- 1Key Laboratory for Plant Diversity and Biogeography of East Asia, Kunming Institute of Botany, Chinese Academy of Sciences, Kunming, China
- 2Department of Ecosystem Science and Management, Pennsylvania State University, University Park, PA, United States
- 3Institute of Botany, University of Basel, Basel, Switzerland
- 4N. Gene Solution of Natural Innovation, Kathmandu, Nepal
- 5School of Development Studies, Lumbini Buddhist University, Devdaha, Nepal
- 6MICD, Faculty of Humanities and Social Science, Mid-West University, Lalitpur, Nepal
- 7Yunnan Key Laboratory for Integrative Conservation of Plant Species with Extremely Small Populations, Kunming Institute of Botany, Chinese Academy of Sciences, Kunming, China
Alpine plants’ distribution is being pushed higher towards mountaintops due to global warming, finally diminishing their range and thereby increasing the risk of extinction. Plants with specialized ‘glasshouse’ structures have adapted well to harsh alpine environments, notably to the extremely low temperatures, which makes them vulnerable to global warming. However, their response to global warming is quite unexplored. Therefore, by compiling occurrences and several environmental strata, we utilized multiple ensemble species distribution modeling (eSDM) to estimate the historical, present-day, and future distribution of two alpine ‘glasshouse’ species Rheum nobile Hook. f. & Thomson and R. alexandrae Batalin. Rheum nobile was predicted to extend its distribution from the Eastern Himalaya (EH) to the Hengduan Mountains (HM), whereas R. alexandrae was restricted exclusively in the HM. Both species witnessed a northward expansion of suitable habitats followed by a southerly retreat in the HM region. Our findings reveal that both species have a considerable range shift under different climate change scenarios, mainly triggered by precipitation rather than temperature. The model predicted northward and upward migration for both species since the last glacial period which is mainly due to expected future climate change scenarios. Further, the observed niche overlap between the two species presented that they are more divergent depending on their habitat, except for certain regions in the HM. However, relocating appropriate habitats to the north and high elevation may not ensure the species’ survival, as it needs to adapt to the extreme climatic circumstances in alpine habitats. Therefore, we advocate for more conservation efforts in these biodiversity hotspots.
Introduction
“Unless we move quickly to protect global biodiversity, we will soon lose most of the species composing life on Earth.” —Edward O. Wilson (1929–2021).
Global biodiversity hotspots (Myers et al., 2000) harbor high levels of species richness and endemism (Sun et al., 2017; Rahbek et al., 2019), yet they are vulnerable to large-scale climatic change (Baker and Moseley, 2007). Two biodiversity hotspots, i.e., the Himalayas and the Mountains of Southwest China (also known as the Hengduan Mountains) (Myers et al., 2000; Mittermeier et al., 2011) known to be the biodiversity reservoir of ‘the Third Pole’, can’t remain intangible by present-day global warming (Qiu, 2008). The alpine areas of this region are characterized by low temperatures, intense solar radiation, strong winds, dense cloudiness, frequent precipitation, and a short growing season (He et al., 2006). Several plant species in this area have evolved specific phenotypes, such as “downy plant”, “cushion plant”, and “glasshouse plant” (Tsukaya and Tsuge, 2001; Körner, 2003) . But those plants, which are well adapted to extreme environments through specialized structures, might be susceptible to climate warming (Doiron et al., 2014). Nevertheless, plant species are known to respond to changes in climate by altering their phenology (Cleland et al., 2007) or shifting their distribution range (Parmesan and Yohe, 2003; Chen et al., 2011). Meanwhile, in the past plant species in the Himalaya-Hengduan Mountains (HHM) have been transformed by quaternary climatic oscillations (Sandel et al., 2011; Muellner-Riehl et al., 2019).
Global warming pushes the plant populations to upslope along elevation and poleward along latitude to track isotherms (Lenoir and Svenning, 2013; Zu et al., 2021). As mountain species migrate upslope to cooler climatic conditions to avoid rising temperatures, it results in a smaller inhabited area and population reduction (Morueta-Holme et al., 2015; Freeman et al., 2018). As they ascend higher in elevation towards mountaintops, the elevational range becomes progressively constrained, perhaps increasing the risk of extinction (Manne et al., 1999; Freeman et al., 2018). Warming temperature drives lowlands species to move upwards, and there may be no record for species adapted to higher temperatures to compensate for the loss (Colwell et al., 2008). Indeed, lowland species from warmer microhabitats may relocate to cooler refuges at the same elevation due to global warming (Bush et al., 2004). However, alpine plants might face summit trap phenomena (Rana et al., 2017; Salick et al., 2019). Due to climate change, plant distribution patterns are changing, with species expanding in more suitable areas and declining in increasingly hostile ones (Kelly and Goulden, 2008). Notably, past temperature rise presented an unusual and persistent upward movement of tree lines for about 1000 m in the Himalayan alpine meadows (Schickhoff et al., 2014) or the HM coniferous forest (Lenoir et al., 2008; Wang et al., 2018; Yao et al., 2020). In line with these predictions, several alpine plants (for instance, Anemone rivularis, Abies delavayi) have shifted their ranges upward, north-westward (Liang et al., 2018), or upslope (Kelly and Goulden, 2008; Lenoir et al., 2008; Rumpf et al., 2018). However, on the other hand, the alpine glasshouse species are rarely explored for their range shifts.
The genus Rheum (Polygonaceae) has around 60 species widely distributed across the QTP’s high latitudes. Only a few species make it to Central and Western Asia and Europe (Losina-Losinskaya, 1936; Kao and Cheng, 1975). Owing to the therapeutic and pharmacological properties of Rheum, the increased market demand has impaired the survival and existence of wild species (Chen et al., 2018). The majority of Rheum species have evolved specific morphologies to cope with the harsh climate (Tsukaya and Tsuge, 2001). The ‘glasshouse’ plants, with their large upper translucent bracts covering the inflorescences, are examples of such adaptations (Ohba, 1988). Despite the fact ‘glasshouse’ morphology may be found in more than ten plant families, including Ranunculaceae, Caryophyllaceae, Lamiaceae, Asteraceae, and Polygonaceae (Yoshida, 2002), the most known alpine ‘glasshouse’ plant species that have piqued the interest of evolutionary and conservation biologists are R. nobile Hook. f. & Thomson and R. alexandrae Batalin. The multifunctional translucent bracts of such plants are likely to have evolved independently in the Himalayas as a response to low temperatures and high irradiance, facilitating an upward range shift in response to climate change (Tsukaya and Tsuge, 2001; Tsukaya, 2002; Sun et al., 2012; Song et al., 2013c; Song et al., 2015; Song et al., 2020a). On the other hand, cold-adapted alpine plants may experience range contractions and/or local extinctions (Giménez-Benavides et al., 2011; Wiens, 2016) due to a slower rate of adaptation than that of climate change (Quintero and Wiens, 2013). Therefore, to fill the unprecedented research gap in Rheum species and to develop a scientific strategy to protect alpine glasshouse species (i.e., R. nobile and R. alexandrae) from the negative consequences of global warming, we used species distribution models and assessed the geographical distribution range shift.
The species distribution models (SDM) combined with geographic information systems have ushered in a new era of research into the consequences of climate change on species ecology, biogeography, and conservation (Guisan and Thuiller, 2005; Warren et al., 2008). Ensemble SDM (eSDM) can discover geographic places with a high possibility of having a focal species present by focusing on biological niche demands (Guisan and Thuiller, 2005). With the recent advancement in SDM approaches (Miller, 2010), multi-model projections have been widely utilized to identify the climatic envelope and range shift for endemic and endangered species (Pearson et al., 2007; Robinson et al., 2018).
This research looks at how global warming affects the distribution ranges of the geographically co-occurring alpine ‘glasshouse’ species R. nobile and R. alexandrae (Polygonaceae). With the following precise goals in mind, we adopted multiple eSDM approaches to 1) characterize the realized niche underpinning the distribution of the two focal species, 2) predict range shifts of the two focal species under various climatic scenarios, and 3) discuss potential adaptive responses to changes in the distributional range of the two focal species in response to global warming for biodiversity conservation.
Materials and methods
Focal species
Rheum nobile and R. alexandrae are two species that have evolved specific adaptations to cope with the cold-foggy and damp conditions prevalent at high elevations in the HHM, respectively. Both species (Figure 1A) are giant perennial herbs endemic to the Eastern Himalayas (EH) and the Hengduan Mountains (HM) (Figure 1D), growing at elevations between 3,400 and 6,000 m a.s.l. (Li and Gao, 1998; Chowdhery and Agrawala, 2009). Rheum nobile is a monocarpic herb with individuals dying after a single reproductive event after ca. 33 years of vegetative growth (Song et al., 2020b), whereas R. alexandrae is a multi-stemmed polycarpic perennial (Song et al. unpublished). Despite their relatedness and morphological similarity (Sun et al., 2012), they have distinct habitat and range distributions. Rheum nobile is found chiefly on alpine open scree and occasionally in open patches of alpine meadows, i.e., in well-drained habitats (Chowdhery and Agrawala, 2009; Song et al., 2013c), whereas the partly sympatric R. alexandrae is found mainly on alpine wetlands, including marshes, swampy meadows, and lakeshores (Chen, 1993).
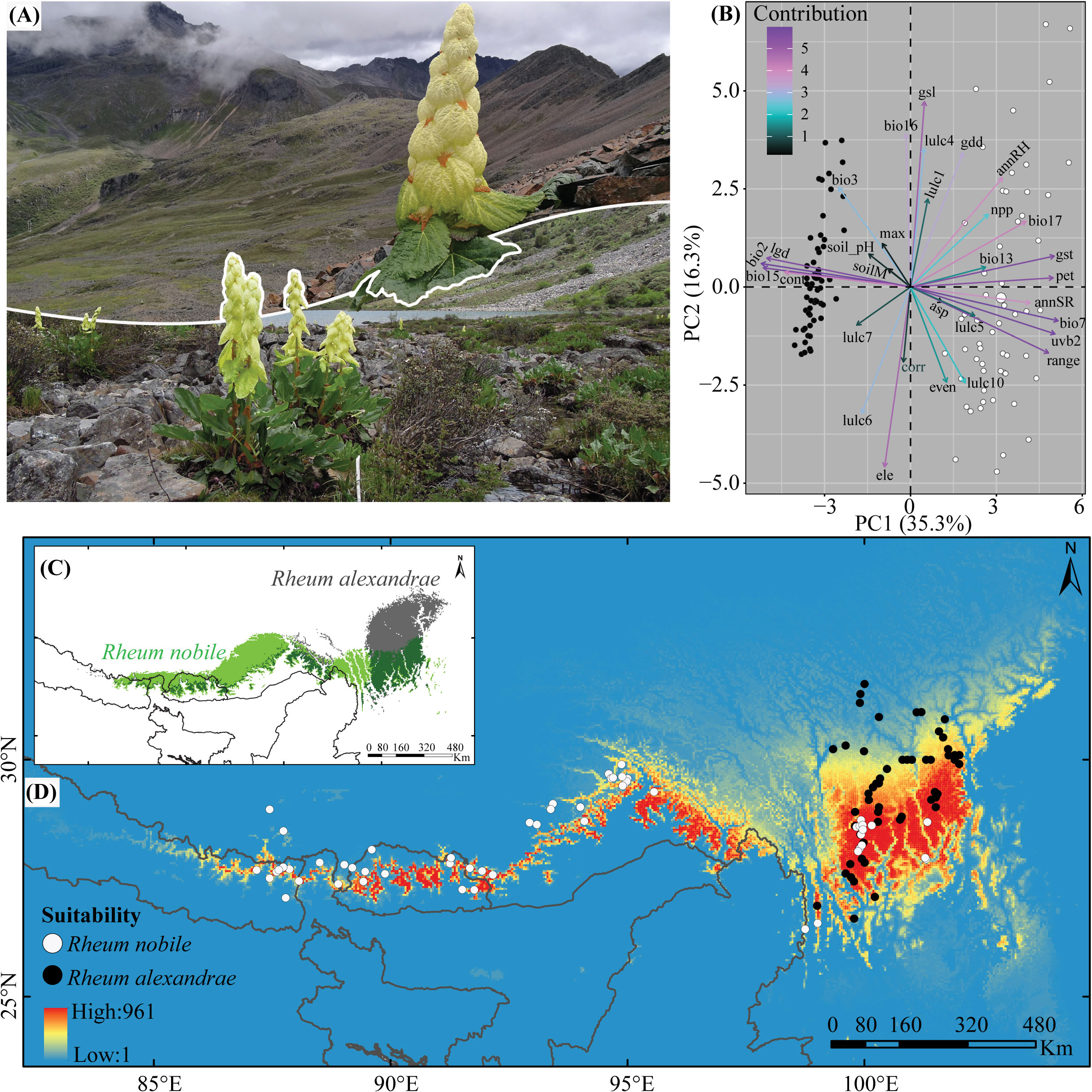
Figure 1 (A) Images of Rheum nobile (above) and Rheum alexandrae (below) in their respective habitats. (B) The relative contribution of the predictive environmental variables (colored arrows) to the two axes of biplots from principal components analysis (PCA). The distribution area of both species as a function of (C) raster overlay and (D) fuzzy overlay depicts their present-day bio-climatically suitable niche in the Eastern Himalaya (EH) and the Hengduan Mountains (HM). The two dots in (B, D) represent the occurrence points for R. nobile (white dot) and R. alexandrae (black dot).
