- 1Departamento de Ciencias Vegetales, Facultad de Agronomía e Ingeniería Forestal, Pontificia Universidad Católica de Chile, Santiago, Chile
- 2Department of Agricultural and Environmental Science, University of Bari Aldo Moro, Bari, Italy
- 3Department of Agricultural and Food Sciences, University of Bologna, Bologna, Italy
- 4Centro Regional Rayentue, Instituto de Investigaciones Agropecuarias (INIA), Rengo, Chile
- 5Centro Regional Quilamapu, Instituto de Investigaciones Agropecuarias (INIA), Chillán, Chile
Abiotic stress strongly affects yield-related traits in durum wheat, in particular drought is one of the main environmental factors that have effect on grain yield and plant architecture. In order to obtain new genotypes well adapted to stress conditions, the highest number of desirable traits needs to be combined in the same genotype. In this context, hundreds of quantitative trait loci (QTL) have been identified for yield-related traits in different genetic backgrounds and environments. Meta-QTL (MQTL) analysis is a useful approach to combine data sets and for creating consensus positions for the QTL detected in independent studies for the reliability of their location and effects. MQTL analysis is a useful method to dissect the genetic architecture of complex traits, which provide an extensive allelic coverage, a higher mapping resolution and allow the identification of putative molecular markers useful for marker-assisted selection (MAS). In the present study, a complete and comprehensive MQTL analysis was carried out to identify genomic regions associated with grain-yield related traits in durum wheat under different water regimes. A total of 724 QTL on all 14 chromosomes (genomes A and B) were collected for the 19 yield-related traits selected, of which 468 were reported under rainfed conditions, and 256 under irrigated conditions. Out of the 590 QTL projected on the consensus map, 421 were grouped into 76 MQTL associated with yield components under both irrigated and rainfed conditions, 12 genomic regions containing stable MQTL on all chromosomes except 1A, 4A, 5A, and 6B. Candidate genes associated to MQTL were identified and an in-silico expression analysis was carried out for 15 genes selected among those that were differentially expressed under drought. These results can be used to increase durum wheat grain yields under different water regimes and to obtain new genotypes adapted to climate change.
Introduction
Durum wheat (Triticum durum Desf.; 2n = 4 × = 28, AABB) is the 10th most important crop in the world with a cultivated area of 16 million ha and a production of 40 million ton in 2017 (Dahl, 2017). In addition, durum wheat is the most important cereal in the Mediterranean regions since it is deeply connected with the history and culinary tradition in those areas (Martínez-Moreno et al., 2020). This cereal plays a key role in human diet because it is primarily used for making pasta and other semolina-based products, such as frike, couscous, bourghul, and unleavened breads, which are widely consumed in many countries of the world (Sharma et al., 2019). The main producers of durum wheat in the world are Spain, France, Italy, and Greece in southern Europe, Morocco, Algeria, and Tunisia in northern Africa, Turkey, and Syria in southwest Asia, and Canada, United States, and Mexico in North America (Martínez-Moreno et al., 2020), and Argentina and Chile in South America (Colasuonno et al., 2019). Durum wheat is commonly grown in arid and semi-arid regions under rainfed conditions, where the precipitations are scarce and irregular across years (Ayed et al., 2021). The water scarcity combined with high temperatures during grain filling period significantly affects the quality and the yields of durum wheat, causing grain yield losses of up to 50% to farmers (Dettori et al., 2017; Soriano et al., 2021).
According to FAO projections, agricultural production requires to increase about 50% by 2,050 to meet the global rising demand for food (Food and Agriculture Organization of the United Nations [FAO], 2018). In this context, the development of new durum wheat high-yielding cultivars and tolerant to abiotic stresses is highly necessary. Therefore, gaining insight into the genetic basis of the grain yield and their responses to drought stress is an important pre-requisite for improvement of durum wheat genotypes, and plant breeders should look for stable loci to improve yields (Arriagada et al., 2020). The identification of quantitative trait loci (QTL) associated with molecular markers is essential for understanding the genetic basis of important traits, and an effective method for improving selection efficiency in breeding programs (Soriano et al., 2021). Hundreds of QTLs, using both linkage analysis and genome-wide association studies (GWAS), have been mapped into the durum wheat genome, which have been summarized in previous works considering grain quality (Colasuonno et al., 2019; Maccaferri et al., 2019; Marcotuli et al., 2020), and grain yield traits (Maccaferri et al., 2019; Arriagada et al., 2020). Despite these considerable advances in the dissection of the genetic basis for different traits related to quality and yield, only a very small fraction of these QTLs and the associated markers have been utilized in breeding programs (Cobb et al., 2019), due that most of those QTLs have minor effects and their expression is highly affected by the environment, the genetic background and their interactions (Zheng et al., 2021).
Meta-QTL (MQTL) analysis is a powerful tool to facilitate and improve the accuracy of QTL detection which is an important pre-requisite to prioritize and better define the loci and associated molecular markers valuable for marker-assisted selection (MAS). MQTL analysis combines data sets and creates consensus positions for the QTL detected in independent studies for the reliability of their location and effects across different genetic backgrounds and environments (Goffinet and Gerber, 2000; Veyrieras et al., 2007). This method also allows to identify QTL that have pleotropic effects by determining regions of the genome (MQTL) that contain QTLs for different traits (Said et al., 2013). The identification of MQTL has proven to be an effective tool for use in MAS because the MQTL generally have reduced confidence intervals (CIs) and improved phenotypic variation explained. In addition, the MQTL are useful for the identification of promising candidate genes associated with the target traits (Colasuonno et al., 2021; Saini et al., 2021).
In common wheat (Triticum aestivum L.), several studies have performed MQTL analysis for root-related traits (Soriano and Alvaro, 2019), adaptation to drought and heat stress (Acuña-Galindo et al., 2015; Kumar et al., 2020), resistance against Fusarium head blight (Liu et al., 2009), grain size and shape (Gegas et al., 2010). In contrast, only two studies have performed MQTL analysis in durum wheat. Recently, Soriano et al. (2021) performed a complete analysis to identified MQTL for quality traits, and tolerance to abiotic and biotic stresses. Previously, Soriano et al. (2017) identified MQTL for phenology, biomass and some yield traits including works from 2008 to 2015. Considering this previous background for purposes of genetic improvement of grain yield, the most appropriate approach is through the simultaneous selection based on grain-yield related traits (Ramazani and Abdipour, 2019). Grain yield is a complex trait governed by hundreds or thousands of loci. Based on this complexity, the genetic dissection of the grain yield inheritance into grain yield components of lower genetic complexity greatly facilitates the identification of the QTL and therefore the MAS efficiency. The aim of the present study was to perform a complete and comprehensive MQTL analysis for grain-yield related traits in durum wheat using articles published in the last 20 years, in order to identify regions of the genome that are useful for durum wheat breeding programs, in which the objective is to increase grain yields of the crop cultivated under different water regimes.
Materials and methods
Quantitative trait loci collection and consensus genetic map
An exhaustive literature review was conducted to find studies reporting QTL for grain-yield related traits in durum wheat grown under different water regimes. The QTL identified in each study were classified as follows: (1) QTL under rainfed conditions which correspond to QTL identified under rainfed, water-limited, and drought conditions, and (2) QTL under irrigated conditions that correspond to QTL identified under well-water, optimal, and irrigated conditions as described by the authors in each study. The country, location of each trial, rainfall and type of classification of the QTL is summarized in Supplementary Table 1. A total of 19 traits associated with the two main yield components (grain weight and grains number per unit area) were selected (Table 1). For the MQTL analysis, only studies that showed the following information were considered: (1) type and size of the mapping population, (2) position of QTL (peak position and/or confidence intervals), (3) LOD (logarithm of the odds) score for each QTL, (4) percentage of phenotypic variance explained for each QTL (PVE or r2). QTL that did not meet these criteria were discarded. Each QTL was treated as an independent QTL, even if some were detected in multiple environments or genetic backgrounds. If the confidence interval (CI, 95%) for the QTL was not reported, it was calculated using the following formulas (Guo et al., 2006):
for back cross (BC) and F2 lines
for double haploid (DH) lines
for Recombinant inbred lines (RIL)
where N is the population size and R2 is the proportion of phenotypic variance of the QTL.
The durum wheat consensus map developed by Maccaferri et al. (2015) was used for QTL projection. The map consisted of 30,144 markers, spanning 2,631 cM, and a density marker of 11 markers per cM.
Projection of quantitative trait loci and meta-QTL analysis
To project the QTL positions detected in the different studies, the original QTL data were projected onto the consensus map following the homothetic approach described by Chardon et al. (2004). The MQTL analysis was conducted with the projected QTL on the consensus map using the software BioMercator V4.2 (Arcade et al., 2004). Two different approaches were performed to MQTL analysis according to the number of QTL per chromosome. When the number of QTL per chromosome was 10 or lower, the approach of Goffinet and Gerber (2000) was carried out. Alternatively, the approach of Veyrieras et al. (2007) was performed when the number of QTL per chromosome was higher than 10. In this case, the best MQTL model was chosen according to Akaike Information Criterion (AIC), corrected Akaike Information Criterion (AICc) and modified AIC with factor 3 (AIC3), Bayesian Information Criterion (BIC) and Average Weight of Evidence (AWE) criteria. The best QTL model was selected when values of the model selection criteria were the lowest in at least three of the five models (Soriano and Alvaro, 2019).
AIC = Akaike information criterion; AICc = corrected Akaike’s information criterion; AIC3 = A variant of AIC that uses 3p as the penalty term.
