- 1State Key Laboratory of Hybrid Rice, College of Life Sciences, Wuhan University, Wuhan, China
- 2Center for Genomics and Biotechnology, Fujian Provincial Key Laboratory of Haixia Applied Plant Systems Biology, Fujian Agriculture and Forestry University, Fuzhou, China
- 3Key Laboratory of Crop Physiology, Ecology and Genetic Breeding (Jiangxi Agricultural University), Ministry of Education of the People’s Republic of China, Nanchang, China
Following the “green revolution,” indica and japonica hybrid breeding has been recognized as a new breakthrough in further improving rice yields. However, heterosis-related grain weight QTLs and the basis of yield advantage among subspecies has not been well elucidated. We herein de novo assembled the chromosome level genomes of an indica/xian rice (Luohui 9) and a japonica/geng rice (RPY geng) and found that gene number differences and structural variations between these two genomes contribute to the differences in agronomic traits and also provide two different favorable allele pools to produce better derived recombinant inbred lines (RILs). In addition, we generated a high-generation (> F15) population of 272 RILs from the cross between Luohui 9 and RPY geng and two testcross hybrid populations derived from the crosses of RILs and two cytoplasmic male sterile lines (YTA, indica and Z7A, japonica). Based on three derived populations, we totally identified eight 1,000-grain weight (KGW) QTLs and eight KGW heterosis loci. Of QTLs, qKGW-6.1 and qKGW-8.1 were accepted as novel KGW QTLs that have not been reported previously. Interestingly, allele genotyping results revealed that heading date related gene (Ghd8) in qKGW-8.1 and qLH-KGW-8.1, can affect grain weight in RILs and rice core accessions and may also play an important role in grain weight heterosis. Our results provided two high-quality genomes and novel gene editing targets for grain weight for future rice yield improvement project.
Introduction
Rice is one of the most important food crops in the world, providing food for more than half of the world’s population (Qin et al., 2021). Over the past two decades, multiple high-quality genomes of indica and japonica subspecies have been assembled, such as Nipponbare (Goff et al., 2002), 9311 (Yu et al., 2002), ZS97 (Zhang et al., 2016a,b), MH63 (Zhang et al., 2016a,b), R498 (Du et al., 2017), IR64 (Tanaka et al., 2020), TN1 (Panibe et al., 2021), Huazhan (Zhang H. et al., 2022), Tianfeng (Zhang H. et al., 2022), etc. Recently, several gap-free reference genomes were completed, namely, ZS97, MH63, PR106, LIMA, LARHAMUGAD, KETANNANGKA, NATELBORO, XL628S, LK638S, J4155S, and HZ (Song et al., 2021; Zhang F. et al., 2022; Zhang Y. et al., 2022). Advances in third-generation sequencing and assembly algorithms have continuously updated the accuracy of the rice pan-genome, revealing some important functionally related structural variations (SVs) and gene copy number variations (gCNVs) (Zhao et al., 2018; Qin et al., 2021; Zhang F. et al., 2022). However, there are dramatically different genetic backgrounds among thousands of rice cultivars, especially between subspecies, including cultivar-specific genes, different alleles of one gene, or gene family expansions (Li et al., 2021). Differences in rice agronomic traits are closely related to these genome variations (Stein et al., 2018; Zhao et al., 2018; Qin et al., 2021). Therefore, the discovery of new genes/alleles related to agronomic traits is inseparable from the comparative analysis of the genomes of elite varieties and the fine mapping in derived populations. For instance, we previously found that the hybrid progeny of the Luohui 9 (xian/indica) and RPY geng (geng/japonica) cross had significant heterosis in yield and resistance traits, and multiple recombinant inbred lines (RILs) derived from Luohui 9 X RPY geng aggregated the advantages of both parents (Kong et al., 2022a). Based on the high-density genetic map, we also obtained some QTLs related to plant height, salt stress tolerance, submerged germination, and grain shape (Kong et al., 2021b, 2022a,b; Deng et al., 2022). But the gene number differences and large structural variations between Luohui 9 and RPY geng, and the effects of these variations/differences on traits and heterosis, remain unclear.
Owing to the impacts of human population growth and limited arable land, breeders and scientists faced the challenge of breeding higher yield potential crops. Rice yield is a complex agronomic trait composed of four main factors including effective panicle number, grain number per panicle, seed setting rate and 1,000-grain weight (KGW) (Zuo and Li, 2014). In addition, heterosis refers to the phenomenon that the phenotype of the hybrid progeny surpasses their parents in biomass, yield, growth vigor, resistance, etc., (Birchler et al., 2010). Yield heterosis between indica and japonica subspecies has been widely used to improve rice yield, causing a worldwide yield revolution (Li et al., 2018). As statistics, hybrid rice shows a 20–30% increase in yield than inbred rice and has effectively solved world food crisis (Xu et al., 2016). Therefore, analyzing the mechanism of rice grain weight (GW) and mining GW-related QTLs and GW-related heterosis loci are important foundations for improving rice yield. Based on different populations and QTL mapping methods, more than 600 QTLs related to grain weight and grain shape have been identified on all 12 chromosomes in rice to date1 (Chan et al., 2021), and more than 20 QTLs have been cloned, including GW2 (Yan S. et al., 2011), GS3 (Liu et al., 2018), TGW6 (Ishimaru et al., 2013), GW6a (Song et al., 2015), WTG1 (Huang et al., 2017), GL7 (Wang et al., 2015), and gw5 (Wan et al., 2005). In fact, multiple reported QTLs may belong to one QTL, but there are differences in the size of the interval. Meta-analysis of QTLs was used to merge multiple QTLs from different rice genetic populations and to identify consensus and stable QTLs (Arcade et al., 2004; Kong et al., 2020), which narrowed down the confidence intervals of QTLs (Martinez et al., 2016; Zhang et al., 2017). Recently, 339 published GW QTLs merged into 34 Meta-QTLs (MQTLs) in rice (Khahani et al., 2020). The new GW QTLs/genes must be urgently explored in indica X japonica derived populations to further improve rice yields.
In this study, we performed the chromosome-level de novo assembly of the Luohui 9 and RPY geng genomes and characterized their genomic differences in genome-wide scale. Additionally, the KGW traits of derived RIL populations from Luohui 9 X RPY geng in four environments were used for QTL mapping. Two testcross populations derived from the crosses of RILs and Z7A (japonica) or YTA (indica) were used to explore the heterosis loci of KGW. These results provided a new insight into the diversity mechanism of grain weight in rice.
Materials and methods
Materials and sequencing
The highly homozygous O. sativa ssp. indica/xian (Luohui 9, 2n = 2 × = 24) and O. sativa ssp. japonica/geng (RPY geng, 2n = 2 × = 24) were planted in the field in Wuhan, China, in 2016, These two subspecies have many significant differences in important agronomic traits, including plant height, number of tillers, and heading date, for example, Luohui 9 has excellent agronomic traits, and RPY geng has an ideal plant architecture (Figure 1A).
