZmDAR1 gene improves maize kernel yield by enhancing starch synthesis. Plant Biotechnol. J. 16, 234–244. doi: 10.1111/pbi.1276
Xin, F., Zhu, T., Wei, S., Han, Y., Zhao, Y., Zhang, D., et al. (2020). QTL mapping of kernel traits and validation of a major QTL for kernel length-width ratio using SNP and bulked segregant analysis in wheat. Sci. Rep. 10, 25. doi: 10.1038/s41598-019-56979-7
Xu, C., Liu, Y., Li, Y., Xu, X., Xu, C., Li, X., et al. (2015). Differential expression of GS5 regulates grain size in rice. J. Exp. Bot. 66, 2611–2623. doi: 10.1093/jxb/erv058
Xu, D., Wen, W., Fu, L., Li, F., Li, J., Xie, L., et al. (2019). Genetic dissection of a major QTL for kernel weight spanning the Rht-B1 locus in bread wheat. Theor. Appl. Genet. 132, 3191–3200. doi: 10.1007/s00122-019-03418-w
Xu, R., Yu, H., Wang, J., Duan, P., Zhang, B., Li, J., et al. (2018). A mitogen-activated protein kinase phosphatase influences grain size and weight in rice. Plant J. 95, 937–946. doi: 10.1111/tpj.13971
Xu, Y., Wang, R., Tong, Y., Zhao, H., Xie, Q., Liu, D., et al. (2014). Mapping QTLs for yield and nitrogen-related traits in wheat: influence of nitrogen and phosphorus fertilization on QTL expression. Theor. Appl. Genet. 127, 59–72. doi: 10.1007/s00122-013-2201-y
Yang, G., Deng, P., Guo, Q., Shi, T., Pan, W., Cui, L., et al. (2022). Population transcriptomic analysis identifies the comprehensive lncRNAs landscape of spike in wheat (Triticum aestivum l.). BMC Plant Biol. 22, 450. doi: 10.1186/s12870-022-03828-x
Yang, L., Zhao, D., Meng, Z., Xu, K., Yan, J., Xia, X., et al. (2020). QTL mapping for grain yield-related traits in bread wheat via SNP-based selective genotyping. Theor. Appl. Genet. 133, 857–872. doi: 10.1007/s00122-019-03511-0
Yu, M., Mao, S. L., Hou, D. B., Chen, G. Y., Pu, Z. E., Li, W., et al. (2018). Analysis of contributors to grain yield in wheat at the individual quantitative trait locus level. Plant Breed. 137, 35–49. doi: 10.1111/pbr.12555
Yu, T., Han, R., Fang, Z., Mu, Z., Zheng, H., Liu, J. (2021). TransRef enables accurate transcriptome assembly by redefining accurate neo-splicing graphs. Briefings Bioinf. 22, bbab261. doi: 10.1093/bib/bbab261
Yuan, Y., Gao, M., Zhang, M., Zheng, H., Zhou, X., Guo, Y., et al. (2017). QTL mapping for phosphorus efficiency and morphological traits at seedling and maturity stages in wheat. Front. Plant Sci. 8. doi: 10.3389/fpls.2017.00614
Zeng, Z., Wang, C., Wang, Z., Han, Z., Wang, L., Lan, C. (2020). Genetic analysis and gene detection of fructan content using DArT molecular markers in spring bread wheat (Triticum aestivum l.) grain. Mol. Breed. 40, 23. doi: 10.1007/s11032-020-1102-4
Zhai, H., Feng, Z., Du, X., Song, Y., Liu, X., Qi, Z., et al. (2018). A novel allele of TaGW2-A1 is located in a finely mapped QTL that increases grain weight but decreases grain number in wheat (Triticum aestivum l.). Theor. Appl. Genet. 131, 539–553. doi: 10.1007/s00122-017-3017-y
Zhang, P., He, Z., Tian, X., Gao, F., Xu, D., Liu, J., et al. (2017). Cloning of TaTPP-6AL1 associated with grain weight in bread wheat and development of functional marker. Mol. Breed. 37, 78. doi: 10.1007/s11032-017-0676-y
Zhang, Y., Li, T., Geng, Y., Wang, Y., Liu, Y., Li, H., et al. (2021). Identification and development of a KASP functional marker of TaTAP46-5A associated with kernel weight in wheat (Triticum aestivum). Plant Breed. 140, 585–594. doi: 10.1111/pbr.12922
Zhang, Y., Liu, J., Xia, X., He, Z. (2014). TaGS-D1, an ortholog of rice OsGS3, is associated with grain weight and grain length in common wheat. Mol. Breed. 34, 1097–1107. doi: 10.1007/s11032-014-0102-7
Zhao, D., Yang, L., Liu, D., Zeng, J., Cao, S., Xia, X., et al. (2021). Fine mapping and validation of a major QTL for grain weight on chromosome 5B in bread wheat. Theor. Appl. Genet. 134, 3731–3741. doi: 10.1007/s00122-021-03925-9
Zhou, J., Li, C., You, J., Tang, H., Mu, Y., Jiang, Q., et al. (2021). Genetic identification and characterization of chromosomal regions for kernel length and width increase from tetraploid wheat. BMC Genomics 22, 706. doi: 10.1186/s12864-021-08024-z
Zhou, S., Xue, H. (2020). The rice PLATZ protein SHORT GRAIN6 determines grain size by regulating spikelet hull cell divisionFA. J. Integr. Plant Biol. 62, 847–864. doi: 10.1111/jipb.12851
Zhou, Y., Tao, Y., Zhu, J., Miao, J., Liu, J., Liu, Y., et al. (2017). GNS4, a novel allele of DWARF11, regulates grain number and grain size in a high-yield rice variety. Rice 10, 34. doi: 10.1186/s12284-017-0171-4
Keywords: QTL mapping, kernel-related traits, putative candidate gene, KASP markers, Triticum aestivum L.
Citation: Zeng Z, Zhao D, Wang C, Yan X, Song J, Chen P, Lan C and Singh RP (2023) QTL cluster analysis and marker development for kernel traits based on DArT markers in spring bread wheat (Triticum aestivum L.). Front. Plant Sci. 14:1072233. doi: 10.3389/fpls.2023.1072233
Received: 17 October 2022; Accepted: 23 January 2023;
Published: 10 February 2023.
Edited by:
Hongwei Wang, Shandong Agricultural University, ChinaReviewed by:
Ana Pontaroli, BioLumic Limited, New ZealandCopyright © 2023 Zeng, Zhao, Wang, Yan, Song, Chen, Lan and Singh. This is an open-access article distributed under the terms of the Creative Commons Attribution License (CC BY). The use, distribution or reproduction in other forums is permitted, provided the original author(s) and the copyright owner(s) are credited and that the original publication in this journal is cited, in accordance with accepted academic practice. No use, distribution or reproduction is permitted which does not comply with these terms.
*Correspondence: Chunping Wang, Y2h1bnBpbmd3QGhhdXN0LmVkdS5jbg==
Disclaimer: All claims expressed in this article are solely those of the authors and do not necessarily represent those of their affiliated organizations, or those of the publisher, the editors and the reviewers. Any product that may be evaluated in this article or claim that may be made by its manufacturer is not guaranteed or endorsed by the publisher.
Research integrity at Frontiers
Learn more about the work of our research integrity team to safeguard the quality of each article we publish.