Rarefaction of species occurrence
We focused on the entire geographic distribution ranges of the two species (Figure 1D). For this, the past field (1937–2020) (Supplementary Table S1) datasets were compiled and cross-checked with the herbarium records from the National Herbarium and Plant Laboratories (KATH, Nepal) and Kunming Institute of Botany, CAS (KUN, China) to construct the geographic distribution of both species. In addition to past field and herbarium collections, we also ground-validated occurrence points (from 2015 to 2022) through GPS in the field and cross-checked with the online databases of the Chinese National Herbarium (PE; http://pe.ibcas.ac.cn/en/), Chinese Virtual Herbarium (CVH; http://www.cvh.ac.cn/), the Global Biodiversity Information Facility (GBIF.org, 2021), the Royal Botanical Garden at Edinburgh (RBGE, United Kingdom; http://data.rbge.org.uk/search/herbarium/) and the Herbarium at the University of Tokyo (TI, Japan; http://umdb.um.u-tokyo.ac.jp/DShokubu/). While compiling the occurrence datasets, we mainly focused on geographic coordinates recorded by the different resource persons during the field. So, the occurrence points from the other herbarium specimens and online databases were discarded in the datasets. Following Barbet-Massin et al. (2012), the background points were randomly chosen within a 25–50 km radius of the occurrence points.
Overfitting or biases in eSDM may result from geo-coding errors in the herbarium label and spatially clustered localities (Hijmans, 2012; Boria et al., 2014; Rana et al., 2021), making it challenging to forecast range distribution and climatic suitability accurately. Therefore, we spatially rarefied the gathered geographic occurrences to avoid model bias (Boria et al., 2014; Rana et al., 2017; Rana et al., 2020b). Due to the filter distances’ effectiveness in spatially high heterogeneous regions, like mountains, the occurrences were rarefied using a 10-km spatial grid (Veloz, 2009; Boria et al., 2014; Rana et al., 2021). Finally, the models were developed using 56 out of 79 R. nobile occurrences and 54 out of 77 R. alexandrae occurrences after rarefaction.
Explanatory environmental variables
The potential distribution of the two focal species was modeled for the present-day (ca. 1990–2000) and projected to the paleo (LIG: last inter-glacial ca. 120,000–140,000 years BP; the LGM: last glacial maximum ca. 22,000 years BP) and future (2070, Representative Concentration Pathways, RCPs4.5) climatic scenarios. The climatic projection was created using a multi-model median (MMM) ensemble of General Circulation Models (GCMs) (Rana et al., 2021) (see Supplementary Table S2 for GCMs).
We used three different eSDM in different environmental strata, each with its unique set of predictive variables. Firstly, bioclimatic variables (http://www.worldclim.org/version2) were used to forecast under paleo climatic (LIG, LGM), present-day, and future scenarios. Secondly, all environmental variables were pooled and forecasted. Finally, ensemble forecasting was performed by combining bioclimatic variables with other environmental variable categories such as geo-climatic (http://www.worldclim.org/version2; http://nelson.wisc.edu/sage; http://www.cgiar-csi.org), habitat heterogeneity (Tuanmu and Jetz, 2015; http://www.earthenv.org/texture), growing days (https://chelsa-climate.org/), ultra-violet radiation (Beckmann et al., 2014; https://www.ufz.de/gluv/) and consensus land-cover (Tuanmu and Jetz, 2014; http://www.earthenv.org/landcover). All these climatic variables (Hijmans et al., 2005) and other environmental variables were used with a spatial resolution of 2.5 arc-min (Table 1).
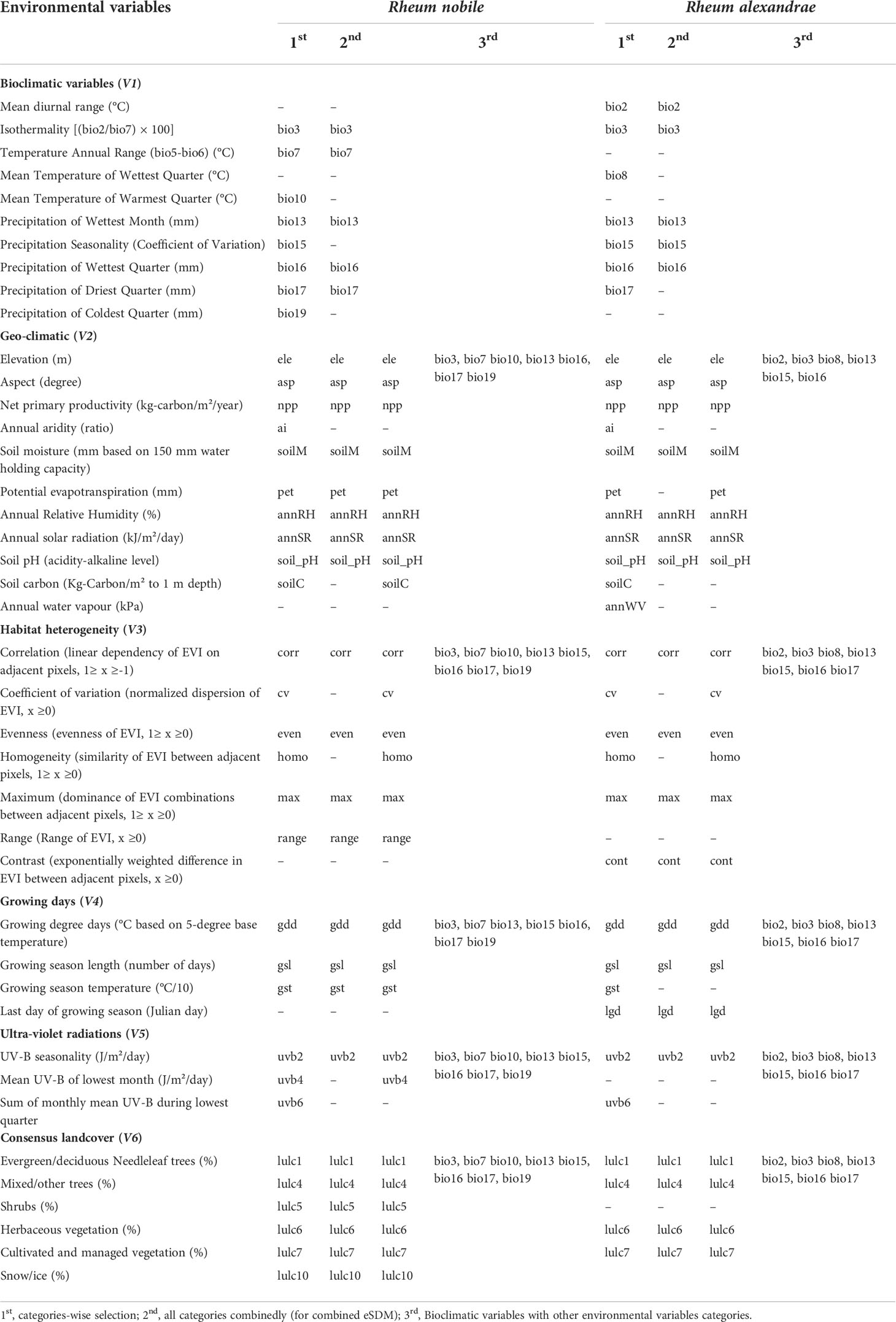
Table 1 Multi-step selection of predictive environmental variables for ensemble species distribution modeling (eSDM) of two alpine ‘glasshouse’ herbs, Rheum nobile and Rheum alexandrae.
The elimination of highly correlated and/or redundant variables reduces the high collinearity, uncertainties, and predictive errors associated with clustered variables (Ranjitkar et al., 2014). Therefore, we used successive steps of variable selection. Firstly, variables were selected category-wise; secondly, all variables from each category were pooled together (for combined ensemble forecasting); and finally, bioclimatic variables were combined with environmental variables from other categories. Initially screened global consensus landcover variables were analyzed with species occurrences larger than a 30% threshold out of all occurrences (Supplementary Table S3). We used the variance inflation factor (VIF; Fox and Weisberg, 2011) (calculated using the R-package ‘car’ fixing elevation as a response variable) in conjunction with a Pearson correlation matrix as the significant core of variable selection. In VIF, the linear function of the model employs numeric response variables and is expressed as VIFj=1/(1–R2j), where R2j is the R2-value obtained by regressing the jth predictor on the remaining predictors (Jackson et al., 2009). Variables with VIFj >10 indicate strong multi-collinearity (Quinn and Keough, 2002), and variables with a high VIFj have a negative impact on the modeling output. Several tests were run with extracted values of occurrences for bioclimatic and other environmental variables until a set of predictors with VIFj values less than 10 was retained. The selection of explanatory variables includes Pearson correlation values of < |0.8| (see Supplementary Tables S4, S5 for correlation analysis results) and VIFj values of < 10 (see Supplementary Tables S6–S10 for VIFj analysis results). Initially, variables were selected based on the Pearson correlation (Supplementary Tables S4, S5) and VIFj analysis (Supplementary Tables S6, S7). In contrast, the remaining steps used VIF (Supplementary Tables S8–S10) as the primary function of the variable selection for both focal species. Several species-specific and environmental variables were used as a subset of predictive variables for forecasting the suitable range (Table 1).
Furthermore, principal component analysis (PCA) was performed to group the occurrences according to the predictive bioclimatic and environmental variables of R. nobile and R. alexandrae using the R-package ‘factoextra’ (Kassambara and Mundt, 2020).
Ensemble model development and validation
For a given species distribution model, we used the standardized approach in ODMAP (Overview, Data, Model, Assessment and Prediction) (Zurell et al., 2020) protocol structure (Supplementary Methods). The ensemble of SDMs was implemented using the R-package ‘Biomod2’ (Thuiller et al., 2020). Biomod2 outperforms single algorithms by incorporating simulations across multi-model classes, parameters, and climatic conditions (Araujo and New, 2007). Moreover, the consistent modeling approach was applied throughout the different strategies implemented for R. nobile and R. alexandrae. The assessment of SDM using niche-based modeling techniques allows for different modeling approaches such as bioclimatic envelopes, regression, classification methods, and machine learning methods (Thuiller et al., 2020) (Table 2). Except for MaxEnt with a maximum iteration of 5000 (Maximum Entropy Models; Phillips et al., 2006), all other models in Biomod2 were run with the default settings.