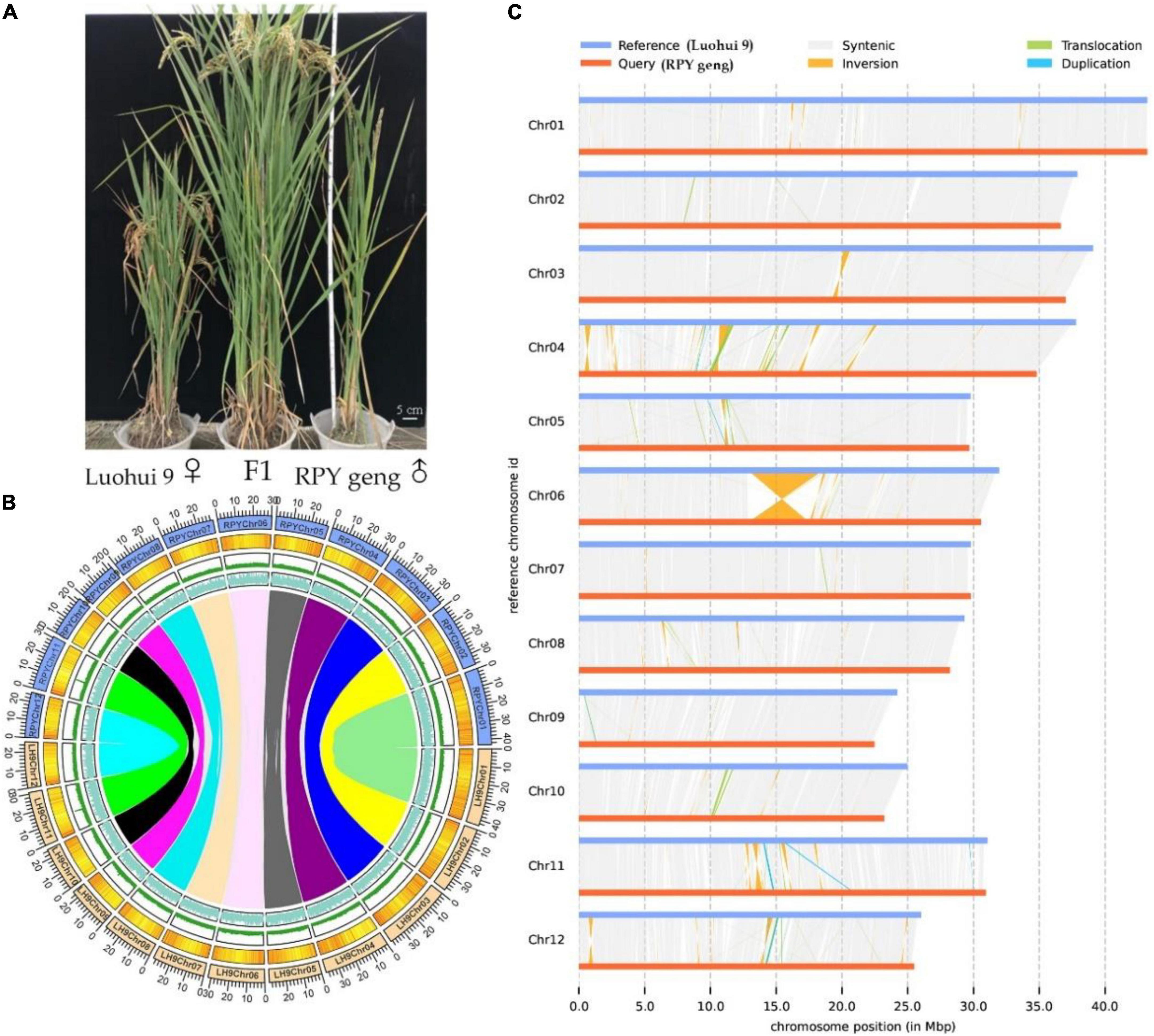
Figure 1. Whole plant phenotype (A), genomic characteristics (B), and large structural variations (C) between RPY geng and Luohui 9. The tracks from outside to inside are the chromosome, gene density, repeat sequence density, and GC content and the different color links represent orthologous gene pairs among chromosomes in (B).
The genomic DNA of these two subspecies was extracted from young leaves using a modified CTAB method and tested using Qubit Quantitation Starter Kit (Invitrogen, United States) and a 1% agarose gel electrophoresis, respectively. Libraries for Illumina short-read and single-molecule real-time (SMRT) sequencing (Pacific Biosciences, United States) were prepared according to the respective manufacturer’s instructions. The short-read DNA libraries were sequenced by paired-end (2 × 150 bp) method on Illumina HiSeqTM 2,500 (Illumina, United States) and the SMRT sequencing were sequenced on the PacBio RS II platform. In addition, Hi-C reads of DNA of young leaves from F1 progeny of Luohui 9 and RPY geng were sequenced on Illumina HiSeqTM 2,500 paired-end (2 × 150 bp) sequencing according to standard protocol. The total RNA of mixed tissues (root, stem, leaf, and young panicle) was extracted for RNA-seq libraries following the manufacturer’s standard protocol. Then, RNA-seq libraries were sequenced on an Illumina HiSeqTM 2,500 paired-end (2 × 150 bp) and raw reads were filtered using Trimmomatic software as described previously (Kong et al., 2020).
Genome assembly and annotation
PacBio RSII sub-reads were filtered by the PacBio SMRT-Analysis package including removing the adapters, low quality, and short length reads (parameters: readScore, 0.75; minSubReadLength, 500) and sub-reads after filtering were corrected by Illumina reads using an error correction module embedded in Canu v1.5 (Koren et al., 2017). The high-quality PacBio sub-reads were used for genome contigs assembly by using Canu v1.5 with default parameters. The contig-level genome was polished by Plion with these parameters: –mindepth 10; –changes; –threads 4; –fix bases (Walker et al., 2014). Hi-C data were used to assist in constructing chromosome-level genome assemblies. The Hi-C data were mapped to the contig-level genomes using BWA aligner software (Li and Durbin, 2009). A total of 46.73 Gb clean Hi-C data was mapped to the genome, with a coverage of 116.83 X. These uniquely mapped Hi-C reads were retained for chromosome-level genome assemblies using LACHESIS software with these parameters: CLUSTER MIN RE SITES = 22; CLUSTER MAX LINK DENSITY = 2; CLUSTER NON-INFORMATIVE RATIO = 2; ORDER MIN N RES IN TRUNK = 10; ORDER MIN N RES IN SHREDS = 10 (Burton et al., 2013). Finally, the chromosome-level genome was further improved and corrected by the high-density binmaps genetic map constructed in this study using all maps software according to the method described (Tang H. B. et al., 2015).
Repeat sequence annotation was performed by EDTA with default parameters (Ou et al., 2019). Coding genes were predicted by de novo, homolog-based, and transcriptome-based strategies. Augustus v2.4 (Stanke and Waack, 2003), Genscan (Burge and Karlin, 1997), GeneID v1.4 (Alioto et al., 2018), GlimmerHMM v3.0.4 (Majoros et al., 2004), and SNAP (version 2006-07-28) (Korf, 2004) were used for de novo prediction. GeMoMa v1.3.1 (Keilwagen et al., 2016) was used for homolog-based prediction. In the transcriptome-based prediction, we used Hisat v2.0.4 and Stringtie v1.2.3 for sequence assembly based on a reference genome (Pertea et al., 2016), and applied TransDecoder v2.02 and GeneMarkS-T v5.1 (Tang S. Y. Y. et al., 2015) for gene prediction; On the other hand, PASA v2.0.2 software (Campbell et al., 2006) was used to perform unigene sequence prediction without reference assembly based on transcriptome sequencing data. Finally, we used the EVM v1.1.1 software (Haas et al., 2008) to integrate all gene prediction results from these three analysis methods. The predicted coding genes were annotated according to alignments against (E value 1e–5) databases including GO, KEGG, KOG, TrEMBL, and Nr databases using BLAST v2.2.31 (Altschul, 2012).
Orthologous clusters analysis and structural variant identification
We extracted the longest-protein sequences from Luohui 9 and RPY geng genomes for orthologous clusters identification using OrthoVenn2, E-value was set 1e–10, and other parameters with default (Xu et al., 2019). Genomic structural variants between Luohui 9 and RPY geng genomes were identified by SyRI (Goel et al., 2019). Luohui 9 genome was used as the reference genome, “nucmer – mum” for sequence alignment, with parameter, -c 100 -l 50 -g 1,000. Then, “delta-filter” was used to filter the comparison results, with parameter, -1 -q -r -i 89 -l 50. Finally, “show-coords,” “syri -c,” and “syri plotsr” steps were done with default parameters.