Identification of candidate genes
QTL involved in grain-yield related traits in durum wheat grown under different water regimes were projected onto the durum wheat consensus map (Maccaferri et al., 2015) for further comparisons. Gene annotations for the most important marker-trait associations (MTAs) was performed using the high-confidence genes reported for the wheat genome sequence (Svevo browser), available at https://wheat.pw.usda.gov/GG3/jbrowse_Durum_Svevo. The marker locations were defined by flanking marker positions and CI of the MQTL. Gene model regulations were obtained through in-silico expression analysis, using the RNAseq data,1 filtered for drought and drought combined with heat stress experiments, with the following identification of the up-regulated genes. Primarily, gene models were identified by the “Chinese spring” annotated sequences and subsequently the homologous genes from “Svevo.” Gene models involved in drought stress during plant development and spike drought during early booting were analyzed using the in-silico expression data using database (see text footnote 1) within the markers flanking the MQTL.
Results
Quantitative trait loci for yield-related traits under different water regimes
A total of 25 studies identifying QTL for yield components published from 2003 to 2021 based on biparental populations were reviewed in Table 2. The studies comprise 26 different populations with 45 parental lines. A total of 724 QTL distributed throughout all 14 chromosomes (genomes A and B) were collected for the 19 yield-related traits selected. Four hundred sixty-eight QTL were reported under rainfed conditions, and 256 QTL under irrigated conditions (Supplementary Table 2). In general, the number of QTL per chromosome ranged from 21 on chromosome 6A to 76 on chromosomes 2A, with an average of 51 QTL per chromosome (Figure 1A). According to the main yield components, the 53.72% of the QTL (389) were reported for traits related to grains number per area, and the 46.27% of the QTL (335) for grain weight. Specifically, the trait with the highest number of reported QTL was 1,000-grain weight (TGW; 204), whereas the trait with the least reported QTL was grain weight per spike (GWPS; 9), whose both traits are associated with the grain weight (Figure 1B). Confidence intervals (CI) ranged from 0 to 145 cM, with an average of 24.3 cM (Figure 1C). The 19.19% of the QTL had a CI lower than 10 cM, and about half (48.20%) had a CI lower than 20 cM. The proportion of phenotypic variance explained (PVE) for each QTL ranged from 0.007 to 0.83, with an average of 0.138 (Figure 1D). Most of the QTL (608) had a PVE lower than 0.20.
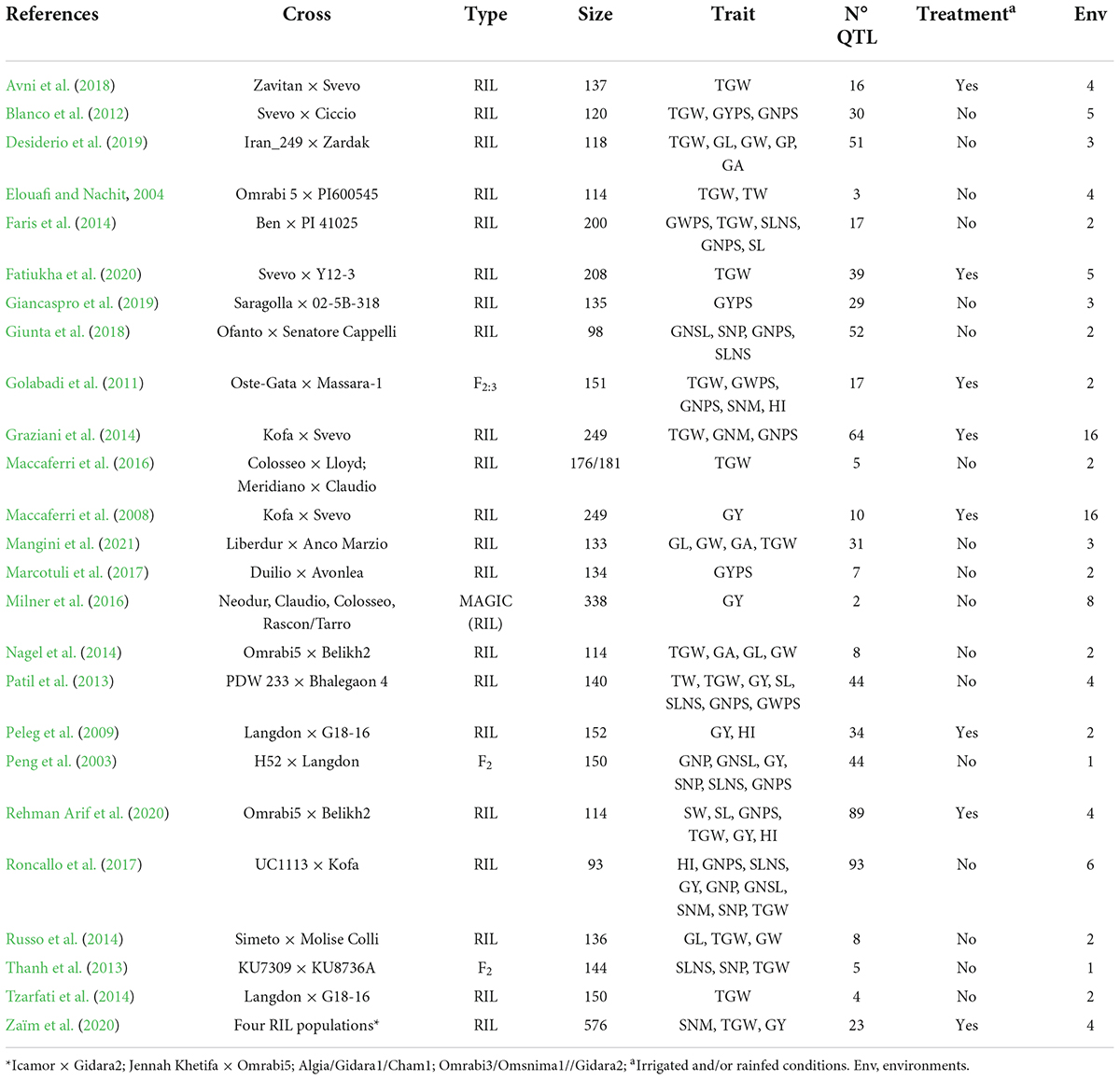
Table 2. Summary of the QTL studies reviewed including reference, mapping populations, type of population, size, traits, number of QTL collected, water regime, and the number of environments.
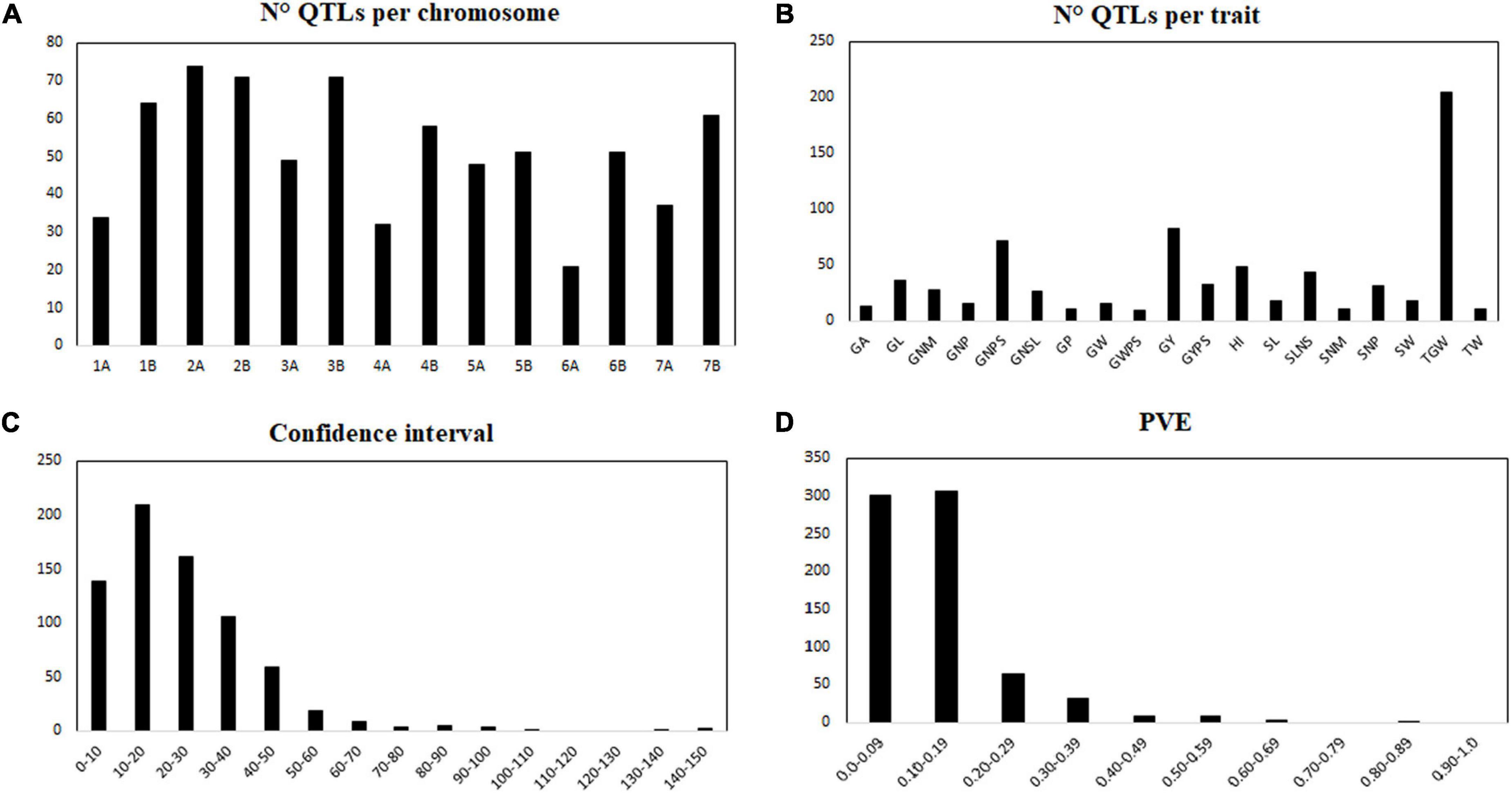
Figure 1. Description of the 724 collected QTLs. Number of QTL per chromosome (A), number of QTL per trait (B), confidence interval (C), phenotypic variance explained (PVE) for each QTL (D). GA, grain area; GL, grain length; GNM, grain number per m2; GNP, grain number per plant; GNPS, grain number per spike; GNSL, grain number per spikelet; GP, grain perimeter; GW, grain width; GWPS, grain weight per spike; GY, grain yield; GYPS, grain yield per spike; HI, harvest index; SL, spike length; SLNS, spikelet number per spike; SNM, spike number per m2; SNP, spike number per plant; SW, spike width; TGW, thousand grain weight; TW, test weight.
