- 1School of Life Science, Luoyang Normal University, Luoyang, China
- 2Royal Botanic Garden Edinburgh, Edinburgh, United Kingdom
- 3Key Laboratory of Adaptation and Evolution of Plateau Biota, Northwest Institute of Plateau Biology, Chinese Academy of Sciences, Xining, China
- 4Senckenberg Research Institute and Natural History Museum, Frankfurt, Germany
- 5Ashworth Laboratories, Institute of Evolutionary Biology, The University of Edinburgh, Edinburgh, United Kingdom
Understanding the evolutionary and ecological processes driving population differentiation and speciation can provide critical insights into the formation of biodiversity. Here, we examine the link between population genetic processes and biogeographic history underlying the generation of diversity in the Hengduan Mountains (HM), a region harboring a rich and dynamic flora. We used restriction site-associated DNA sequencing to generate 1,907 single-nucleotide polymorphisms (SNPs) and four-kb of plastid sequence in species of the Gentiana hexaphylla complex (Gentianaceae). We performed genetic clustering with spatial and non-spatial models, phylogenetic reconstructions, and ancestral range estimation, with the aim of addressing the processes influencing diversification of G. hexaphylla in the HM. We find the G. hexaphylla complex is characterized by geographic genetic structure with clusters corresponding to the South, North and the central HM. Phylogenetic reconstruction and pairwise FST analyses showed deep differentiation between Southern and Northern populations in the HM. The population in Mount Taibai exhibited the highest genetic similarity to the North HM. Ancestral range estimation indicated that the G. hexaphylla complex originated in the central HM and then diverged in the Pliocene and the Early Pleistocene, before dispersing widely, resulting in the current distinct lineages. Overall, we found deep genomic differentiation in the G. hexaphylla complex corresponds to geographic barriers to dispersal in the HM and highlights a critical role of the uplift of the Daxue Mountains and subsequent climatic fluctuations underlying diversification. The colonization of G. hexaphylla in the Mount Taibai region suggests directional dispersal between the alpine flora of the Qinling Mountains and the HM.
Introduction
Alpine floras, those that grow above the tree line, are particularly species-rich communities enriched with endemic taxa adapted to challenging environments with a short growing seasons and harsh winters. These communities have been profoundly shaped by recurrent cycles of historical climatic change, and continue to be affected by climate as conditions warm. Understanding the evolution of these diverse communities must consider not only past climatic changes, but the full range of processes promoting population divergence, range shifts, and speciation (Antonelli et al., 2018; Muellner-Riehl et al., 2019; Kirschner et al., 2020). In particular, in-depth biogeographic studies must consider cryptic species diversity, which may either be a consequence of in situ speciation, colonization by lowland taxa followed by allopatric divergence (local recruitment), or the recurrent immigration of novel and pre-adapted lineages via long-distance dispersal (Muellner-Riehl et al., 2019; Ding et al., 2020). Although relevant in all mountain systems of the world, research on these aspects has primarily focused on a few mountainous regions, such as the Hengduan Mountains (HM).
The Mountains of Southwest China, a global hotspot of biodiversity including the HM (Myers et al., 2000; Marchese, 2015), is an ideal area for exploring the spatial–temporal evolution of alpine communities and the drivers underlying speciation and diversification. This region harbors a rich flora with a high proportion of endemics (Wu, 1988; Boufford, 2014) and is characterized by a high rate of in situ speciation (Xing and Ree, 2017; Ding et al., 2020). There are estimated to be up to 16,550 species in the HM, accounting for approximately 62% of the total number of seed plant species in China, of which at least 3,300 are endemic (Sun et al., 2017). Moreover, the HM hosts a particularly rich alpine flora with an estimated 3,030 species of alpine seed plants (Sun et al., 2017). Furthermore, the complex topography of the HM, characterized by high ruggedness and deeply dissected landscapes, creates fine-scale environmental heterogeneity that may limit dispersal and subdivide distribution ranges, therefore creating complex population genetic structure and promoting divergence and incipient speciation (Scherrer and Körner, 2011; Li et al., 2021).
Major progress has been made in understanding the general processes shaping the evolutionary history of plants across the HM (Liu et al., 2014; Wen et al., 2014; Favre et al., 2015; Sun et al., 2017; Xing and Ree, 2017; Ding et al., 2020). The diversity of plant species is largely a product of vicariance and postglacial recolonization of alpine plants found in this region (Qiu et al., 2011; Liu et al., 2012; Sun et al., 2017; Muellner-Riehl, 2019), with recent in situ diversification in response to local uplift in the HM during the Late Miocene to the Pliocene (Favre et al., 2015; Ding et al., 2020). However, recent comparative analyses of plant species distributions across the region have shown that the HM is not a cohesive entity, but a diverse mosaic of floristic elements shaped by geography, elevation and climate (Li et al., 2021; Muellner-Riehl and Favre, 2021). As such, it is currently unclear how topological changes and changes of connectivity through time within and among each of the seven mountain subranges composing the HM have contributed to species-richness and endemism. The respective role of each of these subranges as regional refugia or sinks is also largely unknown, as case studies often consider the HM as a single entity (Liu et al., 2012; Muellner-Riehl, 2019). Finally, the alpine flora of the HM has not evolved in complete isolation, and adjacent regions such as the Qinling Mountains (QM, 400 km away), which includes Mount Taibai, the highest peak (3,500 m a.s.l.) in Central and East China, may have also been a source for speciation and floristic exchange of species now found in the HM.
The use of high-throughput sequencing is an extremely promising route to elucidate fine-scale genetic structure and the processes underlying speciation among the dynamic flora of the HM (Liu et al., 2014). Of particular value would be to use a large number of nuclear markers, which often provide high-levels of resolution for studying recent species divergence. Recent studies in Europe have emphasized the complex evolutionary history that has shaped the present genetic diversity of refugial populations, and stressed the need to revisit their phylogeographic history with genomic approaches (Dufresnes et al., 2020; Marková et al., 2020). However, genomic data needs to be matched with suitable analytical tools, to account for confounding factors that may obscure evolutionary inference. For example, one challenge is detecting clearly defined genetic units that have evolved independently in different geographic regions, against the background of clinal population structure arising as a result of isolation by distance (IBD) that is a common confounding factor that can obscure the genetic signature of biogeographic barriers (Meirmans, 2012; Perez et al., 2018; Twyford et al., 2020). Such issues can increasingly be accounted for and modelled using appropriate genomic datasets and analytical tools.
Gentiana L. (Gentianaceae), a worldwide alpine genus of about 360 species (Ho and Liu, 2001), is a group where general phylogenetic relationships (Favre et al., 2020) and biogeographic history (Favre et al., 2016) are relatively well understood. It was previously shown that the Qinghai-Tibetan Plateau region, including the HM, is the centre of biodiversity for the genus and the primary source area for colonization to other regions (Favre et al., 2016). The HM is home to 135 Gentiana species of which 66 are endemics (Yu et al., 2020). Phylogenetic and population genetic analyses indicate that climatic changes and mountain uplift are correlated with recent divergence, speciation and diversification in most clades of Gentiana (Zhang et al., 2007, 2009b; Lu et al., 2015; Fu et al., 2018, 2020a, 2021b). However, these previous studies treated the HM as a single geographic entity, and thus our knowledge of the phylogeographic history of the genus in the HM is not well understood at a finer scale.
In this study, we performed population genomic analysis of the gentian species G. hexaphylla Maximowicz ex Kusnezow, which belongs to G. series Verticillatae Marquand, with the aim of investigating fine-scale phylogeographic structure across the topologically complex HM. Previous phylogenetic studies including G. series Verticillatae indicate very young speciation events and recent radiations (Favre et al., 2016; Fu et al., 2021a), making this an ideal study group for investigating the recent biogeographic history of the region. Using genomic data, we address: (1) whether geographical features (e.g., one or more mountain ranges) in the HM acted as barriers to gene flow, and lead to discrete genetic structure and cryptic lineages; (2) whether changes in distribution range during climate oscillations lead to genetic differentiation and possibly speciation; (3) whether migration occurred between the HM and the nearby but disjunct mountain ranges such as the Qinling Mts.
Materials and methods
Study species and sampling
Gentiana hexaphylla is a widespread species with phenotypic variation in some characters [e.g., growth habit, leaf number per whorl and corolla colour (Ho and Liu, 2001)], but has only minor morphological differences from some closely related species in series Verticillatae (8 species in total, Ho and Liu, 2001), including G. ternifolia Franchet, G. tetraphylla Maximowicz ex Kusnezow and G. viatrix Harry Smith. Thus, we considered these closely related species, often co-occurring with G. hexaphylla, to collectively be part of the G. hexaphylla complex in this study. The G. hexaphylla complex occurs widely across the HM (Ho and Pringle, 1995; Ho and Liu, 2001), tends to reproduce sexually via outcrossing in open sunny habitats (Xue et al., 2018), and some species have horticultural value. Despite this interest, taxonomic confusion remains problematic for this group of species. For example, individuals from Mount Taibai have been recognized as G. hexaphylla in the Flora of China (Ho and Pringle, 1995) but treated as G. arethusae by Ho and Liu (2001). These species are very similar, and supposedly differ only by the linear and acuminate upper stem leaves and calyx lobes. Based on numerous field observations, these purported diagnostic traits appear to be highly variable (personal observations) and thus the population from Mount Taibai is treated as G. hexaphylla in this study.
We sampled 15 populations of the G. hexaphylla complex from 10 localities throughout its range, collecting a total of 95 individuals (Table 1; Figure 1). Our sampling aimed to maximize the number of sampling sites across the mountain ranges in the HM, in order to test for the presence of geographic barriers. Within sites, we collected multiple individuals to test for taxonomic clustering when two or more species co-occurred. For sequencing, we selected typical individuals characterized by leaf number per whorl ranging from three to six (Fu et al., 2020b). A total of 10 populations of G. hexaphylla, two populations of G. ternifolia, one population of G. tetraphylla and two populations of G. viatrix were sampled. Young leaves of each individual were dried in silica gel. Voucher specimens were deposited in the herbarium of Luoyang Normal University.
