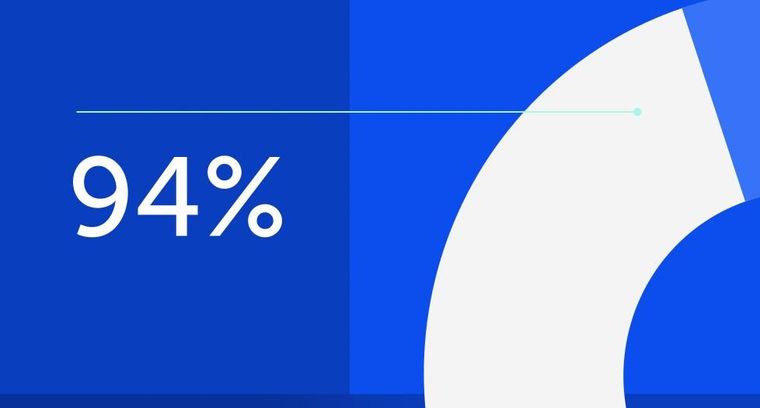
94% of researchers rate our articles as excellent or good
Learn more about the work of our research integrity team to safeguard the quality of each article we publish.
Find out more
ORIGINAL RESEARCH article
Front. Plant Sci., 30 August 2022
Sec. Plant Breeding
Volume 13 - 2022 | https://doi.org/10.3389/fpls.2022.967432
Mining novel and less utilized thousand grain weight (TGW) related genes are useful for improving wheat yield. In this study, a recombinant inbred line population from a cross between Zhongkemai 138 (ZKM138, high TGW) and Chuanmai 44 (CM44, low TGW) was used to construct a new Wheat 50K SNP array-derived genetic map that spanned 1,936.59 cM and contained 4, 139 markers. Based on this map, ninety-one quantitative trait loci (QTL) were detected for eight grain-related traits in six environments. Among 58 QTLs, whose superior alleles were contributed by ZKM138, QTgw.cib-6A was a noticeable major stable QTL and was also highlighted by bulked segregant analysis with RNA sequencing (BSR-Seq). It had a pyramiding effect on TGW enhancement but no significant trade-off effect on grain number per spike or tiller number, with two other QTLs (QTgw.cib-2A.2 and QTgw.cib-6D), possibly explaining the excellent grain performance of ZKM138. After comparison with known loci, QTgw.cib-6A was deduced to be a novel locus that differed from nearby TaGW2 and TaBT1. Seven simple sequence repeat (SSR) and thirty-nine kompetitive allele-specific PCR (KASP) markers were finally developed to narrow the candidate interval of QTgw.cib-6A to 4.1 Mb. Only six genes in this interval were regarded as the most likely candidate genes. QTgw.cib-6A was further validated in different genetic backgrounds and presented 88.6% transmissibility of the ZKM138-genotype and a 16.4% increase of TGW in ZKM138 derivatives. And the geographic pattern of this locus revealed that its superior allele is present in only 6.47% of 433 Chinese modern wheat varieties, indicating its potential contribution to further high-yield breeding.
Bread wheat (Triticum aestivum L.) is one of the most important crops worldwide, providing approximately 20% of calories and 25% of protein for humans (FAO, 2017).1 The global population is reportedly expected to reach nine billion by 2050, which will require an increase in overall food production of at least 70% to meet future food needs.2 Therefore, the steady growth of wheat production is an essential factor in ensuring food security.
Thousand grain weight (TGW), one of three yield components, affects wheat production together with the grain number of spike (GNS) and tiller number (TN). It is a quantitative trait controlled by multiple genes and shows higher heritability than GNS and TN (Simmonds et al., 2014). Previous reports have found that TGW is influenced by multiple grain-related traits (GRTs), including grain size traits [such as grain length (GL), grain width (GW), etc.], and the grain shape traits [such as grain roundness (GR), etc.] (Kumar et al., 2016; Wang et al., 2019a; Guan et al., 2020). These GRTs, especially the grain size traits, are positively correlated with TGW and thus regarded as genetic improvement targets together with TGW in the breeding process (Gegas et al., 2010). Therefore, simultaneously dissecting the novel genetic background controlling TGW and related GRTs can effectively improve wheat yield potential.
Researchers have identified several genes for TGW to facilitate high-yield genetic improvements, such as TaGW2 (Su et al., 2011), TaBT1 (Wang et al., 2019b), as well as TaTPP-6AL (Zhang et al., 2017). TaGW2-6A, an E3 RING ligase gene, is located near the centromere of chromosome 6AS (237.734–237.759 Mb) (Su et al., 2011) and also is one of the most famous and well-studied weight relative genes in common wheat. Previous studies have reported that several single nucleotide polymorphisms (SNPs) or variations could account for yield increases. For instance, SNPs at –593 bp of the promoter region (Hap-6A-A) in Chinese modern varieties (Su et al., 2011) and –494 bp (Hap_5) in Indian wheat (Jaiswal et al., 2015) were discovered, and their corresponding cleaved amplified polymorphic sequence (CAPS) markers were developed for marker-assisted selection(MAS) for improving grain weight. Moreover, the haplotype distributions analysis revealed that the Hap-6A-A was the preferred allele in 265 Chinese mini-core collections and increased TGW (3.11 g). In the 5′ flanking region, Zhai et al. (2018) identified a novel and rare TaGW2-6A allele and found that only three accessions among 848 hexaploid, 238 tetraploid, and 27 diploid wheat accessions contained the favorable allele (TaGW2-A1) increasing TGW. In addition, two mutations in the coding sequence have also been reported to be associated with TGW and grain size: a frame-shift mutation in exon 8 (Yang et al., 2012; Du et al., 2016) and a splice acceptor site mutation in exon 5 (Simmonds et al., 2016). The markers developed based on these reported loci are important tools for detecting TaGW2-6A haplotypes and estimating their effects on TGW.
In addition to the known genes, hundreds of QTLs for TGW were primarily reported overall wheat chromosomes (Cao et al., 2020; Chen et al., 2020b; Hu et al., 2020; Liu et al., 2020; Xin et al., 2020; Li et al., 2022) and a large number of them were co-localized with other GRTs. For example, Li et al. (2022) detected forty-two QTLs controlling TGW, GW, and GL and explaining 3.26–34.06% of phenotypic variation on chromosomes 2A, 3A, 5A, 6A, 3B, and 4B based on the new genetic map from the Wheat 55K SNP array. A major stable QTL for TGW was co-located with that for GW on chromosome 4B, which could increase TGW by 9.64% and was considered a novel locus. In addition, Wang et al. (2019a) reported fourteen QTL clusters for TGW and GRTs based on a Wheat 90K SNP array-derived map, and five of them were likely novel loci and improved the corresponding traits, making them worthy of further exploration and application. Consequently, QTL mapping is an effective method for discovering novel loci and dissecting the genetic basis of multiple complex related traits.
With the advent of draft and complete genome sequences (International Wheat Genome Sequencing, 2014, 2018)3, high-density SNP arrays (Wang et al., 2014; Winfield et al., 2016) have greatly accelerated and facilitated wheat genetic studies. To date, several kinds of wheat SNP arrays have been reported, including 9K (Cavanagh et al., 2013), 35K (King et al., 2017), 50K (Song et al., 2021), 55K (Li et al., 2022), 90K (Wang et al., 2019a), 820K (Winfield et al., 2016), and 660K (Cui et al., 2017) SNP arrays. These SNP arrays have contributed to the detection of important genetic traits and excavation of superior genes in wheat. The technology for transformation and validation of the linked SNPs has also been developed (Wang et al., 2019b; Deng et al., 2022), such as KASP, CASP, and InDel markers (Jaiswal et al., 2015; Zhai et al., 2018; Li et al., 2022), gradually facilitating the detection or validation of the target QTLs. For example, Liu et al. (2020) discovered that the favorable allele of TaFT-D1 had been positively selected during Chinese wheat breeding and increased TGW by 4.33 g by using an effective KASP marker, further indicating these closely linked SNPs can indeed facilitate MAS.
In this study, we developed a recombinant inbred line (RIL) population based on the cross between ZKM138 (high grain weight) and CM44 (low grain weight) with the aims to: (1) construct a genetic map based on the Wheat 50K SNP array to analyze the genetic basis of the superior grain and yield performance of ZKM138; (2) dissect the valuable QTLs controlling TGW and GRTs; (3) develop effective markers to assess the application and distribution of target QTL.
An F7–9 RIL population, including 170 lines from a cross between ZKM138 and CM44 (named BC-RILs), was used for genetic map construction and QTL mapping. ZKM138 is a high-yield wheat variety with excellent TGW and grain performance. It has been consecutively listed as a leading variety in the Chengdu Plain since it was released in 2015 by the Chengdu Institute of Biology, CAS (CIBCAS) (Figure 1A). CM44, released in 2004, is also a representative variety in Sichuan Province with good quality, but its grain weight and size are obviously smaller than those of ZKM138. Another RIL population, derived from a cross between the public parent ZKM138 and a variety with smaller grain (CD1437) (ZC), containing 152 lines, was employed to validate the target QTL in different genetic backgrounds. Additionally, seventy-nine derivatives of ZKM138 also developed by CIBCAS were used to validate the target QTL further and assess its application potential in our actual breeding process. In addition, 433 Chinese varieties and 191 wheat varieties from other countries were employed to evaluate the distribution of the target locus and its potential application value for future TGW improvement.