Quantitative trait loci projection on the consensus map
A total of 590 out of the 724 collected QTLs were projected on the consensus genetic map (Figure 2). One hundred ninety-six QTLs were projected under irrigated conditions, and 394 QTL under rainfed conditions (Supplementary Table 3). The remaining QTLs (134) were not projected because they lacked common markers between the original and the consensus maps, and/or the QTL showed low PVE causing a large CI (Soriano and Alvaro, 2019).
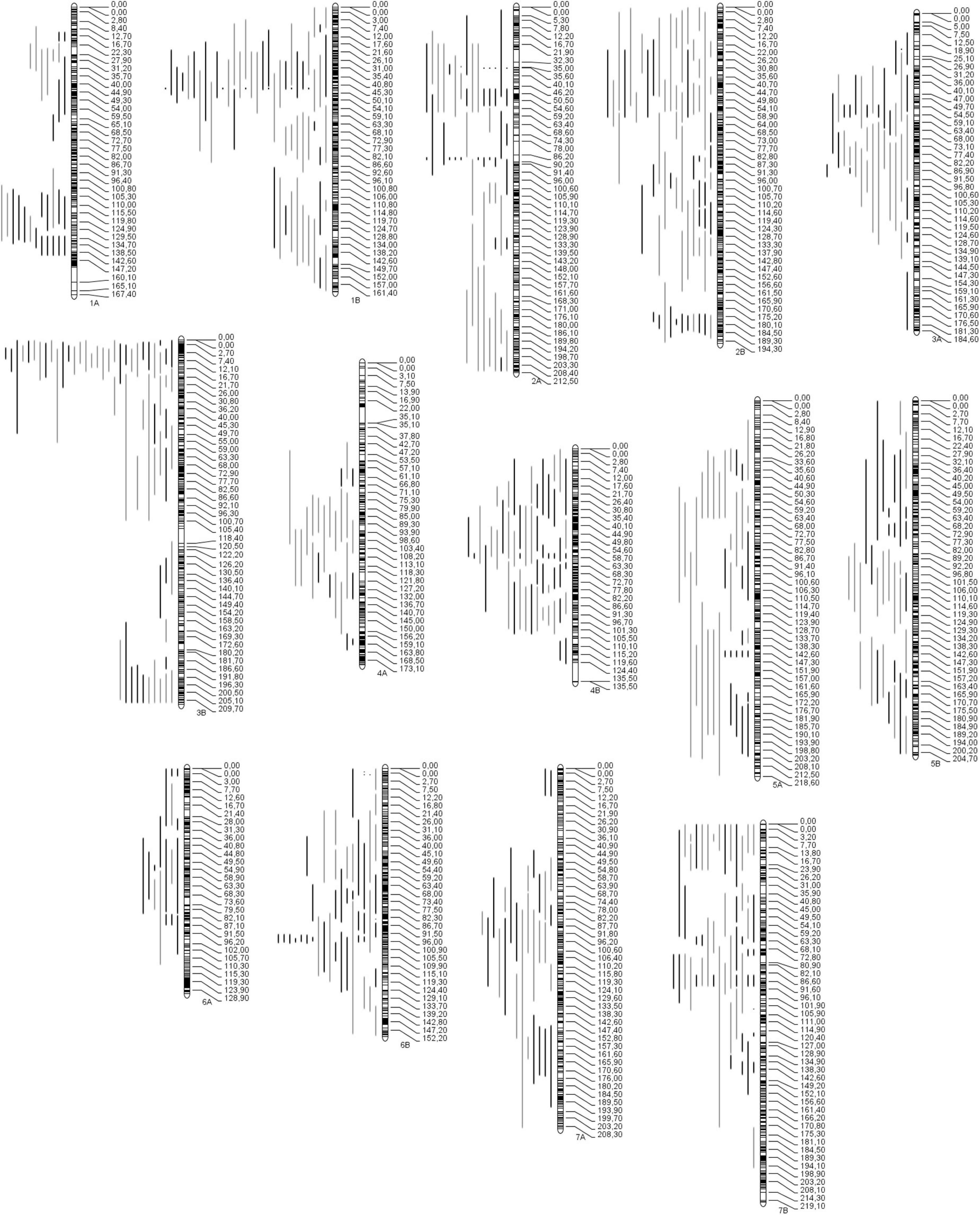
Figure 2. Distribution of the QTL projected throughout all 14 chromosomes (genomes A and B) of durum wheat. QTL were collected for 19 traits related to the main yield components, grains number per area (gray bars) and grain weight (black bars). Black bars within chromosomes represent marker density.
Under irrigated conditions, the chromosomes 7B (26) and 4A (6) had the highest and lowest number of projected QTL, respectively, with an average of 14 QTL per chromosome (Figure 3A). The trait with the highest number of projected QTL was TGW (66 QTL: Figure 3B). The 49.19% of the projected QTL correspond to the grain weight category while the categories of grains number per area has a total of 99 projected QTL. Under rainfed conditions, the number of QTL per chromosome ranged from 10 on chromosome 6A to 44 on chromosome 1B, with an average of 28 QTL per chromosome (Figure 3C). The trait with the highest number of QTL was TGW (114), while those with the lowest number of QTL were GWPS (1) and SL (3) (Figure 3D). Half of the projected QTL (50%) under rainfed conditions correspond to the grain weight category.
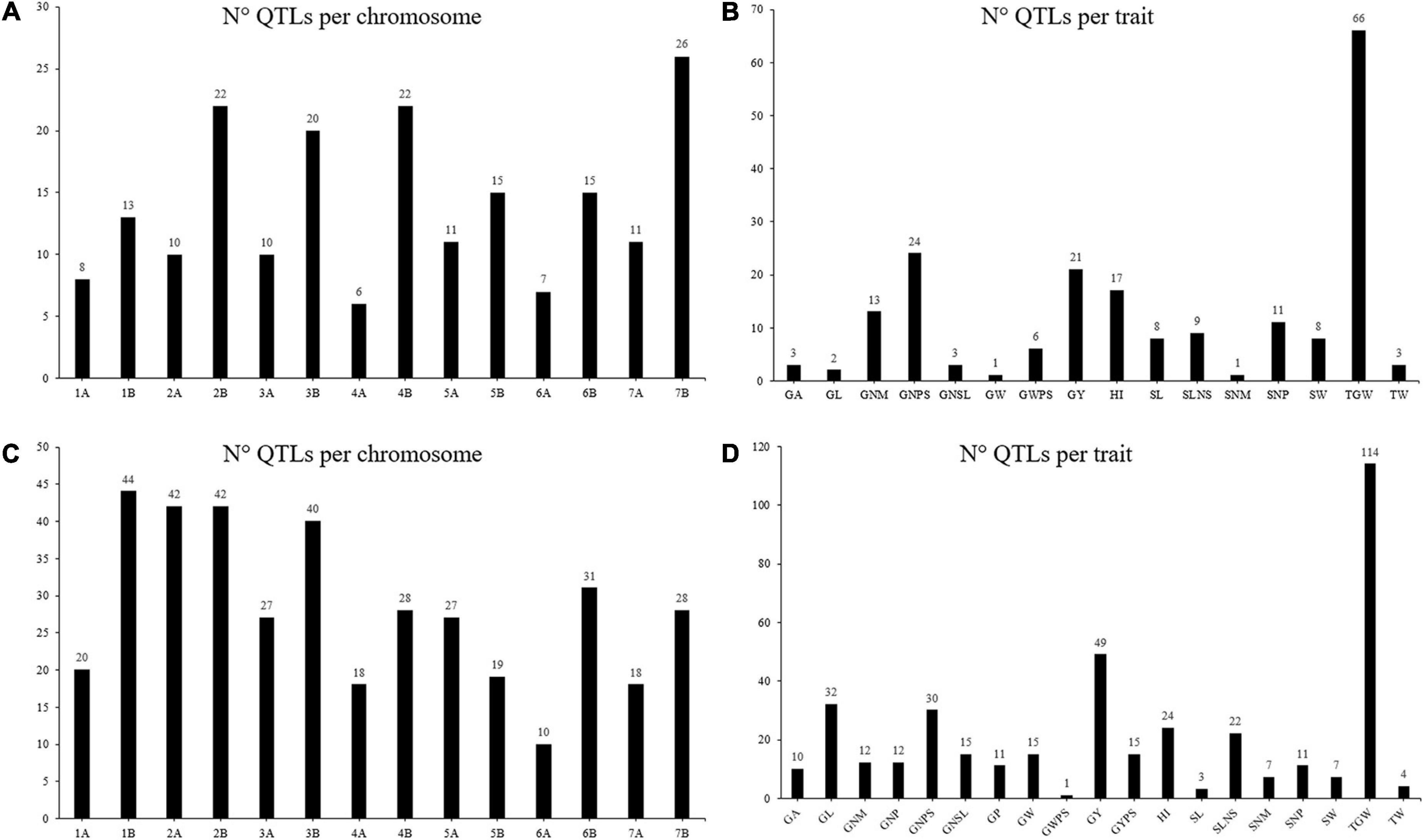
Figure 3. Number of QTL projected under different water regimes. Number of projected QTL per chromosome (A) and per trait (B) under irrigated conditions. Number of projected QTL per chromosome (C) and per trait (D) under rainfed conditions.