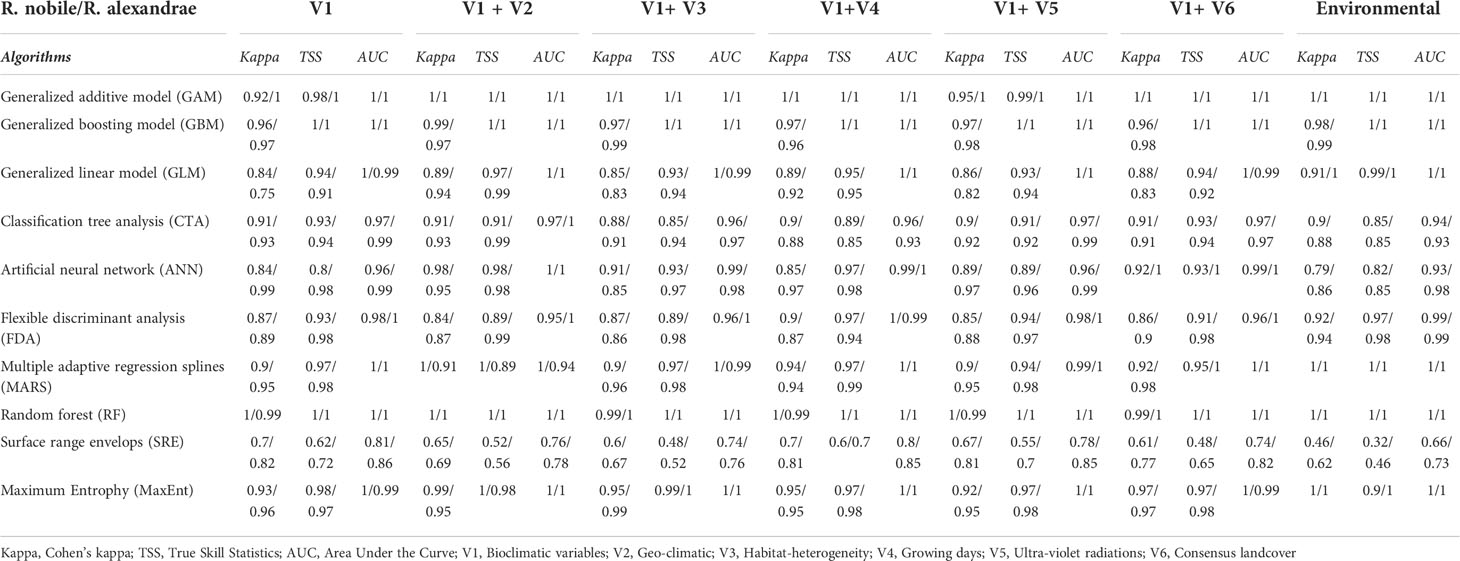
Table 2 Model evaluation indices for the ensemble species distribution modeling (eSDM) of Rheum nobile and Rheum alexandrae using Biomod2 in R-programming language.
Apart from analyzing significant variables, the essential steps in modeling are ensemble model development and validation for consensus mapping, which is generally done using a four-step modeling procedure in Biomod2. The initial step was calibrating ten sub-models with the ‘BIOMOD_Modelling’ function, which defined 4-fold cross-validation using 75% of the data to train the models. The remaining 25% were used to assess the predictive power using True Skill Statistics (TSS), Cohen’s Kappa, and Area Under Curve-Receiver Operating characteristics (AUC) statistics (Araujo et al., 2005; Allouche et al., 2006). The threshold-dependent model accuracy matrices, i.e., TSS and Cohen’s kappa, are independent of prevalence–the ratio of presence to pseudo-absence data in presence-absence predictions (Allouche et al., 2006). It considers both sensitivity and specificity, with the values ranging from −1 to +1, with +1 denoting perfect agreement. Scores between 0.6 to 0.9 suggest medium to good model performance (Allouche et al., 2006). The threshold-independent model evaluation indicator AUC, on the other hand, is likewise independent of prevalence (Phillips et al., 2006) and examines the models’ discriminating abilities. AUC values of less than 0.6 were regarded as poor, 0.6–0.9 were rated moderate, and >0.9 were considered excellent (Phillips et al., 2006). We applied the ‘BIOMOD_EnsembleModeling’ function in the second step, with sub-model weights > 0.9 assessed by TSS for ensemble modeling. The sampling procedure was replicated five times. We then used ‘BIOMOD_Projection’ for projecting the calibrated sub-models into a new space or time. Finally, the ‘BIOMOD_EnsembleForecasting’ function was used to forecast and generate the consensus mapping of species over time and space. The consensus model was then projected onto the past (LIG, LGM) and future (2070) climatic scenarios. Each model’s contribution to the final ensemble model was proportional to its goodness-of-fit statistics.
Mapping the ensemble species distribution model
The spatial conversion of the consensus ensemble model to a binary model (presence/absence) was based on the thresholds (50% of suitable habitats) that suit the present-day distribution of the focal species (Forester et al., 2013; Rana et al., 2020a; Rana et al., 2021). The spatial analyses were carried out in ArcMap 10.4.1 (Environmental Systems Resource Institute (ESRI), 2016) using the extension Spatial Analysis to reclassify changes in LIG, LGM, and future conditions compared to present-day suitability into reduction, stable, and expanded areas of the focal species. The predicted suitable habitat maps of the two focal species were then overlapped to locate overlapping regions under present-day climatic scenarios.
Niche equivalency test
Under the current bioclimatic scenario, we used the ecospat test in R-package ‘ENMTools’ (Warren and Dinnage, 2021) for the two focal species, R. nobile, and R. alexandrae, to test the hypothesis that the environmental niche model formed by population occurrences is identical. The R version of ENMTools has a more straightforward user interface of ecospat hypothesis testing with enmtools.species objects. It further conducts principal component analysis for multiple predictor variables to reduce them to a two-dimensional environment space (Warren et al., 2021). The bioclimatic niches were measured and validated using Schoener’s ‘D’ and Hellinger’s-based ‘I’ through enmtools.ecospat.id function of identity test and enmtools.ecospat.bg function of background test (symmetric and asymmetric) (Warren et al., 2010; Warren et al., 2021). Schoener’s D is a formula that calculates the appropriate range depending on the probability of occupied grid cells. Hellinger’s-based I works in a similar way as Schoener’s D, but without the assumption (Warren and Seifert, 2011). The pairwise similarity values of D and I indices ranged from 0 (complete divergence/no overlap) to 1 (high similarity/complete overlap), indicating that as the score increases, so does the niche overlap.
Results
Explanatory variables analysis and model performance
The series of variable selection resulted in a robust set of bioclimatic and other environmental variables to predict the environmental niche of the two species. Initially screened variable selection through Pearson correlations (r <|0.8|) and VIF (VIFj <10) yielded 36 and 34 least correlated and non-redundant variables for R. nobile and R. alexandrae, respectively. These variables are species-specific to determine the distribution of R. nobile and R. alexandrae (refer to Table 1 for details on predictive variables). Landcover, being an influential factor in determining species range for SDM (Bucklin et al., 2015), we chose the number of occurrences found within each landcover variable (Supplementary Table S3). The first model employs bioclimatic variables, including eight for R. nobile (three temperature-dependent, five precipitations dependent) and seven for R. alexandrae (three temperature-dependent, four precipitations-dependent). Highly contributing algorithm with TSS >0.9 (Table 2) were selected to estimate the response curves of the variables, notably for bioclimatic variables from eSDM (Figure 2) and ENMTools (see Supplementary Figure S1).
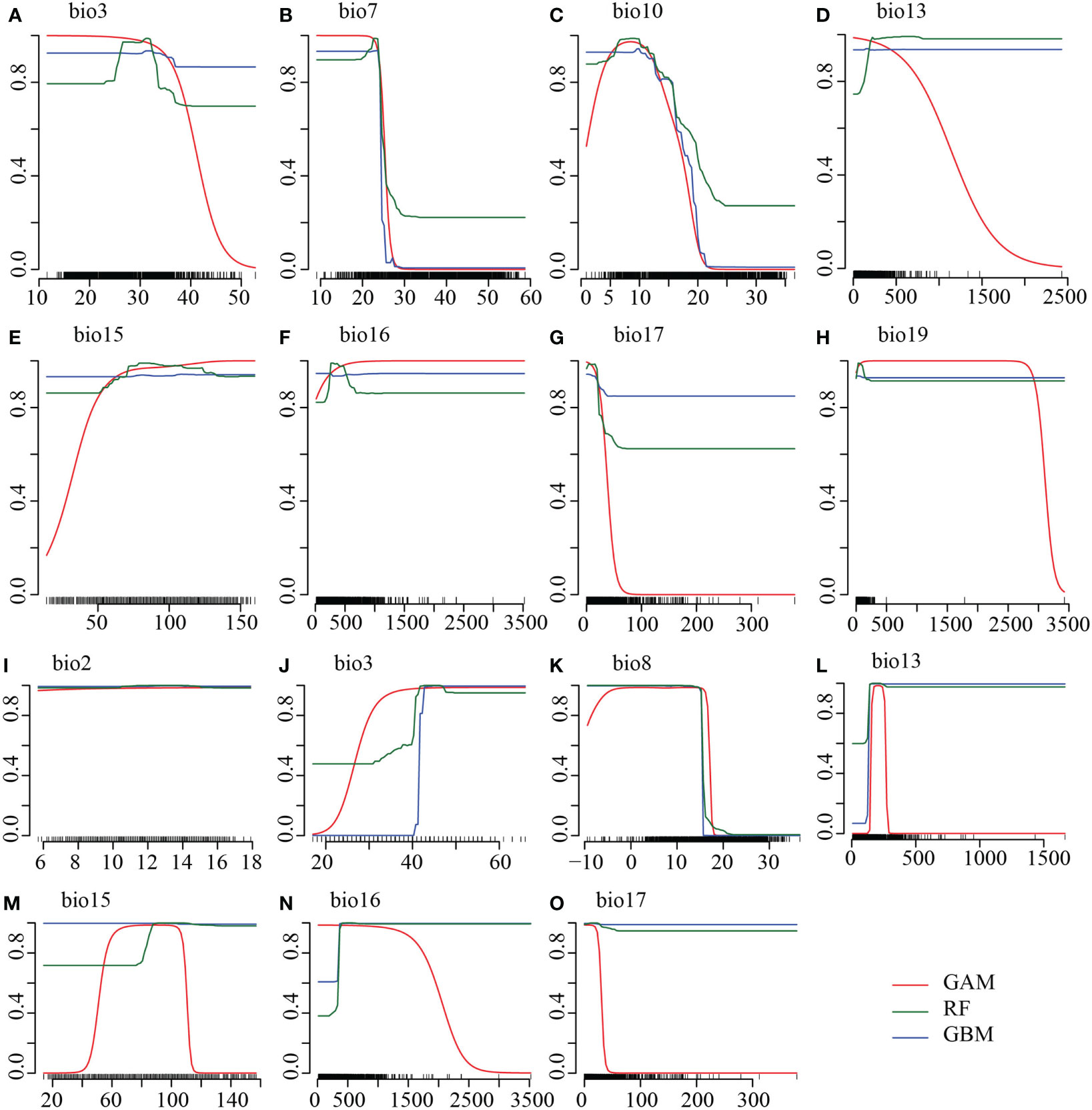
Figure 2 Response curves of the highly predictive top three algorithms showing the probability of presence (y-axis) under the (A–C, I–K) temperature-dependent and (D–H, L–O) precipitation-dependent variables (x-axis) for (A–H) Rheum nobile and (I–O) Rheum alexandrae. The predictive algorithms are the Generalised Additive Model (GAM; red line), Generalised Boosting Models (GBM; blue line), and Random Forest (RF; green line), with high model accuracy TSS > 0.9. Temperatures are expressed in °C (degree Celsius) and precipitation in mm (millimeter). Refer to Table 1 for the bioclimatic variables.
The suitability of R. nobile is more favored by temperature annual range (bio7: ca. 28 ˚C), mean temperature of warmest quarter (bio10: ca. 10 ˚C), precipitation of coldest quarter (bio19: ca. 12 mm) than that of R. alexandrae by mean diurnal range (bio2: ca. 14 ˚C), mean temperature of the wettest quarter (bio8: 8 ˚C) (Figure 2; Supplementary Figure S1). Furthermore, the co-existing niches for both focal species are characterized by isothermality (bio3; ca. 45), precipitation of wettest month (bio13, ca. 200 mm), precipitation seasonality (bio15; ca. 100 mm), precipitation of wettest quarter (bio16; 300–500 mm), and precipitation of driest quarter (bio17; ca. 12 mm) (Figure 2; Supplementary Figure S1). However, other environmental variables contributed to forecasting suitable habitats for the two focal species. When all predictive environmental variables were combined as in the second modeling, robust comparable model algorithms were produced (Table 2). Also, in the third modeling when all these environmental variables were independently modeled with bioclimatic variables only, suitability was found to be predictive.
