- 1School of Biological Sciences, The University of Western Australia, Crawley, WA, Australia
- 2School of BioSciences, University of Melbourne, Parkville, VIC, Australia
Utilising resistance (R) genes, such as LepR1, against Leptosphaeria maculans, the causal agent of blackleg in canola (Brassica napus), could help manage the disease in the field and increase crop yield. Here we present a genome wide association study (GWAS) in B. napus to identify LepR1 candidate genes. Disease phenotyping of 104 B. napus genotypes revealed 30 resistant and 74 susceptible lines. Whole genome re-sequencing of these cultivars yielded over 3 million high quality single nucleotide polymorphisms (SNPs). GWAS in mixed linear model (MLM) revealed a total of 2,166 significant SNPs associated with LepR1 resistance. Of these SNPs, 2108 (97%) were found on chromosome A02 of B. napus cv. Darmor bzh v9 with a delineated LepR1_mlm1 QTL at 15.11-26.08 Mb. In LepR1_mlm1, there are 30 resistance gene analogs (RGAs) (13 nucleotide-binding site-leucine rich repeats (NLRs), 12 receptor-like kinases (RLKs), and 5 transmembrane-coiled-coil (TM-CCs)). Sequence analysis of alleles in resistant and susceptible lines was undertaken to identify candidate genes. This research provides insights into blackleg resistance in B. napus and assists identification of the functional LepR1 blackleg resistance gene.
Introduction
Brassica napus L. (canola, oilseed rape) is one of the most important crops for edible oil, food and industrial purposes (Şensöz et al., 2000; Abbadi and Leckband, 2011). The breeding of canola cultivars has focused on oil quality, seed yield and resistance to pests and diseases (Potter et al., 2016; Salisbury et al., 2016). One of the major diseases affecting canola production is blackleg, caused by the fungal pathogen Leptosphaeria maculans. Blackleg can reduce seed yield by at least 50% (Gugel and Petrie, 1992; West et al., 2001; Van de Wouw et al., 2010; Potter et al., 2016; Salisbury et al., 2016) and also affect oil quality (Fikere et al., 2020a). Blackleg disease is difficult to manage, even with fungicide application and recently there has been identification of fungicide tolerant L. maculans isolates (Kutcher et al., 2010; Van de Wouw et al., 2017; Van de Wouw et al., 2021). Thus, utilising resistant cultivars is recognised as an effective and environmentally safe approach in managing L. maculans and minimising yield loss.
There are two types of resistance against L. maculans in B. napus; qualitative and quantitative resistance (Delourme et al., 2006). Qualitative resistance involves an interaction between a resistance (R) gene and an avirulence (Avr) gene (Flor, 1971). These R genes, also termed major genes, are highly heritable and manifest effectively from the cotyledon stage through to the adult stage (Raman et al., 2012; Raman et al., 2012). On the other hand, quantitative resistance, considered to be governed by multiple minor genes, provides partial resistance to individual isolates and is poorly understood both phenotypically and genotypically (Huang et al., 2009). Both types of blackleg resistance are important in canola and many commercial cultivars have both qualitative and quantitative resistance.
To determine the position of an R gene in the genome, genetic mapping of genotypic variants is frequently undertaken. Two of the most common approaches for genetic mapping are association mapping, or genome wide association studies (GWAS), and biparental linkage mapping. GWAS is an alternative approach to traditional mapping of biparental populations and has been widely used in B. napus (Raman et al., 2014; Zhang et al., 2015; Gacek et al., 2016; Raman et al., 2016; Wu et al., 2016; Fu et al., 2020; Hu et al., 2022). The detection of quantitative trait loci (QTL) through GWAS depends on the level of linkage disequilibrium (LD) between functional loci and markers, which most GWAS models can statistically and easily determine. Generalized linear models (GLM) and mixed linear models (MLM) are the most common GWAS models used in plant studies, as these models are flexible and can detect multiple genotypic variants. Of the two models, MLM simultaneously incorporates kinship and principal component analyses (PCA) to address cryptic relatedness in tested materials (Yu et al., 2006).
Currently 16 qualitative blackleg R genes have been mapped in Brassica species, however only LepR3, Rlm2, Rlm4, Rlm7 and Rlm9 have been cloned to date (Larkan et al., 2013; Larkan et al., 2015; Larkan et al., 2020; Haddadi et al., 2022). LepR3 and Rlm2 are alleles of the same gene, a resistance gene analog (RGA) encoding a receptor-like protein (RLP), while Rlm4, Rlm7 and Rlm9 are also allelic variants of an RGA encoding wall-associated kinases (WAKs) (Larkan et al., 2020; Haddadi et al., 2022). Other blackleg RGAs have also been cloned in Arabidopsis thaliana; RLM1a, RLM1b and RLM3 which encode nucleotide-binding site (NBS)-leucine rich repeats (LRR) (Staal et al., 2006; Staal et al., 2008). One of the blackleg R genes yet to be cloned is LepR1, which was originally introgressed from Brassica rapa L. subsp. sylvestris into B. napus and has been mapped on chromosome A02 of B. napus (Yu et al., 2005; Larkan et al., 2016; Dolatabadian et al., 2019; Fikere et al., 2020b). This study used whole genome re-sequencing (WGRS) data from 104 Australian B. napus genotypes, screened with blackleg isolates containing AvrLep1 to identify a precise location of the QTL harbouring LepR1 and to identify candidate genes within this region. Lastly, sequence analysis was used to identify candidate LepR1 genes in B. napus.
Materials and methods
Blackleg isolates and disease phenotyping
A set of 14 well-characterised differential L. maculans isolates were used to genotype B. napus genotypes for the presence or absence of resistance gene LepR1. All isolates and their genotypes (Table S1) were maintained on 10% V8 media. Pycnidiospores (10-5) of each L. maculans isolate were dropped onto cotyledons of wounded ten-day-old seedlings grown in the glasshouse at 22°C. Disease symptoms were scored at 14 days post-inoculation on a 0-9 scale, as previously described (Marcroft et al., 2012). For each isolate x cultivar interaction, eight replicate plants were inoculated, each with four wounds per plant. Average pathogenicity scores of <5.0 were considered avirulent, whilst average scores of >5.0 were considered virulent.
DNA extraction and whole genome re-sequencing
A total of 104 Australian B. napus genotypes (89 commercial cultivars and 15 breeding lines) were used in this study (Table S2). The genotypes were grown in a glasshouse at 18°C during the day and 13°C at night in a 12-hour photoperiod cycle for two weeks. Approximately 100 mg of young, healthy leaf material was collected, snap-frozen in liquid nitrogen and finely ground using a Geno-Grinder 2010 (SPEX SamplePrep, Mettuchen, Germany). DNA was extracted using the DNeasy Plant Kit (Qiagen©, Hilden, Germany) according to the manufacturer’s instructions. Total DNA was quantified using a Qubit 3.0 Fluorometer and the Qubit dsDNA BR Assay kit (Invitrogen, Carlsbad, United States), while DNA quality was assessed using the LabChip GX Touch 24 (PerkinElmer, Waltham, United States). WGRS of the cultivars was carried out using an Illumina NovaSeq™ 6000 Sequencing System by GeneWiz (Brookes Life Sciences, Guangzhou, China), which generated approximately ~6 Gb of 150 bp paired-end sequencing data per cultivar.
SNP calling and filtering
From the WGRS data, raw FASTQ files were trimmed of adapter sequences and low quality bases using Trimmomatic v0.39 (Bolger et al., 2014) with a maximum mismatch score of 2, a palindrome clip score threshold of 30 and a clip score threshold of 10. Low quality bases with a Phred+ 33 score of < 3 were trimmed from both the start and end of each read. Sliding window trimming was carried out using a 4-base wide window to remove bases with an average quality per base of <15. All the reads with fewer than 36 bases, as well as unpaired reads paired after trimming, were discarded. To verify adapter and read quality trimming, untrimmed and trimmed reads were analysed using FastQC (Andrews, 2010) followed by MultiQC (Ewels et al., 2016), which were compared afterwards.
Trimmed paired reads were then aligned to B. napus genome cv. Darmor bzh v9 (Bayer et al., 2020), using Bowtie2 v2.4.1 (Langmead and Salzberg, 2012) with settings end-to-end, sensitive,-I 0 and -X 1000. Alignments were subsequently converted to bam format and sorted using samtools v.1.10 (Li et al., 2009; https://github.com/samtools/bcftools). Duplicate reads were removed using samtools markdup. SNPs for each individual were called using bcftools v.1.10 (Li et al., 2009) functions mpileup (-q 10 and –Q 20). The vcf files were indexed using tabix (Li, 2011) while the SNPs were merged per chromosome using bcftools.
Merged variants were further filtered using VCFtools v0.1.16 (Danecek et al., 2011). Indels and multiallelic SNPs were omitted (–remove-indels –max-alleles 2 –min-alleles 2). Individuals with > 0.9 missing genotypes were removed before the filtering of SNPs. Genotypes with a depth of < 5 (–minDP 5) were set to missing to minimise the rate of heterozygous alleles incorrectly called as homozygous alleles due to insufficient read depth. SNPs displaying a minor allele frequency (MAF) of < 0.05 (–MAF 0.05) or when genotypes were not present in > 80% of all individuals (–max-missing 0.8) were also discarded. The filtered SNP data was then used as genotype data for GWAS.