QTL mapping of KGW
A 272 RILs and their parents were planted in the Ezhou (30°N, 114°E) Experimental Base of Wuhan University, Wuhan City, Hubei Province in April 2017 (2017EZ) and in April 2018 (2018EZ), the Hybrid Rice Experimental Base of Wuhan University in Lingshui City (18°N, 110°E), Hainan Province in November 2019 (2019LS), and Breeding Experimental Base of Wuhan University Tianyuan Co., Ltd in Hannan District (30°N, 114°E), Wuhan City, Hubei Province in May 2019 (2019HN). Two testcross populations (Z7A-TCF1 and YTA-TCF1) were developed by crossing RILs (F14) with Z7A (japonica) and YTA (indica) in 2019LS. KGWs of Z7A-TCF1, YTA-TCF1, and RILs were investigated in 2019HN.
All plants were planted under standard agricultural management practice (Kong et al., 2022a). KGW was surveyed in the above four environments. Each inbred line counted five individual plants, the average KGW value of five individual plants was considered as the KGW value of each inbred line.
The genetic linkage map of 272 RILs including 4,578 bin blocks with the total bin-map distance 2,356.41 cM was previously constructed in our lab (Kong et al., 2022a). The QTL mapping of KGW was analyzed by R/qtl (Arends et al., 2010), the CIM interval mapping method was adopted and the LOD threshold was set by 3.0. The confidence interval was calculated with the function “lodint” (Dupuis and Siegmund, 1999) and the drop value was set to 1.5.
QTL mapping of KGW heterosis loci
Heterosis related indexes for the KGW trait were calculated by the formulas:
where MPH is middle-parent heterosis, BPH is better-parent heterosis, LPH is lower-parent heterosis, P1 is the high parent, and P2 is the low parent.
The QTL mapping of KGW heterosis related indexes was analyzed by R/qtl (Arends et al., 2010), the CIM interval mapping method was adopted and the LOD threshold was set by 2.5. The confidence interval was calculated with the function “lodint” (Dupuis and Siegmund, 1999) and the drop value was set to 1.5.
Results and discussion
De novo assembly and annotation of RPY geng and Luohui 9 genomes
A total of 25.1 / 36.5 Gb Illumina short reads with 62 / 91 X coverage of the genome and 15.6 / 19.5 Gb PacBio RSII long reads with 39 / 48 X coverage of the genome of RPY geng / Luohui 9 was obtained (Supplementary Table 1). The long reads were polished by the Illumina paired read and the polished long reads were assembled into contigs by Canu V1.5. After three rounds of contig polish by Pilon v1.22 and Hi-C data correction, we obtained a 383.45 Mb RPY geng genome and a 394.43 Luohui 9 genome, with the contig N50 of 2.81 and 2.84 Mb, respectively (Table 1). Luohui 9 was ∼10.98 Mb larger than the genome of RPY geng. Finally, 96.35 and 96.99 % of contigs were anchored onto 12 pseudo-chromosomes of RPY geng and Luohui 9 based on Hi-C interactions and linkage map from RPY geng x Luohui 9 derived population (Kong et al., 2022a), respectively (Table 1 and Supplementary Figure 1).
The percentages of repeat sequences in the genomes of RPY geng and Luohui 9 were 44.29 and 46.96% based on EDTA with default parameters (Supplementary Table 2). A combination of prediction strategies (de novo, homologous based and RNA-seq based) totally identified 39,255 and 39,440 gene models among RPY geng and Luohui 9 genomes, of which 96.81 and 94.75% had at least one annotation result in GO, KEGG, KOG, TrEMBL, or Nr database (Supplementary Tables 3, 4). The results of CEGMA and BUSCO showed that the assembly of RPY geng and Luohui 9 was complete, with more than 95.0% of the core genes. The long terminal repeat (LTR)-assembly index (LAI) of RPY geng and Luohui 9 was 19.44 and 19.37, which is close to the gold genome level (LAI ≥ 20) (Table 1). All the above-mentioned genome indices indicated that the newly assembled genomes of RPY geng and Luohui 9 was of high quality.
Global genome differences between RPY geng and Luohui 9
RPY geng and Luohui 9 showed obvious differences in yield, grain shape (Deng et al., 2022), plant height (Kong et al., 2022a), and abiotic stress resistances (Kong et al., 2020, 2021a,b,2022b), and the hybrid progeny of RPY geng X Luohui 9 had the excellent heterosis (Figure 1A). These essential agronomic differences are inseparable from the number and structural variation of genes between the two subspecies genomes (Zhao et al., 2018; Qin et al., 2021). Benefiting from the completion of the genomes of RPY geng and Luohui 9, we compared their gene numbers and large structural variations at the genome-wide level and highlighted some genes that have potential impact on agronomic traits. A total of 32,720 orthologous clusters including 32,509 orthologous gene pairs were identified (Supplementary Figure 2 and Figure 1B). Luohui 9 unique orthologous clusters were enriched with multiple essential life GO terms, while RPY geng unique orthologous clusters were enriched with multiple stress-related GO terms (Supplementary Table 5), namely, defense response, cellular response to amino acid stimulus, positive regulation of hydrogen peroxide, as well as response to osmotic stress, suggesting that RPY geng has more tolerance-related genes to abiotic stress than Luohui 9. These results are consistent with our previous findings that RPY geng has stronger resistance to salt stress and cold stress and carries important stress tolerance genes (Kong et al., 2020, 2021a,b,2022b).
We further found 190 inversions, 6,852 translocations, 1,279 (Luohui 9)/1,212 (RPY geng) duplications between RPY geng and Luohui 9 involving 2,234 SV-related genes in Luohui 9 and 1,544 SV-related genes in RPY geng (Supplementary Table 6). Notably, at the position of 12.8–18.6 Mb on Luohui 9 chromosome 6 showed a sequence inversion with a length of about 5.7 Mb compared with the RPY geng genome (Figure 1C) and this inversion has also been reported in previous comparative genomic studies between subspecies (Du et al., 2017; Li et al., 2021; Xie et al., 2021), suggesting that this may be an important structural difference between subspecies. To study the potential roles of these SV-related genes in important agronomic traits, we collected 283 important known genes with functional function verifications (Supplementary Table 7) as query sequences to find homologous genes against SV-related genes by BlastP (value E-10). Totally, 337 SV-related genes were identified as homologous genes of 138 known functional genes belonging to cold tolerance, heat tolerance, salt tolerance, insect resistance, disease resistance, drought tolerance, fertility, grain quality, grain shape, heading date, panicle architecture, nutrient utilization, and panicle architecture (Supplementary Table 8). The above results suggested that the genome-wide number and structural differences play essential roles in the trait differences of RPY geng and Luohui 9, which were consistent with their differential trait characteristics.
QTLs of KGW
RPY geng and Luohui 9 belonged to japonica/geng and indica/xian subspecies, respectively, yield traits of their F1 and many RILs showed obvious over-parent dominance. To resolve yield-related genes, we here conducted trait surveys and linkage analysis of KGW based on the previously constructed high-density genetic map (Kong et al., 2022a).
KGW of RILs were investigated in Lingshui, Hannan, or Ezhou among 2017 – 2019. There was extensive variance of KGW in RIL population while there was a minor difference of KGW between the parents (Figure 2 and Supplementary Table 9). KGW of the RIL population showed a normal distribution with high Pearson coefficients in four different environments and transgressive segregations were observed in the RIL population (Figure 2), which indicated that KGW were controlled by multiple genes and indica x japonica hybrid breeding strategy can breed high-yielding rice materials.
