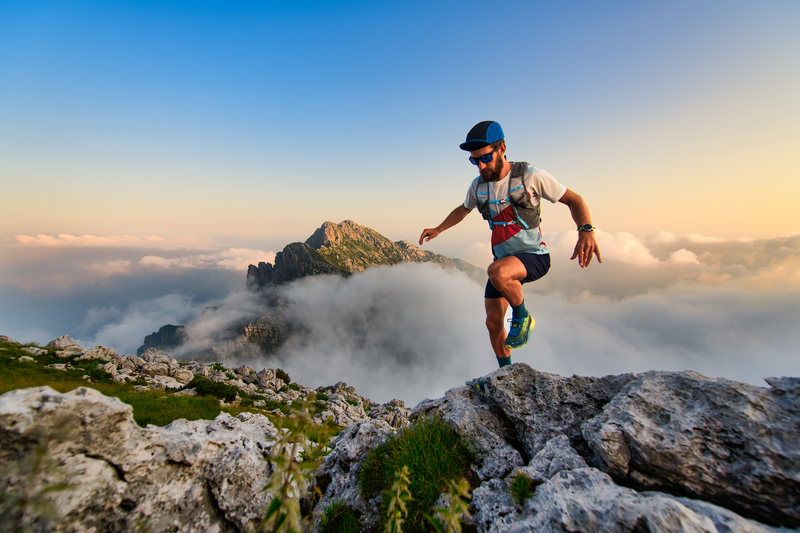
94% of researchers rate our articles as excellent or good
Learn more about the work of our research integrity team to safeguard the quality of each article we publish.
Find out more
ORIGINAL RESEARCH article
Front. Plant Sci. , 22 July 2022
Sec. Plant Bioinformatics
Volume 13 - 2022 | https://doi.org/10.3389/fpls.2022.941231
This article is part of the Research Topic Multi-omics and Computational Biology in Horticultural Plants: From Genotype to Phenotype View all 32 articles
Dendrobium officinale is both a traditional herbal medicine and a plant of high ornamental and medicinal value. Alkaloids, especially terpenoid indole alkaloids (TIAs), with pharmacological activities are present in the tissues of D. officinale. A number of genes involved in alkaloid biosynthetic pathways have been identified. However, the regulatory mechanisms underlying the precursor and methyl jasmonate (MeJA)-induced accumulation of alkaloids in D. officinale are poorly understood. In this study, we collected D. officinale protocorm-like bodies (PLBs) and treated them with TIA precursors (tryptophan and secologanin) and MeJA for 0 (T0), 4 (T4) and 24 h (T24); we also established control samples (C4 and C24). Then, we measured the total alkaloid content of the PLBs and performed transcriptome sequencing using the Illumina HiSeq 2,500 system. The total alkaloid content increased significantly after 4 h of treatment. Go and KEGG analysis suggested that genes from the TIA, isoquinoline alkaloid, tropane alkaloid and jasmonate (JA) biosynthetic pathways were significantly enriched. Weighted gene coexpression network analysis (WGCNA) uncovered brown module related to alkaloid content. Six and seven genes related to alkaloid and JA bisosynthetic pathways, respectively, might encode the key enzymes involved in alkaloid biosynthesis of D. officinale. Moreover, 13 transcription factors (TFs), which mostly belong to AP2/ERF, WRKY, and MYB gene families, were predicted to regulate alkaloid biosynthesis. Our data provide insight for studying the regulatory mechanism underlying TIA precursor and MeJA-induced accumulation of three types of alkaloids in D. officinale.
Dendrobium officinale Kimura et Migo, belonging to the Orchidaceae family, is a rare perennial herb that has antiaging and hypoglycemic properties and is very effective in the treatment of gastrointestinal diseases (Guo et al., 2020). D. officinale is listed separately in the Chinese Pharmacopeia (2010 edition), indicating that it is nationally recognized for its medicinal value (Tang et al., 2017). Several active ingredients, including polysaccharides and alkaloids, reportedly make major contributions to the excellent medicinal effect of D. officinale (Xu et al., 2013). To date, most research has focused on the characterization of polysaccharides, and few studies have examined alkaloids due to their complex chemical compositions and diversity. Some previous studies have shown that the main types of D. officinale alkaloids are pyridines, isoquinolines, purines, amides, and terpenoid indole alkaloids (TIAs) (Mou et al., 2021), with TIAs, such as sempervirine, glycoperine, carapanaubine, and quinine, being the most abundant (Jiao et al., 2018; Cao et al., 2019).
TIAs are a group of natural products with important biological activities. More than 4,000 such alkaloids have been derived from plants in the families Apocynaceae, Rubiaceae, Loganiaceae, and Nyssaceae (Huang et al., 2016). Although the structure of TIAs is complex, they all originate from a common intermediate, strictosidine, which is a product of the condensation of two intermediates, tryptamine and secologanin, catalyzed by the enzyme strictosidine synthase (STR) (Liu et al., 2021). The TIA content in medicinal plants is often low, and the extraction of these compounds is difficult. Moreover, the shortage of natural medicinal plant resources seriously affects the development and utilization of medicinal plants. Adding exogenous substances, such as precursors, elicitors, nutrient elements, and signal molecules, to plant tissue culture systems is an effective method to improve the plant TIA content (Jeet et al., 2020). For example, the accumulation of vinblastine was improved by treatment with tryptophan (Trp) in multiple shoot and callus cultures of Catharanthus roseus (Sharma et al., 2019), and MeJA and JA can be used as signal molecules to regulate the biosynthesis of plant secondary metabolites, such as nicotine, anthocyanin, artemisinin, and TIAs (Wasternack and Strnad, 2019).
In the last few years, transcriptomic technology has become a widely used tool to investigate the biosynthesis and regulatory mechanisms of secondary metabolites. The first transcriptomic analysis paper focused on D. officinale was published in 2013. It revealed several putative alkaloid biosynthetic genes and transcription factor (TF) genes in D. officinale (Guo et al., 2013). To date, a large number of genes involved in alkaloid biosynthesis in D. officinale have been identified by transcriptomic analysis (Shen et al., 2017; Chen et al., 2019; Wang et al., 2020, 2021); however, the regulatory mechanism underlying the accumulation of alkaloids, especially TIAs, in TIA precursor and MeJA-treated D. officinale is largely unknown. In this study, the D. officinale protocorm-like bodies (PLBs) were treated with TIA precursor and MeJA. Then, total alkaloid content of treated and non-treated PLBs was measured, and we used RNA sequencing (RNA-Seq) analysis to study variation in gene expression between the treatment group and control group. Furthermore, WGCNA was used to screen the key modules involved in alkaloid biosynthesis, and the expression of related genes, including key enzymes and TFs, was predicted and analyzed. This work may provide important insights into alkaloids biosynthesis, and reveals the regulation mechanism under precursor and MeJA treatment in D. officinale.