Association analysis
The GWAS results, along with quantile-quantile (QQ) plot in mixed linear model (MLM) with PCA (3 principal components) + Kinship matrix for family relatedness estimates (K) (Yu et al., 2006), were computed using the GAPIT3 R package (Wang and Zhang, 2021). An additional GWAS model; Fixed and random model Circulating Probability Unification (FarmCPU) (Liu et al., 2016), computed through GAPIT3 R package was used to determine consistent significant SNPs across two models. From the GWAS result, CMplot R package (Yin et al., 2021) was used to visualise circular Manhattan and SNP density plots. MAF and adjusted P-value following a false discovery rate (FDR)-controlling procedure (Benjamini and Hochberg, 1995), additional criteria to select SNPs associated to the trait, were also identified using GAPIT3. GAPIT was also used to show the heterozygosity information and LD, represented by R2 which measures two biallelic markers (Hill and Robertson, 1968). The phenotypic variation explained by a SNP marker (PVE) was taken using the following formula: PVE = [(R2 with SNP model value- R2 without SNP model value) x 100] (Sun et al., 2010). Figure plotting of the SNP statistics was also done in GAPIT3 while the other SNP statistics were computed in TASSEL 5.0 (Bradbury et al., 2007).
Current and previous QTL dissection
Putative QTL were derived from the significant SNPs identified through MLM GWAS. A QTL was defined either as a 100 kb upstream and downstream region of each significant SNP (Anderson et al., 2020) or a region with two significant SNPs located ≤ 15 Mb from each other on the same chromosome (Martínez-Montes et al., 2018), also integrating a 100 kb region upstream and downstream from the SNP. For QTL comparison, the previous QTL (chromosome A02) based on LepR1 introgressed lines and structural variants, derived from the results of L. maculans containing AvrLep1 screened at the cotyledon stage (Larkan et al., 2016; Dolatabadian et al., 2019), and based on adult plant survival rate and average internal infection of the stem at maturity stage (Fikere et al., 2020b) (considered as the QTL outlier) were physically mapped to B. napus cv Darmor bzh v9 using MapChart 2.32 for comparison (Voorrips, 2002). Then, the number of genes and RGAs in the derived QTL intervals were identified for comparison.
The RGAugury pipeline (Li et al., 2016) was used to predict RGAs, along with the classes, for example NLR, RLK, RLP, and TM-CC (Transmembrane (TM)- Coiled-Coil (CC)) and subclasses including NBS, CNL (CC-NBS-LRR), CN (CC-NBS), NL (NBS-LRR), TNL (Toll/Interleukin-1 Receptor (TIR)-NBS-LRR), TN (TIR-NBS), TX (TIR with unknown domains), in Darmor bzh v9 (Bayer et al., 2020), then the RGAs within each QTL region were classified. The SNPs of the LepR1 candidate genes (current QTL in A02) were visualised in Geneious R10 (Kearse et al., 2012) and identified as either within a non-coding or coding (CDS) region, non-synonymous or synonymous if it was within the coding region, and non-sense or missense variant if it was a non-synonymous SNP.
Molecular analysis
The LepR1 candidates which had at least 2 SNPs in CDS regions were chosen as the most likely candidates. We also included two flanking RGAs (for comparison) nearest to the LepR1 QTL identified in this study. The marker development was done in two stages. First, markers flanking each candidate were designed using the Primer3 function in Geneious R10 (Kearse et al., 2012) and the markers were tested for allele detection of the resistant and susceptible materials. Then, the amplicons showing segregation between resistant and susceptible lines were purified for sequencing. MiSeq sequencing was done using an Illumina MiSeq platform at the Australian Genome Research Facility (AGRF), Perth, Australia. MiSeq reads were quality-trimmed using Trimmomatic (Bolger et al., 2014) and assembled using Spades version 3.6.0 (Bankevich et al., 2012). The markers showing sequence variation between resistant and susceptible materials were used to design a resistant-specific marker.
Results
Phenotyping
Out of 104 B. napus cultivars, 30 showed a resistance response to L. maculans isolates containing AvrLep1, indicating the presence of LepR1, while 74 showed a susceptible response, indicating they do not harbour LepR1 (Table S2).
WGRS SNP analysis
The WGRS data of 104 B. napus genotypes produced a total of 3,235,008 high quality SNPs that were used in the GWAS (Figure S1). The average number of heterozygous SNPs was 427,970 SNPs per B. napus genotype (7.77% of the 3,235,008 high quality SNPs) (Figure S1, Table S3). LD also showed a slower decay rate (Figure S2) over the previous B. napus LD findings obtained using less dense markers (845 RFLP, 89 and 451 SSR, 8,502 and 251,575 GBS SNP markers) (Bus et al., 2011; Xiao et al., 2012; Horvath et al., 2020; Rahman et al., 2022).
SNPs, QTL and RGAs associated with LepR1 resistance
In MLM GWAS integrated with PCA + Kinship results (Figures S3-4), a total of 2,166 significant SNPs associated with LepR1 resistance were identified across 10 chromosomes (2,108 SNPs on A02, 26 SNPs on C02, 7 SNPs each on A05 and C06, 6 SNPs on A08, 5 SNPs on C08, 3 SNPs on A07, 2 SNPs each on A04 and C01, and a SNP in C07) at the 1.58 E-08 cut-off value, implemented with Bonferroni correction and FDR (Figures 1–2; Tables S4). Significant SNPs had a range of 11.7 – 23.14% PVE (Table S4). The additional FarmCPU GWAS detected 7 significant SNPs but only one SNP (RaGOO_A02_103419) was found significant in the two different GWAS models (Table S5, Figure S5).
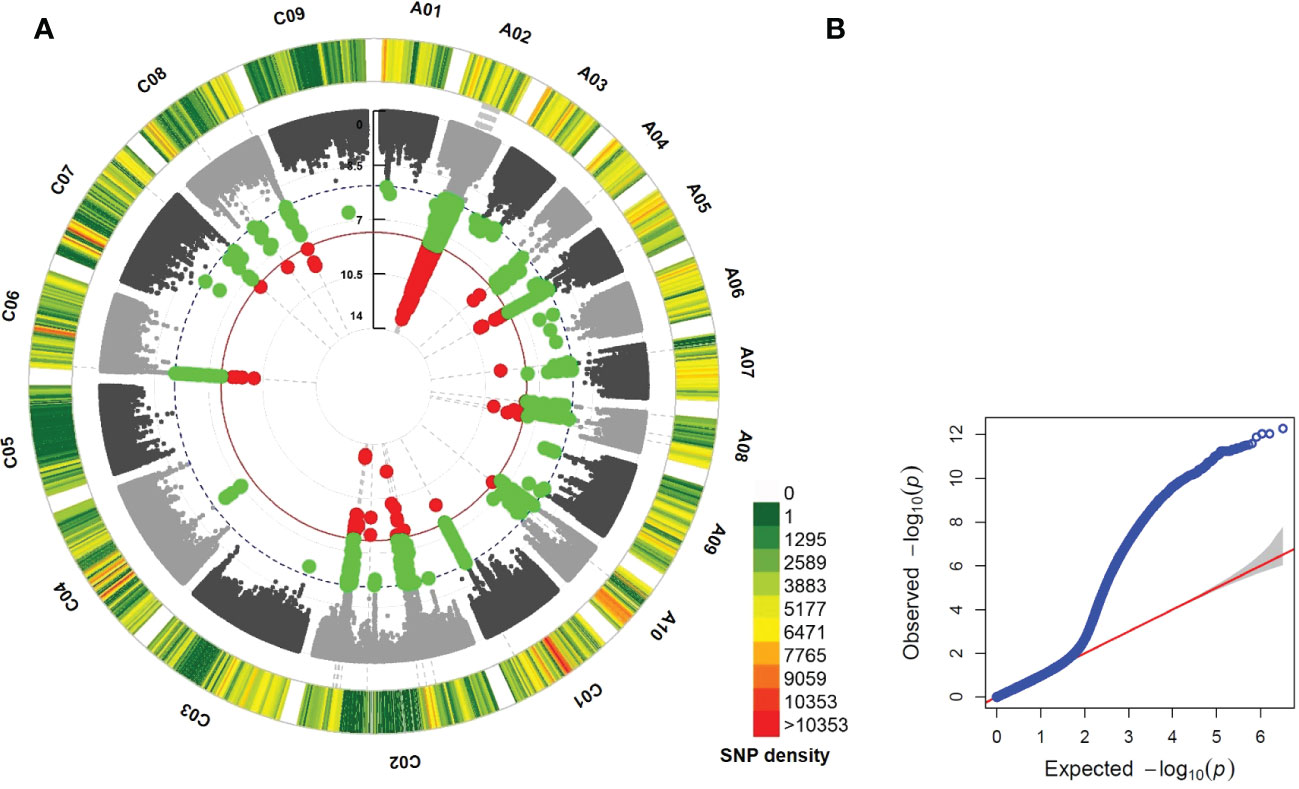
Figure 1 Circular Manhattan (A) and quantile-quantile (QQ) plots (B) showing the significant single nucleotide polymorphisms (SNPs) above the significance threshold line (red dot), 1.58 E-08, in mixed linear model (MLM) of genome wide association study in Brassica napus cv. Darmor bzh v9. Green dot refers to SNPs below the cut-off for significance having at least E-05.