Meta-QTL detection
Overall, out of the 590 QTLs projected on the consensus map, 421 were grouped into 76 MQTL. The rest of the QTLs (169) remained as single QTL since they did not overlap with any MQTL interval, the QTL overlapped with more than one MQTL due to their large CI, or because the predicted QTL peaks were not included within any MQTL. Specifically, 28 and 48 MQTL were identified under irrigated and rainfed conditions, respectively (Tables 3,4). Under rainfed conditions, the number of QTL per MQTL varied from 2 on several chromosomes to more than 20 on chromosomes 1B (YIELD_MQTL1B.1_D; 47.7 cM) and 3B (YIELD_MQTL3B.1_D; 9.8 cM), with an average of 5.89 QTL per MQTL. While under irrigation conditions, the number of QTL per MQTL varied from 2 to 8 on chromosome 3B (YIELD_MQTL3B.3_I; 206.94 cM), with an average of 3.75 QTL per MQTL. The number of MQTL per chromosome varied from 1 on chromosome 1B (under irrigated conditions) to 5 on chromosome 2 (A and B) under rainfed conditions. In addition, no MQTL were detected on chromosome 1A under irrigated conditions. The number of traits involved per MQTL ranged from 1 to 9 in the MQTL YIELD_MQTL1B.1_D (1B), which also contains the largest number of studies (7). Finally, the CI of the MQTL ranged from 0.12 to 25.96 cM with an average of 6.79 cM, which is significantly lower than the average of CI (24.3 cM) considering the original QTL. Interestingly, in 12 regions of the genome (on all chromosomes except 1A, 4A, 5A, and 6B), the MQTL detected under both water conditions were overlapped (Figure 4 and Table 5).
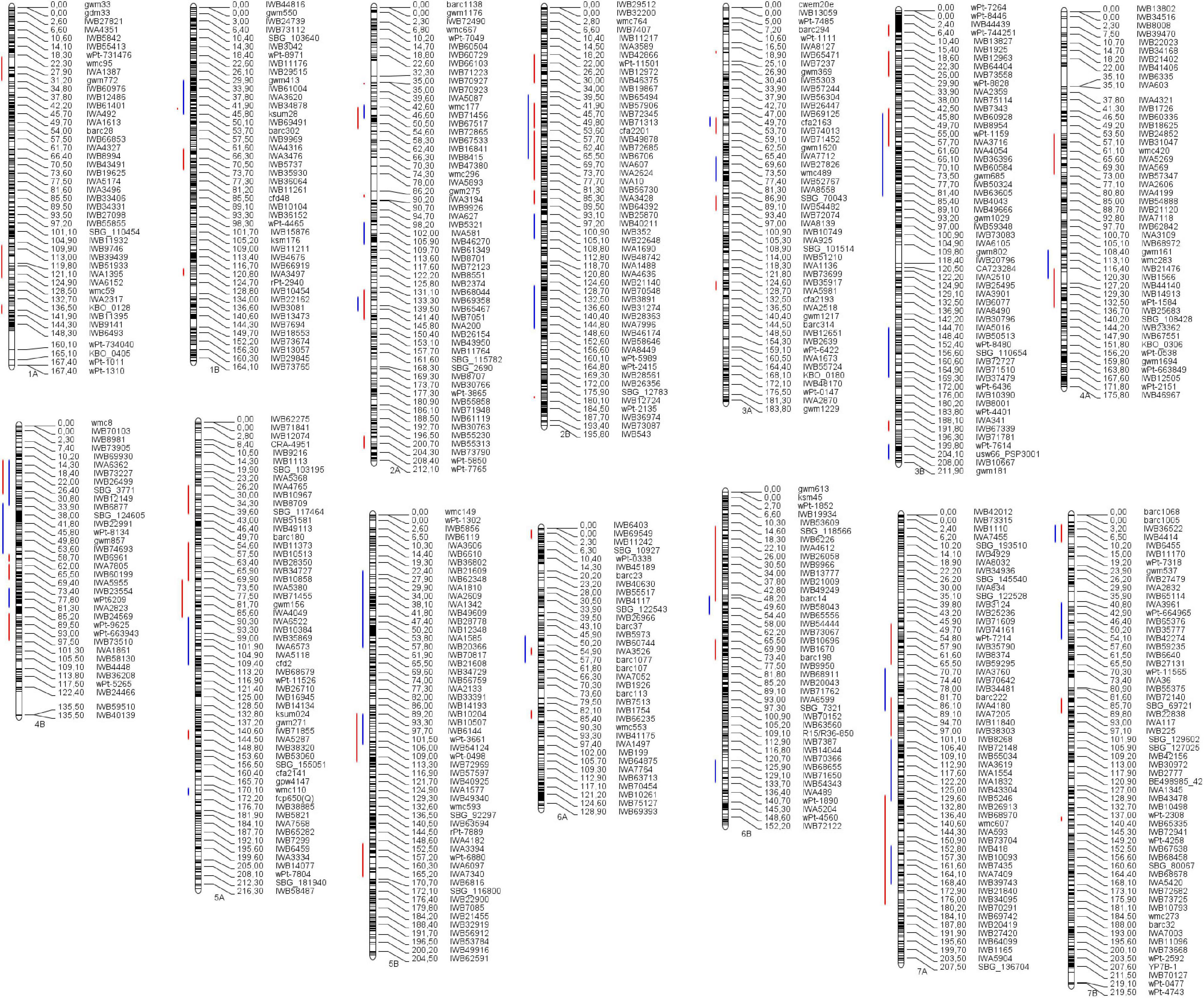
Figure 4. Distribution of the MQTL throughout all 14 chromosomes (genomes A and B) of durum wheat. A total of 76 MQTL were detected under irrigated conditions (blue bars), and under rainfed conditions (red bars). Black bars within chromosomes represent marker density, and to the right is the distance in cM and marker name.
Candidate genes identification for yield-related traits of durum wheat grown under different water regimes
Candidate genes associated with the MQTL detected were identified using the sequences of flanking markers of the CI launched against the genome browser for both “Svevo” (durum wheat) and “Chinese spring” (bread wheat)2 reference genomes. A total of 44 genes were detected and used to determine differentially expressed genes (DEG) up/down regulated under drought/heat conditions using the RNAseq data available at http://www.wheat-expression.com/.
During the exposure to water stress conditions, the 15 most expressed genes (Figure 5), showing the higher expression level (tmp > 3) were associated to MQTL under both irrigated and rainfed conditions. In particular, the following genes were identified: CBL-interacting protein kinase 2-like and endo-1,4-beta-xylanase 1-like on chromosome 2A (YIELD_MQTL2A.2_D and YIELD_MQTL2A.3_D, respectively), zinc finger CCCH domain-containing protein 13-like and cysteine-rich and transmembrane domain-containing protein WIH1-like all on chromosome 3A (YIELD_MQTL3A.2_D and YIELD_MQTL3A.4_D, respectively), DExH-box ATP-dependent RNA helicase DExH3-like, alpha-xylosidase 1-like and ADP-ribosylation factor GTPase-activating protein AGD11 on chromosome 3B (YIELD_MQTL3B.1_D, YIELD_MQTL3B.2_D, and YIELD_MQTL3B.4_D, respectively), heat stress transcription factor A-9-like on chromosome 4B (YIELD_MQTL4B.2_D), disease resistance protein RGA3 like on chromosome 5A (YIELD_MQTL5A.1_D), two disease resistance protein RGA4-like on chromosome 5B (YIELD_MQTL5B.2_D and YIELD_MQTL5B.2_I), transcriptional regulator SLK3 on 7A (YIELD_MQTL7A.1_I), disease resistance protein RGA5-like and methyltransferase on chromosome 7B (YIELD_MQTL7B.1_D and YIELD_MQTL7B.3_D, respectively).
Discussion
Quantitative trait loci for yield component in durum wheat
Increasing productivity under drought stress conditions is one of the main objectives of breeders of staple crops including wheat, due to the need to maintain a sufficient food supply for a growing world population considering the impacts of global warming (Shew et al., 2020). The adaptation to abiotic stress conditions is extremely challenging due to the quantitative genetic basis of the molecular mechanisms adopted by plants to respond to stress (Reynolds et al., 2005). Given that the grain yield components have a quantitative inheritance, and therefore are highly affected by the environment (Nehe et al., 2019), the development of high-yielding varieties must incorporate and accumulate loci associated with yield components that allow them to tolerate the scarcity of water, without affecting significantly their growth and yield. In this sense, numerous studies have been carried out to identify loci associated with the main yield components under irrigated and rainfed conditions in bread wheat (Gupta et al., 2020), and to a lesser extent in durum wheat (Maccaferri et al., 2019; Arriagada et al., 2020).
