- 1State Key Laboratory of Desert and Oasis Ecology, Xinjiang Institute of Ecology and Geography, Chinese Academy of Sciences, Urumqi, China
- 2University of Chinese Academy of Sciences, Beijing, China
- 3Department of Microbiological Sciences, North Dakota State University, Fargo, ND, United States
Drip irrigation under plastic film mulch is a common agricultural practice used to conserve water. However, compared to traditional flood irrigation with film mulch, this practice limit cotton root development from early flowering stage and may cause premature senescence in cotton. Changes of root will consequently shape the composition and activity of rhizosphere microbial communities, however, the effect of this farming practice on cotton rhizosphere microbiota remains poorly understood. This study investigated rhizosphere bacteria and soil functionality in response to different irrigation practices —including how changes in rhizosphere bacterial diversity alter soil nutrient cycling. Drip irrigation under plastic film mulch was shown to enhance bacterial diversity by lowering the salinity and increasing the soil moisture. However, the reduced root biomass and soluble sugar content of roots decreased potential copiotrophic taxa, such as Bacteroidetes, Firmicutes, and Gamma-proteobacteria, and increased potential oligotrophic taxa, such as Actinobacteria, Acidobacteria, and Armatimonadetes. A core network module was strongly correlated with the functional potential of soil. This module not only contained most of the keystone taxa but also comprised taxa belonging to Planctomycetaceae, Gemmatimonadaceae, Nitrosomonadaceae, and Rhodospirillaceae that were positively associated with functional genes involved in nutrient cycling. Drip irrigation significantly decreased the richness of the core module and reduced the functional potential of soil in the rhizosphere. Overall, this study provides evidence that drip irrigation under plastic film mulch alters the core bacterial network module and suppresses soil nutrient cycling.
Introduction
Cotton (Gossypium hirsutum L.) is an important crop in both the agriculture and textile industry (Constable and Bange, 2015). Xinjiang is the largest cotton-producing region in China with a cotton planting area of approximately 2.5 million hectares (ha) (NBSC, 2020). As a result of low temperatures that occur during the seedling stage and water shortages, the combination of drip irrigation and plastic film mulch is extensively used to increase topsoil temperature and save water in dryland farming areas (Zong et al., 2021). Plastic film mulching is applied nearly in all cotton fields in Xinjiang; and cotton fields that use drip irrigation under plastic film mulch currently comprise a nearly 1.2 million ha area (NBSC, 2020). However, compared to traditional flood irrigation with film mulch, this practice reduces root biomass and length (from early flowering stage) and often causes premature senescence in cotton (Mai et al., 2014). In addition, drip irrigation alters the nutrient content in rhizosphere soils (Zhu et al., 2013). This is particularly important because changes in root traits and rhizosphere conditions can affect rhizosphere microbes that are vital for plant growth and fitness (Berendsen et al., 2012; Zhang et al., 2017). While previous studies have explored the effects of drip irrigation on cotton yield, less attention had been paid to its influence on soil biological properties, especially on the rhizosphere microbial community. Thus, knowledge of how rhizosphere soil conditions under drip irrigation alter the rhizosphere microbiome and affect microbially-driven soil functions remains limited.
Rhizosphere microbes determine plant nutrient utilization by actively participating in carbon or nitrogen fixing and releasing available micronutrients. As a result, changes in rhizosphere microbes can influence the development of plants (Zhang et al., 2017). In practice, drip irrigation under plastic film mulch limits primary root development (Mai et al., 2014), and enriches -N in rhizosphere soils (Zhu et al., 2013), potentially impacting the structure and function of rhizosphere microbes. For example, variations in root biomass and length influence the rhizosphere priming effect and the subsequent release of labile organic substances (Zhang et al., 2017). Labile organic substances promote the growth of microbial r-strategist groups such as Beta-proteobacteria, Gamma-proteobacteria, Firmicutes, Gemmatimonadetes, and Bacteroidetes (Zhou et al., 2018) and are linked to the decomposition of soil organic matter and solubilization of insoluble minerals by rhizosphere microbes (Zhang et al., 2017). Soil available N also affects cbbL-carrying autotrophic bacterial composition (Zhou et al., 2019), and N fertilizers suppress various diazotrophic taxa and N fixation rates (Fan et al., 2019). It should be noted that drip irrigation under plastic film mulch increases the amount of residual membrane, which can impose selective pressure on distinct rhizosphere bacterial taxa (Qi et al., 2020). Thus, drip irrigation under plastic film mulch can induce complex rhizosphere conditions and change the characteristics of the rhizosphere microbiome.
Complex potential associations among thousands of soil organisms can be visualized as networks within the microbial community. Networks reveal the factors driving microbial associations in response to environmental disturbance, and provide critical information on microbial taxa associated with soil functioning (Chaffron et al., 2010; Banerjee et al., 2018). For example, it has been found wheat rhizosphere fungal network modules composed of saprotrophs or pathogens; and the modules are affected by tillage (Li et al., 2021). Network analysis studies also have identified several keystone taxa that are critical to microbial community functioning (Banerjee et al., 2021; Zheng et al., 2021). One recent study found that taxa within the core module were positively associated with functional genes involved in maintaining soil nutrient cycling (Fan et al., 2021). Thus, it is worth investigating whether keystone species are also harbored within the core module. Manipulating the rhizosphere microbes can favor plants for sustainable agricultural gains (Zhang et al., 2017) so characterizing the key players involved in nutrient cycling is essential to plant production and supports soil functioning in a more managed ecosystem (Banerjee et al., 2018; Fan et al., 2021). This is especially important after the combined use of plastic film mulch and drip irrigation, which can alter plant traits and soil nutrient availability.
The current study uses three irrigation treatments (flooding irrigation with single plastic film mulch and drip irrigation under single or double plastic film mulch) during a 2-year field experiment in an arid region of China. Shifts in the bacterial community, functional genes, RubisCO activity, and potential rates of nitrification and denitrification were assessed to characterize the bacterial response to drip irrigation-induced effects on rhizosphere nutrient cycling. There were two main objectives: (i) to compare microbial responses to the three different irrigation practices and (ii) to evaluate the effect of irrigation practices on rhizosphere C and N cycling processes. It was hypothesized that keystone taxa in the core module are linked to soil nutrient cycling processes, and drip irrigation under plastic film mulch affects soil functional potential by influencing the core network module.
Materials and methods
Experimental design and sampling
A 2-year field experiment was initially established in 2019 at YuShuGou in the Xinjiang Uyghur Autonomous Region of China (44°09′59″N, 87°59′56″E). This area has a typical temperate continental climate with an average annual precipitation of 190 mm and an average annual temperature of 6.8°C. The experiment consisted of three treatments: flooding irrigation with film mulch (FSM), drip irrigation with single film mulch (DSM), and drip irrigation with double film mulch (DDM) (Figure S1). Each treatment had six replicates (8 m × 20 m per plot for each replicate) arranged in a randomized block design, equaling 18 plots in total with guard rows (width of 1 m) between each plot. Nitrogen fertilizer and nitrogen fertilizer with water applications were managed as described by Mai et al. (2014). In brief, 4000 m3 water/hm2 and 340 kg urea/hm2 were applied each year for drip irrigation with mulch film, and 6000 m3 water/hm2 and 400 kg urea/hm2 were used each year for flooding irrigation with mulch film. The rates and dates of water and fertilizer application were consistent with local cotton agronomic practices (Table S1).
Plants and soil were sampled on 20 August 2020 at the fruiting stage of the cotton (about 18 weeks after sowing) and rhizosphere soil samples were collected as described previously (Mo et al., 2019). To ensure that the sample size of rhizosphere soil was similar and comparable across treatments, roots were collected within a 20-cm soil layer. Loose soil was obtained to filter out film residue. During sample collection, the rhizosphere soil was carefully scraped off the root surface using a sterile scalpel. The samples were then transported on ice to the laboratory for analysis. Some of the soil was stored at -80°C for DNA extraction and the remainder were stored at 4°C for chemical analyses.
Plant traits and soil physicochemical properties
For each treatment, 120 plant samples (20 for each replicate) were dried to a constant weight to determine the biomass. Some of the cotton root samples (10 for each plot) were also used to measure biochemical traits (Wang and Huang, 2015). Phenol content was estimated using the Folin–Ciocalteu assay, soluble sugar content was assessed using Anthrone colorimetry, free amino acids were measured using Ninhydrin colorimetry, and the absorbance was determined using an ultraviolet (UV) spectrophotometer (Cary 60, Agilent Technologies, USA). Soil physicochemical properties, including soil soluble salt content, pH, soil organic matter, nitrate-nitrogen, and ammonium nitrogen, were measured as previously described (Zhao et al., 2019; Zhao et al., 2020). Water-extractable organic carbon (WEOC) was determined as outlined by Zhu et al. (2020).