We totally identified eight KGW QTLs on Chr 2, Chr 3, Chr5, Chr6, Chr8, and Chr10 (Table 2). Of QTLs, qKGW-8.1 was repeatedly detected in 2017EZ, 2018EZ, and 2019LS. qKGW-3.1 and qKGW-3.2 had almost the same interval and qKGW-5.2 was fully contained by qKGW-5.1. These results suggested that these three QTLs had relatively stable effects on KGW in multiple different environments. The remaining QTLs (qKGW-2.1, qKGW-6.1, and qKGW-10.1) were only detected in one specific ecological environment, and were possibly environment-specific KGW QTLs.
Identification and function confirmation of two novel KGW QTLs
To distinguish the new TWG QTLs first discovered in this study, 34 Meta-QTLs from 339 original GW QTLs (Supplementary Table 10) and 126 known GW genes (Supplementary Table 11) were collected (Khahani et al., 2020). Except for qKGW-6.1 and qKGW-8.1, the remaining QTLs all had complete or partial overlap with Meta-QTLs, or contained the known KGW genes in these intervals (Figure 3). For example, qKGW-3.1 and qKGW-3.2 fully covered MQTL-GW18 and included pls2 and SRL2. Sequence alignment result showed that SRL2 had sequence differences in the coding sequence regions (Supplementary Table 12). Similarly, qKGW-2.1 containing OsVPE3, OsMADS6, and OsGRF4, overlapped MQTL-GW7 and MQTL-GW8; qKGW-5.1 and qKGW-5.2 carrying OsPPKL2, SRS3, and GS5 overlapped MQTL-GW18; qKGW-10.1 including FLO7 overlapped MQTL-GW29.
Therefore, qKGW-6.1 and qKGW-8.1 were accepted as novel KGW QTLs. To further confirm the KGW regulation function of qKGW-6.1 and qKGW-8.1, all RILs were divided into different allelic combination based on peak maker genotyping results in genetic map. RPY geng allele (AA) RILs of qKGW-6.1 showed greater KGW than Luohui 9 (BB) RILs (Figure 4A). Interestingly, qKGW-8.1 showed the opposite result relative to qKGW-6.1 (Figure 4B). This suggested that the favorable alleles of these two QTLs are derived from RPY geng and Luohui 9, respectively, and the favorable allele aggregation may enhance KGW of some RILs. As expected, RILs that aggregated favorable alleles (qKGW-6.1 AA + qKGW-8.1 BB) had the largest KGW in all tested environments (Figures 4C–F). These results demonstrated that qKGW-6.1 and qKGW-8.1 are two new KGW loci that can be used to improve rice yield.
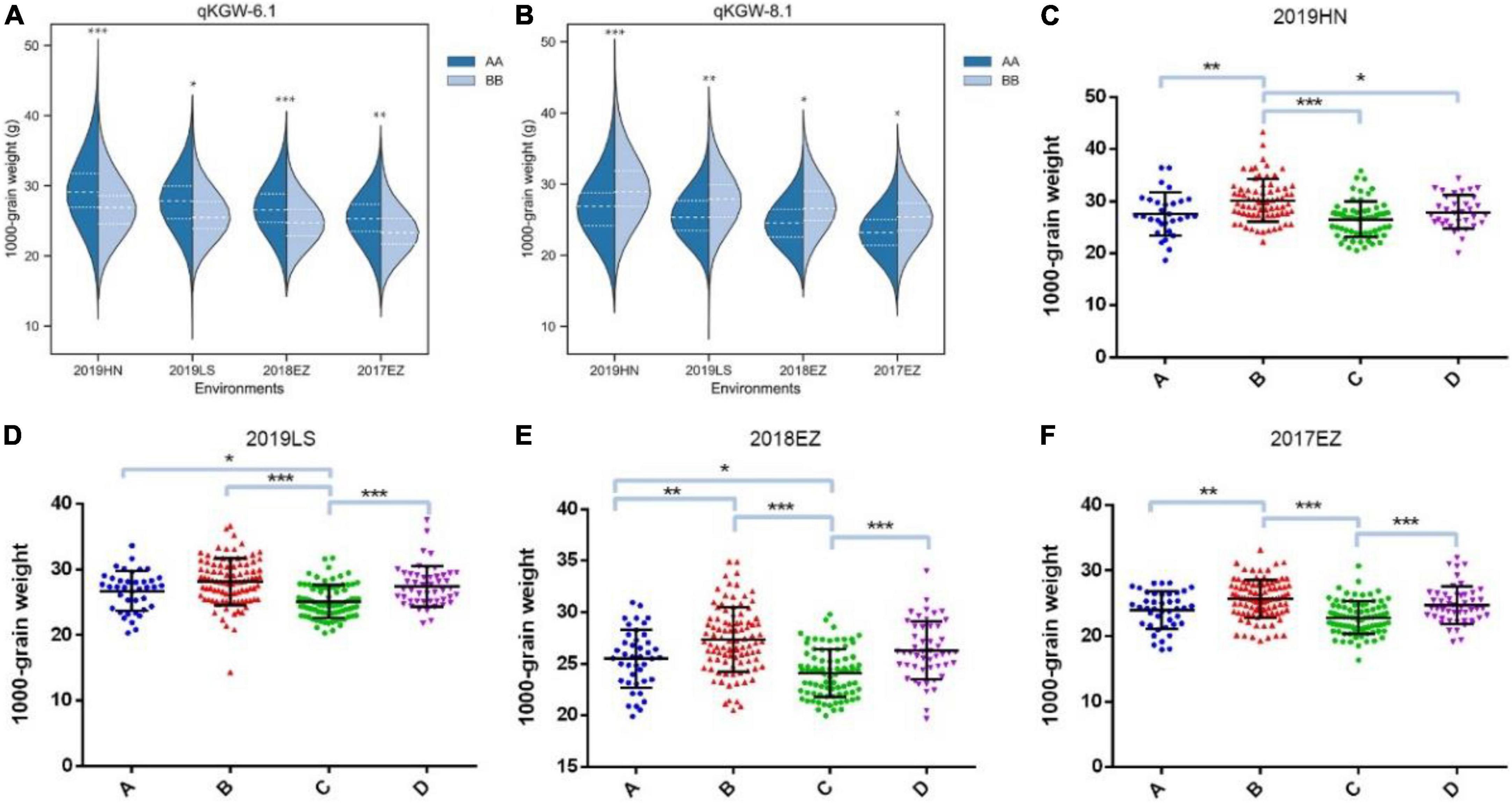
Figure 4. 1000-grain weight of different allele combinations of qKGW-6.1 and qKGW-8.1. In (A), (C–F): qKGW-6.1 AA + qKGW-8.1 AA, (B): qKGW-6.1 AA + qKGW-8.1 BB; (C): qKGW-6.1 BB + qKGW-8.1 AA; (D): qKGW-6.1 BB + qKGW-8.1 AA.
Ghd8 has a potential function in regulating rice grain weight
qKGW-8.1 could be detected repeatedly in three environments with phenotypic interpretation rates of 5.11–9.96. However, no known genes directly related to grain weight were found in qKGW-8.1. We therefore traversed the 92 gene annotation results in qKGW-8.1 and tried to correspond them to the phenotypic differences between the parents. We found that Ghd8 is located within the qKGW-8.1 interval, a gene reported to be closely associated with heading date and yield (Yan W. H. et al., 2011; Dai et al., 2012), which is consistent with parental heading date differences. Whether in Hainan or Hubei, both parents maintained the heading date difference of more than 10 days. We extracted the Ghd8 protein sequences from our newly assembled genome and sequence alignment revealed multiple sequence variations, including seven amino acid substitutions, one amino acid deletion, and a complex C-terminal amino acid variation (Figure 5A).