PLBs of D. officinale with basically the same growth trend were used to inoculate 40 mL of 1/2 MS (Murashige and Skoog) + 0.1 mg/L NAA (α-naphthalene acetic acid) + 30 g/L sucrose + 0.1 g/L lactalbumin hydrolysate (pH 5.8) liquid medium in conical bottles, adding 7.0 g of PLBs to each bottle (Figure 1A). Based on our preliminary experimental results, 9 μmol/L Trp, 6 μmol/L secologanin (S) and 100 μmol/L MeJA were added to the liquid medium (Jiao et al., 2018). PLBs without any treatment were used as the control (CK). Samples were collected at 0, 2, 4, 8, 12, and 24 h after treatment (Ge et al., 2015; Chen et al., 2019). Sampling at each time point was performed for three biological repeats. After collection, the PLBs were cleaned under running water and divided into two groups. One group was dried at 60°C for determination of the total alkaloid content. The other group was quickly frozen with liquid nitrogen and stored at −80°C for subsequent RNA extraction and quantitative real-time polymerase chain reaction (qRT-PCR) analysis. The reagents including tryptophan, secologanin and MeJA were all purchased from Sigma-Aldrich (St. Louis, MO, United States). Other reagents, such as MS, NAA, sucrose and lactalbumin hydrolysate, were purchased from Sinopharm Chemical Reagent Co., Ltd. (Shanghai, China).
Figure 1. Determination of total alkaloid content in D. officinale PLBs under Trp + S + MeJA treatment. (A) The PLBs used for Trp + S + MeJA treatment. (B) The total alkaloid content in PLBs treated with TIA precursors and MeJA for 0, 4 and 24 h. Data represent means ± SD from three biological replicates. Asterisks shows significant differences based on the Student’s t-test (*p < 0.05).
For alkaloid extraction, samples were soaked with ammoniated chloroform. The total alkaloid content was determined according to our previously reported method (Jiao et al., 2018).
The total RNA of D. officinale PLBs was extracted according to the manufacturer’s protocol for the Plant Total RNA Isolation Kit (Sangon, Shanghai, China). The concentration and quality of the extracted RNA were tested using Agilent 2100 bioanalyzer with a RNA Nano 6000 Assay Kit (Agilent, CA, United States). Four key enzyme-encoding genes that may be involved in the biosynthesis of TIAs in D. officinale were identified in the National Center for Biotechnology Information (NCBI) database: 1-deoxy-d-xylose 5-phosphate reductase (DoDXS, GenBank accession number: KF803334), strictosidine synthase (DoSTR, GenBank accession number: KX068707), secologanin synthase (DoSLS, GenBank accession number: XM_020846499.2) and tryptophan decarboxylase (DoTDC, GenBank accession number: MK625691.1). To determine their expression levels in PLBs at the six different treatment times mentioned above, we further selected suitable sampling points (0, 4 and 24 h) for transcriptome sequencing analysis. The qRT-PCR primers for DoDXS, DoSTR, DoSLS and DoTDC are listed in Supplementary Table 1.
Transcriptome sequencing, sequence assembly, and data analysis are provided by Biomarker Biotechnology Co., Ltd. (Beijing, China). The mRNA from these samples collected at the three sampling time points was enriched using Oligo (dT) magnetic beads. Then, the mRNA was fragmented and used as a template for reverse transcription to synthesize cDNA. The purified cDNA was then subjected to end repair and A-tailing, followed by the attachment of a sequencing adapter. A cDNA library was then obtained by PCR amplification and enrichment. Then, the sequencing was executed on an Illumina HiSeq 2,500 platform, and 150 bp paired-end reads were produced. The sequences were further processed with a bioinformatic pipeline tool, BMKCloud13 online platform. Raw data of fastq format were firstly processed through in-house Perl scripts. The raw data were filtered to remove the linker sequences and low-quality reads to obtain high-quality clean data. The Q20, Q30, and GC content of the clean data were calculated.
The clean data were mapped to the genome of D. officinale (version number ASM160598v2).1 HISAT2 software was used to align the obtained clean data to the reference genome, and the comparison efficiency was calculated to evaluate the assembly quality of the selected reference genome. StringTie software was used to assemble the aligned reads, and the obtained unigenes were quantitatively analyzed (Pertea et al., 2016).
Gene expression levels were determined using the fragments per kilobase of transcript per million mapped reads (FPKM) method (Florea et al., 2013). Differential expression analysis of two samples was performed using DESeq2 (version 1.24.0) software (Rapaport et al., 2013). The differentially expressed genes (DEGs) were screened with the threshold false discovery rate (FDR) ≤ 0.01 and | log2FC (fold change)| ≥ 1. Heat maps were generated using TBtools software (version 0.66837) to display genes with significantly altered expression. All the assembled unigenes and DEGs were searched against the NCBI non-redundant protein (NR),2 Gene Ontology (GO),3 Clusters of Orthologous Groups (COG),4 Kyoto Encyclopedia of Genes and Genomes (KEGG)5 and Swiss-Prot databases using a cutoff E-value of 10-5 (Lv et al., 2022).
A weighted gene coexpression network was built using the WGCNA R package to identify modules of high correlated genes based on the FPKM data (Langfelder and Horvath, 2008). Before performing WGCNA analysis, selected gene sets were filtered to remove low quality genes according to median absolute deviation (MAD) value. The soft thresholding power β of 14 was selected to make the networks exhibit an approximate scale-free topology (Supplementary Figure 1). The adjacency matrix was then converted to a topological overlap (TO) matrix using the TOMsimilarity algorithm (Zhan et al., 2015). All genes were hierarchically clustered based on TOMsimilarity, and a gene dendrogram was produced. The Dynamic Hybrid Tree Cut algorithm was used to cut the hierarchal clustering tree and defined modules as branches from the tree cutting. It had a module with default settings: minModuleSize was 30 and the minimum height of the combined module was 0.25 (Li et al., 2021). Modules whose eigengenes were highly correlated (correlation > 0.8) were merged. In order to screen the modules related to alkaloid biosynthesis, Pearson’s correlation between the eigengenes of each module and the alkaloid content were further analyzed. The coexpression network illustration was conducted with Cytoscape software (version 3.8.0).
To confirm the reliability of the RNA-Seq data, the expression levels of 16 candidate genes related to alkaloid biosynthesis and metabolic regulation were checked using qRT-PCR. The primers of the 16 selected unigenes are listed in Supplementary Table 1. cDNAs were reverse-transcribed from total RNA using the PrimeScript RT Reagent Kit (Takara, Tokyo, Japan). All reactions were carried out in a QuantStudio 6 Flex real-time PCR system (Thermo Fisher, Waltham, MA, United States). Each reaction contained 2 μL of diluted cDNA, 1 μL of each primer, 8 μL of SYBR Premix Ex Taq II, and 8 μL of RNase-free double-distilled water (ddH2O). The cycling conditions used for qRT-PCR were as follows: 95°C for 3 min, followed by 40 cycles of 95°C for 10 s, 52°C for 15 s and 72°C for 30 s. A housekeeping gene (β-actin) was used as a reference, and the relative expression level of each gene was calculated using the 2–Δ Δ CT method (Fan et al., 2016).
Three biological replicates were prepared and analyzed in this work, and the data were presented as means ± standard deviations (SD). Student’s t-test and Pearson correlation analysis were performed in SPSS statistical software (version 26.0). Values of p < 0.05 and p < 0.01 were considered to be the significant differences and extremely significant differences, respectively.