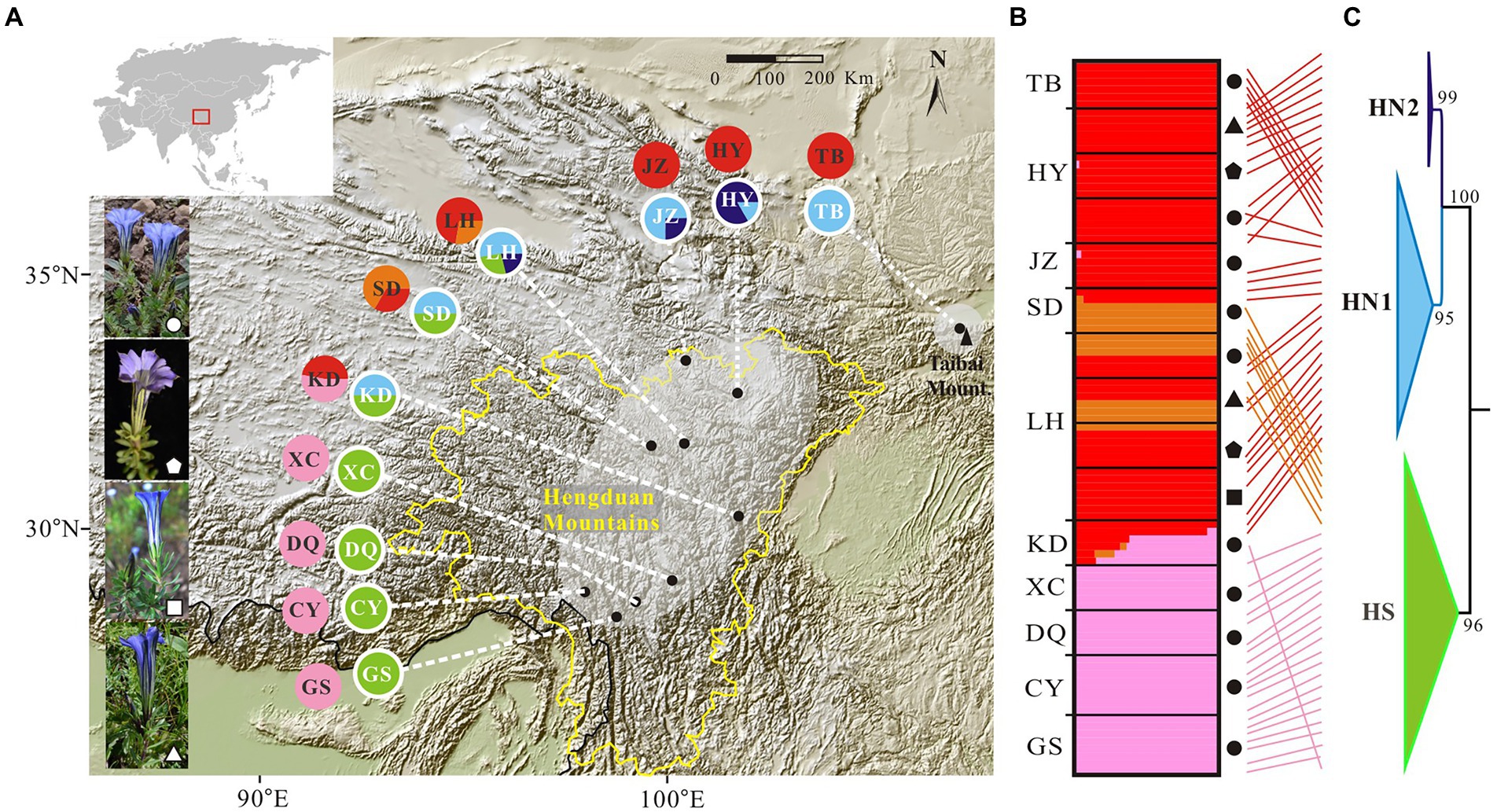
Figure 1. Genetic structure in the Gentiana hexaphylla complex. (A) Pie charts showing frequency of plastid haplotypes (inner circles) and colour-coded groups based on 1,907 SNPs in FastStructure (outer circles) for each sampling site. Black dots represent sampling sites. Map came from the Institute for Planets. (B) Bar plots showing probabilities of ancestral clusters of each sample at K = 3 in FastSTRUCTURE. The sampling site is shown on the left of the bar plot. Dark shapes in panels B,C indicate different species: circles, G. hexaphylla; pentagons, G. viatrix; squares, G. tetraphylla; triangles, and G. ternifolia. (C) Phylogeny of plastid data based on eight plastome fragments. Support values from the maximum likelihood analyses are shown on the nodes. Subclades are named HN1, HN2, and HS. Line connections indicate the same individual in the phylogeny and bar plot. The border of the Hengduan Mountains is indicated with a broken yellow line, and based on Ding et al. (2020). An equal-area projection has been used in the map. White shapes in the photos indicate different species, as used in panels B,C. Photographs: Peng-Cheng Fu.
Library construction, sequencing, and SNP calling
Our genomic sequencing approach aimed to generate many unlinked nuclear SNPs to infer fine-scale population clustering and to estimate genetic divergence, as well as to recover plastid genomic DNA sequence to perform phylogenetic and molecular dating analyses.
Total genomic DNA was extracted from dry leaves using a Dzup plant genomic DNA extraction kit (Sangon, Shanghai, China). DNA concentrations were quantified with a Qubit 2.0 fluorometer (Life Technologies). For RAD library construction and sequencing (Miller et al., 2007), each sample was digested with the restriction enzyme EcoRI followed by the ligation of the P1 adapter by T4 ligase. Fragments were pooled, randomly sheared and size-selected to 350–550 bp. A second adapter (P2) was then ligated. The ligation products were purified and PCR-amplified, followed by gel purification and size selection for fragments in the range of 350–550 bp. Libraries were multiplexed and sequenced using 2 × 150 bp reads generated on the Illumina Novaseq 6,000 (Tianjin, China).
Samples were initially de-multiplexed with the process_radtags script in Stacks 2.0 (Catchen et al., 2011, 2013). Raw reads were filtered and trimmed with Trimmomatic 0.32 (Bolger et al., 2014) with default parameters, to remove adaptor sequences and low-quality reads and sites, and then checked for quality with FastQC 0.11.2. We used Stacks 2.0 (Catchen et al., 2011, 2013) to identify orthologous loci across individuals. Clean sequences were assembled de novo using denovo_map, with a minimum stack depth of three (m = 3), and we tested a range of different mismatches between stacks within and between individuals (M = n = 2, 3 or 4). PCR duplicates were filtered using gstacks following the approach of Rochette et al. (2019). At least 75% of individuals in a population were required to retain a locus (−r 0.75), and SNPs identified in all individuals with a minor allele frequency (MAF) of less than 5% were removed (−min-maf 0.05). SNPs with a missing frequency of less than 50 % among individuals (−max-missing 0.5) were retained using vcftools 0.1.13 (Danecek et al., 2011). Linkage-disequilibrium (LD) SNP pruning was performed in vcftools to excludes variants from closer than 100 bp (−thin 100). Heterogeneous loci were filtered out in TASSEL 5 (Bradbury et al., 2007) to exclude SNPs originating from putative paralogs. We estimated genetic diversity indices including nucleotide diversity (Pi), expected heterozygosity (HE) and observed heterozygosity (HO) using the populations module in Stacks.
To obtain plastid sequences, clean reads were assembled using the GetOrganelle pipeline (Jin et al., 2020) with default parameter. We used the published plastome of G. hexaphylla (MG192305; Sun et al., 2018) as the reference. Contigs longer than 500 bp were mapped back to the plastome of G. hexaphylla in Geneious Basic 5.6.4 (Kearse et al., 2012). Shared plastome regions present in at least one individual per population were extracted, aligned using MAFFT (Katoh et al., 2002) and then concatenated for downstream analyses.
Population genetic structure
To assess the levels of genetic differentiation between populations, we estimated pairwise FST based on nuclear genomic SNPs using the Weir and Cockerham method (Weir and Cockerham, 1984) in vcftools 0.1.13 (Danecek et al., 2011). Pairwise FST values were graphically displayed with the package “pheatmap” using R for Statistical Computing (v. 4.0.1; R Core Team, 2020). Analysis of Molecular Variance (AMOVA) was conducted with GenAlEx 6.5 (Peakall and Smouse, 2006). We tested for IBD (Wright, 1943) by applying a Mantel test using the geographic distance and pairwise genetic distance with zt (Bonnet and Van de Peer, 2002).
For exploring the genetic clusters present within the G. hexaphylla complex, we used a Bayesian clustering method implemented in FastSTRUCTURE (Raj et al., 2014) based on the nuclear SNPs identified above. Following Raj et al. (2014), we used the chooseK.py script to assess model complexity for the data. Graphical representation of individual cluster assignments was performed using DISTRUCT 1.1 (Rosenberg, 2004). As FastSTRUCTURE makes a number of assumptions, such as individuals being in HWE, we also used a non-model-based method in DAPC (Jombart et al., 2010) in the R package “adegenet” to identify genetic clustering. The most likely K value was selected using Bayesian information criterion (BIC; Jombart et al., 2010). Given the strong signal of IBD in our study (see “Results”), we used an additional spatial method implemented in the R package conStruct to infer patterns of genetic structure considering the geographic distance among the sampled populations (Bradburd et al., 2018). This method can be run without spatial information, which will give results similar to other Bayesian admixture approaches, or with spatial information, where it then explicitly accounts for allele frequency differences as expected by IBD, and can therefore reveal discontinuous genetic variation corresponding to barriers to dispersal. We ran a cross-validation analysis with five replicates, comparing the spatial and non-spatial models with K = 1 through 10 for each replicate. Layer contributions were also calculated to interpret cross-validation results. Each training partition (one per replicate) was created by randomly subsampling 90% of the total number of loci and run for 1,000 MCMC iterations.
Phylogenetic inference and divergence time estimation
We constructed a phylogenetic tree based on the nuclear genomic SNPs using maximum likelihood (ML) in IQ-TREE (Nguyen et al., 2015) with 1,000 replicates. To investigate population relationships and model historical migration events, we used TreeMix 1.2 (Pickrell and Pritchard, 2012) using the SNP data. We calculated the percentage of variation explained by the TreeMix analyses with between 0 and 10 migration events using the treemixVarianceExplained scripts in the RADpipe package.1
Using concatenated plastid sequences, ML phylogenetic analyses were conducted with IQ-TREE (Nguyen et al., 2015) implemented in PhyloSuite platform (Zhang et al., 2020) with 1,000 replicates. The substitution model was detected in ModelFinder (Kalyaanamoorthy et al., 2017). Information about outgroup samples is presented in Supplementary Table S1.