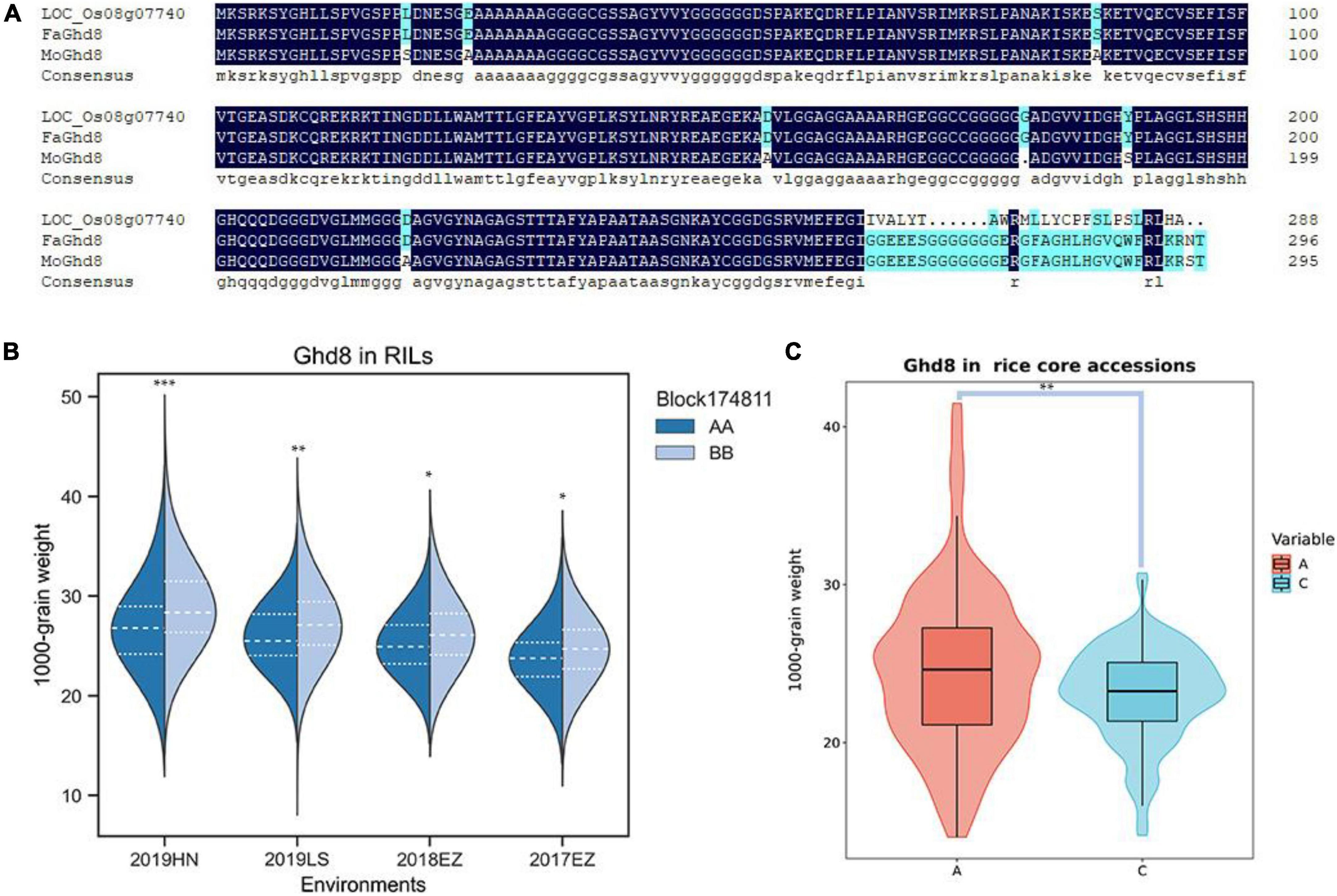
Figure 5. The candidate gene prediction of qKGW-8.1. (A). Protein sequence alignment results of Nipponbare, RPYgeng (FaGhd8), Luohui 9 (MoGhd8). (B) The 1,000-grain weight of RPY geng (AA) and Luohui 9 (BB) allele recombinant inbred lines (RILs). (C) The 1,000-grain weight of different allele rice core accessions.
To test whether Ghd8 has a potential effect on grain weight as a pleiotropic gene, we observed grain weight at different alleles in our RILs and in 532 rice core accessions from RiceVarMap v2.03 (Zhao et al., 2021). In RILs, the allele types of Ghd8 was determined based on a bin maker (Block174811) because Ghd8 is the only gene within block174811. In 532 rice core accessions, a functional snp (vg0804334484) was found in Ghd8 gene, containing A, C, and N alleles, and the N allele was eliminated in further phenotypic comparisons due to uncertainty about its base type. We found that different allele RILs or core accessions displayed significantly different KGW, suggesting that Ghd8 may play a role in KGW regulation (Figures 5B,C).
KGW heterosis loci
We totally identified two QTLs for KGW BPH, two QTLs for KGW MPH, and four QTLs for KGW LPH (Figure 6 and Supplementary Table 13). Three of the eight heterosis-related QTLs overlapped KGW QTLs: qLH-KGW-5.1 and qLH-KGW-5.2 were covered by qKGW-5.1 and qLH-KGW-8.1 overlapped with qKGW-8.1. Interestingly, qLH-KGW-8.1 coincided with a reported yield heterosis locus, RH8 (rice heterosis 8) (Li et al., 2016). Ghd8, as a major gene in RH8, was also located in the qLH-KGW-8.1 interval. This suggested that Ghd8 plays an important role in rice yield heterosis. In addition, two GW-related genes were in heterosis-related QTLs, namely, SRS3 and GS5 in qLH-KGW-5.1, GS5 in qLH-KGW-5.2. Whether these GW-related genes play a role in heterosis remains to be further explored.
