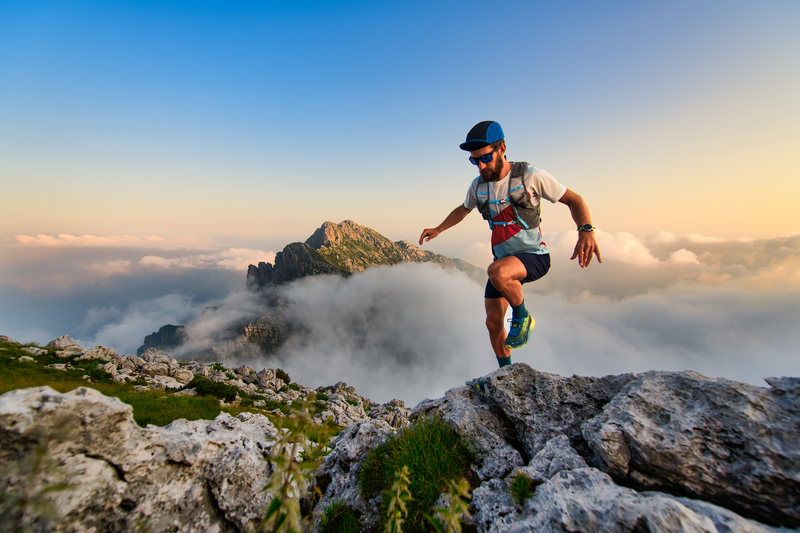
94% of researchers rate our articles as excellent or good
Learn more about the work of our research integrity team to safeguard the quality of each article we publish.
Find out more
ORIGINAL RESEARCH article
Front. Plant Sci. , 22 July 2022
Sec. Plant Pathogen Interactions
Volume 13 - 2022 | https://doi.org/10.3389/fpls.2022.907819
This article is part of the Research Topic Fusarium Pathogenesis: Infection Mechanisms and Disease Progression in Host Plants View all 9 articles
Nitrogen (N) fertilizers are routinely applied to bananas (Musa spp.) to increase production but may exacerbate plant diseases like Fusarium wilt of banana (FWB), which is the most economically important disease. Here, we characterized the effects of N rate and form on banana plant growth, root proteome, bacterial and fungal diversity in the rhizosphere, the concentration of Fusarium oxysporum f.sp. cubense (Foc) in the soil, and the FWB severity. Banana plants (Musa subgroup ABB) were grown under greenhouse conditions in soil with ammonium or nitrate supplemented at five N rates, and with or without inoculation with Foc. The growth of non-inoculated plants was positively correlated with the N rate. In bananas inoculated with Foc, disease severity increased with the N rate, resulting in the Foc-inoculated plant growth being greatest at intermediate N rates. The abundance of Foc in the soil was weakly related to the treatment conditions and was a poor predictor of disease severity. Fungal diversity was consistently affected by Foc inoculation, while bacterial diversity was associated with changes in soil pH resulting from N addition, in particular ammonium. N rate altered the expression of host metabolic pathways associated with carbon fixation, energy usage, amino acid metabolism, and importantly stress response signaling, irrespective of inoculation or N form. Furthermore, in diseased plants, Pathogenesis-related protein 1, a key endpoint for biotic stress response and the salicylic acid defense response to biotrophic pathogens, was negatively correlated with the rate of ammonium fertilizer but not nitrate. As expected, inoculation with Foc altered the expression of a wide range of processes in the banana plant including those of defense and growth. In summary, our results indicate that the severity of FWB was negatively associated with host defenses, which was influenced by N application (particularly ammonium), and shifts in microbial communities associated with ammonium-induced acidification.
The application of synthetic nitrogen (N) fertilizer has enabled humans to dramatically increase agricultural productivity (Erisman et al., 2008). Worldwide use of synthetic N fertilizer is projected to increase to 112 million tonnes in 2022 (FAO, 2019). The use of N fertilizer has substantial benefits, but also some detrimental effects. Excessive N use has been found to increase the severity of diseases such as Fusarium wilt of banana (FWB) (Pittaway et al., 1999; Mur et al., 2017; Segura-Mena et al., 2021). Disease severity is governed by the three-way relationship between host, pathogen and the environment (Agrios, 2005), with N supply an especially important environmental aspect in the context of FWB.
Banana farmers apply N fertilizer to maximize productivity, potentially rendering their plants vulnerable to pathogens such as F. oxysporum f.sp. cubense (Foc), the soil-borne, hemibiotrophic, fungal pathogen responsible for FWB. The association between N fertilization and increased FWB severity remains unclear, as N fertilizers have multiple effects on soils, including changes to nutrient availability, porosity, and pH (Marinari et al., 2000; Neumann and Römheld, 2012). The main forms of N fertilizer used are ammonium, urea, and nitrate, with ammonium and urea being most common due to their lower price. Current evidence indicates that nitrate fertilizer reduces Fusarium wilt severity, whereas ammonium increases it (Woltz and Engelhard, 1973; Jones et al., 1975; Morgan and Timmer, 1984; Woltz et al., 1992; Wang et al., 2016; Zhou et al., 2017) though there are exceptions (Jarvis and Thorpe, 1980). This effect of N form on disease may be due to the acidifying effect of ammonium fertilizer and alkalizing effect of nitrate fertilizer, as soil acidification increases FWB severity (Orr and Nelson, 2018; Teixeira et al., 2021; Segura et al., 2022). Indeed, field trials have sown a greater influence of N fertilizer form on FWB severity at low pH than at high (Jones et al., 1975). Segura-Mena et al. (2021) found that the addition of ammonium nitrate fertilizer increased FWB severity, but less so at pH 5.1 than at 6.1. Only one soil-based study, performed on tomatoes, maintained similar pH between different N fertilizer forms and found nitrate to be significantly more suppressive to disease than ammonium (Woltz et al., 1992), suggesting a mechanism independent of pH. To understand the role of N in the management of FWB it is thus important to consider the effects of N fertilizer form and dose and the resulting change in pH.
Greater N availability has been proposed to cause a growth-defense trade-off in plants to maximize fitness (Herms and Mattson, 1992). Plants that grow more rapidly due to an abundance of N must sacrifice defensive capabilities due to greater metabolic expenditure, leaving them more susceptible to disease (Huot et al., 2014; Neuser et al., 2019). Pittaway et al. (1999) examined the relationship between FWB severity and pathogen invasion and activity under N-fertilized conditions. They hypothesized that the observed increase in disease severity was likely due to a predisposition of the host plant to disease, through a reduction in defense response, rather than changes in the pathogen. If the growth-defense trade-off is responsible for increased banana susceptibility to FWB in the future it may be possible to mitigate the effect through gene manipulation (Wang et al., 2021).
The diversity and biomass of soil bacterial and fungal communities, excluding the pathogen, generally are negatively correlated with the N fertilizer rate (Wang et al., 2018; Yang et al., 2020; Zhou et al., 2020). A reduction in microbial diversity and biomass with increased N fertilizer application is largely attributable to changes in soil pH (Wang et al., 2018; Yang et al., 2020). FWB is less severe in fields with high microbial diversity, possibly due to direct antagonism, resource competition, or support for the host plant defense (Shen et al., 2015; Fu et al., 2017; Rames et al., 2018; Zhou et al., 2019), so the application of N, which reduces microbial diversity of the soil, may increase disease severity. Therefore, it is important to consider both the rate of N applied and the form of fertilizer used.
The aim of this work was to determine the effects of inoculation with Foc, N rate and N form on the severity of FWB and the components of the FWB disease triangle. In controlled conditions, we examined the response of each component of the disease triangle: the pathogen (abundance in the rhizosphere by real-time qPCR), environment (rhizosphere soil chemistry and soil fungal and bacterial population diversity using ITS2 and 16S rRNA gene amplicon sequencing), and host (above- and below-ground growth, tissue N content and isotopic composition, and functional protein content of roots, using SWATH quantitative proteomics).
The top 30 cm of a Liverpool series soil (Dermosol, Murtha, 1986) was collected from a commercial banana (Musa [AAA Group, Cavendish Subgroup] “Williams”) farm that had applied a maximum of 160 kg N/ha/year for the previous seven years. This location was selected to limit the presence of residual N fertilizer. Abiotic characterization of this soil (Site 5, Liverpool) has been published previously as part of a regional survey (Orr and Nelson, 2021). To prevent the spread of soil-borne disease, buckets of soil were sealed for transport and moved to the greenhouse where the experiment was conducted. Transport was at ambient temperature and took ~2 h. The soil was sieved to 10 mm to remove rocks, sticks, and large roots and then homogenized before potting.
To each 4 L pot, 4 kg (dry weight equivalent) of soil was added. Foc Race 1-susceptible in vitro propagated banana plantlets (Musa [ABB Group, Pisang Awak Subgroup] “Ducasse”; Maroochy Research Facility, Maroochydore, Queensland) ~5 cm tall, were decanted and planted directly into soil. After 36 days, ammonium, as ammonium sulphate, or nitrate, as potassium and calcium nitrate, fertilizer were each applied at five rates (6.0, 12.1, 30.2, 48.3, and 66.4 mg N/pot/fortnight; equivalent to field rates of 50, 100, 250, 400, and 550 kg/ha/yr) to each pot. Potassium = 88.1, phosphorus = 6.64, calcium = 36.0, and sulphur = 84.1 mg/pot/fortnight were applied uniformly across the treatments, however the rate of chloride = 72.3 in ammonium pots and 57.0 mg/pot/fortnight in nitrate pots, and sodium differed between treatments (0–139 mg/pot/fortnight).