Analyses of the soil carbon-fixation, nitrification, and denitrification potentials
RubisCO activity was determined as described by Wu et al. (2015). In brief, a soil solution was mixed with the reaction system, and a preparation lacking RuBP was used as a blank. Ribulose bisphosphate (50 μL, 25 mM) was added to the mixture for 30 s, the absorbance was measured at 340 nm, and the RubisCO activity (nmol CO2 min -1 per kg dry soil) was calculated. Soil potential nitrification (PNR) was assessed according to a method described by Stark and Firestone (1995). In brief, field-moist soil (10 g) was added to a 250 ml Erlenmeyer flask with 100 ml nitrification solution consisting of KH2PO4 (280 mM), K2HPO4 (720 mM), and (NH4)2SO4 (500 mM). The samples were continuously shaken at 110 rpm and 10 ml of each sample solution was collected at 24, 48, 72, 96, 130, and 154 hours, filtered, and immediately stored at -20° C until analysis. NH4+-N and -N concentrations were then determined. The denitrification potential (DEA) was tested as described by Griffiths et al. (1998). In brief, 10 g of soil was weighed into a glass container that received 10 ml of a solution containing 1 mM KNO3 and 1 mM glucose. High purity (99.99%) helium was flushed into the container to create anaerobic conditions under atmospheric pressure. For DEA samples, 10 ml of gas was taken out and replaced by injecting 10 ml of acetylene. The slurries were shaken at 100 rpm for 154 h and the gas samples were analyzed. DEA rates were determined for each sample by calculating the slope of the linear regression model at four time points.
DNA extraction and quantitative PCR
Total soil DNA from each sample was extracted using the E.Z.N.A. Soil DNA kit (Omega, USA) and diluted in TE buffer (10 mM Tris-HCl, 1 mM EDTA, pH 7.0). The abundance of each functional gene, cbbL, β-glu, ChiA, nifH, AOB-amoA, narG, nirK, and cnorB was determined by quantitative PCR (qPCR) using the LightCycler® 480 system (Roche Applied Science). A 20 μL reaction contained 10 μL SYBR Premix Ex Taq™ (Takara, Dalian, China), 0.4 μL of each forward and reverse primer, and 2 μL of template DNA (10 ng μL-1). Standard curves showed high correlation coefficients (R2 >0.98), and the PCR efficiency was at least 85% in all cases. Details regarding the primers, thermal cycling conditions, and quality assessment are provided in Table S2.
High-throughput sequencing
The hypervariable V4-V5 region of the bacterial 16S rRNA gene was amplified using 515F (5’-GTGCCAGCMGCCGCGG-3’) and 907R (5’-CCGTCAATTCMTTTRAGTTT-3’) primers (Yusoff et al., 2013). The 25 μL PCR reactions contained 1 μL of each primer (10 μM), 2 μL DNA (10 ng μL−1), and 22 μL Platinum PCR SuperMix (Invitrogen, Shanghai, China). The thermal program was as follows: 95°C for 3 min, followed by 27 cycles at 95°C for 30 s, 50°C for 45 s, and 72°C for 1 min with a final extension at 72°C for 10 min. Sequencing was performed on the Illumina MiSeq platform at Majorbio BioPharm Technology Co., Ltd. (Shanghai, China), and the raw sequences were deposited in GenBank under the BioProject PRJNA793144. Using QIIME 1.9.0, the raw data was processed and trimmed (http://qiime.org; Caporaso et al., 2010). Low-quality reads (length<200 bp and mean quality score<30) were removed, while reads that overlapped by >10 bp were assembled. Chimeras were removed using the Uchime algorithm software (Edgar et al., 2011). The sequences were clustered into operational taxonomic units (OTUs) using QIIME’s pick_open_reference_otus.py with a 97% similarity cut-off. Taxonomic information was obtained using the Ribosomal Database Project classifier at a confidence threshold of 0.80 (Cole et al., 2004). In total, 936,467 effective sequences were retained after sequence information from 18 soil samples was optimized. The reads were rarefied at a 28,795-sequencing depth for each sample.
Co-occurrence network analyses and core module identification
Microbial network analysis was used to identify clusters of microbial taxa that were highly correlated. The top OTUs, accounting for more than 80% of the relative abundance in the total community, were chosen. All pair-wise Spearman correlations between OTUs were calculated using the “Hmsic” package (Harell and Dupont, 2015) in R. To focus on OTUs that strongly co-occurred and were more likely to interact, correlations with a Spearman’s coefficient<0.8 and a P-value >0.01 were removed. The main modules in the network were visualized using Gephi (https://gephi.org/). The relative abundance of each module was calculated by averaging the standardized relative abundances (z-score) of the species to which it belonged. Modules in which taxa correlated positively with the abundance of soil functioning were also identified (Fan et al., 2021). The top 20 nodes with high degree, high closeness centrality, and low betweenness centrality scores were statistically identified as the keystone taxa (Banerjee et al., 2021; Zheng et al., 2021).
Whole bacterial genome downloading and annotation
We selected 494 bacterial genomes from the JGI-IMG database that were previously retrieved from agricultural soils (including rhizosphere and rhizoplane samples) and retrieved their sequences from the NCBI genome database (ftp://ftp.ncbi.nlm.nih.gov/genomes/all/). Finally, a total of 2209 16S-rRNA sequences were extracted from 494 genomes (file format: _rna_from_genomic.fna.gz) using “TBtools” software (Chen et al., 2020). Online BLAST (Nucleotide BLAST: Align two or more sequences using BLAST (nih.gov)) was used to compare the representative sequences of chosen dominant bacterial OTUs (with >97% identity across the sequenced regions of the 16S rRNA genes). In total, there were 112 genomes (Table S3) with small subunit (ssu) rRNA 16S marker genes that could be aligned and built into a phylogenetic tree using MEGA-X and iTOL, within which, 41, 53, and 18 genomes were clustered with the dominant OTUs from Modules #1–3, respectively. The Prokka pipeline was used for gene prediction and annotation; and genes associated with carbon cycling (e.g., abfA, accA, acsA, acsE), and genes associated with nitrogen cycling (e.g., anfG, hao, hdhA, napA) were used as reference to assess potential functions (Fan et al., 2021).
Statistical analyses
The alpha diversity was calculated using the diversity function in the “vegan” R package (Oksanen et al., 2020). Non-metric multidimensional scaling (NMDS) analysis and permutational multivariate analysis (ADONIS) were conducted using the “vegan” package (Oksanen et al., 2020). These used current literature on cultured strains to map prokaryotic clades (e.g., genera or species) to establish metabolic or other ecologically relevant functions (Louca et al., 2016). The hierarchy from phyla to OTUs was illustrated using hierarchical (i.e., tree topology) taxonomic networks in Cytoscape (version: 3.8.0) with manual adjustment (https://apps.cytoscape.org/apps/yfileslayoutalgorithms). Pairwise Spearman correlations between diversity and soil functional potentials [normalized abundance of tested functional genes, carbon-fixation potential, PNR, and DEA (z-score)] were calculated. A random forest analysis was used to estimate the importance of soil properties to explain the carbon-fixation potential, PNR, and DEA rates using the “rfPermute” R package (Eric Archer, 2021). A one-way analysis of variance (ANOVA) with Tukey’s test was conducted for multi-comparisons using R software (version 4.1.2-win).
Results
Changes in soil properties across irrigation practices
Significantly higher (p<0.05) soil moisture, -N, and -N were observed in the rhizosphere soil of the DSM or DDM treatment groups than in the FSM group (Table S4). Higher moisture was also associated with lower salinity and pH in the rhizosphere soil of the DSM or DDM groups. Drip irrigation promoted aboveground biomass, however, the limited moist area promoted shallow root distribution (Figure S1), resulting in lower root biomass (Table S5). A significantly lower (p<0.05) soluble sugar content in the roots and a lower WEOC in the rhizosphere soil were observed from drip irrigation practices (Tables S4 and S5), suggesting that low rhizodeposition might exist under these two treatments.
Rhizosphere bacterial communities and functional potentials across irrigation practices
Bacterial diversity was significantly lower (p<0.05) in the FSM than in the DDM treatment groups (Table S6). Indeed, permutational multivariate analysis (ADONIS) confirmed that the rhizosphere communities differed significantly in response to flooding irrigation versus drip irrigation (Figure S2; Table S7).
Taxa responsible for the observed shifts in β-diversity were further explored at various taxonomic levels from phylum to OTU. Practice-sensitive OTUs were broadly distributed across the taxonomic hierarchy with phyla such as Planctomyces, Actinobacteria, Bacteroidetes, and Gemmatimonadetes exhibiting a clear dispersal of sensitive OTUs (Figure 1). Qualitatively, Bacteroidetes, Omnitrophica, Planctomyces, Gemmatimonadetes, and Gemmatimonadetes had fewer OTUs in the DDM than in the FSM treatment group (Figure 1). Consistent phylum-level differences in the bacterial community were observed among the three irrigation practices (Figure 2). For example, the phyla Actinobacteria, Acidobacteria, and Armatimonadetes showed strong increases in relative abundance under drip irrigation while Bacteroidetes, Gemmatimonadetes, and Firmicutes were more abundant in the rhizosphere soil that received flooding irrigation.