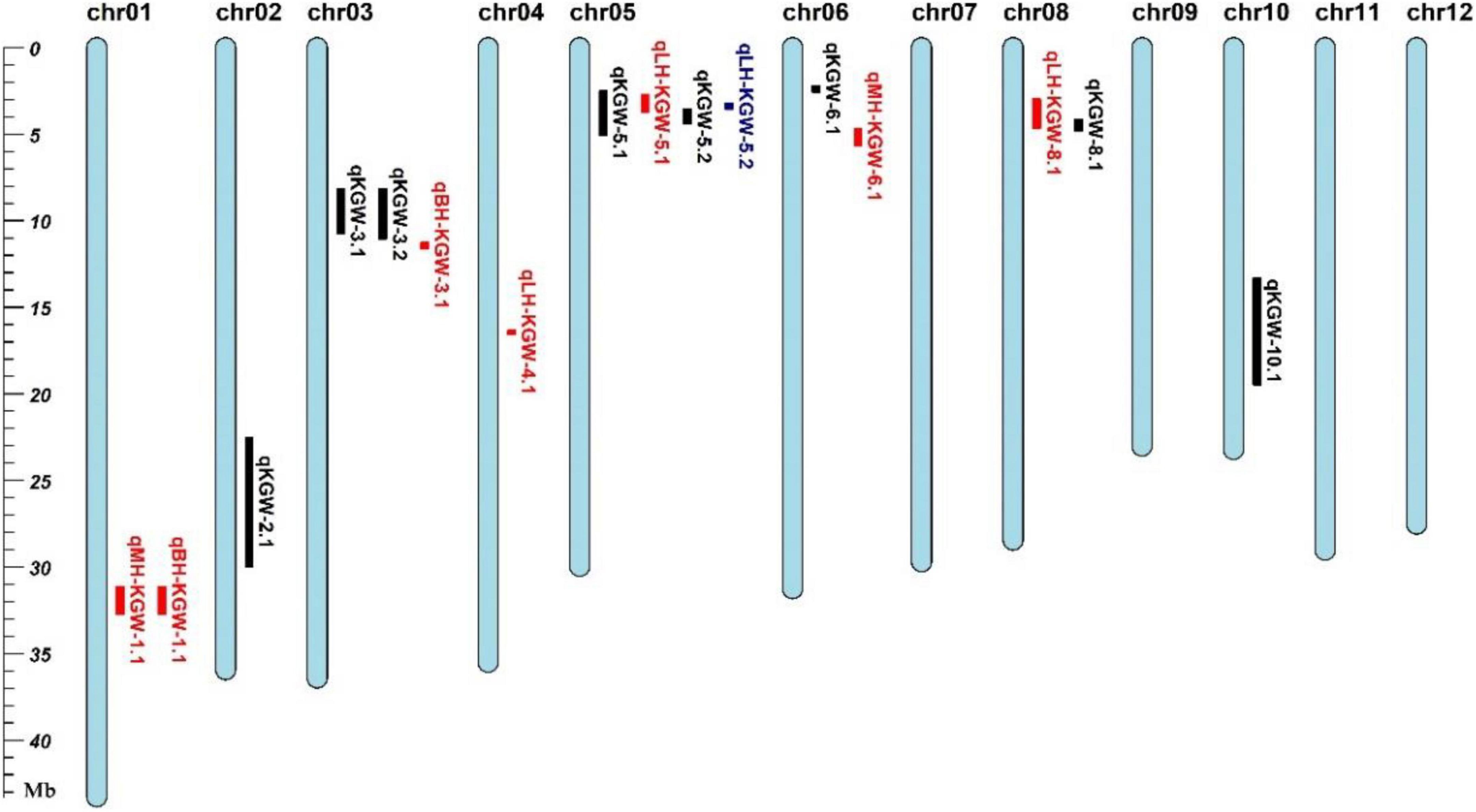
Figure 6. Grain weight heterosis loci in two testcross populations. Black represents QTL loci in recombinant inbred lines (RILs). Red and blue loci represent heterosis-related QTLs of YTA and Z7A testcross population, respectively.
Conclusion
In the present study, we de novo assembled genomes of an indica rice (Luohui 9) and a japonica rice (RPY geng) at the chromosome level and analyzed the KGW trait of their derived RIL populations. We concluded that the substantial genetic diversity of KGW in RILs were closely related to genome variations and allele aggregation difference of KGW QTLs. Importantly, we identified two novel KGW-related QTLs (qKGW-6.1 and qKGW-8.1) and several KGW heterosis loci in three derived population. Based on the genotyping results in RILs and 532 rice core accessions, Ghd8 in qKGW-8.1 was presumed to play an important role in GW regulation.
Data availability statement
The datasets presented in this study can be found in online repositories. The names of the repository/repositories and accession number(s) can be found below: ngdc, PRJCA010706.
Author contributions
YL and WK conceived and designed the experiments. WK performed genome assembly, analyzed the data, prepared the figures and tables, and wrote the manuscript. XD conducted a field survey of agronomic traits, QTL mapping, and allele genotyping in RILs and rice core accessions. ZW participated in the construction of the recombinant inbred lines and revision of the manuscript. YW provided help in genome annotation. ZL performed repetitive sequence annotation of RPY geng and Luohui 9 genomes. MZ collected Meta-QTLs and known genes performed parts of figures and tables. All authors read and approved the final version of the manuscript.
Funding
This work was supported by the National Key Research and Development Program of China (2016YFD0100400), the National Special Key Project for Transgenic Breeding (Grant No. 2016ZX08001001), and the National Natural Science Foundation of China (Grant No. 31760380).
Acknowledgments
We thank Professor Qian Qian (State Key Laboratory of Rice Biology, China National Rice Research Institute, Hangzhou, Zhejiang, China) for giving us RPY geng materials.
Conflict of interest
The authors declare that the research was conducted in the absence of any commercial or financial relationships that could be construed as a potential conflict of interest.
Publisher’s note
All claims expressed in this article are solely those of the authors and do not necessarily represent those of their affiliated organizations, or those of the publisher, the editors and the reviewers. Any product that may be evaluated in this article, or claim that may be made by its manufacturer, is not guaranteed or endorsed by the publisher.
Supplementary material
The Supplementary Material for this article can be found online at: https://www.frontiersin.org/articles/10.3389/fpls.2022.995634/full#supplementary-material
Supplementary Table 1 | The statistic of sequencing data.
Supplementary Table 2 | Repeat sequence statistics of Luohui 9 and RPY geng.
Supplementary Table 3 | Function annotations of RPY geng genes.
Supplementary Table 4 | Function annotations of Luohui 9 genes.
Supplementary Table 5 | GO annotation of RPY geng / Luohui 9 unique orthologous clusters.
Supplementary Table 6 | SV-related genes in Luohui 9 and RPY geng.
Supplementary Table 7 | A 283 important known genes for important agronomic traits.
Supplementary Table 8 | Homologous SV-related genes of 138 known functional genes.
Supplementary Table 9 | The KGW of the RIL population, RPY geng, and Luohui 9.
Supplementary Table 10 | The Meta-QTLs of KGW.
Supplementary Table 11 | The known genes of KGW.
Supplementary Table 12 | The known genes in KGW QTLs.
Supplementary Table 13 | The heterosis-related QTLs of KGW.
Footnotes
References
Alioto, T., Blanco, E., Parra, G., and Guigó, R. (2018). Using geneid to identify genes. Curr. Protoc. Bioinform. 64:e56.
Arcade, A., Labourdette, A., Falque, M., Mangin, B., Chardon, F., Charcosset, A., et al. (2004). BioMercator: Integrating genetic maps and QTL towards discovery of candidate genes. Bioinformatics 20, 2324–2326. doi: 10.1093/bioinformatics/bth230
Arends, D., Prins, P., Jansen, R. C., and Broman, K. W. (2010). R/qtl: High-throughput multiple QTL mapping. Bioinformatics 26, 2990–2992.
Birchler, J. A., Yao, H., Chudalayandi, S., Vaiman, D., and Veitia, R. A. (2010). Heterosis. Plant Cell 22, 2105–2112.
Burge, C., and Karlin, S. (1997). Prediction of complete gene structures in human genomic DNA. J. Mol. Biol. 268, 78–94.
Burton, J. N., Adey, A., Patwardhan, R. P., Qiu, R. L., Kitzman, J. O., and Shendure, J. (2013). Chromosome-scale scaffolding of de novo genome assemblies based on chromatin interactions. Nat. Biotechnol. 31:1119.
Campbell, M. A., Haas, B. J., Hamilton, J. P., Mount, S. M., and Buell, C. R. (2006). Comprehensive analysis of alternative splicing in rice and comparative analyses with Arabidopsis. BMC Genomics 7:327. doi: 10.1186/1471-2164-7-327
Chan, A. N., Wang, L. L., Zhu, Y. J., Fan, Y. Y., Zhuang, J. Y., and Zhang, Z. H. (2021). Identification through fine mapping and verification using CRISPR/Cas9-targeted mutagenesis for a minor QTL controlling grain weight in rice. Theor. Appl. Genet. 134, 327–337. doi: 10.1007/s00122-020-03699-6
Dai, X., Ding, Y., Tan, L., Fu, Y., Liu, F., Zhu, Z., et al. (2012). LHD1, an allele of DTH8/Ghd8, controls late heading date in common wild rice (Oryza rufipogon). J. Integr. Plant Biol. 54, 790–799. doi: 10.1111/j.1744-7909.2012.01166.x
Deng, X., Kong, W., Sun, T., Zhang, C., Zhong, H., Zhao, G., et al. (2022). Bin mapping-based QTL analyses using three genetic populations derived from indica-japonica crosses uncover multiple grain shape heterosis-related loci in rice. Plant Genome 15:e20171. doi: 10.1002/tpg2.20171
Du, H. L., Yu, Y., Ma, Y. F., Gao, Q., Cao, Y. H., Chen, Z., et al. (2017). Sequencing and de novo assembly of a near complete Indica rice genome. Nat. Commun. 8:12. doi: 10.1038/ncomms15324
Dupuis, J., and Siegmund, D. (1999). Statistical methods for mapping quantitative trait loci from a dense set of markers. Genetics 151, 373–386.
