- Key Laboratory of Germplasm Enhancement, Physiology and Ecology of Food Crops in Cold Region, Ministry of Education, Northeast Agricultural University, Harbin, China
Soil salt-alkalization is a common yet critical environmental stress factor for plant growth and development. Discovering and exploiting genes associated with alkaline tolerance in maize (Zea mays L.) is helpful for improving alkaline resistance. Here, an association panel consisting of 200 maize lines was used to identify the genetic loci responsible for alkaline tolerance-related traits in maize seedlings. A total of nine single-nucleotide polymorphisms (SNPs) and their associated candidate genes were found to be significantly associated with alkaline tolerance using a genome-wide association study (GWAS). An additional 200 genes were identified when the screen was extended to include a linkage disequilibrium (LD) decay distance of r2 ≥ 0.2 from the SNPs. RNA-sequencing (RNA-seq) analysis was then conducted to confirm the linkage between the candidate genes and alkali tolerance. From these data, a total of five differentially expressed genes (DEGs; |log2FC| ≥ 0.585, p < 0.05) were verified as the hub genes involved in alkaline tolerance. Subsequently, two candidate genes, Zm00001d038250 and Zm00001d001960, were verified to affect the alkaline tolerance of maize seedlings by qRT-PCR analysis. These genes were putatively involved protein binding and “flavonoid biosynthesis process,” respectively, based on Kyoto Encyclopedia of Genes and Genomes (KEGG) and Gene Ontology (GO) enrichment analyses. Gene promoter region contains elements related to stress and metabolism. The results of this study will help further elucidate the mechanisms of alkaline tolerance in maize, which will provide the groundwork for future breeding projects.
Introduction
Soil salt-alkalization is a major source of stress to plants, and therefor a threat to agricultural development and crop productivity (Zhu, 2016). Due to the increase in global temperature caused by human activity and industrial development, the serious evaporation of soil water, and the lack of drainage consideration during irrigation has led to the deepening of land salinization, threatening crop yields. The effect of salty soils on plants includes salt stress caused by neutral salt (NaCl and Na2SO4; Xu et al., 2019), alkaline salt stress caused by alkaline salt (NaHCO3 and Na2CO3; Yang et al., 2019), and saline-alkali stress caused by both alkaline and neutral salt (Shi and Sheng, 2005). Several studies have shown that alkaline salts, due to their high pH, are more dangerous to plants than neutral salts (Campbell and Nishio, 2000; Hartung et al., 2002; Chen et al., 2012).
Saline-alkali stress induces a variety of plant responses, including changes in plants’ physiological, biochemical, and morphological structures. It also causes ionic imbalance and changes in osmotic pressure (100 mM NaCl; Chaparzadeh et al., 2004; Ambede et al., 2012; Abreu et al., 2013; Mishra et al., 2013). For example, stress accumulation of Na+ leads to competition with K+ for protein binding, which inhibits protein synthesis (Schachtman and Liu, 1999; Pardo and Quintero, 2002). Physiologically speaking, salt stress reduced stomatal number, stomatal density, and photosynthesis, and increased the respiration rate in plants (100 and 200 mM NaCl) (Dikobe et al., 2021). Biochemically, enzyme content such as amount of soluble proteins, total free amino acids, prolines, Na+, and malondialdehyde was increased under alkaline treatment (75 mM Na2CO3) (Abdel Latef and Tran, 2016). Saline soil also leads to decreased seed vigor, reduced germination, damaged root cell structure, reduced nutrient absorption and utilization, and ultimately reduced yield as a culmination of all these factors (mixed salts of NaCl, Na2SO4, NaHCO3, and Na2CO3; Peng et al., 2008; Gao et al., 2014). For example, under different concentrations of saline irrigation, maize yield decreased by 2.08%–3.93% with an increase of the salt concentration (NaCl; Feng et al., 2017). Soil salinization affected the physiological and biochemical indicators, or other resistance, ultimately leading to yield impacts.
Maize (Zea mays L.) is an important cereal crop in the world. Among the cereal crops, it is the most sensitive to alkalinity. Therefore, exploiting genes associated with alkaline tolerance is helpful for improving alkaline resistance in this crop plant. However, not many genes have been associated with alkaline tolerance. Currently, there has been some progress in identifying the genetic loci associated with these tolerances in crop plants. For instance, a major quantitative trait locus (QTL) for photosynthetic alkali tolerance was detected for two consecutive years by the RIL population in rice (8.5 mM Na2CO3; Sun et al., 2019). Three pairs of additive by additive (AA) epistatic QTLs associated with dead leaf rate under alkali tolerance were also identified (pH 8.7 to 8.9, natural alkaline soil; Liang et al., 2014). In maize, researchers identified two QTLs associated with alkali tolerance by using simple sequence repeats (SSR) and specific locus amplified fragment-sequencing (SLAF-seq) markers through 151 F2:3 populations (100 mM Na2CO3; Zhang et al., 2018). For example, the researchers mapped a major QTL for alkali tolerance on Chr 2 in maize by identifying sodium ion content and validating the candidate gene (100 mM NaHCO3; Cao et al., 2020). When maize was treated with alkaline salt, the ZmNSA1 protein bound to Ca2+ for degradation and made H+ efflux, ultimately promoting saline-alkaline tolerance in the treated plants (100 mM NaHCO3 or NaCl; Cao et al., 2020).
In addition to using QTLs to discover alkaline stress-related traits, GWAS have also been used to detect several SNPs associated with this trait. GWAS has been shown to be an effective method to identify genes and alleles associated with certain agronomic traits under complex environments (Yan et al., 2011; Li et al., 2016). Depending on the rapid decay of LD and amount of diversity, GWAS provides a systematic approach to the analysis of complex quantitative traits in many crops, including maize (Li et al., 2013). In the whole wheat genome, 326,570 SNPs were used in the SNP-GWAS method to unearth 20 SNPs that were significantly associated with grain length and 31 SNPs with grain width, among which the IWB32119 marker located on chromosome 2A was associated with the grain weight locus gene TaFlo2-A1 (Sajjad et al., 2017; Li et al., 2019). As a benefit of the rapid decay of maize LD, numerous loci controlling complex traits have been identified in maize by GWAS. Researchers extracted two candidate genes associated with low-temperature tolerance in maize by GWAS mining and used RNA-seq data analysis to confirm that these genes were in fact related to low-temperature tolerance (Zhang et al., 2020). Seven candidate genes associated with drought were identified by combining GWAS, DEGs, and co-expression analysis (Guo et al., 2020). Development of the functional SNP marker Sh2_rs844805326 for the prediction of sweet corn was achieved using GWAS (Ruanjaichon et al., 2021). GWAS has also been used to mine genes that control complex traits in maize, such as leaf development, plant height, and ear height (Li et al., 2016; Miculan et al., 2021). As useful as the GWAS method is, there are few reports on the mining of maize alkali tolerance-related genes using GWAS.
In this study, a panel of 200 different inbred maize lines was used to analyze ten traits associated with alkali resistance by GWAS. Candidate genes were identified and further confirmed using RNA-seq data find the following: (a) SNPs associated with resistance to alkaline, (b) alkali tolerance maize inbred lines, and (c) relevant candidate genes for future agricultural research and breeding applications.
Materials and methods
Plant materials
A total of 200 inbred maize lines with extensive variation in yield traits and biotic stress tolerances were analyzed in this study (Supplementary Table S1; Weng et al., 2011; Liu et al., 2015; Zhang et al., 2017). The seeds were planted in Harbin, Heilongjiang Province, and produced by manual self-pollination. The field site used was well-managed and free from pests and diseases. After seeds were harvested and dried completely, they were stored in a 4°C seed cabinet until ready for use. The 200 maize inbred lines had a >90% germination rate at 25°C, as shown by a previous study conducted. The alkaline-resistant maize line K10 was analyzed using RNA-seq to assess the expression levels of the whole genome.
Seed germination and alkaline stress treatment during seedling stage
To germinate the maize seeds, they were first sterilized in 10 g/l sodium hypochlorite for 20 min. After rinsing the seeds three times with sterilized distilled water, they were imbibed for 6 h at 25°C, then the seeds were subjected to the standard germination: briefly, 30 sterile seeds were placed between two wet germination papers (Li et al., 2018). Seed germination was defined as the observation of a 0.5 cm radicle emergence. After 48 h of seed germination, the seedlings with the same bud length were selected and transferred to pots to continue to grow, with 5 seedlings per pot and 30 seedlings per treatment. Seeds were grown in the growth chamber with an 18 h, 25°C/6 h, 22°C light/dark, and temperature/light cycle (Ma et al., 2020). When the seedlings reached the three-leaf growth stage, they were treated with ½ Hoagland solution containing 25 mmol/l Na2CO3 every day for 10 days (Geng et al., 2020). The control plants were also irrigated with ½ Hoagland solution. The treatment group was watered with ½ Hoagland every 30 days to prevent the excessive accumulation of Na2CO3. Three independent experiments were used for each treatment and the control.
Phenotypic data measurement and analysis
After Na2CO3 treatment for 10 days, maize roots were collected, rinsed with deionized water, and dried by careful blotting before measurements were taken. For root trait data analysis, the Epson Perfection V800 scanner was used to measure root traits to be analyzed by the Regent WinRHIZO (Canada) software. The root traits were root length (RL), root average diameter (RAD), root surface area (RSA), root volume (RV), and root tip number (RTN). Root fresh weight (RFW) and shoot fresh weight (SFW) were measured immediately after observing no moisture in the seedlings. After drying at 105°C for 30 min, roots were transferred to 80°C to continue to dry until they reached a constant weight, then the root dry weight (RDW) and shoot dry weight (SDW) were measured. A ruler was used to measure seed length (SL). The relative performance of the 10 traits was used as an indicator of alkali tolerance. Different treatments consisted of 30 seedlings. Three seedlings were measured for each trait, and the average value of these measurements was taken.
Phenotypic data for the mean, maximum, minimum, kurtosis, skewedness, variance analysis, and standard deviation for each relative trait were analyzed by IBM SPSS Statistics (20.0).1 Correlation analysis of phenotype data was performed with the “Performance Analytics” package in R and IBM SPSS Statistics (20.0). ANOVA for 10 alkali tolerance-related traits was calculated using IBM SPSS Statistics (20.0). The broad-sense heritability (H2) was estimated using the following formula: H2 = VG/VG + VE, with H2 as the heritability estimate, VG as the genotype variance, and VE as the environmental variance.
Genotypic data and GWAS analysis
Using the Illumina BeadStation 500 G SNP developed by Illumina, 41,110 SNPs were selected from 56,110 SNPs with a minor allele frequency (MAF) > 0.05. SNPs with <20% heterozygosity were used for analysis (Weng et al., 2011; Liu et al., 2015; Zhang et al., 2017). From this, a total of 40,697 SNPs were given for association analysis. 7,742 distributed SNP datasets were assessed for structural parameters by using the STRUCTURE 2.3 software (Evanno et al., 2005). ΔK was calculated using Structure Harvester (Earl and vonHoldt, 2011). Using the software TASSEL 5.0, the kinship information for the 200 inbred lines was estimated.