Four structural genes, namely, DXS, STR, SLS, and TDC, are involved in the TIA biosynthetic pathway (Liu et al., 2021). To screen additional DEGs, the expression patterns of these four key enzyme-encoding genes (DoDXS, DoSTR, DoSLS, and DoTDC) in D. officinale PLBs were analyzed. The expression of the four genes was significantly increased under the TIA precursor and MeJA treatment (Figure 2). DoDXS, DoSLS and DoTDC, but not DoSTR, showed strong induction by the precursors and MeJA. The expression of DoDXS and DoSLS peaked after 4 h of treatment; however, DoTDC expression peaked after 8 h of treatment. The expression pattern of DoSTR was different, with a first peak observed after 4 h of treatment, followed by a second peak after 24 h. These results suggested that the expression levels of DoDXS, DoSTR, DoSLS, and DoTDC were markedly increased in the samples treated for 4 and 24 h compared with the control samples. Therefore, we selected PLBs that were treated for 0, 4 and 24 h and grew normally up to 4 and 24 h as the transcriptome sequencing samples.
Figure 2. Expression patterns of four key genes involved in the TIA biosynthetic pathway of D. officinale PLBs under precursor and MeJA treatment.
As alkaloids are the main bioactive constituents in D. officinale, their levels in the control (CK) and Trp + S + MeJA-treated PLBs were measured. We observed no significant differences in total alkaloid content between PLBs treated after 24 h and non-treated samples. However, the total alkaloid content increased significantly from 189 to 319 μg/g DW after 4 h of treatment (Figure 1B). These data indicated an important role of the precursors and MeJA in the accumulation of alkaloids in D. officinale.
To further understand the transcriptional regulatory mechanism of the precursors and MeJA in TIA biosynthesis in D. officinale, 15 cDNA libraries from D. officinale PLBs treated for different durations (0, 4 and 24 h) were constructed and sequenced. Five groups of cDNA libraries (treated after 0 h: T0_1, T0_2, T0_3; treated after 4 h: T4_1, T4_2, T4_3; treated after 24 h: T24_1, T24_2, T24_3; not treated after 4 h: C4_1, C4_2, C4_3; not treated after 24 h: C24_1, C24_2, C24_3) were separately prepared. The raw reads of the libraries were deposited in the NCBI SRA database. The quality of the RNA-Seq data is summarized in Table 1. The average Q20, Q30, and GC content were 97.30, 92.85, and 47.65%, respectively. Correlation analysis revealed that the 15 samples could be divided into five groups and that the replicates had a strong positive correlation (Supplementary Figure 2), indicating high reproducibility and reliability of the transcriptome data. In addition, 89.84% of the clean reads were mapped to the reference genome, suggesting that the selected reference genome assembly could meet the needs for information analysis.
A total of 32,727 unigenes were obtained, of which 29,149 were from the genome of D. officinale and 3,578 were new genes. These unigenes were annotated in public databases, including the NR, GO, COG, Swiss-Prot, and KEGG databases. Among them, 31,979 unigenes were annotated in at least one database.
GO classification was used to classify unigene functions based on the NR annotation. Of the 31,797 assembled unigenes, 12,078 unigenes were successfully assigned to one or more GO terms and classified into three main GO categories and 50 groups (Supplementary Figure 3A). Within the “biological process” (BP) domain, the most evident matches were in the terms “metabolic process” (5,783 unigenes), “cellular process” (5,276 unigenes), and “single-organism process” (3,535 unigenes). In the “cellular component” (CC) domain, most of the unigenes were assigned to the terms “cell” (5,376 unigenes) and “cell part” (5,355 unigenes). In the “molecular function” (MF) domain, the assignments were mostly enriched in the term “catalytic activity” (5,769 unigenes).
All the unigenes were also subjected to a search against the COG database for functional product information and classification. A total of 9,047 unigenes were assigned a COG functional classification and divided into 25 categories (Supplementary Figure 3B). Among them, the most common group was “general function prediction only” (1,003 unigenes, 11.09%), followed by “signal transduction mechanisms” (906 unigenes, 10.01%) and “translation, ribosomal structure and biogenesis” (870 unigenes, 9.62%). Notably, 525 unigenes were annotated in the “secondary metabolites biosynthesis, transport and catabolism” group, suggesting that these unigenes may be related to alkaloid biosynthesis in D. officinale PLBs.
Then, KEGG pathway analysis was performed to functionally classify biochemical pathways associated with the unigenes. A total of 4,783 unigenes were assigned to five KEGG categories with 120 subcategories: “metabolism,” “genetic information processing,” “environmental information processing,” “cellular processes” and “organismal systems” (Supplementary Figure 4). A summary of the findings is presented in Supplementary Table 2. Of the KEGG secondary metabolic pathways, 311 unigenes were assigned to “flavonoid biosynthesis,” “flavone and flavonol biosynthesis,” “phenylpropanoid biosynthesis,” “isoquinoline alkaloid biosynthesis” and “tropane, piperidine and pyridine alkaloid biosynthesis.” The metabolic pathways annotated in the KEGG database, such as “phenylalanine, tyrosine and tryptophan biosynthesis” (ko00400) and “terpenoid backbone biosynthesis” (ko00900), were related to the TIA biosynthesis pathway.
To identify the differentially expressed unigenes between these samples, we used FDR < 0.01 and | log2FC (fold change)| ≥ 1 as the selection parameters. Here, the comparison of C4 vs. T4 and C24 vs. T24 yielded 3,538 and 4,160 DEGs, respectively. A volcano plot was constructed to illustrate the distribution of DEGs in these two comparison groups (Figure 3A). Among the detected DEGs, the C4 vs. T4 group had 2,049 upregulated and 1,489 downregulated genes, and the C24 vs. T24 group had 1,831 upregulated and 2,329 downregulated genes (Figure 3B). In particular, 1,815 DEGs were present in both comparison groups, 1,723 DEGs were present in only the C4 vs. T4 group, and 2,345 DEGs were present in only the C24 vs. T24 group (Figure 3C). In addition, among the 2,731 upregulated DEGs, 1,149 were shared by the two comparison groups, 900 were present in only the C4 vs. T4 group, and 682 DEGs were present in only the C24 vs. T24 group. There were 3,216 downregulated DEGs in the two comparison groups, among which 602 were present in both comparison groups, 887 were present in only the C4 vs. T4 group, and 1,727 DEGs were present in only the C24 vs. T24 group (Figure 3D).
Figure 3. Differentially expressed genes (DEGs) identified by RNA-Seq analysis in Trp + S + MeJA-treated and control samples after induction for 4 and 24 h. (A) Volcano plot of the RNA-Seq data showing DEGs in red and green. The x-axis indicates the fold change in C4 vs. T4 and C24 vs. T24. The y-axis represents the negative -log10-transformed p-values (FDR < 0.01) for differences between the samples. (B) DEG numbers in the C4 vs. T4 and C24 vs. 24 groups. (C) Venn diagram of all DEGs in the C4 vs. T4 and C24 vs. T24 groups. (D) Venn diagram of upregulated and downregulated DEGs in the C4 vs. T4 and C24 vs. T24 groups.