We estimated the divergence times of plastid sequences using the Bayesian method implemented in BEAST 2.4 (Drummond et al., 2012; Bouckaert et al., 2014) under the HKY substitution model (as the best model detected in ModelFinder, Kalyaanamoorthy et al., 2017), the Yule model, and the strict clock model. We constrained one of the nodes with the fossil of G. section Cruciata (G. cruciata L. in Germany, Mai & Walther, 1988; the early Miocene, Mai, 2000) following Fu et al. (2021a), namely with lognormal priors with an offset at 16.0 Ma, a mean of 1.0, and a standard deviation of 1.0. Because the fossil record is very limited for gentians, we also employed a secondary calibration approach, constraining the Gentiana crown age with the estimated divergence time from Janssens et al. (2020), using uniform priors with a lower age of 21.25 Ma and an upper age of 38.21 Ma (Fu et al., 2021a). We ran three independent MCMC chains with 10,000,000 generations, sampling every 1,000th generation and discarding the initial 10% as burn-in. Convergence was confirmed in TRACER 1.52 and judged by ESS values (>200). Trees were summarized using TreeAnnotator 1.7.5 (Drummond et al., 2012) and visualized in FigTree 1.4.3
Ancestral range estimation and species distribution modelling
We used the R package ‘BioGeoBEARS’ (Matzke, 2013, 2014) to compare biogeographical models and estimate the evolution of geographic ranges across the phylogeny which we obtained using BEAST. The distribution of the four lineages of G. hexaphylla were coded for their presence/absence in the four biogeographical regions, which were based on the genetic clusters identified above. Dispersal was restricted to adjacent areas and the maximum range size was set to four, which means no extant cluster can occur in more than the four biogeographical regions. We did not specify an outgroup as the aim of our preliminary analyses was to determine dispersal routes below the species level. We compared six models: dispersal-extinction-cladogenesis (DEC; Ree and Smith, 2008), dispersal-vicariance analysis (DIVA; Ronquist, 1997) and BAYAREA models (Landis et al., 2013), plus all three models separately with the possibility of founder events (+j; Matzke, 2013, 2014). As our main objective was to trace ancestral areas rather than to infer the diversification dynamics or the speciation mode, concerns raised by Ree and Sanmartín (2018) on the DEC + J model are unlikely to have significant effects on our results. The best model was selected using the Akaike information criterion (AIC) and the sample size corrected AIC (AICc) after computing the loglikelihood score (Dupin et al., 2016). The probabilities of the ancestral states at all nodes in the phylogeny were estimated using the best model.
Current distribution data for G. hexaphylla included observations made during the course of our fieldwork, and those available in the Global Biodiversity Information Facility (GBIF).4 Records occurring less than 10 km from each other were removed in ArcGIS 10.2 in order to avoid multicollinearity. The 19 bioclimatic variables at present, mid-Holocene (6 kya) and LGM (Last Glacial Maximum, ~22 kya) were obtained from the WorldClim dataset (Hijmans et al., 2005) with a spatial resolution of 2.5 arc-min. To avoid multicollinearity, a Pearson correlation analysis of the 19 variables was conducted using SPSS 20. Highly correlated variables with correlation coefficients significantly larger than 0.8 (p < 0.05) were removed. MaxEnt 3.4.1 (Phillips et al., 2005) was then applied to predict the potential distribution area. We used 75% of the location data for training and the remaining 25% to test the predictive ability of the model. Effectiveness of the model was evaluated using the receiver operating characteristic (ROC) and the area under the ROC curve (AUC) > 0.9.
Results
Data preprocessing and SNP calling
Illumina sequencing of RAD libraries produced an average of 1.83 × 107 reads per sample. After quality filtering the number of reads retained per sample varied from 4.45 × 106 to 8.07 × 107, with a median value of 1.81 × 107 (Supplementary Table S2). A total of 202,861, 229,804, and 257,315 SNPs were called with the three different Stacks parameter settings of M = n set to 2, 3, and 4, respectively, suggesting broadly similar number of SNPs are recovered regardless of the number of mismatches allowed within (M) or between (n) individuals. After filtering for MAF, LD, missing data and heterogeneous loci, the total number of unlinked SNPs obtained with Stacks for all samples was 1,988, 1,875, and 1,907 when M = n was 2, 3, and 4, respectively.
Population genetic structure and genetic divergence
We firstly used the data set of 1,907 SNPs (m = 3, M = n = 4) to infer population genetic structure in the G. hexaphylla complex. chooseK.py indicated that the marginal likelihood scores from FastSTRUCTURE analyses peaked at K = 3 (Supplementary Figure S1). Generally, the inferred genetic groups were consistent with known geographic barriers present between populations (Figure 1). The northern genetic cluster (TB, JZ, and HY) occurs from the Qionglai Mountains to Mount Taibai and the southern genetic cluster (XC, DQ, GS and CY) from the Shaluli Mountains to Gaoligong Mountains (coloured red and pink, respectively; Figures 1A,B). Populations LH, SD, and KD in the Daxue Mountains in the central HM, was composed of two genetic clusters, and populations LH and SD also formed another genetic cluster (indicated in orange in Figure 1). DAPC analyses suggested an optimal clustering value of K = 3 (Supplementary Figure S2), with population groupings corresponding to their geographic location (Figure 2). None of the four study species consistently formed a separate cluster in both FastSTRUCTURE and DAPC analyses. The other two sets of SNPs (1,988 and 1,875 SNPs) gave almost identical results to the dataset of 1,907 SNPs (Supplementary Figure S1, S2), suggesting these inferences are robust to the parameters in Stacks used to assemble reads into loci, thus we performed all downstream analyses on this dataset.
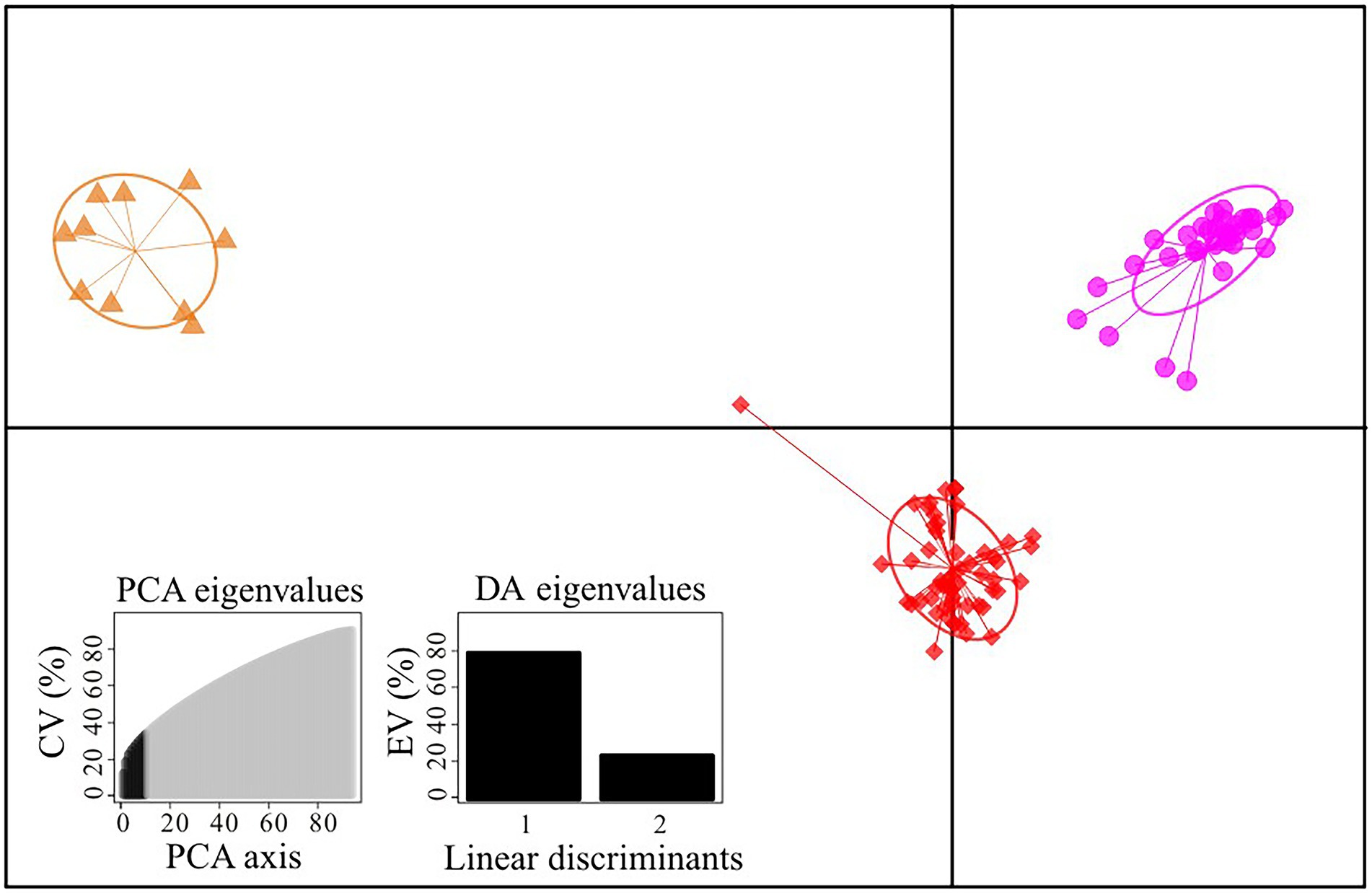
Figure 2. Scatterplot of DAPC analysis in the Gentiana hexaphylla complex using 1,907 SNPs. Clusters and inertia ellipses are shown in different colours, consistent with Figure 1. Each dot represents an individual. Insets show histograms of discriminant analysis eigenvalues. CV, cumulated variance; EV, explained variance.
Mantel tests between genetic and geographical distance showed a significant correlation (R = 0.49, p = 0.0012), indicating strong IBD. The cross-validation analyses in conStruct indicated that the spatial models always had higher predictive accuracy than non-spatial models, with little increase in accuracy when K was greater than three (Supplementary Figure S3). Comparing parametric covariance contributions of each model, layers larger than three generally contributed little to overall covariance across the replicates (Supplementary Figure S3), and are therefore unlikely to be of biological importance. We thus chose three layers for further characterization. In this model, spatial analyses showed genetic clusters separating the northern and southern populations (Figure 3), supporting divergence caused by barriers to dispersal rather than simply IBD in the G. hexaphylla complex.