Figure 1. Performance of the measured grain related traits in the BC-RILs population. (A) Grain phenotype of the parents ZKM138 and CM44, and two representative lines of the BC-RILs population. The white line represents a scale = 1 cm. (B) Correlation analysis between TGW and GRTs in the BC population based on the BLUE dataset. * and ** indicate significance at P ≤ 0.05 and P ≤ 0.01, respectively.
The RILs and parents were evaluated in two field experiments in Shifang (SF, 104°11′E, 31°6′N) and Shuangliu (SL, 103°52′E, 30°34′N), in 2019, 2020, and 2021 planted in a randomized block design. Each field trial was planted with two blocks. Each block contained the complete population lines and parents. The organic fertilizer (300 kg ha–1), nitrogen fertilizer (120 kg ha–1), phosphate fertilizer (50 kg ha–1), and potash fertilizer (50 kg ha–1) as a base fertilizer were applied only before sowing. The soil nutrition conditions in 0–50 cm soil depth were nitrogen (N) content 1.30 g kg–1, phosphate (P) content 820.07 mg kg–1, potash (K) content 29.28 g kg–1, ammonium nitrogen content 11.611 mg kg–1, and nitrate-nitrogen content 8.89 mg kg–1 (Shuangliu) and N content 1.128 g kg–1, P content 772.33 mg kg–1, K content 19.51 g kg–1, ammonium nitrogen content 42.75 mg kg–1, and nitrate-nitrogen content 87.35 mg kg–1 (Shifang), respectively.
The field trials were carried out for the ZC population in four crop seasons of 2016–2018 at Shifang (E1, E3, and E5) and three crop seasons of 2016–2018 (E2, E4, and E6) at Shuangliu. Moreover, seventy-nine derivatives of ZKM138 were planted and evaluated at Shuangliu in 2019–2020.
Each line was single-seed planted in double-row plot with a length of 1 m, 20 seeds per row, and a row spacing of 0.25 m. Field management and disease control were performed according to the common practices for wheat production.
At maturity, at least six representative samples planted in the middle of each line were harvested and evaluated for the following eight traits using SC-G software (Wseen, Hangzhou, Zhejiang, China): TGW (Thousand Grain Weight), GL (Grain Length), GW (Grain Width), GA (Grain Area), GD (Grain Diameter), GP (Grain Perimeter), GR (Grain Roundness), and LWR (Length to Width Ratio) (Kumar et al., 2016).
Basic statistical analyses, frequency distribution, and correlation coefficients among traits were conducted using SPSS version 25.0 for Windows (IBM SPSS, Armonk, NY, United States). The best linear unbiased estimator (BLUE) was calculated using QTL IciMapping software. Broad-sense heritability (H2) was estimated according to the method described by Smith et al. (1998) and Muqaddasi et al. (2019).
At the 15 days after pollination (DPA) stage, the grains of two extreme pools from 20 different lines from BC RILs planted in 2020 in the Shifang experimental field were sampled to construct opposite bulk pairs for RNA sequencing. Total RNA was isolated according to the TRIzol protocol (Invitrogen, Carlsbad, CA, United States). The RNA libraries were sequenced on the Illumina sequencing platform and further analyzed by Genedenovo Biotechnology Co., Ltd. (Guangzhou, China).
The genomic DNA of parents and BC populations was extracted from fresh seedling leaves using the CTAB method and hybridized on the Wheat 50K SNP array by CapitalBio Technology (Beijing, China).
IciMapping 4.1 (Meng et al., 2015) and JoinMap 4.1 were used for genetic construction. First, all markers were analyzed using the BIN function of IciMapping 4.14 based on their parameters of “Missing Rate” and “Distortion Value” being set as 20% and 0.001, respectively. Only one marker from each bin (bin marker) was randomly selected to construct the genetic map. Then, the function of ‘Population’ in JoinMap 4.1 was used to create groups with a limit of detection (LOD) score values ranging from 2 to 10. Last, the Kosambi mapping function was used to order the bin markers, with the parameters being set as LOD ≥ 7 and round = 3, in JoinMap 4.1.
Quantitative trait loci detection in seven datasets (six environments and the BLUE dataset) of BC-RILs was performed by IciMapping 4.1 with the inclusive composite interval mapping (ICIM). Missing phenotype was deleted in the QTL analysis. Single-environment QTLs were detected using the biparental population (BIP) module with a walking step = 0.001 cM and PIN = 0.001, and a test with 1,000 permutations was used to identify the LOD threshold. A LOD value = 2.5 was used to detect putative QTLs. Moreover, a QTL with a LOD value ≥ 3.5 and phenotypic variation > 10% (on average) that was detected in more than two datasets was considered a major stable QTL (Li et al., 2022). If the confidence intervals of corresponding QTLs overlapped, these QTLs were considered and named one QTL.
Besides, except for single-environment QTL detection based on ICIM, multi-environment QTL analysis was conducted by IciMapping 4.1 using the multi-environment trials (MET) with pre-adjusted parameters: Step = 1 cM, PIN = 0.001, and LOD = 2.5. A QTL was considered significantly related to the environment when LOD (AbyE) > 3. QTLs were named according to the International Rules of Genetic Nomenclature, where “cib” represents Chengdu Institute of Biology.
Sixteen functional KASP markers related to 14 reported yield-related genes, including TaSus2-2A, TaCwi, TaGS, TaGS2-B1, TaSus2, GS5, TaTGW6, TaCWI, TaGW2, TaSus1-7A, TaTGW-7A, TaMOS1-7A, TaSus1-7B, and TaGS-D1, were used to detect polymorphisms in parents by China Golden Marker in Beijing.
The physical interval of the target QTL overlapped with TaGW2 (Su et al., 2011) and was adjacent to TaBT1 (Wang et al., 2019b). Thus four markers were used to detectTaGW2 in the parents and lines, including two markers (Su et al., 2011; Simmonds et al., 2016) in the coding region and two markers (Su et al., 2011; Zhai et al., 2018) in the promoter region. Sequence analysis of the promoter region and coding region of TaGW2 was also performed. Meanwhile, two PCR markers listed in Supplementary Table 1 were used to detect TaBT1.
Kompetitive allele-specific polymerase chain reaction markers and SSR markers (Supplementary Table 1) were developed to construct the saturated genetic map of target QTLs based on information from the Wheat 50K SNP array and BSR-Seq in the preliminary QTL interval. According to the previous report (Li et al., 2022), the reaction conditions and systems of the polymerase chain reaction (PCR) system and the KASP arrays were conducted.
Genes within the target region were extracted from the re-sequencing data of the parents. Functional annotation was performed by http://www.uniprot.org/. The gene expression profiles were obtained from the Wheat Expression Browser.5 Graphs of expression values were drawn in TBtools software (v.1.098) (Chen et al., 2020a).
The phenotypic performance of eight traits (TGW, GL, GW, GA, GD, GP, GR, and LWR) in two parents and the BC-RILs across seven datasets are shown in Table 1. Compared with CM44, ZKM138 had significantly higher TGW and five grain size traits, including GL, GW, GA, GD, and GP (Figure 1A), while the grain shape traits (GR and LWR) were not significantly different between the two parents. In the BC population, the absolute values of skewness and kurtosis were almost <1 (Table 1), indicating that the phenotypic data were approximately normally distributed in this population. Strong transgressive segregation exceeding the limits of both parents was observed, indicating that favorable alleles were distributed by the two parents (Table 1). Broad sense heritability (H2) was greater than 0.80 across each environment for all traits.
Table 1. Phenotypic variation and heritability (H2) of thousand grain weight (TGW) and grain-related traits (GRTs), for the parents and the BC-RILs in different environments.
Notably, the correlation analysis (Figure 1B) revealed that TGW was positively correlated with all grain size traits, such as GL, GW, GA, GD, and GP, especially GA and GD, with the highest correlation coefficient being 0.93 in both cases. For GW and GL, the correlation coefficient of TGW-GW was higher (r = 0.89) than TGW-GL (r = 0.67), indicating that there might be a more common genetic basis of TGW with GW than GL in BC-RILs. On the other hand, the correlation of TGW with two grain shape traits (GR and LWR) was relatively weak (r = 0.21/–0.24).