To further understand the biological functions of the DEGs, we compared these DEGs against the GO and KEGG databases. The 2,731 upregulated and 3,216 downregulated DEGs in the above two comparison groups (C4 vs. T4 and C24 vs. T24) were analyzed by GO and KEGG enrichment analysis. In the GO analysis, the “single-organism process,” “biological regulation,” “localization,” “signaling,” “multi-organism process,” “transporter activity,” and “nucleic acid binding transcription factor activity” subcategories contained mainly upregulated DEGs, whereas the other subcategories contained mainly downregulated DEGs (Figure 4). In the KEGG analysis, upregulated DEGs were mainly enriched in alkaloid biosynthesis pathways, including “isoquinoline alkaloid biosynthesis,” “tropane, piperidine and pyridine alkaloid biosynthesis,” and “phenylalanine, tyrosine and tryptophan biosynthesis” (Figure 5A). Some upregulated DEGs were also significantly enriched in “plant hormone signal transduction” and “α-linolenic acid metabolism” (endogenous JA biosynthesis pathway). The downregulated DEGs were mainly related to plant growth and development, fatty acid metabolism, and amino acid and energy metabolism pathways, such as “photosynthesis-antenna proteins,” “carbon fixation in photosynthetic organisms,” “pyrimidine metabolism” and “cutin, suberine and wax biosynthesis” (Figure 5B).
Figure 4. GO enrichment of DEGs in Trp + S + MeJA-treated and non-treated PLBs of D. officinale. The x-axis indicates the percentage of DEGs in the subcategories of each main category. The y-axis indicates the number of DEGs in each subcategory.
Figure 5. KEGG enrichment analysis of DEGs in Trp + S + MeJA-treated and non-treated PLBs of D. officinale. (A) KEGG enrichment analysis of upregulated DEGs. (B) KEGG enrichment analysis of downregulated DEGs. The y-axis and x-axis represent the KEGG pathways and the enrichment factors, respectively. The greater the enrichment factor is, the more obvious the enrichment level of DEGs in the pathway. The color of the block represents the q-value, and the q-value is the p-value corrected by multiple hypothesis tests. The smaller the q-value is, the more reliable the enrichment of DEGs in this pathway is. The size of the circle indicates the number of genes enriched in the pathway.
To identify the genes associated with alkaloid biosynthesis under precursor and MeJA treatment, a WGCNA was performed with all 9,248 genes, resulting in 24 coexpressed modules (Figure 6A). The largest module (“blue”) contained 4,044 genes, while the smallest module (“paleturquoise”) contained 37 genes (Figure 6B). Subsequently, the total alkaloid content was used as a phenotype for correlation analysis with the obtained modules. The “brown” module contained 885 genes was significantly positively correlated with alkaloid, with correlation coefficient (r) of 0.82 (p = 2 × 10–4).
Figure 6. Weighted gene coexpression network analysis (WGCNA) and modules related to alkaloid biosynthesis in D. officinale PLBs. (A) Dendrogram with color annotation. (B) Pearson correlation coefficient (r) and the p-value for alkaloid content and each module. The color scale on the right shows module-trait correlation from −1 (blue) to 1 (red).
Based on the functional annotation and WGCNA results, the genes involved in the TIA biosynthesis [mevalonate (MVA) pathway, 2-C-methyl-D-erythritol 4-phosphate (MEP), seco-iridoid pathway, shikimate pathway and TIA downstream biosynthetic pathway], isoquinoline alkaloid biosynthesis, tropane alkaloid biosynthesis and JA biosynthesis (α-linolenic acid metabolism) were further analyzed. Finally, we identified 125 unigenes annotated as 36 enzymes involved in alkaloid biosynthesis (Supplementary Table 3).
Furthermore, the transcriptomic analysis showed that 35 unigenes exhibited differential expression, and most of them were highly expressed in the Trp + S + MeJA-treated groups (4 and 24 h treated PLBs) compared to the non-treated groups (Figure 7). Of these DEGs, 23 were related to TIA biosynthesis, and 15 showed upregulation under Trp + S + MeJA treatment after 4 or 24 h; these DEGs encode putative HDS (Dca013365), FPS (Dca007721), G10H (Dca009687 and Dca027610), 7DGT (Dca019145), 7DLGT (Dca028752 and Dca029204), DHS (Dca004228 and Dca021396), DHQS (Dca008449), EPSP (Dca016200), CS (Dca015447), AS (Dca008461), TBS (Dca012193) and STR (Dca003087) proteins. However, the expression of DoSLS and DoSK, important genes in the seco-iridoid and shikimate pathways, respectively, decreased after Trp + S + MeJA treatment. Twelve DEGs were identified as being involved in isoquinoline and tropane alkaloid biosynthesis, all of which were upregulated by Trp + S + MeJA treatment except DoADH (Dca009999). The increased expression of these genes subsequently induced alkaloid accumulation, suggesting that the precursors and MeJA could stimulate secondary metabolism via the main biosynthesis pathways and enhance the alkaloid content of PLBs in D. officinale.
Figure 7. Expression patterns of genes putatively involved in the biosynthesis of alkaloids in D. officinale PLBs under precursor and MeJA treatment. The enzymes are marked in red, indicating that genes encoding the enzymes showed different expressions in the C4 vs. T4 or C24 vs. T24 groups. The genes marked in red in the heat map indicates the DEGs identified from the C4 vs. T4 or C24 vs. T24 groups. Blue numbers in brackets represent the correlation coefficient which analyzed between alkaloid content and biosynthesis-related genes. **p < 0.01 and —r— > 0.8. The red triangle indicates that the gene was found in the brown module.
JA derivatives, including jasmonoyl-isoleeucine (JA-ile) and MeJA, are known to promote alkaloid accumulation in various plants (Wasternack and Strnad, 2019). Therefore, we next analyzed the key genes in the JA biosynthesis pathway. As shown in Figure 8A and Supplementary Table 4, we identified 45 unigenes that encoded nine enzymes involved in the biosynthetic pathway of JA. The majority of the genes functioning in this pathway were upregulated, with 18 DEGs in the C4 vs. T4 or C24 vs. T24 group (Figure 8B). Further analysis showed that their expression patterns were similar to those of the DEGs in the alkaloid biosynthesis pathway at different treatment times. Strikingly, the differential expression was more significant at 4 h than at 24 h after Trp + S + MeJA treatment. For example, 29.45- and 1625.08-fold increases in DoLOX (Dca020949) and DoOPR (newGene_9791) expression were observed after 4 h of treatment, but the expression levels increased only 14.56- and 158.31-fold, respectively, at 24 h.
Figure 8. Expression pattern analysis of genes involved in JA biosynthesis in D. officinale PLBs under the precursor and MeJA treatment. (A) JA biosynthesis pathway. The enzymes are marked in red, indicating that genes encoding the enzymes were differentially expressed in the C4 vs. T4 or C24 vs. T24 groups. (B) Heat map of genes associated with JA biosynthesis. The genes marked in red in the heat map indicates the DEGs identified from the C4 vs. T4 or C24 vs. T24 groups. Blue numbers in brackets represent the correlation coefficient which analyzed between alkaloid content and JA biosynthesis-related genes. **p < 0.01 and —r— > 0.8. The red triangle indicates that the gene was found in the brown module.
