- 1College of Agriculture, Nanjing Agricultural University, Nanjing, China
- 2National Engineering and Technology Center for Information Agriculture, Nanjing, China
- 3Engineering Research Center of Smart Agriculture, Ministry of Education, Nanjing, China
- 4Collaborative Innovation Center for Modern Crop Production Co-sponsored by Province and Ministry, Nanjing, China
- 5College of Artificial Intelligence, Nanjing Agricultural University, Nanjing, China
Introduction
Weeds in wheat fields compete with wheat for light, water, fertilizer, and growth space, and therefore are one of the main biohazards that limit the yield and quality formation of wheat (Shiferaw et al., 2013; Singh et al., 2013). Obtaining weed species and location information quickly and accurately is the first step in precise weed control. Existing methods for detecting weeds in wheat fields based on machine learning are highly dependent on the scale and quality of datasets. In particular, deep learning methods usually require massive training samples. However, Multi-modal and Multi-View Image of weed datasets in natural wheat field are very rare currently.
Existing public weed datasets in crop field are shown in Table 1. Compared with other crops such as cotton and sugar beet, the plant space for wheat crop is smaller. Therefore, the background in wheat field is more complex, and the labeling process is more difficult. In addition, the existing weed datasets still have the following drawbacks. First, the number of samples is small, which is difficult to meet the requirements of deep learning. The mode of information is very single and limited. The existing datasets are constructed based on RGB images, which cannot provide a more complete feature space for weed detection. Especially detecting weeds in wheat field, the features extracted from RGB images are easy to detect broadleaf weeds, but it is difficult to detect weeds with similar appearance to wheat. Studies have shown that because of growth competition, height information is an important parameter to discriminate wheat from weeds (Fahad et al., 2015; Xu et al., 2020), and the fusion of RGB image features and height features has become a new method used to improve the efficiency of weed detection. Finally, the existing datasets are mostly based on vertical views for image acquisition (Wu et al., 2021), which is difficult to be applied to weed detection under a complex field background. Leaf overlap and occlusion will have a great impact on detection.
Therefore, we proposed Multi-modal and Multi-view Image Dataset for Weeds Detection in Wheat Field (MMIDDWF) that can be used for deep learning. The dataset contains wheat, broad-leaf weed, and grass weed images of two modes and nine views, and aims to provide a public weed dataset to promote the development of weed detection methods in wheat field.
Value of the data
• A multimodal image dataset will be provided for weed detection in open wheat field, including an RGB image and a depth image of the same scene. Compared with a single RGB image, a depth image can provide three-dimensional structure features for weed detection in wheat field, which is helpful to solve the problem of detecting grass weeds.
• The dataset also contains multi-view images. Images from nine views can provide a more complete feature space for weed detection in open wheat field, thus helping to solve weed detection problems under a complex background such as leaf occlusion and overlapping.
Materials and methods
Experiment design and image acquisition
Experiments on wheat and weeds were carried out from December 2017 to April 2021 at the demonstration base of the National Engineering and Technology Center for Information Agriculture in Rugao county, Nantong City, Jiangsu province, China. Weeds were not controlled during field management, and seeds of six weed species commonly associated with wheat were randomly sown to simulate weed growth in the open field. Alopecurus aequalis, Poa annua, Bromus japonicus, and E. crusgalli are grass weeds; Amaranthus retroflexus and C. bursa-pastoris are broad-leaf weeds; species composition was similar to that of actual weed species in wheat fields.
The data were collected at the peak of weed occurrence in wheat fields, i.e., in the tillering and jointing stages. The acquisition equipment is shown in Figure 1. The RGB and depth images were acquired using Intel® RealSense™ Depth Camera D415 (Integrated Electronics Corporation, Santa Clara, CA, United States), an RGB-D camera that adopts active infrared stereo vision technology. Infrared stereo cameras generate depth images, and the color sensor generates RGB images, both with a resolution of 1,280 × 720.