Using the MLM model in TASSEL 5.0 (Bradbury et al., 2007), the association analysis was performed on the 10 relative traits measured at the seedling stage, using 0.05/Ne to calculate the threshold for association analysis. Because the Bonferroni correction (0.05/41,110 = 1.22e-6) was too conservative, 10 trait-associated loci were considered rare; therefore, the use of a less stringent threshold of −log10(P) > 4 was applied for detecting significant associations (Weng et al., 2011; Zhang et al., 2017; Jia et al., 2020). The Manhattan plot was then generated with the CMplot package in R. When r2 = 0.1, the LD decay was 55, 60, 110, 100, 60, 45, 80, 70, 60, and 110 across chromosomes 1–10, respectively. At r2 = 0.2, the LD decay was 395, 520, 710, 610, 770, 575, 775, 775, 1,125, and 850 across chromosomes 1–10, respectively (Zhang et al., 2020). Significantly associated SNPs with a physical distance less than the LD decay distance that were also located on the same chromosome were defined as one locus. The upstream and downstream ranges of the corresponding LD decay distances for each locus were used to further mining genes. The genetic information of candidate genes was retrieved using the gene model from the B73 RefGen_V4 in the MaizeGDB website.2
Transcriptomic analysis
The maize inbred line K10, which had high alkaline tolerance, was used for RNA-seq analysis. Before germination, the seeds were treated with the same sterilization method described in section Seed Germination and Alkaline Stress Treatment During Seedling Stage. The treated and control groups were sampled after 3 and 4 days Na2CO3 treatment, at the three-leaf stage. Leaves were taken from each plant, frozen in liquid nitrogen, and cryopreserved at −80°C until RNA extraction with Trizol. The maize RNA was sent to DATA Corporation (Beijing, China) for library construction. Genomic DNA was removed using DNase I and mRNA was enriched using oligo (dT) magnetic beads and subsequently fragmented. The fragmented products were then amplified by PCR and sequenced after the samples passed the in-house quality inspection.
For our data analysis, the maize genome ZmB73RefGenv43 was used as the reference genome. Gene expression was calculated using Cuffdiff software and expressed as fragments per kilobase of transcript per million fragments mapped (FPKM) value. The threshold for the significant differential expression of genes was set to p < 0.05 and |log2FC| ≥ 0.585.
Identification and annotation of candidate genes
GO enrichment analysis was used to identify all GO terms for DEGs that were significantly enriched in background genes. All DEGs utilized publicly available databases4 for GO term classification and grouping. A hyper-geometric test and Phytozome5 were used to identify significantly enriched GO terms. GO terms were divided into three categories: “biological process,” “cellular component,” and “molecular function” (Du et al., 2010). To further understand the biological functions of DEGs, enrichment analysis was performed using the KEGG pathway6 (Kanehisa et al., 2008). Genes were annotated using the MaizeGDB and NCBI7 databases. Heatmaps were plotted using an online site.8
Quantitative real-time PCR analysis
A qRT-PCR assay was performed using the same total RNA used for the transcriptome data analysis of the alkaline-tolerant inbred line K10 and the alkaline-sensitive inbred line Mo17. For each sample, the TransScript® One-Step gDNA Removal Kit and cDNA Synthesis SuperMix (TransGen Biotech, Beijing, China) was used to synthesize cDNA. The real-time PCR System (Analytik Jena, Germany) was used for qRT-PCR analysis. As a control, actin was used to normalize the obtained Ct values. Primer5.0 was used to design specific primers for this assay (Supplementary Table S2). The 20 μl reaction mixtures consisted of 2 μl cDNA, 7.2 μl of ddH2O, 0.4 μl each of the forward and reverse primers (10 μM), and 10 μl of 2 × TransStart® Tip Green qPCR SuperMix (TransGen Biotech, Beijing, China). Relative gene expression was calculated using the 2−ΔΔCT analytical method (Livak and Schmittgen, 2001). Three biological replicates were combined to be used for three technical replicates, and the average of the three replicates was used for gene expression analysis.
Results
Phenotypic traits related to alkaline tolerance
The alkaline tolerance of 200 inbred lines at the seedling stage was evaluated (Figure 1). The descriptive statistics for alkaline resistant traits RSL, RSFW, RRFW, RSDW, RRDW, RRL, RRAD, RRSA, RRV, and RRTN evaluated in an artificial environment are presented in Table 1. Under both the control and alkaline conditions, the indicators of alkali tolerance were determined, and descriptive statistics were executed for the related characters, including the determination of the maximum value, minimum value, and standard deviation (SD). For these traits, there were abundant phenotypic variations, they ranged from 0.5487 (RSFW) to 1.0847 (RRAD). The RRL varied from 0.2908 to 0.9935, the RRTN varied from 0.1959 to 0.9916, the RSDW varied from 0.2846 to 0.9972, the RSL varied from 0.4709 to 0.9830, the RSFW varied from 0.1857 to 0.9399, the RRFW varied from 0.4069 to 0.9958, the RRDW varied from 0.4448 to 0.9957, the RRSA varied from 0.3679 to 0.9986, the RRV varied from 0.4152 to 0.9971, and the RRAD varied from 0.8180 to 1.4405. Curiously, the RRAD of some inbred lines had a value of >1.000, suggesting that the relative root average diameter of these inbred lines was actually stimulated by the alkali treatment. In general, the 10 traits analyzed in the panel fit a normal distribution. ANOVA tests showed statistically significant genotypic differences for the 10 relative traits. In the association panel, the broad-sense heritability (H2) of the 10 relative traits ranged from 24.00% (RRAD) to 67.30% (RRTN; Supplementary Table S3).
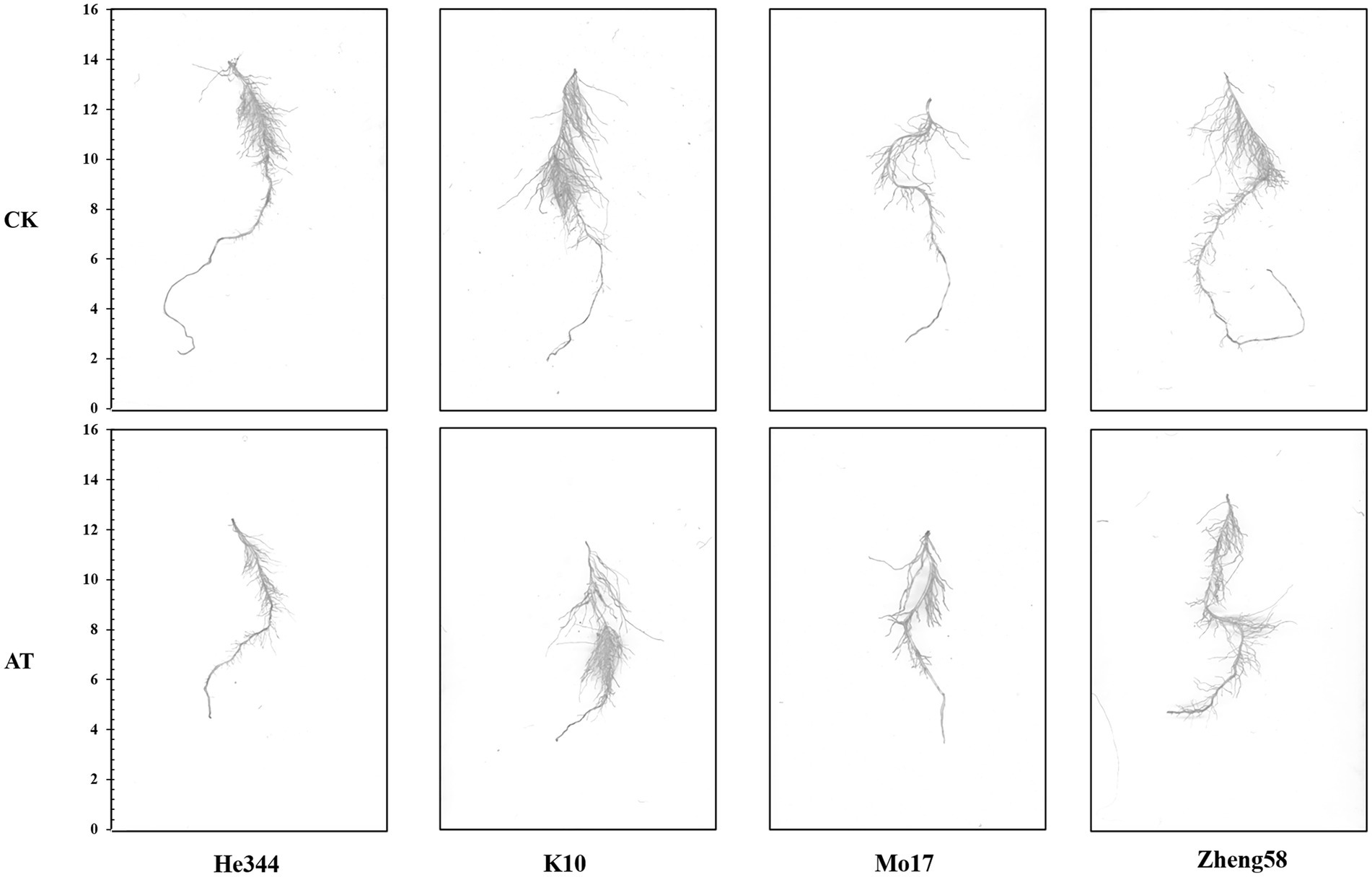
Figure 1. Morphological change in maize under control (CK) and alkaline (AT) treatment. Comparison of roots of maize inbred line He344; inbred line k10; inbred line Mo17; and inbred line Zheng58 under control and alkaline treatment for 10 days. The alkali-sensitive inbred lines Mo17 and He344 are from the Lancaster group, and the alkali-tolerant inbred lines Zheng58 and K10 are from the PA group.
In total, 10 traits were measured (Figure 2) and their correlations with each other were determined using the relative value of each trait under alkali treatment. The traits analyzed at the seedling stage characterized maize growth, indicating that these ten traits can be used as indicators of maize alkali tolerance at the seedling stage. Except for in the case of RRV and RRAD, RSFW and RRAD, RRDW and RRAD, RRAD and the other traits had a significant negative correlation (p < 0.05), such that RRL and RRAD were −0.49, the RRTN and RRAD were −0.42, and the RSL and RRAD were −0.27 (p < 0.001). Except for the RRAD, significant positive correlations were achieved between the other traits (r = 0.17–0.87, p < 0.05). The three traits that were most significantly associated were RRL and RRSA (0.87), RRV and RRSA (0.81), RRL and RRTN (0.71), and RSL and RSDW (0.71; p < 0.001; Supplementary Table S4). The 10 traits were approximated as a normal distribution (kurtosis and skewness< ±1), and showed a significant correlation, indicating the suitability of the 10 traits for further GWAS analysis.