Fertilizers were applied in dissolved form by saturating the soil and then draining off the solution in excess of the soil's water holding capacity. This method allowed the experiment to be carried out in the soil while mimicking some of the benefits of hydroponic systems by redistributing solutes between rhizosphere and bulk soil (which deviated between fertilizer applications due to root activity), equilibrating soil water content between pots (which deviated between pots due to differential transpiration) and removing nitrate (generated by nitrification) from ammonium-treated pots. The water holding capacity of each pot was determined by saturating the known dry weight of soil in each pot with water and then allowing it to drain freely for 24 h and was no longer dripping before reweighing. Prior to each fertilizer application, each pot was watered to 200 ml below water holding capacity by weight. Then the pot was soaked in a fertilizer solution with the correct amount of fertilizer per 200 ml. The pot contents would absorb 200 ml of solution, through small holes at the bottom of the pot over a period of ~5 min, bringing the pot contents to their water holding capacity and required fertilization. Then the pot was removed from the fertilizer solution and allowed to freely drain for 24 h before weighing. The difference between the weight prior to immersion, and that after freely draining was used to confirm the amount of fertilizer retained. The pot weight after free draining was also used to calculate the water required prior to fertilizer addition for the next fertilizer addition 14 days later.
After 61 days, 7.5 ml of millet inoculated with a mixture of two Foc isolates- (Race 1, VCG 0124 [BRIP 43996 and BRIP61873]; Queensland Plant Pathology Herbarium) was added to the inoculated treatment pots. Inoculated millet was prepared following the method of Warman and Aitken (2018) except the millet was not ground prior to inoculation of the pots. Autoclave-sterilized millet was added to the remaining pots, resulting in 20 unique treatment combinations. Inoculated treatments were replicated 5 times, to account for the greater variability of incorporating an extra treatment, and non-inoculated 4 times (n = 90). The millet was mixed into the top 20 mm of soil in each pot, ensuring not to cross contaminate the treatments. All other soil conditions, including temperature and water supply, were consistent between treatments.
Soil samples for pH, nitrate, and ammonium analysis were collected 1, 36, and 52 days after inoculation. Samples for root proteome and rhizosphere soil qPCR and amplicon sequencing were collected 52 and 53 days after inoculation by gently removing the plant and roots from the pot and taking the sample before replanting the plant and remaining roots in the pot. These samples were taken prior to plant harvest to ensure the plant stress response was not due to harvest. Plants were harvested over a 2-day period, 56 and 57 days after inoculation, after severe leaf yellowing and pseudostem splitting had been observed in some plants.
At harvest, the roots were separated from the rhizome and kept separate to minimize soil contamination. The rhizome was sectioned for the determination of disease severity (see below). The above-ground (including rhizome) and root sections were weighed for fresh weight, dried at 60°C until a constant dry weight was achieved, and reweighed to determine the dry weight and water content of the tissue. The entire dried above-ground section of the plant was then ground to a fine powder and homogenized prior to analysis of total N and carbon (C) concentration, and stable isotope composition.
Internal disease severity was determined using the method of Orr et al. (2019). Briefly, after the removal of the roots, the rhizome was laterally sectioned into quarters and the upper side of each section was photographed. Each pixel of the section photographs was classified as either diseased or not diseased using the image analysis program ImageJ v1.52a (Schneider et al., 2012) and a reference grayscale. For sections where the upper side of the lateral section mistakenly included pseudostem tissue rather than the rhizome, a value was not recorded. The proportion of the total rhizome pixels classified as diseased was used as a percentage of disease severity for each rhizome section. The values for each rhizome section were then averaged to provide a disease severity value for that plant.
Soil samples were collected 1, 36, and 52 days after inoculation. A 10 mm internal diameter, 300 mm length, stainless steel tube was inserted to the complete depth of the pot to collect a core. The tube was cleaned and sterilized between samples to avoid cross-contamination. Each core was homogenized and 4 g (dry weight equivalent) of the sample was end-over-end shaken for 1 h with 40 ml of 0.1 M potassium chloride before centrifuging at 3,000 × g for 5 min. After centrifuging the supernatant pH was measured (Ionode IH series probe; Ionode Pty Ltd. Australia) and subsamples of the supernatant were taken for nitrate and ammonium analysis.
Nitrate analysis followed Hach method 8171 (Hach, 2017), using a linear six-point calibration curve from analytical grade standards (Hach, Australia). Ammonium was determined using the Hach method 8038 (Hach, 2017), also using a linear six-point calibration curve from analytical grade standards (Hach Australia).
Total N concentration of the plant (aboveground portion) was measured to determine N uptake, and δ15N was measured to determine if the treatments influenced the proportion of N taken up directly from fertilizer vs. soil or microbially processed N (Denk et al., 2017). The δ13C of the same samples was measured to determine effects on water use efficiency (Cernusak et al., 2013). C and N concentrations, and δ13C and δ15N values of the plant, soil and fertilizer samples were determined using a Costech Elemental Analyzer fitted with a zero-blank auto-sampler coupled via a ConFloIV to a ThermoFinnigan DeltaVPLUS using Continuous-Flow Isotope Ratio Mass Spectrometry (EA-IRMS) at James Cook University's Advanced Analytical Centre (Cairns). Stable isotope results are reported as per mille (‰) deviations from the VPDB and AIR reference standard scale for δ13C and δ15N values, respectively. The standard deviation on internal standards was <0.1 and 0.2% for C and N, respectively. As δ15N differed between the fertilizers used (ammonium sulfate, sodium nitrate, and potassium nitrate), the treatment effect on the isotopic composition of plant material was calculated using δ15NPlant–δ15NFert.
Rhizospheric soil was collected based on previously published methods (Birt et al., 2021, 2022). Briefly, ~70 mm long sections from the apex of three white roots of each plant, with rhizosphere soil attached, were cut with methanol-sterilized scissors and stored at 4°C until processing on the same day. Samples were sonicated in 40 ml phosphate-buffered saline solution to loosen soil and fine roots. Large roots were then removed and the remaining soil suspension was centrifuged for 15 min at 400 × g before removal of the supernatant. The remaining soil pellet (representing the rhizosphere) was frozen at −18°C until DNA extraction the following week.
DNA was extracted using a Qiagen DNeasy Powersoil kit (Qiagen, Australia) according to manufacturer recommendations. The amount of soil extracted for each sample was weighed precisely for use in calculating DNA concentrations in soil. The extracted rhizosphere soil DNA sample was then used for both Foc R1 qPCR and amplicon sequencing.
To survey bacterial communities, 16S rRNA genes were amplified by PCR using the primers 926wF (5′-AAA CTY AAA KGA ATT GRC GG-3′) and 1392R (5′- ACG GGC GGT GWG TRC−3′) (Lane, 1991). Fungal communities were surveyed using the ITS2 rRNA gene primers gITS7 (5′- GTG AAT CAT CGA ATC TTT G-′3) (Ihrmark et al., 2012) and ITS4 (5′- TCC TCC GCT TAT TGA TAT GC-′3) (White et al., 1990). Thermocycling conditions were as follows for all reactions: 98°C for 45 s; then 35 cycles of 98°C for 5 s, 56°C for 5 s, 72°C for 6 s; followed by 72°C for 1 min. Each PCR reaction contained 2 μL DNA of the sample in 5X Phire Green Reaction Buffer (Thermo Fisher), 0.4 μl Phire Green Hot Start II DNA Polymerase (Thermo Fisher), 100 μM of each of the dNTPs (Invitrogen), and 10 mM of each primer. The remaining volume was made to 20 μl with molecular-grade water. A Simpliamp® 96-well Thermocycler (Applied Biosystems) was used to perform PCR reactions. Gel electrophoresis was used to verify blank extraction controls and negative amplification controls.
Magnetic beads (Rohland and Reich, 2012) were used to purify amplicons, which were then dual indexed using the Nextera XT Index Kit (Illumina) according to the manufacturer's instructions. Indexed amplicons were again purified using magnetic beads and quantified using a PicoGreen dsDNA Quantification Kit (Invitrogen). Samples were pooled in equal concentrations and sequenced on an Illumina MiSeq using 30% PhiX Control v3 (Illumina) and a MiSeq Reagent Kit v3 (600 cycles, Illumina) according to the manufacturer's instructions.
Sequence data were processed using a modified UPARSE approach (Edgar, 2013). Firstly, reads were demultiplexed and barcodes removed using the cutadapt tool in QIIME2 (v2017.9.0; Zhang et al., 2000. ITS2 or 16S sequences were then each processed according to a different protocol. For 16S sequences, primers were then removed and trimmed to 250 bp using fastx_truncate of USEARCH (Edgar, 2010). Trimmed reads were then quality filtered using fastq_filter of USEARCH with a maxee score of 1.0. For ITS2 sequences, ITSx (Bengtsson-Palme et al., 2013) was used to extract the ITS2 region with fungi as the specified profile. Chimeras were then removed from the extracted sequences using uchime2_ref of USEARCH against the UNITE 8.2 database (Nilsson et al., 2019). ITS2 and 16S sequences then resumed with the same pipeline after these steps had been completed. Representative sequences were generated using fastx_uniques and cluster_otus of USEARCH with a sequence similarity of 0.97. These representative sequences were used to create an operational taxonomic unit (OTU) table by mapping the remaining reads using the otutab function in USEARCH. Taxonomy was assigned to each OTU using blastn from QIIME2 against the SILVA 128 (Quast et al., 2013) database for 16S sequences and UNITE 8.2 database for ITS2 sequences. Sequences that were not either bacteria or fungi were then removed using taxa filter-table from QIIME2. The 16S sequences were then aligned using MAFFT (Katoh and Standley, 2013) and masked using QIIME2 to generate phylogenetic distance. A midpoint-rooted phylogenetic tree was generated from the alignment using FastTree (v2.1.9) (Price et al., 2010). 16S samples were rarefied to 6950 reads, ITS samples were rarefied to 13,500. Alpha diversity metrics were produced using QIIME2.