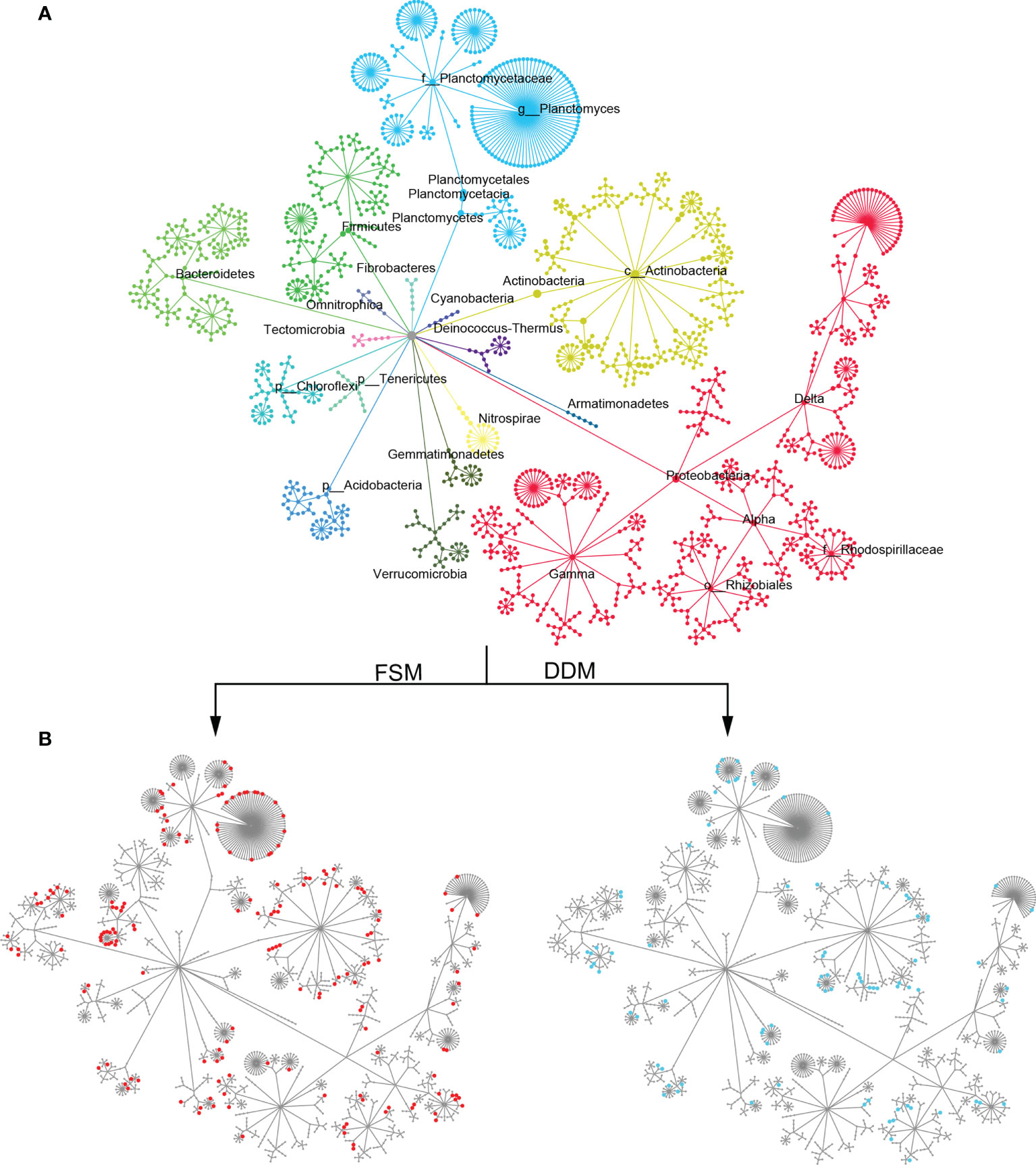
Figure 1 (A) A hierarchical taxonomic tree of bacterial communities showing OTU distribution across the different phyla. Nodes correspond to OTUs and edges represent the taxonomic path from the phylum to the OTU level. OTUs were placed at the level with the lowest possible assignment. Clusters are color-coded by their phylum-level assignment and are labeled with the phylum name. (B) A taxonomic tree with the same topology as shown in panel A, but with node sizes that correspond to the positive relative change (z-transformed) in abundance under flooding irrigation with film mulch (FSM) or drip irrigation with double film mulch (DDM) stands. Node colors correspond to the level of significance from highly significant (red/blue) to not significant (gray). A soft threshold using a color gradient rather than a hard cutoff was used to denote the level of significance. Nodes with p ≤0.05 are completely red/blue.
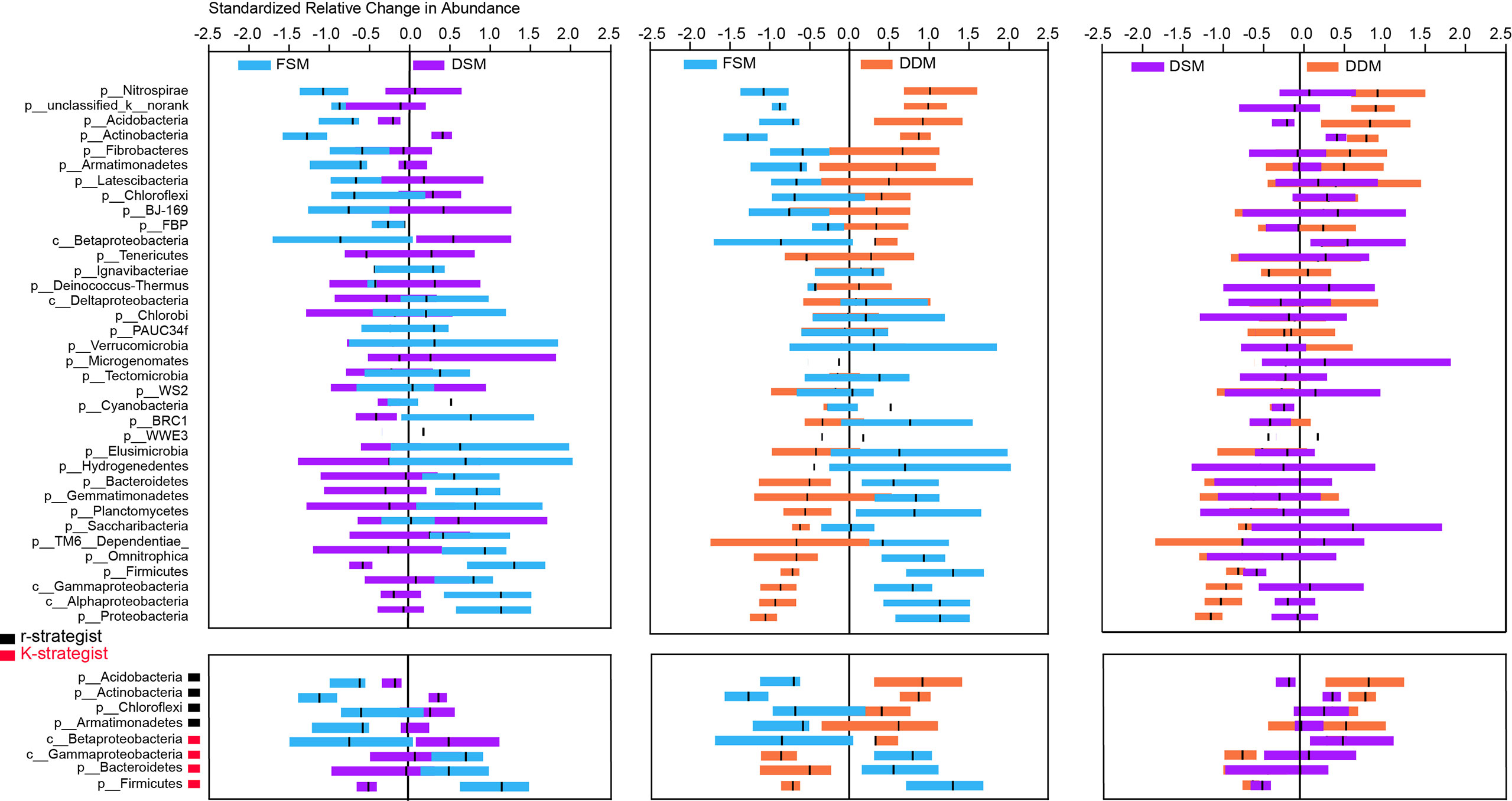
Figure 2 Relative changes (z-transformed) in the abundance of bacterial phyla (upper panel) as well as the major K-strategist and r-strategist groups (lower panel). K-strategist groups: Acidobacteria, Actinobacteria, Chloroflexi; r-strategist groups: Betaproteobacteria, Gammaproteobacteria, Bacteroidetes, Firmicutes.
The abundance of the functional genes, β-glu, cbbL nifH, AOB-amoA, and cnorB was significantly lower under drip irrigation than under flooding irrigation (Figure S3). Meanwhile, lower RubisCO activity, nitrification, and denitrification were observed in response to drip irrigation (Figure S3). The process (i.e., RubisCO activity, PNR, and DEA) was correlated with soil WEOC, SOC, total N, and -N levels (Figure S4).