Meanwhile, the first two components of a PCA with all predictive variables explained 51.6% (PC1: 35.3% and PC2: 16.3%) of the observed variance for the two focal species, and their environmental niches were demarcated from each other in biplots (Figure 1B; Supplementary Figure S2). Bioclimatically (V1), bio2, bio3, and bio15 are the most important for R. alexandrae, whereas bio7 and bio17 are the most important for R. nobile. Although the contributions of the different variables in the biplots are species-specific, R. nobile’s niche is characterized by an assembly of most variables. When compared to R. alexandrae, the niche of R. nobile is characterized largely by environmental variables (refer to Figure 1B and Table 1 for more details on variables contribution). The model evaluation indices AUC and Cohen’s kappa value varied from 0.66 to 1 and 0.46 to 1. The SRE algorithm scored the lowest, and the RF, GAM, and GBM algorithms scored the highest (Table 2). Finally, the consensus model was evaluated and calibrated for all three modelings, with TSS >0.9 (details in Table 2). The result indicates that the consensus models were highly predictive and accurate regarding AUC, Cohen’s kappa, and TSS (Table 2).
Ecological niche analysis
Quantitatively, the average weight of the identity test (D, I < 0.82; Figure 3A) and the low weight of the background test (D, I<0.6; Figures 3B, C) show that the two focal species R. nobile and R. alexandrae, have quite different environmental niches. The ENM similarity score for the present-day occurrence of two species is average compared to what would be expected based on the null hypothesis of niche equivalency, indicating that the two species’ environmental niches may not be similar (Figure 3A). However, the observed overlap between the two species in background tests is lower than expected under the null hypothesis, showing that the two species are more divergent depending on their habitat (Figures 3B, C). However, the predictive power of the niche assemblage differs significantly as a consequence of multiple algorithms. The lowest to average score on the identity test and background test through GAM indicates that the two species are ecologically apart (Supplementary Figures S3A, C, E). In contrast, RF algorithms with the highest identity and background tests imply that the two species’ ecological niches are comparable and co-occur at the same locality (Supplementary Figures S3B, D, F).
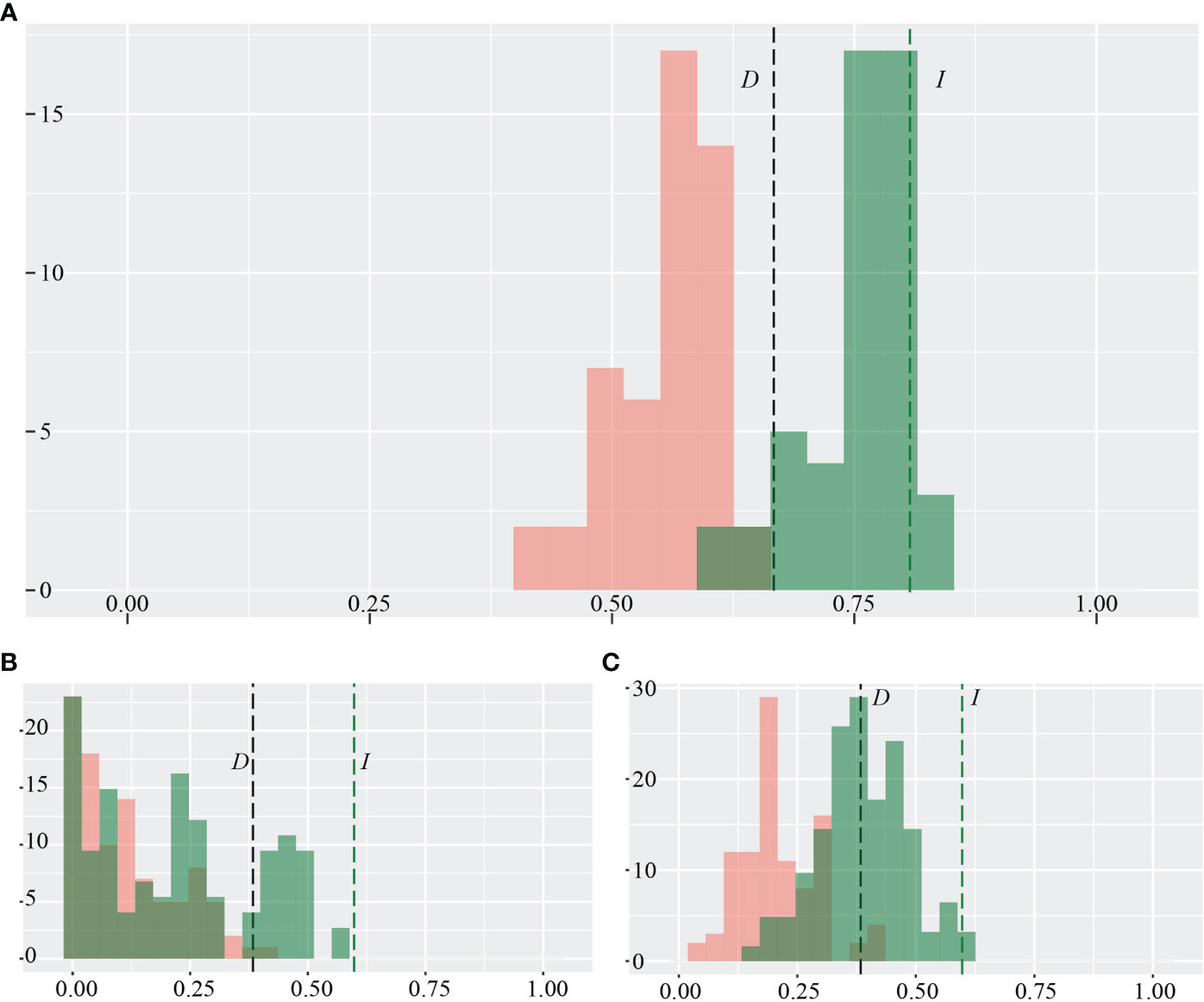
Figure 3 Pairwise niche (A) identity test and background test (B), symmetric; (C), asymmetric calculated between the two alpine ‘glasshouse’ herbs Rheum nobile and Rheum alexandrae as a function of niche.overlap ecospat test implemented in ENMtools. The niche equivalency test as species-wise pair comparisons was measured by Schoener’s D (in black color) and Hellinger’s-based I (in green color) indices.
Climate-induced range shifts of the two alpine ‘glasshouse’ Rheum species
The predicted suitable habitat under the initial bioclimatic modeling inferred the better suitability of R. nobile across the EH than the HM (Figure 4A). On the other hand, R. alexandrae has a robust distribution range in the eastern HM and is scattered across the EH (Figure 4B). The projected suitability using all environmental variables coupled with bioclimatic variables (second modeling) (Supplementary Figures S4A, G) or bioclimatic + each environmental variable (third modeling) (Supplementary Figures S4B–F, H–L) resulted in similar patterns for the distribution ranges. The continuous pattern of the distribution range of R. nobile runs from the EH to the HM (Supplementary Figures S4A–F). In contrast, all three modeling approaches revealed a narrower distribution range of R. alexandrae restricted in the HM (Supplementary Figures S4G–L). The conservative prediction of high suitability in the HM for both species represents the present-day distribution range of the species (Figures 1C, D and 4A, B; Supplementary Figure S4). Although they appear in distinct locations, their range comprises the same geographic region in the HM, namely Huluhai and Hongshan (field observation) (Figures 1B–D).
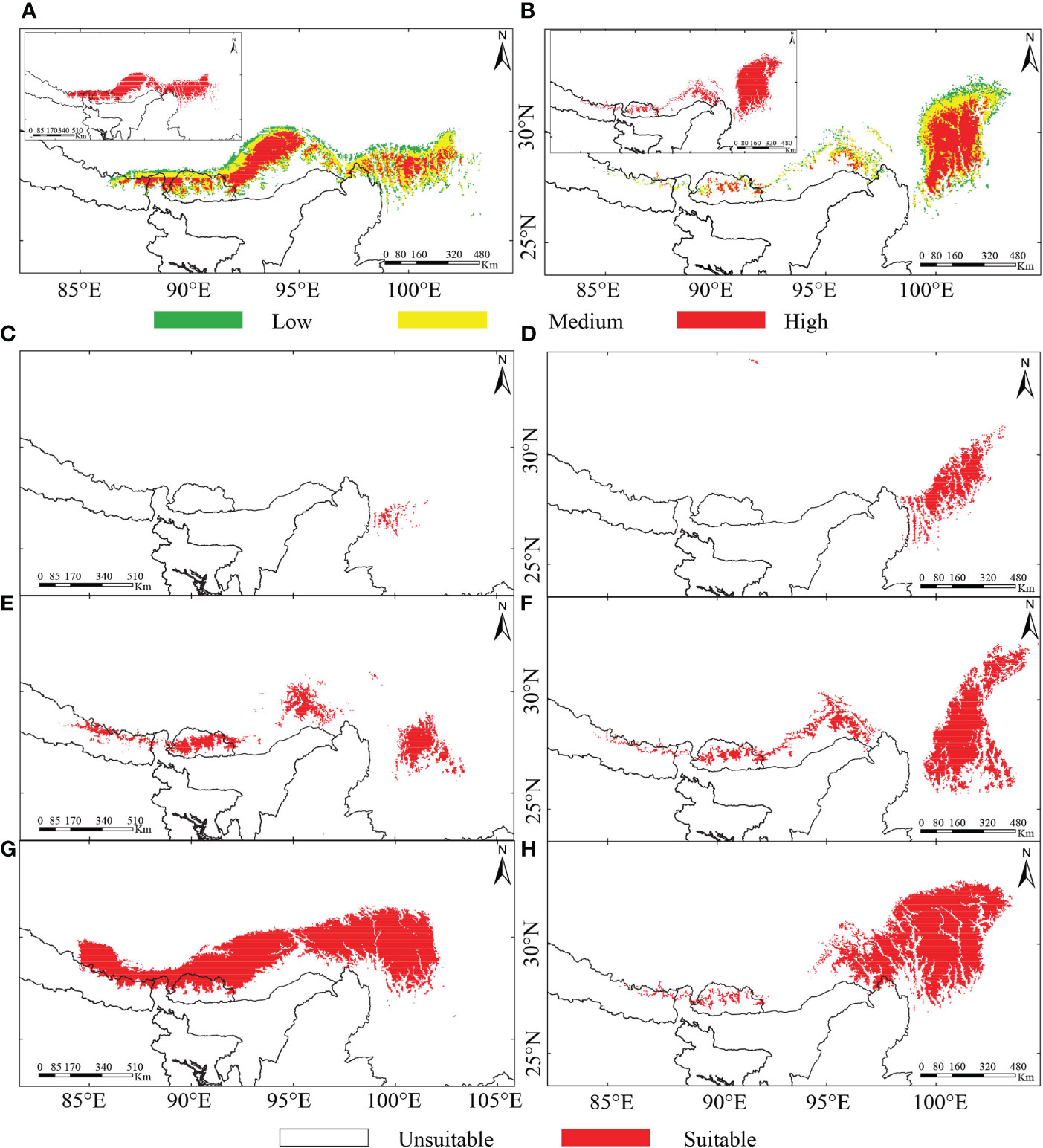
Figure 4 Predicted potentially suitable habitat of two alpine ‘glasshouse’ herbs, (A, C, E, G) Rheum nobile and (B, D, F, H) Rheum alexandrae under (A, B) present-day scenario of bioclimatic variables classifying their suitability level (suitable habitat in the left top corner of each map), (C, D) last-inter glacial (LIG), (E, F) last glacial maximum (LGM), and (G, H) future scenarios.
The prediction under four different climate scenarios (LIG, LGM, present-day, and future) are mainly observed across the Eastern Himalayas and the Hengduan Mountains (Figures 1C, D and 4; Supplementary Figure S4). From LIG to the present-day, Rheum nobile has a varied suitable distribution range, whereas a future range is comparable with the present-day (Figures 4A, C, E, G). Throughout LIG, the paleo-distribution model predicted refugial habitats in the southern HM (Figure 4C), which relocated east and west in distinct regions during the LGM (Figure 4E). Similarly, R. alexandrae’s suitable distribution expands from the LIG to the present-day and then to the future (Figures 4B, D, F, H). R. alexandrae was more common in the central HM during the LIG than R. nobile. Then, the distribution range shifts towards the eastern HM, with some patches towards the EH during the LGM. When projected to the future scenario, the suitable range in the northern HM moves even higher (Figure 4H). Both species were predicted to have suitable distribution ranges and a strong expansion towards the north (Figure 5). However, their distribution ranges were only slightly reduced from the paleo climatic scenario [LIG (Figures 5A, B) and LGM (Figures 5C, D)] to the present-day, and from the present-day to the future (Figures 5E, F). However, in comparison to the present-day situation, the expansion towards the north is accompanied by a habitat contraction in the south, notably in the HM. Conclusively, the ensemble forecasting for the two species shows different range shifts under different climatic scenarios.