Foc abundance was quantified with absolute quantification-based qPCR as previously published (Matthews et al., 2020), except for the use of SsoAdvanced SYBR® SuperMix (BioRad), which has been shown to increase reaction efficiency compared to other qPCR master mixes (Browne et al., 2020). Briefly, Foc-specific DNA sequences were targeted using the forward priming sequence (5′-GACATTTGACGACTTTCTGA-3′), the reverse sequence (5′-GACATTTGACGACTTTCTGA-3′) (Matthews et al., 2020). Each 10 μl total-volume reaction contained 5 ng of extracted DNA, 0.3 μM of desalt-grade primers (Sigma-Aldrich), 5 μl of SsoAdvanced SYBR® SuperMix, and 2 μl molecular grade Ultra-Pure H2O™ (Invitrogen) water. Samples were amplified in a reaction including an initial hot start of 10 min at 95°C, followed by 40 cycles of 10 s at 95°C and 15 s at 66°C. Reactions were followed by a melt curve analysis to ensure primer specificity. Furthermore, technical triplicates were run alongside no template negative controls as well as a sample, extraction, and analysis blank. The entire dilution and technical triplet analysis were also run 2 times to check dilutions. Values from the two dilution sets were consistent and values were averaged. The reaction amplification efficiency was calculated from the gradient of the standard curve titration in accordance with MIQE guidelines (Bustin et al., 2009). Cycle threshold (Ct) scores were converted to DNA copy numbers using a standard curve constructed from a pGEM-T plasmid carrying the Foc specific DNA directed RNA polymerase subunit III gene (i.e. 108−101 copies plasmid/reaction). The target amplicon was 98 base pairs long, reaction efficiency was 98.1%, and standard curve R2 was 0.99. Reactions were measured by QuantStudio 5 Real-Time PCR Machine running QuantStudio Design and Analysis Software (v1.4.3, Applied Biosystems).
When sampling each plant, three healthy root ends (~5 cm long) were cleaned with deionized water to remove soil while attached to the plant, cut, immersed in liquid N, and ground to a fine powder in a liquid-N-cooled mortar and pestle. Samples were then stored at −80°C until processing. Between samples, all surfaces were thoroughly cleaned with methanol to prevent cross-contamination. Peptides were extracted using an acid digestion method adapted from the work of Doellinger et al. (2020). For the full method used, see Supplementary Information 1.
All samples were analyzed with a Ekspert nano-LC415 (Eksigent, USA) liquid chromatography (LC) system running on the water with 0.1% (v/v) formic acid in water (Solvent A) and 0.1% (v/v) formic acid in Acetonitrile (Solvent B), coupled to a TripleTOF 6600 (Sciex, USA) mass spectrometer (MS). Digested peptides were first loaded on a C18 10 mm by 300 μm trap column (Trajan, Australia) under 10 μl/min of Solvent A and separated on a C18 250 mm by 300 μm column (Trajan, Australia) with a linear gradient of 3–35% solvent B over 75 min at 5 μl/min. Mass spectrometer experimental parameters (Sciex software units) were as follows: curtain gas = 35, ion source gas 1 = 25, ion source gas 2 = 30, ionspray voltage floating = 500, and turboheater temperature = 300°C.
A 0.2 μl aliquot of a replicate from each treatment was pooled into two samples and loaded into the LC-MS system. Information Dependent Acquisition mode was set to capture ions with a charge state between 2 and 5 and at a window of 350–750 Da and 745–1,250 Da respectively. A single spectral library was generated from the MS data from both windows for all conditions with the ProteinPilot (Sciex, USA) Paragon method identification workflow under the following settings: Cysteine Alkylation = Iodoacetamide w other Cys mods possible, Digestion = Trypsin, Instrument = TripleTOF 6600, Search effort = Through, ID Focus = Biological modifications, and Unused ProtScore = 0.05. The MS data was matched against a Musa acuminata subsp. malaccensis protein library containing 45,856 protein sequences, obtained from the Banana genome hub in January 2020 (Droc et al., 2013).
The same physical set-up was used in “Sequential Windowed Acquisition of All Theoretical Fragment Ion Mass Spectra” (SWATH) mode to acquire quantitative data for all 90 samples. The SWATH method used 100 windows with 6-50 Da of isolation width, an overlap of 1 Da, and a collision energy spread of 5-10 through the mass range of 399.5 Da to 1249.5 Da. Data was collected over 1,847 cycles with an accumulation time of 25 ms for each window.
SWATH data was processed using the SWATH microapp on the PeakView software (Sciex, USA). The retention time for all SWATH samples was normalized with five selected peptides from the iRT calibrant (Biognosys, Switzerland) ranging from 487.26 to 699.34 m/z. All samples were processed with the previously generated spectral library according to the following SWATH microapp settings: number of peptides per protein = 9, number of transitions per peptide = 9, peptide confidence threshold = 99%, false discovery rate threshold = 1%, XIC extraction window = 20 mins, and XIC width = 50 ppm.
Unless otherwise specified, data were analyzed using an ANCOVA with the ANOVA function in the car package in R software (R Core Team, 2017; Fox and Weisberg, 2019). This method was selected to address the unbalanced study design with respect to inoculation, incorporating type 3 sum of squares error. The predictors included N rate, considered as a continuous variable, N form, a factor with two levels (ammonium and nitrate), and sometimes inoculation when considering both inoculated and non-inoculated plants. The normality of the model residuals was tested using the Shapiro Wilks test and data was transformed if needed. Soil nitrate concentration and fungal Chao1 index were loge(x+1) transformed, plant N tissue concentration and rhizosphere Foc DNA concentration were square root transformed, and the fungal Shannon index was cubed. Soil ammonium concentration and bacterial and fungal Simpson indexes were not normally distributed even when transformed so they were analyzed using non-parametric methods with the ARTool package (Kay et al., 2021) with N rate and form considered as factors. Untransformed data are presented in figures for ease of interpretation. For determining a significant effect in the tested models α = 0.05 was used, unless otherwise specified.
The effects of the treatments on disease severity were analyzed using a beta regression analysis with a logit link in R using the betareg package (Cribari-Neto and Zeileis, 2010). This was selected due to disease severity being a percentage, with a maximum of 100%. Effects on inoculated plant dry weight were analyzed using an ANOVA, but a second order polynomial function was incorporated for the N rate. For both disease severity and inoculated plant weight, a single outlier value was excluded as it was three standard deviations from the mean. The replicate was not an outlier in other analyses possibly indicating an analytical error.
To calculate the treatment effects on microbial beta diversity a PERMANOVA analysis was performed using the adonis function in the vegan R package (Oksanen et al., 2019). For determining a significant effect in the tested models α = 0.05 was used, unless otherwise specified.
Proteomic data were quantile normalized and log2 (x+1) transformed for analysis (Ritchie et al., 2015). PERMANOVA was used to determine the significance of treatments and soil pH on protein expression composition. Redundancy analysis was performed using the vegan package in R (Oksanen et al., 2019), constrained by the treatments of N rate and inoculation as these were found to be significant. Differentially expressed proteins were identified and enrichment was calculated in the limma package in R with a false discovery rate cut-off of 0.10 incorporating a Benjamini–Hochberg correction for multiple testing (Ritchie et al., 2015; Law et al., 2020) using a full model incorporating the treatments of inoculation, N rate and form as well as all interactions. Pathway enrichment was then explored on each main or interaction treatment protein set. Further testing was performed on the expression rates of key individual defensive genes using a full treatment model. Due to concerns regarding the assumption of linearity for protein expression changes, N was also considered as a multilevel factor; however, the results were simply a subset of the linear regression analysis due to the reduction in statistical power so were not reported here.
Gene ontology enrichment was calculated using the topGO package in R (Alexa and Rahnenfuhrer, 2020). Protein IDs were mapped to gene ontology using the Banana Genome Hub (Droc et al., 2013). Fisher's exact test was used to determine enrichment using conservative cut offs (α = 0.01 and >3 proteins per ontology). KEGG pathway enrichment was calculated by annotating the full list of inferred genes analyzed in this experiment using the FASTA sequence blast function in BlastKoala as part of the KEGG mapping service (Kanehisa et al., 2016). KEGG k values from the full experimental protein set were mapped to pathway ko numbers to indicate the protein universe within the samples. Pathway enrichment was determined using a hypergeometric test for each KEGG pathway. This test compared the number of k values (indicating individual proteins or genes) in the differentially expressed protein set with the number of k values in the protein universe. Here, the protein universe was limited to proteins analyzed in this experiment, rather than the entire banana proteome (Bessarabova et al., 2012). Cut offs for enrichment were the same as for gene ontology.
The rate of N fertilizer application significantly affected plant biomass as well as FWB severity (Figure 1; Table 1). Plant dry weight in non-inoculated plants responded linearly to the N rate (Figure 1). Disease severity was significantly related to the N rate in inoculated plants, the rate of increase being constrained by the maximal (100%) internal disease. The combined effects on plant growth and disease severity resulted in a second order polynomial relationship between N rate and plant weight in inoculated plants (Figure 1).
