- 1Crop Foundation Research Division, National Institute of Crop Science, Wanju, South Korea
- 2Residual Agrochemical Assessment Division, National Institute of Agriculture Science, Wanju, South Korea
- 3Bio-Technology of Multidisciplinary Sciences Co., Wanju, South Korea
- 4Department of Environmental Energy Engineering, Kyonggi University, Suwon, South Korea
- 5Reserch Policy Bureau, Rural Development Administration, Wanju, South Korea
- 6Department of Plant Resources, College of Industrial Sciences, Kongju National University, Yesan, South Korea
Ionomics, the study of the composition of mineral nutrients and trace elements in organisms that represent the inorganic component of cells and tissues, has been widely studied to explore to unravel the molecular mechanism regulating the elemental composition of plants. However, the genetic factors of rice subspecies in the interaction between arsenic and functional ions have not yet been explained. Here, the correlation between As and eight essential ions in a rice core collection was analyzed, taking into account growing condition and genetic factors. The results demonstrated that the correlation between As and essential ions was affected by genetic factors and growing condition, but it was confirmed that the genetic factor was slightly larger with the heritability for arsenic content at 53%. In particular, the cluster coefficient of japonica (0.428) was larger than that of indica (0.414) in the co-expression network analysis for 23 arsenic genes, and it was confirmed that the distance between genes involved in As induction and detoxification of japonica was far than that of indica. These findings provide evidence that japonica populations could accumulate more As than indica populations. In addition, the cis-eQTLs of AIR2 (arsenic-induced RING finger protein) were isolated through transcriptome-wide association studies, and it was confirmed that AIR2 expression levels of indica were lower than those of japonica. This was consistent with the functional haplotype results for the genome sequence of AIR2, and finally, eight rice varieties with low AIR2 expression and arsenic content were selected. In addition, As-related QTLs were identified on chromosomes 5 and 6 under flooded and intermittently flooded conditions through genome-scale profiling. Taken together, these results might assist in developing markers and breeding plans to reduce toxic element content and breeding high-quality rice varieties in future.
Introduction
Ionomics is defined as the study of the composition of mineral nutrients and trace elements in organisms and represents the inorganic components in the cells and tissues of an organism (Salt et al., 2008). Early ionomics focused on the identification or characterization of Arabidopsis and yeast mutants (Lahner et al., 2003); however, its application has been extended to high-throughput element profiling to find genes involved in controlling the ionome of an organism (Danku et al., 2013).
Ionomics is a powerful approach to quickly analyzing several samples using the inductively coupled plasma mass spectrometry (ICP-MS). In addition, since transcript and ion profiling can be performed not only on cells regulating plant physiology but also on the whole organism simultaneously, many complex studies on ionomes combined with the genome, transcriptome, proteome, and metabolites have recently been published (Baxter, 2010).
Reportedly, As, a toxic non-essential element, accumulates in rice and other crops due to irrigation with contaminated groundwater, industrialization, mining activity, and use of arsenical pesticides (Duxbury et al., 2003; Liao and Ou, 2005; Williams et al., 2007; Chen et al., 2016). As contamination is not limited to water sources, soil contamination with As induces infertile ears and reduces plant growth, especially in agricultural areas (Kumarathilaka et al., 2018a). Arsenic is a major threat to human health because it is absorbed from the soil and accumulates in edible plant parts (Finnegan and Chen, 2012).
In the environment, As exists in organic and inorganic forms, and the contents of inorganic species, such as arsenate [As(V)] and arsenite [As(III)], are higher in soil than the organic arsenic species, such as monomethylarsonic acid (MMAA) and dimethylarsinic acid (DMAA). The ratio of As(III) and As(V) varies depending on the redox state and redox potential (Eh) of soil; if Eh is negative, As(V) is transformed to As(III), and the proportion of As(III) increases (Yamaguchi et al., 2014). Recent studies based on climate change prediction models have shown that arsenic concentrations in the soil are affected by Co2 and temperature. As(III) concentration decreased with increasing CO2 concentration, but the toxicity of As(III) to humans increased proportionally with an increase in the temperature compared with that of As(V) (Muehe et al., 2019).
Rice, one of the staple crops consumed by more than 50% of the world's population, is known to be roughly 10 times more likely to assimilate As than other crops (Williams et al., 2007), and therefore, it could be a major source of As toxicity in humans. The mechanism of absorption As(V) is closely related to the phosphate uptake system, whereas As(III) is absorbed by the aquaporins of roots (Ullrich-eberius et al., 1989). It is known that several phosphate transporter genes (e.g., OsPht1, 1-OsPht1, and Pht) are involved in As(V) absorption, and nodulin 26-like intrinsic proteins (NIPs) have been shown to absorb As(III) (Paszkowski et al., 2002; Bienert et al., 2008; Isayenkov and Matouis, 2008; Ma et al., 2008; Kirk et al., 2009).
The accumulation of ionomes, including arsenic, in plants is regulated by these genes involved in uptake, binding, transport, and sequestration (Baxter, 2009). Therefore, studies to verify the correlation between arsenic and other inorganic components in various populations and to elucidate genetic factors involved in this have been reported, so far (Zhang et al., 2008; Yang et al., 2018). However, the interactions between ionomes are affected not only by genotypes, but also by the environment or the interaction between the environment and the genotypes (Garcia-Oliveira et al., 2009; Hu et al., 2013; Huang and Salt, 2016).
In this study, the interactions between As and other ions were analyzed under non-stress and stress conditions for rice core collections, including temperate japonica, tropical japonica, indica, and aus, to elucidate the impact of environmental and genotypic differences. In addition, it was attempted to identify the genetic factors regulating As genes through a transcriptome-wide association study on 23 genes known to transport, detoxify, or stress-response (Yang et al., 2012; Nguyen et al., 2014; Most and Papenbrock, 2015; Yamaji et al., 2015; Hwang et al., 2016, 2017; Shi et al., 2016; Das et al., 2017, 2018a,b; Salt, 2017; Latowski et al., 2018; Sun et al., 2018; Wang et al., 2019; Tiwari et al., 2020; Singh et al., 2021).
Materials and Methods
Plant Materials
An allele-mining set of 166 accessions was developed using a heuristic algorithm for 4,046 rice accessions collected from 60 countries held by RDA-Genebank (Kim et al., 2007; Zhao et al., 2010). After the rice core set was constructed, association mapping was conducted on eating quality and amylose content, and a rice core collection for the current 430 accessions was established (Lu and Park, 2012a,b; Zhao et al., 2013). A rice core collection was cultivated in non-contaminated and contaminated paddy soil in 2016 and 2017, and then, 273 accessions overlapped by year were selected. The following subspecies of selected 273 accessions were used: 192 temperate japonica, 19 tropical japonica, 49 indica, 8 aus, 3 admixture, and 2 aromatic.
Field Experiment and Inorganic Component Analysis
Field experiments were conducted on general paddy soil (latitude: 36.670, longitude: 126.855) at Kongju National University and contaminated paddy soil at Hakyeong Mine (latitude: 36.573, longitude: 126.819), Yesan-gun, Chungcheongnam-do, Republic of Korea. The 273 rice accessions were grown in contaminated soil over two years (2016 and 2017), and the ears of each individual were sampled at the yellow ripe stage to analyze accumulation patterns of ionomes in brown rice (Supplementary File 1). In particular, field experiments were conducted under flooded (2016) and intermittently flooded conditions (2017) due to the narrow arable land of the contaminated soil.
The chemical properties of soil were analyzed following the National Academy Aggregation Science (NAAS, 2010). First, soil samples were mixed with distilled water at a 1:5 ratio and kept for 1 h. The pH and EC were then measured (Orion 3 Star, Thermo, USA). To measure cation exchangeability for Ca, Mg, K, and Na in soil, soil samples were mixed with 1 mL of distilled water, 21 mL of HCl, and 7 mL of HNO3. Subsequently, the mixture was decomposed using Kjeldahl (C. Gerhardt GmbH & Co., Northants, UK). Afterward, 1 M NH4OAc (pH 7.0) was added to the decomposed mixture, mixed by shaking, and filtered using a Whatman No. 42 filter paper (Kang et al., 2018).
After the 273 brown rice accessions were ground using a cyclone miller (PX-MFC 90 D, KINEMATICA, Switzerland), they were decomposed by adding 4 mL of HNO3 and 1 mL of distilled water in a microwave oven (UltraWAVE, Milestone, USA). The volume of the decomposed samples was adjusted to 25 mL with distilled water. Micro-elements in plants (As, Zn, Fe, Cu, Mn, Na, and Se) were analyzed using ICP-MS (7700E, Agilent Technologies, USA), and the macro-elements in soil (Ca and Mg) were analyzed using inductively coupled plasma-optical emission spectrometry (Integra XL, GBC, AUS). The R2 of the standard calibration curves for each element was 0.999 or more using multi-element standard (Agilent, USA), and it was confirmed that the recovery rates for inorganic components were 80–120% using IRMM-804 rice flour.
Meanwhile, broad-sense heritability for As content was calculated by QTLmax Global (2022) with the following formula:
, where “” is the genetic variance, “” is the genotype by the annual environmental effect, “σ2” is the error variance, and “e” is the annual environmental effect (Le Sech and Christian, 1991).
DNA Extraction and Whole-Genome Sequencing
DNA and RNA were extracted from the 15-day-old seeds after heading to analyze the SNP and arsenic gene expression levels. Some amino acids or metabolites play important roles in plant growth and development, as well as plant resistance to various stresses (Li et al., 2010). Amino acid metabolism is affected by high night temperature at the early milky stage. As a result, the seed weight and grain quality of rice are determined by changes in gene expression patterns (Liao et al., 2015).