A correlation analysis between alkaloid content and the transcriptional abundance of key genes involving the TIA, isoquinoline alkaloid, tropane alkaloid and JA biosynthesis pathways was further conducted. As shown in Figures 7, 8B, the alkaloid content was highly correlated with the expression of nine and eight genes associated with alkaloid biosynthetic pathway and JA biosynthesis pathway, respectively, (|r| > 0.8 and p < 0.01). In particular, six genes involved in alkaloid biosynthesis, such as DHS (Dca021396), DoADH (Dca025688), DoTYDC (Dca021379), and DoTR (Dca000522, Dca027425, and Dca000521), and seven genes related to JA bisynthesis, including DoLOX (Dca023278), DoAOS (Dca022684) and DoOPR (newGene_9791, newGene_13686, Dca023268, Dca001807, and Dca000910) were also found in the brown module. The above results indicated that these genes were very relevant to alkaloid biosynthesis in D. officinale.
Various TFs have been reported to participate in the biosynthesis of secondary metabolites in plants. In our study, 1,438 unigenes belonging to 48 major TF families were identified in D. officinale PLBs (Supplementary Table 5). Members of the MYB, C2H2, AP2/ERF, NAC, and bHLH families constituted the top five classes, each with more than 80 unigenes, and the MYB family was the largest family, containing 170 members. However, members of the CSD, LIM, Whirly and LFY families had < 4 unigenes.
To systematically study the regulatory networks of alkaloid biosynthesis-related genes and TFs, a total of 66 TFs were found in the brown module, and their expression patterns are presented in Figure 9A. Most of these TFs exhibited similar expression patterns; their expression levels were significantly upregulated at 4 h and remained high at 24 h. As shown in Figure 9A, these TFs belonged to 18 TF families, including AP2/ERF (10), MYB (9), WRKY (9), bHLH (6), Tify (5), bZIP (4), NAC (3), Trihelix (3), MADS-box (3), C3H (2), OFP (2), GRAS (2), C2H2 (2), LOB (2), HSF (1),TCP (1), GRF (1), and GeBP (1). Among them, the expression of 24 TFs were positively correlated with the total alkaloid content (r > 0.8 and p < 0.01). The interaction network diagram analyzed with Cytoscape showed that 13 of the above-mentioned TFs were highly coexpressed with DoFPS (Dca007721), DoDHQS (Dca008449), DoDHS (Dca021396 and Dca004228), DoCS (Dca015447), DoCM (Dca012195), DoTYDC (Dca021379), DoADH (Dca025688), DoTR (Dca027425), DoAOS (Dca022684 and Dca000659), and DoOPR (Dca023268) (Figure 9B), which further illustrated that they play a vital role in the regulation of alkaloid biosynthesis in D. officinale.
Figure 9. Coexpression network of TFs and structure genes related to alkaloid biosynthesis pathway in D. officinale PLBs under the precursor and MeJA treatment. (A) Heat map displaying the expression of 66 TFs in different samples. (B) Construction of regulatory networks of TFs and structure genes related to alkaloid biosynthesis. **p < 0.01 and |r| > 0.8.
To verify the accuracy of the transcriptomic data, the expression levels of five DEGs related to TIA biosynthesis: DoFPS (Dca007721), Do7DGT (Dca019145), Do7DLGT (Dca028752), DoTBS (Dca012193), and DoAS (Dca008461); two DEGs related to isoquinoline alkaloid: DoTYDC (Dca021379) and DoNCS (Dca007610); one DEG related to tropane alkaloid: DoTR (Dca000521); two terpene synthase DEGs: DoLIS (Dca005188) and DoTPS (Dca020940); one DEG involved in JA biosynthesis: DoAOS (Dca022684); and five TFs regulating alkaloid biosynthesis: DoMYC2 (Dca025360), DoAP2/ERF (Dca016049), DoWRKY (Dca028175), DoMYB (Dca025582) and DobZIP (Dca013831) were checked using qRT-PCR, with three biological replicates tested. The relationship between the RNA-Seq and qRT-PCR in different samples was also analyzed based on Pearson’s correlation analysis. Results showed that the relative expression trend of most genes was basically consistent with the RNA-Seq data, and some correlation values were higher than 0.8 (Figure 10), which further demonstrated the reliability of the transcriptome data. These results confirmed that our transcriptome data could serve as a foundation for further research on related genes associated with other medicinal components of D. officinale.
Figure 10. qRT-PCR validation of DEGs related to alkaloid biosynthesis and metabolic regulation in D. officinale PLBs. The left y-axis represents the relative expression levels corresponding to the histogram. The right y-axis represents expression levels calculated by the FPKM method corresponding to the line plots. The relationship between the RNA-Seq and qRT-PCR in T0, C4, T4, C24, and T24 samples was analyzed based on Pearson’s correlation analysis and r represents correlation coefficients. *p < 0.05, **p < 0.01.
D. officinale is well known as one of the most important traditional herbs in China, and its alkaloids are its main medicinal components. However, the regulatory mechanism underlying the precursor and MeJA-induced accumulation of alkaloids in D. officinale PLBs is still unknown. To date, a number of transcriptomic analyses have been performed for different reasons, such as the identification of genes associated with the biosynthesis of bioactive components (polysaccharides, flavonoids, alkaloids, and terpenes) (Ren et al., 2020; Wang et al., 2020; Li et al., 2021), flower development (He et al., 2020; Chen et al., 2021), and environmental responses (Jiang et al., 2020; Zhang et al., 2021). In this study, D. officinale PLBs treated with TIA precursors (Trp and S) and MeJA were used for transcriptome sequencing on the Illumina HiSeq platform. Genes associated with the biosynthesis of three types of alkaloids, namely, TIAs, isoquinoline alkaloids and tropane alkaloids, were annotated in the transcriptomic data. This result was also consistent with our metabonomic analysis (Jiao et al., 2018), which further confirmed that these three types of alkaloids exist in D. officinale PLBs.
Generally, precursors and MeJA can regulate the expression of biosynthetic genes in the TIA pathway (Wei, 2010; Pan et al., 2018; Sharma et al., 2019). The transcriptomes of D. officinale PLBs treated with TIA precursors and MeJA for 4 and 24 h were compared with those of PLBs grown normally for 4 and 24 h, respectively. We found that the TIA precursors and MeJA could positively regulate the transcription of most genes of the TIA, isoquinoline alkaloid and tropane alkaloid biosynthesis pathways to promote the accumulation of alkaloids. Genes in the MVA/MEP pathway (DoHDS and DoFPS), seco-iridoid pathway (DoG10H, Do7DGT, and Do7DLGT), shikimate pathway (DoDHS, DoDHQS, DoEPSP, DoCS, DoAS, and DoTBS) and a key enzyme-encoding gene (DoSTR) were all upregulated significantly (Figure 7). Therefore, it is speculated that the precursors and MeJA promote the accumulation of TIAs by regulating the transcription of genes related to the TIA biosynthesis pathway in Dendrobium officinale PLBs.