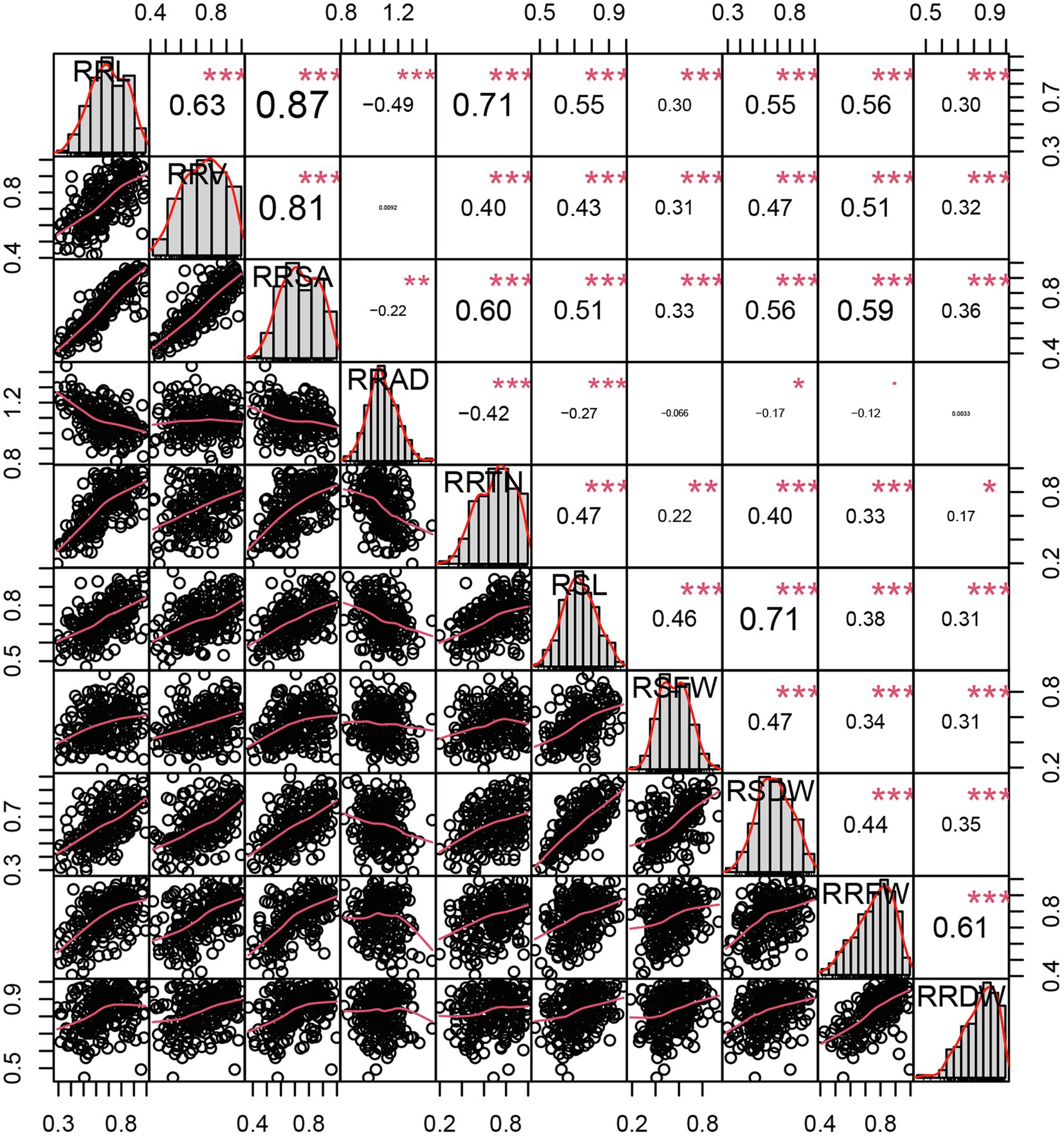
Figure 2. Distributions of and correlations between 10 relative phenotypic traits. The frequency distribution histograms of 10 traits are located on the diagonal line, the area below the diagonal line is the scatter plot of the traits, and the area above is the correlation coefficient between each pair of traits. *, **, and *** indicate significance at p < 0.05, p < 0.01, and p < 0.001, respectively.
Population structure and GWAS analysis
The population structure was calculated using STRUCTURE version 2.3. When K = 6, the 200 inbred lines could be grouped into six large subgroups (Supplementary Figure S1). GWAS was used for the 10 relative traits (RSL, RSFW, RRFW, RSDW, RRDW, RRL, RRAD, RRSA, RRV, and RRTN) for the 200 maize inbred lines. GWAS of the SNP markers and related traits was performed using TASSEL 5.0 software, using a mixed linear model (MLM) combined with population structure and kinship. From this analysis, nine SNPs were shown to be significantly associated with four traits (the p values ranged from 8.1081E-06 to 9.6125E-05; Figure 3).
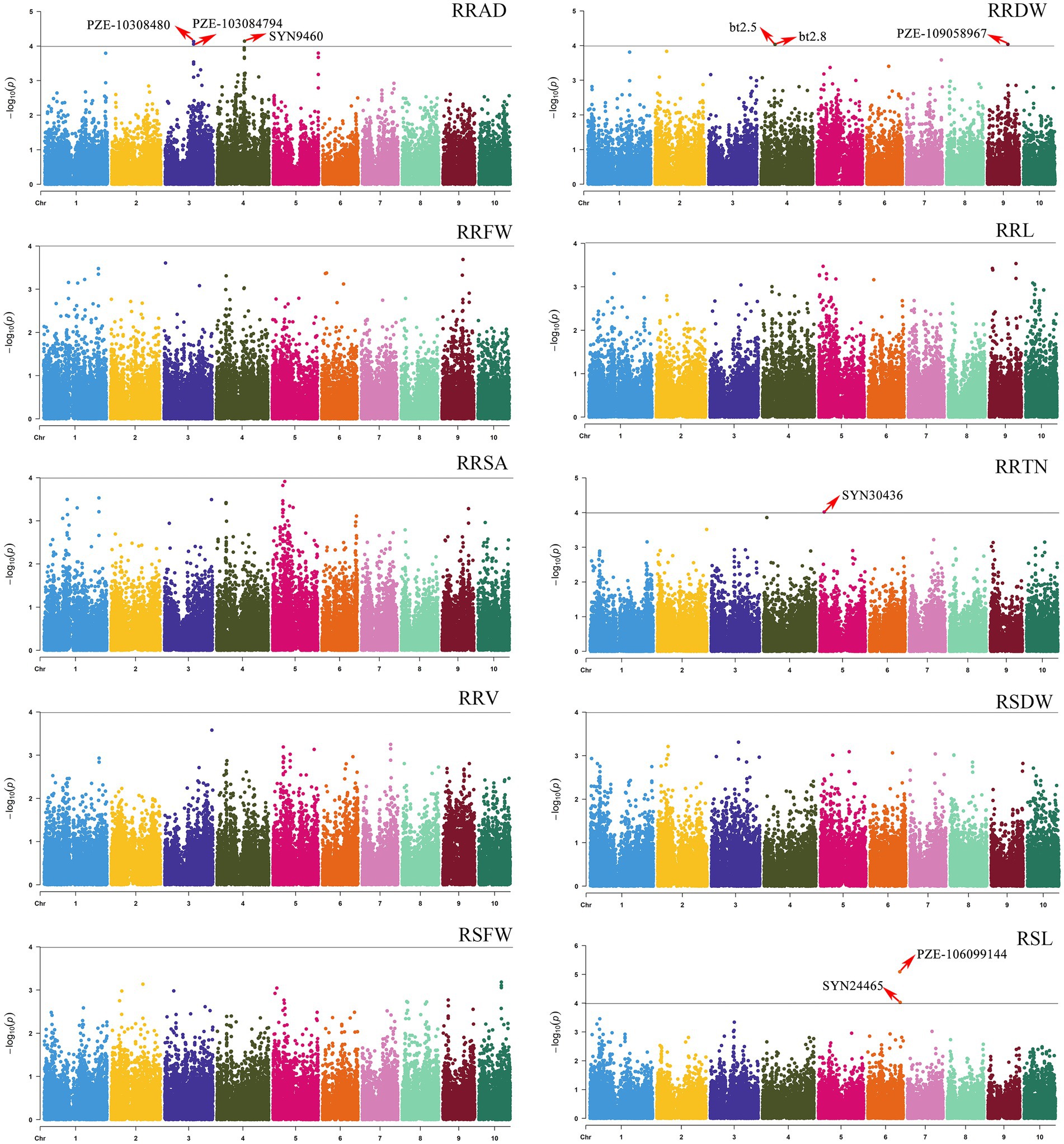
Figure 3. Manhattan plots of GWAS results showing the significant SNPs associated with 10 relative phenotypic traits. The traits included the following: the relative root average diameter (RRAD), relative root fresh weight (RRFW), relative root surface area (RRSA), relative root volume (RRV), relative shoot fresh weight (RSFW), relative root dry weight (RRDW), relative root length (RRL), relative root tip number (RRTN), relative shoot dry weight (RSDW), and relative seeding length (RSL).
Among the nine associated SNPs, the distance between bt2.5 and bt2.8 on chromosome 4 was only 706 bp (for RRDW), and the distance between PZE-103084802 and PZE-103084794 on chromosome 3 was only 42 kb (for RRAD). These SNPs were located on maize chromosomes 3, 4, 5, 6, and 9, with the highest number of SNPs (3) on chromosome 4. A single SNP associated with RRT was discovered. Two SNPs were detected for RSL, and the most significantly associated SNP was for PZE-106099144 (p = 8.1081E-06). The three SNPs were significantly associated with RRAD and were located on chromosomes 4 (SYN9460, p = 7.21E-05), 3 (PZE-103084802, p = 7.45E-05), and 3 (PZE-103084794, p = 8.83E-05). The three SNPs were significantly associated with RRDW and were located on chromosomes 9 (PZE-109058967, p = 9.18E-05), 4 (bt2.5, p = 9.28E-05), and 4 (bt2.8, p = 9.28E-05). Four relative traits containing 9 SNPs explained 7.94%–10.71% of the total phenotypic variation (Table 2).
Among the nine SNPs found for the four traits, nine candidate genes that were directly closest to the physical location of the SNPs were mined using the B73 RefGen_v4 Maize Gene Database (Table 3). Two genes were found to be associated with RSL (Zm00001d038265, and Zm00001d038320). Three genes were found to be associated with RRAD (Zm00001d050905, Zm00001d041766, and Zm00001d041767). Three genes were found to be associated with RRDW (Zm00001d046591, Zm00001d050109, and Zm00001d050110). For RRTN, only one candidate gene was associated (Zm00001d013802). Furthermore, when the LD decay distance was changed to r2 = 0.2, an additional 200 candidate genes were added to the 9 associated SNPs (Supplementary Table S5). They were distributed on chromosomes 1–10: the PZE-106099144 (SL) locus contained 45 genes, the SYN24465 (SL) locus contained 42 genes, the SYN9460 (RAD) locus contained 10 genes, the PZE-103084802 (RAD) locus contained 17 genes, the PZE-103084794 (RAD) locus contained 19 genes, the PZE-109058967 (RDW) locus contained 36 genes, the bt2.5 (RDW) locus contained 9 genes, the bt2.8 (RDW) locus contained 9 genes, and the SYN30436 (RRTN) locus contained 39 genes.
RNA-seq analysis of leaves transcripts in response to alkali stress
To help identify candidate genes, the maize inbred line K10 was selected as an alkali stress tolerant example and its genome-wide gene expression levels were analyzed using RNA-seq. For downregulated DEGs, two were identified in the K4D_ATvsK4D_CK comparison group and 141 DEGs were identified in the K3D_ATvsK3D_CK comparison group. For the upregulated DEGS (|log2FC| ≥ 0.585, p < 0.05), 17 were identified in the K4D_ATvsK4D_CK comparison group, 414 were identified in the K3D_ATvsK3D_CK comparison group, and four were identified in the K4D_ATvsK4D_CK and K3D_ATvsK3D_CK comparison groups (Figure 4).