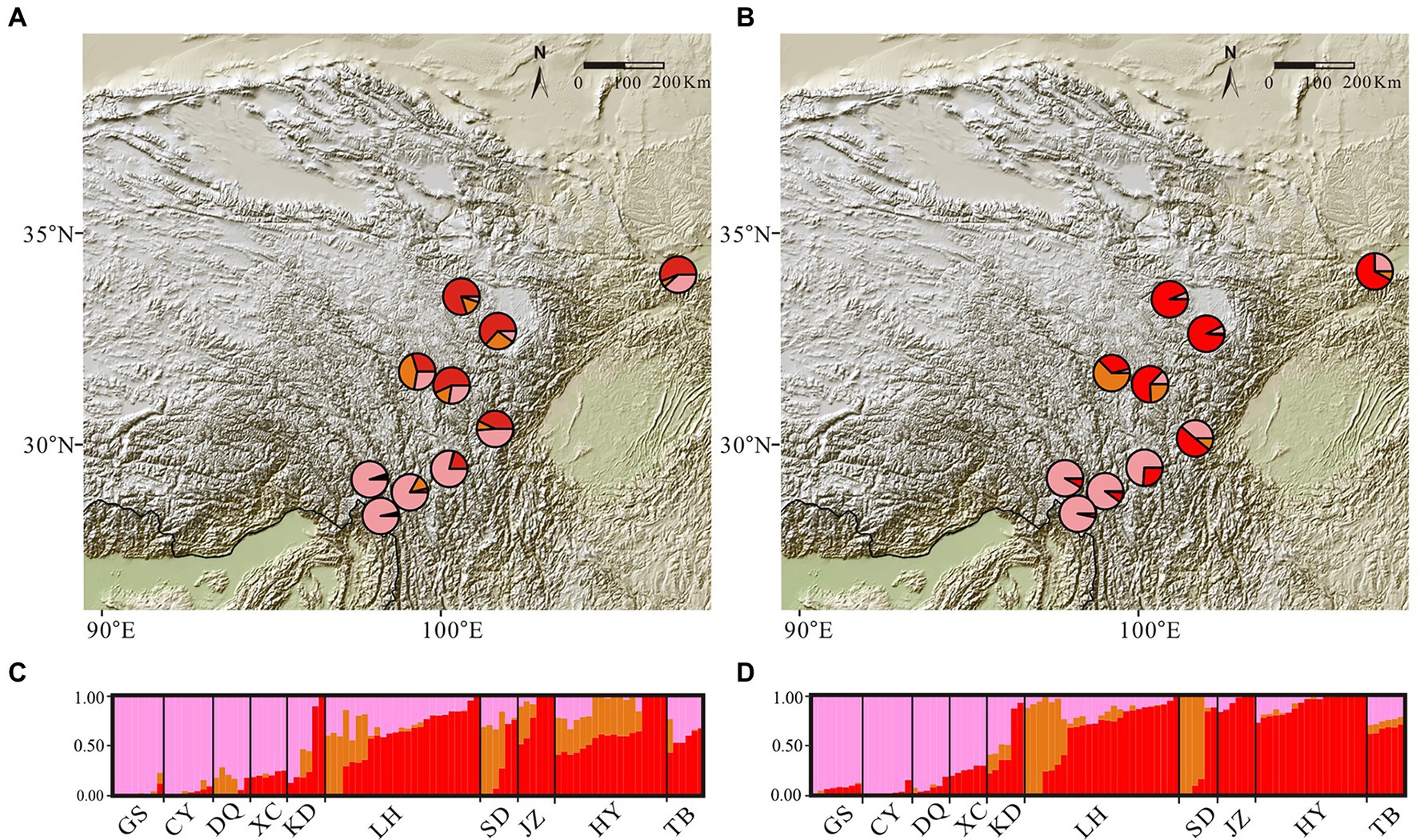
Figure 3. Maps of admixture proportions and bar plots for the Gentiana hexaphylla complex inferred using conStruct spatial and non-spatial analyses, using a K-value of three. Pies show mean admixture proportions across individuals from sampling sites inferred using spatial (panel A) and non-spatial (panel B) models. Admixture bar plots show genetic clustering in spatial (panel C) and non-spatial (panel D) models. Each bar represents an individual. The sampling sites are labelled at the bottom of the bar plots.
The southern populations (XC, DQ, GS, and CY) had higher values of pairwise FST (0.259–0.541) than the northern populations (−0.056–0.320; Figure 4A; Supplementary Table S3). Population (TB) in Mount Taibai had lower values of pairwise FST with the northern than with the southern populations, and the population KD in the Central HM had low values of pairwise FST with both the southern and the northern populations. Populations from the South HM generally had lower nucleotide diversity than other regions (Table 1). Genetic variance mainly occurred among populations (56%) and within populations (37%) rather than among the South, Central and North regions (7%).
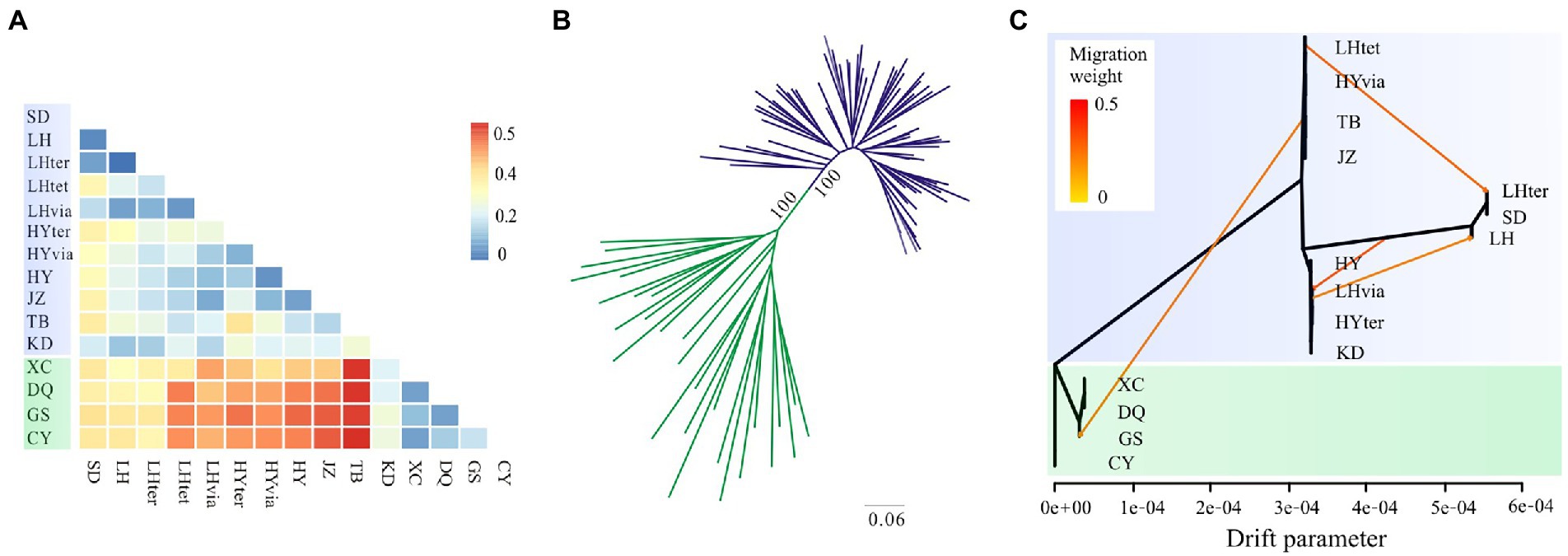
Figure 4. Genetic differentiation and phylogenetic relationships based on genomic SNPs in the Gentiana hexaphylla complex. (A) Heatmap of Weir and Cockerham’s FST between each pair of populations. (B) Unrooted maximum likelihood tree. (C) TreeMix graph showing population splits, and migration edges with migration weights indicated by the colour. The x-axis is the drift parameter reflecting the amount of genetic drift that has occurred between populations and the assumed common ancestral population. Locations in the South HM are showed in green and locations in other regions in blue.
Phylogenetic inference and estimation of divergence time
Using genomic SNPs, the ML tree showed that individuals from the North and the South were assigned to two distinct clades with full support (Figure 4B). None of the species in the G. hexaphylla complex formed a monophyletic lineage. The TreeMix analysis also showed that the southern populations were a distinct clade with populations placed on a long branch (Figure 4C). When no migration was allowed, the variance explained was high (99.17%), indicating a simple model of divergence without migration is a good fit to populations of the G. hexaphylla complex. Adding migration events in the phylogeny produced a marginally better fit (Supplementary Figure S4), and showed some potential historical dispersal events across the northern and central populations as well as to the southern populations (Figure 4C).
Mapping contigs from each sample to the plastome of G. hexaphylla generated eight regions shared among 62 individuals, which covered all populations, and these were concatenated for downstream analyses. The eight aligned regions ranged from 374 bp to 798 bp in length and the aligned concatenated sequences were 4,341 bp. The ML tree indicated that the G. hexaphylla complex had two highly supported clades corresponding to the South (HS) and the North (HN), respectively (Figure 1C; Supplementary Figure S5). The HN clade was further divided into two subclades (HN1 and HN2) with high support. Comparing genetic clustering based on genomic SNPs and plastid data showed that samples in the same group from the south and the north were included in the HS and the HN clade, respectively, while the samples in a distinct clade from the central HM were included in the HN clade (Figures 1B,C; Supplementary Table S4). Bayesian inference in BEAST supported the two subclades in the North HM (HN1 and HN2) and two subclades in the South HM (HS and KD; Supplementary Figure S6). More than one lineage co-occurs at the Central and the North HM (Figure 1A).
Our divergence time analyses based on plastid sequences (Supplementary Figure S6) showed that the HN and HS clades in G. hexaphylla diverged in the Pliocene, approximately 4.01 Ma (95% HPD: 3.04–5.06 Ma). The two subclades in the HN and HS diverged in the Early Pleistocene 1.71 Ma (95% HPD: 1.09–2.38 Ma) and in the Late Pliocene 3.10 Ma (95% HPD: 2.28–3.88 Ma), respectively.
Ancestral range estimation and palaeo-distributional reconstruction
Estimation of the evolution of geographic range in BioGeoBEARS indicated that the BAYAREA model was the best fit to the G. hexaphylla complex, as it received the largest LnL value and the lowest AIC and AICc scores (Table 2). The DEC model also gave a very similar evolutionary scenario to the BAYAREA model. Based on the probability of each estimated ancestral range, the earliest common ancestor of the four lineages in G. hexaphylla complex might have occurred in the central HM (the green area, b) around 4 Ma, implying that the ancestral lineage dispersed out of this area southward and northward independently, which gave rise to different sub-lineages (Figure 5).
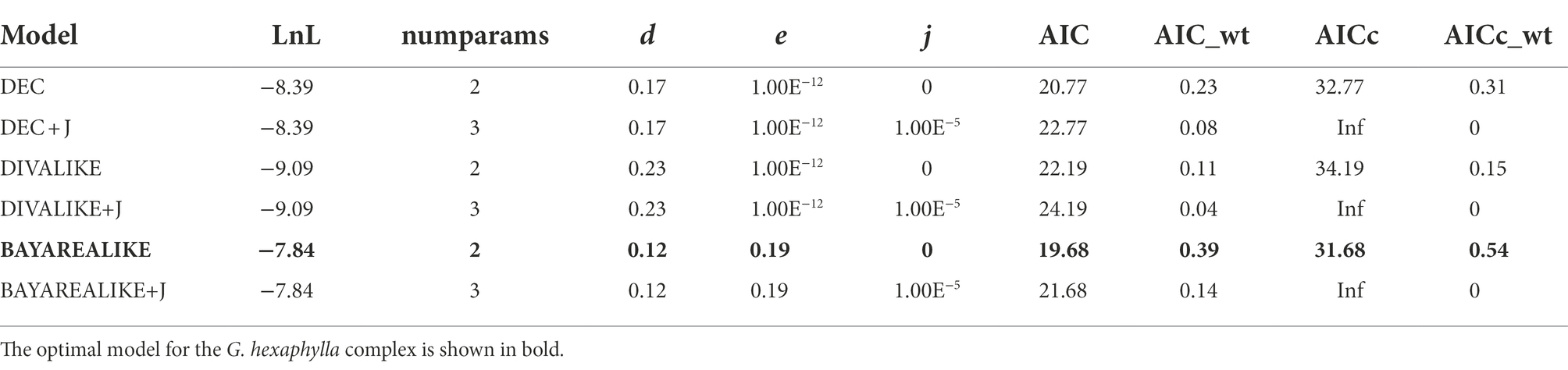
Table 2. Model comparison and parameters (d, dispersal; e, extinction; j, founder speciation) of ancestral area estimation of the Gentiana hexaphylla complex based on BioGeoBEARS.