Network modules involved in soil functions
Three main modules (Modules #1–3) were identified in the network (Figure 3A). The relative abundance of taxa belonging to Module #1 was significantly and positively correlated with the abundance of functional genes related to N and C cycling (Figure 3D). Given its functional importance, Module #1 will be referred to as the core module. The core module was dominated by Actinobacteria, Proteobacteria, Chloroflexi, Planctomycetes, and Firmicutes (Figures 3B, C), among which there were many positive correlations (density = 0.15) (Figure 3B). The relative abundance of the core module was lower in response to drip irrigation (Figure S5). The relative abundance of dominant taxa in Modules #2 and #3 showed fewer direct correlations with the abundance of functional genes than was found in the core module (Figure 3D). The associations between other modules and functional potential are shown in Figure S6. Of the 20 keystone species, 13 were in the core module (Table S8). They primarily belonged to Chloroflexi (Caldilineaceae and unclassified Chloroflexi), Actinobacteria (Blastocatellaceae subgroup 4 and unclassified Actinobacteria), and Gemmatimonadetes (Gemmatimonadaceae). Other members belonged to Planctomycetes, Nitrospirae, and Proteobacteria. Keystone taxa in Module #2 included members of Myxococcales, Cytophagales, and Rhizobiales (Table S8).
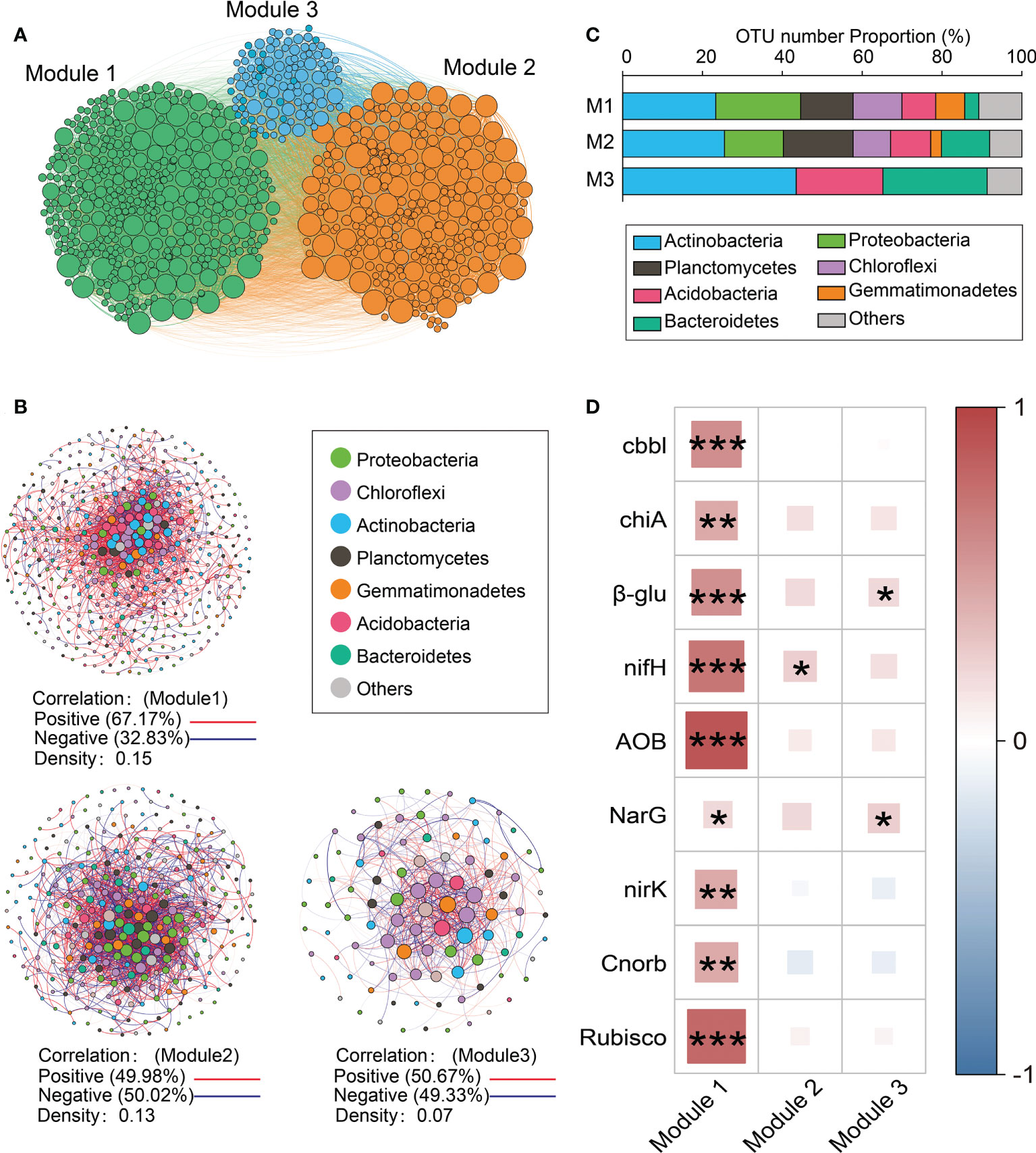
Figure 3 Modules based on networks. (A) A Network diagram with nodes colored according to each of the three main modules (Modules #1–3); (B) Operational taxonomic unit (OTU) number properties of the dominant taxa in the main modules; (C) Dominant taxa (relative abundance >0.05) from Modules #1–3, respectively. A connection stands for a strong (Spearman’s r >0.8) and significant (P<0.001) correlation. The co-occurrence network is colored by phylum. The size of each node is proportional to the relative abundance of each taxa. A red edge indicates a positive correlation between two individual nodes, while a blue edge indicates a negative correlation; (D) Spearman correlations between the abundance of functional genes and the relative abundance of dominant taxa from Modules #1–3 (z-score). *P < 0.05, **P < 0.01, ***P < 0.001.
Relationships between key taxa and soil functions
Further phylogenetic analysis showed that the key taxa (major taxa in Module #1) were more often phylogenetically clustered within specific bacterial taxa (e.g., Actinobacteria: Actinomycetia; Planctomycetes: Planctomycetaceae; Gemmatimonadetes: Gemmatimonadaceae; Alpha-proteobacteria: Rhodobacteraceae, Beta-proteobacteria: Nitrosomonadaceae) than the dominant taxa in other modules (Figure 4). The normalized per genome copy numbers of functional genes were significantly higher in the genomes clustered from key taxa than those clustered within the other two modules (Figure 4). In addition, the richness of the core module had a significantly positive effect on the functional potentials (Figure 5). We used additional examples to illustrate the link between genetic function and taxa in the core module (Figure S7). Specifically, the abundance of the cbbL and nifH genes correlated significantly with the richness of Alpha- and Gamma-proteobacteria and the richness of key taxa including Verrucomicrobia, Planctomycetes and Gemmatimonadetes correlated significantly with the abundance of β-glu, AOB-amoA, and cnorB.