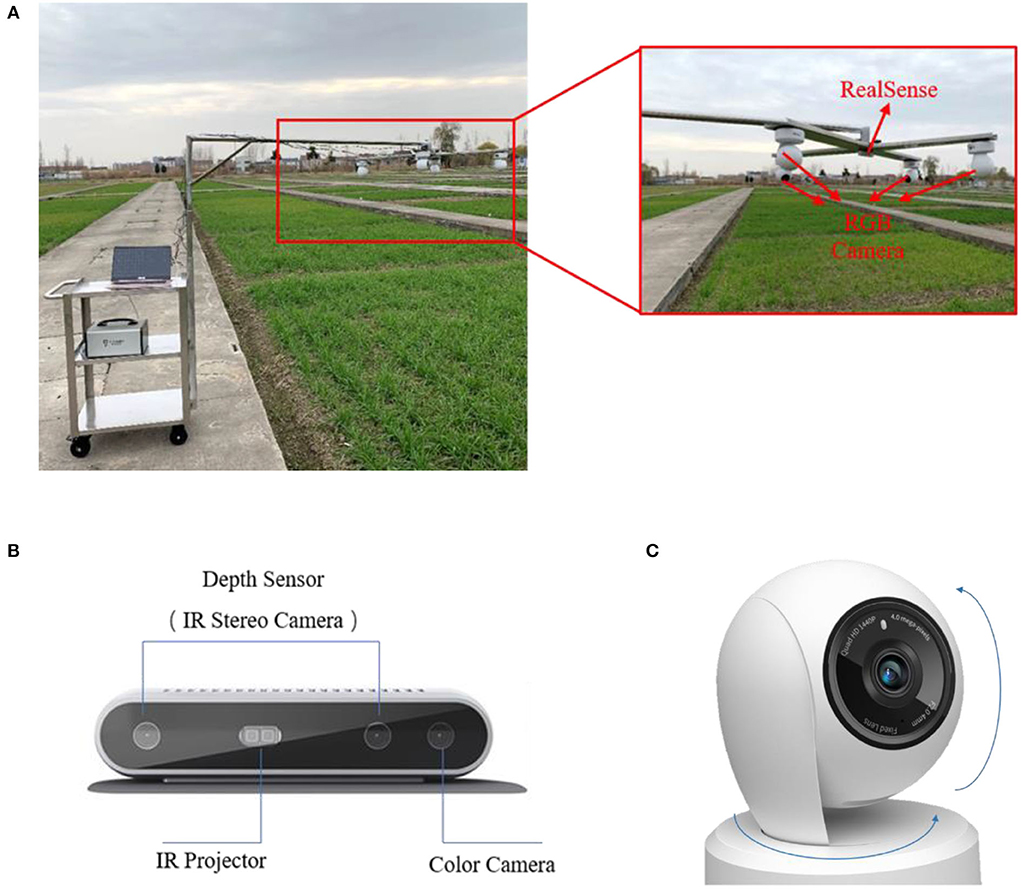
Figure 1. (A) Image acquisition equipment, (B) Intel® RealSense™ Depth Camera D415, and (C) TL-IPC44AN-4camera.
RGB and depth field images under natural conditions were obtained during the wheat tillering and jointing stages. Multi-view images were collected with TL-IPC44AN-4(TP-Link Corporation, Shenzhen, China) cameras in four positions at angles of 15 and 30° horizontally. Image collection was conducted from 9 a.m. to 4 p.m. under clear and windless weather conditions. The camera was 70 cm above the crop canopy. Images were transmitted to a computer in real time via USB 3.0.
Image annotation and dataset production
The original depth information is less representative. In particular, feature extraction from depth images with a convolutional neural network generates feature maps of distance rather than geometric structures with physical significance. Therefore, single-channel depth images were transformed to three-channel images by re-encoding the original images to make them more representative and structurally similar to RGB images (Gupta et al., 2014). The three channels of re-encoded images are phase, height above ground, and angle with gravity, and re-encoded images are referred to as PHA images. For the image re-encoding method, refer to Xu et al. (2021). Therefore, the multimodal image dataset in MMIDDWF includes three parts: RGB images, single-channel depth images corresponding to RGB images, and PHA images obtained by recoding depth images. In MMIDDWF, each type includes 1,288 images measuring 500 × 500 pixels. The multi-view image dataset contains 692 images, including 79 RGB images from vertical view, 79 vertical depth images corresponding to vertical view RGB images, and 534 images from eight other views. The details of the dataset are shown in Table 2. LabelImg is employed to annotate broadleaf and grass weeds in images as shown in Figure 2A, and the annotation information of RGB images corresponds to that of depth and PHA images. Figure 2B shows the detection results of weeds in wheat fields based on the multimodal dataset (Xu et al., 2021), which achieved precise detection results in an open wheat field by proposing a dual-channel convolutional neural network and fusing multimodal information.