Grain yield components and their interactions determine the wheat yield (Li et al., 2019). According to our results, among the grain yield components, grain weight is the component most studied in the QTL studies of durum wheat, being TGW the trait most evaluated. This result agrees with the MQTL analyses carried out in bread wheat, in which grains number per spike (GNPS) and TGW are the most evaluated traits under different environmental conditions (Zhang et al., 2010; Gupta et al., 2020). These results can be explained because the main approach to augmenting crop yield is to increase the number and the weight of grains. In fact, TGW is the most important limiting factor affecting wheat yield (Liang et al., 2017). The weight of the grain is the last component of the yield that is formed, and it is highly dependent on the speed and the duration of the grain filling period (Takai et al., 2005), and it is greatly affected by the environment (Li et al., 2019). Therefore, exploring the genetic basis of TGW and its related traits is an effective approach to increase wheat yields (Würschum et al., 2018). According to the distribution of QTLs through the durum wheat genome, the chromosomes with the highest number of QTLs were 2A (76), 2B (71), and 3B (71), whereas chromosome 6A was the one with the lowest number of QTLs (21). These chromosomes consistently contain the greatest number of QTLs for root-related traits (Soriano and Alvaro, 2019), and for quality-related traits, as well as abiotic and biotic stress in durum wheat (Soriano et al., 2021).
Meta-QTL for yield under different water regimens in durum wheat
In the last decades, many QTL studies have been performed to identify loci associated with grain-yield related traits in bread and durum wheat. However, only a small fraction of these QTLs and the associated markers have been utilized in breeding programs (Cobb et al., 2019), due that most of those QTLs have minor effects and their expression is greatly affected by the environment and the genetic background (Zheng et al., 2021). In this sense, the MQTL analysis has been widely used for collecting data and information of QTL from different populations with different sizes and evaluated under different environmental conditions to identify stable QTL in the plant genomes (Shariatipour et al., 2021). This method allows to identify genome regions (MQTL) implicated in trait variation and reducing the confidence intervals of the QTL. Therefore, the MQTL are useful in marker-assisted breeding programs. In addition, it allows the identification of candidate genes within the MQTL detected in the genome of the target species (Veyrieras et al., 2007).
Several MQTL analyzes have been performed on several important crops such as rice (Islam et al., 2019), maize (Martinez et al., 2016), and barley (Zhang et al., 2017). In wheat, the highest number of MQTL analyzes have been performed in common wheat (Quraishi et al., 2011; Tyagi et al., 2015; Kumar et al., 2020; Zheng et al., 2021). In durum wheat, there are few previous studies of MQTL analysis (Soriano et al., 2017, 2021). In the present paper, we compared the genomic regions involved in durum wheat yield performance under rainfed and irrigated conditions, comparing MQTL in order to identify the most import regions associated to stress tolerance and the candidate genes underlying them. The number of MQTL detected under irrigated conditions is lower than those detected under rainfed conditions, because most durum wheat is sown under rainfed conditions. Twelve regions of the genome overlapping for both rainfed and irrigated conditions. A new MQTL was detected on chromosome 5A (YIELD_MQTL5A.1_D), underlying genes activated only during stress conditions. QTL for stress condition were reported also by Soriano et al. (2021) on chromosome 5A. The chromosome 5A seems to have an important role in yield and adaptation trait, and this can be due to the presence of the vernalization genes Vrn-A1, favorable alleles for this gene during breeding helps develop spring habit without cold requirements for flowering, thus this can be used as a strategy for introgressing important target traits from non-adapted pre-breeding materials combining the most favorable vernalization alleles (Soriano et al., 2021).
In the present study, an interesting MQTL on chromosome 2A YIELD_MQTL2A.2_D (map position 51.86 cM) linked to TGW and HI was co-localized with a MQTL previously described for different traits in durum wheat by Soriano et al. (2021). These authors, in fact, identified a MQTL on the chromosome 2A at 50.8 cM (durumMQTL2A.3) associated with traits related to abiotic stress. Specifically, normalized difference vegetation index (NDVI) and chlorophyll content (SPAD) were identified in that genetic region, which are associated to grain yield under drought stress (Cairns et al., 2012). Considering the map position of the two MQTL could be coincident, this strong MQTL for stress and for yield under rainfed conditions can be useful in durum wheat breeding programs, in which the objective is to increase grain yield under drought conditions.
Identification of candidate genes underlying the stable meta-QTL
This is the first study that identifies and compares wheat MQTL associated with yield components under irrigated and rainfed conditions. Many different genes have been detected and associated to MQTL for yield-related traits grown under different water regimes, some of them related directly to water stress, some others related to secondary mechanism activated by stresses, and finally genes associated to plant development and differentiation.
A gene model identified on chromosome 2A and associated with a MQTL for harvest index and spike length was the CBL-interacting protein kinase 2-like involved in the CIPK serine-threonine protein kinases interaction with the activation of the kinase in a calcium-dependent manner. This gene plays a positive regulatory effect in drought stress response, in fact, Wang et al. (2016) found that the over-expression of the TaCBL-CIPK2 gene confers drought tolerance in transgenic tobacco plants, by regulating stomatal closure. Another detected important gene on chromosome 2A was endo-1,4-beta-xylanase 1-like, involved in the hydrolyzation of the xylan backbones into shorter and soluble xylo-oligo saccharides. The xylanase is strongly expressed in tolerant barley genotype under drought stress for the mobilization of the nutrients from the aleurone layer and endosperm to the developing seed (Hajibarat et al., 2022). Among the gene models detected, different disease resistance protein RGA were identified and specifically RGA3, and RGA4 (two different), and RGA5 on chromosomes 5A, 5B, and 7B under both irrigated and rainfed condition. The RGA genes have been identified primarily in response to biotic stresses such as fungal pathogens (Huang and Gill, 2001; Césari et al., 2014) and subsequently for drought stress, due to the interaction with other proteins, which positively affect the ABA biosynthesis in seed germinations (Skubacz et al., 2016) and flag leaves (Onyemaobi et al., 2021).
On chromosome 3A, we reported the zing finger CCCH domain protein 13-like which was found to have a function on plant development and tolerance to abiotic stresses such as salt, drought, flooding, cold temperatures and oxidative stress (Han et al., 2021). In addition, we identified cysteine-rich and transmembrane domain-containing protein WIH1-like, which is involved in megasporogenesis and germ cell formation from somatic precursor cells (Lieber et al., 2011). A DExH-box ATP-dependent RNA helicase DExH3-like (DExH-box RHs) gene, which is involved in biotic and abiotic stresses response as well as plant development, was also identified and associated to MQTL on chromosome 3B for most of the traits for grain weight and grain number per area which have been considered. Recently, the relationship between DExH-box RHs and temperature stress tolerance has been reported in Arabidopsis (Liu and Imai, 2018). Another gene model detected on chromosome 3B was the α-xylosidase 1-like, which contributes to maintain the mechanical integrity of the primary cell wall in the growing and pre-growing tissues. Additionally, in Arabidospis mutant for α-xylosidase the expression of genes encoding specific abscisic acid and gibberellin enzymes was altered in accordance with the aberrant germination phenotype (Shigeyama et al., 2016). Considering that the abscisic acid is involved in plant adaptation to environmental stresses (Audran et al., 2001), we can assume an indirect correlation between the expression of the α-xylosidase 1-like in response to water regimes. One additional gene identified on chromosome 3B was ADP-ribosylation factors GTPase-activating protein AGD11, which has a function in diverse physiological and molecular activities and recently an involvement on conferring tolerance to biotic and abiotic stresses in in rice and foxtail millet (Muthamilarasan et al., 2016).
The heat stress transcription factors have been detected for MQTL on chromosome 4B. These factors have been largely studied in plants and play a crucial role in response to high temperature, salinity, and drought because they adversely affect the survival, growth, and reproduction by regulating the expression of stress-responsive genes, such as heat shock proteins (Guo et al., 2016).
One gene was identified on chromosome 7A associated with a MQTL for TW, GWPS, GNPS, TGW, the transcriptional regulator SLK3, which encodes a regulator of AGAMOUS gene and functions together with a repressor of the AGAMOUS gene, the LEUNIG gene. One experiment in Arabidopsis with loss-of-function mutants of the AGAMOUS, showed that the repression of the gene by transcriptional regulator SLK3 induced a replacement of the stamens with the petals, and carpels with a new flower (Franks et al., 2001). On chromosome 7B, a methyltransferase involved in DNA methylation at cytosine residues and required for gene expression control and genome stability (Thomas et al., 2014), was detected and it correlates to a MQTL for TGW, GL, SLNS. This gene has been characterized and appeared to be express as a response to stress for the regulation of developmental events such as dormancy (Gianoglio et al., 2017), and against stress-inducing treatment, such as damaged proteins (Krzewska et al., 2021).
Conclusion
In conclusion, the yield components are complex traits controlled by many QTLs with small effect. In this sense, the MQTL studies provide valuable information for QTL fine mapping and key genes for cloning. We performed the first meta-analysis study that identifies and compares durum wheat MQTL associated with yield components under irrigated and rainfed conditions. In this study, a total of 74 MQTLs were detected, where a total of 35 candidate genes associated with drought stress tolerance and yield were identified. A valuable novel aspect of this work was the identification of 12 genomic regions containing stable MQTLs on all chromosomes, except 1A, 4A, 5A, and 6B. Finally, 15 correlated genes that were differentially expressed under drought were reported, which can be very useful in durum wheat breeding programs to increase the grain yields regardless of the water regime used.
Data availability statement
The datasets presented in this study can be found in online repositories. The names of the repository/repositories and accession number (s) can be found in the article/Supplementary material.