The 15-day-old young seeds were collected and powdered using mortar–pestle. Afterward, genomic DNA was extracted using DNeasy® Plant Mini Kit (QIAGEN, Germany). The concentration of the sample was adjusted to 30 ng μL-1. For next-generation sequencing library preparation, quality control (QC) analysis was performed to ensure that the fragment of the DNA was of the desired size. The extracted DNA samples were quantified using the Quant-iT™ dsDNA High-Sensitivity Assay Kit (Invitrogen, Carlsbad, CA, USA) on an Agilent 2100 Bioanalyzer (Agilent Technologies, Santa Clara, CA, USA). Optical density was measured using Tecan F200 (Tecan, Switzerland), and the quality of the extracted DNA was confirmed by electrophoresis on a 0.7% agarose gel. Short-read sequences were obtained using HiSeq 2500 (Illumina), and next-generation sequencing was performed for genome analysis (Supplementary File 2).
RNA Sequencing and Transcriptome Analyzes
Total RNA from the samples was extracted using the Total RNA Prep Kit for plant tissues (QIAGEN, Germany). The quality of the extracted RNA was confirmed by electrophoresis on 0.7% agarose gel, and its absorbance was measured using a UV spectrophotometer (UV-2600, SHIMADAZU). The purity and quantification were performed using NanoDrop ND-1000 (Dupont Agricultural Genomics Laboratory). The concentration of the sample was adjusted to 20 ng μL−1. The short-read sequence obtained from RNA sequencing was aligned, and the Bowtie (version 1.1.2) and Tophat (version 2.1.0) were used to compare and map with International Rice Genome Sequencing Project 1.0 (Heo et al., 2017; Supplementary File 3).
Model Selection for GWAS
GWAS is the most powerful statistical tool to analyze the association between traits and SNP markers, and various genetic models are applied to identify quantitative traits. Whole-genome resequencing data were imputed using the Beagle (Browning and Browning, 2007), and then, 3,110,974 SNPs for the rice core collection (273 accessions) were obtained from 808,686 SNPs by adjusting the minor allele frequency (MAF) to be less than 5% and removing the proportion of missing SNPs by 80%. In addition, accurate genome-scale profiling for As was performed by applying the linear mixed model (LMM).
The formula of the LMM is as follows:
where “y” is the observed phenotype, “β” indicates the marker information that is a fixed effect, “u” indicates the object information that is a random effect, “ε” is the random residual effect, and “X” and “Z” are the associated design metrics. The random effect assumes that u is proportional to the normal distribution for the mean and covariates [u~N(0, G)] and that ε is proportional to the normal distribution for the means and sum of the error squares [ε~N(0, I)] (Piepho et al., 2008).
On the contrary, in the 2016 and 2017 GWAS results applying GLM, the lambda values were 1.234507 and 1.58868, respectively, which were inflated compared with the LMM (0.781438 and 0.950722) (Supplementary File 4).
Significant QTLs with –log10(p) > 5 from GWAS results applied with LMM were selected, and linkage disequilibrium was analyzed by calculating allele frequency as r2 for each QTL. Candidate genes were identified at the range of ±50 kbp using genome browser of the Rice Annotation Project (https://rapdb.dna.affrc.go.jp/) (Kawahara et al., 2013; Sakai et al., 2013) for QTLs with a low recombination rate (r2 ≤ 1) (Supplementary File 5). In addition, functional haplotypes for candidate genes were investigated by using genomic sequence information, including the promoter and coding regions. The phenotypic variation for the haplotype group was statistically validated with a one-way ANOVA test.
Statistical and Network Analyses
Jamovi (version 1.6.12) was used for the correlation analysis between inorganic components. The one-way analysis of variance (ANOVA) was performed for genotypic differences (japonica, indica, and aus) of the rice core collection. Cytoscape (version 3.7.2) was used for the network analyses between inorganic components based on the Pearson correlation coefficient. However, the network analysis excluded Admixture and Aromatic collections with small sample numbers (n < 5).
Results
Variations in the Ionome Among the 273 Rice Accessions
The variations of As, Se, Na, Ca, Mn, Fe, Cu, Zn, and Mg contents accumulated in brown rice of 273 accessions were analyzed. Under the non-contaminated soil condition, As contents of rice varieties ranged from 0.0407 to 0.1775 mg kg−1, with an average content of 0.0833 mg kg−1. In 2016, As contents of varieties grown under contaminated soil conditions ranged from 0.1306 to 0.6923 mg kg−1, with an average content of 0.2768 mg kg−1. This average content increased by approximately 332% compared with the non-contaminated soil condition. The As content of the cultivars in 2017 was grown under the same polluted soil conditions as in 2016, As contents ranged from 0.0391 to 0.4184 mg kg−1, with an average of 0.1471 mg kg−1, a decrease of approximately 53% compared with the previous year. Functional inorganic components, such as Na, Ca, Mn, Fe, Cu, Zn, and Mg, showed statistically significant differences (p < 0.05) in all environmental conditions. It was confirmed that under the contaminated soil condition in 2017, other inorganic components except for Na, Cu, Fe, and Mg increased compared with the contaminated soil condition of the previous year (Figure 1). However, there were no significant differences in Se contents under the contaminated soil conditions in both years. In the 273 rice accessions grown in contaminated soil, the broad-sense heritability (H2) of As content in 2016 and 2017 was about 54%, which was found to be affected by both environmental and genetic factors (Figure 2).
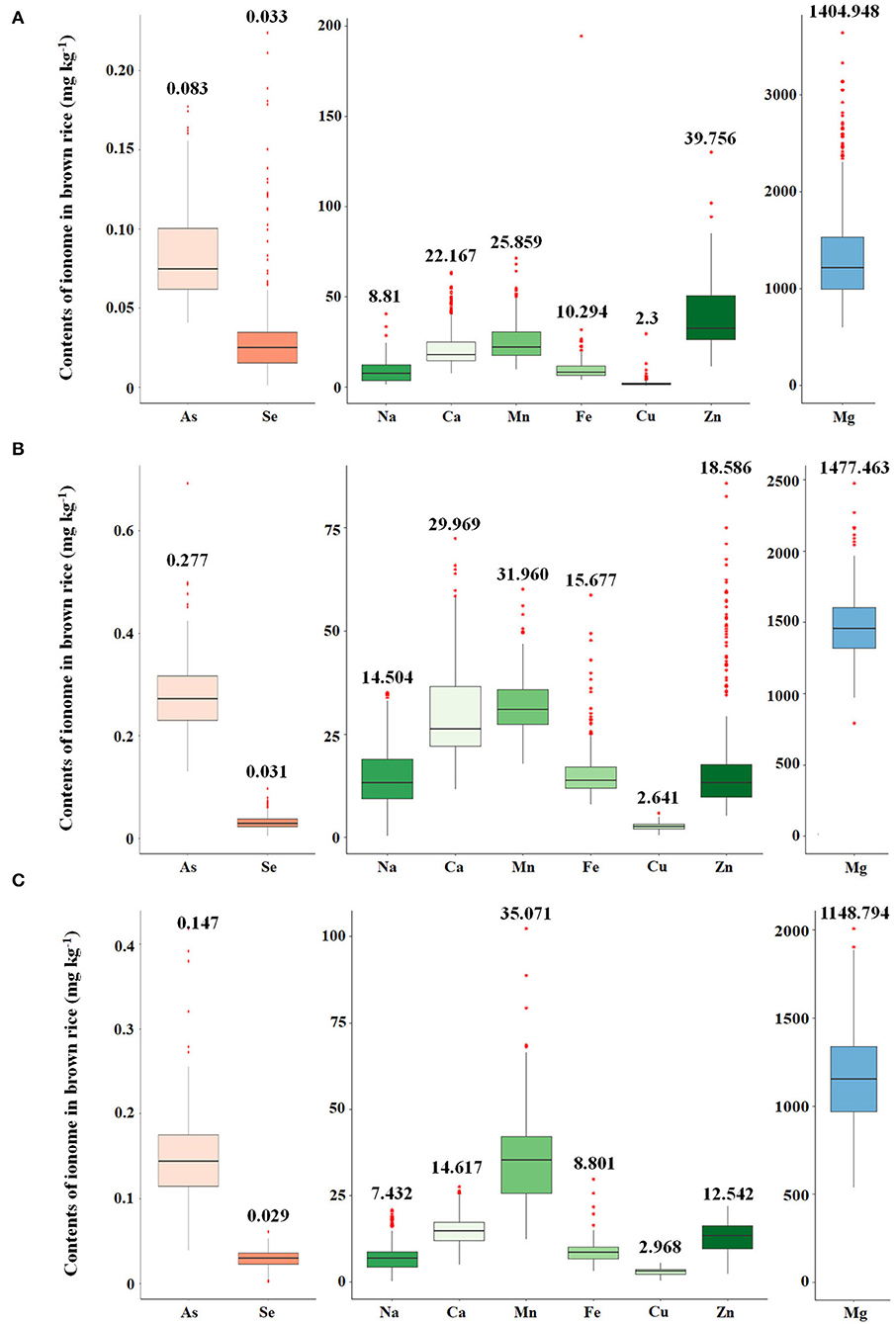
Figure 1. Variation of arsenic and functional ion content for the 273 rice accessions. (A) Ion contents in 273 rice grains in non-contaminated soil. (B) Ion contents in 273 rice grains under the flooded condition in contaminated soil. (C) Ion contents of 273 rice grains under the intermittently flooded condition in contaminated soil.
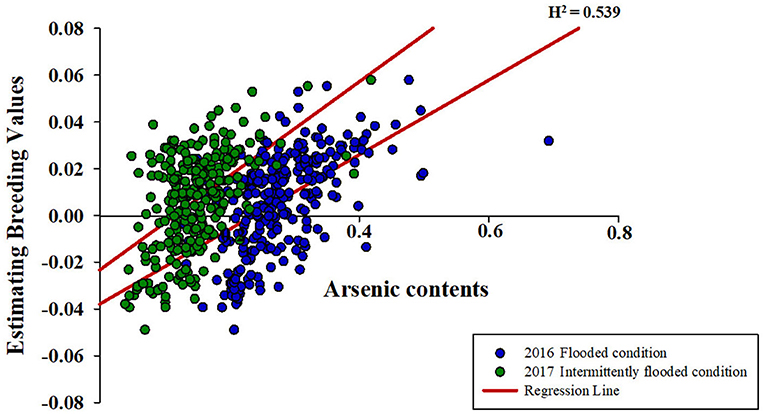
Figure 2. Correlations between estimating breeding values (EBVs) and arsenic contents. Green circle indicates arsenic contents of the 273 rice accessions grown under the flooded condition in contaminated soil. Blue circle indicates arsenic contents of the 273 rice accessions grown under the intermittently flooded condition in contaminated soil.