Goel, M., Sun, H. Q., Jiao, W. B., and Schneeberger, K. (2019). SyRI: Finding genomic rearrangements and local sequence differences from whole-genome assemblies. Genome Biol. 20:277. doi: 10.1186/s13059-019-1911-0
Goff, S. A., Ricke, D., Lan, T. H., Presting, G., Wang, R. L., Dunn, M., et al. (2002). A draft sequence of the rice genome (Oryza sativa L. ssp japonica). Science 296, 92–100.
Haas, B. J., Salzberg, S. L., Zhu, W., Pertea, M., Allen, J. E., Orvis, J., et al. (2008). Automated eukaryotic gene structure annotation using EVidenceModeler and the program to assemble spliced alignments. Genome Biol. 9:22. doi: 10.1186/gb-2008-9-1-r7
Huang, K., Wang, D., Duan, P., Zhang, B., Xu, R., Li, N., et al. (2017). WIDE AND THICK GRAIN 1, which encodes an otubain-like protease with deubiquitination activity, influences grain size and shape in rice. Plant J. 5, 849–860. doi: 10.1111/tpj.13613
Ishimaru, K., Hirotsu, N., Madoka, Y., Murakami, N., Hara, N., Onodera, H., et al. (2013). Loss of function of the IAA-glucose hydrolase gene TGW6 enhances rice grain weight and increases yield. Nat. Genet. 45, 707–711. doi: 10.1038/ng.2612
Keilwagen, J., Wenk, M., Erickson, J. L., Schattat, M. H., Grau, J., and Hartung, F. (2016). Using intron position conservation for homology-based gene prediction. Nucleic Acids Res. 44:11.
Khahani, B., Tavakol, E., Shariati, V., and Fornara, F. (2020). Genome wide screening and comparative genome analysis for Meta-QTLs, ortho-MQTLs and candidate genes controlling yield and yield-related traits in rice. BMC Genomics 21, 294–318. doi: 10.1186/s12864-020-6702-1
Kong, W. L., Zhang, C. H., Qiang, Y. L., Zhong, H., Zhao, G. Q., and Li, Y. S. (2020). Integrated RNA-seq analysis and Meta-QTLs mapping provide insights into cold stress response in rice seedling roots. Int. J. Mol. Sci. 21:14. doi: 10.3390/ijms21134615
Kong, W., Deng, X., Yang, J., Zhang, C., Sun, T., Ji, W., et al. (2022a). High-resolution bin-based linkage mapping uncovers the genetic architecture and heterosis-related loci of plant height in Indica-japonica derived populations. Plant J. 110, 814–827. doi: 10.1111/tpj.15705
Kong, W., Li, S., Zhang, C., Qiang, Y., and Li, Y. (2022b). Combination of quantitative trait locus (QTL) mapping and transcriptome analysis reveals submerged germination QTLs and candidate genes controlling coleoptile length in rice. Food Energy Security 11:e354.
Kong, W., Sun, T., Zhang, C., Deng, X., and Li, Y. (2021a). Comparative Transcriptome analysis reveals the mechanisms underlying differences in salt tolerance between Indica and japonica rice at seedling stage. Front. Plant Sci. 12:725436. doi: 10.3389/fpls.2021.725436
Kong, W., Zhang, C., Zhang, S., Qiang, Y., Zhang, Y., Zhong, H., et al. (2021b). Uncovering the novel qtls and candidate genes of salt tolerance in rice with linkage mapping, RTM-Gwas, and RNA-seq. Rice 14:93. doi: 10.1186/s12284-021-00535-3
Koren, S., Walenz, B. P., Berlin, K., Miller, J. R., Bergman, N. H., and Phillippy, A. M. (2017). Canu: Scalable and accurate long-read assembly via adaptive k-mer weighting and repeat separation. Genome Res. 27, 722–736. doi: 10.1101/gr.215087.116
Li, D., Huang, Z., Song, S., Xin, Y., Mao, D., Lv, Q., et al. (2016). Integrated analysis of phenome, genome, and transcriptome of hybrid rice uncovered multiple heterosis-related loci for yield increase. Proc. Natl. Acad. Sci. U.S.A. 113, E6026–E6035. doi: 10.1073/pnas.1610115113
Li, F., Gao, Y., Wu, B., Cai, Q., and Wang, S. (2021). High-quality de novo genome assembly of Huajingxian 74, a receptor parent of single segment substitution lines. Rice Sci. 28, 109–113.
Li, H., and Durbin, R. (2009). Fast and accurate short read alignment with Burrows-Wheeler transform. Bioinformatics 25, 1754–1760.
Li, X. K., Wu, L., Wang, J. H., Sun, J., Xia, X. H., Geng, X., et al. (2018). Genome sequencing of rice subspecies and genetic analysis of recombinant lines reveals regional yield- and quality-associated loci. BMC Biol. 16:12. doi: 10.1186/s12915-018-0572-x
Liu, Q., Han, R., Wu, K., Zhang, J., Ye, Y., Wang, S., et al. (2018). G-protein βγ subunits determine grain size through interaction with MADS-domain transcription factors in rice. Nat. Commun. 9:852. doi: 10.1038/s41467-018-03047-9
Majoros, W., Pertea, M., and Salzberg, S. (2004). TigrScan and GlimmerHMM: Two open source ab initio eukaryotic gene-finders. Bioinformatics 20, 2878–2879. doi: 10.1093/bioinformatics/bth315
Martinez, A. K., Soriano, J. M., Tuberosa, R., Koumproglou, R., Jahrmann, T., and Salvi, S. (2016). Yield QTLome distribution correlates with gene density in maize. Plant Sci. 242, 300–309. doi: 10.1016/j.plantsci.2015.09.022
Ou, S. J., Su, W. J., Liao, Y., Chougule, K., Agda, J. R. A., Hellinga, A. J., et al. (2019). Benchmarking transposable element annotation methods for creation of a streamlined, comprehensive pipeline. Genome Biol. 20:275.