The scores for the probes were classified into six categories by Affymetrix software: (1) Poly High Resolution (PHR) (16724; 25.02%); (2) No Minor Homozygote (NMH) (25307; 37.86%); (3) Off-target Variant (OTV) (1888; 2.82%); (4) Mono High Resolution (MHR) (3306; 4.95%); (5) Call Rate Below Threshold (CRBT) (1,361; 2.04%); and (6) Other (18,249; 27.3%) (Supplementary Figure 1). Of the 66,834 markers called from the Wheat 50K SNP array, 7,725 (11.56%) markers were polymorphic between the two parents. After removing SNPs with more than 20% missing data or a segregation distortion test P < 0.001, 4,139 markers were used for map construction and linkage analysis. These markers were divided into 948 bins. Only one marker was selected from each bin. In total, 467 bins contained only one marker, and the largest bin contained 110 markers on chromosome 1A. Finally, a novel genetic map consisting of 24 linkage groups was mapped on 21 chromosomes of wheat. Chromosomes 3A, 5A, and 6D contained two linkage groups (Supplementary Figure 2 and Supplementary Table 2). Based on the genetic information, all SNP markers, including bin markers and redundant markers, were integrated into the genetic map with a total length of 1,939.78 cM and average interval distance of 2.04 cM per bin and 0.47 cM per marker (Supplementary Table 3). The mapped markers were located in the A (28.78%), B (42.67%), and D (28.56%) genomes with lengths of 596.68, 730.44, and 612.66 cM, respectively. In addition, the lengths of the constructed linkage maps ranged from 10.66 (6D2) to 131.21 cM (7D); the markers on linkage maps ranged from 21 (3A2) to 396 (7B); the average interval distance between adjacent markers ranges from 0.08 (6D2) to 2.11 (5D); and the average interval distance between adjacent bin markers ranged from 1.18 (6D2) to 4.22 cM (1D).
Based on the physical locations of these SNPs in the reference genome (IWGSC RefSeq v1.1), 4,019 markers showed best hits to the CS contigs, and 120 markers (2.90%) were mapped to their homologous and non-homologous linkage groups (Supplementary Figure 3). Marker order was relatively consistent with that of the wheat genome assembly on most chromosomes (Supplementary Figure 3).
For single environment QTL detection, ninety-one QTLs were identified for eight traits in seven datasets with phenotypic variations ranging from 3.10 to 18.04% and LOD values of 2.50–11.85. And forty-five, fifteen, and thirty-one QTLs were distributed on A, B, and D genomes, respectively; the favorable alleles of fifty-eight (63.74%) QTLs were donated from ZKM138. Thirty-six QTLs were stable QTLs that could be detected in more than two datasets and are presented in detail below, and the remaining fifty-five QTLs were detected in only one dataset (Supplementary Table 4).
For TGW, five stable QTLs were detected on chromosomes 2A, 6A, and 6D1. Among these QTLs, a major stable QTL, QTgw.cib-6A, was detected in all datasets, explaining 6.84–14.84% of phenotypic variation. The other QTLs, QTgw.cib-2A.1, QTgw.cib-2A.2, QTgw.cib-2A.3, and QTgw.cib-6D, explained 6.90–9.43, 9.38–12.74, 5.96–8.33, and 7.93–8.53%, respectively, of phenotypic variation. Interestingly, the positive alleles of all five loci were contributed by ZKM138, the parent with better grain performance.
For GL, eight stable QTLs were identified on chromosomes 2A, 4A, 5A1, 6A, and 6D1. Two major stable QTLs, QGl.cib-2A.1 and QGl.cib-6A, explained 6.99–14.76 and 7.84–12.80%, respectively, of phenotypic variation. QGl.cib-4A explained 4.76-7.77% of phenotypic variation. Five QTLs, QGl.cib-2A.2, QGl.cib-2A.3, QGl.cib-5A1, QGl.cib-6D1.1, and QGl.cib-6D1.2, explained 3.70–4.30, 7.47–9.81, 4.90–6.75, 4.09–4.49, and 7.34–10.26%, respectively, of phenotypic variation. The positive alleles at seven loci (except QGl.cib-2A.1) were contributed by ZKM138.
For GW, three stable QTLs were identified on chromosomes 2A, 6A, and 6D1. QGw.cib-2A.1, QGw.cib-6A, and QGw.cib-6D1 explained 8.78–9.08, 6.41–9.61, and 7.13–10.18% of phenotypic variation, respectively. Similarly, ZKM138 contributed to the beneficial alleles at all QTLs.
For GA, three stable QTLs were detected on chromosomes 2A, 6A, and 6D1. QGa.cib-6A, a major stable QTL, explained 8.14–14.34% of phenotypic variation. The other QTLs QGa.cib-2A and QGa.cib-6D1 explained 7.89–8.12% and 6.61–7.11% of phenotypic variation, respectively. The superior alleles of the three QTLs were also obtained from ZKM138.
For GD, four stable QTLs were identified on chromosomes 2A, 6A, and 6D1. A major stable QTL, QGd.cib-6A, explained 8.91–14.21% of phenotypic variation. QGd.cib-2A.1, QGd.cib-2A.2, and QGd.cib-6D1 explained 8.57–8.59, 8.33–8.91, and 6.94–6.95%, respectively, of phenotypic variation. Additionally, the beneficial alleles of these QTLs were all from ZKM138.
For GP, seven stable QTLs were detected on chromosomes 2A, 5A1, 6A, and 6D1. A major stable QTL, QGp.cib-6A, explained 8.83–15.51% of phenotypic variation. The remaining QTLs, QGp.cib-2A.1, QGp.cib-2A.2, QGp.cib-2A.3, QGp.cib-5A, QGp.cib-6D.1, and QGp.cib-6D.2, explained 6.66–9.42, 9.02–10.10, 3.10–7.62, 7.26–7.60, 4.96–7.06, and 7.04–12.21%, respectively, of phenotypic variation. The positive alleles of these QTLs (except QGp.cib-2A.1) were contributed by ZKM138.
For GR, three stable QTLs were detected on chromosomes 2A, 4A, and 5A1. A major stable QTL, QGr.cib-2A, was detected in four datasets, explaining 8.11–17.12% of phenotypic variation. QGr.cib-4A and QGr.cib-5A1 explained 5.14–7.52% and 4.48–10.35% of phenotypic variation, respectively. The superior allele increasing GR at QGr.cib-2A was contributed by ZKM138, while CM44 contributed to the positive alleles of QGr.cib-4A and QGr.cib-5A1.
For LWR, three stable QTLs were identified on chromosomes 2A, 4A, and 5A1. QLwr.cib-2A was a major stable QTL that explained 8.11–17.12% of phenotypic variation. QLwr.cib-4A and QLwr.cib-5A1 explained 4.71–8.60 and 4.98–9.40% of phenotypic variation, respectively. The positive alleles of QLwr.cib-4A and QLwr.cib-5A1 were donated by ZKM138, while CM44 contributed to the favorable alleles of QLwr.cib-2A. Consistent with expectations, these results were contrary to QTLs for GR.
Based on the stable QTLs detected in a single environment (Supplementary Table 4), eight QTL clusters containing nine major stable QTLs for different traits were highlighted (Table 2). These clustered QTLs shared confidence intervals and thus were inactive of potential pleiotropic effect on the corresponding traits. They were mainly mapped on 2A (three clusters), 4A (one cluster), 5A1 (one cluster), 6A (one cluster), and 6D1 (two clusters), respectively (Figure 2). Notably, the superior alleles on C2A.2, C2A.3, C6A, C6D1.1, and C6D1.2 were all from ZKM138, which increased the corresponding traits.
Table 2. Quantitative trait loci (QTL) clusters simultaneously affecting thousand grain weight (TGW) and grain-related traits (GRTs) in the BC-RILs.
Figure 2. The highlighted QTL regions for TGW and GRTs. (A) Summary of QTL clusters detected in the BC-RILs (except C6A). (B) The target QTL cluster on chromosome 6A (C6A). (C) The LOD value of the major stable QTL (QTgw.cib-6A) for TGW in the BC population. The dotted line represents a logarithm of the odds (LOD) = 2.5. (D) Identification of candidate SNPs using BSR-Seq. Δ (SNP-index) > 99%.
C6A contained a major stable QTL for TGW (QTgw.cib-6A), which was detected in all seven datasets and was simultaneously clustered with the other four major stable QTLs (QGl.cib-6A, QGa.cib-6A, QGd.cib-6A, and QGp.cib-6A) and one other stable QTL (QGw.cib-6A), with the ZKM138-derived alleles increasing all these traits.
C2A.2 contained a major stable QTL for TGW (QTgw.cib-2A.2) which co-localized with four QTLs for grain size traits (QGl.cib-2A.3, QGa.cib-2A.3, QGd.cib-2A.1, and QGp.cib-2A.2). ZKM138 also contributed to the beneficial alleles for all QTLs.
Additionally, three clusters associated with grain shape traits were located on chromosomes 2A (C2A.1), 4A (C4A), and 5A1 (C5A). C2A.1 consisted of three major stable QTLs (QGl.cib-2A.1, QGr.cib-2A, and QLwr.cib-2A), with ZKM138-derived alleles decreasing GL and LWR but increasing GR. Both C4A and C5A contained QTLs for GR, LWR, and GL, indicating that these three loci might control grain shape mainly by moderating grain length.