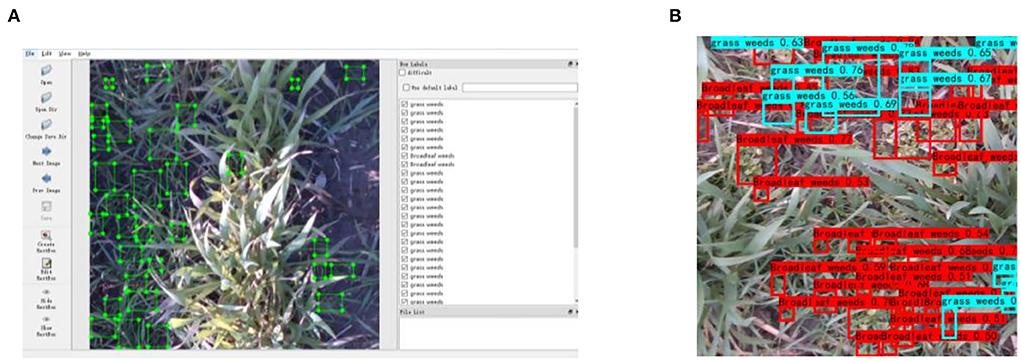
Figure 2. (A) Labeling of grass and broadleaf weeds in wheat fields using LabelImg and (B) weed detection result in wheat field.
Data availability statement
The datasets presented in this study can be found in online repositories. The names of the repository/repositories and accession number(s) can be found below: https://github.com/cocococoxu/MMDWWF.
Author contributions
JN, YZ, KX, and WC designed the research. KX, ZJ, QL, and QX conducted the experiment. KX and ZJ analyzed the data and wrote the manuscript. All authors have read and approved the final version of the manuscript.
Funding
This study was supported by the National Natural Science Foundation of China (Grant No: 31871524), Modern Agricultural Machinery Equipment and Technology Demonstration and Promotion of Jiangsu Province (Grant No: NJ2021-58), Primary Research and Development Plan of Jiangsu Province of China (Grant No: BE2021304), and Six Talent Peaks Project in Jiangsu Province (Grant No: XYDXX-049).
Conflict of interest
The authors declare that the research was conducted in the absence of any commercial or financial relationships that could be construed as a potential conflict of interest.
Publisher's note
All claims expressed in this article are solely those of the authors and do not necessarily represent those of their affiliated organizations, or those of the publisher, the editors and the reviewers. Any product that may be evaluated in this article, or claim that may be made by its manufacturer, is not guaranteed or endorsed by the publisher.
References
Champ, J., Mora-Fallas, A., Goëau, H., Mata-Montero, E., Bonnet, P., and Joly, A. (2020). Instance segmentation for the fine detection of crop and weed plants by precision agricultural robots. Appl. Plant Sci. 8, e11373. doi: 10.1002/aps3.11373
Chebrolu, N., Lottes, P., and Schaefer, Winterhalter, Burgard Stachniss. (2017). Agricultural robot dataset for plant classification, localization and mapping on sugar beet fields. Int. J. Robot Res. 36, 1045–1052. doi: 10.1177/0278364917720510
Coleman, G. (2021). 20201014 - Cobbity Wheat BFLY. Weed-AI. Available online at: https://weed-ai.sydney.edu.au/datasets/73468c19-b098-406a-86fa-df172caaec16.
Espejo-Garcia, B., Mylonas, N., Athanasakos, L., Fountas, S., and Vasilakoglou, I. (2020). Towards weeds identification assistance through transfer learning. Comput. Electron. Agric. 171, 105306. doi: 10.1016/j.compag.2020.105306
Fahad, S., Hussain, S., Chauhan, B. S., Saud, S., Wu, C., Hassan, S., et al. (2015). Weed growth and crop yield loss in wheat as influenced by row spacing and weed emergence times. Crop Protect. 71, 101–108. doi: 10.1016/j.cropro.2015.02.005
Giselsson, T. M., Jørgensen, R. N., Jensen, P. K., Dyrmann, M., and Midtiby, H. S. (2017). A public image database for benchmark of plant seedling classification algorithms. arXiv. 1711.05458.