Figure 1. The effect of nitrogen fertilizer rate on total plant dry weight in plants that were not inoculated with Fusarium oxysporum f.sp. cubense (A), disease severity in inoculated plants (B) and dry weight in inoculated plants (C). For regression model statistics see Table 1. Points have been jittered in the x dimension to avoid overplotting.
Table 1. The model outputs for the relationship between plant weight and treatments for plants inoculated with Fusarium oxysporum f.sp. cubense (quadratic) and not inoculated (linear) as shown in Figure 1.
The concentration of ammonium in soil was unaffected by the rate, form, or interaction of rate and form of N fertilizer used, whereas the concentration of nitrate in soil was positively correlated with the N application rate (F(1,86) = 44.9, p < 0.001), but not the form or the interaction of rate and form (Figure 2). Soil pH was significantly affected by the interaction of rate and form (F(1,86) = 51.4, p < 0.001) with ammonium addition decreasing soil pH (Figure 2).
Figure 2. The effect of ammonium and nitrate fertilizer addition on the concentration of soil ammonium (A), nitrate (B), and pH (C) at the time of harvest.
The N concentration of aboveground plant tissue increased significantly with N fertilizer application rate [0.87−3.28 %, F(1,82) = 128.7, p < 0.001], but was not significantly affected by N fertilizer form, inoculation, or any interactions. The difference between δ15NPlant and δ15NFert was significantly affected by N rate [F(1,82) = 228.7, p < 0.001], N form [F(1,82) = 100.2, p < 0.001], and the interaction of N rate and form [Figure 3, F(1,82) = 31.7, p < 0.001], but not inoculation or any other treatment combinations. When fertilizer rates were low, δ15NPlant values were similar to that of unfertilized soil, but as fertilizer rates increased δ15NPlant decreased, approaching that of the fertilizer (difference of zero). δ13C was significantly increased by N rate [Figure 3, F(1,82) = 62.7, p < 0.001], but no other treatments or combinations.
Figure 3. (A) The effect of nitrate and ammonium fertilizer addition on the δ15N of aboveground plant tissue, showing values for the original soil (dotted line) and fertilizer (large colored points for each fertilizer type and rate, red = ammonium, blue = nitrate). (B) δ13C of aboveground plant tissue. Points have been jittered in the x dimension to avoid overplotting.
The concentration of Foc DNA in rhizosphere soil of inoculated pots had no relationship with banana internal disease severity (Figure 4). Foc DNA concentration was not significantly affected by N rate, form, or their interaction but was marginally negatively related to soil pH [F(1,48) = 2.8, p = 0.098] (Figure 4).
Figure 4. Fusarium oxysporum f.sp. cubense DNA concentration in banana rhizosphere soil in relation to nitrogen fertilizer form and (A) Internal disease severity of banana plants, (B) Nitrogen fertilizer rate, and (C) Soil pH.
Bacterial alpha diversity was significantly decreased by N rate, but not significantly affected by N form or inoculation (Sobs, Chao1, Shannon, Simpson, and PD indices) (Figure 5, Supplementary Information 2). An ANOVA with N form and either soil pH or N rate was run to test the relative influence of soil pH and N rate on Shannon diversity, as well as the importance of the interaction of these terms. Both soil pH and N rate were significant predictors of Shannon diversity but soil pH was stronger. In addition, there was a significant interaction between N rate and N form (F = 4.3, p = 0.042) but not between soil pH and N form (F = 1.4, p = 0.234). Bacterial beta diversity, as tested with a PERMANOVA, was significantly affected by the main effects of inoculation (F = 1.5, R2 = 0.017, p < 0.001), N rate (F = 2.2, R2 = 0.024, p < 0.001) and form (F = 1.9, R2 = 0.020, p < 0.001), as well as the interactions of N rate and form (F = 1.7, R2 = 0.018, p < 0.001) and N rate and inoculation (F = 1.2, R2 = 0.012, p = 0.042). Soil pH and N form both significantly affected bacterial beta diversity but their interaction was not significant (F = 1.1, R2 = 0.012, p = 0.265), whereas when N rate and N form were considered, the interaction was significant (F = 1.7, R2 = 0.018, p < 0.001).
Figure 5. Bacterial alpha diversity (Shannon index) as affected by the rate and form of nitrogen fertiliser addition (A) and soil pH (B). Points in the left panel have been jittered in the x dimension to minimize overplotting.
Fungal beta diversity was affected by inoculation (F = 6.8, R2 = 0.072, p < 0.001; Figure 6), but was not significantly affected by N rate, N form, or any interactions. The species F. solani was most representative of uninoculated samples while the fungal OTU most affected by inoculation was the genus Fusarium (Figure 6). Fungal alpha diversity measures that considered only richness (Chao1 and Sobs) were not affected by inoculation; however, those that also considered evenness (Shannon and Simpson) were indicating that some OTUs were reduced in abundance but not removed in entirety (Supplementary Information 2).
Figure 6. A redundancy plot of the fungal community with inoculation of Fusarium oxysporum f.sp. cubense (Y) or not (N) constrained by inoculation. Circles represent samples and crosses OTUs. The far-left cross represents the genus Fusarium, which exerts considerable influence on the significance of the treatment effect.
A full factorial PERMANOVA analysis of the proteome dataset considering inoculation, N rate and form demonstrated a large effect of inoculation (F = 6.0, R2 = 0.059, p < 0.001), and N rate (F = 6.0, R2 = 0.060, p < 0.001) and the interaction of all three treatments was significant (F = 2.0, R2 = 0.019, p < 0.027). N form and the other interactions were not significant (Figure 7). When soil pH was included in the model it was not significant, nor were any interactions with it.
Figure 7. Redundancy analysis of the proteomic output constrained by factors of inoculation, nitrogen form and nitrogen rate. Point colours indicates nitrogen rate and ellipses with text labels indicate combinations of inoculation (Y or N) with nitrogen form (Ammonium or Nitrate).
Examination of differential expression on the individual protein level showed that the main effects of N rate and inoculation had the greatest effect, but there was no main effect of N form. N form and inoculation had the sole interaction with differential expression though none of the identified proteins were related to growth or defense. Therefore, further investigation was focused on those proteins differentially expressed under the main effects of N rate and inoculation. The false discovery rate used for differential expression was highly conservative due to the large number of tests performed and the multiple testing adjustments required. A separate investigation of key defensive proteins included all treatments and their interactions.
Inoculation with Foc resulted in differential expression of the proteins associated with the “Defense response” process as well as a wide range of other molecular functions (Supplementary Information 3). KEGG pathway analysis identified differentially expressed groups of proteins primarily related to the metabolism of sugars (Supplementary Information 3).
Gene ontology and KEGG pathway enrichments affected by N rate were primarily processes and functions associated with energy fixation and use (Tables 2, 3). The KEGG MAPK signaling pathway was also affected, indicating that the addition of N may have altered the plants' ability to respond to biotic and abiotic stressors (Table 3).
Table 3. Significantly differently expressed KEGG pathways, based on proteins that were differentially related to nitrogen rate.
Pathogenesis-related protein 1 (PR1) expression rates were examined due to their importance as late-stage biotic infection response proteins. Inoculation significantly increased the expression of all three PR1 proteins (Table 4; Figure 8). All three forms of PR1 showed strong and complex interactions between the three treatments so the N rate response was examined separately for each combination of inoculation and N form. Increased N rate significantly reduced PR1 expression in inoculated plants treated with ammonium but there was not a significant effect of N rate on inoculated plants treated with nitrate, nor on disease-free plants fertilized with either form of N (Figure 8).
Table 4. Effects of inoculation, nitrogen (N) rate and N form on the three forms of Pathogenesis related protein 1 measured.
Figure 8. Log2 transformed expression rates of measured forms of pathogenesis related protein 1 (PR1), shown with gene locations, with treatments of inoculation with Fusarium oxysporum f.sp. cubense, nitrogen rate and form.
Disease severity, determined here by the proportion of plant tissue that was necrotic, was examined in response to Foc infection, N fertilizer rate and form, and the interaction of these treatments. In Foc inoculated plants, disease severity was positively correlated with N fertilizer rate in either N form irrespective of changes to soil pH, resulting in curvilinear growth response to increased N (Figure 1). This result agrees with previous findings that N is positively correlated with disease severity of both Foc Race 1 and Tropical Race 4 (Segura-Mena et al., 2021; Teixeira et al., 2021), though disagrees with the general trend that acidification associated with the ammonium use increases Fusarium wilt and FWB severity, whereas nitrate use is protective (Orr and Nelson, 2018). δ13C was also positively associated with the N rate in both inoculated and disease-free plants indicating that water use efficiency of photosynthesis, of which δ13C is a proxy (Farquhar et al., 1982), was affected by N availability as expected (Evans, 1989) but not by the presence of water restricting wilt symptoms.
The disease is the result of the interactions between the three components of the disease triangle: plant, pathogen, and environmental influences (Agrios, 2005). We found that soil Foc load was a poor predictor of disease severity and only marginally related to N rate or soil pH, in agreement with Pittaway et al. (1999). Soil bacteria and fungi populations were more consistently affected by soil pH and the introduction of the pathogen than by N rate per se as the N rate effect differed between N forms (Figure 4; Supplementary Information 2). High rates of N fertilizer, and associated increased plant growth, are often linked with increases in disease due to a trade-off between growth and defense (Herms and Mattson, 1992; Huot et al., 2014). The expression of proteins associated with the “MAPK signaling pathway—plant,” plant stress and defense pathway, was significantly changed by increased N supply, independent of the presence of the pathogen. PR1 expression, a key endpoint for the “MAPK signaling pathway—plant” and salicylic acid defense response was negatively affected by ammonium fertilization in diseased plants. N fertilization enriched the “Carbon fixation in photosynthetic organisms” pathway that is a key contributor to growth. This change was likely associated with an increase in photosynthetic efficiency shown by the measured enrichment in δ13C with N fertilization. Taken together, these findings support the growth-defense trade-off hypothesis, which suggests that an increase in disease severity results from a reduction in host plant defense resulting from an increase in growth, and a pH effect on the soil microbial community.