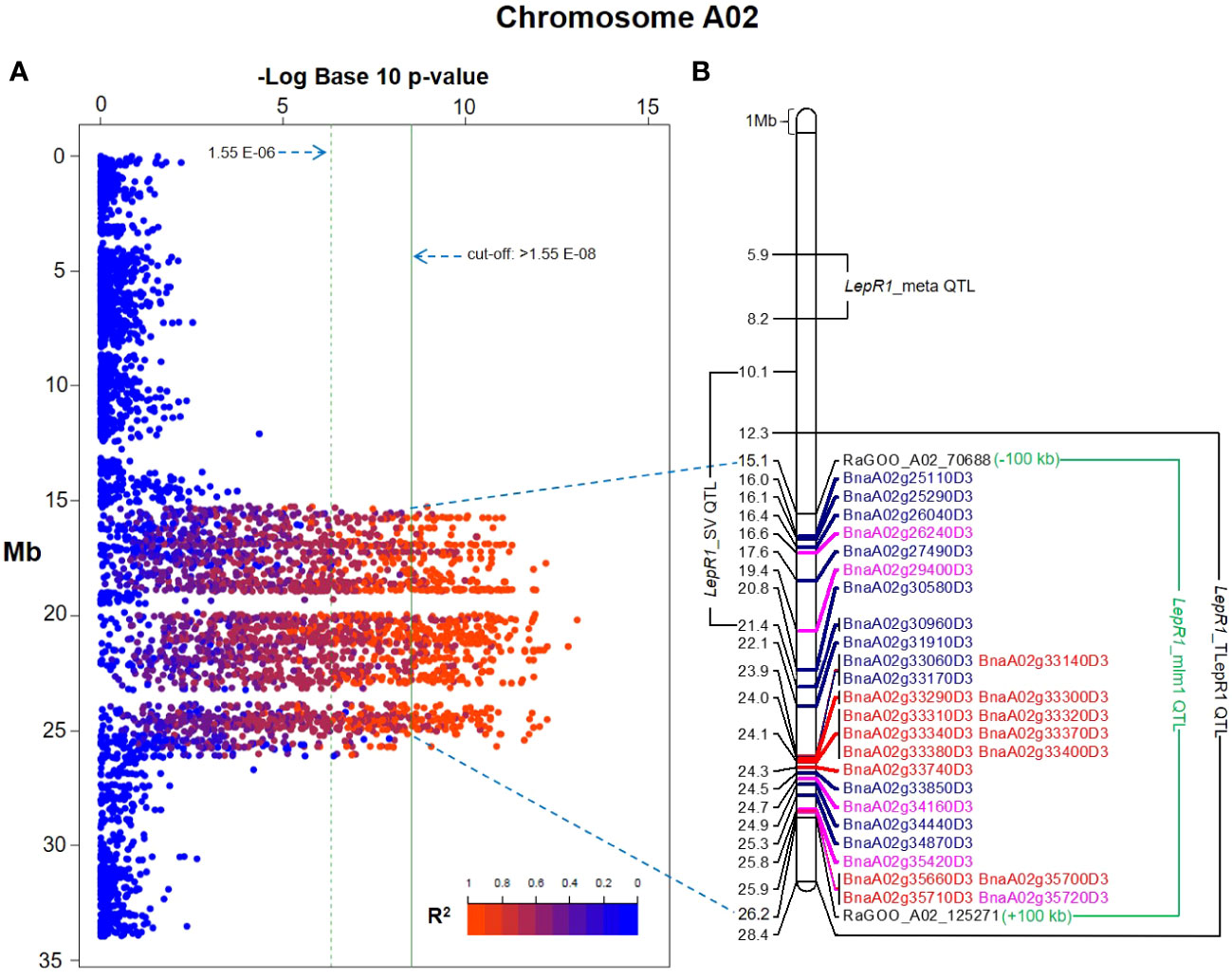
Figure 2 Mixed linear model (MLM)- genome-wide association study (GWAS) Manhattan plot in chromosome A02 of Brassica napus cv. Darmor bzh v9 showing SNPs and their linkage disequilibrium estimates in R2 (A). Physical map of the flanking significant SNPs, RaGOO_A02_70688 and _125271, delineating the current quantitative trait loci (QTL) along its resistance gene analogs (RGAs) (red font=nucleotide binding site leucine rich repeats, blue font=receptor-like protein kinase, pink font=transmembrane-coiled coil) and previous QTL associated to LepR1 blackleg resistance in Brassica napus (B).
From the significant SNPs in MLM GWAS, we derived 15 QTL (containing 3,055 genes in total), however only 9 of them contained RGAs (Table 1, S6) and were considered further. These 9 QTL were located on chromosomes A02, C01 and C02, harbouring 62 RGAs in total (28 NLRs (12 TXs, 9 TNLs, 5 NLs, 4 NBS, 2 CNLs and 2 CN), 24 RLKs, 14 TM-CCs, and 3 RLPs) (Tables 1, 2; S7)
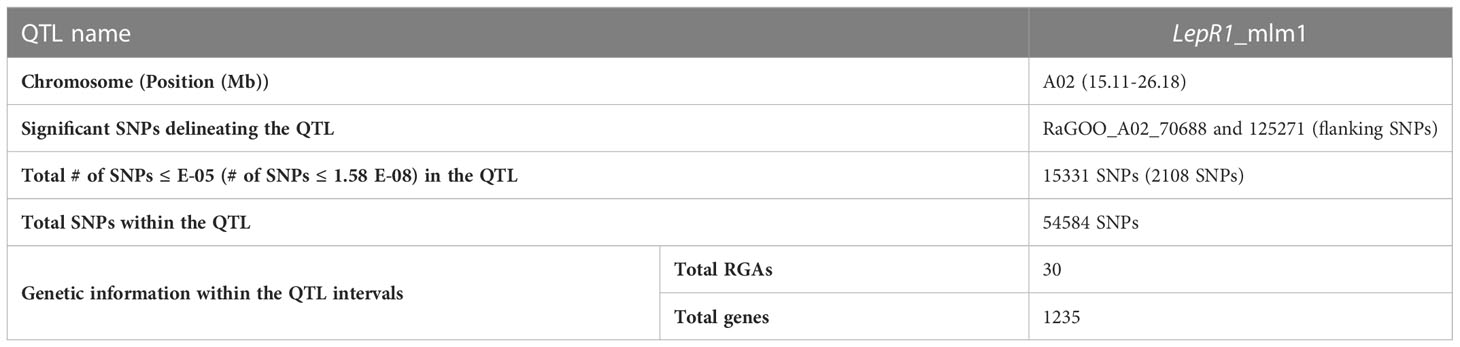
Table 1 Delineation of the LepR1_mlm1 quantitative trait loci (QTL) including number of genes and the resistance gene analogs (RGAs) content using the mixed linear model (MLM) genome-wide association study (GWAS) and its significant single nucleotide polymorphism (SNP) associated with LepR1 blackleg resistance in Brassica napus.