Panibe, J. P., Wang, L., Li, J., Li, M. Y., Lee, Y. C., Wang, C. S., et al. (2021). Chromosomal-level genome assembly of the semi-dwarf rice Taichung Native 1, an initiator of Green Revolution. Genomics 113, 2656–2674. doi: 10.1016/j.ygeno.2021.06.006
Pertea, M., Kim, D., Pertea, G. M., Leek, J. T., and Salzberg, S. L. (2016). Transcript-level expression analysis of RNA-seq experiments with HISAT, StringTie and Ballgown. Nat. Protoc. 11, 1650–1667. doi: 10.1038/nprot.2016.095
Qin, P., Lu, H., Du, H., Wang, H., Chen, W., Chen, Z., et al. (2021). Pan-genome analysis of 33 genetically diverse rice accessions reveals hidden genomic variations. Cell 184, 3542–3558.e16. doi: 10.1016/j.cell.2021.04.046
Song, J. M., Xie, W. Z., Wang, S., Guo, Y. X., Koo, D. H., Kudrna, D., et al. (2021). Two gap-free reference genomes and a global view of the centromere architecture in rice. Mol. Plant 14, 1757–1767. doi: 10.1016/j.molp.2021.06.018
Song, X. J., Kuroha, T., Ayano, M., Furuta, T., Nagai, K., Komeda, N., et al. (2015). Rare allele of a previously unidentified histone H4 acetyltransferase enhances grain weight, yield, and plant biomass in rice. Proc. Natl. Acad. Sci. U.S.A. 112, 76–81. doi: 10.1073/pnas.1421127112
Stanke, M., and Waack, S. (2003). Gene prediction with a hidden Markov model and a new intron submodel. Bioinformatics 19, II215–II225. doi: 10.1093/bioinformatics/btg1080
Stein, J. C., Yu, Y., Copetti, D., Zwickl, D. J., Zhang, L., Zhang, C., et al. (2018). Genomes of 13 domesticated and wild rice relatives highlight genetic conservation, turnover and innovation across the genus Oryza. Nat. Genet. 50, 285–296. doi: 10.1038/s41588-018-0040-0
Tanaka, T., Nishijima, R., Teramoto, S., Kitomi, Y., Hayashi, T., Uga, Y., et al. (2020). De novo genome assembly of the Indica rice variety IR64 using linked-read sequencing and nanopore sequencing. G3 (Bethesda) 10, 1495–1501. doi: 10.1534/g3.119.400871
Tang, H. B., Zhang, X. T., Miao, C. Y., Zhang, J. S., Ming, R., Schnable, J. C., et al. (2015). ALLMAPS: Robust scaffold ordering based on multiple maps. Genome Biol. 16:15. doi: 10.1186/s13059-014-0573-1
Tang, S. Y. Y., Lomsadze, A., and Borodovsky, M. (2015). Identification of protein coding regions in RNA transcripts. Nucleic Acids Res. 43:10.
Walker, B. J., Abeel, T., Shea, T., Priest, M., Abouelliel, A., Sakthikumar, S., et al. (2014). Pilon: An integrated tool for comprehensive microbial variant detection and genome assembly improvement. PLoS One 9:14. doi: 10.1371/journal.pone.0112963
Wan, X. Y., Wan, J. M., Weng, J. F., Jiang, L., Bi, J. C., Wang, C. M., et al. (2005). Stability of QTLs for rice grain dimension and endosperm chalkiness characteristics across eight environments. Theor. Appl. Genet. 110, 1334–1346. doi: 10.1007/s00122-005-1976-x
Wang, Y., Xiong, G., Hu, J., Jiang, L., Yu, H., Xu, J., et al. (2015). Copy number variation at the GL7 locus contributes to grain size diversity in rice. Nat. Genet. 47, 944–948. doi: 10.1038/ng.3346
Xie, X., Du, H., Tang, H., Tang, J., Tan, X., Liu, W., et al. (2021). A chromosome-level genome assembly of the wild rice Oryza rufipogon facilitates tracing the origins of Asian cultivated rice. Sci. China Life Sci. 64, 282–293. doi: 10.1007/s11427-020-1738-x
Xu, L., Dong, Z. B., Fang, L., Luo, Y. J., Wei, Z. Y., Guo, H. L., et al. (2019). OrthoVenn2: A web server for whole-genome comparison and annotation of orthologous clusters across multiple species. Nucleic Acids Res. 47, W52–W58. doi: 10.1093/nar/gkz333
Xu, S. Z., Xu, Y., Gong, L., and Zhang, Q. F. (2016). Metabolomic prediction of yield in hybrid rice. Plant J. 88, 219–227.
Yan, S., Zou, G., Li, S., Wang, H., Liu, H., Zhai, G., et al. (2011). Seed size is determined by the combinations of the genes controlling different seed characteristics in rice. Theor. Appl. Genet. 123, 1173–1181.
Yan, W. H., Wang, P., Chen, H. X., Zhou, H. J., Li, Q. P., Wang, C. R., et al. (2011). A major QTL, Ghd8, plays pleiotropic roles in regulating grain productivity, plant height, and heading date in rice. Mol. Plant 4, 319–330. doi: 10.1093/mp/ssq070
Yu, J., Hu, S. N., Wang, J., Wong, G. K. S., Li, S. G., Liu, B., et al. (2002). A draft sequence of the rice genome (Oryza sativa L. ssp Indica). Science 296, 79–92.
Zhang, F., Xue, H., Dong, X., Li, M., Zheng, X., Li, Z., et al. (2022). Long-read sequencing of 111 rice genomes reveals significantly larger pan-genomes. Genome Res 32, 853–863. doi: 10.1101/gr.276015.121
Zhang, H., Wang, Y., Deng, C., Zhao, S., Zhang, P., Feng, J., et al. (2022). High-quality genome assembly of Huazhan and Tianfeng, the parents of an elite rice hybrid Tian-you-hua-zhan. Sci. China Life Sci. 65, 398–411. doi: 10.1007/s11427-020-1940-9
Zhang, J. W., Chen, L. L., Sun, S., Kudrna, D., Copetti, D., Li, W. M., et al. (2016a). Building two Indica rice reference genomes with PacBio long-read and Illumina paired-end sequencing data. Sci. Data 3:160076. doi: 10.1038/sdata.2016.76
Zhang, J. W., Chen, L. L., Xing, F., Kudrna, D. A., Yao, W., Copetti, D., et al. (2016b). Extensive sequence divergence between the reference genomes of two elite Indica rice varieties Zhenshan 97 and Minghui 63. Proc. Natl. Acad. Sci. U.S.A. 113, E5163–E5171. doi: 10.1073/pnas.1611012113
Zhang, X. C., Shabala, S., Koutoulis, A., Shabala, L., and Zhou, M. X. (2017). Meta-analysis of major QTL for abiotic stress tolerance in barley and implications for barley breeding. Planta 245, 283–295. doi: 10.1007/s00425-016-2605-4
Zhang, Y., Fu, J., Wang, K., Han, X., Yan, T., Su, Y., et al. (2022). The telomere-to-telomere gap-free genome of four rice parents reveals SV and PAV patterns in hybrid rice breeding. Plant Biotechnol. J. doi: 10.1111/pbi.13880
Zhao, H., Li, J. C., Yang, L., Qin, G., Xia, C. J., Xu, X. B., et al. (2021). An inferred functional impact map of genetic variants in rice. Mol. Plant 14, 1584–1599.
Zhao, Q., Feng, Q., Lu, H., Li, Y., Wang, A., Tian, Q., et al. (2018). Pan-genome analysis highlights the extent of genomic variation in cultivated and wild rice. Nat. Genet. 50, 278–284.
Keywords: rice, genome sequencing, yield improvement, 1,000-grain weight, heterosis loci
Citation: Kong W, Deng X, Liao Z, Wang Y, Zhou M, Wang Z and Li Y (2022) De novo assembly of two chromosome-level rice genomes and bin-based QTL mapping reveal genetic diversity of grain weight trait in rice. Front. Plant Sci. 13:995634. doi: 10.3389/fpls.2022.995634
Received: 16 July 2022; Accepted: 01 August 2022;
Published: 22 August 2022.
Edited by:
Weihua Pan, Agricultural Genomics Institute at Shenzhen (CAAS), ChinaReviewed by:
Jingping Fang, Fujian Normal University, ChinaLuomiao Yang, Northeast Agricultural University, China
Showkat Ganie, Royal Holloway, University of London, United Kingdom
Copyright © 2022 Kong, Deng, Liao, Wang, Zhou, Wang and Li. This is an open-access article distributed under the terms of the Creative Commons Attribution License (CC BY). The use, distribution or reproduction in other forums is permitted, provided the original author(s) and the copyright owner(s) are credited and that the original publication in this journal is cited, in accordance with accepted academic practice. No use, distribution or reproduction is permitted which does not comply with these terms.
*Correspondence: Zhaohai Wang, xzhaohai_wang@163.com; Yangsheng Li, lysh2001@whu.edu.cn
†These authors have contributed equally to this work