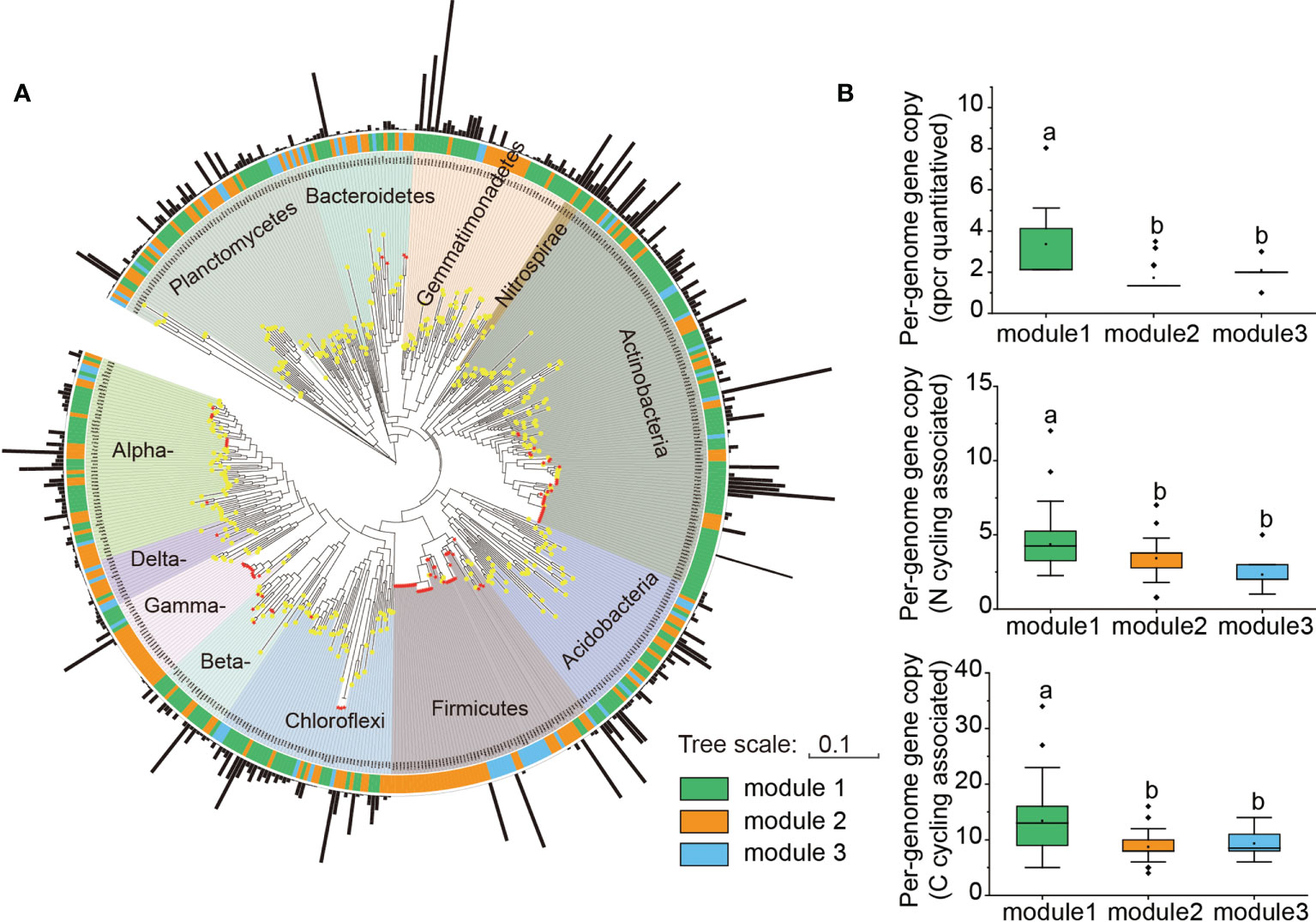
Figure 4 Phylogenetic properties and per-genome gene copies of mapped taxa from different modules. (A) Phylogenetic tree of dominant OTUs from Modules, and 35 whole genomes (with >97% identity across the sequenced region of the 16S rRNA gene) downloaded from the JGI-IMG websites (https://img.jgi.doe.gov/). Black bar: relative abundance of each OTU. Red star on the tree: ssu rRNA 16S marker genes of the whole genomes. (B) The normalized per-genome copy numbers of eight tested genes, and other reference genes associated with carbon (18) and nitrogen (14). The normalized copy numbers are shown as the percentage (%) of genes per genome. The diamond symbol in each box plot represents the mean value.
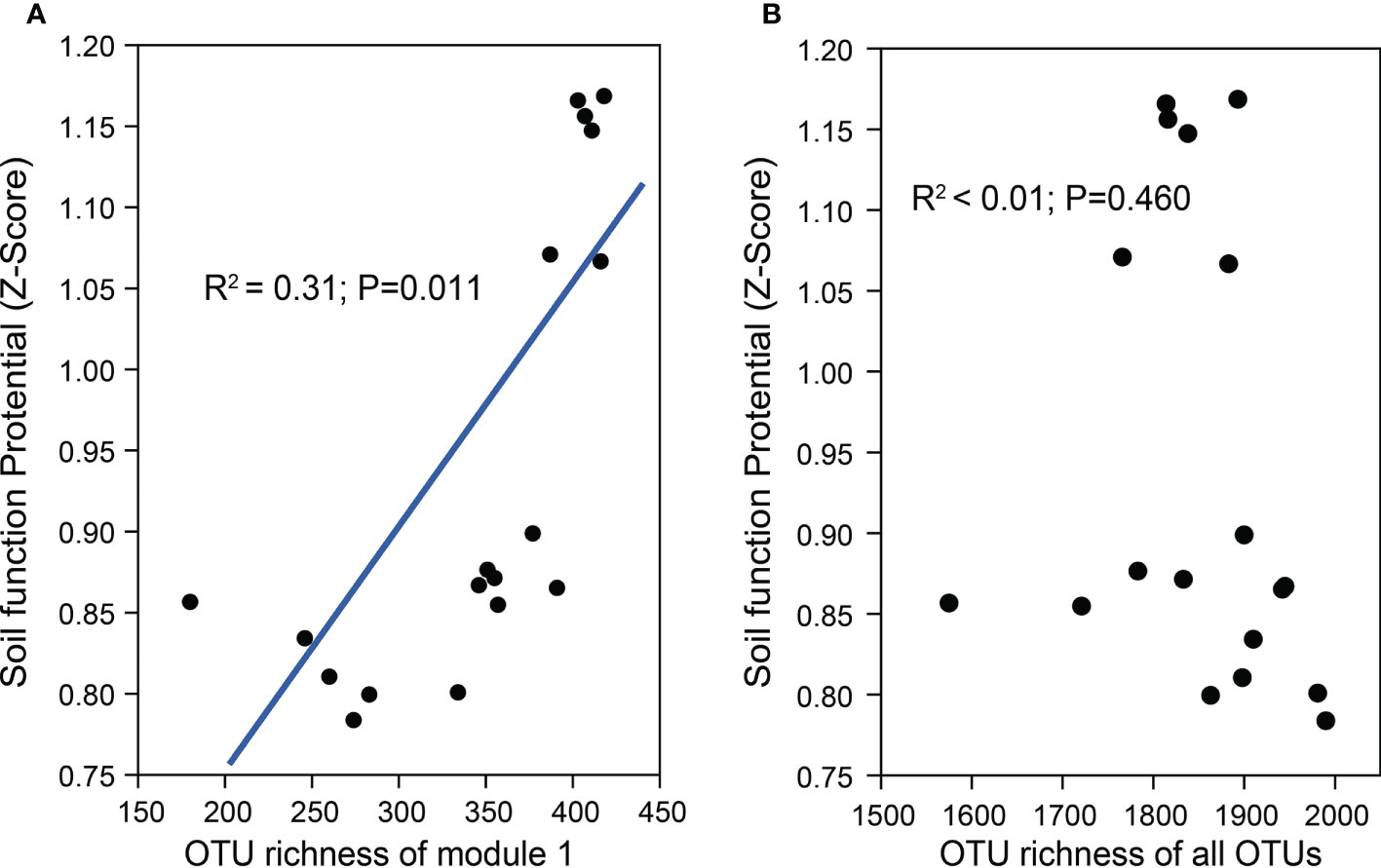
Figure 5 The regression relationships between the richness of the core module (Module #1) (A), the richness of the whole community (B), and the soil functional potentials [normalized abundance of test functional genes, RubisCO activity, PNR, and DEA (z-score)].
Discussion
Distinct responses of bacterial communities to irrigation practices
There were only subtle differences in bacterial α-diversity between the two drip irrigation practices. However, the Shannon indices of both drip irrigation practices were significantly higher than the index associated with flooding irrigation (Table S6), suggesting that drip irrigation is associated with increased rhizosphere bacterial diversity. This is not surprising given that lower salinity and high moisture are suitable for bacterial growth. At the phylum level, Bacteroidetes, Firmicutes, and Gamma-proteobacteria were more abundant in response to flooding irrigation, while Actinobacteria, Acidobacteria, and Armatimonadetes were more abundant under drip irrigation (Figure 1). The K - and - r selection theory (Fierer et al., 2007) may partially explain shifts in taxonomic groups. For example, Actinobacteria play a vital role in the soil C-cycle by degrading recalcitrant carbon and are highly resistant to C starvation (Rosenberg et al., 2014; Hartmann et al., 2017). Acidobacteria and Armatimonadetes are also able to metabolize complex carbohydrates (Kielak et al., 2016; Hartmann et al., 2017). These K-strategist groups often dominate in soils with low carbon availability (Ali et al., 2018; Razanamalala et al., 2018). In contrast, fast-growing r-strategist organisms such as Gamma-proteobacteria, Bacteroidetes and Firmicutes predominate in soils with highly labile organic substrates (Fierer et al., 2007; Fierer et al., 2012; Li et al., 2019). Taken together, it is likely that decreased carbon availability (e.g., SOC, WEOC) under drip irrigation allowed K-strategists to be outcompeted in the rhizosphere. However, it was notable that Beta-proteobacteria, which is considered an r-strategist (Fierer et al., 2007), was highly abundant in response to drip irrigation (Figure 2). Thus, an assessment of the effects of different irrigation treatments on the bacterial activity at different levels is necessary for a more complete understanding of the environment.