Using the MET module under multiple environmental conditions, a total of four hundred QTLs for TGW and GRTs were detected (Supplementary Table 5). Consistent with Cui et al. (2014) and Fan et al. (2019), all QTLs detected by the BIP module could be repeatedly identified in the MET module, indicating that the QTLs detected in this study have high reliability and consistency. Among them, fifty-five QTLs were detected in a single environment, indicating that they are greatly affected by the environment and cannot be stably expressed. For example, QGd.cib-6D1.2, one of the environmentally sensitive QTLs with a high PVE (AbyE) of 6.15% (Supplementary Table 4), was specifically expressed in E2 (Supplementary Table 4). However, the PVE (AbyE) of QTgw.cib-6A was only 1.23%, while its PVE (A) was higher (7.04%) (Supplementary Table 5), indicating that this locus is relatively stable in different environments and mainly affected by genetic factors.
The polymorphism between the two parents was tested by sixteen functional markers related to fourteen genes for grain weight and yield (Supplementary Table 6). Only TaGS (Zhang et al., 2014) and TaTGW6 (Hanif et al., 2016) presented polymorphism between parents, but they did not overlap with any of the QTLs detected in this study (Supplementary Figure 4A).
Additionally, based on the detection of markers reported in previous studies, no divergence was found between the parents and lines for TaGW2 and TaBT1 (Supplementary Figure 4B). The sequencing of the promoter region and coding region of TaGW2 further showed that they were consistent between parents (Supplementary Figures 4C,D). Therefore, QTgw.cib-6A was deduced to probably be a novel locus that differed from its nearby TaGW2 and TaBT1. Therefore, QTgw.cib-6A was deduced to be a novel locus that differed from its nearby TaGW2 and TaBT1.
The additive effects of the positive alleles at the three QTLs for TGW (QTgw.cib-2A.2, QTgw.cib-6A, and QTgw.cib-6D) were analyzed (Figure 3A). Compared with those without favorable alleles, RILs carrying one, two, or three alleles showed TGW significantly increased TGW by 9.87–26.28%. The combination of the three superior alleles had the largest pyramiding effect on increasing TGW, followed by two superior alleles, and only one superior allele exhibited the smallest positive effect on TGW. This is further supported by line BC-168 carrying the ZKM138-derived allele at the three loci displaying a higher TGW than line BC-161 carrying the CM44-derived allele (Figure 1A). Moreover, these QTLs had no significant trade-off effect on TN (Figure 3B) and GNS (Figure 3C).
Figure 3. Pyramiding effect analysis of QTgw.cib-2A.2, QTgw.cib-6A and QTgw.cib-6D on TGW (A), TN (B), and GNS (C). *, **, and *** represent significance at P < 0.05, P < 0.01, and P < 0.001, respectively and ns represents non-significance; + and - represent lines with and without the positive alleles of the target QTLs based on the flanking markers of the corresponding QTL, respectively; AA and BB represent lines with and without the positive alleles of the target QTLs based on the flanking markers of the corresponding QTL, respectively.
Using the BSR-Seq approach, a set of candidate SNPs (70.43%) between the parents putatively associated with TGW were identified on chromosome 6A (Figure 2D), consistent with the QTL mapping results (Figure 2B). Combining the information from the candidate SNPs of BSR-Seq and the Wheat 50K SNP array, a new integrated genetic map for the candidate region of QTgw.cib-6A was reconstructed with thirty-nine KASP markers and seven SSR markers (Supplementary Table 7). After remapping based on the new map, the original candidate region (101 Mb) was narrowed to only 4.1 Mb (Figure 4).
Figure 4. Delimitation and validation of QTgw.cib-6A. (A) The new integrated genetic map of QTgw.cib-6A; markers in blue represent the preliminary QTL interval of QTgw.cib-6A; markers in red represent tightly linked marker; and the bar in red represents the new interval of QTgw.cib-6A based on the integrated genetic map. (B) Effect estimation of QTgw.cib-6A on TGW in the BC population; the red and blue boxes indicate the TGW (g) performance of the lines with alleles from ZKM138 and CM44, respectively; *, **, and ** represent significance at P < 0.05, P < 0.01, and P < 0.001, respectively. (C) Effect estimation of QTgw.cib-6A on TGW in the ZC population. The red and green boxes indicate the TGW (g) performance of the lines with alleles from ZKM138 and CD1437, respectively; *, **, and *** represent significance at P < 0.05, P < 0.01, and P < 0.001, respectively.
The effects of QTgw.cib-6A on TGW and GRTs were further examined using the newly developed and linked marker (KASP6A-2121) in all datasets. Significant differences (P < 0.001) were observed in TGW (Figure 4) and all five grain size traits (GA, GD, GL, GW, and GP) (Supplementary Figure 5) between the lines with homozygous alleles from ZKM138 and CM44. However, no significant differences were found in grain shape traits, such as GR and LWR, at this locus (Supplementary Figure 5).
Considering that the favorable allele of QTgw.cib-6A was from ZKM138, the ZC-RILs also derived from the common parent ZKM138 were used to preliminarily validate the effects of QTgw.cib-6A under different genetic backgrounds. The lines containing different alleles showed significant differences in TGW (P < 0.05) (Figure 4). Moreover, the lines with the ZKM138-derived allele had significantly (P < 0.05) higher GW, GA, GP, and GD than those homozygous for the CD1437 allele (Supplementary Figure 5). To a certain extent, it was confirmed that the ZKM138-derived allele at this locus improves grain weight and most grain size traits in ZC-RILs.
ZKM138 has been an important backbone parent during our breeding process and derived several varieties and stable lines. In this study, a total of seventy-nine ZKM138 derivatives were used to further validate the effect of QTgw.cib-6A and estimate its transmissibility. In total, 88.6% of these lines harbored a ZKM138-derived allele, indicating that this candidate gene had been selected during the small-scale breeding process that used ZKM138 as the major parent (Figure 5A). The group harboring the superior alleles also exhibited significantly (P < 0.001) increased TGW (16.4%), which further confirmed the contribution of the ZKM138-derived allele to effective increases in TGW (Figure 5A).
Figure 5. Transmissibility and geographic patterns of the genotype of QTgw.cib-6A. (A) Contributions of QTgw.cib-6A to TGW enhancement in 79 ZKM138-derivatives. Numbers in white represent the mean value of TGW; *** represents significance at P < 0.001. Geographic distributions of the superior allele of QTgw.cib-6A in 624 worldwide samples (B) and 433 Chinese varieties (C). I, Northern Winter Wheat Zone; II, Yellow and Huai River Valleys Facultative Wheat Zone; III, Middle and Lower Yangtze Valleys Autumn-Sown Spring Wheat Zone; IV, Southwestern Autumn-Sown Spring Wheat Zone; V, Southern Autumn-Sown Spring Wheat Zone; VI, Northeastern Spring Wheat Zone; VII, Northern Spring Wheat Zone; VIII, Northwestern Spring Wheat Zone; IX, Qinghai-Tibetan Plateau Spring-Winter Wheat Zone; X, Xinjiang Winter-Spring Wheat Zone; AA and BB represent varieties with and without the superior allele of QTgw.cib-6A, respectively.
To determine whether the superior allele was applied to a broader breeding range, using marker KASP6A-2121, we preliminarily investigated the geographic distribution of this genotype in 433 Chinese wheat varieties and 191 germplasms varieties from other fifteen countries belonging to Asian, Europe, Oceania, and Africa, respectively. As shown in Figure 5, the samples with AA genotype in 624 worldwide varieties (consistent with ZKM138) accounted for only 4.97% (31/624), of which 5.22% (29/556) was from Asian and 4% (2/50) was from Europe. No samples contained superior allele in Oceania and Africa. Notably, the ZKM138-derived allele was detected in 28 of 433 (6.47%) Chinese varieties, which were derived from wheat zones I, II, III, IV, VI, and IX. The frequency of favorable allele in Chinese wheat zones was in the order of IV (11.32%) > II (3.92%) > I, III, VI, and IX (0%) (Figures 5B,C and Supplementary Table 8). These results suggested that the superior allele could be used more widely as an insufficiently selected and applied locus to increase yield potential in the future.
According to the re-sequencing data of the parents, fourteen genes in the candidate region of QTgw.cib-6A (213.1-217.2 Mb) had different SNPs in the gene body of the parents. These genes were annotated based on the Ensembl Plant database6 (Supplementary Table 9). The initial spatial expression pattern analysis showed that nine genes were expressed in various tissues (root, stem, leaf, spike, and grain) based on the public expression database7 (Borrill et al., 2016; Ramírez-González et al., 2018; Figure 6). Only six of them (TraesCS6A02G185400, TraesCS6A02G183500, TraesCS6A02G185000, TraesCS6A02G184000, TraesCS6A02 G183900, and TraesCS6A02G184500) were specifically expressed in the spike and grain, and might be the most likely candidate genes needing further validation.