Gupta, S., Girshick, R., Arbelaez, P., and Malik, J. (2014). “Learning rich features from RGB-D images for object detection and segmentation,” 13th European Conference on Computer Vision (ECCV). Zurich, Switzerland (Cham: Springer). p. 345–360.
Haug, S., and Ostermann, J. (2015). “A crop/weed field image dataset for the evaluation of computer vision based precision agriculture tasks,” 13th European Conference on Computer Vision (ECCV). Zurich, Switzerland (Cham: Springer). p.105–116.
Lameski, P., Zdravevski, E., Trajkovik, V., and Kulakov, A. (2017). “Weed detection dataset with RG, images taken under variable light conditions,” International Conference on IC, Innovations. (Cham: Springer). p.112–119.
Olsen, A., Konovalov, D. A., Philippa, B., Ridd, P., Wood, J. C., Johns, J., et al. (2019). deepweeds: a multiclass weed species image dataset for deep learning. Sci. Rep. 9. doi: 10.1038/s41598-018-38343-3
Rayner, G. (2022). RadishWheatDataset. Weed-AI. Available online at: https://weed-ai.sydney.edu.au/datasets/8b8f134f-ede4-4792-b1f7-d38fc05d8127.
Salazar-Gomez, A., Darbyshire, M., Gao, J., Sklar, E. I., and Parsons, S. (2021). Towards practical object detection for weed spraying in precision agriculture. arXiv. 2109.11048. doi: 10.48550/arXiv.2109.11048
Shiferaw, B., Smale, M., Braun, H. J., Duveiller, E., and Muricho, G. (2013). Crops that feed the world 10. Past successes and future challenges to the role played by wheat in global food security. Food Sec. 5. doi: 10.1007/s12571-013-0263-y
Singh, V., Singha, H., and Raghubanshi, A. S. (2013). Competitive interactions of wheat with Phalaris minor or Rumex dentatus: a replacement series study. Pans Pest Articles News Summaries. 59, 245–258. doi: 10.1080/09670874.2013.845320
Sudars, K., Jasko, J., Namatevs, I., Ozola, L., and Badaukis, N. (2020). Dataset of annotated food crops and weed images for robotic computer vision control. Data in Brief. 31, 105833. doi: 10.1016/j.dib.2020.105833
Vo Hoang, T., Gwang-Hyun, Y., Dang Thanh, V., and Jin-Young, K. (2020). Late fusion of multimodal deep neural networks for weeds classification. Comput. Electron. Agric. 175. doi: 10.1016/j.compag.2020.105506
Wu, Z., Chen, Y., Zhao, B., Kang, X., and Ding, Y. (2021). Review of weed detection methods based on computer vision. Sensors. 21, 3647. doi: 10.3390/s21113647
Xu, K., Li, H., Cao, W., Zhu, Y., and Ni, J. (2020). Recognition of Weeds in Wheat Fields Based on the Fusion of RGB Images and Depth Images. IEEE Access. 1–1. doi: 10.1109/ACCESS.2020.3001999
Keywords: multi-modal image, multi-view image, grass weeds detection, wheat field, machine learning, deep learning
Citation: Xu K, Jiang Z, Liu Q, Xie Q, Zhu Y, Cao W and Ni J (2022) Multi-modal and multi-view image dataset for weeds detection in wheat field. Front. Plant Sci. 13:936748. doi: 10.3389/fpls.2022.936748
Received: 05 May 2022; Accepted: 20 July 2022;
Published: 22 August 2022.
Edited by:
Yuzhen Lu, Mississippi State University, United StatesReviewed by:
Borja Espejo-Garca, Agricultural University of Athens, GreeceFengying Dang, Michigan State University, United States
Chetan Badgujar, Kansas State University, United States
Copyright © 2022 Xu, Jiang, Liu, Xie, Zhu, Cao and Ni. This is an open-access article distributed under the terms of the Creative Commons Attribution License (CC BY). The use, distribution or reproduction in other forums is permitted, provided the original author(s) and the copyright owner(s) are credited and that the original publication in this journal is cited, in accordance with accepted academic practice. No use, distribution or reproduction is permitted which does not comply with these terms.
*Correspondence: Jun Ni, bmlqdW5AbmphdS5lZHUuY24=
†These authors have contributed equally to this work and share first authorship