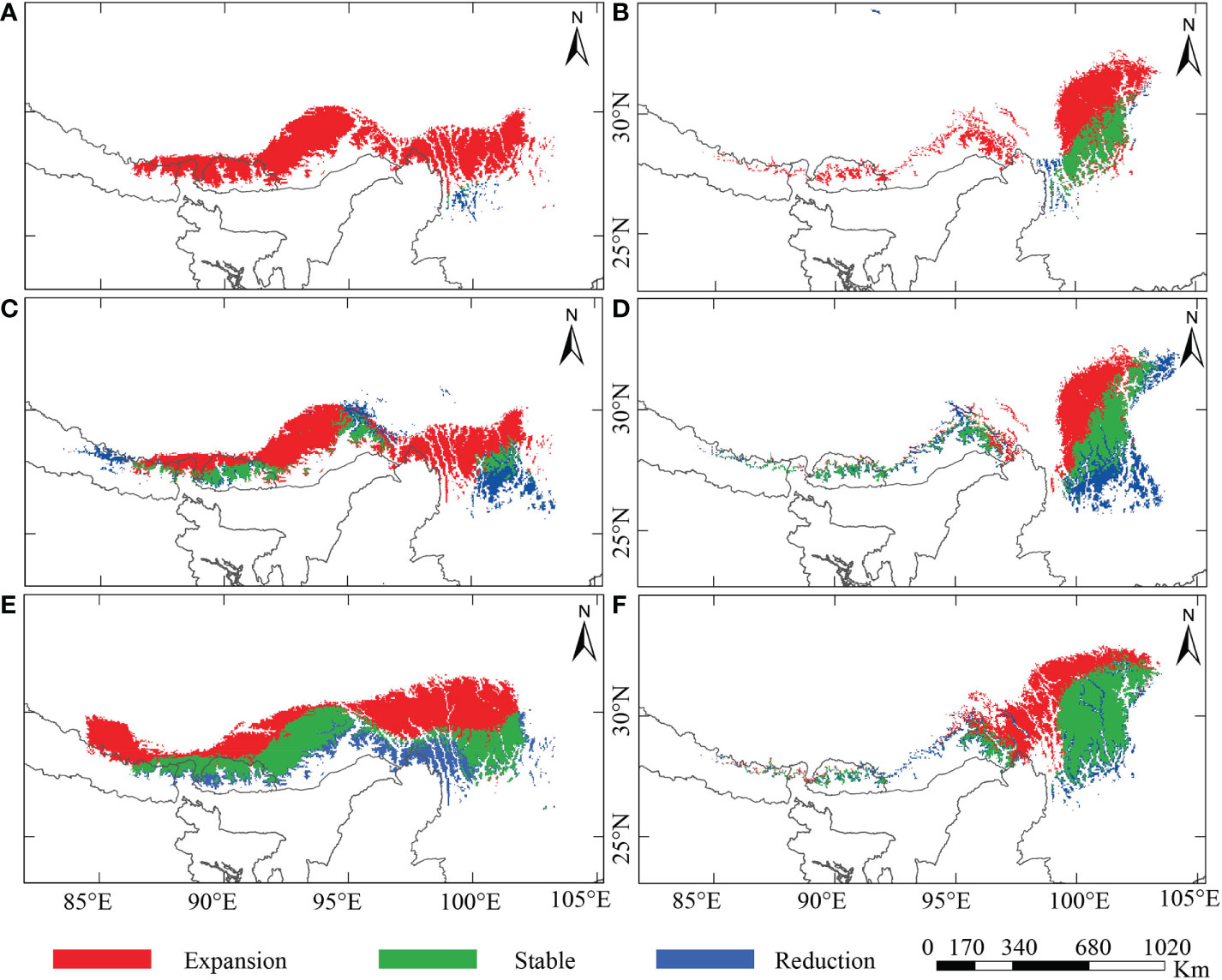
Figure 5 Potentially reduced, stable, and expanded range for the alpine ‘glasshouse’ herbs, (A, C, E) Rheum nobile and (B, D, F) Rheum alexandrae compared among different climate scenarios: (A, B) last interglacial (LIG) versus present-day; (C, D) last glacial maximum (LGM) versus present-day; and (E, F) present-day versus future [2070, Representative Concentration Pathway (RCP) 4.5].
Discussion
Predictiveness of the multi-model median for eSDM
Climate change in mountainous regions is projected to shift plant distribution upwards, consequently, declining their populations in those areas where it is no longer suited for them. The capacity to model such alterations in plant distribution ranges is heavily reliant on the availability of sufficient environmental data and algorithms to analyze the temporal changes. Ensemble modeling has proven to be robust and predictive, overcoming the uncertainty and variability of single models across multiple GCMs (Murphy et al., 2004; Pierce et al., 2009; Rana et al., 2021). The use of ensemble modeling in conjunction with consensus projections not only reduces the predictive uncertainty of single models (Araujo and New, 2007; Ranjitkar et al., 2014) but also improves accuracy (Marmion et al., 2009) and addresses uncertainties inherent in SDM techniques (Barry and Elith, 2006). However, it is equally important to consider the selective nature of variables selected through a series of variable filtration used in the different modeling. Our study is the first to use an ensemble forecasting procedure in an assemblage of variables related to bioclimatic, geo-climatic, habitat heterogeneity, the number of growing days, landcover, and ultraviolet radiation to predict the range shift of plant species. It is anticipated to have a comparable predictive value when forecasting the range shift of montane plants based on only bioclimatic variables (Rana et al., 2021). Nonetheless, alpine plants must use bioclimatic variables with macro/micro-climatic environmental variables, habitat heterogeneity, landcover, canopy cover, and UV radiation (Austin and van Niel, 2011; Robinson et al., 2018). As a result, model uncertainty is minimized, and the algorithm’s accuracy is improved. We analyzed and evaluated the algorithms using threshold-independent (i.e., AUC) and threshold-dependent (i.e., TSS and Cohen’s kappa) model performance measures and outlined the accuracy and predictiveness (matrices values >0.9) of algorithms in different eSDM for both species.
Ecological niche characterization of the two alpine ‘glasshouse’ Rheum species
Global warming will significantly impact the distribution and resilience of the earth’s ecological communities (Mclaughlin and Zavaleta, 2012). These impacts will most likely be felt in mountainous regions, as many alpine plants’ ranges are projected to migrate upwards/polewards (IPCC, 2014). Meanwhile, we found that the distribution of alpine plants, such as the two ‘glasshouse’ Rheum species, are characterized by precipitation-dependent variables more than temperature-dependent variables, thereby confirming Fu et al. (2018) and Sun et al. (2016). Along with that, the climatic niche for R. nobile is also characterized by a ‘temperature annual range’ with peak suitability at ca. 28˚C, higher than Wang (2006) estimation (i.e., 15 ˚C). Rheum alexandrae, on the other hand, has a ‘mean temperature of warmest quarter’ of ca. 8 ˚C. Therefore, the temperature-induced shift in bioclimatic zones is the decisive factor responsible for the decline in the suitability range of the two alpine species. However, their local occurrence and ecological microhabitat are frequently associated with soil water conditions. Rheum alexandrae is found chiefly in alpine wetlands, including marsh, swampy meadows, and lakeshores (Chen, 1993); while R. nobile, usually occurs on alpine scree and rarely in open patches of alpine meadow, i.e., in well-drained habitats (Song et al., 2013a; Song et al., 2013b).
Specifically, climate regulates the distribution limitations of the two Rheum species. The species’ cool range limits are governed directly by climatic factors, while the warm range limits are more impacted by biotic interactions, such as predation, mutualism, and resource competition (Paquette and Hargreaves, 2021). Furthermore, our model exemplifies the hypothesis of a combined latitudinal/upward range shift of species with cool range limitations. Despite their close relationship and similar morphology (Sun et al., 2012; Song et al., 2020b), R. alexandrae and R. nobile exhibit distinct life histories, distribution limits, and ecological niches related to soil water conditions. Consequently, their specific local niches might underpin the biogeographic patterns of alpine ‘glasshouse’ species. Their specific areas of occurrence were barely apart from each other. They are in comparable geographical places. However, they had never existed together in the same microhabitat. Consequently, niche identity and background analyses for the two ‘glasshouse’ species revealed minimal evidence of comparable niche composition.
Global warming affects the distribution range of the two alpine ‘glasshouse’ Rheum species
Will species be able to adapt in the face of distribution range shift as a result of climate warming? Such topics have been hotly debated, but it is still poorly understood in science. Many researchers argue that species that have previously adapted to worsening climate conditions may be threatened more seriously. In contrast, others fear that the shift in species ranges caused by global warming may result in species extinction (Williams et al., 2007). For mountainous plant species, global warming is expected to act as an ‘escalator to extinction’ (Freeman et al., 2018). This is especially true for species living near mountaintops that cannot move higher. Our model illustrates the range shifts due to global warming for the two alpine ‘glasshouse’ Rheum species. The model identified their distinct niches, influencing their responses to predicted climate change and their shift across the Himalayas and the Hengduan Mountains (You et al., 2018). Further, our model suggested that the appropriate range for Rheum species has risen since the past LIG, as a result of climate change. The existence of suitable habitats in the HM for both the species during glacial-interglacial periods, especially for R. alexandrae, reflects that the HM was not completely glaciated and likely served as a refugium for many plants during glacial periods (Qiu et al., 2011; Zhang et al., 2018; Muellner-Riehl, 2019). The specific nature of the habitat niches for the two Rheum species led to an increased suitable range from LIG to LGM.
The southern HM provided a refugium for R. nobile and the southeast HM for R. alexandrae. As a result of global cooling, the eSDM suggested that both species were able to expand their geographic range from LIG to LGM in different magnitudes (Retallack, 2001; Meng et al., 2017). Possibly, glaciations during the LGM might have pushed the suitable range of R. nobile towards the EH and east of the HM. At the same time, R. alexandrae, during the LIG, slightly retreated its area of occurrence towards the southern HM. However, an occasional long-distance dispersal of the seed by wind (Song et al., 2013b) may have shaped the current HHM distribution for R. nobile. The present-day model of the suitable distribution range represents the actual distribution for both species, except for that of R. alexandrae in the EH. Rheum nobile is predicted to have a wide suitable range in the EH and the HM, but for R. alexandrae only in the HM. The HHM endemic R. nobile conjunctly occurs in the HM (especially in the Huluhai and Hongshan regions) but in the distinct areas from R. alexandrae.
The present-day similar distribution ranges of the two species in the EH and the HM will no longer exist under climate warming (Figures 4, 5). We speculate that super-competitor species that increase their range northward may benefit from identical niche requirements due to climate change (Liang et al., 2018; Rumpf et al., 2018). Species’ ability to adapt genetically or modify their physiological tolerance will determine their local survival. The dramatic temperature fluctuation during the quaternary glacial cycles has led plant species to move downslope during the LGM and then return upslope with the Holocene warming (Flenley, 1998; Bush et al., 2004). Similarly, since glacial-interglacial periods, our model of warming scenarios predicted north- and upwards expansion of suitable habitats in the HHM and retreat of suitable habitats in the south. The complex topography in the high HM provides enough land surface, allowing upward relocation of suitable habitats and a northward expansion into adjacent areas with suitable habitats (Liang et al., 2018). Similar results were found in SDM studies for other Himalayan species (Song et al., 2004; Xiaodan et al., 2011; Zhao et al., 2011; Manish et al., 2016; Lamsal et al., 2017), which predicted a northward vegetation shift and re-shuffling of plant assemblages in the future due to global warming. Apart from that, comparable findings were observed for Australian plants (Auld et al., 2022), along with other mountain species in the European Alps (Thuiller et al., 2005; Benito et al., 2011; Dullinger et al., 2012), the Andes (Ruiz et al., 2008; Feeley and Silman, 2010), and in arctic regions of the northern hemisphere (Kaplan and New, 2006). However, such range shifts may not be sufficient to ensure population and species survival (Rana et al., 2017). Alpine species may need to change physiologically to cope with the specific climatic circumstances found at the higher elevations (Körner, 2003); seeds, in particular, must be viable for germination and resistant to the ‘summit trap phenomena’ (Pertoldi and Bach, 2007). A failure to adapt might cause mountaintop species to face the risk of ‘mountaintop extinction’ unless they have disjunct populations elsewhere on higher mountains or in colder latitudes (Williams et al., 2007; Lenoir et al., 2008).