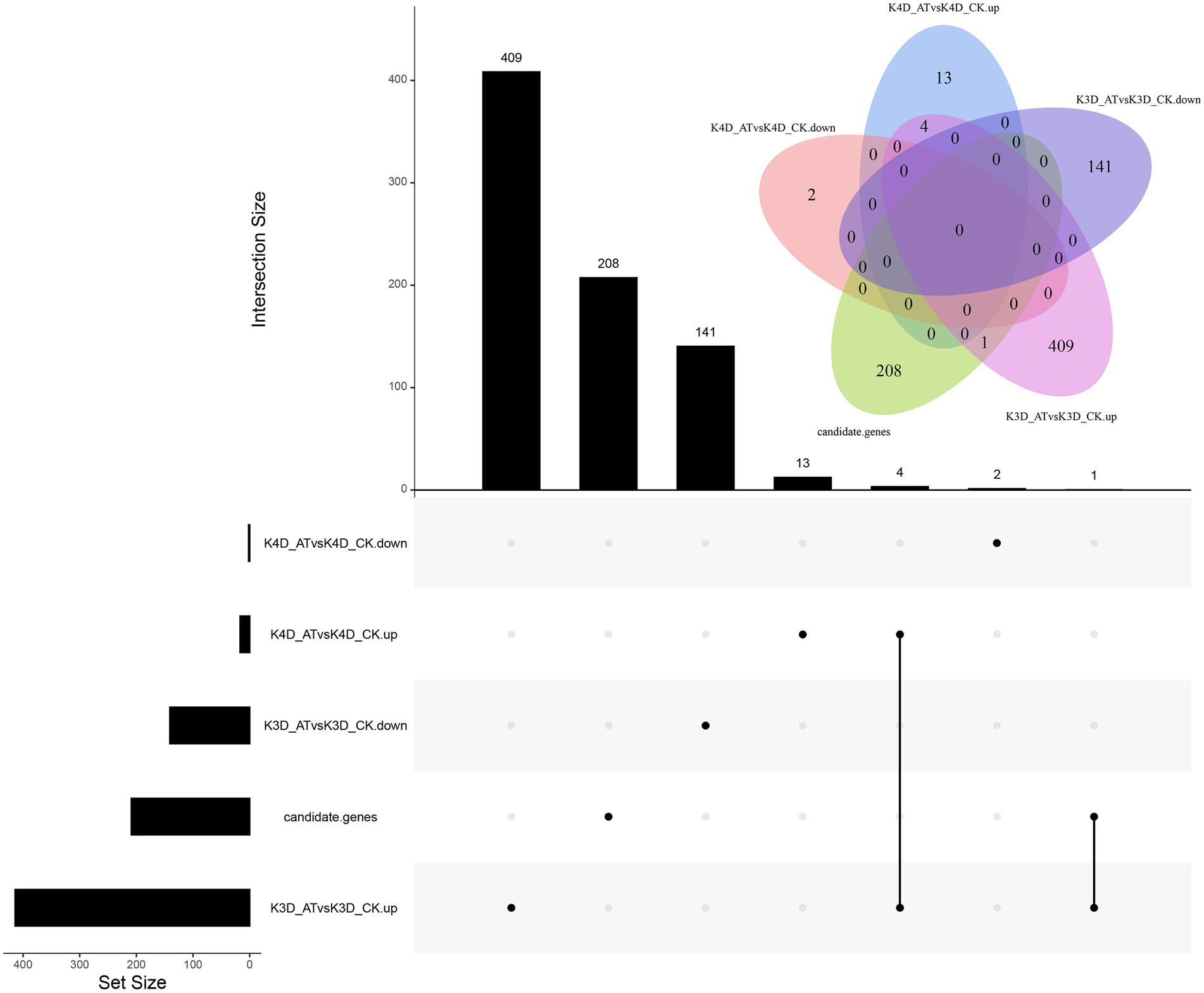
Figure 4. Venn diagram of DEGs distributed in maize inbred lines K10. The treatments included the normal control treatment for 3 and 4 days (CK), and the alkaline treatment for 3 and 4 days (AT). The alkaline treatment for 3 and 4 days are labeled “K3D_AT”and “K4D_AT,” respectively. The five treatment-line comparisons groups included the following: alkaline treatment for 3 days vs. normal control treatment for 3 days, upregulated expression (K3D_ATvsK3D_CK.up); alkaline treatment for 3 days vs. normal control treatment for 3 days, downregulated expression (K3D_ATvsK3D_CK.down); alkaline treatment for 4 days vs. normal control treatment for 4 days, upregulated expression (K4D_ATvsK4D_CK.up); alkaline treatment for 4 days vs. normal control treatment for 4 days, downregulated expression (K4D_ATvsK4D_CK.down); and candidate genes.
A total of 209 candidate genes were associated with SNPs, and one important DEG was identified, including one candidate gene (Zm00001d038250) that was only upregulated in the K3D_ATvsK3D_CK comparison group. Four DEGs were upregulated in the K3D_ATvsK3D_CK and K4D_ATvsK4D_CK comparison group, including four candidate genes (Zm00001d027619, Zm00001d032973, Zm00001d001820, and Zm00001d001960). Putative protein functions are listed in Table 4, determined by NCBI. Zm00001d038250 encoded a HSP40/DNAJ peptide-binding protein, Zm00001d027619 encoded a beta-amylase, Zm00001d032973 encoded a glycerol-3-phosphate acyltransferase7, Zm00001d001820 encoded a protochlorophyllide reductase 1, and Zm00001d001960 encoded a flavanone 3-dioxygenase 1.
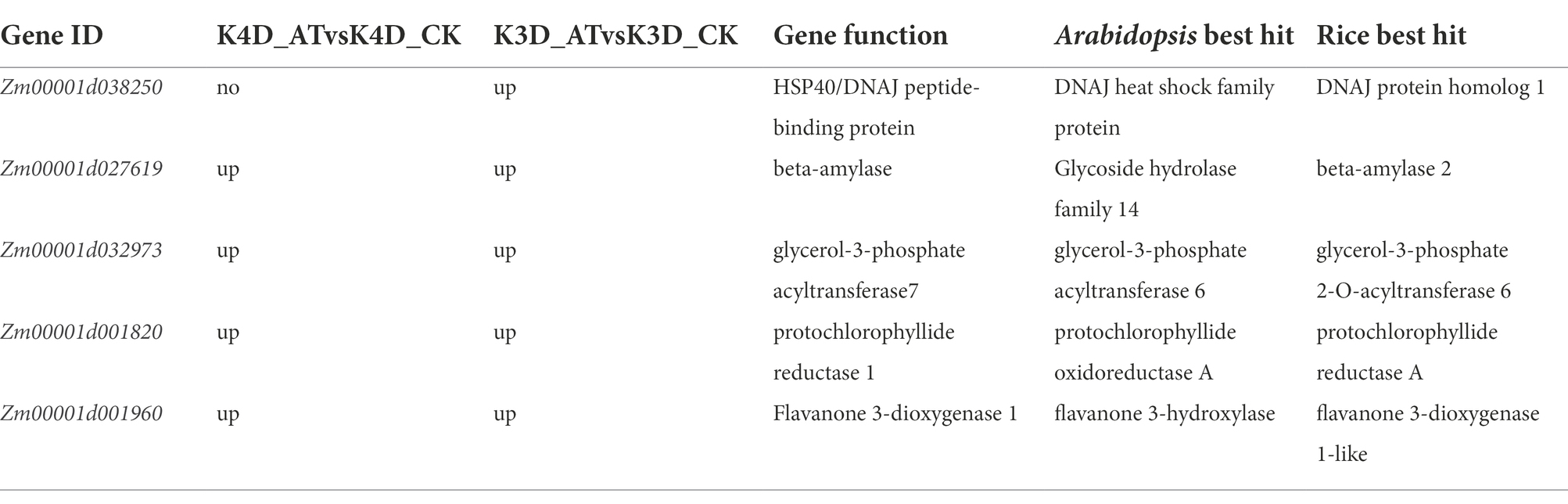
Table 4. Candidate DEGs that are consistent between the K4D_ATvsK4D_CK and K3D_ATvsK3D_CK comparison groups.
Functional prediction of candidate genes by GWAS and RNA-seq analysis
There were a total of 37 GO terms associated with the five candidate genes, three candidate genes were associated with four KEGG pathways, and one KEGG pathway (zma01110) was co-enriched by the three genes (Tables 5, 6). These GO terms were functionally related to three broad categories. The first function described was cellular components, including cytoplasmic part (Zm00001d001820 and Zm00001d038250), cytoplasm (Zm00001d001820 and Zm00001d038250), cell part (Zm00001d038250 and Zm00001d001820), cell (Zm00001d001820 and Zm00001d038250), cytosol (Zm00001d038250), intracellular part (Zm00001d038250 and Zm00001d001820), intracellular (Zm00001d038250), membrane (Zm00001d032973 and Zm00001d001820), plastid (Zm00001d001820), membrane-bounded organelle (Zm00001d001820), intracellular membrane-bounded organelle (Zm00001d001820), intracellular organelle (Zm00001d001820), and organelle (Zm00001d001820). The second type described was biological processes, which involved cellular process (Zm00001d038250 and Zm00001d032973), metabolic process (Zm00001d027619, Zm00001d032973, Zm00001d001820, and Zm00001d001960), catabolic process (Zm00001d027619), carbohydrate metabolic process (Zm00001d027619), primary metabolic process (Zm00001d027619), organic substance metabolic process (Zm00001d027619), biosynthetic process (Zm00001d032973, Zm00001d001820 and Zm00001d001960), response to abiotic stimulus (Zm00001d001960), response to stimulus (Zm00001d001960), photosynthesis (Zm00001d001820), and cellular metabolic process (Zm00001d001820). The third type of function described was molecular functions, which involved binding (Zm00001d001960 and Zm00001d038250), protein binding (Zm00001d038250), catalytic activity (Zm00001d027619, Zm00001d032973, Zm00001d001820, and Zm00001d001960), hydrolase activity (Zm00001d027619 and Zm00001d032973), and transferase activity (Zm00001d032973; Table 5; Figures 5A,B). Two major classes of KEGG pathways were identified: the flavonoid synthesis pathway and the metabolic pathway, including starch and sucrose metabolism, and porphyrin and chlorophyll metabolism (Table 6; Figure 5C).