Interaction of Ionomes in Rice Core Collection
It was confirmed that the accumulation of ionomes in rice is affected by environmental conditions, and the contents of ionomes vary greatly depending on the rice varieties. This is predicted to be due to the genotypic differences due to subspecies, and the ionome content and their interactions were analyzed by classifying them into temperate japonica, tropical japonica, indica, and aus. Compared with other subspecies, temperate japonica exhibited more complex interactions between ionomes under both non-contaminated and contaminated soil conditions (Figures 3A,B). On the contrary, the correlations between ionomes in the rice grown under contaminated soil conditions were increased in all subspecies in the second year (2017) compared with that in the previous year (Figures 3B,C). The direction of correlation between As and other functional minerals varied depending on subspecies and environmental conditions.
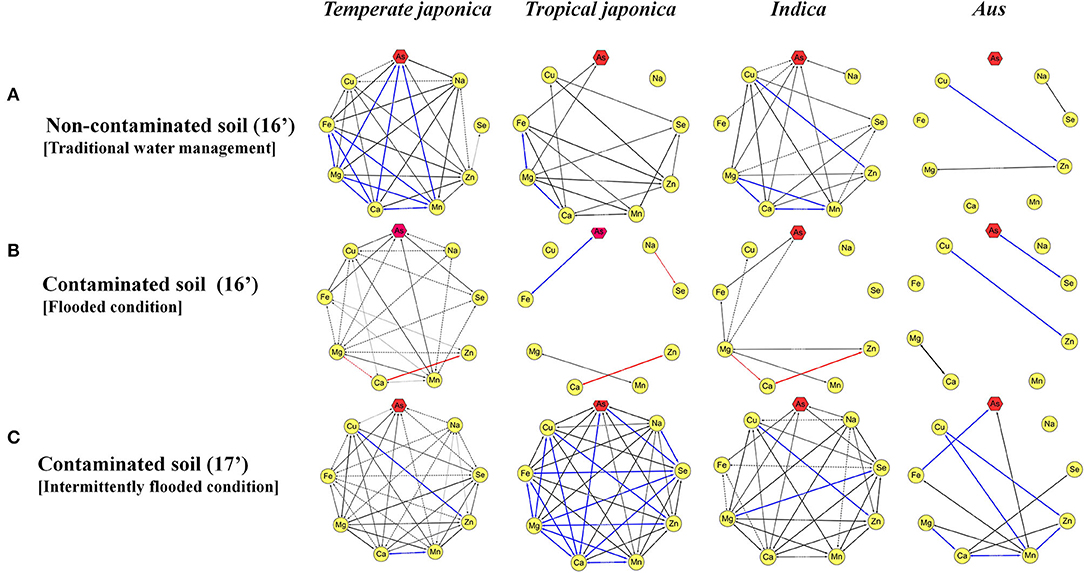
Figure 3. Interaction between arsenic and other inorganic components. The correlation between ionomes was based on the Pearson coefficient value. Blue line: positive correlation (0.8 < r <1.0), red line: negative correlation (−1.0 < r < −0.8), black line: positive correlation (0.4 < r <0.8), and dotted line: positive correlation (0.2 < r <0.4). (A) The interaction between ionomes of 273 rice grains in non-contaminated soil. (B) The interaction between ionomes of 273 rice grains under the flooded condition in contaminated soil. (C) The interaction between ionomes of 273 rice grains under the intermittently flooded condition in contaminated soil.
Co-Expression Network Analysis
The subspecies of rice were largely classified into japonica and indica population; then, the relationship between expression levels and genotypes of 23 genes known to be involved in As transport, detoxification, or As-induced stress response was analyzed for each population (Table 1). The expression networks for 23 As genes were performed using RNA-seq data from the rice accessions grown in unpolluted paddy soils.
The network average clustering coefficient for 23 As genes expression levels was 0.428 in the japonica population, which was larger than that in the indica population (0.414). That is, it was confirmed that the expression network of the indica population was denser than that of japonica, so seven essential genes (STR5, STR6, STR8, MYB1, multidrug and toxic compound extrusion 2 [MATE2], NIP1.1, and NIP3.2) were identified in the japonica population, and these genes are related to sulfur transferase and As transporter. Seven essential genes (STR5, STR8, GRX4, MRP1, ACR2.1, RCS, and AIR2) involved in sulfur transferase, As translation, and As induction were identified in the indica population (Figure 4). As a result of comparing As contents accumulated in grains of rice core collection cultivated in contaminated soil based on network analyses for rice subspecies, the As content of japonica under the flooded condition was 0.283 mg kg−1, which was significantly higher than that of indica (0.253 mg kg−1). In addition, the arsenic content of japonica was 0.153 even under the intermittently flooded condition, which was significantly higher than that of indica (0.126 mg kg−1).
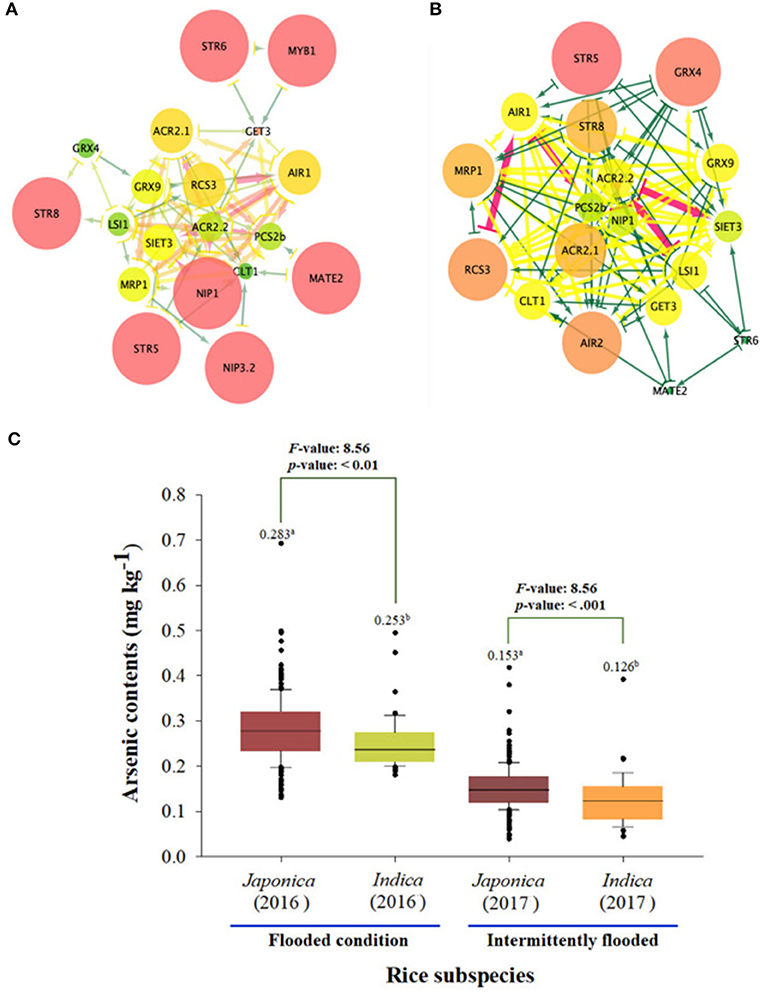
Figure 4. Expression network analysis of As genes between japonica and indica population. (A) The expression network of As-related genes within the japonica population. (B) The expression network of As-related genes within the indica population. (C) As content of japonica and indica under flooded and intermittently flooded conditions. a and b indicates whether there is a statistically significant difference between groups.
Expression QTL Analyzes
Twelve genes were identified as essential genes in the expression network for As genes. To identify the genetic factors regulating the expression of these genes, the association between the whole genome and the gene expression level was analyzed for the 273 rice accessions. As a result, the associations between expression levels and genotypes for STR5, STR8, and AIR2 were significant as -log10(p) > 5 (Figure 5). The expression levels of STR5 were associated with trans-eQTLs ranging from 25.4 to 33.6 Mb on chromosome 1, and the -log10(p) for trans-eQTLs ranged from 5.03 to 7.21 (Figure 5A). The 18,967 SNPs were identified at trans-eQTLs of STR5. These SNPs were clustered into three groups by variations, and 90% japonica varieties and 97% indica varieties were included in Group 1 and Group 3, respectively. STR5 expression levels of each group were 0.23, 0.35, and 0.30, but there was no statistical significance (Table 2). A total of 121 trans-eQTL genes were identified at the trans-eQTLs, including 5 at 25 Mb and 31 at 33 Mb. Os01g0635400 (protein-binding) and transcription-regulating Os01g0635550 (ZF-HD homeobox) were detected at 25 Mb, and genes involved in nodulin 20 (Os01g0786500), ABC transporter (Os01g0786000), peroxidase (Os01g0787000), and transcription factor (Os01g0788800) were detected at 33 Mb (Supplementary File 6).