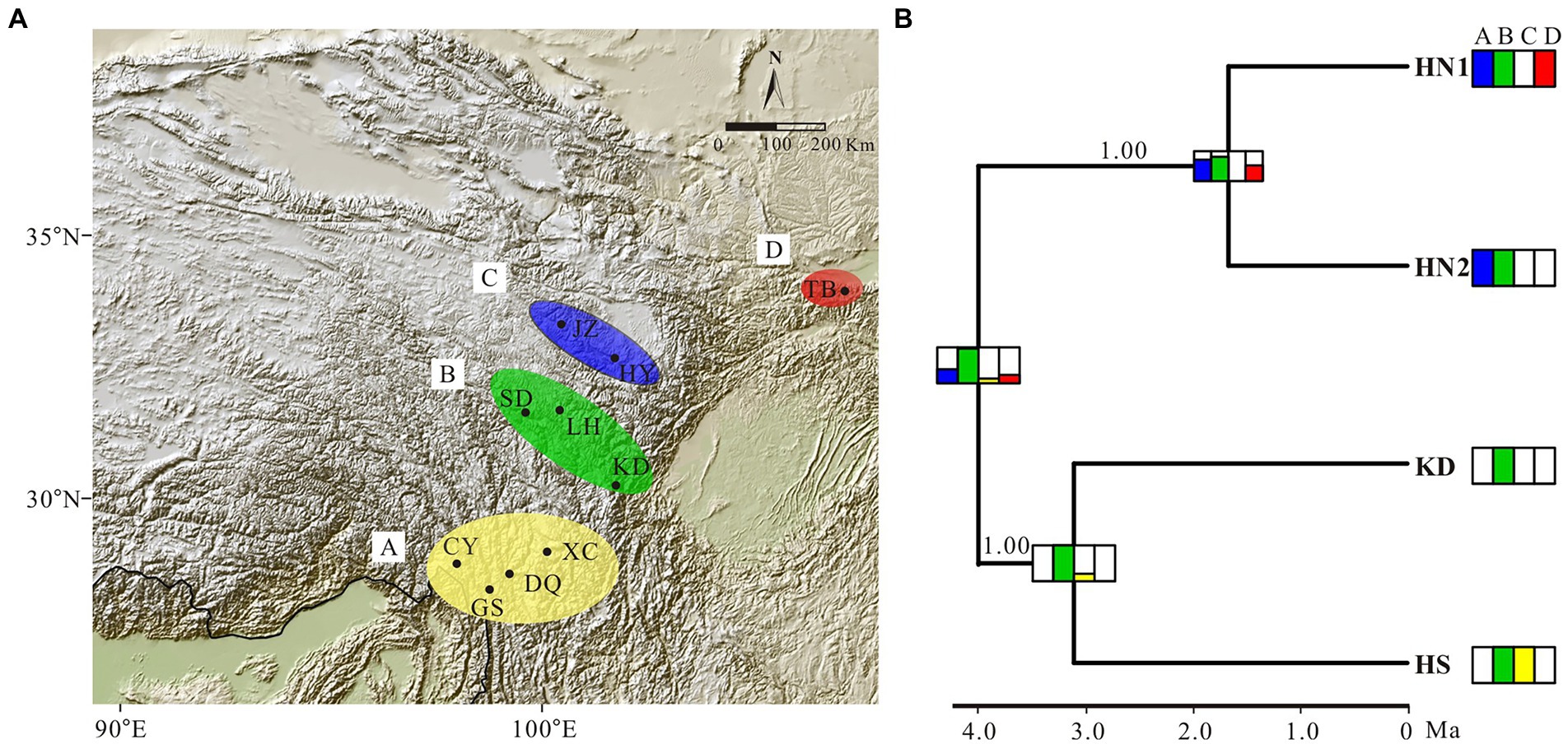
Figure 5. Ancestral area estimation of the Gentiana hexaphylla complex based on BioGeoBEARS. (A) Area definition for ancestral area estimation, based on the extant distribution of the G. hexaphylla complex. (B) Ancestral range estimation based on BAYAREALIKE model implemented in BioGeoBEARS based on the result from BEAST. Extant species distributions used for the ancestral area estimations are provided in coloured boxes next to each lineage. Boxes on each node represent the cumulative probabilities for the estimated ancestral range. Phylogenetic support values for Bayesian inference are shown on branches.
After the Pearson correlation analysis, nine bioclimatic variables (bio1–bio4, bio7, bio12–bio15) were kept for distributional reconstruction. The palaeo-distributional reconstruction showed that during the LGM the potential habitat of G. hexaphylla was restricted to the Himalayas and the South to East of the HM (Figure 6). Afterwards, from the LGM to today, its range significantly expanded into the HM, but experienced a slight contraction in the Northeast.
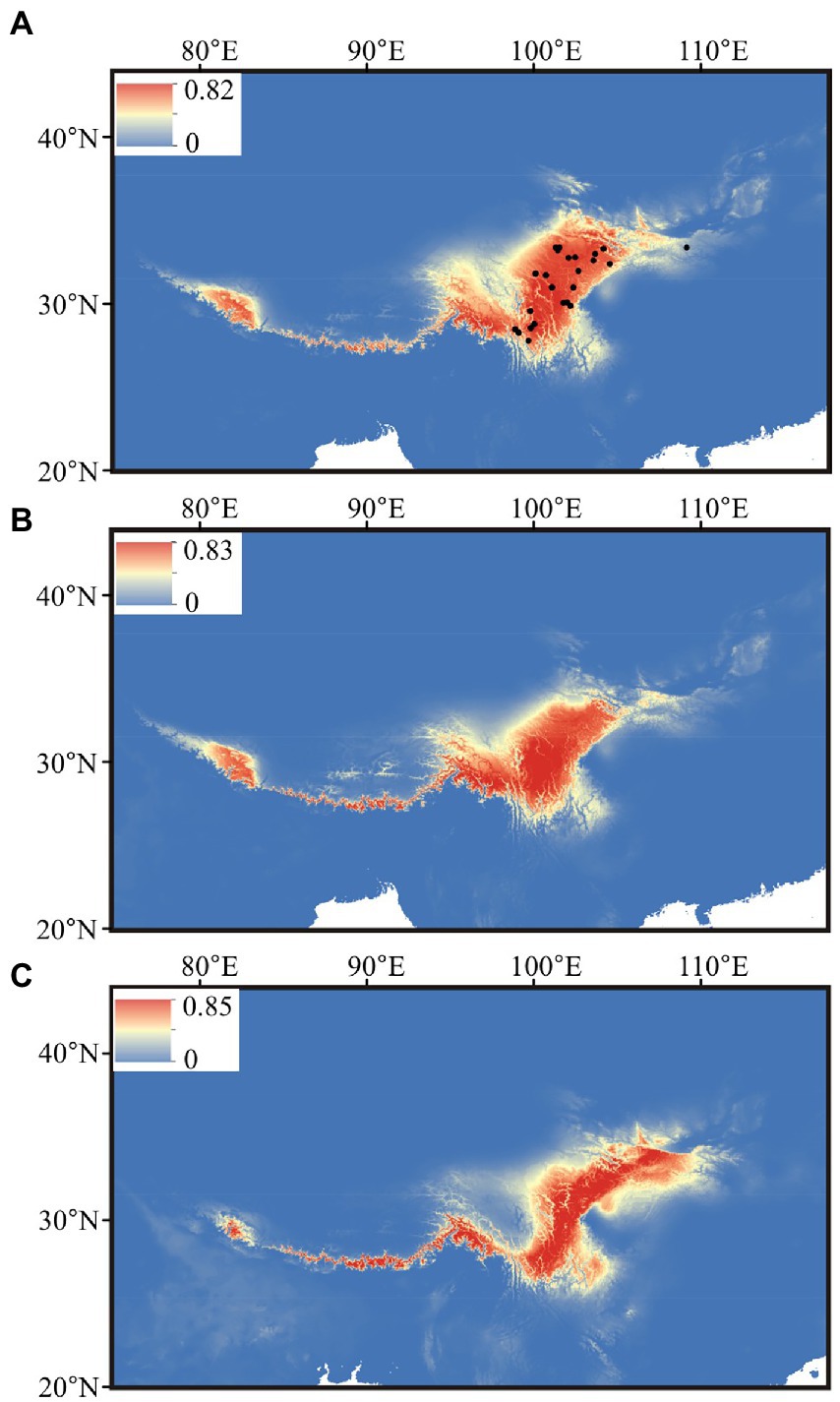
Figure 6. Estimated climatic niches for Gentiana hexaphylla in the Qinghai-Tibetan Plateau. Maps are shown at (A) present, (B) mid-Holocene (~6 kya), and (C) LGM (~22 kya). The value of predicted habitat suitability is indicated by the bars in each panel. Current distribution records are shown in the present map by black dots.
Discussion
As the longest continuously existing alpine flora (Ding et al., 2020), the HM alpine flora offers an excellent opportunity to explore spatial–temporal changes in the distribution ranges of species. Such studies can test how range changes may have been driven by geological or climatic modifications and resulted in divergence, speciation and ultimately diversification. Using genomic data for a common Gentian species, we detected deep genetic divergence corresponding to geological barriers in the HM, with divergence likely promoted by both mountain uplift and climatic fluctuations. While we detected divergence in populations spread across the landscape, there was notably high genetic similarity between populations in Mount Taibai and the North HM, indicating a connection between the alpine flora of the Qinling Mts and the HM. Here, we discuss our results in terms of the biogeographic history of this important hotspot for alpine species, and consider the implications for future studies.