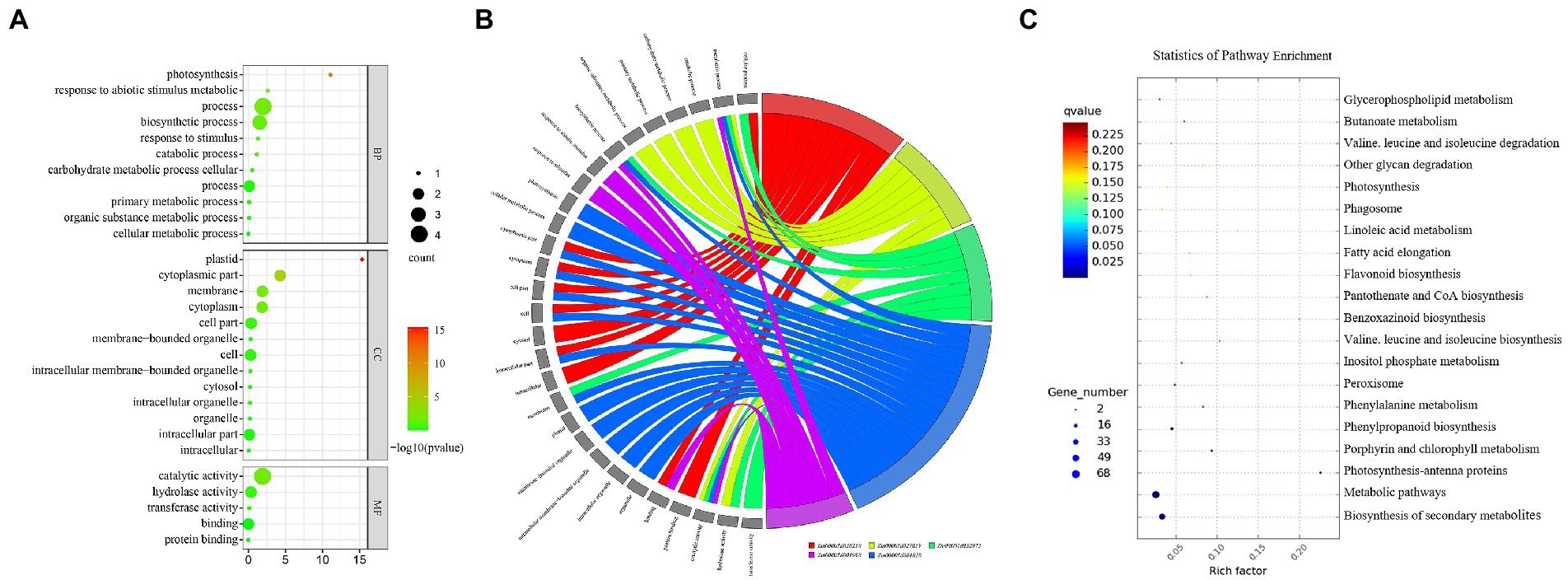
Figure 5. GO annotations and KEGG pathways of DEGs. (A) Bubble chart of GO classifications of five DEGs. (B) Chord chart of GO annotation corresponding to five DEGs. (C) The red box is the KEGG pathway map of Zm00001d02761, Zm00001d001820, and Zm00001d001960 genes.
qRT-PCR validation for differentially expressed genes
Five candidate genes were selected based on RNA-seq (|log2FC| ≥ 0.585, p < 0.05) for qRT-PCR analysis: Zm00001d038250, Zm00001d027619, Zm00001d032973, Zm00001d001820, and Zm00001d001960. When alkali treated for 3 days, the expression level of one gene (Zm00001d038250) in K10 was significantly higher (1.49) than that in Mo17 (−0.74) (p < 0.01). The expression level of this gene showed a downward trend after 4 days of treatment, but the expression level in B73 (0.410) was still higher than that in Mo17 (−0.237). The expression level of gene Zm00001d027619 in K10 (0.263) was significantly lower than that in Mo17 (2.140) when treated for 3 days, and there was no significant difference when treated for 4 days. The expression levels of the other two genes (Zm00001d032973 and Zm00001d001820,) in K10 (2.617 and 1.787) were significantly higher than those in Mo17 (1.610 and −3.867) when treated with alkali for 3 days but showed the opposite pattern when treated for 4 days: the expression level in Mo17 (3.533 and 3.353) was significantly higher than that in K10 (0.233 and 0.337). The expression level of the last gene (Zm00001d001960) in K10 (2.933 and 0.960) was significantly higher than that of Mo17 (−0.593 and −1.130) at 3 and 4 days of alkali treatment (Figure 6). The results of RNA-seq and qRT-PCR analysis showed that the expression trends of the five genes were consistent: the expressions were all upregulated after 3 or 4 days of alkaline treatment.
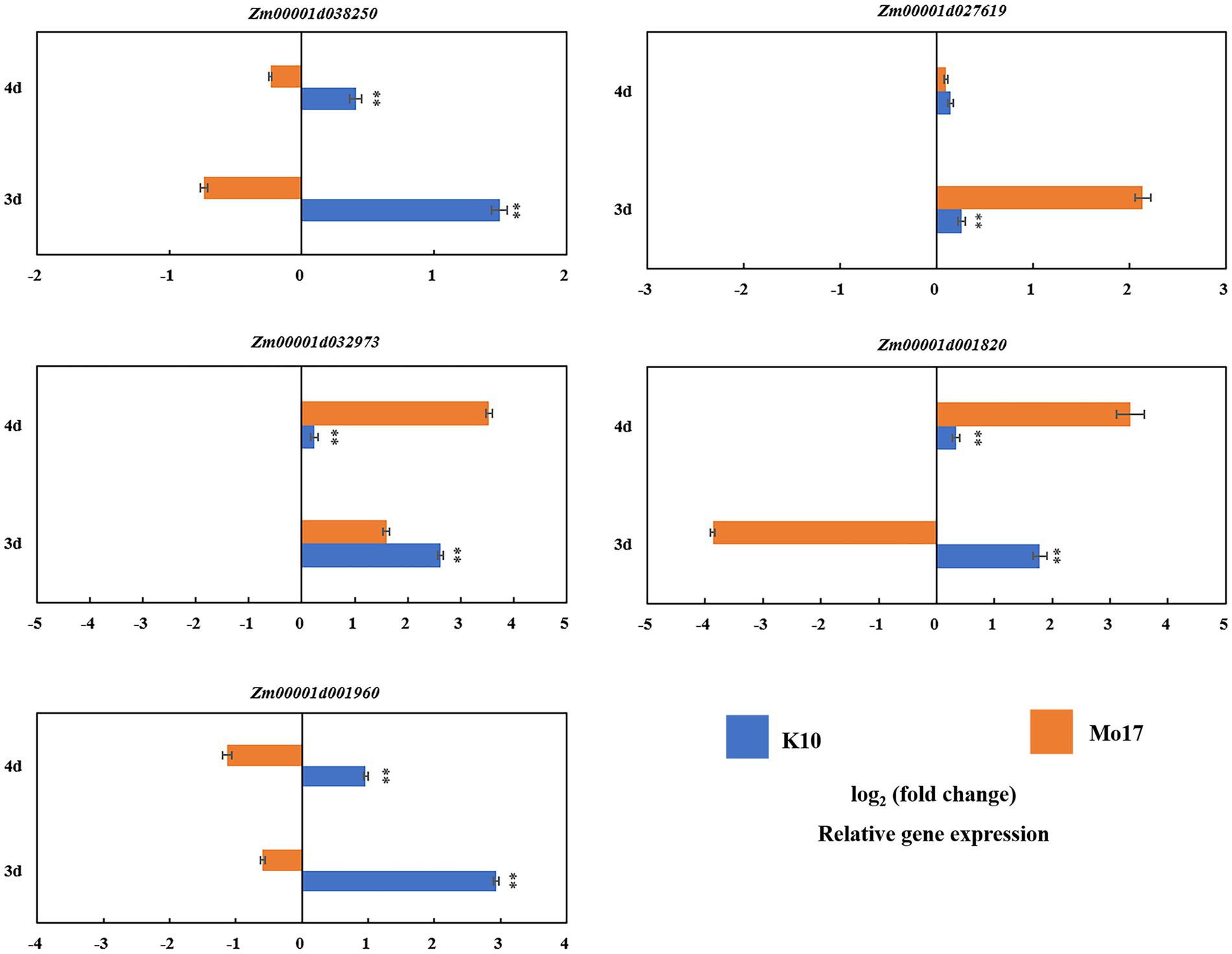
Figure 6. qRT-PCR validation of the GWAS and RNA-seq results. Expression of five candidate genes in alkaline-tolerant inbred line K10 and the alkaline-sensitive inbred line Mo17. Expression analysis was conducted on leaf that were collected at 3 and 4 days under normal control treatment and alkaline treatment, respectively. ** indicate significance at p < 0.01.
Two candidate genes were shown to be more attractive than the others: Zm00001d038250 and Zm00001d001960, as they were upregulated in K10 and downregulated in Mo17 under both the treatment durations (3 or 4 days). Gene function annotation suggested that they may be involved in alkaline tolerance-related functions, such as heat shock proteins and flavonoid metabolic pathways.
Discussion
Maize is an alkali-sensitive crop that is grown on salt-contaminated soil due to soil salinization (Luo et al., 2021). Soil salinization ultimately affects maize yield, but the effect of salinization at the seedling stage is reflected in traits such as seedling length and root fresh weight. In previous studies, the SL, SFW, RFW, SDW, and RDW were used to assess the salinity tolerance of maize, highlighting that these traits showed a significant positive correlation to salinity tolerance (Cui et al., 2014; Luo et al., 2019, 2021). In addition to using the above traits, traits such as RL and RAD were added in this study. A total of 10 relative traits were determined at the seedling stage for GWAS. Among the 200 maize inbred lines that were studied, a large range of phenotypic variation was observed. Except for RAD, which was significantly negatively correlated with other traits, the remaining traits showed significant positive correlations that were consistent with previous studies. The newly added trait, RL, was also significantly positively correlated with other traits but extremely significantly negatively correlated with RRAD (r2 = −0.49, p < 0.001). The mean RRAD of 200 inbred lines was 1.0847, and the average RRL of 200 inbred lines was 0.6664. This indicated that maize may resist external alkali stress by thickening and shortening its roots.
GWAS is now commonly used to detect complex trait variants and predict candidate genes. However, there are still problems with false positives using this method (Wang et al., 2019). RNA-seq has emerged as an important tool for analyzing genome-wide expression patterns (Tai et al., 2016). Nevertheless, potential candidate genes are difficult to identify from the large number of DEGs obtained by RNA-seq. In recent years, GWAS combined in combination with RNA-seq has been used to detect novel genes associated with complex traits in crops. For example, five candidate genes potentially involved in maize root development were predicted using GWAS in combination with RNA-seq. Furthermore, ten candidate genes related to low-temperature tolerance were also identified (Wang et al., 2019; Zhang et al., 2020). This study identified five candidate genes (Zm00001d038250, Zm00001d027619, Zm00001d032973, Zm00001d001820, and Zm00001d001960) associated with alkali tolerance through this method. Among the identified genes, Zm00001d038250 and Zm00001d001960 had significant differences in expression between the sensitive and tolerant maize lines, may provide valuable genetic information on the mechanisms of alkali tolerance in maize at the seedling stage.
To assess the reliability of the SNPs in this study, the nine SNPs obtained from this research were compared with previously identified and published QTLs and SNPs. Three of the loci overlapped with the physical locations of previously published QTLs. SYN30436 on chromosome 5 was located in proline-associated qPC-5-1 under alkaline treatment (Zhang et al., 2018). Two SNPs located on chromosome 3 were located on QTLs associated with shoot dry weight (Luo et al., 2019). The parallel between the examples that have been previously described and the examples identified in this study proved that the SNPs of the alkali tolerance-related traits in this study were reliable for maize breeding. The contribution rate of SNPs in this study was 8.4%–10.7%, which may be because root-related traits are controlled by many small-effect QTLs (Burton et al., 2014; Li et al., 2015).
Zm00001d038250 encoded the DNAJ protein, also known as HSP40, which is a member of the conserved co-chaperone protein family. It is homologous to rice LOC_ OS05G0427900 and Arabidopsis AT1G44160. As a co-chaperone of HSP70, the DNAJ protein can promote the ATPase activity of HSP70 under stress conditions and participate in the maintenance of intracellular protein folding, complex depolymerization, and other vital activities (Rajan and D’Silva, 2009; Chen et al., 2014). It was found that Zm00001d027619 in maize encoded β-amylase protein and contains an AmyAc conserved domain. However, little research has been presented on β-amylase and its response to abiotic stress using unstructured carbon composed of soluble sugars and starches (Dickman et al., 2019; Zhen et al., 2020).