Figure 6. Expression patterns of candidate genes in the physical interval of QTgw.cib-6A in different tissues. * represents genes specifically expressed in the spike and grain; the data of expression profiles were obtained from the public online database (http://202.194.139.32/expression/wheat.html).
Grain shape and size traits are two kinds of grain features affecting the cereal milling process and yield formation, respectively (Peng et al., 2003). In this study, consistent with previous reports (Cheng et al., 2017; Wang et al., 2019a), wheat grain size traits showed a higher positive correlation coefficient with TGW (r > 0.67) than grain shape traits (r < 0.24), thus facilitating final yield, which could be partially explained by 12 co-localized QTLs (Figure 1B and Supplementary Table 4). These QTLs all presented the same direction of additive effects, while no stable QTLs affecting both TGW and grain shape traits were identified in this study, further indicating that modifying grain size might be an effective method to increase grain weight and that grain shape is more likely to be independent of TGW.
Unlike in the rice breeding process, where grain shape is under strong selection because of its contribution to rice processing and cooking quality (such as milling rate) (Jain et al., 2004; Zheng et al., 2010), grain shape does not appear to be a major target in the wheat selection and breeding process (Kovach et al., 2007). In fact, previous studies found that the flour extraction rate was higher for semispherical grains (62.1%) than for long grains (57.4%) in wheat (Cheng et al., 2020), indicating the equal importance of grain shape and grain size for wheat grain improvement. Thus, as shown in this study, pleiotropic QTLs for grain size and TGW could be used to enhance grain yield, and two major stable QTLs (QGr.cib-2A and QLwr.cib-2A) for grain shape might also play a role of in process quality improvement.
Identifying beneficial alleles has been an effective method for wheat genomic research (Chen et al., 2017). In this study, there were significant differences between parents for TGW and other grain traits (Figure 1A). ZKM138 was found to present superior genotypes at only three genes (Supplementary Table 6), including TaTGW6 (Hanif et al., 2016), TaTGW7 (Hu et al., 2016), TaSus1-7A (Hou et al., 2014), and carry negative genotypes at all of the other ten genes (Supplementary Table 6), suggesting the possibility of the undiscovered loci supporting high TGW performance in ZKM138. In this study, eight QTL clusters containing stable QTLs for TGW and GRTs were detected, but most of them were previously reported, except C6A harboring QTgw.cib-6A. Specifically, the TGW relative gene, TaFlo2-A1, located on deletion bin “2AL1–0.85–1.00” (Sajjad et al., 2017), was overlapped with the CI of C2A.3, which was consistent with numerous reported QTLs for TGW (Ma et al., 2018; Sukumaran et al., 2018; Liu et al., 2019), and thus may be responsible for this region affecting TGW. And another gene associated with TGW, TaGS1a (Guo et al., 2013), was located in the region of C6D1.2 and thus might be the gene responsible for this QTL cluster.
At the QTL level, the CI of C2A.1, close to Ppd-A1 (a well-known photoperiod locus also affecting GRTs) (Beales et al., 2007), was overlapped with several reported QTLs for TGW (Mohler et al., 2016; Liu et al., 2019). Additionally, QTLs for TGW and grain size traits previously detected in this region by Mangini et al. (2021) in durum wheat. However, considering that this region was mainly associated with grain shape and did not affect TGW, differences between C2A.1 and the above reported QTLs were possible. Another cluster mainly controlling grain shape, C5A1, was located in a 12.5 cM interval corresponding to 0.64–3.65 Mb. In this region, only one QTL for GL was reported at 2.79 Mb (Sun et al., 2017), which might correspond to C5A1, which also harbored a stable QTL for GL (QGl.cib-5A1) (Table 2).
For grain size related loci, the QTL cluster C2A.2, associated with TGW and four grain size traits (GL, GA, GD, and GP), might include QTLs for TGW and grain size traits that have been previously reported in hexaploid wheat (Cui et al., 2014; Guan et al., 2018; Li et al., 2022) and in durum wheat (Peleg et al., 2011; Sun et al., 2020). Moreover, the region of C6D1.1 contained QTLs for four grain size traits that were consistent with these previously reported QTLs for TGW (Chen et al., 2019; Liu et al., 2019), GL, and GW (Chen et al., 2019).
Wheat yield is a polygenic trait primarily determined by three yield components, i.e., TGW, GNS, and TN (Simmonds et al., 2016). Commonly, TGW is negatively associated with GNS (Jia et al., 2013; Schulthess et al., 2017; Zhai et al., 2018), and thus, many TGW-related genes present a trade-off effect on grain yield (Sukumaran et al., 2018). For example, Zhai et al. (2018) reported a novel and rare TaGW2-A1 allele that increased TGW by reducing GNS. Identifying QTLs for TGW with no negative effect on GNS is beneficial for enhancing yield potential. However, at the QTL level, Xie and Sparkes (2021) reported that 96% of MQTL had a trade-off between TGW and GNS. In this study, three stable QTLs for TGW (QTgw.cib-2A.2, QTgw.cib-6A, and QTgw.cib-6D1) with superior alleles from ZKM138 were detected. The combination of two or three of them showed a significant additive effect on TGW and had no noticeable negative effect on GNS (Figure 3), suggesting that they have potential value for wheat breeding. Similar results were reported by Griffiths et al. (2015) and Golan et al. (2019). Golan et al. (2019) found a unique GNI-A1 allele in wild emmer that promoted grain weight without significantly affecting grain number, contributing to breeding efforts to enhance grain yield.
Linked maker is useful for conveniently detecting target loci (Collard and Mackill, 2008). In this study, a major stable QTL QTgw.cib-6A for TGW and grain size was mapped and finally narrowed to a 213.1-217.2 Mb region (∼4.1 Mb) on chromosome 6A with a tightly linked KASP marker (KASP6A-2121) (Figure 4). In previous studies, linked markers were used to investigate the transmissibility (Fan et al., 2018) and distribution (Liu et al., 2020) of a particular genotype of a target QTL. These reports found that many favorable alleles or genotypes have been widely used in genetic improvement (Chen et al., 2022; Deng et al., 2022). For instance, the detection of functional markers of FT-D1 (Chen et al., 2022) and TaGW2 (Su et al., 2011) revealed that their superior haplotypes had been frequently selected among Chinese varieties. For instance, the frequency of the positive allele of TaGW2 has gradually increased (from 58.3 to 77.4%) in recent decades. Additionally, the excellent allele of FT-D1 occurs at a high frequency (more than 60%) in different worldwide wheat regions (Chen et al., 2022). The high frequency and range of applications of these well-known genes indicate their large contributions to yield improvement in the artificial breeding process. On the other hand, a possible novel gene with a relatively low frequency distribution might provide a new target for genetic improvement and an extra opportunity for further yield enhancement.
In this study, among the 79 derivatives of ZKM138, the strong artificial selection of the ZKM138 genotype representing high TGW was observed (88.6%), possibly because its effect on increasing yield has been passively noticed and selected during the breeding process, mainly with ZKM138 used as a parent. In addition, only 4.97% (31/624) of the worldwide varieties carried superior genotypes increasing TGW, with the highest frequency 11.32% (18/159) in Southwestern Wheat Zone in China, suggesting that this locus more likely harbored a new and underutilized gene controlling TGW with potential application value in the subsequent high-yield variety breeding process.
In this study, we performed QTL analysis using seven datasets from the BC-RILs via a new genetic map based on the Wheat 50K SNP array. A total of ninety-one QTLs for TGW and GRTs were detected on fourteen chromosomes. Among them, thirty-six stable QTLs were identified in more than two datasets, and eight genomic clusters were highlighted. The pyramiding of three of the QTLs (QTgw.cib-2A.2, QTgw.cib-6A, and QTgw.cib-6D1) for TGW was found to contribute greatly to high wheat yield, which might primarily support the excellent grain weight and yield performance of ZKM138. Notably, QTgw.cib-6A was identified in all datasets and finally delimitated to a physical interval of approximately 4.1 Mb. Further transmissibility and geographic pattern analysis revealed that the superior allele was selected during our actual breeding process based on ZKM138 but is still rare in worldwide modern wheat varieties. Thus, the superior allele of QTgw.cib-6A and its linked markers might be valuable for further improving TGW and yield.
The data presented in the study are deposited in the NCBI repository, accession number: PRJNA850613.
XL undertook the field trials and subsequent analysis of all available data including the phenotyping and population genotyping and drafted this manuscript. ZX assisted in field trials. BF, QZ, GJ, SL, SG, and DL participated in phenotyping. ZX, XF, and TW developed the BC population. XL, XF, ZX, and TW discussed the results. XF and TW designed the experiments, guided the entire study, participated in data analysis, discussed the results, and revised the manuscript. All authors contributed to the article and approved the submitted version.
This work was supported by the Strategic Priority Research Program of Chinese Academy of Sciences (no. XDA24030402) and the National Natural Science Foundation of China (no. 31971934).
The authors declare that the research was conducted in the absence of any commercial or financial relationships that could be construed as a potential conflict of interest.