Strong geographic genetic differentiation between the North and South HM
The HM is well known for its extraordinary diversity and high rate of in situ alpine speciation (Sun et al., 2017; Xing and Ree, 2017; Ding et al., 2020). However, how genetic subdivision of populations corresponds to major geological features within the HM and surrounding areas remains to be characterized in detail. To address this issue, studies of fine-scale population divergence across this region are necessary, but they have been hampered by the poor resolution offered by traditional markers and/or by the complex evolutionary history of this region (Qiu et al., 2011; Liu et al., 2012; Muellner-Riehl, 2019). Using genomic data, our results clearly show that deep genetic differentiation in the G. hexaphylla complex occurs between the North and South HM, two areas which are geographically separated by the Daxue Mountains. Although we detected a significant pattern of IBD, once geographic distance is accounted for, we confirmed that there are clearly defined geographic genetic clusters rather than structure corresponding to a cline (Twyford et al., 2020). Our genomic data showed much clearer genetic structure and geographical divergence in the G. hexaphylla complex than previous work on the same species complex using one plastid fragment but with denser population sampling (Fu et al., 2020b). Recently, other genomic studies in plants of the HM, including Pinus armandii (Liu et al., 2019) and the Rheum palmatum complex (Feng et al., 2020), have also found similar results. Together with other studies (e.g., Dufresnes et al., 2020; Marková et al., 2020), these results show how genomic data can resolve complex and potentially cryptic genetic patterns, even in topographically complex settings.
The deeply dissected landscape of the HM is expected to generate numerous barriers to gene flow and thus to promote genetic differentiation and speciation. This was supported by the high FST value in our study, as well as the high variance explained by the TreeMix model without migration, as well as by the presence of private haplotypes in previous work (Fu et al., 2020b). Our finding of a North–South divide in the HM is in line with others studies, for example in Pinus armandii (Liu et al., 2019) and the Rheum palmatum complex (Feng et al., 2020). In addition, changes in species richness and composition can be observed across this zone (Zhang et al., 2009a). Thus, the Daxue Mountains, which create a North–South divide, is the primary geological barrier for this species complex. To the contrary, our study did not detect the Nu River—the notorious Salween-Mekong divide isolating the Gaoligong Mountains (around population GS in this study) from the rest of the HM—as a barrier to gene flow, as has been previously found in yew trees (Liu et al., 2013). Taken together, our analyses as well as previous work show geological features in the HM create significant barriers to gene flow and lead to discrete population genetic structure, but the specific patterns are likely to be idiosyncratic to different biomes and taxa.
In addition, by sequencing typical individuals of each species in the G. hexaphylla complex, our genomic data show that all samples of species co-occurring with G. hexaphylla cluster together based on their geographical origin rather than their morphological traits (taxonomic attribution). Thus, our results not only point to geographical structuring, but also highlight the need for taxonomic clarification in this species complex.
Divergence correlates with uplift and climate change
The timing of geological events leading to the current topological conformation in the HM is still debated (Favre et al., 2015; Spicer et al., 2020), but most studies agree that at least some parts of the HM (in the east) have experienced local uplift in the HM during the Late Miocene to the Pliocene (Favre et al., 2015; Ding et al., 2020), and that this caused in situ diversification of many alpine groups (Xing and Ree, 2017; Muellner-Riehl et al., 2019; Ye et al., 2019). However, other studies suggest that the extent of Pleistocene climate fluctuation are a key factor causing divergence, rather than geological processes (Muellner-Riehl, 2019). The divergence between the two main lineages in the G. hexaphylla complex dated to the Pliocene, and correlated with the uplift of the Daxue Mountains, including Mt. Gonggar (7,556 m above sea level, a.s.l.), which may then have acted as barrier to dispersal. This is likely to be similar to Pinus armandii (Liu et al., 2019). Our ancestral area estimation also indicated that the G. hexaphylla complex originated in the central HM and then dispersed northward and southward, suggesting that the species occurred in the region of the Daxue Mountains and then experienced divergence associated with mountain uplift. However, climatic oscillations lead to variable connectivity among sky-islands in mountain systems, as previously shown in the HM (Deng et al., 2020) and considered in the Flickering Connectivity System’ proposed for the Andean flora (Flantua et al., 2019). Through vertical displacement as climate oscillates, some areas may be characterized by cycles of extinction and colonization, while other areas may be colonized anew. Thus, the dispersal from the central HM to other regions, as well as differentiation in isolation in each of these regions, may have been caused by climate oscillations. Therefore, the G. hexaphylla complex may bear the marks of a species-pump effect, as predicted by the Mountain-Geobiodiversity Hypothesis (Mosbrugger et al., 2018).
Colonization from the HM to Mount Taibai
The G. hexaphylla complex is distributed across two biogeographically disjunct regions, namely the HM and the QM. The QM provides a natural boundary between northern and southern China, and served as a geographical and ecological barrier for species with low dispersal ability (Yan et al., 2010; Hu et al., 2021). Its isolation also promoted the divergence of some relict species (Shahzad et al., 2020). Mount Taibai, belonging to the QM, is the highest peak (3,500 m a.s.l.) in Central and East China and sits more than 400 km northeast of the HM. At its highest elevation it harbors an alpine flora including several Gentiana species (Ho and Pringle, 1995), for example the endemic G. apiata N.E. Brown (Ho and Liu, 2001). Our species distribution modelling showed that this region may have been suitable for G. hexaphylla since the LGM. As Mount Taibai was glaciated during the LGM (Rost, 1994; Zhang et al., 2016), G. hexaphylla individuals now occurring in this region are likely to be the result of uphill migration as glaciation receded. This colonization scenario would be consistent with climate change from cold and humid to warm and dry from 18 kya to present in the QM (Zhao et al., 2014). This inference is also supported by our pairwise FST values and the genetic clustering results, which both showed less genetic differentiation between population TB and the northern populations of the HM. This result indicates that G. hexaphylla is likely to have colonized the QM from the North HM. To our knowledge, our study is the first to show the dispersal directionality between the QM and the North HM, although at the genus level, Gentiana is known to match the out-of-Tibet hypothesis (Favre et al., 2016). Although more case studies are needed to evaluate the relative role of the different modes of assembly of the alpine biome in the QM, our results do improve our understanding of how Gentiana, and likely other alpine lineages, may have colonized these mountains.
Conclusion
Using genomic data, this study recovered deep differentiation between populations of the G. hexaphylla complex along two sides of the Daxue Mountains in the Central HM, from where the complex originated. Divergence is likely to have been driven by a combination of mountain uplift, climatic fluctuations and geographical isolation. We also found that the QM were colonized from the HM by G. hexaphylla relatively recently, probably aided by changes in climatic conditions.
Data availability statement
The data presented in the study are deposited in Dryad (doi: 10.5061/dryad.0gb5mkm08).
Author contributions
P-CF collected the samples, analyzed the data, and wrote the manuscript. S-SS did lab work and prepared the tables and figures. PH and AF revised the manuscript. S-LC collected the samples. AT guided the analysis and revised the manuscript. All authors contributed to the article and approved the submitted version.
Funding
This study was supported by the National Natural Science Foundation of China (grant no. 31600296) and Chinese Scholarship Council to P-CF. AF was supported by the German Science Foundation (Deutsche Forschungsgemeinschaft) project no. FA1117/1–2.
Acknowledgments
We thank Yan-Qian Ding of the University of Edinburgh for the suggestions for running conStruct, and Henan Research Center of Wetland Restoration in the Middle and Lower Reaches of the Yellow River for sample collection.
Conflict of interest
The authors declare that the research was conducted in the absence of any commercial or financial relationships that could be construed as a potential conflict of interest.
Publisher’s note
All claims expressed in this article are solely those of the authors and do not necessarily represent those of their affiliated organizations, or those of the publisher, the editors and the reviewers. Any product that may be evaluated in this article, or claim that may be made by its manufacturer, is not guaranteed or endorsed by the publisher.
Supplementary material
The Supplementary material for this article can be found online at: https://www.frontiersin.org/articles/10.3389/fpls.2022.936761/full#supplementary-material
Footnotes
1. ^https://github.com/darencard/RADpipe
2. ^http://tree.bio.ed.ac.uk/software/tracer/
References
Antonelli, A., Kissling, W. D., Flantua, S. G. A., Bermudez, M. A., Mulch, A., Muellner-Riehl, A. N., et al. (2018). Geological and climatic influences on mountain biodiversity. Nat. Geosci. 11, 718–725. doi: 10.1038/s41561-018-0236-z
Bolger, A. M., Lohse, M., and Usadel, B. (2014). Trimmomatic: a flexible trimmer for Illumina sequence data. Bioinformatics 30, 2114–2120. doi: 10.1093/bioinformatics/btu170
Bonnet, E., and Van de Peer, Y. (2002). Zt: A sofware tool for simple and partial mantel tests. J. Statist. Softw. 7, 1–12. doi: 10.18637/jss.v007.i10