Conservation implication in response to the rise in global temperature
Local extinction of mountain species is a significant driver altering ecosystem structure and misbalancing biodiversity in hotspots. The Himalayas and the Mountains of Southwest China (also known as the HM) are two crucial biodiversity hotspots (Myers et al., 2000; Mittermeier et al., 2011) with a high proportion of endemic mountaintop species. Endemic high mountain species like R. nobile and R. alexandrae are more vulnerable to local extinction due to global warming as their distribution range is pushed higher and higher. The species’ ability to survive in extreme conditions may be jeopardized if its distribution range expands towards mountaintops. Instead, despite having the adaptive capacity to endure challenging conditions at the mountaintops, the species must suffer local extinction due to increased elevational range contraction (Freeman et al., 2018). Therefore, a centerpiece of biodiversity conservation always warrants the conservation of remaining habitats from further loss.
Moreover, the quantification and mapping of the suitable range of the alpine ‘glasshouse’ Rheum in response to climate change have implications for conservation policies aimed at protecting their natural habitats. Our future-predicted map of suitable habitats can be used to identify critical habitat areas and prioritize conservation requirements, reducing biodiversity loss due to habitat degradation and loss. As a result, given the current period of biodiversity loss, there is a desperate need to understand the mechanisms driving the biodiversity dynamics in hotspots, as these findings will aid in the development of conservation policies to safeguard species threatened by environmental changes and human influences. We know that conservation efforts will be challenged by the severity of climate change and the different range shifts of certain species due to climate change (Williams et al., 2007). Furthermore, rising anthropogenic pressure from increased root harvesting for Tibetan medicinal purposes (Song et al., 2020b) has enhanced the need for conservation. Additionally, grazing pressure by yaks has dramatically increased in grassland during summer and autumn (Yang and Du, 1990). It is likely to pose a threat to the rare R. nobile even by moderate grazing and thus this flagship species could be endangered by intensified traditional pastoralism (Song et al., 2020b). The information presented here about future climate refugia should be utilized to drive the establishment of conservation areas and conservation initiatives.
Conclusions
Our research investigates the range shift pattern of two alpine ‘glasshouse’ species, R. nobile and R. alexandrae, under different climate change scenarios using different eSDM. We explored climatic variables in conjunction with environmental variables to forecast the species’ geographical distribution range. This research demonstrated the importance of applying eSDM and multi-matrices model evaluation methodologies for robust alpine plant range shift projections. Our model predicted the two Rheum species’ northward and upward migration from the last glacial period to a future climate change scenario. We not only predicted a shift in the two focus species’ distribution ranges but also exemplified the sympatric coexistence of two Rheum species, albeit in distinct ecological niches. Despite having evolved to deal with extreme alpine climatic conditions, the predicted distribution range north- and upwards of the two Rheum species may not ensure their survival in the future. Our findings highlight the necessity of combining mechanistic research with knowledge of the physiological and molecular mechanisms that underpin distribution range and current biodiversity patterns. In this era of accelerating biodiversity loss, it is critical to understand the mechanism that drives biodiversity dynamics in hotspots. Through eSDM, we argue for a better understanding of alpine plants’ species range shifts and better biogeographer insight into adaptations and biodiversity conservation objectives.
Data availability statement
The datasets presented in this study can be found in online repositories. The names of the repository/repositories and accession number(s) can be found below: Dryad depository (https://doi.org/10.5061/dryad.jq2bvq8b3).
Author contributions
HS, SKR and BS conceived the ideas. SKR, BS and HKR collected the data. SKR and HKR analyzed the data with the support of JS, SR; and SKR wrote the manuscript with the support of HS, BS, JS, HKR, and SR. All authors contributed to the article and approved the submitted version.
Funding
The first author is supported by the ‘CAS President’s International Fellowship Initiative’ (PIFI) postdoctoral fellowship (2021PB0034). This study was funded by the Second Tibetan Plateau Scientific Expedition and Research (STEP) program (2019QZKK0502), the Strategic Priority Research Program of the Chinese Academy of Sciences (XDA20050203), the National Natural Science Foundation of China (31770249, 32071669 and 32150410356), the Key Projects of the Joint Fund of the National Natural Science Foundation of China (U1802232).
Acknowledgments
The authors thank numerous collaborators for their contribution to gathering the occurrence of the Rheum species, especially curators of the Herbarium of the Kunming Institute of Botany, CAS (KUN) and the National Herbarium and Plant Laboratories, Nepal (KATH).
Conflict of interest
The authors declare that the research was conducted in the absence of any commercial or financial relationships that could be construed as a potential conflict of interest.
Publisher’s note
All claims expressed in this article are solely those of the authors and do not necessarily represent those of their affiliated organizations, or those of the publisher, the editors and the reviewers. Any product that may be evaluated in this article, or claim that may be made by its manufacturer, is not guaranteed or endorsed by the publisher.
Supplementary material
The Supplementary Material for this article can be found online at: https://www.frontiersin.org/articles/10.3389/fpls.2022.925296/full#supplementary-material
Supplementary methods | _ODMAP Protocol.
Supplementary Figure 1 | Response curves of the highly predictive top two algorithms showing the probability of presence and suitability (y-axis) under the (A–C, I–K) temperature-dependent and (D–H, L–O) precipitation-dependent variables (x-axis) for (A–H) Rheum nobile and (I–O) Rheum alexandrae under the function of ENMTools. The predictive algorithms are the Generalised Additive Model (GAM; red line) and Random Forest (RF; green line). Temperatures are expressed in °C (degree Celsius) and precipitation in mm (millimeter). Refer to for the bioclimatic variables.
Supplementary Figure 2 | (A) The scree plot representing the variance explained by the first ten principal components of predictive environmental variables. (B, C) The contribution of top predictive environmental variables under the PC1 (B) and PC2 (C) scores. The Red dashed line indicates the expected average contribution if variable contributions were uniform. Refer to for abbreviated environmental variables.
Supplementary Figure 3 | Pairwise niche (A, B) identity test and background test (C, D, symmetric; E, F, asymmetric test) for the highly predictive top two algorithms (A, C, E) Generalized additive model (GAM) and (B, D, F) Random forest (RF) model, between the two alpine ‘glasshouse’ herbs Rheum nobile and Rheum alexandrae, calculated as a function of niche.overlap ecospat test using ENMtools. The niche equivalency test as species-wise pair comparisons was measured by Schoener’s D (in black color) and Hellinger’s-based I (in green color) indices.
Supplementary Figure 4 | Predicted potentially suitable habitat of two alpine ‘glasshouse’ herbs, (A–F) Rheum nobile and (G–L) Rheum alexandrae, under the present-day scenario of predictive (A, G) environmental variables, (B, H) geo-climatic, (C, I) habitat heterogeneity, (D, J) growing days, (E, K) ultra-violet radiations, and (F, L) consensus landcover.
References
Allouche, O., Tsoar, A., Kadmon, R. (2006). Assessing the accuracy of species distribution models: prevalence, kappa and the true skill statistic (TSS). J. Appl. Ecol. 43, 1223–1232. doi: 10.1111/j.1365-2664.2006.01214.x
Araujo, M. B., New, M. (2007). Ensemble forecasting of species distributions. Trends Ecol. Evol. 22, 42–47. doi: 10.1016/j.tree.2006.09.010
Araujo, M. B., Pearson, R. G., Thuiller, W., Erhard, M. (2005). Validation of species-climate impact models under climate change. Glob. Change Biol. 11, 1504–1513. doi: 10.1111/j.1365-2486.2005.01000.x
Auld, J., Everingham, S. E., Hemmings, F. A., Moles, A. T. (2022). Apline plants are on the move: Quantifying distribution shifts of Australian alpine plants through time. Divers. Distrib. 28, 943–955. doi: 10.1111/ddi.13494
Austin, M. P., van Niel, K. P. (2011). Improving species distribution models for climate change studies: variable selection and scale. J. Biogeogr. 38, 1–8. doi: 10.1111/j.1365-2699.2010.02416.x
Baker, B. B., Moseley, R. K. (2007). Advancing treeline and retreating glaciers: Implications for conservation in yunnan, PR China. Arct. Antarct. Alp. Res. 39, 200–209. doi: 10.1657/1523-0430(2007)39[200:ATARGI]2.0.CO;2
Barbet-Massin, M., Jiguet, F., Albert, C. H., Thuiller, W. (2012). Selecting pseudo-absences for species distribution models: how, where and how many? Methods Ecol. Evol. 3, 327–338. doi: 10.1111/j.2041-210X.2011.00172.x
Barry, S., Elith, J. (2006). Error and uncertainty in habitat models. J. Appl. Ecol. 43, 413–423. doi: 10.1111/j.1365-2664.2006.01136.x
Beckmann, M., Václavík, T., Manceur, A. M., Šprtová, L., von Wehrden, H., Welk, E., et al. (2014). glUV: a global UV-b radiation data set for macroecological studies. Methods Ecol. Evol. 5, 372–383. doi: 10.1111/2041-210X.12168
Benito, B., Lorite, J., Peñas, J. (2011). Simulating potential effects of climatic warming on altitudinal patterns of key species in Mediterranean-alpine ecosystems. Clim. Change 108, 471–483. doi: 10.1007/s10584-010-0015-3
Boria, R. A., Olson, L. E., Goodman, S. M., Anderson, R. P. (2014). Spatial filtering to reduce sampling bias can improve the performance of ecological niche models. Ecol. Model. 275, 73–77. doi: 10.1016/j.ecolmodel.2013.12.012
Bucklin, D. N., Basille, M., Benscoter, A. M., Brandt, L. A., Mazzotti, F. J., Romanach, S. S., et al. (2015). Comparing species distribution models constructed with different subsets of environmental predictors. Divers. Distrib. 21, 23–35. doi: 10.1111/ddi.12247
Bush, M. B., Silman, M. R., Urrego, D. H. (2004). 48,000 years of climate and forest change in a biodiversity hotspot. Science 303, 827–829. doi: 10.1126/science.1090795
Chen, S. (1993). “Polygonaceae” in Vascular plants of the hengduan mountains. Eds. Wang, W., Wu, S., Lang, K., Li, P., Pu, F., Chen, S. (Beijing, China: Science Press), 349–369.
Chen, I., Hill, J. K., Ohlemüller, R., Roy, D. B., Thomas, C. D. (2011). Rapid range shifts of species associated with high levels of climate warming. Science 333, 1024–1026. doi: 10.1126/science.1206432
Chen, T., Yang, X., Wang, N., Li, H., Zhao, J., Li, Y. (2018). Separation of six compounds including two n-butyrophenone isomers and two stibene isomers from Rheum tanguticum maxim by recycling high speed counter-current chromatography and preparative high-performance liquid chromatography. J. Sep. Sci. 41, 3660–3668. doi: 10.1002/jssc.201800411
Chowdhery, H. J., Agrawala, D. K. (2009). Rheum nobile hook. f. and thoms. (Polygonaceae)-a rare and highly specialized plant of Himalayan region. Indian J. Bot. 32, 145–148.