Author contributions
OA and AS contributed for the conception and design of the study, organized the database and performed all data analysis, and wrote the first draft of the manuscript. AG and IlM performed the analysis and identification of candidate genes and wrote sections of the manuscript. MM, MC, SR, CA, and IvM made important improvements to the manuscript through their revisions and feedback. All authors revised and edited the manuscript, read, and approved the final manuscript.
Funding
This article processing charge was funded by the Agencia Nacional de Investigación y Desasrrollo de Chile (ANID) (Fondecyt Regular n° 1210092 grant). This work was partially supported by the ANID (grants Fondecyt Regular n° 1210092 [AS, IvM, and CA] and Fondecyt Postdoctorado n°3200981 [OA]). This research was also partially funded by the project “CerealMed”—Enhancing diversity in Mediterranean cereal farming systems, project funded by PRIMA Section 2—Multi-topic 2019 and MUR (Ministero dell’Università e della Ricerca), the Attraction and International Mobility PON-AIM Project AIM1812334 (Ministero dell’Istruzione, dell’Università e della Ricerca, Italy); PSR Puglia 2014–2020, sottomisura 16.2, “FILIERA FRUMENTO DURO: INNOVAZIONE VARIETALE, QUALITA’ E TRACCIABILITA’ DELLE PRODUZIONI PUGLIESI”/IPERDURUM DDS N. 130 del 30/06/2020 CUP: B39J20000160009 (AG and IlM).
Conflict of interest
The authors declare that the research was conducted in the absence of any commercial or financial relationships that could be construed as a potential conflict of interest.
Publisher’s note
All claims expressed in this article are solely those of the authors and do not necessarily represent those of their affiliated organizations, or those of the publisher, the editors and the reviewers. Any product that may be evaluated in this article, or claim that may be made by its manufacturer, is not guaranteed or endorsed by the publisher.
Supplementary material
The Supplementary Material for this article can be found online at: https://www.frontiersin.org/articles/10.3389/fpls.2022.984269/full#supplementary-material
Footnotes
References
Acuña-Galindo, M. A., Mason, R. E., Subramanian, N. K., and Hays, D. B. (2015). Meta-analysis of wheat QTL regions associated with adaptation to drought and heat stress. Crop Sci. 55, 477–492. doi: 10.2135/cropsci2013.11.0793
Arcade, A., Labourdette, A., Falque, M., Mangin, B., Chardon, F., Charcosset, A., et al. (2004). BioMercator: Integrating genetic maps and QTL towards discovery of candidate genes. Bioinformatics 20, 2324–2326. doi: 10.1093/bioinformatics/bth230
Arriagada, O., Marcotuli, I., Gadaleta, A., and Schwember, A. R. (2020). Molecular mapping and genomics of grain yield in durum wheat: A review. Int. J. Mol. Sci. 21:7021. doi: 10.3390/ijms21197021
Audran, C., Liotenberg, S., Gonneau, M., North, H., Frey, A., Tap-Waksman, K., et al. (2001). Localisation and expression of zeaxanthin epoxidase mRNA in Arabidopsis in response to drought stress and during seed development. Funct. Plant Biol. 28, 1161–1173. doi: 10.1071/PP00134
Avni, R., Oren, L., Shabtay, G., Assili, S., Pozniak, C., Hale, I., et al. (2018). Genome based meta-QTL analysis of grain weight in tetraploid wheat identifies rare alleles of GRF4 associated with larger grains. Genes 9:636.
Ayed, S., Othmani, A., Bouhaouel, I., and Teixeira da Silva, J. A. (2021). Multi-Environment screening of durum wheat genotypes for drought tolerance in changing climatic events. Agronomy 11:875. doi: 10.3390/agronomy11050875
Blanco, A., Mangini, G., Giancaspro, A., Giove, S., Colasuonno, P., and Simeone, R. (2012). Relationships between grain protein content and grain yield components through quantitative trait locus analyses in a recombinant inbred line population derived from two elite durum wheat cultivars. Mol. Breed. 30, 79–92.
Cairns, J. E., Sanchez, C., Vargas, M., Ordoñez, R., and Araus, J. L. (2012). Dissecting maize productivity: Ideotypes associated with grain yield under drought stress and well-watered conditions. J. Integr. Plant Biol. 54, 1007–1020. doi: 10.1111/j.1744-7909.2012.01156.x
Césari, S., Kanzaki, H., Fujiwara, T., Bernoux, M., Chalvon, V., Kawano, Y., et al. (2014). The NB-LRR proteins RGA4 and RGA5 interact functionally and physically to confer disease resistance. EMBO J. 33, 1941–1959. doi: 10.15252/embj.201487923
Chardon, F., Virlon, B., Moreau, L., Falque, M., Joets, J., Decousset, L., et al. (2004). Genetic architecture of flowering time in maize as inferred from quantitative trait loci meta-analysis and synteny conservation with the rice genome. Genetics 168, 2169–2185. doi: 10.1534/genetics.104.032375
Cobb, J. N., Biswas, P. S., and Platten, J. D. (2019). Back to the future: Revisiting MAS as a tool for modern plant breeding. Theor. Appl. Genet. 132, 647–667. doi: 10.1007/s00122-018-3266-4
Colasuonno, P., Marcotuli, I., Blanco, A., Maccaferri, M., Condorelli, G. E., Tuberosa, R., et al. (2019). Carotenoid pigment content in durum wheat (Triticum turgidum L. var durum): An overview of quantitative trait loci and candidate genes. Front. Plant Sci. 10:1347. doi: 10.3389/fpls.2019.01347
Colasuonno, P., Marcotuli, I., Gadaleta, A., and Soriano, J. M. (2021). From genetic maps to QTL cloning: An overview for durum wheat. Plants 10:315. doi: 10.3390/plants10020315
Dahl, C. (2017). Global Durum Outlook. Available online at: http://www.italmopa.com/wp-content/uploads/2017/05/144_all_1.pdf (accessed on Aug 15, 2021).
Desiderio, F., Zarei, L., Licciardello, S., Cheghamirza, K., Farshadfar, E., Virzi, N., et al. (2019). Genomic regions from an Iranian landrace increase kernel size in durum wheat. Front. Plant Sci. 10:448. doi: 10.3389/fpls.2019.00448
Dettori, M., Cesaraccio, C., and Duce, P. (2017). Simulation of climate change impacts on production and phenology of durum wheat in Mediterranean environments using CERES-Wheat model. Field Crop Res. 206, 43–53. doi: 10.1016/j.fcr.2017.02.013
Elouafi, I., and Nachit, M. M. (2004). A genetic linkage map of the Durum × Triticum dicoccoides backcross population based on SSRs and AFLP markers, and QTL analysis for milling traits. Theor. Appl. Genet. 108, 401–413. doi: 10.1007/s00122-003-1440-8
Faris, J. D., Zhang, Q., Chao, S., Zhang, Z., and Xu, S. S. (2014). Analysis of agronomic and domestication traits in a durum × cultivated emmer wheat population using a high-density single nucleotide polymorphism-based linkage map. Theor. Appl. Genet. 127, 2333–2348. doi: 10.1007/s00122-014-2380-1
Fatiukha, A., Filler, N., Lupo, I., Lidzbarsky, G., Klymiuk, V., Korol, A. B., et al. (2020). Grain protein content and thousand kernel weight QTLs identified in a durum × wild emmer wheat mapping population tested in five environments. Theor. Appl. Genet. 133, 119–131. doi: 10.1007/s00122-019-03444-8
Food and Agriculture Organization of the United Nations [FAO] (2018). The Future Of Food And Agriculture Alternative Pathways To 2050. Rome: Food and Agriculture Organization of the United Nations.