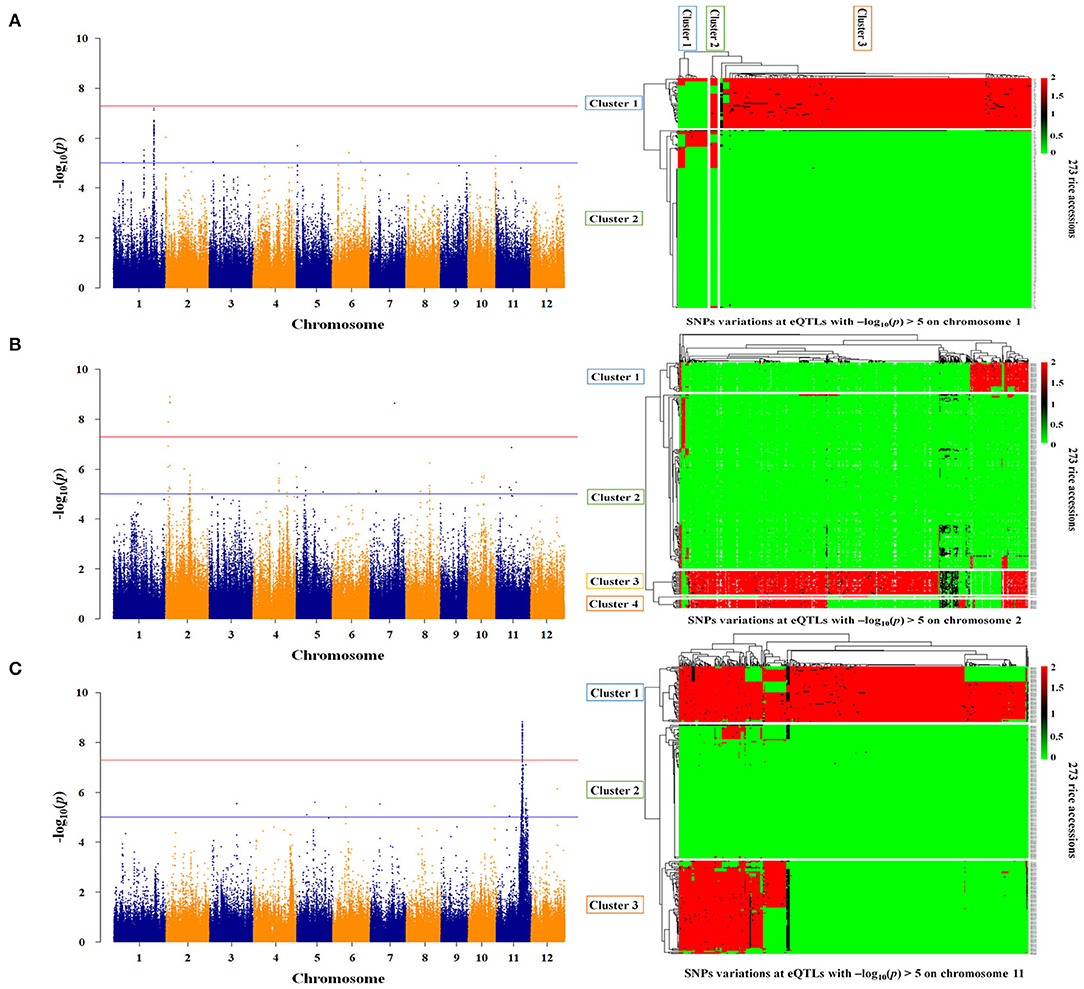
Figure 5. Regulatory factors associated with As gene expression. (A) Genome-scale profiling for STR5 expression levels, and SNPs variations at trans-eQTLs (25.4–33.6 Mb) in the japonica and indica populations. (B) Genome-scale profiling for STR8 expression levels and SNPs variations at cis-eQTLs (3.0–3.1 Mb and 19.4–20.0 Mb) in the japonica and indica populations. (C) Genome-scale profiling for AIR2 expression levels and SNPs variations at cis-eQTLs (19.2 Mb−25.8 Mb) in the japonica and indica populations. The major allele was indicated as 0 (green), the minor allele as 2 (red), and the heterologous allele as 1 (black).
The expression levels of STR8 and AIR2 showed high association at cis-eQTLs on chromosome 2 and chromosome 11, respectively. The cis-eQTLs for STR8 were identified at 3.0 to 3.1 Mb and 19.4 to 20.0 Mb on chromosome 2. The –log10(p) for cis-eQTLs ranged from 5.1 to 8.9 (Figure 5B). The 226 SNPs at 3 Mb were identified and clustered into three groups by SNPs variations. All japonica and 63% indica varieties were included in Group 1, and 26% indica varieties were included in Group 3. The expression levels of STR8 in each group were 0.22, 0.33, and 0.21, but there was no significant difference between groups. The 376 SNPs were identified and clustered into two groups at cis-eQTLs within 19.4–20 Mb. All japonica cultivars and 72% indica varieties were included in Groups 1 and 2, respectively, but there was no association between STR8 expression and SNPs variations at cis-eQTLs (Table 2). The 20 cis-eQTL genes were detected at cis-eQTLs of STR8. The cis-eQTLs genes involved in leucine-rich repeat domain (e.g., Os02g0156400) and arsenate reductase (Os02g0157600) were identified at 3 Mb. In addition, five cis-eQTLs genes, including chloroplast development protein (Os02g0539600), were identified at 19.2–20 Mb (Supplementary File 6).
The 15,388 SNPs were identified at 19.2 Mb−25.8 Mb associated with AIR2 expression, and -log10(p) for cis-eQTLs ranged from 5 to 8.84 (Figure 5C). The cis-eQTLs were clustered into three groups, and 75% japonica and all indica varieties were included in Groups 1 and 2, respectively. AIR2 expression levels were 1.89, 1.85, and 1.67 in each group, and there was a significant difference (p < 0.001) in Group 1 and Group 3 (Table 2). The 591 cis-eQTL genes for AIR2 were detected, and it was confirmed that a number of genes are related to leucine-rich repeat domains (e.g., Os11g0568800), serine/threonine kinase activity (Os11g0569300), heat shock (Os11g0578500), and RNA binding (Os11g0579900). In addition, the AIR2 was detected as a cis-eQTLs gene (Supplementary File 6).
QTLs Identification for As Content Through GWAS
To identify additional As-related genes other than known major genes and to investigate genetic factors associated with arsenic in plants by varying growing conditions under the same stress environment, we performed GWAS of the rice core collections, grown under contaminated soil conditions in 2016 and 2017. The contaminated soil was maintained under the flooded condition in 2016 and was maintained under the intermittently flooded condition in 2017. Under the flooded condition, As-associated QTLs (As-QTLs) were identified at 8.0 and 8.32 Mb on chromosome 6 and p-values were significant as 5.12 and 5.36, respectively (Figure 6A). As a result of LD (linkage disequilibrium) for As-QTLs, it was confirmed that the linkage equilibrium was weakly linked (R2 <0.5) within 8.32 Mb and strongly linked (R2 ≤ 1) between arsenic-related SNPs within 8.0 Mb (Supplementary File 5). Ten candidate genes were identified within ±50 kb based on 8.0 Mb, and a number of candidate genes were identified that respond to abiotic stress (Figure 6C). In particular, Os06g0254200 for ion channel activity and potassium transport, Os06g0254300 for calcium ion binding, and Os06g0255100, an iron-dependent oxygenase protein, were detected as As-related candidate genes (Supplementary File 7).
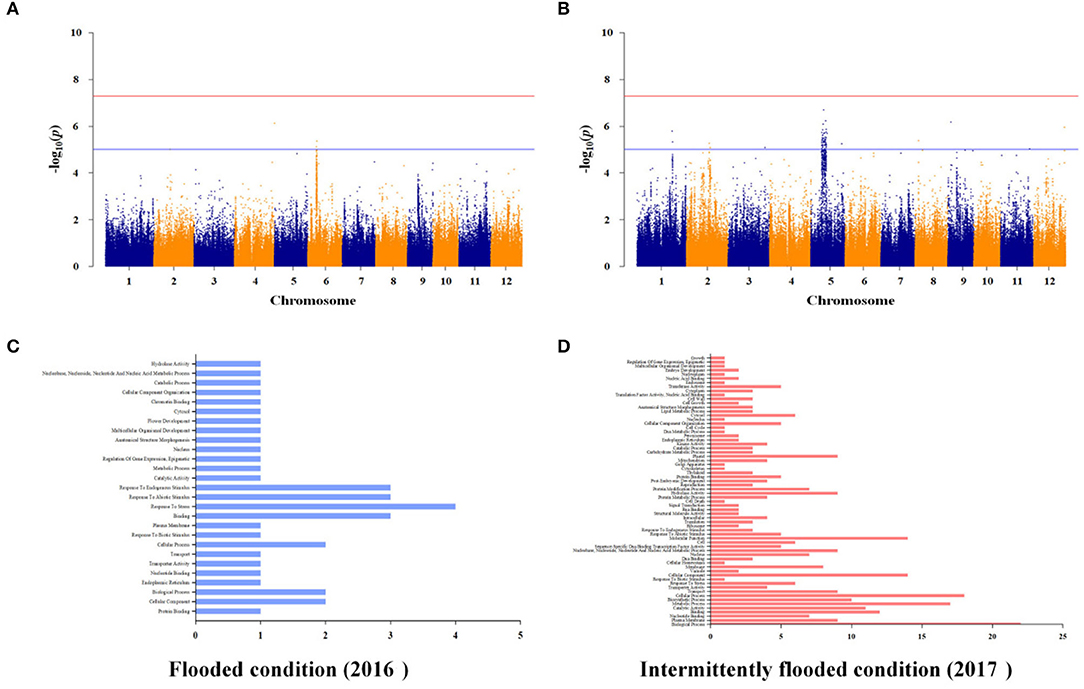
Figure 6. Whole genome-scale profiling for As under flooded and intermittently flooded conditions in contaminated soil. (A) Significant SNPs with -log10(p) > 5 were identified on chromosome 6 under the flooded condition. (B) Significant SNPs with -log10(p) > 5 identified on chromosomes 1 and 5 under the intermittently flooded condition. (C) Ten candidate genes under the flooded condition were identified within strongly linked SNPs (R2 ≤ 1), and a number of candidate genes were involved in abiotic stress response. (D) The 150 candidate genes under the intermittently flooded condition were identified within strongly linked SNPs (R2 ≤ 1), and a number of candidate genes were involved in biosynthetic process, membrane, and plastid.