Next, Zm00001d032973 encoded a glycerol-3-phosphate acyltransferase protein (GPAT). Three types of GPATs have been found in plant cells, which are located in the plastids (including chloroplasts), endoplasmic reticulum, and mitochondria (Murata and Tasaka, 1997; Zheng et al., 2003; Gidda et al., 2009). Among these, GPATs in plastids/chloroplasts are soluble proteins, which affect plants’ cold tolerance by affecting the saturation of chloroplast membrane lipid molecules (Murata and Tasaka, 1997; Yokoi et al., 1998; Zhu et al., 2009; Sui et al., 2017). GPATs distributed in the endoplasmic reticulum and mitochondria are membrane-bound proteins, which participate in the biosynthesis of triacylglycerol (TAGs) in seeds, thereby affecting the accumulation of seed oil (Zheng et al., 2003). Another gene, Zm00001d001820, encoded a protochlorophyllide reductase1 protein (pcr1). A homologous gene in Arabidopsis thaliana, AT5G54190, was shown to be involved in NYEs/SGRs-mediated chlorophyll degradation for detoxification during seed maturation (Li et al., 2017b). The last gene identified in this study, Zm00001d001960, encoded flavanone 3-hydroxylase 1 protein (fht1). It is homologous to rice LOC_ OS04G0662600 and Arabidopsis AT3G51240. In general, flavanone 3-hydroxylase 1 improved stress tolerance by upregulating its expression under water-related stress and increasing the content of flavonoids (Klimek-Chodacka et al., 2016; Hodaei et al., 2018).
The two candidate genes (Zm00001d038250 and Zm00001d001960) are thought to function in alkali tolerance, similar to the HSP40 protein and flavanone 3-hydroxylase 1 activity. Zm00001d038250 encoded the HSP40 protein (sHSP), a heat shock protein (HSP), which is a special protein produced by the body in response to stress. Its structure is highly conserved, and it mainly acts as a molecular chaperone to play biological functions such as folding and transportation (Voellmy and Boellmann, 2007). Maize mitochondrial sHsps improved mitochondrial electron transport during salt stress, mainly by protecting NADH: ubiquinone oxidoreductase activity (Hamilton and Heckathorn, 2001). Overexpression of HSP40 in Arabidopsis improved salt tolerance by increasing root length under 120 mM NaCl treatment (Zhao et al., 2010). The protein BIL2 in the Arabidopsis HSP40 family induced cell elongation during BR signaling by promoting ATP synthesis in mitochondria, thereby increasing inflorescence root length in response to salt and light stress (Bekh-Ochir et al., 2013). Zm00001d038250 and ZmPWZ18936.1 proteins (NCBI accession number: PWZ18936.1) are evolutionarily on the same branch, and may have some similarities in function. The Zm00001d038250 gene promoter region contained not only CAAT-box core elements but also hormone-, light-, defense-, metabolism-, and stress-related elements (Figures 7A,C; Supplementary Table S6).
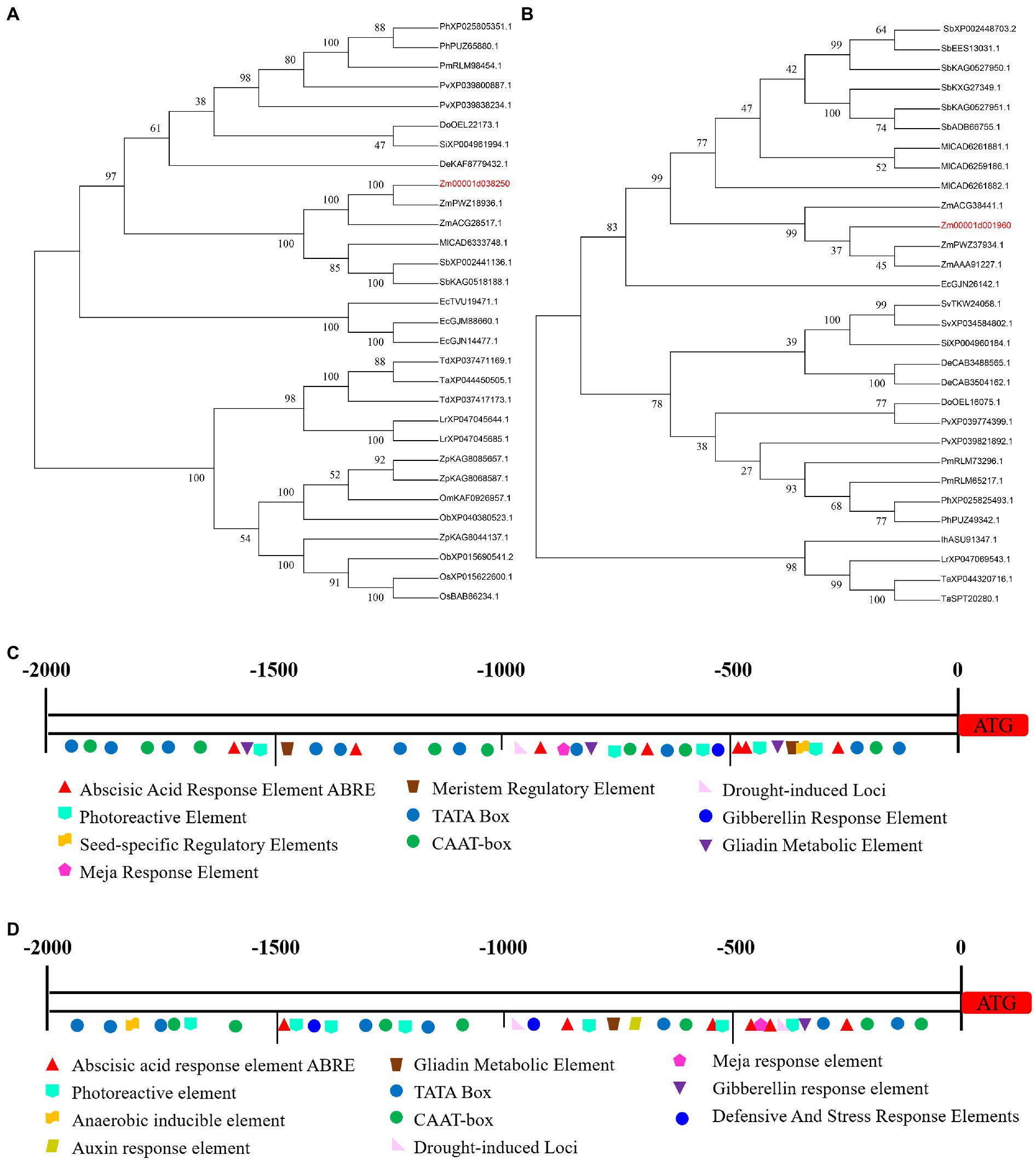
Figure 7. The characterization of candidate genes. (A) Evolutionary tree of Zm00001d038250 protein. (B) Evolutionary tree of Zm00001d001960 protein. (C) The promoter region of Zm00001d038250 gene. (D) The promoter region of Zm00001d001960 gene.
Zm00001d001960 putatively encoded flavanone 3-hydroxylase 1(F3H). Flavonoids are a group of substances with various phenolic structures, which participate in anti-oxidative stress processes, and also participate in some biotic and abiotic stress response processes (Winket-Shirley, 2002; Panche et al., 2016). Researchers have found that recombinant or overexpression of F3H in different crops could modulate naringenin to alleviate growth inhibition and enhance tolerance to salt stress (Li et al., 2017a; Si et al., 2022). Through proteomic analysis of beet seedlings treated with 50 mM NaCl, 135 differentially expressed proteins, including F3H, were mined (Wu et al., 2018). In addition, it was found that under the combined salt and heat stress in rice, overexpression of F3H increased the kaempferol and quercetin content, and then scavenged reactive oxygen species. The content of heat stress transcription factors (HSFs) and heat shock proteins (HSPs) also increased significantly to improve tolerance (Jan et al., 2021). Zm00001d001960 is involved in metabolic pathway and flavonoid biosynthesis pathway, which may regulate alkali tolerance through this pathway. The Zm00001d001960 protein is evolutionarily in the same large clade with the ZmPWZ37934.1 (NCBI accession number: PWZ37934.1) protein and the ZmAAA91227.1 (NCBI accession number: AAA91227.1) protein, but its credibility is low, it may be that there is only some similarity in their structure. The Zm00001d001960 gene promoter region contained not only CAAT-box core elements but also hormone-, light-, and metabolism-related elements. (Figures 7B,D; Supplementary Table S7).
In conclusion, the Zm00001d038250 and Zm00001d001960 genes were significantly and differentially expressed in tolerant materials under alkaline conditions based on functional analysis and GO enrichment analysis. It was hypothesized that the HSP40 protein encoded by Zm00001d038250 could limit the ion transport mode, and the F3H protein encoded by Zm00001d001960 could improve the antioxidant capacity by metabolizing flavonoids, which, in turn, could affect the alkali tolerance of maize seedlings, though this requires further research. Therefore, these genes are attractive genes for the future study of alkali tolerance in maize seedling stage.
Conclusion
In a population composed of 200 maize inbred lines, we detected 10 alkali tolerance-related traits at the seedling stage and found nine SNPs through GWAS. These SNPs were all related to alkali tolerance during the seedling stage. Through RNA-seq analysis and qPRT-PCR verification, we found that two candidate genes, Zm00001d038250 and Zm00001d001960, were differentially expressed under alkaline treatment. This study provides a genetic basis for molecular-assisted breeding of alkali-tolerant maize seedlings.
Data availability statement
The datasets presented in this study can be found in online repositories. The names of the repository/repositories and accession number(s) can be found at: https://www.ncbi.nlm.nih.gov/, PRJNA846593.
Author contributions
ZW and HD: conception or design of the work. CL, YJ, and LL: performed the experiment. RZ, MC, and YZ: analyzed the data. CL and YJ: wrote the manuscript. All authors contributed to the article and approved the submitted version.
Funding
This research was supported by the National Natural Science Foundation of China (32072128) and Genetically Modified Organisms Breeding Major Projects of China (2016ZX08003003).
Conflict of interest
The authors declare that the research was conducted in the absence of any commercial or financial relationships that could be construed as a potential conflict of interest.
Publisher’s note
All claims expressed in this article are solely those of the authors and do not necessarily represent those of their affiliated organizations, or those of the publisher, the editors and the reviewers. Any product that may be evaluated in this article, or claim that may be made by its manufacturer, is not guaranteed or endorsed by the publisher.
Acknowledgments
We would like to thank Xinhai Li and Jianfeng Weng for providing the plant materials.