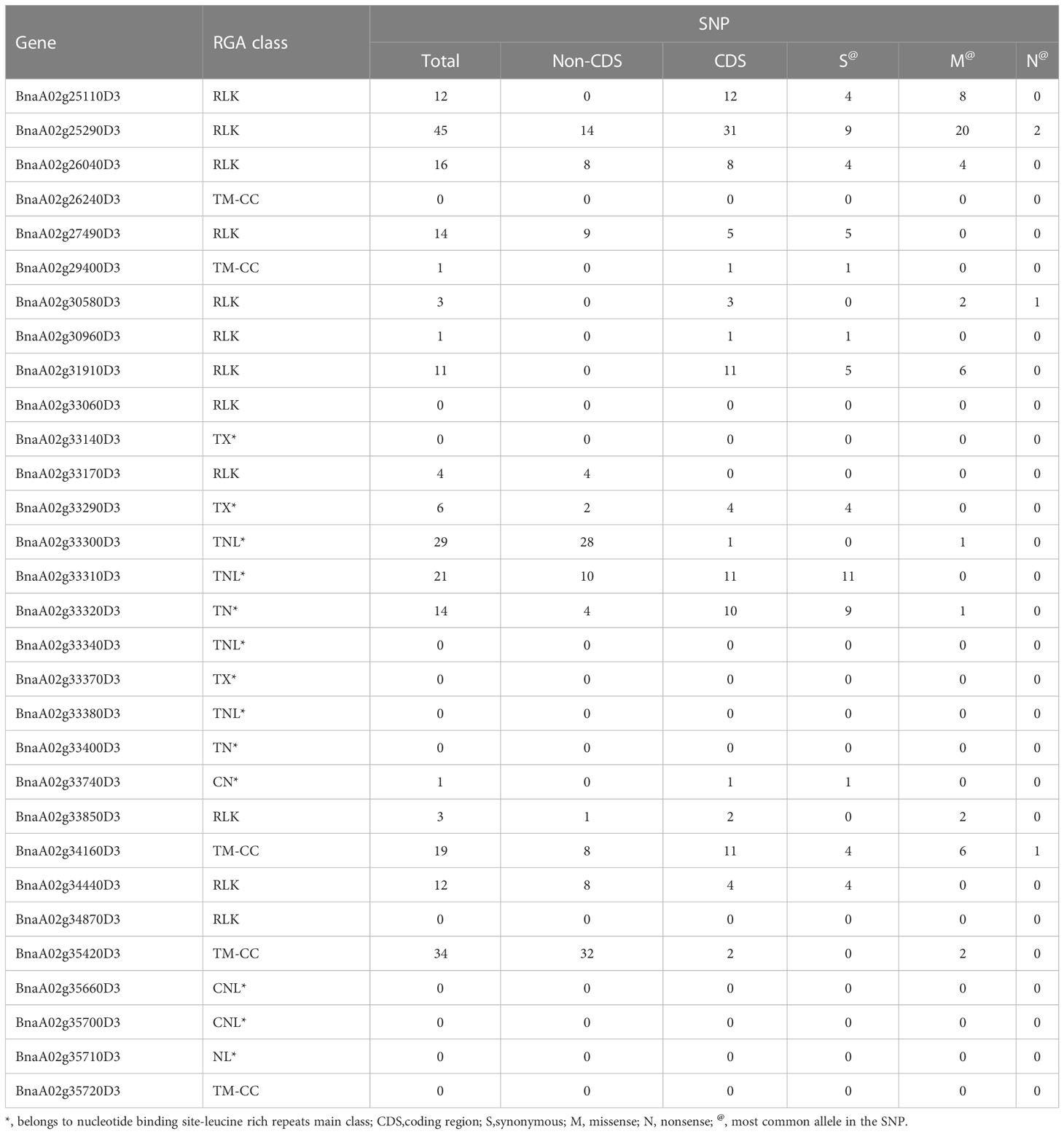
Table 2 List of resistance gene analogs (RGA) and their single nucleotide polymorphism (SNP) information within LepR1_mlm1 on chromosome A02 of Brassica napus cv. Darmor bzh v9 identified using mixed linear (MLM) genome wide association study (GWAS).
A02 as the chromosome for LepR1 in B. napus
This study showed 97% (2,108 out of 2,167 SNPs) of the total significant SNPs associated with LepR1 blackleg resistance were found in chromosome A02 in B. napus cv. Darmor bzh v9 (Figure 1; Table S3). The delineated QTL on A02 (LepR1_mlm1) was 11.07 Mb in length (15.11-26.18 Mb) (Figure 2). Analysis of LD revealed closely associated SNPs within the QTL interval with a R2 ranging between 0.802-0.923 (Figure 2; Table S4).
The QTL in other studies were based on the LepR1 introgression line (cv. Topas), mapped in genome cv. Darmor bzh v4.1 (Larkan et al., 2016), the structural variants of LepR1 candidates mapped in cv. Darmor bzh v8.1 (Dolatabadian et al., 2019), and meta-analysis of blackleg resistance mapped in cv. Darmor bzh v4.1 (Fikere et al., 2020b), which were named in this study as LepR1_TLepR1, LepR1_SV, and LepR1_meta, respectively (Figure 2; Table 3). In Darmor bzh v9, LepR1_TLepR1, LepR1_SV, and LepR1_meta were found at 12.27-28.44 Mb totalling 16.17 Mb size, 10.09-21.4 Mb totalling 11.31 Mb size, and 5.91-8.22 Mb totalling 2.31 Mb size in A02, respectively (Figure 2). It can be noted that LepR1_mlm1 is shorter compared to the sizes of the LepR1_TLepR1 and LepR1_SV, but larger than the QTL outlier, LepR1_meta. Between the positions of these QTL, LepR1_mlm1 was nearer to LepR1_TLepR1 and LepR1_SV than LepR1_meta (Figure 2). LepR1_mlm1 contained 1235 genes (30 RGAs), LepR1_TLepR1, LepR1_SV, and LepR1_meta contained 1915 genes (46 RGAs), 344 genes (8 RGAs), and 1434 genes (20 RGAs), respectively (Tables 1, 3). Of the previous QTL, only LepR1_TLepR1 and LepR1_SV had an overlapping or co-localised position with LepR1_mlm1 (Figure 2). Eight out of 30 RGAs in LepR1_mlm1 (RLKs BnaA02g25110D3, BnaA02g25290D3, BnaA02g26040D3, BnaA02g27490D3, BnaA02g30580D3, and BnaA02g30960D3, TM-CCs BnaA02g26240D3 and BnaA02g29400D3) were also within the intervals of the LepR1_TLepR1 and LepR1_SV (Figure 2).
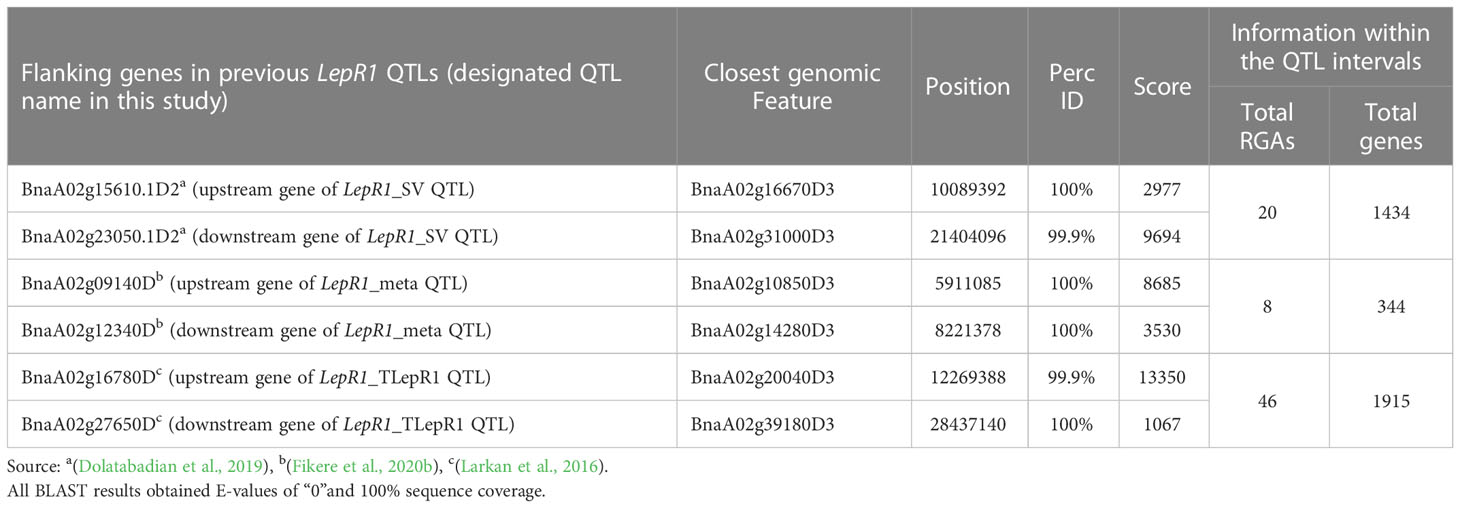
Table 3 Alignment (basic local alignment sequence tool or BLAST) of the flanking genes from previous LepR1 quantitative trait loci (QTL) in chromosome A02 of Brassica napus to Darmor bzh v9.
Eighteen (60%) out of the 30 RGAs underlying LepR1_mlm1 contained SNPs (246 SNPs in total), with 17 having SNPs in the CDS region (CDS-SNPs) (118 CDS-SNPs in total) (Table 2). BnaA02g25290D3 (RLK) contained the most CDS-SNPs, 31. The next highest number of CDS-SNPs in a LepR1 candidate gene were 12 in BnaA02g25110D3 (RLK), 11 in BnaA02g31910D3 (RLK), BnaA02g33310D3 (RLK) and BnaA02g34160D3 (TM-CC), 10 in BnaA02g33320D3 (NLR) and 8 in BnaA02g26040D3 (RLK) (Table 2). Among the candidate genes, only BnaA02g25290D3, BnaA02g30580D3 (RLK), and BnaA02g34160D3 contained nonsense SNPs. For instance, BnaA02g25290D3 had the “T” allele of RaGOO_A02_75077 (16,131,870 bp in A02) and RaGOO_A02_75078 (16,131,906 bp in A02) was identified as a STOP CODON, “TGA” (Table S7). The positions of RaGOO_A02_75077 and RaGOO_A02_75078 correspond to the 16,131,870-16,131,872 bp and 16,131,906-16,906,908 bp with a three-nucleotide “AGA” (Arginine) in reference to the Darmor bzh v9 (Table S8). BnaA02g25290D3 also contained the highest number of possible missense SNP alleles, 20, followed by 8 in BnaA02g25110D3 and 6 to both BnaA02g31910D3 and BnaA02g34160D3 (Table 2).