Under the intermittently flooded condition, As-QTLs were identified from 30.7 to 30.8 Mb and 9.6 to 14.2 Mb and 27.1 Mb on chromosomes 1 and 5, respectively (Figure 6B). The p-values of As-QTLs ranged from 5 to 6.7, and linkage equilibrium was strongly linked with R2 ≤ 1 (Supplementary File 5). The 150 candidate genes on chromosomes 1 and 5 were identified within ±50 kb based on each As-QTL. Candidate genes involved in biosynthetic process, membrane, and plastid were detected under the intermittently flooded condition, unlike candidate genes identified under the flooded condition (Figure 6D). In addition, Os01g0733001 (NRAMP3), which transports metal ions, and Os05g0279400 (RFP), which regulates innate immunity and disease resistance, were detected at As-QTLs (Supplementary File 7). GWAS results for other functional ions are not presented in the main text and are given in Supplementary File 8.
Selection of Low-Arsenic Rice Varieties
AIR2 showed a significant difference in expression between japonica and indica at the cis-eQTL (p < 0.001), so it was verified whether the arsenic content differs by rice subspecies in genomic sequences of AIR2. The haplotype group for AIR2 was classified into three groups, and SNP variations between groups were confirmed in nonsynonymous SNPs (Ala134 → Thr134, Gly130 → Ser130, Ile101 → Thr101). The 104 japonica varieties belonged to Hap1, and the average As content under flooded and intermittently flooded conditions was 0.295 and 0.161 mg kg−1, respectively. Hap2 included 54 japonica and 18 indica varieties, and the average As content under flooded and intermittently flooded conditions was 0.261 and 0.143 mg kg−1, respectively. Hap3 included 19 japonica and 25 indica varieties, and the average As content under flooded and intermittently flooded conditions was 0.251 and 0.134 mg kg−1, respectively, which were significantly lower (p < 0.01) than those of Hap1 and Hap2.
The haplotype groups for AIR2 were similar to the groups clustered in the cis-eQTLs of AIR2. Based on the results of these analyses, rice varieties with low expression levels and low As content were selected from japonica and indica, respectively (Figure 7).
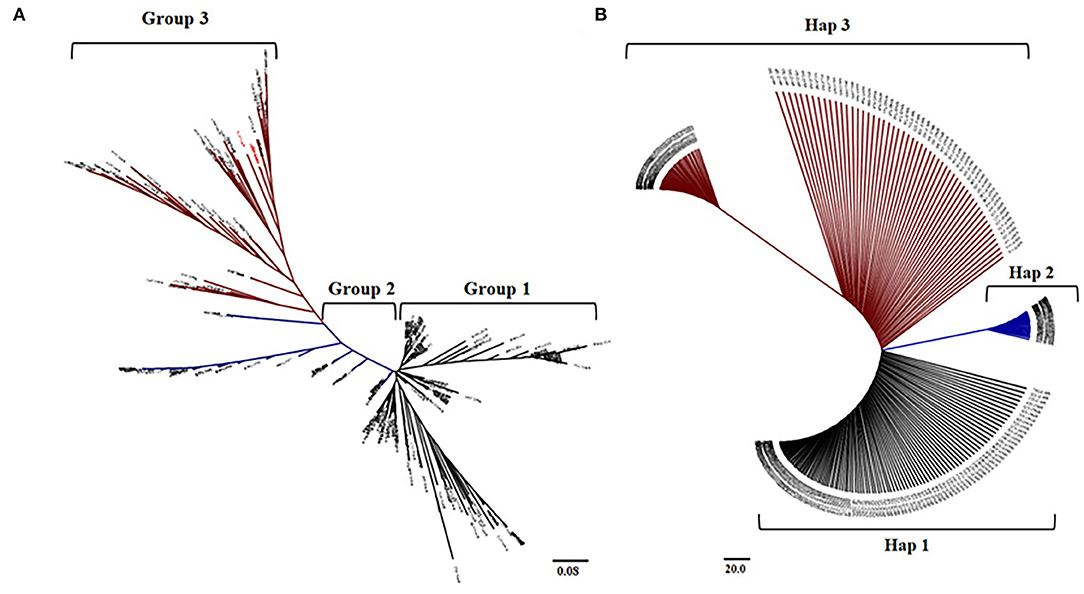
Figure 7. Comparison between haplotype group and cis-eQTLs of AIR2. (A) Neighbor-joining tree and clustering groups for 273 accessions at the cis-eQTLs associated with AIR2 expression levels. (B) Neighboring binding trees and haplotype groups of 273 accessions to the genomic sequence of AIR2.
Discussion
Variation and Interaction of Ionomes in Rice Core Collection
Partitioning the core collection based on rice subspecies and estimation of the interaction between As and other functional minerals demonstrated that environmental and genetic factors contribute to these interactions. The ionome accumulation of 273 rice grains cultivated in contaminated paddy soil was generally increased compared with unpolluted paddy soil conditions in the same year. Na, Mn, Fe, Cu, and Mg including arsenic increased, while Se, Ca, and Zn decreased in the contaminated soil (flooded condition). Nicula et al. (2013) investigated whether the properties of metals and the particularities of each species affect the uptake capacity in Phaseolus vulgaris and Zea mays and found that Fe, Cu, and Zn content of the two species was increased in contaminated soil compared with unpolluted soil. In addition, Williams et al. (2009) confirmed that Zn and Se significantly decreased in rice as the arsenic concentration increased. The results of these previous studies are consistent with the variations in ionome contents for the 273 rice accessions.
The correlations between ionomes were decreased in contaminated soil (flooded condition) compared with unpolluted soil, and it was confirmed that there was a difference by rice subspecies. The findings also revealed that the correlations between ionomes under the intermittently flooded condition were increased compared with those under the flooded condition. Therefore, it can be inferred that the contents and interaction between As and functional ions vary depending on the rice subspecies and water management. Although the genetic basis for the correlation between trace elements or toxic elements has not yet been established (Tan et al., 2020), studies have demonstrated the influence of subspecies on As, Hg, Pb, and Cd contents in japonica and indica rice (Meharg et al., 2009; Jiang et al., 2012; Norton et al., 2012). In addition, Wang et al. (2020) reported that the variation in accumulation and translocation of As among 74 main rice cultivars, including 66 japonica and 8 indica cultivars, was influenced by Si, P, Fe, and Mn contents.
Water management is an effective way to reduce the absorption of toxic ions, such as As, in crops. Rahaman and Ashim (2013) reported that arsenic content by approximately 24% is reduced and Fe and Zn are also reduced to lower levels under the intermittently flooded condition. Although there are environmental factors for the annual As content of 273 rice varieties, it is consistent with previous studies in that As content was decreased by 46.8% under the intermittently flooded condition compared with the flooded condition, and Na, Fe, and Zn also were decreased by 43.8 and 32.5%, respectively. However, the intermittently flooded condition induces oxidative stress and facilitates Cd accumulation, particularly in crops, so the rice core collections were classified into subspecies, and eQTL analyses were performed on 23 genes known as As induction or resistance to identify the genetic factors.
Co-Expression of As-Related Genes
STR5, STR6, STR8, MYB1, MATE, NIP1.1, and NIP3.2 were identified as essential proteins in the expression networks of 23 As genes of the japonica population, and the clustering coefficient of these genes was 0.5, which was larger than that of other genes. The clustering coefficient of GET3 was the smallest at 0.23. STR5, STR8, GRX4, MRP1, ACR2.1, RCS, and AIR2 were identified as essential genes with clustering coefficients ranging from 0.45 to 0.5 in the expression network of the indica population. The clustering coefficients of STR6 and MATE2 were the smallest at 0.23. The average clustering coefficient of japonica population was 4.28, which was larger than that of indica population, but the shortest paths accounted for 30% of the total path, which was less than that of indica. Here, the clustering coefficient describes the relatedness of two proteins and measures the importance of edges in a protein–protein interaction network, thus facilitating identification of key proteins in populations (Wang et al., 2012). That is, although many essential arsenic genes are present in the japonica population, the distance between As-induced and As-resistant genes is longer than that of indica, so it is likely to be easily exposed to the risk of As. This hypothesis was indirectly verified through the results of the As content of japonica and indica in the rice core collections grown in contaminated soil. On the contrary, the expression levels of STR5, STR8, and AIR2 among the 14 essential genes were -log10(p) > 5, which had high associations with the SNPs of core collections. Reportedly, sulfur assimilation and GSH are upregulated in response to oxidative stress in plants exposed to heavy metals (Na and Salt, 2011). Sulfur assimilation plays an important role in minimizing As absorption and transfers to crops and arsenic detoxification (Dixit et al., 2015). STR5, STR6, and STR8 are genes associated with sulfur assimilation and are known to regulate the As accumulation (Most and Papenbrock, 2015).
AIR2 (As-induced RING E3 ligase 2) encodes RING E3 ubiquitin ligase. Heterogeneous overexpression of OsAIR2 has been reported to positively regulate a plant growth in Arabidopsis or rice in response to As(V) stress. In addition, OsKAT1 (3-ketoacyl-CoA thiolase protein), the physical interaction partner of OsAIR2, is degraded and ubiquitinated by OsAIR2 through the 26S proteasome degradation pathway (Lim et al., 2014; Hwang et al., 2016, 2017). The eQTLs of STR5 and STR8 were not directly related to the gene expression, but the expression level of AIR2 showed a significant difference between groups at the cis-eQTLs. In particular, these cis-eQTLs include AIR2 genomic sequences, and homogeneous and heterogeneous SNPs were observed in Groups 1 (75% japonica) and 3 (100% indica), respectively. These findings suggest that SNP variations at the cis-eQTL associated with OsAIR2 regulate resistance to As stress, and it can be inferred that indica is less likely to accumulate As than japonica.