Auxin-induced plant growth, triggered by the addition of N, suppresses defensive processes such as salicylic and jasmonic acid signaling (Yaeno and Iba, 2008; Guo et al., 2018; Van Butselaar and Van Den Ackerveken, 2020). Jasmonic acid production is the primary response to necrotrophic pathogens, whereas salicylic acid production is the primary response to biotrophic and hemibiotrophic pathogens such as F. oxysporum f.sp. cubense, though there is important crosstalk between the two responses (Di et al., 2016; Sun et al., 2020; Fernandes and Ghag, 2022). The induction of auxin and target of rapamycin pathways, which are associated with plant growth, has been shown to suppress the salicylic acid response, PR1, and plant resistance (Van Butselaar and Van Den Ackerveken, 2020). The trade-off between auxin synthesis, related to growth, and salicylic acid accumulation, related to defense, is particularly marked in root tissue (Denancé et al., 2013), which was the focus of this study. The salicylic acid response and the production of PR1 have been previously identified as a key defense indicator differentiating Fusarium wilt resistant and susceptible banana cultivars (Van Den Berg et al., 2007; Wang et al., 2015; Ramu et al., 2016; Li et al., 2017; Zhang et al., 2019, 2020). The exogenous application of salicylic acid has also been demonstrated to induce partial resistance to Fusarium wilt in bananas (Wang et al., 2015; Emilda et al., 2020). Systemic acquired resistance, in parallel with salicylic acid defense is likely controlled by the production of nitric oxide, itself controlled by N nutrition (Sun et al., 2020). Mur et al. (2017) suggest nitric oxide-dependent defense is enhanced by nitrate fertilization and reduced by ammonium. Our findings agree that PR1 expression decreased as more ammonium fertilizer was added to the system, though we did not find a significant increase from nitrate fertilization, nor an N form effect on the “MAPK signaling pathway—plant” pathway. Further examination of the proteins that contribute to PR1 expression would be useful to understand how the full signaling pathways respond to N fertilization.
The expression of PR1 at low fertilization rates is greater with ammonium than with nitrate and the decrease of PR1 in response to ammonium is much greater than the increase in response to nitrate causing the expression rates based on the two N forms to intersect at high rates (Figure 8). This is despite the δ15N findings indicating that at low zfertilization rates all plants are likely taking up nitrate (Figures 2, 3) and there is minimal pH difference between the two fertilizer forms (Figure 2). This suggests that a separate mechanism may be responsible and warrants further investigation. There was no statistically significant N form effect on either disease severity or inoculated plant dry weight at low N rates despite the difference in PR1 expression between the two N forms so there may be additional important factors not considered here.
Initiation of defensive signaling in bananas as a response to Foc exposure has been shown to affect the level of banana resistance to Foc (Zhang et al., 2019). The group of proteins categorized under the gene ontology of “Defense response”, which was differentially regulated in inoculated compared with uninoculated samples, is the primary response to the presence of a foreign body or injury (Gene Ontology, 2021). The “Chitin binding” gene ontology also could be a defense-associated response to the fungal pathogen, due to the importance of chitin in fungal cell walls (Zhang et al., 2019). The “Signaling receptor activity” pathway, which includes immune receptor activity, was also affected by Foc inoculation possibly indicating a reduction in the transmission of the defense response (Supplementary Information 3). In agreement with previous studies, inoculation with Foc caused a substantial upregulation of PR1, demonstrating the importance of PR1 to the banana's defense against Foc (Li et al., 2015; Zhang et al., 2019).
The effect of N fertilizer application on disease severity is complicated by a change in soil pH, which affects pathogen abundance and soil bacteria. Soil pH was reduced by the addition of ammonium and slightly increased, though statistically insignificant, by nitrate addition. Nitrate fertilizer has been previously shown to provide protection to plants from Fusarium wilts whereas ammonium typically increases disease severity (Morgan and Timmer, 1984; Wang et al., 2016; Mur et al., 2017; Zhou et al., 2017). However, we found that increased application of both nitrate and ammonium was significantly positively correlated with internal disease severity (Figure 1), suggesting that in our experiment soil pH change may not be the principal determinant of disease severity. Morgan and Timmer (1984) found ammonium and nitrate-treated soil had a final pH of 5.8 and 6.9, respectively. The soils we used had a greater pH buffering capacity, possibly limiting the suppressive potential of pH increase and explaining the disparity between our findings and that of previous researchers.
Our δ15N findings provide further insight into the uptake of the two N forms (Figure 3). At low N fertilizer rates, the nitrate-fertilized plants took up less than half of their N as nitrate, directly from the fertilizer (δ15N of plants nearer to that of soil than fertilizer) whereas the ammonium-fertilized plants did not take up the equivalent amount of fertilizer-derived ammonium N. Either they took up all their N from the soil N pool or from fertilizer-derived N that had nitrified to nitrate (Figure 2). Therefore, at low N fertilizer rates, plants appeared to take up mostly nitrate, irrespective of the form applied. Conversely, at high fertilizer rates plants drew N from the fertilizer supplied but in a combination of forms despite nitrate concentrations being far higher than ammonium concentrations irrespective of the form applied (Figure 2).
Separating the effect of N rate from the effect of pH decline caused by ammonium on Fusarium wilt disease symptoms and the growth of Foc is difficult (Orr and Nelson, 2018; Segura-Mena et al., 2021). Organisms differ in their capacities to function at low pH. Generally, plants are regarded as having greater tolerance to low pH, fungi moderate tolerance, and bacteria are intolerant to reductions in pH (Rousk et al., 2009, 2010). Soil pH was negatively correlated with soil bacterial alpha diversity (Figure 5) and to a minor extent Foc DNA concentration, indicating that the effect of N on the soil microbiome may be occurring at least partially via pH change. However, changes in Foc DNA concentration were unrelated to banana plant disease severity (Figure 4) indicating that Foc abundance was not a driver of disease severity in this case. Our results agree with those of Pittaway et al. (1999) who found pathogen activity was unrelated to disease severity. We inoculated the upper layer of the soil but measured the pathogen population in the rhizosphere where the pathogen and host interact. Growth of the pathogen through the soil exposes the pathogen to the treatments and other members of the disease triangle, resulting in either suppressiveness or conduciveness. The downside of this approach is that the distance between the soil surface and plant root will vary between replicates, adding to variability in the rhizosphere pathogen population. Another limitation of our approach is that we did not measure virulence, which may or may not be linked to abundance. Virulence factors such as Fusaric acid production, or virulence-related gene expression, may have responded to N supply irrespective of abundance (Divon et al., 2006; Bolton and Thomma, 2008; López-Berges et al., 2010; Ding et al., 2018). Li et al. (2019) recently applied simultaneous transcript profiling of Foc and the banana plant during infection. This approach may offer further insight into the effect of N on pathogenicity in the future by directly coupling the response of the plant and pathogen.
Soil pH was not the only environmental factor altered by the nitrogen treatments in this experiment. Most major ions were equalized between treatments, however, the rate of chloride and sodium applied as fertilizer differed between treatments. Despite these differences, it is unlikely that either of these ions was responsible for the treatment effects. There is limited evidence that either chloride or sodium affects Fusarium wilt severity except under high concentrations (Orr and Nelson, 2018). Treatments demonstrating a relationship between Fusarium wilt severity and chloride, sodium or electrical conductivity were orders of magnitude larger than the differences in our fertilizer applications (Woltz et al., 1992; Dominguez et al., 2001; Triky-Dotan et al., 2005).
Inoculation with Foc was significantly associated with changes in the beta diversity of the bacterial (16S) and fungal (ITS) rhizosphere communities and inoculated samples had lower fungal alpha diversity. Contrary to the findings of Effendi et al. (2019), we found that inoculation was not associated with a reduction in the alpha diversity of the bacterial community. Ou et al. (2019) determined that inoculation with Foc increased bacterial alpha diversity in disease suppressive soils and decreased it in conducive soils. The soil we used was relatively disease conducive compared to other soils cultivated for bananas in Australia (Bowen et al., 2019) though there is no way to compare our soils to those of Ou et al. (2019) as conduciveness is a relative measure. It is worth noting that by measuring rhizosphere soil DNA we inherently included residual DNA from organisms that were present prior to inoculation possibly artificially increasing the diversity of the inoculated samples (Carini et al., 2016).
The controlled growing conditions determined in this study differed from commercial situations, and the effects demonstrated the need for field investigation across soil types. However, several previous studies suggest that our findings should be applicable to field situations. For example, a meta-analysis of the effect of N addition on fungal plant pathogens identified no difference between field and pot trials or when the pathogen was naturally vs. artificially introduced (Veresoglou et al., 2013). Additionally, the effect of N on Fusarium wilt severity caused by Race 1 and Tropical Race 4 are similar (Segura-Mena et al., 2021) and infection with these two races initiates a similar defense response (Li et al., 2013). The similarities between Foc Races and agreement between field and pot experiments suggest that the results of our experiment may be applicable to wider conditions though it would be valuable to test this.