Molecular analysis in LepR1 resistant and susceptible materials
Only 13 LepR1 candidates (BnaA02g25110D3, BnaA02g25290D3, BnaA02g26040D3, BnaA02g27490D3, BnaA02g30580D3, BnaA02g31910D3, BnaA02g33290D3, BnaA02g33310D3, BnaA02g33320D3, BnaA02g34160D3, BnaA02g34440D3, BnaA02g33850D3, and BnaA02g35420D3) had at least 4 SNPs in their CDS regions between resistant and susceptible lines (Table 2). Among the 13 selected LepR1 candidates and the 2 flanking RGAs, only BnaA02g33310D3, BnaA02g33850D3, BnaA02g34440D3, and the flanking RGA BnaA02g25030D3 were reproducibly amplified (Table S9), which were sent for sequencing analysis.
BnaA02g33310D3 and BnaA02g25030D3 had a sequence difference between the resistant and susceptible alleles, while BnaA02g33850D3 and BnaA02g34440D3 had similar sequences throughout the tested materials. SNP differences among the alleles of the resistant cultivars was also observed in BnaA02g25030D3. In all, BnaA02g33310D3 had 92 SNP differences between the resistant and susceptible B. napus cultivars (Figure S5). There were also two large deletions in positions 697 to 706 bp and 2836 to 2854 bp observed in the resistant allele, while there was one large deletion in position 2881 and 2894 bp in the susceptible allele (Figure S5). For the resistant-specific marker developed based on the sequencing result (Table S10), 22 out of 25 LepR1 resistant cultivars had a band while no band was detected in the 31 susceptible cultivars (Figure S6).
Discussion
Leptosphaeria maculans isolates containing specific Avr gene profiles are widely used either to verify known blackleg R genes or discover novel blackleg R genes in B. napus (Balesdent et al., 2005; Van de Wouw et al., 2009; Marcroft et al., 2012; Jiquel et al., 2021). However, a mild or inconsistent hypersensitive response in B. napus cultivars can be observed due to different genomic backgrounds (Rouxel et al., 2003; Balesdent et al., 2005; Larkan et al., 2016). Nevertheless, LepR1 is a single dominant gene and its interaction to AvrLep1 interaction in B. napus follows a gene-for-gene relationship (Yu et al., 2002; Yu et al., 2005). Thus, the phenotype data from L. maculans isolates containing AvrLep1 confirmed the presence of the R gene in the resistant cultivars and the absence of the R gene in the susceptible cultivars in this study.
The use of WGRS in crop studies is advantageous because it can identify a large number of SNPs that are densely dispersed throughout the entire genome, therefore improving the ability for GWAS to identify functional QTL. The genotype data used here comprised over 3 million SNP markers, which has a better coverage and was more dense compared to low coverage markers such as RFLPs, SSRs, the Brassica Infinium 60K SNP array, and imputed SNPs, that were used to detect previous LepR1 QTL in B. napus (Yu et al., 2005; Yu et al., 2012; Larkan et al., 2016; Fikere et al., 2020b). In previous studies which had defined positions of the LepR1 QTL, they used previous versions (with different quality and assembly size) of Darmor bzh genomes (v4.1 or v8.1). For instance, Darmor bzh v9 has a total size of 1,043.4 Mb compared to the 850.3 Mb of Darmor bzh v4.1 (Bayer et al., 2020).
GWAS is commonly used to reveal genetic mechanisms underlying disease resistance as it integrates historical recombination of the different cultivars and thus, uncovers significant associations between genotype and phenotype. Among the GWAS models, MLM is widely regarded as the most common model in analysing marker trait associations in crop research. Kinship and PCA results were integrated into the MLM to overcome any bias from genetic ancestry (relatedness) (Price et al., 2006; Yu et al., 2006; VanRaden, 2008).
LepR1_meta was based on adult plant survival rate and average internal infection of the stem at maturity stage paired with imputed SNPs, a methodology that would identify quantitative instead of qualitative blackleg resistance in B. napus (Raman et al., 2018; Fikere et al., 2020b). This is likely the reason why LepR1_meta was located 6.89 Mb away from LepR1_mlm1 of this study. Our LepR1_mlm1 corresponds to qualitative blackleg resistance. Similarly, LepR1_TLepR1 was a result from L. maculans containing AvrLep1 screened at the cotyledon stage of the LepR1 introgressed lines (Larkan et al., 2016). LepR1_TLepR1 was also used as the basis to define structural variants of LepR1 in LepR1_SV (Dolatabadian et al., 2019). Taken together, our QTL was consistent with previous LepR1 QTL based on gene-for-gene screening which were all mapped to A02 (Larkan et al., 2016; Dolatabadian et al., 2019; Fikere et al., 2020b).
The few significant SNP associations on C01 and C02 (having RGAs in the QTL) could possibly be due the incorrect mapping of DNA sequences containing notable SNPs that occurred during the genome assembly process in B. napus as SNPs were commonly observed in a genome due to their abundance. Another reason is due to homology between chromosomes, as C02 is homeologous to A02 in B. napus (Parkin et al., 2005). Another reason could be that some genes might have a role (for example as a helper or decoy gene) in the gene-for-gene interaction between LepR1 and AvrLep1. RNA sequencing in previous studies has revealed several significantly activated genes in the LepR1-AvrLep1 interaction on chromosomes C01 and C02 (Becker et al., 2017; Haddadi et al., 2019), indicating the possible roles of other genes in the LepR1-AvrLep1 interaction in B. napus. Recent findings in the B. napus-L. maculans pathosystem indicate that the simple gene-for-gene interaction may indeed be more complicated than once thought (Cantila et al., 2021). Functional gene studies revealed that B. napus mitogen-activated protein (MAP) kinase 9 (BnMPK9), BnaCnng11720D or C05p48150.1_BnaDar on C05 (Rousseau-Gueutin et al., 2020), is an essential partner to the gene-for-gene mediated resistance of blackleg R gene Rlm1 on A07 and pathogen effector AvrLm1 (Ma et al., 2018). Furthermore, Suppresor of BAK1-interacting receptor like kinase 1 (SOBIR1) genes on A03 and C03 (BnaA03g14760D and BnaC03g17800D) are also required for the blackleg resistance of the alleles LepR3 and Rlm2 (BnaA10g20720D) of A10 to effectors AvrLm1 and AvrLm2, respectively (Larkan et al., 2015; Ma et al., 2018).
RGAs with CDS-SNPs are promising candidates for the LepR1 gene. The CDS-SNPs of these RGAs could alter amino acids (aa) of the predicted proteins explaining the difference between susceptible and resistant phenotypes. Among the SNP types, a nonsense SNP leads to a premature stoppage of the amino acids, potentially changing the gene expression among cultivars with different genotypes. Although this study did not examine gene expression between cultivars with different LepR1 phenotypes, previous studies have shown that RLKs and NLRs were significantly expressed in LepR1 resistant cultivars (Becker et al., 2017; Ferdous et al., 2020).
Among the candidates, only BnaA02g33310D3 showed interesting SNP variation, including large deletions, between LepR1 resistant and susceptible materials, indicating the gene may be considered further as a candidate gene. Large deleted sequences were also found in functionally characterised (mutated) genes RPM1 against Pseudomonas syringae (Grant et al., 1998) and LepR3/Rlm2 against L. maculans (Larkan et al., 2013; Larkan et al., 2015) compared to its respective wild gene. Also, SNP variation has been the basis to develop specific markers with blackleg resistance (Van de Wouw et al., 2022). This study’s resistant-specific marker for BnaA02g33310D3 also signified the gene as a candidate in B. napus and will be useful for MAS. On the other hand, the other candidates which did not have successful band detection can still be considered candidates for the LepR1 but they require further analysis.
Conclusion
This study has narrowed down the LepR1 resistance in B. napus by comparing the current QTL to the previous LepR1 QTLs. While all RGAs in LepR1_mlm1 are promising candidates, those co-localised within the intervals in previous LepR1 QTLs aligned in Darmor bzh v9 and having CDS-SNPs, especially with nonsense SNPs, should be prioritised for validation. In addition, RGAs in the QTLs identified on chromosomes other than A02 in this study can be tested for the possible roles of accessory, helper or decoy genes in LepR1 gene-for-gene resistance in B. napus. Our identified RGAs especially BnaA02g33310D3 are a useful resource to identify the functional LepR1 gene in B. napus.
LepR1 can also be an significant source of blackleg resistance for the cultivars in Australia, which has virulent L. maculans population causing the breakdown of the cultivars containing R gene like LepR3 and ineffectiveness of R genes like Rlm1 and Rlm3 (Li et al., 2005; Van de Wouw et al., 2014; Van de Wouw et al., 2021). Other studies have also shown that cultivars with LepR1 are more effective than those with R genes Rlm1, Rlm3, Rlm4, and LepR3 (Alnajar et al., 2022; Rashid et al., 2022).
Data availability statement
The datasets presented in this study can be found in online repositories. The names of the repository/repositories and accession number(s) can be found below: NCBI accession PRJNA885991.