Bouckaert, R., Heled, J., Kühnert, D., Vaughan, T., Wu, C. H., Xie, D., et al. (2014). BEAST 2: a software platform for Bayesian evolutionary analysis. PLoS Comput. Biol. 10:e1003537. doi: 10.1371/journal.pcbi.1003537
Bradburd, G. S., Coop, G. M., and Ralph, P. L. (2018). Inferring continuous and discrete population genetic structure across space. Genetics 210, 33–52. doi: 10.1534/genetics.118.301333
Bradbury, P. J., Zhang, Z., Kroon, D. E., Casstevens, T. M., Ramdoss, Y., and Buckler, E. S. (2007). TASSEL: software for association mapping of complex traits in diverse samples. Bioinformatics 23, 2633–2635. doi: 10.1093/bioinformatics/btm308
Catchen, J., Hohenlohe, P. A., Bassham, S., Amores, A., and Cresko, W. A. (2013). Stacks: an analysis tool set for population genomics. Mol. Ecol. 22, 3124–3140. doi: 10.1111/mec.12354
Catchen, J. M., Amores, A., Hohenlohe, P., Cresko, W., and Postlethwait, J. H. (2011). Stacks: building and genotyping loci de novo from short-read sequences. G3: Genes, genomes, genetics 1, 171–182. doi: 10.1534/g3.111.000240
Danecek, P., Auton, A., Abecasis, G., Albers, C. A., Banks, E., DePristo, M. A., et al. (2011). The variant call format and VCFtools. Bioinformatics 27, 2156–2158. doi: 10.1093/bioinformatics/btr330
Deng, J. Y., Fu, R. H., Compton, S. G., Liu, M., Wang, Q., Yuan, C., et al. (2020). Sky islands as foci for divergence of fig trees and their pollinators in Southwest China. Mol. Ecol. 29, 762–782. doi: 10.1111/mec.15353
Ding, W. N., Ree, R. H., Spicer, R. A., and Xing, Y. W. (2020). Ancient orogenic and monsoon-driven assembly of the world’s richest temperate alpine flora. Science 369, 578–581. doi: 10.1126/science.abb4484
Drummond, A. J., Suchard, M. A., Xie, D., and Rambaut, A. (2012). Bayesian phylogenetics with BEAUti and the BEAST 1.7. Mol.Biolo. Evol. 29, 1969–1973. doi: 10.1093/molbev/mss075
Dufresnes, C., Nicieza, A. G., Litvinchuk, S. N., Rodrigues, N., Jeffries, D. L., Vences, M., et al. (2020). Are glacial refugia hotspots of speciation and cytonuclear discordances? Answers from the genomic phylogeography of Spanish common frogs. Mol. Ecol. 29, 986–1000. doi: 10.1111/mec.15368
Dupin, J., Matzke, N. J., Särkinen, T., Knapp, S., Olmstead, R. G., Bohs, L., et al. (2016). Bayesian estimation of the global biogeographical history of the Solanaceae. J. Biogeogr. 44, 887–899. doi: 10.1111/jbi.12898
Favre, A., Michalak, I., Chen, C. H., Wang, J. C., Pringle, J. S., Matuszak, S., et al. (2016). Out-of-Tibet: the spatio-temporal evolution of Gentiana (Gentianaceae). J. Biogeogr. 43, 1967–1978. doi: 10.1111/jbi.12840
Favre, A., Päckert, M., Pauls, S. U., Jähnig, S. C., Uhl, D., Michalak, I., et al. (2015). The role of the uplift of the Qinghai-Tibetan plateau for the evolution of Tibetan biotas. Biol. Rev. 90, 236–253. doi: 10.1111/brv.12107
Favre, A., Pringle, J. S., Heckenhauer, J., Kozuharova, E., Gao, Q., Lemmon, E. M., et al. (2020). Phylogenetic relationships and sectional delineation within Gentiana (Gentianaceae). Taxon 69, 1221–1238. doi: 10.1002/tax.12405
Feng, L., Ruhsam, M., Wang, Y. H., Li, Z. H., and Wang, X. M. (2020). Using demographic model selection to untangle allopatric divergence and diversification mechanisms in the Rheum palmatum complex in the eastern Asiatic region. Mol. Ecol. 29, 1791–1805. doi: 10.1111/mec.15448
Flantua, S. G., O’Dea, A., Onstein, R. E., Giraldo, C., and Hooghiemstra, H. (2019). The flickering connectivity system of the north Andean páramos. J. Biogeogr. 46, 1808–1825. doi: 10.1111/jbi.13607
Fu, P. C., Sun, S. S., Khan, G., Dong, X. X., Tan, J. Z., Favre, A., et al. (2020a). Population subdivision and hybridization in a species complex of Gentiana in the Qinghai-Tibetan plateau. Ann. Bot. 125, 677–690. doi: 10.1093/aob/mcaa003
Fu, P. C., Sun, S. S., Twyford, A. D., Li, B. B., Zhou, R. Q., Chen, S. L., et al. (2021a). Lineage-specific plastid degradation in subtribe Gentianinae (Gentianaceae). Ecol. Evol. 11, 3286–3299. doi: 10.1002/ece3.7281
Fu, P. C., Tan, J. Z., Wang, H. Y., and Sun, S. S. (2020b). Genetic divergence and demographic history of Gentiana hexaphylla Maximowicz ex Kusnezow (Gentianaceae) complex in Hengduan Mountains. Plant Sci. J. 38, 390–399. doi: 10.11913/PSJ.2095-0837.2020.30390
Fu, P. C., Twyford, A. D., Sun, S. S., Wang, H. Y., Xia, M. Z., Tan, C. X., et al. (2021b). Recurrent hybridization underlies the evolution of novelty in Gentiana (Gentianaceae) in the Qinghai-Tibetan plateau. AoB Plants 13:plaa068. doi: 10.1093/aobpla/plaa068
Fu, P. C., Ya, H. Y., Liu, Q. W., Cai, H. M., and Chen, S. L. (2018). Out of refugia: population genetic structure and evolutionary history of the alpine medicinal plant Gentiana lawrencei var. farreri (Gentianaceae). Front. Genet. 9:564. doi: 10.3389/fgene.2018.00564
Hijmans, R. J., Cameron, S. E., Parra, J. L., Jones, P. G., and Jarvis, A. (2005). Very high resolution interpolated climate surfaces for global land areas. Int. J. Climatol. 25, 1965–1978. doi: 10.1002/joc.1276
Ho, T. N., and Pringle, J. S. (1995). “Gentianaceae” in Flora of China. eds. Z. Y. Wu and P. H. Raven, Vol. 16 (Beijing: Science press, St. Louis: Missouri botanical garden), 1–140.
Hu, C., Yuan, S., Sun, W., Chen, W., Liu, W., Li, P., et al. (2021). Spatial genetic structure and demographic history of the wild boar in the Qinling Mountains, China. Animals 11:346. doi: 10.3390/ani11020346
Janssens, S. B., Couvreur, T. L. P., Mertens, A., Dauby, G., Dagallier, L. M. J., Abeele, S. V., et al. (2020). A large-scale species level dated angiosperm phylogeny for evolutionary and ecological analyses. Biodivers Data J. 8:e39677. doi: 10.3897/BDJ.8.e39677
Jin, J. J., Yu, W. B., Yang, J. B., Song, Y., DePamphilis, C. W., Yi, T. S., et al. (2020). GetOrganelle: a fast and versatile toolkit for accurate de novo assembly of organelle genomes. Gen. Biol. 21, 241–231. doi: 10.1186/s13059-020-02154-5
Jombart, T., Devillard, S., and Balloux, F. (2010). Discriminant analysis of principal components: a new method for the analysis of genetically structured populations. BMC Genet. 11:94. doi: 10.1186/1471-2156-11-94
Kalyaanamoorthy, S., Minh, B. Q., Wong, T. K., von Haeseler, A., and Jermiin, L. S. (2017). ModelFinder: fast model selection for accurate phylogenetic estimates. Nat. Methods 14, 587–589. doi: 10.1038/nmeth.4285
Katoh, K., Misawa, K., Kuma, K. I., and Miyata, T. (2002). MAFFT: A novel method for rapid multiple sequence alignment based on fast Fourier transform. Nucleic Acids Res. 30, 3059–3066. doi: 10.1093/nar/gkf436
Kearse, M., Moir, R., Wilson, A., Stones-Havas, S., Cheung, M., Sturrock, S., et al. (2012). Geneious basic: An integrated and extendable desktop software platform for the organization and analysis of sequence data. Bioinformatics 28, 1647–1649. doi: 10.1093/bioinformatics/bts199
Kirschner, P., Záveská, E., Gamisch, A., Hilpold, A., Trucchi, E., Paun, O., et al. (2020). Long-term isolation of European steppe outposts boosts the biome’s conservation value. Nat. Commun. 11:1968. doi: 10.1038/s41467-020-15620-2
Landis, M. J., Matzke, N. J., Moore, B. R., and Huelsenbeck, J. P. (2013). Bayesian analysis of biogeography when the number of areas is large. Syst. Biol. 62, 789–804. doi: 10.1093/sysbio/syt040
Li, Q., Sun, H., Boufford, D. E., Bartholomew, B., Fritsch, P. W., Chen, J. H., et al. (2021). Grade of membership models reveal geographical and environmental correlates of floristic structure in a temperate biodiversity hotspot. New Phytol. 232, 1424–1435. doi: 10.1111/nph.17443
Liu, J., Möller, M., Provan, J., Gao, L. M., Poudel, R. C., and Li, D. Z. (2013). Geological and ecological factors drive cryptic speciation of yews in a biodiversity hotspot. New Phytol. 199, 1093–1108. doi: 10.1111/nph.12336
Liu, J. Q., Duan, Y. W., Hao, G., Ge, X. J., and Sun, H. (2014). Evolutionary history and underlying adaptation of alpine plants on the Qinghai–Tibet Plateau. J. Syst. Evol. 52, 241–249. doi: 10.1111/jse.12094
Liu, J. Q., Sun, Y. S., Ge, X. J., Gao, L. M., and Qiu, Y. X. (2012). Phylogeographic studies of plants in China: advances in the past and directions in the future. J. Syst. Evol. 50, 267–275. doi: 10.1111/j.1759-6831.2012.00214.x
Liu, Y. Y., Jin, W. T., Wei, X. X., and Wang, X. Q. (2019). Cryptic speciation in the Chinese white pine (Pinus armandii): implications for the high species diversity of conifers in the Hengduan Mountains, a global biodiversity hotspot. Mol. Phylogenet. Evol. 138, 114–125. doi: 10.1016/j.ympev.2019.05.015
Lu, Z., Chen, P., Bai, X., Xu, J., He, X., Niu, Z., et al. (2015). Initial diversification, glacial survival, and continuous range expansion of Gentiana straminea (Gentianaceae) in the Qinghai–Tibet plateau. Biochem. Syst. Ecol. 62, 219–228. doi: 10.1016/j.bse.2015.09.005
Mai, D. H. (2000). Die mittelmiozänen und obermiozänen Floren aus der Meuroer und Raunoer Folge in der Lausitz. Teil I: Farnpflanzen, Koniferen und Monokotyledonen. Palaeontographica, Abteilung B, Pälaophytologie 256, 1–68. doi: 10.1127/palb/256/2000/1
Mai, D. H., and Walther, H. (1988). Die pliozaenen Floren von Thüringen, Deutsche Demokratische Republik. Quartaerpalaeontologie 7, 55–297.
Marchese, C. (2015). Biodiversity hotspots: A shortcut for a more complicated concept. Glob. Ecol. Conserv. 3, 297–309. doi: 10.1016/j.gecco.2014.12.008
Marková, S., Horníková, M., Lanier, H. C., Henttonen, H., Searle, J. B., Weider, L. J., et al. (2020). High genomic diversity in the bank vole at the northern apex of a range expansion: the role of multiple colonizations and end-glacial refugia. Mol. Ecol. 29, 1730–1744. doi: 10.1111/mec.15427
Matzke, N. J. (2013). BioGeoBEARS: biogeography with Bayesian (and Likelihood) evolutionary analysis in R scripts R package, version 0.2.1. Available at: http://CRAN.R-project.org/package=BioGeoBEARS (accessed 27 May 2020).
Matzke, N. J. (2014). Model selection in historical biogeography reveals that founder-event speciation is a crucial process in island clades. Syst. Biol. 63, 951–970. doi: 10.1093/sysbio/syu056
Meirmans, P. G. (2012). The trouble with isolation by distance. Mol. Ecol. 21, 2839–2846. doi: 10.1111/j.1365-294X.2012.05578.x
Miller, M. R., Dunham, J. P., Amores, A., Cresko, W. A., and Johnson, E. A. (2007). Rapid and cost-effective polymorphism identification and genotyping using restriction site associated DNA (RAD) markers. Genome Res. 17, 240–248. doi: 10.1101/gr.5681207
Mosbrugger, V., Favre, A., Muellner-Riehl, A. N., Päckert, M., and Mulch, A. (2018). “Cenozoic evolution of geo-biodiversity in the Tibeto-Himalayan region,” in Mountains, climate, and biodiversity. Hoboken: Wiley eds. C. Hoorn, A. Perrigo, and A. Antonelli.