Our results demonstrated that a high rate of N fertilizer led to an increase in the severity of the Fusarium wilt of bananas and suggest this may be partially due to a reduction in the defensive capabilities of the plant. The effect of N form requires further investigation, as PR1, a key defensive compound was not affected by nitrate, despite nitrate fertilizer being positively correlated with disease severity. The increase in disease severity may also have been partially due to a reduction in bacterial diversity caused by a decrease in soil pH following ammonium addition. The abundance of the pathogen in the rhizosphere appeared to have no effect on disease severity but was weakly related to soil pH. It would appear there is a threshold of N application, above which elevated internal disease severity is detrimental to plant dry weight. It may in the future be possible to apply fertilizer to maximize banana plant growth while avoiding the penalty of increased internal Fusarium wilt severity, by enhancing the expression of PR1 or related defense genes, decoupling the plant growth—defense trade-off, and by preventing soil pH decline.
The datasets presented in this study can be found in online repositories. The names of the repository/repositories and accession number(s) can be found below: https://massive.ucsd.edu/ProteoSAFe/static/massive.jsp, MSV000089270.
RO, PD, AP, and PN contributed to the conception and experimental design. RO carried out the trial, collected the samples, and wrote the first draft of the manuscript. PD, YW, DB, HB, and HL-G performed sample analysis and assisted with statistical analysis. MC assisted with statistical analysis. All authors contributed to manuscript revision, read, and approved the submitted version.
This project has been funded by Hort Innovation, using the Hort Innovation banana research and development levy, co-investment from Queensland Government and contributions from the Australian Government (Project Code BA14014). Hort Innovation is the grower-owned, not-for-profit research and development corporation for Australian horticulture.
The authors declare that the research was conducted in the absence of any commercial or financial relationships that could be construed as a potential conflict of interest.
All claims expressed in this article are solely those of the authors and do not necessarily represent those of their affiliated organizations, or those of the publisher, the editors and the reviewers. Any product that may be evaluated in this article, or claim that may be made by its manufacturer, is not guaranteed or endorsed by the publisher.
The Supplementary Material for this article can be found online at: https://www.frontiersin.org/articles/10.3389/fpls.2022.907819/full#supplementary-material
Alexa, A., and Rahnenfuhrer, J. (2020). “topGO: Enrichment Analysis for Gene Ontology.” R package version 2.40.0 ed.).
Bengtsson-Palme, J., Ryberg, M., Hartmann, M., Branco, S., Wang, Z., Godhe, A., et al. (2013). Improved software detection and extraction of ITS1 and ITS2 from ribosomal ITS sequences of fungi and other eukaryotes for analysis of environmental sequencing data. Methods Ecol. Evol. 4, 914–919. doi: 10.1111/2041-210X.12073
Bessarabova, M., Ishkin, A., Jebailey, L., Nikolskaya, T., and Nikolsky, Y. (2012). Knowledge-based analysis of proteomics data. BMC Bioinform. 13, S13–S13. doi: 10.1186/1471-2105-13-S16-S13
Birt, H. W. G., Pattison, A. B., and Dennis, P. G. (2021). “Sampling microbiomes associated with different plant compartments,” in The Plant Microbiome: Methods and Protocols, eds. L.C. Carvalhais and P.G. Dennis. (New York, NY: Springer), 23–29. doi: 10.1007/978-1-0716-1040-4_2
Birt, H. W. G., Pattison, A. B., Skarshewski, A., Daniells, J., Raghavendra, A., and Dennis, P. G. (2022). The Core Bacterial Microbiome of Banana (Musa spp.). Research Square. doi: 10.21203/rs.3.rs-1495474/v1
Bolton, M. D., and Thomma, B. P. H. J. (2008). The complexity of nitrogen metabolism and nitrogen-regulated gene expression in plant pathogenic fungi. Physiologic. Mol. Plant Pathol. 72, 104–110. doi: 10.1016/j.pmpp.2008.07.001
Bowen, A., Orr, R., Mcbeath, A. V., Pattison, A., and Nelson, P. N. (2019). Suppressiveness or conduciveness to Fusarium wilt of bananas differs between key Australian soils. Soil Res. 57, 158–165. doi: 10.1071/SR18159
Browne, D. J., Brady, J. L., Waardenberg, A. J., Loiseau, C., and Doolan, D. L. (2020). An Analytically and diagnostically sensitive RNA extraction and RT-qPCR protocol for peripheral blood mononuclear cells. Front. Immunol. 11, 20. doi: 10.3389/fimmu.2020.00402
Bustin, S. A., Benes, V., Garson, J. A., Hellemans, J., Huggett, J., Kubista, M., et al. (2009). The MIQE guidelines: minimum information for publication of quantitative real-time PCR experiments. Clin. Chem. 55, 611–622. doi: 10.1373/clinchem.2008.112797
Carini, P., Marsden, P. J., Leff, J. W., Morgan, E. E., Strickland, M. S., and Fierer, N. (2016). Relic DNA is abundant in soil and obscures estimates of soil microbial diversity. Nature Microbiol.y 2, 16242. doi: 10.1101/043372
Cernusak, L. A., Ubierna, N., Winter, K., Holtum, J.a.M, Marshall, J. D., et al. (2013). Environmental and physiological determinants of carbon isotope discrimination in terrestrial plants. New Phytolo. 200, 950–965. doi: 10.1111/nph.12423
Cribari-Neto, F., and Zeileis, A. (2010). Beta Regression in R. 2010 34,24. doi: 10.18637/jss.v034.i02
Denancé, N., Ranocha, P., Oria, N., Barlet, X., Rivière, M.-P., Yadeta, K. A., et al. (2013). Arabidopsis wat1 (walls are thin1)-mediated resistance to the bacterial vascular pathogen, Ralstonia solanacearum, is accompanied by cross-regulation of salicylic acid and tryptophan metabolism. Plant J. 73, 225–239. doi: 10.1111/tpj.12027
Denk, T. R. A., Mohn, J., Decock, C., Lewicka-Szczebak, D., Harris, E., Butterbach-Bahl, K., et al. (2017). The nitrogen cycle: a review of isotope effects and isotope modeling approaches. Soil Biol. Biochemistr. 105, 121–137. doi: 10.1016/j.soilbio.2016.11.015
Di, X., Takken, F. L. W., and Tintor, N. (2016). How phytohormones shape interactions between plants and the soil-borne fungus fusarium oxysporum. Front. Plant Sci. 7, 12. doi: 10.3389/fpls.2016.00170
Ding, Z., Yang, L., Wang, G., Guo, L., Liu, L., Wang, J., et al. (2018). Fusaric acid is a virulence factor of Fusarium oxysporum f. sp. cubense on banana plantlets. Tropic. Plant Pathol. 43, 297–305. doi: 10.1007/s40858-018-0230-4
Divon, H. H., Ziv, C., Davydov, O., Yarden, O., and Fluhr, R. (2006). The global nitrogen regulator, FNR1, regulates fungal nutrition-genes and fitness during Fusarium oxysporum pathogenesis. Mol. Plant Pathol. 7, 485–497. doi: 10.1111/j.1364-3703.2006.00354.x
Doellinger, J., Schneider, A., Hoeller, M., and Lasch, P. (2020). Sample preparation by easy extraction and digestion (SPEED)—a universal, rapid, and detergent-free protocol for proteomics based on acid extraction. Mol Cell Proteom. 19, 209–222. doi: 10.1074/mcp.TIR119.001616
Dominguez, J., Negrin, M. A., and Rodriguez, C. M. (2001). Aggregate water-stability, particle size and soil solution properties in conducive and suppressive soils to Fusarium wilt of banana from Canary Islands (Spain). Soil Biol. Biochemistr. 33, 449–455. doi: 10.1016/S0038-0717(00)00184-X
Droc G. Larivière D. Guignon V. Yahiaoui N. This D. Garsmeur O.. (2013). The banana genome hub. Database 2013, 253. doi: 10.1093/database/bat035
Edgar, R. C. (2010). Search and clustering orders of magnitude faster than BLAST. Bioinformatics 26, 2460–2461. doi: 10.1093/bioinformatics/btq461
Edgar, R. C. (2013). UPARSE: highly accurate OTU sequences from microbial amplicon reads. Nat. Meth. 10, 996–998. doi: 10.1038/nmeth.2604
Effendi, Y., Pambudi, A., and Pancoro, A. (2019). Metagenomic analysis of Fusarium oxysporum f. sp. cubense-infected soil in banana plantation, Sukabumi, Indonesia. Biodiversitas J. Biologic. Div. 20, 234. doi: 10.13057/biodiv/d200721
Emilda, D., Sutanto, A., and Sukartini, J. (2020). “Application of salicylic acid to induce disease resistance against fusarium wilt on banana,” in IOP Conference Series: Earth and Environmental Science. IOP Conference Series: Earth and Environmental Science). doi: 10.1088/1755-1315/468/1/012026
Erisman, J. W., Sutton, M. A., Galloway, J., Klimont, Z., and Winiwarter, W. (2008). How a century of ammonia synthesis changed the world. Nat. Geosci. 1, 636–639. doi: 10.1038/ngeo325
Evans, J. R. (1989). Photosynthesis and nitrogen relationships in leaves of C3 plants. Oecologia 78, 9–19. doi: 10.1007/BF00377192
Farquhar, G., O'leary, M., and Berry, J. (1982). On the relationship between carbon isotope discrimination and the intercellular carbon dioxide concentration in leaves. Funct. Plant Biol. 9, 121–137. doi: 10.1071/PP9820121
Fernandes, L. B., and Ghag, S. B. (2022). Molecular insights into the jasmonate signaling and associated defense responses against wilt caused by Fusarium oxysporum. Plant Physiol. Biochemistr. 174, 22–34. doi: 10.1016/j.plaphy.2022.01.032
Fox, J., and Weisberg, S. (2019). An R Companion to Applied Regression, Third Edition. Thousand Oaks, California: Sage.