All claims expressed in this article are solely those of the authors and do not necessarily represent those of their affiliated organizations, or those of the publisher, the editors and the reviewers. Any product that may be evaluated in this article, or claim that may be made by its manufacturer, is not guaranteed or endorsed by the publisher.
The Supplementary Material for this article can be found online at: https://www.frontiersin.org/articles/10.3389/fpls.2022.967432/full#supplementary-material
Beales, J., Turner, A., Griffiths, S., Snape, J. W., and Laurie, D. A. (2007). A Pseudo-Response Regulator is misexpressed in the photoperiod insensitive Ppd-D1a mutant of wheat (Triticum aestivum L.). Theor. Appl. Genet. 115, 721–733. doi: 10.1007/s00122-007-0603-4
Borrill, P., Ramirez-Gonzalez, R., and Uauy, C. (2016). expVIP: A customizable RNA-Seq data analysis and visualization platform. Plant Physiol. 170, 2172–2186. doi: 10.1104/pp.15.01667
Cao, S. H., Xu, D. G., Hanif, M., Xia, X. C., and He, Z. H. (2020). Genetic architecture underpinning yield component traits in wheat. Theor. Appl. Genet. 133, 1811–1823. doi: 10.1007/s00122-020-03562-8
Cavanagh, C. R., Chao, S. M., Wang, S. C., and Akhunovet, E. (2013). Genome-wide comparative diversity uncovers multiple targets of selection for improvement in hexaploid wheat landraces and cultivars. PNAS 110, 8057–8062. doi: 10.1073/pnas.1217133110
Chen, C. J., Chen, H., Zhang, Y., Thomas, H. R., Frank, M. H., He, Y. H., et al. (2020a). TBtools: An integrative toolkit developed for interactive analyses of big biological data. Mol. Plant 13, 1194–1202. doi: 10.1016/j.molp.2020.06.009
Chen, Y., Yan, Y., Wu, T. T., Zhang, G. L., Yin, H. R., Chen, W., et al. (2020b). Cloning of wheat keto-acyl thiolase 2B reveals a role of jasmonic acid in grain weight determination. Nat. Commun. 11:6626. doi: 10.1038/s41467-020-20133-z
Chen, G. F., Wu, R. G., Li, D. M., Yu, H. X., Deng, Z. Y., and Tian, J. C. (2017). Genomewide association study for seeding emergence and tiller number using SNP markers in an elite winter wheat population. J. Genet. 96, 177–186. doi: 10.1007/s12041-016-0731-1
Chen, Z. Y., Cheng, X. J., Chai, L. L., Wang, Z. H., Bian, R., Li, J., et al. (2019). Dissection of genetic factors underlying grain size and fine mapping of QTgw.cau-7D in common wheat (Triticum aestivum L.). Theor. Appl. Genet. 133, 149–162. doi: 10.1007/s00122-019-03447-5
Chen, Z. Y., Ke, W. S., He, F., Chai, L. L., Cheng, X. J., Xu, H. W., et al. (2022). A single nucleotide deletion in the third exon of FT-D1 increases the spikelet number and delays heading date in wheat (Triticum aestivum L.). Plant Biotechnol. J. 20, 920–933. doi: 10.1111/pbi.13773
Cheng, R. R., Kong, Z. X., Zhang, L. W., Xie, Q., Jia, H. Y., Yu, D., et al. (2017). Mapping QTLs controlling kernel dimensions in a wheat inter-varietal RIL mapping population. Theor. Appl. Genet. 130, 1405–1414. doi: 10.1007/s00122-017-2896-2
Cheng, X. J., Xin, M. M., Xu, R. B., Chen, Z. Y., Cai, W. L., Chai, L. L., et al. (2020). A single amino acid substitution in STKc_GSK3 kinase conferring semispherical grains and its implications for the origin of Triticum sphaerococcum. Plant Cell. 32, 923–934. doi: 10.1105/TPC.19.00580
Collard, B. C. Y., and Mackill, D. J. (2008). Marker-assisted selection: An approach for precision plant breeding in the twenty-first century. Philos. Trans. R. Soc. Lond. B Biol. Sci. 363, 557–572. doi: 10.1098/rstb.2007.2170
Cui, F., Zhang, N., Fan, X. L., Zhang, W., Zhao, C. H., Yang, L. J., et al. (2017). Utilization of a Wheat 660K SNP array-derived high-density genetic map for high-resolution mapping of a major QTL for kernel number. Sci. Rep. 7:3788. doi: 10.1038/s41598-017-04028-6
Cui, F., Zhao, C. H., Ding, A. M., Li, J., Wang, L., Li, X. F., et al. (2014). Construction of an integrative linkage map and QTL mapping of grain yield-related traits using three related wheat RIL populations. Theor. Appl. Genet. 127, 659–675. doi: 10.1007/s00122-013-2249-8
Deng, M., Li, L., Cheng, Y. K., Yao, F. J., Guan, F. N., Wang, Y. Q., et al. (2022). Mapping a stable adult-plant stripe rust resistance QTL on chromosome 6AL in Chinese wheat landrace Yibinzhuermai. Crop J. 10, 1111–1119. doi: 10.1016/j.cj.2021.10.011
Dixon, L. E., Greenwood, J. R., Bencivenga, S., Zhang, P., Cockram, J., Mellers, G., et al. (2018). TEOSINTE BRANCHED1 regulates inflorescence architecture and development in cread wheat (Triticum aestivum). Plant Cell 30, 563–581. doi: 10.1105/tpc.17.00961
Du, D. F., Gao, X., Geng, J., Li, Q. Y., Li, L. Q., Lv, Q., et al. (2016). Identification of key proteins and networks related to grain development in wheat (Triticum aestivum L.) by comparative transcription and proteomic analysis of allelic variants in TaGW2-6A. Front. Plant Sci. 7:922. doi: 10.3389/fpls.2016.00922
Fan, X. L., Cui, F., Ji, J., Zhang, W., Zhao, X. Q., Liu, J. J., et al. (2019). Dissection of pleiotropic QTL regions controlling wheat spike characteristics under different nitrogen treatments using traditional and conditional QTL mapping. Front. Plant Sci. 10:187. doi: 10.3389/fpls.2019.00187
Fan, X. L., Zhang, W., Zhang, N., Chen, M., Zheng, S. S., Zhao, G. H., et al. (2018). Identification of QTL regions for seedling root traits and their effect on nitrogen use efficiency in wheat (Triticum aestivum L.). Theor. Appl. Genet. 131, 2677–2698. doi: 10.1007/s00122-018-3183-6
Fatiukha, A., Filler, N., Lupo, I., Lidzbarsky, G., Klymiuk, V., Korol, A. B., et al. (2020). Grain protein content and thousand kernel weight QTLs identified in a durum×wild emmer wheat mapping population tested in five environments. Theor. Appl. Genet. 133, 119–131. doi: 10.1007/s00122-019-03444-8
Gegas, V. C., Nazari, A., Griffiths, S., Simmonds, J., Fish, L., Orford, S., et al. (2010). A genetic framework for grain size and shape variation in wheat. Plant Cell. 22, 1046–1056. doi: 10.1105/tpc.110.074153
Golan, G., Ayalon, I., Perry, A., Zimran, G., Ade-Ajayi, T., Mosquna, A., et al. (2019). GNI-A1 mediates trade-off between grain number and grain weight in tetraploid wheat. Theor. Appl. Genet. 132, 2353–2365. doi: 10.1007/s00122-019-03358-5
Griffiths, S., Wingen, L., Pietragalla, J., Garcia, G., Hasan, A., Miralles, D., et al. (2015). Genetic dissection of grain size and grain number trade-offs in CIMMYT wheat germplasm. PLoS One 10:e0118847. doi: 10.1371/journal.pone.0118847
Guan, P. F., Lu, L. H., Jia, L. J., Kabir, M. R., Zhang, J. B., Lan, T. Y., et al. (2018). Global QTL analysis identifies genomic regions on chromosomes 4A and 4B harboring stable loci for yield-related traits across different environments in wheat (Triticum aestivum L.). Front. Plant Sci. 9:529. doi: 10.3389/fpls.2018.00529
Guan, P. F., Shen, X. Y., Mu, Q., Wang, Y. F., Chen, Y. M., Zhao, Y., et al. (2020). Dissection and validation of a QTL cluster linked to Rht-B1 locus controlling grain weight in common wheat (Triticum aestivum L.) using near-isogenic lines. Theor. Appl. Genet. 133, 2639–2653. doi: 10.1007/s00122-020-03622-z
Guo, Y., Sun, J. J., Zhang, G. Z., Wang, Y. Y., Kong, F. M., Zhao, Y., et al. (2013). Haplotype, molecular marker and phenotype effects associated with mineral nutrient and grain size traits of TaGS1a in wheat. Field Crop Res. 154, 119–125. doi: 10.1016/j.fcr.2013.07.012
Hanif, M., Gao, F. M., Liu, J. D., Wen, W. E., Zhang, Y. J., Rasheed, A., et al. (2016). TaTGW6-A1, an ortholog of rice TGW6, is associated with grain weight and yield in bread wheat. Mol. Breeding 36:1. doi: 10.1007/s11032-015-0425-z