Author contributions
AC and JB conceptualized the paper. AC wrote the original draft along with formal analyses. WT, PB, DE, and AV helped improve the paper by suggesting additional ideas and by thorough revision/editing. AC and NS planted the B. napus materials including DNA extraction. AV provided the phenotype data. AS-E, NS and RA provided bioinformatics support by calling SNPs on the reference B. napus cv. Darmor-bzh v9 genome and statistical codes. AC designed the primers/markers and prepared the materials for sequencing analysis. JB supervised, reviewed, and suggested revisions to the paper. All authors contributed to the article and approved the submitted version.
Funding
This study is funded by the Australian Research Council projects DP200100762, and DP210100296 and the Grains Research and Development Corporation (UWA1905-006RTX).
Acknowledgments
All authors acknowledge the University of Western Australia Research Training Program economic support during AYC, WJWT, NSMS and RA’s respective doctoral studies. WJWT would also like to acknowledge the support of the Grains Research and Development Corporation. The authors are also grateful to Yueqi Zhang and Aria Dolatabadian for their assistance in the lab and advice analyzing data.
Conflict of interest
The authors declare that the research was conducted in the absence of any commercial or financial relationships that could be construed as a potential conflict of interest.
Publisher’s note
All claims expressed in this article are solely those of the authors and do not necessarily represent those of their affiliated organizations, or those of the publisher, the editors and the reviewers. Any product that may be evaluated in this article, or claim that may be made by its manufacturer, is not guaranteed or endorsed by the publisher.
Supplementary material
The Supplementary Material for this article can be found online at: https://www.frontiersin.org/articles/10.3389/fpls.2023.1051994/full#supplementary-material
References
Abbadi, A., Leckband, G. (2011). Rapeseed breeding for oil content, quality, and sustainability. Eur. J. Lipid Sci. Technology. 113 (10), 1198–1206. doi: 10.1002/ejlt.201100063
Alnajar, D., von Tiedemann, A., Koopmann, B. (2022). Efficacy of blackleg major resistance genes in b. napus in Germany. Pathogens. 11 (4), 461. doi: 10.3390/pathogens11040461
Anderson, R., Edwards, D., Batley, J., Bayer, P. E. (2020). Genome-wide association studies in plants. eLS, 1–7. doi: 10.1002/9780470015902.a0027950
Balesdent, M. H., Barbetti, M. J., Li, H., Sivasithamparam, K., Gout, L., Rouxel, T. (2005). Analysis of leptosphaeria maculans race structure in a worldwide collection of isolates. Phytopathology. 95 (9), 1061. doi: 10.1094/PHYTO-95-1061
Bankevich, A., Nurk, S., Antipov, D., Gurevich, A. A., Dvorkin, M., Kulikov, A. S., et al. (2012). SPAdes: a new genome assembly algorithm and its applications to single-cell sequencing. J. Comput. Biol. 19 (5), 455–477. doi: 10.1089/cmb.2012.0021
Bayer, P., Scheben, A. P., Golicz, A., Yuan, A., Faure, S., Lee, J., et al. (2020). Brassica oleracea, rapa, napus pangenome data. Ed. Perth, W. A. (35 Stirling Hwy, Crawley WA, Australia: The University of Western Australia). doi: 10.26182/5f1936836a1c4
Becker, M. G., Zhang, X., Walker, P. L., Wan, J. C., Millar, J. L., Khan, D., et al. (2017). Transcriptome analysis of the brassica napus–leptosphaeria maculans pathosystem identifies receptor, signaling and structural genes underlying plant resistance. Plant J. 90 (3), 573–586. doi: 10.1111/tpj.13514
Benjamini, Y., Hochberg, Y. (1995). Controlling the false discovery rate: A practical and powerful approach to multiple testing. J. R. Stat. Soc. Ser. B (Methodological). 57 (1), 289–300. doi: 10.1111/j.2517-6161.1995.tb02031.x
Bolger, A. M., Lohse, M., Usadel, B. (2014). Trimmomatic: a flexible trimmer for illumina sequence data. Bioinformatics. 30 (15), 2114–2120. doi: 10.1093/bioinformatics/btu170
Bradbury, P. J., Zhang, Z., Kroon, D. E., Casstevens, T. M., Ramdoss, Y., Buckler, E. S. (2007). TASSEL: software for association mapping of complex traits in diverse samples. Bioinf. (Oxford England). 23 (19), 2633–2635. doi: 10.1093/bioinformatics/btm308
Bus, A., Korber, N., Snowdon, R. J., Stich, B. (2011). Patterns of molecular variation in a species-wide germplasm set of brassica napus. TAG Theor. Appl. Genet. Theoretische und angewandte Genetik 123 (8), 1413–1423. doi: 10.1007/s00122-011-1676-7
Cantila, A. Y., Saad, N. S., Amas, J. C., Edwards, D., Batley, J. (2021). Recent findings unravel genes and genetic factors underlying leptosphaeria maculans resistance in brassica napus and its relatives. Int. J. Mol. Sci. 22 (1). doi: 10.3390/ijms22010313
Danecek, P., Auton, A., Abecasis, G., Albers, C. A., Banks, E., DePristo, M. A., et al. (2011). The variant call format and VCFtools. Bioinf. (Oxford England). 27 (15), 2156–2158. doi: 10.1093/bioinformatics/btr330
Delourme, R., Chèvre, A. M., Brun, H., Rouxel, T., Balesdent, M. H., Dias, J. S., et al. (2006). Major gene and polygenic resistance to leptosphaeria maculans in oilseed rape (Brassica napus). Eur. J. Plant Pathology. 114 (1), 41–52. doi: 10.1007/s10658-005-2108-9
Dolatabadian, A., Bayer, P. E., Tirnaz, S., Hurgobin, B., Edwards, D., Batley, J. (2019). Characterization of disease resistance genes in the brassica napus pangenome reveals significant structural variation. Plant Biotechnol. J. 18 (4), 969–982. doi: 10.1111/pbi.13262
Ewels, P., Magnusson, M., Lundin, S., Käller, M. (2016). MultiQC: summarize analysis results for multiple tools and samples in a single report. Bioinf. (Oxford England). 32 (19), 3047–3048. doi: 10.1093/bioinformatics/btw354
Ferdous, M. J., Hossain, M. R., Park, J.-I., Kim, H.-T., Robin, A. H. K., Natarajan, S., et al. (2020). In silico characterization and expression of disease-resistance-related genes within the collinear region of brassica napus blackleg resistant locus LepR1 in b. oleracea. J. Gen. Plant Pathology. 86, 442–456. doi: 10.1007/s10327-020-00946-y
Fikere, M., Barbulescu, D. M., Malmberg, M. M., Maharjan, P., Salisbury, P. A., Kant, S., et al. (2020a). Genomic prediction and genetic correlation of agronomic, blackleg disease, and seed quality traits in canola (Brassica napus l.). Plants 9 (6), 719. doi: 10.3390/plants9060719
Fikere, M., Barbulescu, D. M., Malmberg, M. M., Spangenberg, G. C., Cogan, N. O. I., Daetwyler, H. D. (2020b). Meta-analysis of GWAS in canola blackleg (Leptosphaeria maculans) disease traits demonstrates increased power from imputed whole-genome sequence. Sci. Rep. 10 (1), 14300. doi: 10.1038/s41598-020-71274-6
Flor, H. H. (1971). Current status of the gene-For-Gene concept. Annu. Rev. Phytopathology. 9, 275–296. doi: 10.1146/annurev.py.09.090171.001423
Fu, F., Zhang, X., Liu, F., Peng, G., Yu, F., Fernando, D. (2020). Identification of resistance loci in Chinese and Canadian canola/rapeseed varieties against leptosphaeria maculans based on genome-wide association studies. BMC Genomics 21 (1), 501. doi: 10.1186/s12864-020-06893-4
Gacek, K., Bayer, P. E., Bartkowiak-Broda, I., Szala, L., Bocianowski, J., Edwards, D., et al. (2016). Genome-wide association study of genetic control of seed fatty acid biosynthesis in brassica napus. Front. Plant Sci. 7, 2062. doi: 10.3389/fpls.2016.02062
Grant, M. R., McDowell, J. M., Sharpe, A. G., de Torres Zabala, M., Lydiate, D. J., Dangl, J. L. (1998). “Independent deletions of a pathogen-resistance gene in brassica and arabidopsis,” in Proceedings of the National Academy of Sciences, 95(26). 15843–15848. doi: 10.1073/pnas.95.26.15843
Gugel, R. K., Petrie, G. A. (1992). History, occurrence, impact, and control of blackleg of rapeseed. Can. J. Plant Pathology. 14 (1), 36–45. doi: 10.1080/07060669209500904