Fu, L., Penton, C. R., Ruan, Y., Shen, Z., Xue, C., Li, R., et al. (2017). Inducing the rhizosphere microbiome by biofertilizer application to suppress banana Fusarium wilt disease. Soil Biol. Biochemistr. 104, 39–48. doi: 10.1016/j.soilbio.2016.10.008
Gene Ontology, C. (2021). The Gene Ontology resource: enriching a GOld mine. Nucleic Acids Res. 49, D325–D334. doi: 10.1093/nar/gkaa1113
Guo, Q., Major, I. T., and Howe, G. A. (2018). Resolution of growth–defense conflict: mechanistic insights from jasmonate signaling. Curr. Opin. Plant Biol. 44, 72–81. doi: 10.1016/j.pbi.2018.02.009
Herms, D. A., and Mattson, W. J. (1992). The dilemma of plants: to grow or defend. Q. Rev. Biol. 67, 283–335. doi: 10.1086/417659
Huot, B., Yao, J., Montgomery, B. L., and He, S. Y. (2014). Growth–defense tradeoffs in plants: a balancing act to optimize fitness. Mol. Plant 7, 1267–1287. doi: 10.1093/mp/ssu049
Ihrmark, K., Bödeker, I. T. M., Cruz-Martinez, K., Friberg, H., Kubartova, A., Schenck, J., et al. (2012). New primers to amplify the fungal ITS2 region—evaluation by 454-sequencing of artificial and natural communities. FEMS Microbiol. Ecol. 82, 666–677. doi: 10.1111/j.1574-6941.2012.01437.x
Jarvis, W. R., and Thorpe, H. J. (1980). Effects of nitrate and ammonium nitrogen on severity of Fusarium foot and root rot and on yield of greenhouse tomatoes. Plant Dis. 64, 309–310. doi: 10.1094/PD-64-309
Jones, J. P., Woltz, S. S., and Everett, P. H. (1975). Effect of liming and nitrogen source on Fusarium wilt of cucumber and watermelon. Proceed. Florida State Horticult. Soc. 88, 200–203.
Kanehisa, M., Sato, Y., and Morishima, K. (2016). BlastKOALA and GhostKOALA: KEGG Tools for functional characterization of genome and metagenome sequences. J. Mol. Biol. 428, 726–731. doi: 10.1016/j.jmb.2015.11.006
Katoh, K., and Standley, D. M. (2013). MAFFT multiple sequence alignment software version 7: improvements in performance and usability. Mol. Biol. Evol. 30, 772–780. doi: 10.1093/molbev/mst010
Kay, M., Elkin, L., Higgins, J., and Wobbrock, J. (2021). ARTool: Aligned Rank Transform for Nonparametric Factorial ANOVAs.
Law, C., Zeglinski, K., Dong, X., Alhamdoosh, M., Smyth, G., and Ritchie, M. (2020). A guide to creating design matrices for gene expression experiments [version 1; peer review: 2 approved]. F1000Research 9. doi: 10.12688/f1000research.27893.1
Li, C., Shao, J., Wang, Y., Li, W., Guo, D., Yan, B., et al. (2013). Analysis of banana transcriptome and global gene expression profiles in banana roots in response to infection by race 1 and tropical race 4 of Fusarium oxysporum f. sp. cubense. BMC Genom. 14, 851–851. doi: 10.1186/1471-2164-14-851
Li, W., Ge, X., Wu, W., Wang, W., Hu, Y., Mo, Y., et al. (2015). Identification of defense-related genes in banana roots infected by Fusarium oxysporum f. sp. cubense tropical race 4. Euphytica 205, 837–849. doi: 10.1007/s10681-015-1418-z
Li, W., Li, C., Sun, J., and Peng, M. (2017). Metabolomic, biochemical, and gene expression analyses reveal the underlying responses of resistant and susceptible banana species during early infection with fusarium oxysporum f. sp. cubense. Plant Dis. 101, 534–543. doi: 10.1094/PDIS-09-16-1245-RE
Li, W., Wang, X., Li, C., Sun, J., Li, S., and Peng, M. (2019). Dual species transcript profiling during the interaction between banana (Musa acuminata) and the fungal pathogen Fusarium oxysporum f. sp. cubense. BMC Genom. 20, 519. doi: 10.1186/s12864-019-5902-z
López-Berges, M. S., Rispail, N., Prados-Rosales, R. C., and Di Pietro, A. (2010). A nitrogen response pathway regulates virulence in plant pathogenic fungi. Plant Signal. Behav. 5, 1623–1625. doi: 10.4161/psb.5.12.13729
Marinari, S., Masciandaro, G., Ceccanti, B., and Grego, S. (2000). Influence of organic and mineral fertilisers on soil biological and physical properties. Bioresour. Technol. 72, 9–17. doi: 10.1016/S0960-8524(99)00094-2
Matthews, M. C., Mostert, D., Ndayihanzamaso, P., Rose, L. J., and Viljoen, A. (2020). Quantitative detection of economically important Fusarium oxysporum f. sp. cubense strains in Africa in plants, soil and water. Plos One 15, e0236110. doi: 10.1371/journal.pone.0236110
Morgan, K. T., and Timmer, L. W. (1984). Effect of inoculum density, nitrogen source and saprophytic fungi on Fusarium wilt of Mexican lime. Plant Soil 79, 203–210. doi: 10.1007/BF02182342
Mur, L. A., Simpson, C., Kumari, A., Gupta, A. K., and Gupta, K. J. (2017). Moving nitrogen to the centre of plant defence against pathogens. Annal. Bot. 119, 703–709. doi: 10.1093/aob/mcw179
Neumann, G., and Römheld, V. (2012). “Chapter 14—rhizosphere chemistry in relation to plant nutrition a2—marschner, Petra,” in Marschner's Mineral Nutrition of Higher Plants (Third Edition) (San Diego: Academic Press), 347–368. doi: 10.1016/B978-0-12-384905-2.00014-5
Neuser, J., Metzen, C. C., Dreyer, B. H., Feulner, C., Van Dongen, J. T., Schmidt, R. R., et al. (2019). HBI1 mediates the trade-off between growth and immunity through its impact on apoplastic ROS homeostasis. Cell Reports 28, 1670–1678. doi: 10.1016/j.celrep.2019.07.029
Nilsson, R. H., Larsson, K. H., Taylor, A. F. S., Bengtsson-Palme, J., Jeppesen, T. S., Schigel, D., et al. (2019). The UNITE database for molecular identification of fungi: handling dark taxa and parallel taxonomic classifications. Nucleic. Acids Res. 47, D259–d264. doi: 10.1093/nar/gky1022
Oksanen, J., Blanchet, F. G., Friendly, M., Kindt, R., Legendre, P., Mcglinn, D., et al. (2019). vegan: Community Ecology Package. R package version 2.5–6. 2019.
Orr, R., and Nelson, P. N. (2018). Impacts of soil abiotic attributes on Fusarium wilt, focusing on bananas. Appl. Soil Ecol. 132, 20–33. doi: 10.1016/j.apsoil.2018.06.019
Orr, R., Pattison, A., East, D., Warman, N., O'neill, W., Czislowski, E., et al. (2019). Image-based quantification of Fusarium wilt severity in banana. Austral. Plant Dis. Notes 14, 14. doi: 10.1007/s13314-019-0344-7
Ou, Y., Penton, C. R., Geisen, S., Shen, Z., Sun, Y., Lv, N., et al. (2019). Deciphering underlying drivers of disease suppressiveness against pathogenic fusarium oxysporum. Front. Microbiol. 10, 123. doi: 10.3389/fmicb.2019.02535
Pittaway, P. A., Nasir, N., and Pegg, K. G. (1999). Soil receptivity and host-pathogen dynamics in soils naturally infested with Fusarium oxysporum f. sp. cubense, the cause of Panama disease in bananas. Austral. J. Agricult. Res. 50, 623–628. doi: 10.1071/A98152
Price, M. N., Dehal, P. S., and Arkin, A. P. (2010). FastTree 2—approximately maximum-likelihood trees for large alignments. PLoS ONE 5, e9490. doi: 10.1371/journal.pone.0009490
Quast, C., Pruesse, E., Yilmaz, P., Gerken, J., Schweer, T., Yarza, P., et al. (2013). The SILVA ribosomal RNA gene database project: improved data processing and web-based tools. Nucleic Acids Res. 41, D.590-D.596. doi: 10.1093/nar/gks1219
R Core Team (2017). R: A Language and Environment for Statistical Computing. Vienna, Austria: R Foundation for Statistical Computing.