Franks, R. G., Wang, C., Levin, J. Z., and Liu, Z. (2001). SEUSS, a member of a novel family of plant regulatory proteins, represses floral homeotic gene expression with LEUNIG. Development 129, 253–263. doi: 10.1242/dev.129.1.253
Gegas, V. C., Nazari, A., Griffiths, S., Simmonds, J., Fish, L., Orford, S., et al. (2010). A genetic framework for grain size and shape variation in wheat. Plant Cell 22, 1046–1056. doi: 10.1105/tpc.110.074153
Giancaspro, A., Giove, S. L., Zacheo, S. A., Blanco, A., and Gadaleta, A. (2019). Genetic variation for protein content and yield-related traits in a durum population derived from an inter-specific cross between hexaploid and tetraploid wheat cultivars. Front. Plant Sci. 10:1509. doi: 10.3389/fpls.2019.01509
Gianoglio, S., Moglia, A., Acquadro, A., Comino, C., and Portis, E. (2017). The genome-wide identification and transcriptional levels of DNA methyltransferases and demethylases in globe artichoke. PLoS One 12:e0181669. doi: 10.1371/journal.pone.0181669
Giunta, F., De Vita, P., Mastrangelo, A. M., Sanna, G., and Motzo, R. (2018). Environmental and genetic variation for yield-related traits of durum wheat as affected by development. Front. Plant Sci. 9:8. doi: 10.3389/fpls.2018.00008
Goffinet, B., and Gerber, S. (2000). Quantitative trait loci: A meta-analysis. Genetics 155, 463–473. doi: 10.1093/genetics/155.1.463
Golabadi, M., Arzani, A., Maibody, S. M., Tabatabaei, B. S., and Mohammadi, S. A. (2011). Identification of microsatellite markers linked with yield components under drought stress at terminal growth stages in durum wheat. Euphytica 177, 207–221. doi: 10.1007/s10681-010-0242-8
Graziani, M., Maccaferri, M., Royo, C., Salvatorelli, F., and Tuberosa, R. (2014). QTL dissection of yield components and morpho-physiological traits in a durum wheat elite population tested in contrasting thermo-pluviometric conditions. Crop Pasture Sci. 65, 80–95. doi: 10.1071/CP13349
Guo, B., Sleper, D. A., Lu, P., Shannon, J. G., Nguyen, H. T., and Arelli, P. R. (2006). QTLs associated with resistance to soybean cyst nematode in soybean: Meta-analysis of QTL locations. Crop Sci. 46, 202–208. doi: 10.2135/cropsci2005.04-0036
Guo, M., Liu, J.-H., Ma, X., Luo, D.-X., Gong, Z.-H., and Lu, M.-H. (2016). The plant heat stress transcription factors (HSFs): Structure, regulation, and function in response to abiotic stresses. Front. Plant Sci. 7:114. doi: 10.3389/fpls.2016.00114
Gupta, P. K., Balyan, H. S., Sharma, S., and Kumar, R. (2020). Genetics of yield, abiotic stress tolerance and biofortification in wheat (Triticum aestivum L.). Theor. Appl. Genet. 133, 1569–1602. doi: 10.1007/s00122-020-03583-3
Hajibarat, Z., Saidi, A., Shahbazi, M., Zeinalabedini, M., Gorji, A. M., Mirzaei, M., et al. (2022). Comparative proteome analysis of the penultimate internodes of Barley genotypes differing in stem reserve remobilization under drought stress. Res Square [Preprint]. doi: 10.21203/rs.3.rs-1178453/v1
Han, G., Qiao, Z., Li, Y., Wang, C., and Wang, B. (2021). The roles of CCCH Zinc-Finger proteins in plant abiotic stress tolerance. Int. J. Mol. Sci. 22:8327. doi: 10.3390/ijms22158327
Huang, L., and Gill, B. (2001). An RGA – like marker detects all known Lr21 leaf rust resistance gene family members in Aegilops tauschii and wheat. Theor. Appl. Genet. 103, 1007–1013. doi: 10.1007/s001220100701
Islam, M., Ontoy, J., and Subudhi, P. K. (2019). Meta-analysis of quantitative trait loci associated with seedling-stage salt tolerance in rice (Oryza sativa L.). Plants 8:33. doi: 10.3390/plants8020033
Krzewska, M., Dubas, E., Gołȩbiowska, G., Nowicka, A., Janas, A., Zieliński, K., et al. (2021). Comparative proteomic analysis provides new insights into regulation of microspore embryogenesis induction in winter triticale (×Triticosecale Wittm.) after 5-azacytidine treatment. Sci. Rep. 11:22215. doi: 10.1038/s41598-021-01671-y
Kumar, A., Saripalli, G., Jan, I., Kumar, K., Sharma, P. K., Balyan, H. S., et al. (2020). Meta-QTL analysis and identification of candidate genes for drought tolerance in bread wheat (Triticum aestivum L.). Physiol. Mol. Biol. Plants 26, 1713–1725. doi: 10.1007/s12298-020-00847-6
Li, J., Zhang, Z., Liu, Y., Yao, C., Song, W., Xu, X., et al. (2019). Effects of micro-sprinkling with different irrigation amount on grain yield and water use efficiency of winter wheat in the North China Plain. Agric. Water Manage. 224:105736. doi: 10.1016/j.agwat.2019.105736
Liang, W., Zhang, Z., Wen, X., Liao, Y., and Liu, Y. (2017). Effect of non-structural carbohydrate accumulation in the stem pre-anthesis on grain filling of wheat inferior grain. Field Crop Res. 211, 66–76. doi: 10.1016/j.fcr.2017.06.016
Lieber, D., Lora, J., Schrempp, S., Lenhard, M., and Laux, T. (2011). Arabidopsis WIH1 and WIH2 genes act in the transition from somatic to reproductive cell fate. Curr. Biol. 21, 1009–1017. doi: 10.1016/j.cub.2011.05.015
Liu, S., Hall, M. D., Griffey, C. A., and McKendry, A. L. (2009). Meta-analysis of QTL associated with Fusarium head blight resistance in wheat. Crop Sci. 49, 1955–1968. doi: 10.2135/cropsci2009.03.0115
Liu, Y., and Imai, R. (2018). Function of plant DExD/H-Box RNA helicases associated with ribosomal RNA biogenesis. Front. Plant Sci. 9:125. doi: 10.3389/fpls.2018.00125
Maccaferri, M., El-Feki, W., Nazemi, G., Salvi, S., Canè, M. A., and Colalongo, M. C. (2016). Prioritizing quantitative trait loci for root system architecture in tetraploid wheat. J. Exp. Bot. 67, 1161–1178. doi: 10.1093/jxb/erw039
Maccaferri, M., Harris, N. S., Twardziok, S. O., Pasam, R. K., Gundlach, H., Spannagl, M., et al. (2019). Durum wheat genome highlights past domestication signatures and future improvement targets. Nat. Genet. 51, 885–895. doi: 10.1038/s41588-019-0381-3
Maccaferri, M., Ricci, A., Salvi, S., Milner, S. G., Noli, E., Martelli, P. L., et al. (2015). A high-density, SNP-based consensus map of tetraploid wheat as a bridge to integrate durum and bread wheat genomics and breeding. Plant Biotechnol. J. 13, 648–663. doi: 10.1111/pbi.12288
Maccaferri, M., Sanguineti, M. C., Corneti, S., Ortega, J. L. A., Salem, M. B., and Bort, J. (2008). Quantitative trait loci for grain yield and adaptation of durum wheat (Triticum durum Desf.) across a wide range of water availability. Genetics 178, 489–511. doi: 10.1534/genetics.107.077297
Mangini, G., Blanco, A., Nigro, D., Signorile, M. A., and Simeone, R. (2021). Candidate genes and quantitative trait loci for grain yield and seed size in durum wheat. Plants 10:312. doi: 10.3390/plants10020312
Marcotuli, I., Colasuonno, P., Hsieh, Y. S. Y., Fincher, G. B., and Gadaleta, A. (2020). Non-starch polysaccharides in durum wheat: A review. Int. J. Mol. Sci. 21:2933. doi: 10.3390/ijms21082933
Marcotuli, I., Gadaleta, A., Mangini, G., Signorile, A. M., Zacheo, S. A., and Blanco, A. (2017). Development of a high-density SNP-based linkage map and detection of QTL for β-glucans, protein content, grain yield per spike and heading time in durum wheat. Int. J. Mol. Sci. 18:1329. doi: 10.3390/ijms18061329
Martinez, A. K., Soriano, J. M., Tuberosa, R., Koumproglou, R., Jahrmann, T., and Salvi, S. (2016). Yield QTLome distribution correlates with gene density in maize. Plant Sci. 242, 300–309. doi: 10.1016/j.plantsci.2015.09.022
Martínez-Moreno, F., Solís, I., Noguero, D., Blanco, A., Özberk, I., Nsarellah, N., et al. (2020). Durum wheat in the mediterranean rim: Historical evolution and genetic resources. Genet. Resour. Crop Evol. 67, 1415–1436.
Milner, S. G., Maccaferri, M., Huang, B. E., Mantovani, P., Massi, A., and Frascaroli, E. (2016). A multiparental cross population for mapping QTL for agronomic traits in durum wheat (Triticum turgidum ssp. durum). Plant Biotechnol. J. 14, 735–748. doi: 10.1111/pbi.12424
Muthamilarasan, M., Mangu, V. R., Zandkarimi, H., Prasad, M., and Baisakh, N. (2016). Structure, organization and evolution of ADP-ribosylation factors in rice and foxtail millet, and their expression in rice. Sci. Rep. 6:24008.
Nagel, M., Navakode, S., Scheibal, V., Baum, M., Nachit, M., Röder, M. S., et al. (2014). The genetic basis of durum wheat germination and seedling growth under osmotic stress. Biol. Plant. 58, 681–688. doi: 10.1007/s10535-014-0436-3
Nehe, A., Akin, B., Sanal, T., Evlice, A. K., Ünsal, R., Dinçer, N., et al. (2019). Genotype x environment interaction and genetic gain for grain yield and grain quality traits in Turkish spring wheat released between 1964 and 2010. PLoS One 14:e0219432. doi: 10.1371/journal.pone.0219432
Onyemaobi, O., Sangma, H., Garg, G., Wallace, X., Kleven, S., Suwanchaikasem, P., et al. (2021). Reproductive stage drought tolerance in wheat: Importance of stomatal conductance and plant growth regulators. Genes 12:1742. doi: 10.3390/genes12111742
Patil, R. M., Tamhankar, S. A., Oak, M. D., Raut, A. L., Honrao, B. K., Rao, V. S., et al. (2013). Mapping of QTL for agronomic traits and kernel characters in durum wheat (Triticum durum Desf.). Euphytica 190, 117–129. doi: 10.1007/s10681-012-0785-y
Peleg, Z. V. I., Fahima, T., Krugman, T., Abbo, S., Yakir, D. A. N., Korol, A. B., et al. (2009). Genomic dissection of drought resistance in durum wheat × wild emmer wheat recombinant inbreed line population. Plant Cell Environ. 32, 758–779. doi: 10.1111/j.1365-3040.2009.01956.x
Peng, J. H., Ronin, Y., Fahima, T., Röder, M. S., Li, Y., Nevo, E., et al. (2003). Domestication quantitative trait loci in Triticum dicoccoides, the progenitor of wheat. Proc. Natl. Acad. Sci. U.S.A. 100, 2489–2494. doi: 10.1073/pnas.252763199
Quraishi, U. M., Abrouk, M., Murat, F., Pont, C., Foucrier, S., Desmaizieres, G., et al. (2011). Cross-genome map based dissection of a nitrogen use efficiency ortho-metaQTL in bread wheat unravels concerted cereal genome evolution. Plant J. 65, 745–756. doi: 10.1111/j.1365-313X.2010.04461.x
Ramazani, S. H. R., and Abdipour, M. (2019). Statistical analysis of grain yield in Iranian cultivars of barley (Hordeum vulgare). Agric. Res. 8, 239–246. doi: 10.1007/s40003-018-0360-4
Rehman Arif, M. A., Attaria, F., Shokat, S., Akram, S., Waheed, M. Q., Arif, A., et al. (2020). Mapping of QTLs associated with yield and yield related traits in durum wheat (Triticum durum Desf.) under irrigated and drought conditions. Int. J. Mol. Sci. 21:2372. doi: 10.3390/ijms21072372
Reynolds, M. P., Mujeeb-Kazi, A., and Sawkins, M. (2005). Prospects for utilising plant-adaptive mechanisms to improve wheat and other crops in drought-and salinity-prone environments. Ann. Appl. Biol. 146, 239–259. doi: 10.1111/j.1744-7348.2005.040058.x
Roncallo, P. F., Akkiraju, P. C., Cervigni, G. L., and Echenique, V. C. (2017). QTL mapping and analysis of epistatic interactions for grain yield and yield-related traits in Triticum turgidum L. var. durum. Euphytica 213:277. doi: 10.1007/s10681-017-2058-2
Russo, M. A., Ficco, D. B. M., Laido, G., Marone, D., Papa, R., Blanco, A., et al. (2014). A dense durum wheat × T. dicoccum linkage map based on SNP markers for the study of seed morphology. Mol. Breed. 34, 1579–1597.