Core module association with soil functions
The richness of bacterial taxa in the core module (Module #1), including Alpha-, Beta- and Gamma-proteobacteria, Ardenticatenia, Verrucomicrobia, Planctomycetes, and Gemmatimonadetes was positively associated with the abundance of functional genes (Figure 3). Alpha- and Gamma-proteobacteria were the common autotrophic bacterial classes (Xiao et al., 2018). Some taxa from these two classes that were included in the current study, Paracoccus and Dokdonella, are important contributors to microbial CO2 assimilation (Zhou et al., 2019), while other taxa, including Azospirillum, Bradyrhizobium, and Rhodospirillaceae are N fixers (Nelson et al., 2016). Moreover, the taxa of Nitrosomonadaceae (Nitrosomonadaceae) from the core module, are shown to contain ammonia oxidizers (Prosser et al., 2014). Ardenticatenia belongs to Chloroflexi and members of this phylum are positively correlated with simple carbohydrate metabolic pathways (Pérez Castro et al., 2019). Verrucomicrobia, Planctomycetes, and Gemmatimonadetes are significantly positively correlated with the abundance of functional genes including those involved in C fixation, C degradation, and N cycling (Fierer et al., 2013; Shi et al., 2020). Phyla Gemmatimonadetes and Verrucomicrobia are also considered as N cycle-related bacteria and are enriched in healthy plant rhizosphere (Ge et al., 2021). Taxa from the core module also had higher normalized per-genome copy numbers of functional genes associated with C and N cycling (Figure 4), suggesting that this module had greater nutrient cycling potential.
It is important that most of the keystone taxa in this study were associated with the core module (Table S8). The disappearance of keystone taxa may cause disruptions in network modules (Guimerà and Nunes Amaral, 2005), suggesting that the core module is critical for maintaining network structure and soil functioning in the rhizosphere. Other keystone taxa, including those from Rhizobiales, Myxococcales, and Cytophagaceae, exist in Module #2. Rhizobiales members are found as keystone taxa across different ecosystems (Banerjee et al., 2018). Myxococcales and Cytophagaceae (Cytophagales) are groups known for their predatory lifestyle (Emmett et al., 2021), which may explain why Module #2 had the highest negative correlation.
Drip irrigation practices reduced core module richness and soil functioning
Drip irrigation also reduced the rhizosphere soil functional potential by affecting the richness of the core module (Figure 5). Many variations caused by drip irrigation were able to inhibit microbial extracellular enzyme and functional gene expression. For example, two labile carbon hydrolase-coding genes, β-glu and chi-A, decreased by different amounts, suggesting that drip irrigation may reduce the production of the corresponding extracellular enzymes, and lower the efficiency of labile carbon decomposition. This result may be explained by the reduced richness of the Verrucomicrobia, Planctomycetes, and Gemmatimonadetes taxa. In addition, cbbL-carrying obligate autotrophs, not facultative autotrophs, are strongly and positively correlated with RubisCO activity (Yuan et al., 2012a; Yuan et al., 2012b; Wu et al., 2015; Lynn et al., 2017). Available N (e.g., -N) decreases soil RubisCO activity by reducing obligate autotrophs (Zhou et al., 2019). Thus, the accumulation of -N under drip irrigation may decrease soil obligate autotroph abundance. According to previous studies, N fertilizes against N fixation and specific groups of N fixers (Fan et al., 2019) and high is negatively correlated with the abundance of denitrifying microbes (Shen et al., 2010). In addition, soil carbon is critical for the activity of denitrifiers, and denitrification is usually found in soils with both a high TOC and moisture content (Enwall et al., 2010; Banerjee and Siciliano, 2012; Schulz et al., 2017). In the current study, drip irrigation enriched -N but decreased SOC and WEOC, which could be because fewer key taxa were involved in N fixation or nitrification and denitrification.
In summary, this study built a theoretical framework to characterize the effects of drip irrigation with film mulch on the rhizosphere bacterial communities (Figure 6). Results showed that rhizosphere bacteria, which benefit from reduced salinity and moisture, may promote community diversity, while limited carbon substrate availability caused many K-strategist groups to occupy the communities. Furthermore, WEOC, SOC, and -N induced under drip irrigation may reduce the richness of the core module, which could suppress the biogeochemical cycling of C and N. It should be noted, however, that plant and soil sampling was only conducted during one growth stage in this study. Rhizosphere fungal communities can be impacted by the interactions between tillage practices and growth stage (Li et al., 2021). In addition, beneficial and tolerant microbes can be stimulated by certain long-term agricultural management practices (Fan et al., 2021). Thus, it will be necessary to evaluate the temporal consistency of these findings.
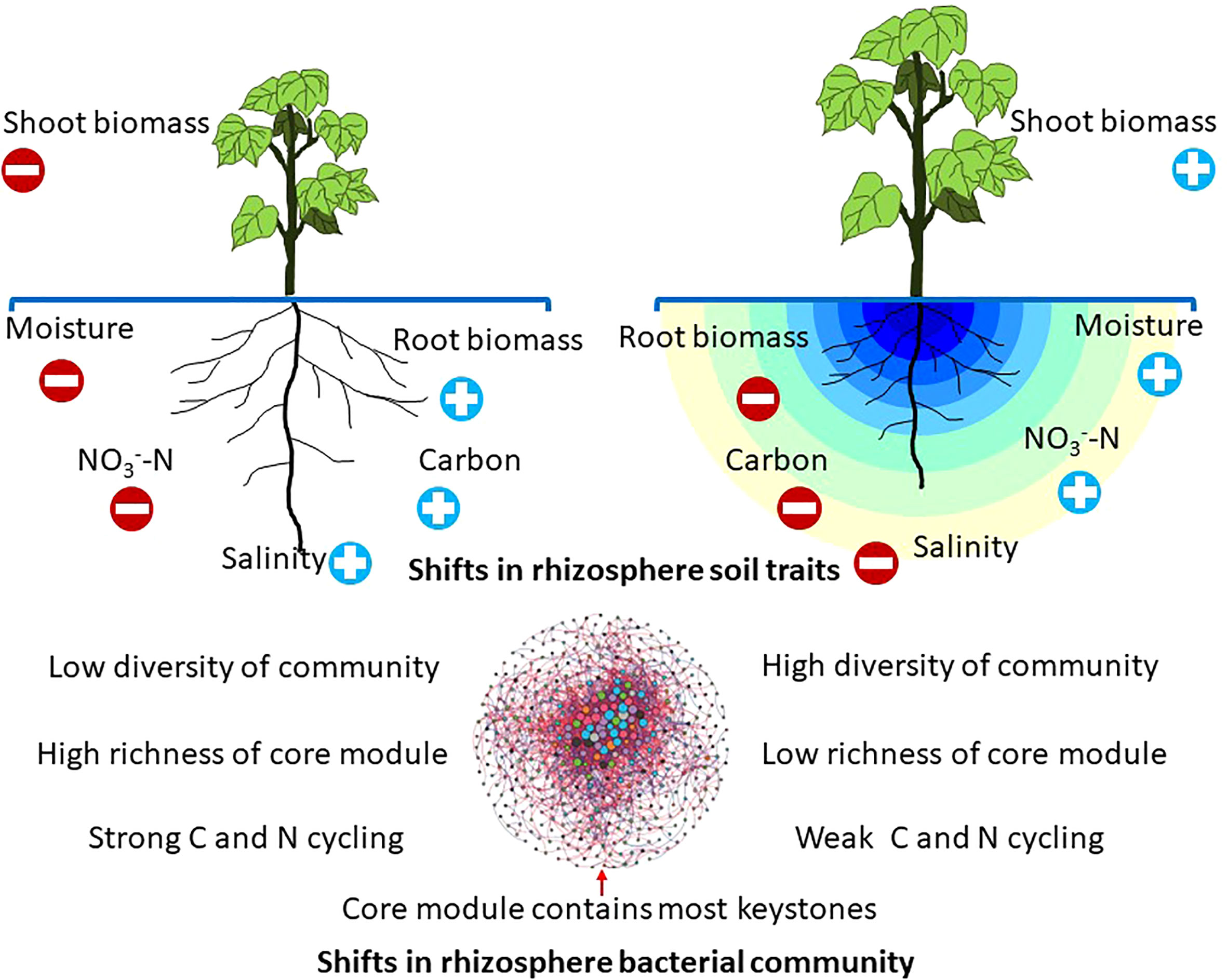
Figure 6 A theoretical framework exploring the effects of different practices on bacteria (structure, functional potential) in relation to multiple factors. Practices affect plant growth and rhizosphere soils, which in turn influence the rhizosphere bacterial community. The richness of the core module can be altered by agricultural practice. The pivotal changes in the bacteria-driven nutrient process are presented.
Conclusions
This study revealed a strong effect of drip irrigation on the rhizosphere bacterial community of cotton. While this practice enhanced bacterial diversity, changes in the quantity and/or quality of plant-derived inputs, such as root biomass and carbon, and -N concentration may suppress the richness of the core module and its associated functional C and N cycling potentials. These findings contribute to our understanding of the linkage between functional potentials and the rhizosphere bacterial community under different irrigation practices.
Data availability statement
The datasets presented in this study can be found in online repositories. The names of the repository/repositories and accession number(s) can be found below: https://www.ncbi.nlm.nih.gov/genbank/, PRJNA793144.
Author contributions
SZ and CT: Conceptualization, Investigation. SZ and BP: Software, Writing- Original draft preparation. WM: Formal analysis. SB and SZ: Validation, Writing - Review and Editing. All authors contributed to the article and approved the submitted version.