Rames, E. K., Pattison, A. B., Czislowski, E., and Smith, M. K. (2018). Soil microbial community changes associated with ground cover management in cultivation of Ducasse banana (Musa sp. ABB, Pisang Awak subgroup) and suppression of Fusarium oxysporum. Austral. Plant Pathol. 47, 449–462. doi: 10.1007/s13313-018-0578-4
Ramu, V., Venkatarangaiah, K., Krishnappa, P., Rajanna, S. K. S., Deeplanaik, N., Pal, A. C., et al. (2016). Identification of biomarkers for resistance to Fusarium oxysporum f. sp. cubense infection and in silico studies in Musa paradisiaca cultivar puttabale through proteomic approach. Proteomes 4, 245. doi: 10.3390/proteomes4010009
Ritchie, M. E., Phipson, B., Wu, D., Hu, Y., Law, C. W., Shi, W., et al. (2015). limma powers differential expression analyses for RNA-sequencing and microarray studies. Nucleic Acids Res. 43, e47–e47. doi: 10.1093/nar/gkv007
Rohland, N., and Reich, D. (2012). Cost-effective, high-throughput DNA sequencing libraries for multiplexed target capture. Genome Res. 22, 939–946. doi: 10.1101/gr.128124.111
Rousk, J., Baath, E., Brookes, P. C., Lauber, C. L., Lozupone, C., Caporaso, J. G., et al. (2010). Soil bacterial and fungal communities across a pH gradient in an arable soil. ISME J. 4, 1340–1351. doi: 10.1038/ismej.2010.58
Rousk, J., Brookes, P. C., and Bååth, E. (2009). Contrasting soil pH effects on fungal and bacterial growth suggest functional redundancy in carbon mineralization. Appl. Environ. Microbiol. 75, 1589–1596. doi: 10.1128/AEM.02775-08
Schneider, C. A., Rasband, W. S., and Eliceiri, K. W. (2012). NIH Image to ImageJ: 25 years of image analysis. Nat. Methods 9, 671–675. doi: 10.1038/nmeth.2089
Segura, R. A., Stoorvogel, J. J., and Sandoval, J. A. (2022). The effect of soil properties on the relation between soil management and Fusarium wilt expression in Gros Michel bananas. Plant Soil. 471, 89–100. doi: 10.1007/s11104-021-05192-5
Segura-Mena, R. A., Stoorvogel, J. J., García-Bastidas, F., Salacinas-Niez, M., Kema, G. H. J., and Sandoval, J. A. (2021). Evaluating the potential of soil management to reduce the effect of Fusarium oxysporum f. sp. cubense in banana (Musa AAA). Euro. J. Plant Pathol. 160, 441–455. doi: 10.1007/s10658-021-02255-2
Shen, Z., Ruan, Y., Xue, C., Zhong, S., Li, R., and Shen, Q. (2015). Soils naturally suppressive to banana Fusarium wilt disease harbor unique bacterial communities. Plant Soil 393, 21–33. doi: 10.1007/s11104-015-2474-9
Sun, Y., Wang, M., Mur, L.a,.J, Shen, Q., and Guo, S. (2020). Unravelling the roles of nitrogen nutrition in plant disease defences. Int. J. Mol. Sci. 21, 572. doi: 10.3390/ijms21020572
Teixeira, L., Heck, D., Nomura, E., Vieira, H., and Dita, M. (2021). Soil attributes, plant nutrition, and Fusarium wilt of banana in São Paulo, Brazil. Tropic. Plant Pathol. 46, 443–454. doi: 10.1007/s40858-021-00428-2
Triky-Dotan, S., Yermiyahu, U., Katan, J., and Gamliel, A. (2005). Development of crown and root rot disease of tomato under irrigation with saline water. Phytopathology 95, 1438–1444. doi: 10.1094/PHYTO-95-1438
Van Butselaar, T., and Van Den Ackerveken, G. (2020). Salicylic acid steers the growth-immunity tradeoff. Trends Plant Sci. doi: 10.1016/j.tplants.2020.02.002
Van Den Berg, N., Berger, D. K., Hein, I., Birch, P. R., Wingfield, M. J., and Viljoen, A. (2007). Tolerance in banana to Fusarium wilt is associated with early up-regulation of cell wall-strengthening genes in the roots. Mol. Plant Pathol. 8, 333–341. doi: 10.1111/j.1364-3703.2007.00389.x
Veresoglou, S. D., Barto, E. K., Menexes, G., and Rillig, M. C. (2013). Fertilization affects severity of disease caused by fungal plant pathogens. Plant Pathol. 62, 961–969. doi: 10.1111/ppa.12014
Wang, C., Liu, D., and Bai, E. (2018). Decreasing soil microbial diversity is associated with decreasing microbial biomass under nitrogen addition. Soil Biol. Biochemistr. 120, 126–133. doi: 10.1016/j.soilbio.2018.02.003
Wang, M., Sun, Y., Gu, Z., Wang, R., Sun, G., Zhu, C., et al. (2016). Nitrate protects cucumber plants against fusarium oxysporum by regulating citrate exudation. Plant Cell Physiol. 57, 2001–2012. doi: 10.1093/pcp/pcw124
Wang, X., Yu, R., and Li, J. (2021). Using genetic engineering techniques to develop banana cultivars with fusarium wilt resistance and ideal plant architecture. Front. Plant Sci. 11, 34. doi: 10.3389/fpls.2020.617528
Wang, Z., Jia, C., Li, J., Huang, S., Xu, B., and Jin, Z. (2015). Activation of salicylic acid metabolism and signal transduction can enhance resistance to Fusarium wilt in banana (Musa acuminata L. AAA group, cv. Cavendish). Funct. Integrat. Genom. 15, 47–62. doi: 10.1007/s10142-014-0402-3
Warman, N. M., and Aitken, E. B. (2018). The Movement of Fusarium oxysporum f.sp. cubense (Sub-Tropical Race 4) in Susceptible Cultivars of Banana. Front. Plant Sci. 9, 23. doi: 10.3389/fpls.2018.01748
White, T. J., Bruns, T., Lee, S., and Taylor, J. (1990). Amplification and direct sequencing of fungal ribosomal RNA genes for phylogenetics. PCR Protocols Guide Method Applic. 18, 315–322. doi: 10.1016/B978-0-12-372180-8.50042-1
Woltz, S., and Engelhard, A. (1973). Fusarium wilt of chrysanthemum: effect of nitrogen source and lime on disease development. Phytopathology. 63, 155–157. doi: 10.1094/Phyto-63-155
Woltz, S. S., Jones, J. P., and Scott, J. W. (1992). Sodium chloride, nitrogen source, and lime influence fusarium crown rot severity in tomato. HortScience 27, 1087–1088. doi: 10.21273/HORTSCI.27.10.1087
Yaeno, T., and Iba, K. (2008). BAH1/NLA, a RING-Type Ubiquitin E3 Ligase, Regulates the Accumulation of Salicylic Acid and Immune Responses to Pseudomonas syringae DC3000 Plant Physiol. 148, 1032–1041. doi: 10.1104/pp.108.124529
Yang, Y., Cheng, H., Gao, H., and An, S. (2020). Response and driving factors of soil microbial diversity related to global nitrogen addition. Land Degradat. Develop. 31, 190–204. doi: 10.1002/ldr.3439
Zhang, L., Cenci, A., Rouard, M., Zhang, D., Wang, Y., Tang, W., et al. (2019). Transcriptomic analysis of resistant and susceptible banana corms in response to infection by Fusarium oxysporum f. sp. cubense tropical race 4. Scientific Rep. 9, 8199. doi: 10.1038/s41598-019-44637-x
Zhang, L., Liu, L., Li, S., Bai, T., Xu, S., Fan, H., et al. (2020). “Proteomic analysis of banana xylem sap provides insight into resistant mechanisms to Fusarium oxysporum f. sp. cubense Tropical Race 4,” in Research Square. doi: 10.21203/rs.3.rs-26869/v1
Zhang, Z., Schwartz, S., Wagner, L., and Miller, W. (2000). A greedy algorithm for aligning DNA sequences. J. Comput. Biol. 7, 203–214. doi: 10.1089/10665270050081478
Zhou, D., Jing, T., Chen, Y., Wang, F., Qi, D., Feng, R., et al. (2019). Deciphering microbial diversity associated with Fusarium wilt-diseased and disease-free banana rhizosphere soil. BMC Microbiol. 19, 161. doi: 10.1186/s12866-019-1531-6
Zhou, J., Wang, M., Sun, Y., Gu, Z., Wang, R., Saydin, A., et al. (2017). Nitrate increased cucumber tolerance to fusarium wilt by regulating fungal toxin production and distribution. Toxins 9, 100. doi: 10.3390/toxins9030100
Keywords: disease triangle, proteomics, ammonium, nitrate, qPCR, banana, nitrogen fertilisation, Fusarium wilt (causal agent Fusarium oxysporum)
Citation: Orr R, Dennis PG, Wong Y, Browne DJ, Cooper M, Birt HWG, Lapis-Gaza HR, Pattison AB and Nelson PN (2022) Nitrogen fertilizer rate but not form affects the severity of Fusarium wilt in banana. Front. Plant Sci. 13:907819. doi: 10.3389/fpls.2022.907819
Received: 30 March 2022; Accepted: 29 June 2022;
Published: 22 July 2022.
Edited by:
Giovanni Beccari, University of Perugia, ItalyReviewed by:
Rony Swennen, International Institute of Tropical Agriculture (IITA), NigeriaCopyright © 2022 Orr, Dennis, Wong, Browne, Cooper, Birt, Lapis-Gaza, Pattison and Nelson. This is an open-access article distributed under the terms of the Creative Commons Attribution License (CC BY). The use, distribution or reproduction in other forums is permitted, provided the original author(s) and the copyright owner(s) are credited and that the original publication in this journal is cited, in accordance with accepted academic practice. No use, distribution or reproduction is permitted which does not comply with these terms.
*Correspondence: Ryan Orr, cnlhbi5vcnJAZGFmLnFsZC5nb3YuYXU=; cnlhbi5vcnJAamN1LmVkdS5hdQ==
Disclaimer: All claims expressed in this article are solely those of the authors and do not necessarily represent those of their affiliated organizations, or those of the publisher, the editors and the reviewers. Any product that may be evaluated in this article or claim that may be made by its manufacturer is not guaranteed or endorsed by the publisher.
Research integrity at Frontiers
Learn more about the work of our research integrity team to safeguard the quality of each article we publish.