A number of trans and cis-eQTL genes were identified, such as nodulin 20, ABC transporters, leucine-rich repeat domains, and transcription factors at the eQTLs associated with the expression of STR5, STR8, and AIR2. Nodulin 20 (EN20) is a signaling mediator that activates plant defenses under stress, and OsABCC1, a type C ATP-binding cassette transporter (OsABCC) family, is known to detoxify As in grains (Wu et al., 2011; Song et al., 2014). In addition, the leucine-rich repeat domain (LRR) is involved in negative regulator-programmed cell death, tolerance to oxidative stress, and salt stress, but the biological function of most of the LRR gene has not been clearly elucidated in the plant genome (De Lorenzo et al., 2009; Oh et al., 2010; Pitorre et al., 2010; Hwang et al., 2011).
Identification of QTLs and Candidate Genes for as Content
In general, GWAS is the most powerful statistical method that provides a genetic basis for complex traits and has been applied to major agricultural traits, including toxic minerals, yield, flowering time, and disease resistance, among others, in rice (Huang et al., 2010, 2012; Famoso et al., 2011; McCouch et al., 2016). In rice, the alleles with large effects have been retained and fixed by evolution, human selection, and inbreeding, and therefore, GWAS is widely used to investigate these complex alleles in rice to uncover the genetic basis and effects associated with various traits.
In this study, GWAS was performed by applying LMM to arsenic content of the rice core collection under different water management conditions in the contaminated soil to uncover the genetic factors associated with As. GWAS results applied with LMM were verified by comparison with a general linear model (GLM). A lambda value ranging from 0 to 1 is a measure of asymmetric association indicating the strength of the relationship between the independent and dependent variables and is used to validate the p-value from the GWAS results (Hartwig, 1973; Kim et al., 2019).
As-associated SNPs were mapped on chromosomes 6 and 5 under flooded conditions and intermittently flooded conditions, respectively. The association of arsenic with the genome in crops may vary depending on growing conditions. Norton et al. (2019) reported various QTLs associated with arsenic through annual comparisons by water management systems. Moreover, it is known that microorganisms directly or indirectly affect the mobility and biological availability of arsenic in rice paddies, depending on the water management system; then, arsenic uptake and translocation can be decreased or increased by altered expression of transporter genes in plants (Kumarathilaka et al., 2018b). Concordant with the findings of previous studies, this study confirmed that As-related QTLs showed different patterns under water management conditions and that there were several candidate genes involved in physiological responses under intermittently flooded conditions. In a follow-up study, it is necessary not only to verify these candidate genes based on the GWAS results for As, but also to further prove the genetic relationship between arsenic and functional ions by exploring SNPs co-localized with other functional ions.
Selection of Low-Arsenic Rice Varieties
The 273 rice accessions were classified according to rice subspecies in genomic sequences and cis-eQTLs of AIR2. The groups clustered in cis-eQTLs mostly coincided with the haplotype groups of AIR2, and indica varieties had lower AIR2 expression and As content compared with japonica. These results suggest that indica is relatively less likely to be exposed to As risk compared with japonica and also means that low expression of AIR2 can reduce As accumulation in rice. Therefore, rice varieties (eight indica, two temperate japonica, and two tropical japonica) with low AIR2 expression and low As content were finally selected based on these results.
Conclusion
The correlations between arsenic and functional ions were affected by rice subspecies and growing condition through the water management. As was reduced under the intermittently flooded condition compared with the flooded condition, but As reduction through the water management has limitations in terms of the rice-growing condition. Therefore, it was verified that the arsenic accumulation was lower in indica than in japonica through the association studies between transcriptome and genome data of rice core collections. AIR2 (arsenic-induced RING finger protein) expression for indica was relatively lower than that of japonica in its cis-eQTLs, and it was confirmed that the arsenic content of the group including a number of indica was also low in haplotype group for AIR2. Finally, rice varieties with low AIR2 expression and low arsenic content were selected. Therefore, the selected rice varieties are valuable as breeding materials, so verification through follow-up studies is required. In addition, it is necessary to develop a low-arsenic marker that can be applied to all rice varieties by converting three nonsynonymous SNPs into KASP markers in future through the SNP mutation information of AIR2.
Data Availability Statement
The whole genome resequencing information for the rice core collection (273 rice accessions) is available in “Rice evolution analysis based on the chloroplast genome” by NCBI (https://www.ncbi.nlm.nih.gov/).
Author Contributions
S-BL and S-WP designed the research. S-BL performed the experiments and wrote the initial manuscript. J-DS provided technical advice. G-JK and G-HC analyzed arsenic contents of rice core collection. S-WP and Y-JP supervised the study. Y-JP preprocessed rice whole-genome resequencing information and whole transcriptome data used in this study. S-KP, J-DS, and WC reviewed the manuscript. All authors contributed to the article and approved the submitted version.
Funding
This study was funded by the Next-Biogreen 21 Programme, under grant agreement no. PJ013405, Studies on the metabolic behavior of harmful substances, such as soil persistence pesticides and crop uptake no. PJ01594403, and Development of low-allergy soybean breeding materials and molecular marker to improve added value no. PJ01678402.
Conflict of Interest
J-DS was employed by Bio-Technology of Multidisciplinary Sciences Co.
The remaining authors declare that the research was conducted in the absence of any commercial or financial relationships that could be construed as a potential conflict of interest.
Publisher's Note
All claims expressed in this article are solely those of the authors and do not necessarily represent those of their affiliated organizations, or those of the publisher, the editors and the reviewers. Any product that may be evaluated in this article, or claim that may be made by its manufacturer, is not guaranteed or endorsed by the publisher.
Acknowledgments
We thank Dool-Yi Kim, Mi-Suk Seo, Jung-Kyung Moon, and Yuna Kim at the National Institute of Crop Science (NICS) and Kyu-Won Kim, Sang-Ho Chu, and Myeong-Hyeon Min at the Kongju National University for their assistance in carrying out the project. We also thank Kyeong Ae Son and Ji-Hyock Ryu at the National Institute of Agricultural Sciences (NIAS) for providing technical advice and support during fieldwork.
Supplementary Material
The Supplementary Material for this article can be found online at: https://www.frontiersin.org/articles/10.3389/fpls.2022.905842/full#supplementary-material
Supplementary File 1. Regional climate information for 2016 and 2017 in Yesan-gun, Chungcheongnam-do, Republic of Korea.
Supplementary File 2. DNA sequencing and rice core collection information for 279 accessions.
Supplementary File 3. Expression levels (FPKM) of arsenic genes for 234 rice accessions.
Supplementary File 4. Differences between genetic models applied to GWAS for arsenic content in brown rice. It was verified that the p-value of the GWAS result was inflated using the lambda values (a value between 0 and 1). (A) GWAS results of applying the general linear model (GLM) and the linear mixed model (LMM) to the arsenic content under the flooded condition. (B) GWAS results of applying the general linear model (GLM) and the linear mixed model (LMM) to the arsenic content under the intermittently flooded condition.
Supplementary File 5. Linkage disequilibrium for As-related QTLs. (A,B) Linkage disequilibrium for arsenic-associated QTLs on chromosome 6 under the flooded condition. (C–E) Linkage disequilibrium for arsenic-associated QTLs on chromosome 5 under the intermittently flooded condition. (F,G) Linkage disequilibrium for arsenic-associated QTLs on chromosome 1 under the intermittently flooded condition.
Supplementary File 6. eQTLs candidate genes associated with STR5, STR8, and AIR2 expression levels.
Supplementary File 7. Candidate genes identified from GWAS for arsenic contents under flooded and intermittently flooded conditions.
Supplementary File 8. Genome-wide scale profiling for functional ions. (A) QTLs associated with functional ions in brown rice under the flooded condition. (B) QTLs associated with functional ions in brown rice under the intermittently flooded condition.
References
Baxter, I. (2009). Ionomics: studying the social network of mineral nutrients. Curr. Opin. Plant Biol. 12, 381–386. doi: 10.1016/j.pbi.2009.05.002
Baxter, I. (2010). Ionomics: The functional genomics of elements. Brief. Funct. Genomics 9, 149–156. doi: 10.1093/bfgp/elp055
Bienert, G. P., Thorsen, M., Schüssler, M. D., Nilsson, H. R., Wagner, A., Tamás, M. J., et al. (2008). A subgroup of plant aquaporins facilitate the bi-directional diffusion of As(OH)3 and Sb(OH)3 across membranes. BMC Biol. 6, 1–15. doi: 10.1186/1741-7007-6-26
Browning, B. L., and Browning, S. R. (2007). Efficient multilocus association testing for whole genome association studies using localized haplotype clustering. Genet. Epidemiol. 31, 365–375. doi: 10.1002/gepi.20216
Chen, W. Q., Shi, Y. L., Wu, S. L., and Zhu, Y. G. (2016). Anthropogenic arsenic cycles: a research framework and features. J. Clean. Prod. 139, 328–336 doi: 10.1016/j.jclepro.2016.08.050
Danku, J. M. C., Lahner, B., Yakubova, E., and Salt, D. E. (2013). Large-Scale Plant Ionomics. Plant Mineral Nutrients. Totowa, NJ: Humana Press.