Said, J. I., Lin, Z., Zhang, X., Song, M., and Zhang, J. (2013). A comprehensive meta QTL analysis for fiber quality, yield, yield related and morphological traits, drought tolerance, and disease resistance in tetraploid cotton. BMC Genomics 14:776. doi: 10.1186/1471-2164-14-776
Saini, D. K., Chopra, Y., Pal, N., Chahal, A., Srivastava, P., and Gupta, P. K. (2021). Meta-QTLs, ortho-MQTLs and candidate genes for nitrogen use efficiency and root system architecture in bread wheat (Triticum aestivum L.). Physiol. Mol. Biol. Plants 27, 2245–2267. doi: 10.1007/s12298-021-01085-0
Shariatipour, N., Heidari, B., and Richards, C. M. (2021). Meta-analysis of QTLome for grain zinc and iron contents in wheat (Triticum aestivum L.). Euphytica 217:86. doi: 10.1007/s10681-021-02818-8
Sharma, J. S., Running, K. L., Xu, S. S., Zhang, Q., Haugrud, A. R. P., Sharma, S., et al. (2019). Genetic analysis of threshability and other spike traits in the evolution of cultivated emmer to fully domesticated durum wheat. Mol. Genet. Genomics 294, 757–771. doi: 10.1007/s00438-019-01544-0
Shew, A. M., Tack, J. B., Nalley, L. L., and Chaminuka, P. (2020). Yield reduction under climate warming varies among wheat cultivars in South Africa. Nat. Commun. 11:4408. doi: 10.1038/s41467-020-18317-8
Shigeyama, T., Watanabe, A., Tokuchi, K., Toh, S., Sakurai, N., Shibuya, N., et al. (2016). α-Xylosidase plays essential roles in xyloglucan remodelling, maintenance of cell wall integrity, and seed germination in Arabidopsis thaliana. J. Exp. Bot. 67, 5615–5629. doi: 10.1093/jxb/erw321
Skubacz, A., Daszkowska-Golec, A., and Szarejko, I. (2016). The role and regulation of ABI5 (ABA-Insensitive 5) in plant development, abiotic stress responses and phytohormone crosstalk. Front. Plant Sci. 7:1884. doi: 10.3389/fpls.2016.01884
Soriano, J. M., and Alvaro, F. (2019). Discovering consensus genomic regions in wheat for root-related traits by QTL meta-analysis. Sci. Rep. 9:0537. doi: 10.1038/s41598-019-47038-2
Soriano, J. M., Colasuonno, P., Marcotuli, I., and Gadaleta, A. (2021). Meta-QTL analysis and identification of candidate genes for quality, abiotic and biotic stress in durum wheat. Sci. Rep. 11:11877. doi: 10.1038/s41598-021-91446-2
Soriano, J. M., Malosetti, M., Roselló, M., Sorrells, M. E., and Royo, C. (2017). Dissecting the old Mediterranean durum wheat genetic architecture for phenology, biomass and yield formation by association mapping and QTL meta-analysis. PLoS One 12:e0178290. doi: 10.1371/journal.pone.0178290
Takai, T., Fukuta, Y., Shiraiwa, T., and Horie, T. (2005). Time-related mapping of quantitative trait loci controlling grain-filling in rice (Oryza sativa L.). J. Exp. Bot. 56, 2107–2118. doi: 10.1093/jxb/eri209
Thanh, P. T., Vladutu, C. I., Kianian, S. F., Thanh, P. T., Ishii, T., Nitta, M., et al. (2013). Molecular genetic analysis of domestication traits in emmer wheat. I: Map construction and QTL analysis using an F2 pupulation. Biotechnol. Biotechnol. Equip. 27, 3627–3637. doi: 10.5504/BBEQ.2013.0008
Thomas, M., Pingault, L., Poulet, A., Duarte, J., Throude, M., Faure, S., et al. (2014). Evolutionary history of methyltransferase 1 genes in hexaploid wheat. BMC Genomics 15:922. doi: 10.1186/1471-2164-15-922
Tyagi, S., Mir, R. R., Balyan, H. S., and Gupta, P. K. (2015). Interval mapping and meta-QTL analysis of grain traits in common wheat (Triticum aestivum L.). Euphytica 201, 367–380. doi: 10.1007/s10681-014-1217-y
Tzarfati, R., Barak, V., Krugman, T., Fahima, T., Abbo, S., Saranga, Y., et al. (2014). Novel quantitative trait loci underlying major domestication traits in tetraploid wheat. Mol. Breed. 34, 1613–1628. doi: 10.1007/s11032-014-0182-4
Veyrieras, J. B., Goffinet, B., and Charcosset, A. (2007). MetaQTL: A package of new computational methods for the meta-analysis of QTL mapping experiments. BMC Bioinformatics 8:49. doi: 10.1186/1471-2105-8-49
Wang, Y., Sun, T., Li, T., Wang, M., Yang, G., and He, G. (2016). A CBL-Interacting Protein Kinase TaCIPK2 confers drought tolerance in transgenic tobacco plants through regulating the stomatal movement. PLoS One 11:e0167962. doi: 10.1371/journal.pone.0167962
Würschum, T., Leiser, W. L., Langer, S. M., Tucker, M. R., and Longin, C. F. H. (2018). Phenotypic and genetic analysis of spike and kernel characteristics in wheat reveals long-term genetic trends of grain yield components. Theor. Appl. Genet. 131, 2071–2084. doi: 10.1007/s00122-018-3133-3
Zaïm, M., Kabbaj, H., Kehel, Z., Gorjanc, G., Filali-Maltouf, A., Belkadi, B., et al. (2020). Combining QTL analysis and genomic predictions for four durum wheat populations under drought conditions. Front. Genet. 11:316. doi: 10.3389/fgene.2020.00316
Zhang, L., Liu, D. C., Guo, X. L., Yang, W. L., Sun, J. Z., Wang, D. W., et al. (2010). Genomic distribution of quantitative trait loci for yield and yield-related traits in common wheat. J. Integr. Plant Biol. 52, 996–1007. doi: 10.1111/j.1744-7909.2010.00967.x
Zhang, X., Shabala, S., Koutoulis, A., Shabala, L., and Zhou, M. (2017). Meta-analysis of major QTL for abiotic stress tolerance in barley and implications for barley breeding. Planta 245, 283–295. doi: 10.1007/s00425-016-2605-4
Keywords: meta-QTL analysis, yield component, QTL, rainfed, drought, durum wheat
Citation: Arriagada O, Gadaleta A, Marcotuli I, Maccaferri M, Campana M, Reveco S, Alfaro C, Matus I and Schwember AR (2022) A comprehensive meta-QTL analysis for yield-related traits of durum wheat (Triticum turgidum L. var. durum) grown under different water regimes. Front. Plant Sci. 13:984269. doi: 10.3389/fpls.2022.984269
Received: 01 July 2022; Accepted: 18 August 2022;
Published: 06 September 2022.
Edited by:
Salvatore Ceccarelli, Bioversity International, ItalyReviewed by:
Reza Mohammadi, Dryland Agricultural Research Institute, Agricultural Research, Education and Extension Organization, IranJai Prakash Jaiswal, G. B. Pant University of Agriculture and Technology, India
Copyright © 2022 Arriagada, Gadaleta, Marcotuli, Maccaferri, Campana, Reveco, Alfaro, Matus and Schwember. This is an open-access article distributed under the terms of the Creative Commons Attribution License (CC BY). The use, distribution or reproduction in other forums is permitted, provided the original author(s) and the copyright owner(s) are credited and that the original publication in this journal is cited, in accordance with accepted academic practice. No use, distribution or reproduction is permitted which does not comply with these terms.
*Correspondence: Andrés R. Schwember, YXNjaHdlbWJlckB1Yy5jbA==