Haddadi, P., Larkan, N. J., Borhan, M. H. (2019). Dissecting r gene and host genetic background effect on the brassica napus defense response to leptosphaeria maculans. Sci. Rep. 9 (1), 6947. doi: 10.1038/s41598-019-43419-9
Haddadi, P., Larkan, N. J., Van deWouw, A., Zhang, Y., Xiang Neik, T., Beynon, E., et al. (2022). Brassica napus genes Rlm4 and Rlm7, conferring resistance to leptosphaeria maculans, are alleles of the Rlm9 wall-associated kinase-like resistance locus. Plant Biotechnol. J. 20 (7), 1–3. doi: 10.1111/pbi.13818
Hill, W., Robertson, A. (1968). Linkage disequilibrium in finite populations. Theor. Appl. Genet. 38 (6), 226–231. doi: 10.1007/BF01245622
Horvath, D. P., Stamm, M., Talukder, Z. I., Fiedler, J., Horvath, A. P., Horvath, G. A., et al. (2020). A new diversity panel for winter rapeseed (Brassica napus l.) genome-wide association studies. Agronomy 10 (12). doi: 10.3390/agronomy10122006
Huang, Y. J., Pirie, E. J., Evans, N., Delourme, R., King, G. J., Fitt, B. D. L. (2009). Quantitative resistance to symptomless growth of leptosphaeria maculans (phoma stem canker) in brassica napus (oilseed rape). Plant Pathology. 58 (2), 314–323. doi: 10.1111/j.1365-3059.2008.01957.x
Hu, J., Chen, B., Zhao, J., Zhang, F., Xie, T., Xu, K., et al. (2022). Genomic selection and genetic architecture of agronomic traits during modern rapeseed breeding. Nat. Genet. 54 (5), 694–704. doi: 10.1038/s41588-022-01055-6
Jiquel, A., Gervais, J., Geistodt-Kiener, A., Delourme, R., Gay, E. J., Ollivier, B., et al. (2021). A gene-for-gene interaction involving a 'late' effector contributes to quantitative resistance to the stem canker disease in brassica napus. New phytologist. 231 (4), 1510–1524. doi: 10.1111/nph.17292
Kearse, M., Moir, R., Wilson, A., Stones-Havas, S., Cheung, M., Sturrock, S., et al. (2012). Geneious basic: An integrated and extendable desktop software platform for the organization and analysis of sequence data. Bioinf. (Oxford England). 28 (12), 1647–1649. doi: 10.1093/bioinformatics/bts199
Kutcher, H. R., Yu, F., Brun, H. (2010). Improving blackleg disease management of brassica napus from knowledge of genetic interactions with leptosphaeria maculans. Can. J. Plant Pathology. 32 (1), 29–34. doi: 10.1080/07060661003620961
Langmead, B., Salzberg, S. L. (2012). Fast gapped-read alignment with bowtie 2. Nat. Methods 9 (4), 357–359. doi: 10.1038/nmeth.1923
Larkan, N. J., Lydiate, D. J., Parkin, I. A., Nelson, M. N., Epp, D. J., Cowling, W. A., et al. (2013). The brassica napus blackleg resistance gene LepR3 encodes a receptor-like protein triggered by the leptosphaeria maculans effector AVRLM1. New Phytol. 197 (2), 595–605. doi: 10.1111/nph.12043
Larkan, N. J., Ma, L., Borhan, M. H. (2015). The brassica napus receptor-like protein RLM2 is encoded by a second allele of the LepR3/Rlm2 blackleg resistance locus. Plant Biotechnol. J. 13 (7), 983–992. doi: 10.1111/pbi.12341
Larkan, N. J., Ma, L., Haddadi, P., Buchwaldt, M., Parkin, I. A. P., Djavaheri, M., et al. (2020). The brassica napus wall-associated kinase-like (WAKL) gene Rlm9 provides race-specific blackleg resistance. Plant J. 104 (4):892–900. doi: 10.1111/tpj.14966
Larkan, N. J., Yu, F., Lydiate, D. J., Rimmer, S. R., Borhan, M. H. (2016). Single r gene introgression lines for accurate dissection of the brassica - leptosphaeria pathosystem. Front. Plant Sci. 7 (1771). doi: 10.3389/fpls.2016.01771
Li, H. (2011). Tabix: fast retrieval of sequence features from generic TAB-delimited files. Bioinf. (Oxford England). 27 (5), 718–719. doi: 10.1093/bioinformatics/btq671
Li, H., Barbetti, M. J., Sivasithamparam, K. (2005). Hazard from reliance on cruciferous hosts as sources of major gene-based resistance for managing blackleg (Leptosphaeria maculans) disease. Field Crops Res. 91, 185–198. doi: 10.1016/j.fcr.2004.06.006
Li, H., Handsaker, B., Wysoker, A., Fennell, T., Ruan, J., Homer, N., et al. (2009). The sequence Alignment/Map format and SAMtools. Bioinf. (Oxford England). 25 (16), 2078–2079. doi: 10.1093/bioinformatics/btp352
Li, P., Quan, X., Jia, G., Xiao, J., Cloutier, S., You, F. M. (2016). RGAugury: a pipeline for genome-wide prediction of resistance gene analogs (RGAs) in plants. BMC Genomics 17 (1), 852. doi: 10.1186/s12864-016-3197-x
Liu, X., Huang, M., Fan, B., Buckler, E. S., Zhang, Z. (2016). Iterative usage of fixed and random effect models for powerful and efficient genome-wide association studies. PloS Genet. 12 (2), e1005767. doi: 10.1371/journal.pgen.1005767
Ma, L., Djavaheri, M., Wang, H., Larkan, N. J., Haddadi, P., Beynon, E., et al. (2018). Leptosphaeria maculans effector protein AvrLm1 modulates plant immunity by enhancing MAP kinase 9 phosphorylation. iScience. 3, 177–191. doi: 10.1016/j.isci.2018.04.015
Marcroft, S. J., Elliott, V. L., Cozijnsen, A. J., Salisbury, P. A., Howlett, B. J., Van de Wouw, A. P. (2012). Identifying resistance genes to in Australian cultivars based on reactions to isolates with known avirulence genotypes. Crop Pasture Science. 63 (4), 338–350. doi: 10.1071/CP11341
Martínez-Montes, ÁM, Fernández, A., Muñoz, M., Noguera, J. L., Folch, J. M., Fernández, A. I. (2018). Using genome wide association studies to identify common QTL regions in three different genetic backgrounds based on Iberian pig breed. PloS One 13 (3), e0190184-e. doi: 10.1371/journal.pone.0190184
Parkin, I. A. P., Gulden, S. M., Sharpe, A. G., Lukens, L., Trick, M., Osborn, T. C., et al. (2005). Segmental structure of the brassica napus genome based on comparative analysis with arabidopsis thaliana. Genetics. 171 (2), 765. doi: 10.1534/genetics.105.042093
Potter, T., Burton, W., Edwards, J., Wratten, N., Mailer, R., Salisbury, P., et al. (2016). Assessing progress in breeding to improve grain yield, quality and blackleg (Leptosphaeria maculans) resistance in selected Australian canola cultivars (1978–2012). Crop Pasture Sci. 67 (3–4), 308–316. doi: 10.1071/CP15290
Price, A. L., Patterson, N. J., Plenge, R. M., Weinblatt, M. E., Shadick, N. A., Reich, D. (2006). Principal components analysis corrects for stratification in genome-wide association studies. Nat. Genet. 38 (8), 904–909. doi: 10.1038/ng1847
Rahman, M., Hoque, A., Roy, J. (2022). Linkage disequilibrium and population structure in a core collection of brassica napus (L.). PloS One 17 (3), e0250310. doi: 10.1371/journal.pone.0250310
Raman, H., Raman, R., Coombes, N., Song, J., Diffey, S., Kilian, A., et al. (2016). Genome-wide association study identifies new loci for resistance to leptosphaeria maculans in canola. Front. Plant science. 7, 1513. doi: 10.3389/fpls.2016.01513
Raman, H., Raman, R., Diffey, S., Qiu, Y., McVittie, B., Barbulescu, D. M., et al. (2018). Stable quantitative resistance loci to blackleg disease in canola (Brassica napus l.) over continents. Front. Plant Sci. 9 (1622). doi: 10.3389/fpls.2018.01622
Raman, H., Raman, R., Kilian, A., Detering, F., Carling, J., Coombes, N., et al. (2014). Genome-wide delineation of natural variation for pod shatter resistance in brassica napus. PloS One 9 (7), e101673. doi: 10.1371/journal.pone.0101673
Raman, R., Taylor, B., Lindbeck, K., Coombes, N., Barbulescu, D., Salisbury, P., et al. (2012). Molecular mapping and validation of Rlm1 gene for resistance to leptosphaeria maculans in canola (Brassica napus l.). Crop Pasture Sci. 63 (10), 1007–17, 11. doi: 10.1071/CP12255
Raman, R., Taylor, B., Marcroft, S., Stiller, J., Eckermann, P., Coombes, N., et al. (2012). Molecular mapping of qualitative and quantitative loci for resistance to leptosphaeria maculans causing blackleg disease in canola (Brassica napus l.). TAG Theor. Appl. Genet. Theoretische und angewandte Genetik 125 (2), 405–418. doi: 10.1007/s00122-012-1842-6