A similar phenomenon was also observed for isoquinoline and tropane alkaloid biosynthetic genes, including DoADH, DoCM, DoTYDC, and DoNCS in the isoquinoline alkaloid biosynthetic pathway and DoTR in the tropane alkaloid biosynthetic pathway, which showed a significant upward trend at the transcriptional level. As reported previously, transcriptome analysis of Papaver somniferum treated with MeJA showed that the expression of PsNCS, which belongs to the isoquinoline alkaloid biosynthesis pathway, was significantly upregulated (Gurkok et al., 2015). This result is consistent with our study. Thus, we speculate that precursors and MeJA can promote the biosynthesis of isoquinoline alkaloids (such as xanthoplanine, which was identified in our previous study) by positively regulating the expression levels of DoADH, DoCM, DoTYDC, and DoNCS. Tropinone reductase (TR), a stereospecific NADPH-dependent reductase, is a key enzyme involved in the metabolism of tropinone (Kohnen-Johannsen and Kayser, 2019). TR has been found in Dendrobium huoshanense and Dendrobium nobile, and its expression was also induced by MeJA (Wang et al., 2020). In our study, the expression of DoTR was significantly upregulated after Trp + S + MeJA treatment (Figure 7). This indicated that DoTR was involved in regulating the biosynthesis of tigloidine (a type of tropane alkaloid found in our previous study) in D. officinale PLBs.
Exogenous MeJA can induce the expression of genes related to the endogenous JA biosynthesis pathway to improve the level of endogenous JA in plants, which constitutes the self-activation of JA biosynthesis (Wasternack and Strnad, 2019; Griffiths, 2020). Here, our transcriptomic analysis showed that the expression levels of endogenous JA biosynthesis pathway-related genes, such as DoLOX, DoAOS, DoOPR, and DoJMT, increased significantly in Trp + S + MeJA-treated D. officinale PLBs. It is speculated that treatment with TIA precursors and MeJA leads to the upregulation of the transcription of genes related to the JA biosynthesis pathway to improve the expression of α-linolenic acid and the endogenous JA levels (Jiao et al., 2018).
WGCNA was used to analyze the correlation between physiological indicators and genes (Yang et al., 2021). This method has been applied to study the regulatory networks of the triterpene saponin biosynthesis in tea (Camellia sinensis) (Chen et al., 2022), anthocyanin accumulation in apple (Malus domestica) (Song et al., 2019), and lignin metabolism in pomelo (Citrus maxima) (Li et al., 2022). In our study, WGCNA was also used to further analyze the association between the coexpressed gene modules formed by all genes and the alkaloid content. The brown module was highly positively correlated (0.82) with the alkaloid content and contained 885 genes, indicating these genes might play an important role in alkaloid biosynthesis (Figure 6B). In the brown module, a total of six genes involved in alkaloid biosynthesis, such as DoDHS, DoADH, DoTYDC, and DoTR, and seven genes related to JA bisynthesis, such as DoLOX, DoAOS, and DoOPR, were significantly correlated with the alkaloid content and may play a vital role in the alkaloid biosynthesis of D. officinale.
As reported previously, the TFs involved in regulating the biosynthesis of TIAs or isoquinoline alkaloids are mainly bHLH, AP2/ERF, WRKY, MYB, and bZIP family members (Zhou and Memelink, 2016). In C. roseus, it has been suggested that CrMYC1 and CrMYC2 (bHLH TFs), CrORCA1, CrORCA2, and CrORCA3 (AP2/ERF TFs), CrWRKY1 (a WRKY TF), CrBPF1 (an MYB TF), and CrGBF1, and CrGBF2 (bZIP TFs) can regulate the expression of genes related to the TIA biosynthesis pathway (Sibéril et al., 2001; Zhou and Memelink, 2016). Furthermore, CjWRKY1 and CjbHLH1 TFs, which can regulate the biosynthesis of isoquinoline alkaloids, have been found in Coptis japonica (Kato et al., 2007). Besides, JAZ proteins, belonging to the plant-specific Tify family, also play important regulatory role in alkaloids biosynthesis (Yamada and Sato, 2021). In this study, a total of 66 TFs, which mostly belonged to AP2/ERF, MYB, bHLH, WRKY, bZIP, and Tify families, were obtained after WGCNA analysis, and their expression were almost positively related to the alkaloid content. Moreover, 13 of the above TFs might control alkaloid biosynthesis by transcriptionally regulating DoFPS (Dca007721), DoDHQS (Dca008449), DoDHS (Dca021396 and Dca004228), DoCS (Dca015447), DoCM (Dca012195), DoTYDC (Dca021379), DoADH (Dca025688), DoTR (Dca027425), DoAOS (Dca022684 and Dca000659), and DoOPR (Dca023268) (Figure 9B). So far, the knowledge on the TFs to alkaloid biosynthesis in D. officinale are still limited. More studies are needed to further explore the regulatory network of alkaloid metabolism in D. officinale.
In this study, we compared the total alkaloid content in treated (Trp + S + MeJA) and non-treated D. officinale PLBs, and a comparative transcriptomic analysis was performed. The total alkaloid content in 4 h-treated PLBs was much higher than that in 4 h-non-treated samples. 13 genes encoding DoDHS, DoADH, DoTYDC, DoTR, DoLOX, DoAOS, and DoOPR enzymes, might be the key genes involved in alkaloid biosynthesis. In addition, most of the 13 TFs belonging to AP2/ERF, WRKY, and MYB gene families, were predicted to be associated with alkaloid accumulation by WGCNA, and deserve to be further investigated as key regulators of alkaloid metabolism. This study will facilitate future research on transcriptional regulation mechanisms in D. officinale and other traditional Chinese medicinal plants.
Our RNA-seq data of 15 cDNA libraries have been deposited in the repositories of NCBI Sequence Read Archive with Bioproject accession number: PRJNA816308 and SRA accession numbers: SRR18338800-SRR18338814.
QJ, HF, and YC conceived the study and participated in its design and coordination. CJ performed the experimental measurements, processed the experimental data, and wrote the manuscript. MW and CS interpreted the experimental data. ZW and CS helped in revising the manuscript and pictures. All authors contributed to the article and approved the submitted version.
This work was supported by the Natural Science Research Project of Provincial Natural Science Foundation of Anhui (2108085MC80), the Foundation of State Key Laboratory of Utilization of Woody Oil Resource (GZKF202103), the National College Students Innovation and Entrepreneurship Training Program (202114098068), the Innovation and Entrepreneurship Training Program for College Students in Anhui Province (14098013 and 14098014), the School-Level Scientific Research Project of Hefei Normal University (2021KJQN07), and the High-level Talent Scientific Research Start-up Fund Project of West Anhui University (WGKQ2022025).
The authors declare that the research was conducted in the absence of any commercial or financial relationships that could be construed as a potential conflict of interest.
The handling editor, YC, declared a past co-authorship with the authors, YC and CS.
All claims expressed in this article are solely those of the authors and do not necessarily represent those of their affiliated organizations, or those of the publisher, the editors and the reviewers. Any product that may be evaluated in this article, or claim that may be made by its manufacturer, is not guaranteed or endorsed by the publisher.