Hou, J., Jiang, Q. Y., Hao, C. Y., Wang, Y. Q., Zhang, H. N., Zhang, X. Y., et al. (2014). Global selection on sucrose synthase haplotypes during a century of wheat breeding. Plant Physiol. 164, 1918–1929. doi: 10.1104/pp.113.232454
Hu, J. M., Wang, X. Q., Zhang, G. X., Jiang, P., Cheng, W. Y., Hao, Y. C., et al. (2020). QTL mapping for yield-related traits in wheat based on four RIL populations. Theor. Appl. Genet. 133, 917–933. doi: 10.1007/s00122-019-03515-w
Hu, M. J., Zhang, H. P., Liu, K., Cao, J. J., Wang, S. X., Jiang, H., et al. (2016). Cloning and characterization of TaTGW-7A gene associated with grain weight in wheat via SLAF-seq-BSA. Front. Plant Sci. 7:e0145970. doi: 10.3389/fpls.2016.01902
Jain, S., Jain, R. K., and McCouch, S. R. (2004). Genetic analysis of Indian aromatic and quality rice (Oryza sativa L.) germplasm using panels of fluorescently-labeled microsatellite markers. Theor. Appl. Genet. 109, 965–977. doi: 10.1007/s00122-004-1700-2
Jaiswal, V., Gahlaut, V., Mathur, S., Agarwal, P., Khandelwal, M. K., Khurana, J. P., et al. (2015). Identification of novel SNP in promoter sequence of TaGW2-6A associated with grain weight and other agronomic traits in wheat (Triticum aestivum L.). PLoS One 10:e129400. doi: 10.1371/journal.pone.0129400
Jia, H. Y., Wan, H. S., Yang, S. H., Zhang, Z. Z., Kong, Z. X., Xue, S. L., et al. (2013). Genetic dissection of yield-related traits in a recombinant inbred line population created using a key breeding parent in China’s wheat breeding. Theor. Appl. Genet 126, 2123–2139. doi: 10.1007/s00122-013-2123-8
Jiang, Q. Y., Hou, J., Hao, C. Y., Wang, L. F., Ge, H. M., Dong, Y. S., et al. (2011). The wheat (T. aestivum) sucrose synthase 2 gene (TaSus2) active in endosperm development is associated with yield traits. Funct. Integr. Genomic. 11, 49–61. doi: 10.1007/s10142-010-0188-x
Jiang, Y. M., Jiang, Q. Y., Hao, C. Y., Hou, J., Wang, L. F., Zhang, H. N., et al. (2015). A yield-associated gene TaCWI, in wheat: Its function, selection and evolution in global breeding revealed by haplotype analysis. Theor. Appl. Genet. 128, 131–143. doi: 10.1007/s00122-014-2417-5
King, J. L., Grewal, S., Yang, C. Y., Hubbart, S., Scholefield, D., Ashling, S., et al. (2017). A step change in the transfer of interspecific variation into wheat from Amblyopyrum muticum. Plant. Biotechnol. J. 15, 217–226. doi: 10.1111/pbi.12606
Kovach, M. J., Sweeney, M. T., and Mccouch, S. R. (2007). New insights into the history of rice domestication. Trends Genet. 23, 578–587. doi: 10.1016/j.tig.2007.08.012
Kumar, A., Mantovani, E. E., Seetan, R., Soltani, A., Echeverry-Solarte, M., Jain, S., et al. (2016). Dissection of genetic factors underlying wheat kernel shape and size in an Elite × Nonadapted cross using a high density SNP linkage map. Plant Genome 9, 1–22. doi: 10.3835/plantgenome2015.09.0081
Lee, H. S., Jung, J., Kang, C., Heo, H., and Park, C. S. (2014). Mapping of QTL for yield and its related traits in a doubled haploid population of Korean wheat. Plant Biotechnol. Rep. 8, 443–454. doi: 10.1007/s11816-014-0337-0
Li, T., Deng, G. B., Su, Y., Yang, Z., Tang, Y. Y., Wang, J. H., et al. (2022). Genetic dissection of quantitative trait loci for grain size and weight by high-resolution genetic mapping in bread wheat (Triticum aestivum L.). Theor. Appl. Genet. 135, 257–271. doi: 10.1007/s00122-021-03964-2
Li, X. P., Zhao, X. Q., He, X., Zhao, G. Y., Li, B., Liu, D. C., et al. (2011). Haplotype analysis of the genes encoding glutamine synthetase plastic isoforms and their association with nitrogen-use- and yield-related traits in bread wheat. New Phytol. 189, 449–458. doi: 10.1111/j.1469-8137.2010.03490.x
Liu, G., Jia, L. J., Lu, L. H., Qin, D. D., Zhang, J. P., Guan, P. F., et al. (2014). Mapping QTLs of yield-related traits using RIL population derived from common wheat and Tibetan semi-wild wheat. Theor. Appl. Genet. 127, 2415–2432. doi: 10.1007/s00122-014-2387-7
Liu, J., Wu, B. H., Singh, R. P., and Velu, G. (2019). QTL mapping for micronutrients concentration and yield component traits in a hexaploid wheat mapping population. J. Cereal. Sci. 88, 57–64. doi: 10.1016/j.jcs.2019.05.008
Liu, T., Wu, L. J., Gan, X. L., Chen, W. J., Liu, B. L., Fedak, G., et al. (2020). Mapping quantitative trait loci for 1000-grain weight in a double haploid population of common wheat. Int. J. Mol. Sci. 21:3960. doi: 10.3390/ijms21113960
Ma, D. Y., Yan, J., He, Z. H., Wu, L., and Xia, X. C. (2012). Characterization of a cell wall invertase gene TaCwi-A1 on common wheat chromosome 2A and development of functional markers. Mol. Breeding 29, 43–52. doi: 10.1007/s11032-010-9524-z
Ma, F. F., Xu, Y. F., Ma, Z. Q., Li, L. H., and An, D. G. (2018). Genome-wide association and validation of key loci for yield-related traits in wheat founder parent Xiaoyan 6. Mol. Breeding 38:91. doi: 10.1007/s11032-018-0837-7
Mangini, G., Blanco, A., Nigro, D., Signorile, M. A., and Simeone, R. (2021). Candidate genes and quantitative trait loci for grain yield and seed size in durum wheat. Plants 10:312. doi: 10.3390/plants10020312
Meng, L., Li, H. H., Zhang, L. Y., and Wang, J. K. (2015). QTL IciMapping: Integrated software for genetic linkage map construction and quantitative trait locus mapping in biparental populations. Crop. J. 3, 269–283. doi: 10.1016/j.cj.2015.01.001
Mohler, V., Albrecht, T., Castell, A., Diethelm, M., Schweizer, G., and Hartl, L. (2016). Considering causal genes in the genetic dissection of kernel traits in common wheat. J. Appl. Genet. 57, 467–476. doi: 10.1007/s13353-016-0349-2
Muqaddasi, Q. H., Jayakodi, M., Rörner, A., and Röder, M. S. (2019). Identification of consistent QTL with large effect on anther extrusion in doubled haploid populations developed from spring wheat accessions in German Federal exsitu Genebank. Theor. Appl. Genet. 132, 3035–3045. doi: 10.1007/s00122-019-03404-2
Peleg, Z., Fahima, T., Korol, A. B., Abbo, S., and Saranga, Y. (2011). Genetic analysis of wheat domestication and evolution under domestication. J. Exp. Bot. 62, 5051–5061. doi: 10.1093/jxb/err206
Peng, J. H., Ronin, Y., Fhima, T., Röder, M. S., Li, Y. C., Nevo, E., et al. (2003). Domestication quantitative trait loci in Triticum dicoccoides, the progenitor of wheat. PNAS 100, 2489–2494. doi: 10.1073/pnas.252763199
Peng, J. R., Richards, D. E., Hartley, N. M., Murphy, G. P., Devos, K. M., Flintham, J. E., et al. (1999). ‘Green revolution’ genes encode mutant gibberellin response modulators. Nature 400, 256–261. doi: 10.1038/22307
Ramírez-González, R. H., Borrill, P., Lang, D., Harrington, S. A., Brinton, J., Venturini, L., et al. (2018). The transcriptional landscape of polyploid wheat. Science 361:eaar6089. doi: 10.1126/science.aar6089
Sajjad, M., Ma, X. L., Khan, S. H., Shoaib, M., Yan, H. S., Wen, L. Y., et al. (2017). TaFlo2-A1, an ortholog of rice Flo2, is associated with thousand grain weight in bread wheat (Triticum aestivum L.). BMC Plant Biol. 17:164. doi: 10.1186/s12870-017-1114-3
Schulthess, A. W., Reif, J. C., Ling, J., Plieske, J., Kollers, S., Ebmeyer, E., et al. (2017). The roles of pleiotropy and close linkage as revealed by association mapping of yield and correlated traits of wheat (Triticum aestivum L.). J. Exp. Bot. 68, 4089–4101. doi: 10.1093/jxb/erx214