Funding
This work was supported by the National Natural Science Foundation of China (grant nos. 31971448 and U1803233), the Foundation of Science & Technology Department of Xinjiang Uygur Autonomous Region (No. 2019XS28), the “Western young scholars” project (Grant NO. 2019-XBQNXZ-A-006), the research programs (grant nos. 2020DB001, 131965KYSB20190083 and ZD2022D001) and the Youth Innovation Promotion Association CAS (grant no. 2020433).
Acknowledgments
We thank the editor and reviewers for their constructive suggestions and insightful comments. We are grateful to Dr. Ke Zhang and Dr. Shoule Wang for their help in collecting samples.
Conflict of interest
The authors declare that the research was conducted in the absence of any commercial or financial relationships that could be construed as a potential conflict of interest.
Publisher’s note
All claims expressed in this article are solely those of the authors and do not necessarily represent those of their affiliated organizations, or those of the publisher, the editors and the reviewers. Any product that may be evaluated in this article, or claim that may be made by its manufacturer, is not guaranteed or endorsed by the publisher.
Supplementary material
The Supplementary Material for this article can be found online at: https://www.frontiersin.org/articles/10.3389/fpls.2022.973919/full#supplementary-material
References
Ali, R. S., Poll, C., Kandeler, E. (2018). Dynamics of soil respiration and microbial communities: Interactive controls of temperature and substrate quality. Soil Biol. Biochem. 127, 60–70. doi: 10.1016/j.soilbio.2018.09.010
Archer, E. (2021). rfPermute: Estimate permutation p-values for random forest importance metrics. R package version 2.5. Available at: https://CRAN.R-project.org/package=rfPermute.
Banerjee, S., Schlaeppi, K., van der Heijden, M. G. A. (2018). Keystone taxa as drivers of microbiome structure and functioning. Nat. Rev. Microbiol. 16, 567–576. doi: 10.1038/s41579-018-0024-1
Banerjee, S., Siciliano, S. D. (2012). Spatially tripartite interactions of denitrifiers in arctic ecosystems: Activities, functional groups and soil resources: Denitrifier spatial patterns in arctic soils. Environ. Microbiol. 14, 2601–2613. doi: 10.1111/j.1462-2920.2012.02814.x
Banerjee, S., Zhao, C., Kirkby, C. A., Coggins, S., Zhao, S., Bissett, A., et al. (2021). Microbial interkingdom associations across soil depths reveal network connectivity and keystone taxa linked to soil fine-fraction carbon content. Agric. Ecosyst. Environ. 320, 107559. doi: 10.1016/j.agee.2021.107559
Berendsen, R. L., Pieterse, C. M. J., Bakker, P. A. H. M. (2012). The rhizosphere microbiome and plant health. Trends Plant Sci. 17, 478–486. doi: 10.1016/j.tplants.2012.04.001
Caporaso, J. G., Kuczynski, J., Stombaugh, J., Bittinger, K., Bushman, F. D., Costello, E. K., et al. (2010). QIIME allows analysis of high-throughput community sequencing data. Nat Methods. 7, 335–336. doi: 10.1038/nmeth.f.303
Chaffron, S., Rehrauer, H., Pernthaler, J., von Mering, C. (2010). A global network of coexisting microbes from environmental and whole-genome sequence data. Genome Res. 20, 947–959. doi: 10.1101/gr.104521.109
Chen, C. J., Chen, H., Zhang, Y., Thomas, H. R., Frank, M. H., He, Y. H., et al. (2020). TBtools: An integrative toolkit developed for interactive analyses of big biological. Data. Mol. Plant 13, 1194–1202. doi: 10.1016/j.molp.2020.06.009
Cole, J. R. (2004). The ribosomal database project (RDP-II): Sequences and tools for high-throughput rRNA analysis. Nucleic Acids Res. 33, D294–D296. doi: 10.1093/nar/gki038
Constable, G. A., Bange, M. P. (2015). The yield potential of cotton (Gossypium hirsutum l.). Field Crops Res. 182, 98–106. doi: 10.1016/j.fcr.2015.07.017
Edgar, R. C., Haas, B. J., Clemente, J. C., Quince, C., Knight, R. (2011). UCHIME improves sensitivity and speed of chimera detection. Bioinformatics 27, 2194–2200. doi: 10.1093/bioinformatics/btr381
Emmett, B. D., Lévesque-Tremblay, V., Harrison, M. J. (2021). Conserved and reproducible bacterial communities associate with extraradical hyphae of arbuscular mycorrhizal fungi. ISME J. 15, 2276–2288. doi: 10.1038/s41396-021-00920-2
Enwall, K., Throbäck, I. N., Stenberg, M., Söderström, M., Hallin, S. (2010). Soil resources influence spatial patterns of denitrifying communities at scales compatible with land management. Appl. Environ. Microbiol. 76, 2243–2250. doi: 10.1128/AEM.02197-09
Fan, K. K., Delgado-Baquerizo, M., Guo, X. S., Wang, D. Z., Wu, Y. Y., Zhu, M., et al. (2019). Suppressed n fixation and diazotrophs after four decades of fertilization. Microbiome 7, 143. doi: 10.1186/s40168-019-0757-8
Fan, K. K., Delgado-Baquerizo, M., Guo, X. S., Wang, D. Z., Zhu, Y. G., Chu, H. Y. (2021). Biodiversity of key-stone phylotypes determines crop production in a 4-decade fertilization experiment. ISME J. 15, 550–561. doi: 10.1038/s41396-020-00796-8
Fierer, N., Bradford, M. A., Jackson, R. B. (2007). Toward an ecological classification of soil bacteria. Ecology 88, 1354–1364. doi: 10.1890/05-1839
Fierer, N., Ladau, J., Clemente, J. C., Leff, J. W., Owens, S. M., Pollard, K. S., et al. (2013). Reconstructing the microbial diversity and function of pre-agricultural tallgrass prairie soils in the United States. Science 342, 621–624. doi: 10.1126/science.1243768
Fierer, N., Lauber, C. L., Ramirez, K. S., Zaneveld, J., Bradford, M. A., Knight, R. (2012). Comparative metagenomic, phylogenetic and physiological analyses of soil microbial communities across nitrogen gradients. ISME J. 6, 1007–1017. doi: 10.1038/ismej.2011.159
Ge, A. H., Liang, Z. H., Xiao, J. L., Zhang, Y., Zeng, Q., Xiong, C., et al. (2021). Microbial assembly and association network in watermelon rhizosphere after soil fumigation for fusarium wilt control. Agric. Ecosyst. Environ. 312, 107336. doi: 10.1016/j.agee.2021.107336
Griffiths, R. P., Homann, P. S., Riley, R. (1998). Denitrification enzyme activity of Douglas-fir and red alder forest soils of the pacific Northwest. Soil Biol. Biochem. 30, 1147–1157. doi: 10.1016/S0038-0717(97)00185-5
Guimerà, R., Nunes Amaral, L. A. (2005). Functional cartography of complex metabolic networks. Nature 433, 895–900. doi: 10.1038/nature03288
Harell, F.B.Jr, Dupont, C. (2015). Hmisc: Harrell miscellaneous. R Package Version 3.16-0. Available at: http://CRAN.R-project.org/package=Hmisc.
Hartmann, M., Brunner, I., Hagedorn, F., Bardgett, R. D., Stierli, B., Herzog, C., et al. (2017). A decade of irrigation transforms the soil microbiome of a semi-arid pine forest. Mol. Ecol. 26, 1190–1206. doi: 10.1111/mec.13995
Kielak, A. M., Barreto, C. C., Kowalchuk, G. A., van Veen, J. A., Kuramae, E. E. (2016). The ecology of acidobacteria: Moving beyond genes and genomes. Front. Microbiol. 7. doi: 10.3389/fmicb.2016.00744
Li, Y., Wang, Z., Li, T., Zhao, D., Han, J., Liao, Y. (2021). Wheat rhizosphere fungal community is affected by tillage and plant growth. Agric. Ecosyst. Environ. 317, 107475. doi: 10.1016/j.agee.2021.107475
Li, H., Zhang, Y. Y., Yang, S., Wang, Z. R., Feng, X., Liu, H. Y., et al. (2019). Variations in soil bacterial taxonomic profiles and putative functions in response to straw incorporation combined with n fertilization during the maize growing season. Agric. Ecosyst. Environ. 283, 106578. doi: 10.1016/j.agee.2019.106578
Louca, S., Parfrey, L. W., Doebeli, M. (2016). Decoupling function and taxonomy in the global ocean microbiome. Science 353, 1272–1277. doi: 10.1126/science.aaf4507
Lynn, T. M., Ge, T. D., Yuan, H. Z., Wei, X. M., Wu, X. H., Xiao, K. Q., et al. (2017). Soil carbon-fixation rates and associated bacterial diversity and abundance in three natural ecosystems. Microb. Ecol. 73, 645–657. doi: 10.1007/s00248-016-0890-x
Mai, W. X., Tian, C. Y., Lo, L. (2014). Localized salt accumulation: the main reason for cotton root length decrease during advanced growth stages under drip irrigation with mulch film in a saline soil. J. Arid Land 6, 361–370. doi: 10.1007/s40333-014-0001-0
Mo, F., Zhang, Y. Y., Li, T., Wang, Z. T., Yu, K. L., Wen, X. X., et al. (2019). Fate of photosynthesized carbon as regulated by long–term tillage management in a dryland wheat cropping system. Soil biol. Biochem 138, 107581. doi: 10.1016/j.soilbio.2019.107581
Nelson, M. B., Martiny, A. C., Martiny, J. B. H. (2016). Global biogeography of microbial nitrogen-cycling traits in soil. Proc. Natl. Acad. Sci. 113, 8033–8040. doi: 10.1073/pnas.1601070113
Oksanen, J., Blanchet, F. G., Friendly, M., Kindt, R., Legendre, P., McGlinn, D., et al. (2020). Vegan: Community ecology package. R package version 2.5-7. Available at: https://CRAN.R-project.org/package=vegan.