Das, C. K., Bastia, D., Swain, S. C., and Mahapatra, S. S. (2018a). Computational analysis of genes encoding for molecular determinants of arsenic tolerance in rice (Oryza sativa L.) to engineer low arsenic content varieties. ORYZA-An Int. J. Rice 55, 248–259. doi: 10.5958/2249-5266.2018.00031.0
Das, N., Bhattacharya, S., Bhattacharyya, S., and Maiti, M. K. (2017). Identification of alternatively spliced transcripts of rice phytochelatin synthase 2 gene OsPCS2 involved in mitigation of cadmium and arsenic stresses. Plant Mol. Biol. 94, 167–183. doi: 10.1007/s11103-017-0600-1
Das, N., Bhattacharya, S., Bhattacharyya, S., and Maiti, M. K. (2018b). Expression of rice MATE family transporter OsMATE2 modulates arsenic accumulation in tobacco and rice. Plant Mol. Biol. 98, 101–120. doi: 10.1007/s11103-018-0766-1
De Lorenzo, L., Merchan, F., Laporte, P., Thompson, R., Clarke, J., Sousa, C., et al. (2009). A novel plant leucine-rich repeat receptor kinase regulates the response of Medicago truncatula roots to salt stress. Plant Cell 21, 668–680. doi: 10.1105/tpc.108.059576
Dixit, G., Singh, A. P., Kumar, A., Dwivedi, S., Deeba, F., Kumar, S., et al. (2015). Sulfur alleviates arsenic toxicity by reducing its accumulation and modulating proteome, amino acids and thiol metabolism in rice leaves. Sci. Rep. 5, 1–16. doi: 10.1038/srep16205
Duxbury, J. M., Mayer, A. B., Lauren, J. G., and Hassan, N. (2003). Food Chain aspects of arsenic contamination in bangladesh: effects on quality and productivity of rice. J. Environ. Sci. Health A 38, 61–69 doi: 10.1081/ESE-120016881
Famoso, A. N., Zhao, K., Clark, R. T., Tung, C. W., Wright, M. H., Bustamante, C., et al. (2011). Genetic architecture of aluminum tolerance in rice (Oryza sativa) determined through genome-wide association analysis and QTL mapping. PLoS Genet. 7, e1002221. doi: 10.1371/journal.pgen.1002221
Finnegan, P. M., and Chen, W. (2012). Arsenic toxicity: the effects on plant metabolism. Front. Physiol. 3, 182. doi: 10.3389/fphys.2012.00182
Garcia-Oliveira, A. L., Tan, L., Fu, Y., and Sun, C. (2009). Genetic identification of quantitative trait loci for contents of mineral nutrients in rice grain. J. Integr. Plant Biol. 51, 84–92. doi: 10.1111/j.1744-7909.2008.00730.x
Hartwig, F. (1973). Statistical significance of the lambda coefficients. Behav. Sci. 18, 307–310 doi: 10.1002/bs.3830180409
Heo, E. B., Yoo, J. M., Lee, W. D., Chu, S. H., Kim, K. W., Cho, Y. H., et al. (2017). Integrated genome-wide association studies to dissect natural variation for magnesium ion contents in rice germplasm. Korean J. Breed. Sci. 49, 141–149. doi: 10.9787/KJBS.2017.49.3.141
Hu, P., Huang, J., Ouyang, Y., Wu, L., Song, J., Wang, S., et al. (2013). Water management affects arsenic and cadmium accumulation in different rice cultivars. Environ. Geochem. Health. 35, 767–778. doi: 10.1007/s10653-013-9533-z
Huang, X., Wei, X., Sang, T., Zhao, Q., Feng, Q., Zhao, Y., et al. (2010). Genome-wide association studies of 14 agronomic traits in rice landraces. Nat. Genet. 42, 961–967. doi: 10.1038/ng.695
Huang, X., Zhao, Y., Wei, X., Li, C., Wang, A., Zhao, Q., et al. (2012). Genome-wide association study of flowering time and grain yield traits in a worldwide collection of rice germplasm. Nat. Genet. 44, 32–39. doi: 10.1038/ng.1018
Huang, X. Y., and Salt, D. E. (2016). Plant ionomics: from elemental profiling to environmental adaptation. Mol. Plant. 9, 787–797. doi: 10.1016/j.molp.2016.05.003
Hwang, S. G., Chapagain, S., Han, A. R., Park, Y. C., Park, H. M., Kim, Y. H., et al. (2017). Molecular characterization of rice arsenic-induced RING finger E3 ligase 2 (OsAIR2) and its heterogeneous overexpression in Arabidopsis thaliana. Physiol. Plant. 161, 372–384. doi: 10.1111/ppl.12607
Hwang, S. G., Kim, D. S., and Jang, C. S. (2011). Comparative analysis of evolutionary dynamics of genes encoding leucine-rich repeat receptor-like kinase between rice and Arabidopsis. Genetica 139, 1023–1032. doi: 10.1007/s10709-011-9604-y
Hwang, S. G., Park, H. M., Han, A. R., and Jang, C. S. (2016). Molecular characterization of Oryza sativa arsenic-induced RING E3 ligase 1 (OsAIR1): expression patterns, localization, functional interaction, and heterogeneous overexpression. J. Plant Physiol. 191, 140–148. doi: 10.1016/j.jplph.2015.12.010
Isayenkov, S. V., and Matouis, F. J. M. (2008). The Arabidopsis thaliana aquaglyceroporin AtNIP7;1 is a pathway for arsenite uptake. FEBS Lett. 582, 1625–1628. doi: 10.1016/j.febslet.2008.04.022
Jiang, S., Shi, C., and Wu, J. (2012). Genotypic differences in arsenic, mercury, lead and cadmium in milled rice (Oryza sativa L.). Int. J. Food Sci. Nutr. 63, 468–475. doi: 10.3109/09637486.2011.636343
Kang, D. W., Kim, D. Y., Yoo, J. H., Park, S. W., Oh, K. S., Kwon, O. K., et al. (2018). Effect of soil amendments on arsenic reduction of brown rice in paddy fields. Korean J. Soil Sci. Fertilizer. 51, 101–110. doi: 10.7745/KJSSF.2018.51.2.101
Kawahara, Y., de la Bastide, M., Hamilton, J. P., Kanamori, H., McCombie, W. R., Ouyang, S., et al. (2013). Improvement of the Oryza sativa Nipponbare reference genome using next generation sequence and optical map data. Rice 6, 1–10. doi: 10.1186/1939-8433-6-4
Kim, B., Dai, X., Zhang, W., Zhuang, Z., Sanchez, D. L., Lübberstedt, T., et al. (2019). GWASpro: a high-performance genome-wide association analysis server. Bioinformatics 35, 2512–2514. doi: 10.1093/bioinformatics/bty989
Kim, K. W., Chung, H. K., Cho, G. T., Ma, K. H., Chandrabalan, D., Gwag, J. G., et al. (2007). PowerCore: a program applying the advanced M strategy with a heuristic search for establishing core sets. Bioinformatics 23, 2155–2162. doi: 10.1093/bioinformatics/btm313
Kirk, C., Jensen, M., Kjaer, C. N., Smedskjaer, M. M., Larsen, K. L., Wimmer, R., et al. (2009). Aqueous batch rebinding and selectivity studies on sucrose imprinted polymers. Biosensors Bioelectr. 25, 623–628. doi: 10.1016/j.bios.2009.01.021
Kumarathilaka, P., Seneweera, S., Meharg, A., and Bundschuh, J. (2018a). Arsenic speciation dynamics in paddy rice soil-water environment: sources, physico-chemical, and biological factors-a review. Water Res. 140, 403–414. doi: 10.1016/j.watres.2018.04.034
Kumarathilaka, P., Seneweera, S., Meharg, A., and Bundschuh, J. (2018b). Arsenic accumulation in rice (Oryza sativa L.) is influenced by environment and genetic factors. Sci. Total Environ. 642, 485–496. doi: 10.1016/j.scitotenv.2018.06.030
Lahner, B., Gong, J., Mahmoudian, M., Smith, E. L., Abid, K. B., Rogers, E. E., et al. (2003). Genomic scale profiling of nutrient and trace elements in Arabidopsis thaliana. Nat. Biotechnol. 21, 1215–1221. doi: 10.1038/nbt865
Latowski, D., Kowalczyk, A., Nawieśniak, K., and Listwan, S. (2018). “Arsenic uptake and transportation in plants,” in Mechanisms of Arsenic Toxicity and Tolerance in Plants (Singapore: Springer), 1–26.
Le Sech, L., and Christian, H. (1991). Diallel analysis in white lupin: consequences for breeding. Agronomie 11, 719–726. doi: 10.1051/agro:19910902
Li, L., Ye, H., Guo, H., and Yin, Y. (2010). Arabidopsis IWS1 interacts with transcription factor BES1 and is involved in plant steroid hormone brassinosteroid regulated gene expression. Proc. Natl. Acad. Sci. U.S.A. 107, 3918–3923. doi: 10.1073/pnas.0909198107
Liao, J. L., Zhou, H. W., Peng, Q., Zhong, P. A., Zhang, H. Y., He, C., et al. (2015). Transcriptome changes in rice (Oryza sativa L.) in response to high night temperature stress at the early milky stage. BMC Genomics 16, 18. doi: 10.1186/s12864-015-1222-0
Liao, V. H. C., and Ou, K. L. (2005). Development and testing of a green fluorescent protein-based bacterial biosensor for measuring bioavailable arsenic in contaminated groundwater samples. Environ. Toxicol. Chem. 24, 1624–1631. doi: 10.1897/04-500R.1
Lim, S. D., Hwang, J. G., Han, A. R., Park, Y. C., Lee, C., Ok, Y. S., et al. (2014). Positive regulation of rice RING E3 ligase OsHIR1 in arsenic and cadmium uptakes. Plant Mol. Biol. 85, 365–379. doi: 10.1007/s11103-014-0190-0
Lu, F. H., and Park, Y. J. (2012a). Sequence variations in OsAGPase significantly associated with amylose content and viscosity properties in rice (Oryza sativa L.). Genet. Res. 94, 179–189. doi: 10.1017/S0016672312000390
Lu, F. H., and Park, Y. J. (2012b). An SNP downstream of the OsBEIIb gene is significantly associated with amylose content and viscosity properties in rice (Oryza sativa L.). J. Cereal Sci. 56, 706–712. doi: 10.1016/j.jcs.2012.08.007
Ma, J. F., Yamaji, N., Mitani, N., Xu, X. Y., Su, Y. H., McGrath, S. P., et al. (2008). Transporters of arsenite in rice and their role in arsenic accumulation in rice grain. Proc. Natl. Acad. Sci. U.S.A. 105, 9931–9935. doi: 10.1073/pnas.0802361105
McCouch, S. R., Wright, M. H., Tung, C. W., Maron, L. G., McNally, K. L., Fitzgerald, M., et al. (2016). Open access resources for genome-wide association mapping in rice. Nature 7, 1–14. doi: 10.1038/ncomms10532
Meharg, A. A., Williams, P. N., Adomako, A., Lawgali, Y. Y., Deacon, C., Villada, A., et al. (2009). Geographical variation in total and inorganic arsenic content of polished (White) rice. Environ. Sci. Technol. 43, 1612–1617. doi: 10.1021/es802612a
Most, P., and Papenbrock, J. (2015). Possible roles of plant sulfurtransferases in detoxification of cyanide, reactive oxygen species, selected heavy metals and arsenate. Molecules 20, 1410–1423. doi: 10.3390/molecules20011410
Muehe, E. M., Wang, T., Kerl, C. F., Planer-Friedrich, B., and Fendorf, S. (2019). Rice production threatened by coupled stresses of climate and soil arsenic. Nat. Commun. 10, 1–10. doi: 10.1038/s41467-019-12946-4
Na, G. N., and Salt, D. E. (2011). The role of sulfur assimilation and sulfur-containing compounds in trace element homeostasis in plants. Environ. Exp. Bot. 72, 18–25. doi: 10.1016/j.envexpbot.2010.04.004
NAAS (2010). Analysis methods for soil chemical properties. National Academy of Agriculture Science, Republic of Korea Publication No. 11-1390802-000282-01.