The Supplementary Material for this article can be found online at: https://www.frontiersin.org/articles/10.3389/fpls.2022.941231/full#supplementary-material
Cao, H., Ji, Y., Li, S., Lu, L., Tian, M., Yang, W., et al. (2019). Extensive metabolic profiles of leaves and stems from the medicinal plant Dendrobium officinale Kimura et Migo. Metabolites 9:215. doi: 10.3390/metabo9100215
Chen, C., Zhu, H., Kang, J., Warusawitharana, H. K., Chen, S., Wang, K., et al. (2022). Comparative transcriptome and phytochemical analysis provides insight into triterpene saponin biosynthesis in seeds and flowers of the tea plant (Camellia sinensis). Metabolites 12:204. doi: 10.3390/metabo12030204
Chen, Y., Hu, B., Zhang, F., Luo, X., and Xie, J. (2021). Cytological observation and transcriptome comparative analysis of self-pollination and cross-pollination in Dendrobium officinale. Genes 12:432. doi: 10.3390/genes12030432
Chen, Y., Wang, Y., Lyu, P., Chen, L., Shen, C., and Sun, C. (2019). Comparative transcriptomic analysis reveal the regulation mechanism underlying MeJA-induced accumulation of alkaloids in Dendrobium officinale. J. Plant Res. 132, 419–429. doi: 10.1007/s10265-019-01099-6
Fan, H., Wu, Q., Wang, X., Wu, L., Cai, Y., and Lin, Y. (2016). Molecular cloning and expression of 1-deoxy-d-xylulose-5-phosphate synthase and 1-deoxy-d-xylulose-5-phosphate reductoisomerase in Dendrobium officinale. Plant Cell Tiss. Organ Cult. 125, 381–385. doi: 10.1007/s11240-016-0945-1
Florea, L., Song, L., and Salzberg, S. L. (2013). Thousands of exon skipping events differentiate among splicing patterns in sixteen human tissues. F1000Res. 2:188. doi: 10.12688/f1000research.2-188.v1
Ge, Q., Zhang, Y., Hua, W. P., Wu, Y. C., Jin, X. X., Song, S. H., et al. (2015). Combination of transcriptomic and metabolomic analyses reveals a JAZ repressor in the jasmonate signaling pathway of Salvia miltiorrhiza. Sci. Rep. 5:14048. doi: 10.1038/srep14048
Griffiths, G. (2020). Jasmonates: biosynthesis, perception and signal transduction. Essays Biochem. 64, 501–512. doi: 10.1042/EBC20190085
Guo, L., Qi, J., Du, D., Liu, Y., and Jiang, X. (2020). Current advances of Dendrobium officinale polysaccharides in dermatology: a literature review. Pharm. Biol. 58, 664–673. doi: 10.1080/13880209.2020.1787470
Guo, X., Li, Y., Li, C., Luo, H., Wang, L., Qian, J., et al. (2013). Analysis of the Dendrobium officinale transcriptome reveals putative alkaloid biosynthetic genes and genetic markers. Gene 527, 131–138. doi: 10.1016/j.gene.2013.05.073
Gurkok, T., Turktas, M., Parmaksiz, I., and Unver, T. (2015). Transcriptome profiling of alkaloid biosynthesis in elicitor induced opium poppy. Plant Mol. Biol. Rep. 33, 673–688. doi: 10.1007/s11105-014-0772-7
He, C., Liu, X., Teixeira da Silva, J. A., Liu, N., Zhang, M., and Duan, J. (2020). Transcriptome sequencing and metabolite profiling analyses provide comprehensive insight into molecular mechanisms of flower development in Dendrobium officinale (Orchidaceae). Plant Mol. Biol. 104, 529–548. doi: 10.1007/s11103-020-01058-z
Huang, Y., Tan, H., Guo, Z., Wu, X., Zhang, Q., Zhang, L., et al. (2016). The biosynthesis and genetic engineering of bioactive indole alkaloids in plants. J. Plant Biol. 59, 203–214. doi: 10.1007/s12374-016-0032-5
Jeet, A., Singh, Y., Singh, P., Nimoriya, R., Bilung, C. J., Kanojiya, S., et al. (2020). Strategies for indole alkaloids enrichment through callus culture from Alstonia scholaris (L.) R. Br. Plant Growth Regul. 90, 383–392. doi: 10.1007/s10725-019-00570-7
Jiang, W., Wu, Z., Wang, T., Mantri, N., Huang, H., Li, H., et al. (2020). Physiological and transcriptomic analyses of cadmium stress response in Dendrobium officinale seedling. Plant Physiol. Biochem. 148, 152–165. doi: 10.1016/j.plaphy.2020.01.010
Jiao, C., Song, C., Zheng, S., Zhu, Y., Jin, Q., Cai, Y., et al. (2018). Metabolic profiling of Dendrobium officinale in response to precursors and methyl jasmonate. Int. J. Mol. Sci. 19:728. doi: 10.3390/ijms19030728
Kato, N., Dubouzet, E., Kokabu, Y., Yoshida, S., Taniguchi, Y., Dubouzet, J. G., et al. (2007). Identification of a WRKY protein as a transcriptional regulator of benzylisoquinoline alkaloid biosynthesis in Coptis japonica. Plant Cell Physiol. 48, 8–18. doi: 10.1093/pcp/pcl041
Kohnen-Johannsen, K. L., and Kayser, O. (2019). Tropane alkaloids: chemistry, pharmacology, biosynthesis and production. Molecules 24:796. doi: 10.3390/molecules24040796
Langfelder, P., and Horvath, S. (2008). WGCNA: an R package for weighted correlation network analysis. BMC Bioinformatics 9:559. doi: 10.1186/1471-2105-9-559
Li, N., Dong, Y., Lv, M., Qian, L., Sun, X., Liu, L., et al. (2021). Combined analysis of volatile terpenoid metabolism and transcriptome reveals transcription factors related to terpene synthase in two cultivars of Dendrobium officinale flowers. Front. Genet. 12:661296. doi: 10.3389/fgene.2021.661296
Li, X., Huang, H., Rizwan, H. M., Wang, N., Jiang, J., She, W., et al. (2022). Transcriptome analysis reveals candidate lignin-related genes and transcription factors during fruit development in Pomelo (Citrus maxima). Genes 13:845. doi: 10.3390/genes13050845
Liu, Y., Patra, B., Singh, S. K., Paul, P., Zhou, Y., Li, Y., et al. (2021). Terpenoid indole alkaloid biosynthesis in Catharanthus roseus: effects and prospects of environmental factors in metabolic engineering. Biotechnol. Lett. 43, 2085–2103. doi: 10.1007/s10529-021-03179-x
Lv, D., Wang, G., Zhang, Q., Yu, Y., Qing, P. C., Pang, J. A., et al. (2022). Comparative transcriptome analysis of hard and tender fruit spines of cucumber to identify genes involved in the morphological development of fruit spines. Front. Plant Sci. 13:797433. doi: 10.3389/fpls.2022.797433
Mou, Z., Zhao, Y., Ye, F., Shi, Y., Kennelly, E. J., Chen, S., et al. (2021). Identification, biological activities and biosynthetic pathway of Dendrobium alkaloids. Front. Pharmacol. 12:605994. doi: 10.3389/fphar.2021.605994