Pérez Castro, S., Cleland, E. E., Wagner, R., Sawad, R. A., Lipson, D. A. (2019). Soil microbial responses to drought and exotic plants shift carbon metabolism. ISME J. 13, 1776–1787. doi: 10.1038/s41396-019-0389-9
Prosser, J. ,. I., Head, I. M., Stein, L. Y. (2014). The family nitrosomonadaceae (Berlin Heidelberg: Springer). doi: 10.1007/978-3-642-30197-1_372
Qi, Y. L., Ossowicki, A., Yang, X. M., Huerta Lwanga, E., Dini-Andreote, F., Geissen, V., et al. (2020). Effects of plastic mulch film residues on wheat rhizosphere and soil properties. J. Hazard Mater. 387, 121711. doi: 10.1016/j.jhazmat.2019.121711
Razanamalala, K., Razafimbelo, T., Maron, P. A., Ranjard, L., Chemidlin, N., Lelièvre, M., et al. (2018). Soil microbial diversity drives the priming effect along climate gradients: A case study in Madagascar. ISME J. 12, 451–462. doi: 10.1038/ismej.2017.178
E., Rosenberg, DeLong, E. F., Lory, S., Stackebrandt, E., Thompson, F. (Eds.) (2014). The prokaryotes: Actinobacteria (Berlin, Heidelberg: Springer Berlin Heidelberg). doi: 10.1007/978-3-642-30138-4
Schulz, S., Kölbl, A., Ebli, M., Buegger, F., Schloter, M., Fiedler, S. (2017). Field-scale pattern of denitrifying microorganisms and N2O emission rates indicate a high potential for complete denitrification in an agriculturally used organic soil. Microb. Ecol. 74, 765–770. doi: 10.1007/s00248-017-0991-1
Shen, W. S., Lin, X. G., Shi, W. M., Min, J., Gao, N., Zhang, H. Y., et al. (2010). Higher rates of nitrogen fertilization decrease soil enzyme activities, microbial functional diversity and nitrification capacity in a Chinese polytunnel greenhouse vegetable land. Plant Soil 337, 137–150. doi: 10.1007/s11104-010-0511-2
Shi, Y., Delgado-Baquerizo, M., Li, Y. T., Yang, Y. F., Zhu, Y. G., Peñuelas, J., et al. (2020). Abundance of kinless hubs within soil microbial networks are associated with high functional potential in agricultural ecosystems. Environ. Int. 142, 105869. doi: 10.1016/j.envint.2020.105869
Stark, J. M., Firestone, M. K. (1995). Mechanisms for soil moisture effects on activity of nitrifying bacteria. Appl. Environ. Microbiol. 61, 218–221. doi: 10.1128/aem.61.1.218-221.199
Wang, X. K., Huang, J. L. (2015). Principles and techniques of plant physiological biochemical experiment (Beijing: Higher Education Press).
Wu, X. H., Ge, T. D., Wang, W., Yuan, H. Z., Wegner, C. E., Zhu, Z. K., et al. (2015). Cropping systems modulate the rate and magnitude of soil microbial autotrophic CO2 fixation in soil. Front. Microbiol. 6. doi: 10.3389/fmicb.2015.00379
Xiao, H. B., Li, Z. W., Chang, X. F., Deng, L., Nie, X. D., Liu, C., et al. (2018). Microbial CO2 assimilation is not limited by the decrease in autotrophic bacterial abundance and diversity in eroded watershed. Biol. Fertil. Soils 54, 595–605. doi: 10.1007/s00374-018-1284-7
Yuan, H. Z., Ge, T. D., Chen, C. Y., O’Donnell, A. G., Wu, J. S. (2012a). Significant role for microbial autotrophy in the sequestration of soil carbon. Appl. Environ. Microbiol. 78, 2328–2336. doi: 10.1128/AEM.06881-11
Yuan, H. Z., Ge, T. D., Wu, X. H., Liu, S. L., Tong, C. L., Qin, H. L., et al. (2012b). Long-term field fertilization alters the diversity of autotrophic bacteria based on the ribulose-1,5-biphosphate carboxylase/oxygenase (RubisCO) large-subunit genes in paddy soil. Appl. Microbiol. Biotechnol. 95, 1061–1071. doi: 10.1007/s00253-011-3760-y
Yusoff, M.M., Hu, A., Feng, C., Maeda, T., Shirai, Y., Hassan, M.A., et al. (2013). Influence of pretreated activated sludge for electricity generation in microbial fuel cell application. Bioresource Technol. 145, 90–96. doi: 10.1016/j.biortech.2013.03.003
Zhang, R. F., Vivanco, J. M., Shen, Q. R. (2017). The unseen rhizosphere root–soil–microbe interactions for crop production. Curr. Opin. Microbiol. 37, 8–14. doi: 10.1016/j.mib.2017.03.008
Zhao, S., Liu, J. J., Banerjee, S., Zhou, N., Zhao, Z. Y., Zhang, K., et al. (2020). Biogeographical distribution of bacterial communities in saline agricultural soil. Geoderma 361, 114095. doi: 10.1016/j.geoderma.2019.114095
Zhao, X., Liu, H. L., Yang, P., Zhai, Y. P., Wang, S. M., Zhang, X. (2019). Effects of drip irrigation on bacterial diversity and community structure in rhizosphere soil of alfalfa (in chinese). Microbiol. China. 46, 2579–2590. doi: 10.13344/j.microbiol.china.180874
Zheng, H. P., Yang, T. J., Bao, Y. Z., He, P. P., Yang, K. M., Mei, X. L., et al. (2021). Network analysis and subsequent culturing reveal keystone taxa involved in microbial litter decomposition dynamics. Soil Biol. Biochem. 157, 108230. doi: 10.1016/j.soilbio.2021.108230
Zhou, Z. H., Wang, C. K., Luo, Y. Q. (2018). Effects of forest degradation on microbial communities and soil carbon cycling: A global meta-analysis. Glob. Ecol. Biogeogr. 27, 110–124. doi: 10.1111/geb.12663
Zhou, Z. F., Wei, W. L., Shi, X. J., Liu, Y. M., He, X. H., Wang, M. X. (2019). Twenty-six years of chemical fertilization decreased soil RubisCO activity and changed the ecological characteristics of soil cbbL-carrying bacteria in an entisol. Appl. Soil Ecol. 141, 1–9. doi: 10.1016/j.apsoil.2019.05.005
Zhu, E. X., Liu, T., Zhou, L., Wang, S. M., Wang, X., Zhang, Z. H., et al. (2020). Leaching of organic carbon from grassland soils under anaerobiosis. Soil Biol. Biochem. 141, 107684. doi: 10.1016/j.soilbio.2019.107684
Zhu, Q. C., Wei, C. Z., Li, M. N., Zhu, J. L., Wang, J. (2013). Nutrient availability in the rhizosphere of rice grown with plastic film mulch and drip irrigation. J. Soil Sci. Plant Nutr. 13, 943–953. doi: 10.4067/S0718-95162013005000074
Keywords: cotton, drip irrigation, rhizosphere, bacterial community, functional potential
Citation: Peng B, Zhao S, Banerjee S, Mai W and Tian C (2022) Contrasting effect of irrigation practices on the cotton rhizosphere microbiota and soil functionality in fields. Front. Plant Sci. 13:973919. doi: 10.3389/fpls.2022.973919
Received: 20 June 2022; Accepted: 03 October 2022;
Published: 18 October 2022.
Edited by:
Linkun Wu, Fujian Agriculture and Forestry University, ChinaReviewed by:
Tengxiang Lian, South China Agricultural University, ChinaLeslie A. Weston, Charles Sturt University, Australia
Yanfeng Hu, Key Laboratory of Soybean Molecular Design and Breeding (CAS), China
Copyright © 2022 Peng, Zhao, Banerjee, Mai and Tian. This is an open-access article distributed under the terms of the Creative Commons Attribution License (CC BY). The use, distribution or reproduction in other forums is permitted, provided the original author(s) and the copyright owner(s) are credited and that the original publication in this journal is cited, in accordance with accepted academic practice. No use, distribution or reproduction is permitted which does not comply with these terms.
*Correspondence: Shuai Zhao, emhhb3NodWFpQG1zLnhqYi5hYy5jbg==