Muellner-Riehl, A. N. (2019). Mountains as evolutionary arenas: patterns, emerging approaches, paradigm shifts, and their implications for plant phylogeographic research in the Tibeto-Himalayan region. Front. Plant Sci. 10, 195. doi: 10.3389/fpls.2019.00195
Muellner-Riehl, A. N., and Favre, A. (2021). Mountain biogeography coming full circle: a new ‘3D’ floristic approach provides units for reconstructing evolutionary trajectories. New Phytol. 232, 964–966. doi: 10.1111/nph.17645
Muellner-Riehl, A. N., Schnitzler, J., Kissling, W. D., Mosbrugger, V., Rijsdijk, K. F., Seijmonsbergen, A. C., et al. (2019). Origins of global mountain plant biodiversity: testing the ‘mountain-geobiodiversity hypothesis’. J. Biogeogr. 46, 2826–2838. doi: 10.1111/jbi.13715
Myers, N., Mittermeier, R. A., Mittermeier, C. G., Da Fonseca, G. A., and Kent, J. (2000). Biodiversity hotspots for conservation priorities. Nature 403, 853–858. doi: 10.1038/35002501
Nguyen, L. T., Schmidt, H. A., Von Haeseler, A., and Minh, B. Q. (2015). IQ-TREE: a fast and effective stochastic algorithm for estimating maximum-likelihood phylogenies. Mol. Biol. Evol. 32, 268–274. doi: 10.1093/molbev/msu300
Peakall, R. O. D., and Smouse, P. E. (2006). GENALEX 6: genetic analysis in excel. Population genetic software for teaching and research. Mol. Ecol. Notes 6, 288–295. doi: 10.1111/j.1471-8286.2005.01155.x
Perez, M. F., Franco, F. F., Bombonato, J. R., Bonatelli, I. A., Khan, G., Romeiro-Brito, M., et al. (2018). Assessing population structure in the face of isolation by distance: are we neglecting the problem? Divers. Distrib. 24, 1883–1889. doi: 10.1111/ddi.12816
Phillips, S. J., Dudík, M., and Schapire, R. E. (2005). Maxent software for modeling species niches and distributions (version 3.4.1). Available at: http://biodiversityinformatics.amnh.org/open_source/maxent/ (accessed on 2020-8-17).
Pickrell, J., and Pritchard, J. (2012). Inference of population splits and mixtures from genome-wide allele frequency data. Nature Precedings 1:1. doi: 10.1038/npre.2012.6956.1
Qiu, Y. X., Fu, C. X., and Comes, H. P. (2011). Plant molecular phylogeography in China and adjacent regions: tracing the genetic imprints of quaternary climate and environmental change in the world’s most diverse temperate flora. Mol. Phylogenet. Evol. 59, 225–244. doi: 10.1016/j.ympev.2011.01.012
R Core Team (2020). R: A Language and Environment for Statistical Computing. R Foundation for Statistical Computing, Vienna, Austria.
Raj, A., Stephens, M., and Pritchard, J. K. (2014). fastSTRUCTURE: variational inference of population structure in large SNP data sets. Genetics 197, 573–589. doi: 10.1534/genetics.114.164350
Ree, R. H., and Sanmartín, I. (2018). Conceptual and statistical problems with the DEC +J model of founder-event speciation and its comparison with DEC via model selection. J. Biogeogr. 45, 741–749. doi: 10.1111/jbi.13173
Ree, R. H., and Smith, S. A. (2008). Maximum likelihood inference of geographic range evolution by dispersal, local extinction, and cladogenesis. Syst. Biol. 57, 4–14. doi: 10.1080/10635150701883881
Rochette, N. C., Rivera-Colón, A. G., and Catchen, J. M. (2019). Stacks 2: analytical methods for paired-end sequencing improve RADseq-based population genomics. Mol. Ecol. 28, 4737–4754. doi: 10.1111/mec.15253
Ronquist, F. (1997). Dispersal-vicariance analysis: a new approach to the quantification of historical biogeography. Syst. Biol. 46, 195–203. doi: 10.1093/sysbio/46.1.195
Rosenberg, N. E. (2004). DISTRUCT: a program for the graphical display of population structure. Mol. Ecol. Notes 4, 137–138. doi: 10.1046/j.1471-8286.2003.00566.x
Rost, K. T. (1994). Paleoclimatic field studies in and along the Qinling Shan (Central China). GeoJournal 34, 107–120. doi: 10.1007/BF00813974
Scherrer, D., and Körner, C. (2011). Topographically controlled thermal-habitat differentiation buffers alpine plant diversity against climate warming. J. Biogeogr. 38, 406–416. doi: 10.1111/j.1365-2699.2010.02407.x
Shahzad, K., Liu, M. L., Zhao, Y. H., Zhang, T. T., Liu, J. N., and Li, Z. H. (2020). Evolutionary history of endangered and relict tree species Dipteronia sinensis in response to geological and climatic events in the Qinling Mountains and adjacent areas. Ecol. Evol. 10, 14052–14066. doi: 10.1002/ece3.6996
Spicer, R. A., Farnsworth, A., and Su, T. (2020). Cenozoic topography, monsoons and biodiversity conservation within the Tibetan region: An evolving story. Plant Diversity 42, 229–254. doi: 10.1016/j.pld.2020.06.011
Sun, H., Zhang, J., Deng, T., and Boufford, D. E. (2017). Origins and evolution of plant diversity in the Hengduan Mountains, China. Plant Diversity 39, 161–166. doi: 10.1016/j.pld.2017.09.004
Sun, S. S., Fu, P. C., Zhou, X. J., Cheng, Y. W., Zhang, F. Q., Chen, S. L., et al. (2018). The complete plastome sequences of seven species in Gentiana sect. Kudoa (Gentianaceae): insights into plastid gene loss and molecular evolution. Front. Plant Sci. 9:493. doi: 10.3389/fpls.2018.00493
Twyford, A. D., Wong, E. L. Y., and Friedman, J. (2020). Multi-level patterns of genetic structure and isolation by distance in the widespread plant Mimulus guttatus. Heredity 125, 227–239. doi: 10.1038/s41437-020-0335-7
Weir, B. S., and Cockerham, C. C. (1984). Estimating F-statistics for the analysis of population structure. Evolution 38, 1358–1370. doi: 10.1111/j.1558-5646.1984.tb05657.x
Wen, J., Zhang, J., Nie, Z. L., Zhong, Y., and Sun, H. (2014). Evolutionary diversifications of plants on the Qinghai-Tibetan plateau. Front. Genet. 5, 4. doi: 10.3389/fgene.2014.00004
Xing, Y., and Ree, R. H. (2017). Uplift-driven diversification in the Hengduan Mountains, a temperate biodiversity hotspot. P. Natl. A. Sci. 114, E3444–E3451. doi: 10.1073/pnas.1616063114
Xue, J., He, J., Wang, L., Gao, J., and Wu, Y. (2018). Plant traits and biomass allocation of Gentiana hexaphylla on different slope aspects at the eastern margin of Qinghai-Tibet plateau. Appl. Ecol. Env. Res. 16, 1835–1853. doi: 10.15666/aeer/1602_18351853
Yan, J., Wang, Q., Chang, Q., Ji, X., and Zhou, K. (2010). The divergence of two independent lineages of an endemic Chinese gecko, Gekko swinhonis, launched by the Qinling orogenic belt. Mol. Ecol. 19, 2490–2500. doi: 10.1111/j.1365-294X.2010.04660.x
Ye, X. Y., Ma, P. F., Yang, G. Q., Guo, C., Zhang, Y. X., Chen, Y. M., et al. (2019). Rapid diversification of alpine bamboos associated with the uplift of the Hengduan Mountains. J. Biogeogr. 46, 2678–2689. doi: 10.1111/jbi.13723
Yu, H., Miao, S., Xie, G., Guo, X., Chen, Z., and Favre, A. (2020). Contrasting floristic diversity of the Hengduan Mountains, the Himalayas and the Qinghai-Tibet plateau sensu stricto in China. Front. Ecol. Evol. 8:136. doi: 10.3389/fevo.2020.00136
Zhang, D., Gao, F., Jakovlić, I., Zou, H., Zhang, J., Li, W. X., et al. (2020). PhyloSuite: an integrated and scalable desktop platform for streamlined molecular sequence data management and evolutionary phylogenetics studies. Mol. Ecol. Resour. 20, 348–355. doi: 10.1111/1755-0998.13096
Zhang, D. C., Boufford, D. E., Ree, R. H., and Sun, H. (2009a). The 29 N latitudinal line: an important division in the Hengduan Mountains, a biodiversity hotspot in Southwest China. Nord. J. Bot. 27, 405–412. doi: 10.1111/j.1756-1051.2008.00235.x
Zhang, W., Liu, L., Chen, Y., Liu, B., Harbor, J. M., Cui, Z., et al. (2016). Late glacial 10Be ages for glacial landforms in the upper region of the Taibai glaciation in the Qinling Mountain range, China. J. Asian Earth Sci. 115, 383–392. doi: 10.1016/j.jseaes.2015.10.011
Zhang, X. L., Wang, Y. J., Ge, X. J., Yuan, Y. M., Yang, H. L., and Liu, J. Q. (2009b). Molecular phylogeny and biogeography of Gentiana sect. Cruciata (Gentianaceae) based on four plastid DNA datasets. Taxon 58, 862–870. doi: 10.1002/tax.583014
Zhang, X. L., Yuan, Y. M., and Ge, X. J. (2007). Genetic structure and differentiation of Gentiana atuntsiensis WW Smith and G. striolata TN Ho (Gentianaceae) as revealed by ISSR markers. Bot. J. Linn. Soc. 154, 225–232. doi: 10.1111/j.1095-8339.2007.00635.x
Keywords: ancestral range estimation, Gentiana hexaphylla, Hengduan Mountains, isolation by distance, plastid, nuclear SNPs
Citation: Fu P-C, Sun S-S, Hollingsworth PM, Chen S-L, Favre A and Twyford AD (2022) Population genomics reveal deep divergence and strong geographical structure in gentians in the Hengduan Mountains. Front. Plant Sci. 13:936761. doi: 10.3389/fpls.2022.936761
Edited by:
Shu-Miaw Chaw, Biodiversity Research Center, Academia Sinica, TaiwanReviewed by:
Sara V. Good, University of Winnipeg, CanadaJuan Carlos Villarreal A., Laval University, Canada
Copyright © 2022 Fu, Sun, Hollingsworth, Chen, Favre and Twyford. This is an open-access article distributed under the terms of the Creative Commons Attribution License (CC BY). The use, distribution or reproduction in other forums is permitted, provided the original author(s) and the copyright owner(s) are credited and that the original publication in this journal is cited, in accordance with accepted academic practice. No use, distribution or reproduction is permitted which does not comply with these terms.
*Correspondence: Peng-Cheng Fu, ZnVwZW5nY0BzaW5hLmNvbQ==; Alex D. Twyford, QWxleC5Ud3lmb3JkQGVkLmFjLnVr