Supplementary materials
The Supplementary Materials for this article can be found online at: https://www.frontiersin.org/articles/10.3389/fpls.2022.963874/full#supplementary-material
Footnotes
1. ^https://www.ibm.com/support/pages/node/230551
3. ^https://www.ncbi.nlm.nih.gov/data-hub/genome/GCA_000005005.6/
4. ^http://www.geneontology.org/
5. ^https://phytozome-next.jgi.doe.gov/
6. ^http://www.genome.ad.jp/kegg/kegg2.html
References
Abdel Latef, A. A., and Tran, L. S. (2016). Impacts of priming with silicon on the growth and tolerance of maize plants to alkaline stress. Front. Plant Sci. 7:243. doi: 10.3389/fpls.2016.00243
Abreu, I. A., Farinha, A. P., Negrao, S., Goncalves, N., Fonseca, C., Rodrigues, M., et al. (2013). Coping with abiotic stress: proteome changes for crop improvement. J. Proteomics 93, 145–168. doi: 10.1016/j.jprot.2013.07.014
Ambede, J. G., Netondo, G. W., Mwai, G. N., and Musyimi, M. (2012). NaCl salinity affects germination, growth, physiology, and biochemistry of bambara groundnut. Brazilian J. Plant Physiol. 24, 151–160. doi: 10.1590/S1677-04202012000300002
Bekh-Ochir, D., Shimada, S., Yamagami, A., Kanda, S., Ogawa, K., Nakazawa, M., et al. (2013). A novel mitochondrial DnaJ/Hsp40 family protein BIL2 promotes plant growth and resistance against environmental stress in brassinosteroid signaling. Planta 237, 1509–1525. doi: 10.1007/s00425-013-1859-3
Bradbury, P. J., Zhang, Z., Kroon, D. E., Casstevens, T. M., Ramdoss, Y., and Buckler, E. S. (2007). TASSEL: software for association mapping of complex traits in diverse samples. Bioinformatics 23, 2633–2635. doi: 10.1093/bioinformatics/btm308
Burton, A. L., Johnson, J. M., Foerster, J. M., Hirsch, C. N., Buell, C. R., Hanlon, M. T., et al. (2014). QTL mapping and phenotypic variation for root architectural traits in maize (Zea mays L.). Theor. Appl. Genet. 127, 2293–2311. doi: 10.1007/s00122-014-2353-4
Campbell, S. A., and Nishio, J. N. (2000). Iron deficiency studies of sugar beet using an improved sodium bicarbonate-buffered hydroponic growth system. J. Plant Nutr. 23, 741–757. doi: 10.1080/01904160009382056
Cao, Y. B., Zhang, M., Liang, X. Y., Li, F. R., Shi, Y. L., Yang, X. H., et al. (2020). Natural variation of an EF-hand Ca2+-binding-protein coding gene confers saline-alkaline tolerance in maize. Nat. Commun. 11, 186–200. doi: 10.1038/s41467-019-14027-y
Chaparzadeh, N., D’Amico, M. L., Khavari-Nejad, R. A., Izzo, R., and Navari-Izzo, F. (2004). Antioxidative responses of Calendula officinalis under salinity conditions. Plant Physiol. Biochem. 42, 695–701. doi: 10.1016/j.plaphy.2004.07.001
Chen, D. H., Huang, Y., Liu, C. L., Ruan, Y., and Shen, W. H. (2014). Functional conservation and divergence of J-domain-containing ZUO1/ZRF orthologs throughout evolution. Planta 239, 1159–1173. doi: 10.1007/s00425-014-2058-6
Chen, S. S., Xing, J. J., and Lan, H. Y. (2012). Comparative effects of neutral salt and alkaline salt stress on seed germination, early seedling growth and physiological response of a halophyte species Chenopodium glaucum. Afr. J. Biotechnol. 11, 9572–9581. doi: 10.5897/ajb12.320
Cui, D. Z., Wu, D. D., Somarathna, Y., Xu, C. Y., Li, S., Li, P., et al. (2014). QTL mapping for salt tolerance based on snp markers at the seedling stage in maize (Zea mays L.). Euphytica 203, 273–283. doi: 10.1007/s10681-014-1250-x
Dickman, L. T., McDowell, N. G., Grossiord, C., Collins, A. D., Wolfe, B. T., Detto, M., et al. (2019). Homoeostatic maintenance of nonstructural carbohydrates during the 2015-2016 El Nino drought across a tropical forest precipitation gradient. Plant Cell Environ. 42, 1705–1714. doi: 10.1111/pce.13501
Dikobe, T. B., Mashile, B., Sinthumule, R. R., and Ruzvidzo, O. (2021). Distinct Morpho-physiological responses of maize to salinity stress. Am. J. Plant Sci. 12, 946–959. doi: 10.4236/ajps.2021.126064
Du, Z., Zhou, X., Ling, Y., Zhang, Z. H., and Su, Z. (2010). agriGO: a GO analysis toolkit for the agricultural community. Nucleic Acids Res. 38, W64–W70. doi: 10.1093/nar/gkq310
Earl, D. A., and vonHoldt, B. M. (2011). STRUCTURE HARVESTER: a website and program for visualizing STRUCTURE output and implementing the Evanno method. Conserv. Genet. Resour. 4, 359–361. doi: 10.1007/s12686-011-9548-7
Evanno, G., Regnaut, S., and Goudet, J. (2005). Detecting the number of clusters of individuals using the software STRUCTURE: a simulation study. Mol. Ecol. 14, 2611–2620. doi: 10.1111/j.1365-294X.2005.02553.x
Feng, G. X., Zhang, Z. Y., Wan, C. Y., Lu, P. R., and Bakour, A. (2017). Effects of saline water irrigation on soil salinity and yield of summer maize (Zea mays L.) in subsurface drainage system. Agric. Water Manag 193, 205–213. doi: 10.1016/j.agwat.2017.07.026
Gao, Z. W., Han, J. Y., Mu, C. S., Lin, J. X., Li, X. Y., Lin, L. D., et al. (2014). Effects of saline and alkaline stresses on growth and physiological changes in oat (Avena sativa L.) seedlings. Notulae Botanicae Horti Agrobotanici Cluj-Napoca. 42, 357–362. doi: 10.15835/nbha4229441
Geng, G., Li, R. R., Stevanato, P., Lv, C. H., Lu, Z. Y., Yu, L. H., et al. (2020). Physiological and transcriptome analysis of sugar beet reveals different mechanisms of response to neutral salt and alkaline salt stresses. Front. Plant Sci. 11:571864. doi: 10.3389/fpls.2020.571864
Gidda, S. K., Shockey, J. M., Rothstein, S. J., Dyer, J. M., and Mullen, R. T. (2009). Arabidopsis thaliana GPAT8 and GPAT9 are localized to the ER and possess distinct ER retrieval signals: functional divergence of the dilysine ER retrieval motif in plant cells. Plant Physiol. Biochem. 47, 867–879. doi: 10.1016/j.plaphy.2009.05.008
Guo, J., Li, C. H., Zhang, X. Q., Li, Y. X., Zhang, D. F., Shi, Y. S., et al. (2020). Transcriptome and GWAS analyses reveal candidate gene for seminal root length of maize seedlings under drought stress. Plant Sci. 292:110380. doi: 10.1016/j.plantsci.2019.110380
Hamilton, E. W., and Heckathorn, S. A. (2001). Mitochondrial adaptations to NaCl. Complex I is protected by anti-oxidants and small heat shock proteins, Whereas complex II is protected by Proline and Betaine. Plant Physiol. 126, 1266–1274. doi: 10.1104/pp.126.3.1266
Hartung, W., Leport, L., Ratcliffe, R. G., Sauter, A., Duda, R., and Turner, N. C. (2002). Abscisic acid concentration, root pH and anatomy do not explain growth differences of chickpea (Cicer arietinum L.) and lupin (Lupinusangustifolius L.) on acid and alkaline soils. Plant and Soil 240, 191–199. doi: 10.1023/A:1015831610452
Hodaei, M., Rahimmalek, M., Arzani, A., and Talebi, M. (2018). The effect of water stress on phytochemical accumulation, bioactive compounds and expression of key genes involved in flavonoid biosynthesis in Chrysanthemum morifolium L. Ind. Crop Prod. 120, 295–304. doi: 10.1016/j.indcrop.2018.04.073
Jan, R., Kim, N., Lee, S. H., Khan, M. A., Asaf, S., Asif, S., et al. (2021). Enhanced flavonoid accumulation reduces combined salt and heat stress Through regulation of transcriptional and hormonal mechanisms. Front. Plant Sci. 12:796956. doi: 10.3389/fpls.2021.796956
Jia, T. J., Wang, L. F., Li, J. J., Ma, J., Cao, Y. Y., Lübberstedt, T., et al. (2020). Integrating a genome-wide association study with transcriptomic analysis to detect genes controlling grain drying rate in maize (Zea may, L.). Theor. Appl. Genet. 133, 623–634. doi: 10.1007/s00122-019-03492-0
Kanehisa, M., Araki, M., Goto, S., Hattori, M., Hirakawa, M., Itoh, M., et al. (2008). KEGG for linking genomes to life and the environment. Nucleic Acids Res. 36, D480–D484. doi: 10.1093/nar/gkm882
Klimek-Chodacka, M., Sadłowska, K., Kamińska, I., and Barański, R. (2016). Expression of the flavonoid pathway genes in carrot plants tolerant to salt stress. N. Biotechnol. 33:S166. doi: 10.1016/j.nbt.2016.06.1294
Li, P. C., Chen, F. J., Cai, H. G., Liu, J. C., Pan, Q. C., Liu, Z. G., et al. (2015). A genetic relationship between nitrogen use efficiency and seedling root traits in maize as revealed by QTL analysis. J. Exp. Bot. 66, 3175–3188. doi: 10.1093/jxb/erv127
Li, C. C., Liu, S. H., Yao, X. H., Wang, J., Wang, T. L., Zhang, Z. H., et al. (2017a). PnF3H, a flavanone 3-hydroxylase from the Antarctic moss Pohlia nutans, confers tolerance to salt stress and ABA treatment in transgenic Arabidopsis. Plant Growth Regul. 83, 489–500. doi: 10.1007/s10725-017-0314-z
Li, H., Peng, Z. Y., Yang, X. H., Wang, W. D., Fu, J. J., Wang, J. H., et al. (2013). Genome-wide association study dissects the genetic architecture of oil biosynthesis in maize kernels. Nat. Genet. 45, 43–50. doi: 10.1038/ng.2484
Li, X. H., Wang, G. H., Fu, J. J., Li, L., Jia, G. Y., Ren, L. S., et al. (2018). QTL mapping in three connected populations reveals a set of consensus genomic regions for low temperature germination ability in Zea mays L. Front. Plant Sci. 9:65. doi: 10.3389/fpls.2018.00065
Li, F. J., Wen, W. E., Liu, J. D., Zhang, Y., Cao, S. H., He, Z. H., et al. (2019). Genetic architecture of grain yield in bread wheat based on genome-wide association studies. BMC Plant Biol. 19:168. doi: 10.1186/s12870-019-1781-3
Li, Z. P., Wu, S. X., Chen, J. Y., Wang, X. Y., Gao, J., Ren, G. D., et al. (2017b). NYEs/SGRs-mediated chlorophyll degradation is critical for detoxification during seed maturation in Arabidopsis. Plant J. 92, 650–661. doi: 10.1111/tpj.13710
Li, X. P., Zhou, Z. J., Ding, J. Q., Wu, Y. B., Zhou, B., Wang, R. X., et al. (2016). Combined linkage and association mapping reveals QTL and candidate genes for plant and ear height in maize. Front. Plant Sci. 7:833. doi: 10.3389/fpls.2016.00833
Liang, J. L., Qu, Y. P., Yang, C. G., Ma, X. D., Cao, G. L., Zhao, Z. W., et al. (2014). Identification of QTLs associated with salt or alkaline tolerance at the seedling stage in rice under salt or alkaline stress. Euphytica 201, 441–452. doi: 10.1007/s10681-014-1236-8