Rashid, M. H., Liban, S. H., Zhang, X., Parks, P. S., Borhan, H., Fernando, W. G. D. (2022). Comparing the effectiveness of r genes in a 2-year canola–wheat rotation against leptosphaeria maculans, the causal agent of blackleg disease in brassica species. Eur. J. Plant Pathol 163(3), 573–586. doi: 10.1007/s10658-022-02498-7
Rousseau-Gueutin, M., Belser, C., Da Silva, C., Richard, G., Istace, B., Cruaud, C., et al. (2020). Long-read assembly of the brassica napus reference genome darmor-bzh. gigascience 9 (12), 16. doi: 10.1093/gigascience/giaa137
Rouxel, T., Willner, E., Coudard, L., Balesdent, M.-H. (2003). Screening and identification of resistance to leptosphaeria maculans (stem canker) in brassica napus accessions. Euphytica. 133 (2), 219–231. doi: 10.1023/A:1025597622490
Şensöz, S., Angın, D., Yorgun, S. (2000). Influence of particle size on the pyrolysis of rapeseed (Brassica napus l.): Fuel properties of bio-oil. Biomass Bioenergy 19, 271–279. doi: 10.1016/S0961-9534(00)00041-6
Salisbury, P. A., Cowling, W. A., Potter, T. D. (2016). Continuing innovation in Australian canola breeding. Crop Pasture Sci. 67 (3–4), 266–272. doi: 10.1071/CP15262
Staal, J., Kaliff, M., Bohman, S., Dixelius, C. (2006). Transgressive segregation reveals two arabidopsis TIR-NB-LRR resistance genes effective against leptosphaeria maculans, causal agent of blackleg disease. Plant J. 46 (2), 218–230. doi: 10.1111/j.1365-313X.2006.02688.x
Staal, J., Kaliff, M., Dewaele, E., Persson, M., Dixelius, C. (2008). RLM3, a TIR domain encoding gene involved in broad-range immunity of arabidopsis to necrotrophic fungal pathogens. Plant J. Cell Mol. Biol. 55 (2), 188–200. doi: 10.1111/j.1365-313X.2008.03503.x
Sun, G., Zhu, C., Kramer, M., Yang, S., Song, W., Piepho, H., et al. (2010). Variation explained in mixed-model association mapping. Heredity. 105 (4), 333–340. doi: 10.1038/hdy.2010.11
Van de Wouw, A. P., Cozijnsen, A. J., Hane, J. K., Brunner, P. C., McDonald, B. A., Oliver, R. P., et al. (2010). Evolution of linked avirulence effectors in leptosphaeria maculans is affected by genomic environment and exposure to resistance genes in host plants. PloS Pathogens. 6 (11), e1001180. doi: 10.1371/journal.ppat.1001180
Van de Wouw, A. P., Elliott, V. L., Chang, S., López-Ruiz, F. J., Marcroft, S. J., Idnurm, A. (2017). Identification of isolates of the plant pathogen leptosphaeria maculans with resistance to the triazole fungicide fluquinconazole using a novel in planta assay. PloS One 12 (11), e0188106. doi: 10.1371/journal.pone.0188106
Van de Wouw, A. P., Marcroft, S. J., Barbetti, M. J., Hua, L., Salisbury, P. A., Gout, L., et al. (2009). Dual control of avirulence in leptosphaeria maculans towards a brassica napus cultivar with ‘sylvestris-derived’ resistance suggests involvement of two resistance genes. Plant Pathology. 58 (2), 305–313. doi: 10.1111/j.1365-3059.2008.01982.x
Van de Wouw, A. P., Marcroft, S. J., Sprague, S. J., Scanlan, J. L., Vesk, P. A., Idnurm, A. (2021). Epidemiology and management of blackleg of canola in response to changing farming practices in Australia. Australas. Plant Pathology. 50 (2), 137–149. doi: 10.1007/s13313-020-00767-9
Van de Wouw, A., Marcroft, S., Ware, A., Lindbeck, K., Khangura, R., Howlett, B. (2014). Breakdown of resistance to the fungal disease, blackleg, is averted in commercial canola (Brassica napus) crops in Australia. Field Crops Res. 166, 144–151. doi: 10.1016/j.fcr.2014.06.023
Van de Wouw, A. P., Scanlan, J. L., Marcroft, S. J., Smith, A. J., Sheedy, E. M., Perndt, N. W., et al. (2021). Fungicide sensitivity and resistance in the blackleg fungus, Leptosphaeria maculans, across canola growing regions in Australia. Crop Pasture Science. 72 (12), 994–1007, 14. doi: 10.1071/CP21369
Van de Wouw, A. P., Zhang, Y., Mohd Saad, N. S., Yang, H., Sheedy, E., Elliott, C. E., et al. (2022). Molecular markers for identifying resistance genes in brassica napus. Agronomy. 12 (5), 985. doi: 10.3390/agronomy12050985
VanRaden, P. M. (2008). Efficient methods to compute genomic predictions. J. dairy science. 91 (11), 4414–4423. doi: 10.3168/jds.2007-0980
Voorrips, R. E. (2002). MapChart: Software for the graphical presentation of linkage maps and QTLs. J. Heredity. 93 (1), 77–78. doi: 10.1093/jhered/93.1.77
Wang, J., Zhang, Z. (2021). GAPIT version 3: Boosting power and accuracy for genomic association and prediction. Genomics Proteomics Bioinf 19 (4), 629–640. doi: 10.1016/j.gpb.2021.08.005
West, J. S., Kharbanda, P. D., Barbetti, M. J., Fitt, B. D. L. (2001). Epidemiology and management of leptosphaeria maculans (phoma stem canker) on oilseed rape in Australia, Canada and Europe. Plant Pathology. 50 (1), 10–27. doi: 10.1046/j.1365-3059.2001.00546.x
Wu, J., Zhao, Q., Liu, S., Shahid, M., Lan, L., Cai, G., et al. (2016). Genome-wide association study identifies new loci for resistance to sclerotinia stem rot in brassica napus. Front. Plant Sci. 7 (1418). doi: 10.3389/fpls.2016.01418
Xiao, Y., Cai, D., Yang, W., Ye, W., Younas, M., Wu, J., et al. (2012). Genetic structure and linkage disequilibrium pattern of a rapeseed (Brassica napus l.) association mapping panel revealed by microsatellites. Theor. Appl. Genet. 125 (3), 437–447. doi: 10.1007/s00122-012-1843-5
Yin, L., Zhang, H., Tang, Z., Xu, J., Yin, D., Zhang, Z., et al. (2021). rMVP: A memory-efficient, visualization-enhanced, and parallel-accelerated tool for genome-wide association study. Genomics Proteomics Bioinf 19 (4), 619–628. doi: 10.1016/j.gpb.2020.10.007
Yu, F., Lydiate, D. J., Gugel, R. K., Sharpe, A. G., Rimmer, S. R. (2012). Introgression of brassica rapa subsp. sylvestris blackleg resistance into b. napus. Mol. Breeding. 30 (3), 1495–1506. doi: 10.1007/s11032-012-9735-6
Yu, F., Lydiate, D. J., Rimmer, S. R. (2005). Identification of two novel genes for blackleg resistance in brassica napus. TAG Theor. Appl. Genet. Theoretische und angewandte Genetik 110 (5), 969–979. doi: 10.1007/s00122-004-1919-y
Yu, J., Pressoir, G., Briggs, W. H., Vroh Bi, I., Yamasaki, M., Doebley, J. F., et al. (2006). A unified mixed-model method for association mapping that accounts for multiple levels of relatedness. Nat. Genet. 38 (2), 203–208. doi: 10.1038/ng1702
Yu, F., Rimmer, S. R., Lydiate, D. J., Buzza, G. (2002). Identification of two loci in brassica napus for resistance to leptosphaeria maculans, Saskatchewan regional meeting, 2001, the Canadian phytopathological society. Can. J. Plant Pathology. 24 (1), 95–97.
Keywords: association mapping, blackleg resistance, canola, gene sequencing, LepR1 resistant-specific marker
Citation: Cantila AY, Thomas WJW, Saad NSM, Severn-Ellis AA, Anderson R, Bayer PE, Edwards D, Van de Wouw AP and Batley J (2023) Identification of candidate genes for LepR1 resistance against Leptosphaeria maculans in Brassica napus. Front. Plant Sci. 14:1051994. doi: 10.3389/fpls.2023.1051994
Received: 23 September 2022; Accepted: 19 January 2023;
Published: 14 February 2023.
Edited by:
Sateesh Kagale, National Research Council Canada (NRC), CanadaReviewed by:
Bianyun Yu, National Research Council Canada (NRC-CNRC), CanadaBerisso Demo Kebede, University of Alberta, Canada
Copyright © 2023 Cantila, Thomas, Saad, Severn-Ellis, Anderson, Bayer, Edwards, Van de Wouw and Batley. This is an open-access article distributed under the terms of the Creative Commons Attribution License (CC BY). The use, distribution or reproduction in other forums is permitted, provided the original author(s) and the copyright owner(s) are credited and that the original publication in this journal is cited, in accordance with accepted academic practice. No use, distribution or reproduction is permitted which does not comply with these terms.
*Correspondence: Jacqueline Batley, jacqueline.batley@uwa.edu.au