Cleland, E. E., Chuine, I., Menzel, A., Mooney, H. A., Schwartz, M. D. (2007). Shifting plant phenology in response to global change. Trends Ecol. Evol. 22, 357–365. doi: 10.1016/j.tree.2007.04.003
Colwell, R. K., Brehm, G., Cardelús, C. L., Gilman, A. C., Longino, J. T. (2008). Global warming, elevational range shifts, and lowland biotic attrition in the wet tropics. Science 322, 258–261. doi: 10.1126/science.1162547
Doiron, M., Gauthier, G., Levesque, E. (2014). Effects of experimental warming on nitrogen concentration and biomass of forage plants for an arctic herbivore. J. Ecol. 102, 508–517. doi: 10.1111/1365-2745.12213
Dullinger, S., Gattringer, A., Thuiller, W., Moser, D., Zimmermann, N. E., Guisan, A., et al. (2012). Extinction debt of high-mountain plants under twenty-first-century climate change. Nat. Clim. Change 2, 619–622. doi: 10.1038/nclimate1514
Feeley, K. J., Silman, M. R. (2010). Land-use and climate change effects on population size and extinction risk of Andean plants. Glob. Change Biol. 16, 3215–3222. doi: 10.1111/j.1365-2486.2010.02197.x
Flenley, J. R. (1998). Tropical forests under the climates of the last 30,000 years. Clim. Change 39, 177–197. doi: 10.1023/A:1005367822750
Forester, B. R., DeChaine, E. G., Bunn, A. G. (2013). Integrating ensemble species distribution modelling and statistical phylogeography to inform projections of climate change impacts on species distributions. Divers. Distrib. 19, 1480–1495. doi: 10.1111/ddi.12098
Freeman, B. G., Scholer, M. N., Ruiz-Gutierrez, V., Fitzpatrick, J. W. (2018). Climate change causes upslope shifts and mountaintop extirpations in a tropical bird community. Proc. Natl. Acad. Sci. U.S.A. 115, 11982–11987. doi: 10.1073/pnas.1804224115
Fu, G., Shen, Z., Zhang, X. (2018). Increased precipitation has stronger effects on plant production of an alpine meadow than does experimental warming in the northern Tibetan plateau. Agric. Forest. Meteorol. 249, 11–21. doi: 10.1016/j.agrformet.2017.11.017
GBIF.org (2021) GBIF home page. Available at: https://www.gbif.org (Accessed 10 August 2021).
Giménez-Benavides, L., Albert, M. J., Iriondo, J. M., Escudero, A. (2011). Demographic processes of upward range contraction in a long-lived Mediterranean high mountain plant. Ecography 34, 85–93. doi: 10.1111/j.1600-0587.2010.06250.x
Guisan, A., Thuiller, W. (2005). Predicting species distributions: Offering more than simple habitat models. Ecol. Lett. 8, 993–1009. doi: 10.1111/j.1461-0248.2005.00792.x
He, Y. P., Duan, Y. W., Liu., J. Q., Smith, W. K. (2006). Floral closure in response to temperature and pollination in Gentiana straminea maxim. (Gentianaceae), an alpine perennial in the qinghai-Tibetan plateau. Plant Syst. Evol. 256, 17–33. doi: 10.1007/s00606-005-0345-1
Hijmans, R. J. (2012). Cross-validation of species distribution models: removing spatial sorting bias and calibration with a null model. Ecology 93, 679–688. doi: 10.1890/11-0826.1
Hijmans, R. J., Cameron, S. E., Parra, J. L., Jones, P. G., Jarvis, A. (2005). Very high resolution interpolated climate surfaces for global land areas. Int. J. Climatol. 25, 1965–1978. doi: 10.1002/joc.1276
IPCC, Core Writing Team (2014). “Climate change 2014: Synthesis report”, in Contribution of working groups I, II and III to the fifth assessment report of the intergovernmental panel on climate change. Eds. Pachauri, R. K., Meyer, L. A. (Switzerland: IPCC Geneva), 151.
Jackson, L. S., Carslaw, N., Carslaw, D. C., Emmerson, K. M. (2009). Modelling trends in OH radical concentrations using generalized additive models. Atmos. Chem. Phys. 9, 2021–2033. doi: 10.5194/acp-9-2021-2009
Kaplan, J. O., New, M. (2006). Arctic Climate change with a 2 °C global warming: timing, climate patterns and vegetation change. Clim. Change 79, 213–241. doi: 10.1007/s10584-006-9113-7
Kassambara, A., Mundt, F. (2020) Factoextra: Extract and visualize the results of multivariate data analyses (R package version 1.0.7). Available at: https://cran.r-project.org/web/packages/factoextra/index.html (Accessed January 3, 2021).
Kelly, A. E., Goulden, M. L. (2008). Rapid shifts in plant distribution with recent climate change. Proc. Natl. Acad. Sci. U.S.A. 105 (33), 11823–11826. doi: 10.1073/pnas.0802891105
Lamsal, P., Kumar, L., Shabani, F., Atreya, K. (2017). The greening of the Himalayas and Tibetan plateau under climate change. Glob. Planet. Change 159, 77–92. doi: 10.1016/j.gloplacha.2017.09.010
Lenoir, J., Gegout, J., Marquet, P., de Ruffray, P., Brisse, H. (2008). A significant upward shift in plant species optimum elevation during the 20th century. Science 320, 1768–1771. doi: 10.1126/science.1156831
Lenoir, J., Svenning, J. C. (2013). “Latitudinal and elevational range shifts under contemporary climate change”, in Encyclopedia biodiversity. Ed. Levin, S. (Waltham, MA: Academic Press), 599–611. doi: 10.1016/B978-0-12-384719-5.00375-0
Liang, Q., Xu, X., Mao, K., Wang, M., Wang, K., Xi, Z, et al. (2018). Shift in plant distributions in response to climate warming in a biodiversity hotspot, the hengduan mountains. J. Biogeogr. 45, 1334–1444. doi: 10.1111/jbi.13229
Losina-Losinskaya, A. S. (1936). The genus Rheum and its species. Acta Inst. Bot. Acad. Sci. Union. Rerum. Public. Sovet. Soc Ser. 1, 5–141.
Manish, K., Telwala, Y., Nautiyal, D. C., Pandit, M. K. (2016). Modelling the impacts of future climate change on plant communities in the himalaya: a case study from Eastern himalaya, India. Model. Earth Syst. Environ. 2, 1–12. doi: 10.1007/s40808-016-0163-1
Manne, L. L., Brooks, T. M., Pimm, S. L. (1999). Relative risk of extinction of passerine birds on continents and islands. Nature 399, 258–261. doi: 10.1038/20436
Marmion, M., Parviainen, M., Luoto, M., Heikkinen, R. K., Thuiller, W. (2009). Evaluation of consensus methods in predictive species distribution modelling. Divers. Distrib. 15, 59–69. doi: 10.1111/j.1472-4642.2008.00491.x
Mclaughlin, B. C., Zavaleta, E. S. (2012). Predicting species responses to climate change: demography and climate microrefugia in California valley oak (Quercus lobata). Glob. Change Biol. 18, 2301–2312. doi: 10.1111/j.1365-2486.2011.02630.x
Meng, H., Su, T., Gao, X., Li, J., Jiang, X., Sun, H., et al. (2017). Warm-cold colonization: response of oaks to uplift of the himalaya–hengduan mountains. Mol. Ecol. 26, 3276–3294. doi: 10.1111/mec.14092
Miller, J. (2010). Species distribution modeling. Geogr. Compass 4, 490–509. doi: 10.1111/j.1749-8198.2010.00351.x
Mittermeier, R. A., Turner, W. A., Larsen, F. W., Brooks, T. M., Gascon, C. (2011). “Global biodiversity conservation: the critical role of hotspots”, in Biodiversity hotspots. Eds. Zachos, F., Habel, J. (Heidelberg, Berlin: Springer), 3–22.
Morueta-Holme, N., Engemann, K., Sandoval-Acuna, P., Jonas, J. D., Segnitz, R. M., Svenning, J., et al. (2015). Strong upslope shifts in chimborazo’s vegetation over two centuries since Humboldt. Proc. Natl. Acad. Sci. USA. 112, 12741–12745. doi: 10.1073/pnas.1509938112
Muellner-Riehl, A. N. (2019). Mountains as evolutionary arenas: Patterns, emerging approaches, paradigm shifts, and their implications for plant phylogeographic research in the tibeto-Himalayan region. Front. Plant Sci. 10. doi: 10.3389/fpls.2019.00195
Muellner-Riehl, A. N., Schnitzler, J., Kissling, W. D., Moosbrugger, V., Rijsdijk, K. F., Seijmonsbergen, A. C, et al. (2019). Origins of global mountain plant biodiversity: Testing the ‘mountain geobiodiversity hypothesis’. J. Biogeogr. 46, 2826–2838. doi: 10.1111/jbi.13715
Murphy, J. M., Sexton, D. M., Barnett, D. N., Jones, G. S., Webb, M. J., Collins, M, et al. (2004). Quantification of modelling uncertainties in a large ensemble of climate change simulations. Nature 430, 768–772. doi: 10.1038/nature02771
Myers, N., Mittermeier, R. A., Mittermeier, C. G., da Fonseca, G. A., Kent, J. (2000). Biodiversity hotspots for conservation priorities. Nature 403, 853–858. doi: 10.1038/35002501
Ohba, H. (1988). “The alpine flora of the Nepal Himalayas: an introductory note”, in The Himalayan plants, vol. vol. 1 . Eds. Ohba, H., Malla, S. B. (Tokyo: University of Tokyo Press), 19–46.
Paquette, A., Hargreaves, A. L. (2021). Biotic interactions are more often important at species’ warm versus cool range edges. Ecol. Lett. 24, 2427–2438. doi: 10.1111/ele.13864
Parmesan, C., Yohe, G. (2003). A globally coherent fingerprint of climate change impacts across natural systems. Nature 421, 37–42. doi: 10.1038/nature01286
Pearson, R. G., Raxworthy, C. J., Nakamura, M., Peterson, A. T. (2007). Predicting species distributions from small numbers of occurrence records: A test case using cryptic geckos in Madagascar. J. Biogeogr. 34, 102–117. doi: 10.1111/j.1365-2699.2006.01594.x
Pertoldi, C., Bach, L. (2007). Evolutionary aspects of climate-induced changes and the need for multidisciplinarity. J. Therm. Biol. 32, 118–124. doi: 10.1016/j.jtherbio.2007.01.011
Phillips, S. J., Anderson, R. P., Schapire, R. E. (2006). Maximum entropy modeling of species geographic distributions. Ecol. Model. 190, 231–259. doi: 10.1016/j.ecolmodel.2005.03.026
Pierce, D. W., Barnett, T. P., Santer, B. D., Gleckler, P. J. (2009). Selecting global climate models for regional climate change studies. Proc. Natl. Acad. Sci. U.S.A. 106, 8441–8446. doi: 10.1073/pnas.0900094106
Qiu, Y., Fu, C., Comes, H. P. (2011). Plant molecular phylogeography in China and adjacent regions: Tracing the genetic imprints of quaternary climate and environmental change in the world’s most diverse temperate flora. Mol. Phylogenet. Evol. 59, 225–244. doi: 10.1016/j.ympev.2011.01.012
Quinn, G. P., Keough, M. J. (2002). Experimental design and data analysis for biologists (Cambridge: Cambridge University Press), 111–154.