Liu, C. L., Hao, Z. F., Zhang, D. G., Xie, C. X., Li, M. S., Zhang, X. C., et al. (2015). Genetic properties of 240 maize inbred lines and identity-by-descent segments revealed by high-density SNP markers. Mol. Breed. 35, 146–158. doi: 10.1007/s11032-015-0344-z
Livak, K. J., and Schmittgen, T. D. (2001). Analysis of relative gene expression data using real-time quantitative PCR and the 2(-Delta Delta C(T)) method. Methods 25, 402–408. doi: 10.1006/meth.2001.1262
Luo, M. J., Zhang, Y. X., Chen, K., Kong, M. S., Song, W., Lu, B. S., et al. (2019). Mapping of quantitative trait loci for seedling salt tolerance in maize. Mol. Breed. 39, 64–76. doi: 10.1007/s11032-019-0974-7
Luo, M. J., Zhang, Y. X., Li, J. N., Zhang, P. P., Chen, K., Song, W., et al. (2021). Molecular dissection of maize seedling salt tolerance using a genome-wide association analysis method. Plant Biotechnol. J. 19, 1937–1951. doi: 10.1111/pbi.13607
Ma, L. L., Qing, C. Y., Frei, U., Shen, Y. O., and Lübberstedt, T. (2020). Association mapping for root system architecture traits under two nitrogen conditions in germplasm enhancement of maize doubled haploid lines. Crop J. 8, 213–226. doi: 10.1016/j.cj.2019.11.004
Miculan, M., Nelissen, H., Ben Hassen, M., Marroni, F., Inzè, D., EnricoPè, M., et al. (2021). A forward genetics approach integrating genome-wide association study and expression quantitative trait locus mapping to dissect leaf development in maize (Zea mays). Plant J. 107, 1056–1071. doi: 10.1111/tpj.15364
Mishra, P., Bhoomika, K., and Dubey, R. S. (2013). Differential responses of antioxidative defense system to prolonged salinity stress in salt-tolerant and salt-sensitive Indica rice (Oryza sativa L.) seedlings. Protoplasma 250, 3–19. doi: 10.1007/s00709-011-0365-3
Murata, N., and Tasaka, Y. (1997). Glycerol-3-phosphate acyltransferase in plants. Biochim. Biophys. Acta 1348, 10–16. doi: 10.1016/s0005-2760(97)00115-x
Panche, A. N., Diwan, A. D., and Chandra, S. R. (2016). Flavonoids: an overview. J. Nutr. Sci. 5:e47. doi: 10.1017/jns.2016.41
Pardo, J. M., and Quintero, F. J. (2002). Plants and sodium ions: keeping company with the enemy. Genome Biol. 3:reviews1017. doi: 10.1186/gb-2002-3-6-reviews1017
Peng, Y. L., Gao, Z. W., Gao, Y., Liu, G. F., Sheng, L. X., and Wang, D. L. (2008). Eco-physiological characteristics of alfalfa seedlings in response to various mixed salt-alkaline stresses. J. Integr. Plant Biol. 50, 29–39. doi: 10.1111/j.1744-7909.2007.00607.x
Rajan, V. B., and D’Silva, P. (2009). Arabidopsis thaliana J-class heat shock proteins: cellular stress sensors. Funct. Integr. Genomics 9, 433–446. doi: 10.1007/s10142-009-0132-0
Ruanjaichon, V., Khammona, K., Thunnom, B., Suriharn, K., Kerdsri, C., Aesomnuk, W., et al. (2021). Identification of gene associated with sweetness in corn (Zea mays L.) by genome-wide association study (GWAS) and development of a functional SNP marker for predicting sweet corn. Plants. 10, 1239–1250. doi: 10.3390/plants10061239
Sajjad, M., Ma, X. L., Habibullah Khan, S., Shoaib, M., Song, Y. H., Yang, W. L., et al. (2017). TaFlo2-A1, an ortholog of rice Flo2, is associated with thousand grain weight in bread wheat (Triticum aestivum L.). BMC Plant Biol. 17:164. doi: 10.1186/s12870-017-1114-3
Schachtman, D., and Liu, W. (1999). Molecular pieces to the puzzle of the interaction between potassium and sodium uptake in plants. Trends Plant Sci. 4, 281–287. doi: 10.1016/S1360-1385(99)01428-4
Shi, D. C., and Sheng, Y. M. (2005). Effect of various salt–alkaline mixed stress conditions on sunflower seedlings and analysis of their stress factors. Environ. Exp. Bot. 54, 8–21. doi: 10.1016/j.envexpbot.2004.05.003
Si, C., Dong, W., da Silva, J. A. T., He, C. M., Yu, Z. M., Zhang, M. Z., et al. (2022). Functional analysis of Flavanone 3-hydroxylase (F3H) from Dendrobium officinale, which confers abiotic stress tolerance. Hortic. Plant J. doi: 10.1016/j.hpj.2022.03.006 (in press).
Sui, N., Tian, S. S., Wang, W. Q., Wang, M. J., and Fan, H. (2017). Overexpression of Glycerol-3-phosphate Acyltransferase from Suaeda salsa improves salt tolerance in Arabidopsis. Front. Plant Sci. 8:1337. doi: 10.3389/fpls.2017.01337
Sun, J., Xie, D. W., Zhang, E. Y., Zheng, H. L., Wang, J. G., Liu, H. L., et al. (2019). QTL mapping of photosynthetic-related traits in rice under salt and alkali stresses. Euphytica 215, 147–161. doi: 10.1007/s10681-019-2470-x
Tai, H. H., Lu, X., Opitz, N., Marcon, C., Paschold, A., Lithio, A., et al. (2016). Transcriptomic and anatomical complexity of primary, seminal, and crown roots highlight root type-specific functional diversity in maize (Zea mays L.). J. Exp. Bot. 67, 1123–1135. doi: 10.1093/jxb/erv513
Voellmy, R., and Boellmann, F. (2007). Chaperone regulation of the heat shock protein response. Adv. Exp. Med. Biol. 594, 89–99. doi: 10.1007/978-0-387-39975-1_9
Wang, H. M., Wei, J., Li, P. C., Wang, Y. Y., Ge, Z. Z., Qian, J. Y., et al. (2019). Integrating GWAS and gene expression analysis identifies candidate genes for root morphology traits in maize at the seedling stage. Genes 10, 773–789. doi: 10.3390/genes10100773
Weng, J. F., Xie, C. X., Hao, Z. F., Wang, J. J., Liu, C. L., Li, M. S., et al. (2011). Genome-wide association study identifies candidate genes that affect plant height in Chinese elite maize (Zea mays L.) inbred lines. PLoS One 6:e29229. doi: 10.1371/journal.pone.0029229
Winket-Shirley, B. (2002). Biosynthesis of flavonoids and effects of stress. Curr. Opin. Plant Biol. 5, 218–223. doi: 10.1016/S1369-5266(02)00256-X
Wu, G. Q., Wang, J. L., Feng, R. J., Li, S. J., and Wang, C. M. (2018). iTRAQ-based comparative proteomic analysis provides insights into molecular mechanisms of salt tolerance in sugar beet (Beta vulgaris L.). Int. J. Mol. Sci. 19, 3866–3886. doi: 10.3390/ijms19123866
Xu, J. J., Liu, T., Yang, S. C., Jin, X. Q., Qu, F., Huang, N., et al. (2019). Polyamines are involved in GABA-regulated salinity-alkalinity stress tolerance in muskmelon. Environ. Exp. Bot. 164, 181–189. doi: 10.1016/j.envexpbot.2019.05.011
Yan, J. B., Warburton, M., and Crouch, J. (2011). Association mapping for enhancing maize (Zea mays L.) genetic improvement. Crop. Sci. 51, 433–449. doi: 10.2135/cropsci2010.04.0233
Yang, Y. Q., Wu, Y. J., Ma, L., Yang, Z. J., Dong, Q. Y., Li, Q. P., et al. (2019). The Ca2+ sensor SCaBP3/CBL7 modulates plasma membrane H+-ATPase activity and promotes alkali tolerance in Arabidopsis. Plant Cell 31, 1367–1384. doi: 10.1105/tpc.18.00568
Yokoi, S., Higashi, S., Kishitani, S., Murata, N., and Toriyama, K. (1998). Introduction of the cDNA for Arabidopsis glycerol-3-phosphate acyltransferase (GPAT) confers unsaturation of fatty acids and chilling tolerance of photosynthesis on rice. Mol. Breed. 4, 269–275. doi: 10.1023/A:1009671231614
Zhang, C. X., Jin, F. X., Li, S. F., Liu, W. P., Ma, X. J., Yang, S., et al. (2018). Fine mapping of major QTLs for alkaline tolerance at the seedling stage in maize (Zea mays L.) through genetic linkage analysis combined with high-throughput DNA sequencing. Euphytica 214, 120–132. doi: 10.1007/s10681-018-2190-7
Zhang, H., Zhang, J. Y., Xu, Q. Y., Wang, D. D., Di, H., Huang, J., et al. (2020). Identification of candidate tolerance genes to low-temperature during maize germination by GWAS and RNA-seqapproaches. BMC Plant Biol. 20:333. doi: 10.1186/s12870-020-02543-9
Zhang, C. S., Zhou, Z. Q., Yong, H. J., Zhang, X. C., Hao, Z. F., Zhang, F. J., et al. (2017). Analysis of the genetic architecture of maize ear and grain morphological traits by combined linkage and association mapping. Theor. Appl. Genet. 130, 1011–1029. doi: 10.1007/s00122-017-2867-7
Zhao, Z., Zhang, W., Yan, J., Zhang, J., Liu, Z. L. X., and Yi, Y. (2010). Over-expression of Arabidopsis DnaJ (Hsp40) contributes to NaCl-stress tolerance. Afr. J. Biotechnol. 9, 972–978. doi: 10.5897/ajb09.1450
Zhen, F. X., Zhou, J. J., Mahmood, A., Wang, W., Chang, X. N., Liu, B., et al. (2020). Quantifying the effects of short-term heat stress at booting stage on nonstructural carbohydrates remobilization in rice. Crop J. 8, 194–212. doi: 10.1016/j.cj.2019.07.002
Zheng, Z. F., Xia, Q., Dauk, M., Shen, W. Y., Selvaraj, G., and Zou, J. T. (2003). Arabidopsis AtGPAT1, a member of the membrane-bound glycerol-3-phosphate acyltransferase gene family, is essential for tapetum differentiation and male fertility. Plant Cell 15, 1872–1887. doi: 10.1105/tpc.012427
Zhu, J. K. (2016). Abiotic stress signaling and responses in plants. Cell 167, 313–324. doi: 10.1016/j.cell.2016.08.029
Keywords: maize, alkali tolerance, genome-wide association study, RNA-seq, candidate genes
Citation: Li C, Jia Y, Zhou R, Liu L, Cao M, Zhou Y, Wang Z and Di H (2022) GWAS and RNA-seq analysis uncover candidate genes associated with alkaline stress tolerance in maize (Zea mays L.) seedlings. Front. Plant Sci. 13:963874. doi: 10.3389/fpls.2022.963874
Edited by:
Xia Xin, Institute of Crop Sciences (CAAS), ChinaReviewed by:
Zhoufei Wang, South China Agricultural University, ChinaBaoshen Liu, Shandong Agricultural University, China
Copyright © 2022 Li, Jia, Zhou, Liu, Cao, Zhou, Wang and Di. This is an open-access article distributed under the terms of the Creative Commons Attribution License (CC BY). The use, distribution or reproduction in other forums is permitted, provided the original author(s) and the copyright owner(s) are credited and that the original publication in this journal is cited, in accordance with accepted academic practice. No use, distribution or reproduction is permitted which does not comply with these terms.
*Correspondence: Zhenhua Wang, emhlbmh1YXdhbmdfMjAwNkAxNjMuY29t; Hong Di, ZGlob25nZGhAMTYzLmNvbQ==
†These authors have contributed equally to this work