Pan, Y. J., Lin, Y. C., Yu, B. F., Zu, Y. G., Yu, F., and Tang, Z. H. (2018). Transcriptomics comparison reveals the diversity of ethylene and methyl-jasmonate in roles of TIA metabolism in Catharanthus roseus. BMC Genomics 19:508. doi: 10.1186/s12864-018-4879-3
Pertea, M., Kim, D., Pertea, G. M., Leek, J. T., and Salzberg, S. L. (2016). Transcript-level expression analysis of RNA-seq experiments with HISAT, StringTie and Ballgown. Nat. Protoc. 11, 1650–1667. doi: 10.1038/nprot.2016.095
Rapaport, F., Khanin, R., Liang, Y., Pirun, M., Krek, A., Zumbo, P., et al. (2013). Comprehensive evaluation of differential gene expression analysis methods for RNA-seq data. Genome Biol. 14:R95. doi: 10.1186/gb-2013-14-9-r95
Ren, Z., Qiu, F., Wang, Y., Yu, W., Liu, C., Sun, Y., et al. (2020). Network analysis of transcriptome and LC-MS reveals a possible biosynthesis pathway of anthocyanins in Dendrobium officinale. BioMed Res. Int. 2020:6512895. doi: 10.1155/2020/6512895
Sharma, A., Mathur, A. K., Ganpathy, J., Joshi, B., and Patel, P. (2019). Effect of abiotic elicitation and pathway precursors feeding over terpenoid indole alkaloids production in multiple shoot and callus cultures of Catharanthus roseus. Biologia 74, 543–553. doi: 10.2478/s11756-019-00202-5
Shen, C., Guo, H., Chen, H., Shi, Y., Meng, Y., Lu, J., et al. (2017). Identification and analysis of genes associated with the synthesis of bioactive constituents in Dendrobium officinale using RNA-Seq. Sci. Rep. 7:187. doi: 10.1038/s41598-017-00292-8
Sibéril, Y., Benhamron, S., Memelink, J., Giglioli-Guivarc’h, N., Thiersault, M., Boisson, B., et al. (2001). Catharanthus roseus G-box binding factors 1 and 2 act as repressors of strictosidine synthase gene expression in cell cultures. Plant Mol. Biol. 45, 477–488. doi: 10.1023/A:1010650906695
Song, T., Li, K., Wu, T., Wang, Y., Zhang, X., Xu, X., et al. (2019). Identification of new regulators through transcriptome analysis that regulate anthocyanin biosynthesis in apple leaves at low temperatures. PLoS One 14:e0210672. doi: 10.1371/journal.pone.0210672
Tang, H., Zhao, T., Sheng, Y., Zheng, T., Fu, L., and Zhang, Y. (2017). Dendrobium officinale Kimura et Migo: a review on its ethnopharmacology, phytochemistry, pharmacology, and industrialization. Evid. Based Complement. Alternat. Med. 2017:7436259. doi: 10.1155/2017/7436259
Wang, Z., Jiang, W., Liu, Y., Meng, X., Su, X., Cao, M., et al. (2021). Putative genes in alkaloid biosynthesis identified in Dendrobium officinale by correlating the contents of major bioactive metabolites with genes expression between Protocorm-like bodies and leaves. BMC Genomics 22:579. doi: 10.1186/s12864-021-07887-6
Wang, Z., Zhao, M., Cui, H., Li, J., and Wang, M. (2020). Transcriptomic landscape of medicinal Dendrobium reveals genes associated with the biosynthesis of bioactive components. Front. Plant Sci. 11:391. doi: 10.3389/fpls.2020.00391
Wasternack, C., and Strnad, M. (2019). Jasmonates are signals in the biosynthesis of secondary metabolites—pathways, transcription factors and applied aspects-a brief review. New Biotechnol. 48, 1–11. doi: 10.1016/j.nbt.2017.09.007
Wei, S. (2010). Methyl jasmonic acid induced expression pattern of terpenoid indole alkaloid pathway genes in Catharanthus roseus seedlings. Plant Growth Regul. 61, 243–251. doi: 10.1007/s10725-010-9468-7
Xu, J., Han, Q. B., Li, S. L., Chen, X. J., Wang, X. N., Zhao, Z. Z., et al. (2013). Chemistry, bioactivity and quality control of Dendrobium, a commonly used tonic herb in traditional Chinese medicine. Phytochem. Rev. 12, 341–367. doi: 10.1007/s11101-013-9310-8
Yamada, Y., and Sato, F. (2021). Transcription factors in alkaloid engineering. Biomolecules 11:1719. doi: 10.3390/biom11111719
Yang, J., Ren, Y., Zhang, D., Chen, X., Huang, J., Xu, Y., et al. (2021). Transcriptome-based WGCNA analysis reveals regulated metabolite fluxes between floral color and scent in Narcissus tazetta flower. Int. J. Mol. Sci. 22:8249. doi: 10.3390/ijms22158249
Zhan, J., Thakare, D., Ma, C., Lloyd, A., Nixon, N. M., Arakaki, A. M., et al. (2015). RNA sequencing of laser-capture microdissected compartments of the maize kernel identifies regulatory modules associated with endosperm cell differentiation. Plant Cell 27, 513–531. doi: 10.1105/tpc.114.135657
Zhang, M., Yu, Z., Zeng, D., Si, C., Zhao, C., Wang, H., et al. (2021). Transcriptome and metabolome reveal salt-stress responses of leaf tissues from Dendrobium officinale. Biomolecules 11:736. doi: 10.3390/biom11050736
Keywords: Dendrobium officinale, alkaloid biosynthesis, precursors, methyl jasmonate, transcriptome
Citation: Jiao C, Wei M, Fan H, Song C, Wang Z, Cai Y and Jin Q (2022) Transcriptomic analysis of genes related to alkaloid biosynthesis and the regulation mechanism under precursor and methyl jasmonate treatment in Dendrobium officinale. Front. Plant Sci. 13:941231. doi: 10.3389/fpls.2022.941231
Received: 11 May 2022; Accepted: 28 June 2022;
Published: 22 July 2022.
Edited by:
Yunpeng Cao, Chinese Academy of Sciences (CAS), ChinaReviewed by:
Lihu Wang, Hebei University of Engineering, ChinaCopyright © 2022 Jiao, Wei, Fan, Song, Wang, Cai and Jin. This is an open-access article distributed under the terms of the Creative Commons Attribution License (CC BY). The use, distribution or reproduction in other forums is permitted, provided the original author(s) and the copyright owner(s) are credited and that the original publication in this journal is cited, in accordance with accepted academic practice. No use, distribution or reproduction is permitted which does not comply with these terms.
*Correspondence: Qing Jin, cWluZ2ppbkBhaGF1LmVkdS5jbg==
Disclaimer: All claims expressed in this article are solely those of the authors and do not necessarily represent those of their affiliated organizations, or those of the publisher, the editors and the reviewers. Any product that may be evaluated in this article or claim that may be made by its manufacturer is not guaranteed or endorsed by the publisher.
Research integrity at Frontiers
Learn more about the work of our research integrity team to safeguard the quality of each article we publish.