Quintero, I., Wiens, J. J. (2013). Rates of projected climate change dramatically exceed past rates of climatic niche evolution among vertebrate species. Ecol. Lett. 16, 1095–1103. doi: 10.1111/ele.12144
Rahbek, C., Borregaard, M. K., Antonelli, A., Colwell, R. K., Holt, B. G., Nogues-Bravo, D, et al. (2019). Building mountain biodiversity: Geological and evolutionary processes. Science 365, 1114–1119. doi: 10.1126/science.aax0151
Rana, H. K., Luo, D., Rana, S. K., Sun, H. (2020a). Geological and climatic factors affect the population genetic connectivity in Mirabilis himalaica (Nyctaginaceae): Insight from phylogeography and dispersal corridors in the himalaya-hengduan biodiversity hotspot. Front. Plant Sci. 10, 1721. doi: 10.3389/fpls.2019.01721
Rana, S. K., Rana, H. K., Ghimire, S. K., Shrestha, K. K., Ranjitkar, S. (2017). Predicting the impact of climate change on the distribution of two threatened Himalayan medicinal plants of liliaceae in Nepal. J. Mt. Sci. 14, 558–570. doi: 10.1007/s11629-015-3822-1
Rana, S. K., Rana, H. K., Luo, D., Sun, H. (2021). Estimating climate-induced ‘Nowhere to go’ range shifts of the Himalayan incarvillea juss. using multi-model median ensemble species distribution models. Ecol. Indic. 121, 107127. doi: 10.1016/j.ecolind.2020.107127
Rana, S. K., Rana, H. K., Ranjitkar, S., Ghimire, S. K., Gurmachhan, C. M., O'Neill, A. R, et al. (2020b). Climate-change threats to distribution, habitats, sustainability and conservation of highly traded medicinal and aromatic plants in Nepal. Ecol. Indic. 115, 106435. doi: 10.1016/j.ecolind.2020.106435
Ranjitkar, S., Xu, J., Shrestha, K. K., Kindt, R. (2014). Ensemble forecast of climate suitability for the trans-Himalayan nyctaginaceae species. Ecol. Model. 282, 18–24. doi: 10.1016/j.ecolmodel.2014.03.003
Retallack, G. J. (2001). Cenozoic Expansion of grasslands and climatic cooling. J. Geol. 109, 407–426. doi: 10.1086/320791
Robinson, T. P., Di Virgilio, G., Temple-Smith, D., Hesford, J., Wardell-Johnson, G. W. (2018). Characterisation of range restriction amongst the rare flora of banded ironstone formation ranges in semiarid south-western Australia. Aust. J. Bot. 67, 234–247. doi: 10.1071/BT18111
Ruiz, D., Moreno, H. A., Gutiérrez, M. E., Zapata, P. A. (2008). Changing climate and endangered high mountain ecosystems in Colombia. Sci. Total Environ. 398, 122–132. doi: 10.1016/j.scitotenv.2008.02.038
Rumpf, S. B., Hülber, K., Klonner, G., Moser, D., Schütz, M., Wessely, J, et al. (2018). Range dynamics of mountain plants decrease with elevation. Proc. Natl. Acad. Sci. U.S.A. 115, 1848–1853. doi: 10.1073/pnas.1713936115
Salick, J., Fang, Z. D., Hart, R. (2019). Rapid changes in eastern Himalayan alpine flora with climate change. Am. J. Bot. 106, 520–530. doi: 10.1002/ajb2.1263
Sandel, B., Arge, L., Dalsgaard, B., Davies, R. G., Gaston, K. J., Sutherland, W. J., et al. (2011). The influence of late quaternary climate-change velocity on species endemism. Science 334, 660–664. doi: 10.1126/science.1210173
Schickhoff, U., Bobrowski, M., Böhner, J., Bürzle, B., Chaudhary, R. P., Gerlitz, L., et al. (2014). Do Himalayan treelines respond to recent climate change? An evaluation of sensitivity indicators. Earth Syst. Dynam. Discuss., 5, 1407–1461. doi: 10.5194/esdd-5-1407-2014
Song, B., Gao, Y., Stöcklin, J., Song, M., Sun, L., Sun, H., et al. (2020a). Ultraviolet screening increases with elevation in translucent bracts of Rheum nobile (Polygonaceae), an alpine ‘glasshouse’ plant from the high Himalayas. Bot. J. Linn. Soc 193, 276–286. doi: 10.1093/botlinnean/boaa005
Song, B., Stöcklin, J., Gao, Y., Zhang, Z., Yang, Y., Li, Z., et al. (2013a). Habitat-specific responses of seed germination and seedling establishment to soil water condition in two Rheum species in the high sino-Himalayas. Ecol. Res. 28, 643–651. doi: 10.1007/s11284-013-1057-6
Song, B., Stöcklin, J., Peng, D., Gao, Y., Sun, H. (2015). The bracts of the alpine ‘glasshouse’ plant Rheum alexandrae (Polygonaceae) enhance reproductive fitness of its pollinating seed-consuming mutualist. Bot. J. Linn. Soc 179, 349–359. doi: 10.1111/boj.12312
Song, B., Stöcklin, J., Zhang, Z., Yang, Y., Sun, H. (2013b). Seed and microsite limitation in Rheum nobile, a rare and endemic plant from the subnival zone of sino-himalaya. Plant Ecol. Divers. 6 (3–4), 503–509. doi: 10.1080/17550874.2013.788568
Song, B., Stoll, P., Peng, D., Sun, H., Stöcklin, J. (2020b). Demography of the giant monocarpic herb Rheum nobile in the Himalayas and the effect of disturbances by grazing. Ann. Bot. 125, 447–458. doi: 10.1093/aob/mcz178
Song, B., Zhang, Z., Stocklin, J., Yang, Y., Niu, Y., Chen, J, et al. (2013c). Multifunctional bracts enhance plant fitness during flowering and seed development in Rheum nobile (Polygonaceae), a giant herb endemic to the high Himalayas. Oecologia 172, 359–370. doi: 10.1007/s00442-012-2518-2
Song, M., Zhou, C., Ouyang, H. (2004). Distributions of dominant tree species on the Tibetan plateau under current and future climate scenarios. Mt. Res. Dev. 24, 166–173. doi: 10.1659/0276-4741(2004)024[0166:DODTSO]2.0.CO;2
Sun, J., Qin, X., Yang, J. (2016). The response of vegetation dynamics of the different alpine grassland types to temperature and precipitation on the Tibetan plateau. Environ. Monit. Assess. 188, 20. doi: 10.1007/s10661-015-5014-4
Sun, Y., Wang, A., Wan, D., Wang, Q., Liu, J. (2012). Rapid radiation of Rheum (Polygonaceae) and parallel evolution of morphological traits. Mol. Phylogenet. Evol. 63, 150–158. doi: 10.1016/j.ympev.2012.01.002
Sun, H., Zhang, J., Deng, T., Boufford, D. E. (2017). Origins and evolution of plant diversity in the hengduan mountains, China. Plant Divers. 39, 161–166. doi: 10.1016/j.pld.2017.09.004
Thuiller, W., Georges, D., Engler, R., Breiner, F. (2020) Biomod2: Ensemble platform for species distribution modeling (R package version 3.4.12). Available at: https://cran.r-project.org/web/packages/biomod2/index.html (Accessed November 3, 2020).
Thuiller, W., Lavorel, S., Araujo, M. B., Sykes, M. T., Prentice, I. C. (2005). Climate change threats to plant diversity in Europe. Proc. Natl. Acad. Sci. U.S.A. 102, 8245–8250. doi: 10.1073/pnas.0409902102
Tsukaya, H. (2002). Optical and anatomical characteristics of bracts from the Chinese ‘glasshouse’ plant, Rheum alexandrae batalin (Polygonaceae), in yunnan, China. J. Plant Res. 115, 59–63. doi: 10.1007/s102650200009
Tsukaya, H., Tsuge, T. (2001). Morphological adaptation of inflorescences in plants that develop at low temperatures in early spring: the convergent evolution of “downy plants”. Plant Biol. 3, 536–543. doi: 10.1055/s-2001-17727
Tuanmu, M. N., Jetz, W. (2014). A global 1-km consensus land-cover product for biodiversity and ecosystem modelling. Glob. Ecol. Biogeogr. 23, 1031–1045. doi: 10.1111/geb.12182
Tuanmu, M. N., Jetz, W. (2015). A global, remote sensing-based characterization of terrestrial habitat heterogeneity for biodiversity and ecosystem modelling. Glob. Ecol. Biogeogr. 24, 1329–1339. doi: 10.1111/geb.12365
Veloz, S. D. (2009). Spatially autocorrelated sampling falsely inflates measures of accuracy for presence-only niche models. J. Biogeogr. 36, 2290–2299. doi: 10.1111/j.1365-2699.2009.02174.x
Wang, Y. (2006). Yunnan mountain climate (Chinese) Science and technology publisher (Kunming, China:Science and Technology Publisher).
Wang, X., Yao, Y., Wortley, A. H., Qiao, H., Blackmore, S., Wang, Y., et al. (2018). Vegetation responses to the warming at the younger dryas-Holocene transition in the hengduan mountains, southwestern China. Quat. Sci. Rev. 192, 236–248. doi: 10.1016/j.quascirev.2018.06.007
Warren, D. L., Dinnage, R. (2021) ENMTools: Analysis of niche evolution using niche and distribution models (R package version 1.0.3). Available at: https://cran.r-project.org/web/packages/ENMTools/index.html (Accessed June 2, 2021).
Warren, D. L., Glor, R. E., Turelli, M. (2008). Environmental niche equivalency versus conservatism: quantitative approaches to niche evolution. Evolution 62, 2868–2883. doi: 10.1111/j.1558-5646.2008.00482.x
Warren, D. L., Glor, R. E., Turelli, M. (2010). ENMTools: a toolbox for comparative studies of environmental niche models. Ecography 33, 607–611. doi: 10.1111/j.1600-0587.2009.06142.x
Warren, D. L., Matzke, N. J., Cardillo, M., Baumgartner, J. B., Beaumont, L. J., Turelli, M., et al. (2021). ENMTools 1.0: an r package for comparative ecological biogeography. Ecography 44, 504–511. doi: 10.1111/ecog.05485
Warren, D. L., Seifert, S. N. (2011). Ecological niche modeling in maxent: the importance of model complexity and the performance of model selection criteria. Ecol. Appl. 21, 335–342. doi: 10.1890/10-1171.1
Wiens, J. J. (2016). Climate-related local extinctions are already widespread among plant and animal species. PloS Biol. 14, e2001104. doi: 10.1371/journal.pbio.2001104
Williams, J. W., Jackson, S. T., Kutzbach, J. E. (2007). Projected distributions of novel and disappearing climates by 2100AD. Proc. Natl. Acad. Sci. U.S.A. 104, 5738–5742. doi: 10.1073/pnas.0606292104
Xiaodan, W., Genwei, C., Xianghao, Z. (2011). Assessing potential impacts of climatic change on subalpine forests on the eastern Tibetan plateau. Clim. Change 108, 225–241. doi: 10.1007/s10584-010-0008-2
Yang, Q. Y., Du, Z. (1990). On altitudinal land use zonation of the hengduan mountain region in southwestern China. GeoJournal 20, 69–374. doi: 10.1007/bf00174977
Yao, Y., Wang, X., Qin, F., Wortley, A. H., Li, S., Blackmore, S, et al. (2020). Evidence for climate instability during the younger dryas interval in the hengduan mountains, yunnan, southwestern China. Paleogeogr. Paleoclimatol. Paleoecol. 554, 109798. doi: 10.1016/j.palaeo.2020.109798
Yoshida, T. (2002). “Adaptive strategies of alpine plants in nepal”, in Himalayan Botany in the twentieth and twenty-first centuries. Eds. Noshiro, S., Rajbhandari, K. R. (Tokyo: The Society of Himalayan Botany), 105–111.
You, J., Qin, X., Ranjitkar, S., Lougheed, S. C., Wang, M., Zhou, W., et al. (2018). Response to climate change of montane herbaceous plants in the genus Rhodiola predicted by ecological niche modelling. Sci. Rep. 8, 5879. doi: 10.1038/s41598-018-24360-9
Zhang, J., Zhong, D., Song, W., Zhu, R., Sun, W. (2018). Climate is not all: Evidence from phylogeography of Rhodiola fastigiate (Crassulaceae) and comparison to its closest relatives. Front. Plant Sci. 9. doi: 10.3389/fpls.2018.00462
Zhao, D., Wu, S., Yin, Y., Yin, Z. (2011). Vegetation distribution on Tibetan plateau under climate change scenario. Reg. Environ. Change 11, 905–915. doi: 10.1007/s10113-011-0228-7
Zurell, D., Franklin, J., König, C., Bouchet, P. J., Dormann, C. F., Elith, J., et al. (2020). A standard protocol for reporting species distribution models. Ecography 43, 1261–1277. doi: 10.1111/ecog.04960
Keywords: biodiversity hotspots, biomod2, climate change, glasshouse, range shifts, Rheum
Citation: Rana SK, Rana HK, Stöcklin J, Ranjitkar S, Sun H and Song B (2022) Global warming pushes the distribution range of the two alpine ‘glasshouse’ Rheum species north- and upwards in the Eastern Himalayas and the Hengduan Mountains. Front. Plant Sci. 13:925296. doi: 10.3389/fpls.2022.925296
Received: 21 April 2022; Accepted: 15 September 2022;
Published: 07 October 2022.
Edited by:
Boris Rewald, University of Natural Resources and Life Sciences Vienna, AustriaReviewed by:
Robbie Hart, Missouri Botanical Garden, United StatesJinlong Zhang, Kadoorie Farm and Botanic Garden, Hong Kong SAR, China
Copyright © 2022 Rana, Rana, Stöcklin, Ranjitkar, Sun and Song. This is an open-access article distributed under the terms of the Creative Commons Attribution License (CC BY). The use, distribution or reproduction in other forums is permitted, provided the original author(s) and the copyright owner(s) are credited and that the original publication in this journal is cited, in accordance with accepted academic practice. No use, distribution or reproduction is permitted which does not comply with these terms.
*Correspondence: Bo Song, c29uZ2JvQG1haWwua2liLmFjLmNu; Hang Sun, c3VuaGFuZ0BtYWlsLmtpYi5hYy5jbg==
†These authors have contributed equally to this work