Simmonds, J., Scott, P., Brinton, J., Mestre, T. C., Bush, M., Blanco, A. D., et al. (2016). A splice acceptor site mutation in TaGW2-A1 increases thousand grain weight in tetraploid and hexaploid wheat through wider and longer grains. Theor. Appl. Genet. 129, 1099–1112. doi: 10.1007/s00122-016-2686-2
Simmonds, J., Scott, P., Leverington-Waite, M., Turner, A. S., Brinton, J., Korzun, V., et al. (2014). Identification and independent validation of a stable yield and thousand grain weight QTL on chromosome 6A of hexaploid wheat (Triticum aestivum L.). BMC Plant Biol. 14:191. doi: 10.1186/s12870-014-0191-9
Smith, S. E., Kuehl, R. O., Ray, I. M., Hui, R., and Soleri, D. (1998). Evaluation of simple methods for estimating broad-sense heritability in stands of randomly planted genotypes. Crop. Sci. 38, 1125–1129. doi: 10.2135/cropsci1998.0011183X003800050003x
Song, P. B., Wang, X., Wang, X. X., Zhou, F., Xu, X. W., Wu, B. J., et al. (2021). Application of 50K Chip-based genetic map to QTL mapping of stem-related traits in wheat. Crop. Pasture Sci. 72, 105–112. doi: 10.1071/CP20326
Su, Q. N., Zhang, X. L., Zhang, W., Zhang, N., Song, L. Q., Liu, L., et al. (2018). QTL detection for kernel size and weight in bread wheat (Triticum aestivum L.) using a high-density SNP and SSR-based linkage map. Front. Plant Sci. 9:1484. doi: 10.3389/fpls.2018.01484
Su, Z. Q., Hao, C. Y., Wang, L. F., Dong, Y. C., and Zhang, X. Y. (2011). Identification and development of a functional marker of TaGW2 associated with grain weight in bread wheat (Triticum aestivum L.). Theor. Appl. Genet. 122, 211–223. doi: 10.1007/s00122-010-1437-z
Sukumaran, S., Lopes, M., Dreisigacker, S., and Reynolds, M. (2018). Genetic analysis of multi-environmental spring wheat trials identifies genomic regions for locus-specific trade-offs for grain weight and grain number. Theor. Appl. Genet. 131, 985–998. doi: 10.1007/s00122-017-3037-7
Sun, C. W., Zhang, F. Y., Yan, X. F., Zhang, X. F., Dong, Z. D., Cui, D. Q., et al. (2017). Genome-wide association study for 13 agronomic traits reveals distribution of superior alleles in bread wheat from the Yellow and Huai Valley of China. Plant Biotechnol. J. 15, 953–969. doi: 10.1111/pbi.12690
Sun, L. Q., Huang, S. S., Sun, G. L., Zhang, Y. J., Hu, X., Nevo, E., et al. (2020). SNP-based association study of kernel architecture in a worldwide collection of durum wheat germplasm. PLoS One 15:e0229159. doi: 10.1371/journal.pone.0229159
Wang, S. C., Wong, D., Forrest, K., Forrest, K., Allen, A., Chao, S. M., et al. (2014). Characterization of polyploid wheat genomic diversity using a high-density 90 000 single nucleotide polymorphism array. Plant Biotechnol. J. 12, 787–796. doi: 10.1111/pbi.12183
Wang, X. Q., Dong, L. H., Hu, J. M., Pang, Y. L., Hu, L. Q., Xiao, G. L., et al. (2019a). Dissecting genetic loci affecting grain morphological traits to improve grain weight via nested association mapping. Theor. Appl. Genet. 132, 3115–3128. doi: 10.1007/s00122-019-03410-4
Wang, Y. M., Hou, J., Liu, H., Li, H., Wang, K., Hao, C. Y., et al. (2019b). TaBT1, affecting starch synthesis and thousand kernel weight, underwent strong selection during wheat improvement. J. Exp. Bot. 70, 1497–1511. doi: 10.1093/jxb/erz032
Winfield, M. O., Allen, A. M., Burridge, A. J., Barker, G. L. A., Benbow, H. R., Wilkinson, P. A., et al. (2016). High-density SNP genotyping array for hexaploid wheat and its secondary and tertiary gene pool. Plant Biotechnol. J. 14, 1195–1206. doi: 10.1111/pbi.12485
Würschum, T., Leiser, W. L., Langer, S. M., Tucker, M. R., and Longin, C. F. H. (2018). Phenotypic and genetic analysis of spike and kernel characteristics in wheat reveals long-term genetic trends of grain yield components. Theor. Appl. Genet. 131, 2071–2084. doi: 10.1007/s00122-018-3133-3
Xie, Q., and Sparkes, D. L. (2021). Dissecting the trade-off of grain number and size in wheat. Planta 254:3. doi: 10.1007/s00425-021-03658-5
Xin, F., Zhu, T., Wei, S. W., Han, Y. C., Zhao, Y., Zhang, D. Z., et al. (2020). QTL mapping of kernel traits and validation of a major QTL for kernel length-width ratio using SNP and bulked segregant analysis in wheat. Sci. Rep. 10:25. doi: 10.1038/s41598-019-56979-7
Yang, Z. B., Bai, Z. Y., Li, X. L., Wang, P., Wu, Q. X., Yang, L., et al. (2012). SNP identification and allelic-specific PCR markers development for TaGW2, a gene linked to wheat kernel weight. Theor. Appl. Genet. 125, 1057–1068.
Yao, J., Wang, L. X., Liu, L. H., Zhao, C. P., and Zheng, Y. L. (2009). Association mapping of agronomic traits on chromosome 2A of wheat. Genetica 137, 67–75. doi: 10.1007/s10709-009-9351-5
Zhai, H. J., Feng, Z. Y., Du, X. F., Song, Y. N., Liu, X. Y., Qi, Z. Q., et al. (2018). A novel allele of TaGW2-A1 is located in a finely mapped QTL that increases grain weight but decreases grain number in wheat (Triticum aestivum L.). Theor. Appl. Genet. 131, 539–553. doi: 10.1007/s00122-017-3017-y
Zhang, B., Liu, X., Xu, W. N., Chang, J. Z., Li, A., Mao, X. G., et al. (2015). Novel function of a putative MOC1 ortholog associated with spikelet number per spike in common wheat. Sci. Rep. 5:12211. doi: 10.1038/srep12211
Zhang, P. F., He, Z. H., Tian, X. L., Gao, F. M., Xu, D. G., Liu, J. D., et al. (2017). Cloning of TaTPP-6AL1 associated with grain weight in bread wheat and development of functional marker. Mol. Breeding 37:78. doi: 10.1007/s11032-017-0676-y
Zhang, Y., Li, D., Zhang, D. B., Zhao, X. G., Cao, X. M., Dong, L. L., et al. (2018). Analysis of the functions of TaGW2 homoeologs in wheat grain weight and protein content traits. Plant J. 94, 857–866. doi: 10.1111/tpj.13903
Zhang, Y. J., Liu, J. D., Xia, X. C., and He, Z. H. (2014). TaGS-D1, an ortholog of rice OsGS3, is associated with grain weight and grain length in common wheat. Mol. Breeding 34, 1097–1107. doi: 10.1007/s11032-014-0102-7
Keywords: wheat, genetic map, QTL analysis, grain weight, breeding value
Citation: Liu X, Xu Z, Feng B, Zhou Q, Ji G, Guo S, Liao S, Lin D, Fan X and Wang T (2022) Quantitative trait loci identification and breeding value estimation of grain weight-related traits based on a new wheat 50K single nucleotide polymorphism array-derived genetic map. Front. Plant Sci. 13:967432. doi: 10.3389/fpls.2022.967432
Received: 12 June 2022; Accepted: 04 August 2022;
Published: 30 August 2022.
Edited by:
Luigi Cattivelli, Council for Agricultural and Economics Research, ItalyReviewed by:
Ana Pontaroli, BioLumic Limited, New ZealandCopyright © 2022 Liu, Xu, Feng, Zhou, Ji, Guo, Liao, Lin, Fan and Wang. This is an open-access article distributed under the terms of the Creative Commons Attribution License (CC BY). The use, distribution or reproduction in other forums is permitted, provided the original author(s) and the copyright owner(s) are credited and that the original publication in this journal is cited, in accordance with accepted academic practice. No use, distribution or reproduction is permitted which does not comply with these terms.
*Correspondence: Xiaoli Fan, fanxl@cib.ac.cn; Tao Wang, wangtao@cib.ac.cn
Disclaimer: All claims expressed in this article are solely those of the authors and do not necessarily represent those of their affiliated organizations, or those of the publisher, the editors and the reviewers. Any product that may be evaluated in this article or claim that may be made by its manufacturer is not guaranteed or endorsed by the publisher.
Research integrity at Frontiers
Learn more about the work of our research integrity team to safeguard the quality of each article we publish.