Nguyen, Q. T. T., Huang, T. L., and Huang, H. J. (2014). Identification of genes related to arsenic detoxification in rice roots using microarray analysis. Int. J. Biosci. Biochem. Bioinform. 4, 22. doi: 10.7763/IJBBB.2014.V4.304
Nicula, C., Peter, A., Mihaly-Cozmuta, L., and Mihaly-Cozmuta, A. (2013). The uptake of heavy metals in phaseolus vulgaris and zea mays seeds harvested from polluted and unpolluted areas. Carpathian. J. Food Sci. Technol. 5, 1–8. Available online at: http://stiinte.ubm.ro/multimedia/Chimie/index.html
Norton, G. J., Pinson, S. R. M., Alexander, J., Mckay, S., Hansen, H., Duan, G. L., et al. (2012). Variation in grain arsenic assessed in a diverse panel of rice (Oryza sativa) grown in multiple sites. New Phytol. 193, 650–664. doi: 10.1111/j.1469-8137.2011.03983.x
Norton, G. J., Travis, A. J., Talukdar, P., Hossain, M., Islam, M. R., Douglas, A., et al. (2019). Genetic loci regulating arsenic content in rice grains when grown flooded or under alternative wetting and drying irrigation. Rice 12, 1–15. doi: 10.1186/s12284-019-0307-9
Oh, M. H., Wang, X., Wu, X., Zhao, Y., Clouse, S. D., and Huber, S. C. (2010). Autophosphorylation of Tyr-610 in the receptor kinase BAK1 plays a role in brassinosteroid signaling and basal defense gene expression. Proc. Natl. Acad. Sci. U.S.A. 107, 17827–17832. doi: 10.1073/pnas.0915064107
Paszkowski, U., Kroken, S., Roux, C., and Briggs, S. P. (2002). Rice phosphate transporters include an evolutionarily divergent gene specifically activated in arbuscular mycorrhizal symbiosis. Proc. Natl. Acad. Sci. U.S.A. 99, 13324–13329. doi: 10.1073/pnas.202474599
Piepho, H. P., Möhring, J., Melchinger, A. E., and Büchse, A. (2008). BLUP for phenotypic selection in plant breeding and variety testing. Euphytica 161, 209–228. doi: 10.1007/s10681-007-9449-8
Pitorre, D., Llauro, C., Jobet, E., Guilleminot, J., Brizard, J. P., Delseny, M., et al. (2010). RLK7, a leucine-rich repeat receptor-like kinase, is required for proper germination speed and tolerance to oxidative stress in Arabidopsis thaliana. Planta 232, 1339–1353. doi: 10.1007/s00425-010-1260-4
QTLmax Global. (2022). QTLMax 3.0: An Analytical Toolset for Enterprises in Agribusiness. Katy, TX. Available online at: https://www.qtlmax.com
Rahaman, S., and Ashim, C. S. (2013). Water regimes: an approach of mitigation arsenic in summer rice (Oryza sativa L.) under different topo sequences on arsenic-contaminated soils of Bengal delta. Paddy Water Environ. 11, 397–410. doi: 10.1007/s10333-012-0331-5
Sakai, H., Lee, S. S., Tanaka, T., Numa, H., Kim, J., Kawahara, Y., et al. (2013). Rice annotation project database (RAP-DB): an integrative and interactive database for rice genomics. Plant Cell Physiol. 54, e6–e6. doi: 10.1093/pcp/pcs183
Salt, D. E. (2017). Would the real arsenate reductase please stand up?. New Phytol. 215, 926–928. doi: 10.1111/nph.14691
Salt, D. E., Baxter, I., and Lahner, B. (2008). Ionomics and the study of the plant ionome. Annu. Rev. Plant Biol. 59, 709–733. doi: 10.1146/annurev.arplant.59.032607.092942
Shi, S., Wang, T., Chen, Z., Tang, Z., Wu, Z., Salt, D. E., et al. (2016). OsHAC1; 1 and OsHAC1; 2 function as arsenate reductases and regulate arsenic accumulation. Plant Physiol. 172, 1708–1719. doi: 10.1104/pp.16.01332
Singh, P., Khan, A., and Srivastava, A. (2021). “Biological means of arsenic minimization with special reference to siderophore,” in Arsenic Toxicity: Challenges and Solutions (Singapore: Springer), 253–278.
Song, W. Y., Yamaki, T., Yamaji, N., Ko, D., Jung, K. H., Fujii-Kashino, M., et al. (2014). A rice ABC transporter, OsABCC1, reduces arsenic accumulation in the grain. Proc. Natl. Acad. Sci. U.S.A. 111, 15699–15704. doi: 10.1073/pnas.1414968111
Sun, S. K., Chen, Y., Che, J., Konishi, N., Tang, Z., Miller, A. J., et al. (2018). Decreasing arsenic accumulation in rice by overexpressing Os NIP 1; 1 and Os NIP 3; 3 through disrupting arsenite radial transport in roots. New Phytol. 219, 641–653. doi: 10.1111/nph.15190
Tan, Y., Sun, L., Song, Q., Mao, D., Zhou, J., Jiang, Y., et al. (2020). Genetic architecture of subspecies divergence in trace mineral accumulation and elemental correlations in the rice grain. Theor. Appl. Genet. 133, 529–545. doi: 10.1007/s00122-019-03485-z
Tiwari, P., Indoliya, Y., Chauhan, A. S., Singh, P., Singh, P. K., Singh, P. C., et al. (2020). Auxin-salicylic acid cross-talk ameliorates OsMYB–R1 mediated defense towards heavy metal, drought and fungal stress. J. Hazard. Mater. 399, 122811. doi: 10.1016/j.jhazmat.2020.122811
Ullrich-eberius, C. I., Sanz, A., and Novacky, A. J. (1989). Evaluation of arsenate- and vanadate-associated changes of electrical membrane potential and phosphate transport in lemna gibba G1. J.Exp. Bot. 40, 119–128. doi: 10.1093/jxb/40.1.119
Wang, C., Tang, Z., and Zhao, F. J. (2019). “OsRCS3 functions as a cytosolic O-acetylserine (thiol) lyase and regulates arsenic accumulation in rice,” in Environmental Arsenic in a Changing World: Proceedings of the 7th International Congress and Exhibition on Arsenic in the Environment (AS 2018), July 1-6, 2018, Beijing, PR China (Beijing: CRC Press), 343.
Wang, J., Li, M., Wang, H., and Pan, Y. (2012). Identification of essential proteins based on edge clustering coefficient. IEEE/ACM Trans. Comput. Biol. Bioinform. 9, 1070–1080. doi: 10.1109/TCBB.2011.147
Wang, Y., Lv, K., Shi, C., Shi, C., Li, Y., Chen, X., et al. (2020). Variation in arsenic accumulation and translocation among 74 main rice cultivars in Jiangsu Province, China. Environ. Sci. Pollut. Res. 27, 26249–26261. doi: 10.1007/s11356-020-08994-9
Williams, P. N., Islam, S., Islam, R., Jahiruddin, M., Adomako, E., Soliaman, A. R. M., et al. (2009). Arsenic limits trace mineral nutrition (selenium, zinc, and nickel) in Bangladesh rice grain. Environ. Sci. Technol. 43, 8430–8436. doi: 10.1021/es901825t
Williams, P. N., Villada, A., Deacon, C, Raab, A., Figuerola, J., Green, A. J., et al. (2007). Greatly enhanced arsenic shoot assimilation in rice leads to elevated grain levels compared to wheat and barley. Environ. Sci. Technol. 41, 6854–6859. doi: 10.1021/es070627i
Wu, H., Shen, Y., Hu, Y., Tan, S., and Lin, Z. (2011). A phytocyanin-related early nodulin-like gene, BcBCP1, cloned from Boea crassifolia enhances osmotic tolerance in transgenic tobacco. J. Plant Physiol. 168, 935–943. doi: 10.1016/j.jplph.2010.09.019
Yamaguchi, N., Ohkura, T., Takahashi, Y., Yuji Maejima, Y., and Arao, T. (2014). Arsenic distribution and speciation near rice roots influenced by iron plaques and redox conditions of the soil matrix. Environ. Sci. Technol. 48, 1549–1556. doi: 10.1021/es402739a
Yamaji, N., Sakurai, G., Mitani-Ueno, N., and Ma, J. F. (2015). Orchestration of three transporters and distinct vascular structures in node for intervascular transfer of silicon in rice. Proc. Natl. Acad. Sci. U.S.A. 112, 11401–11406. doi: 10.1073/pnas.1508987112
Yang, H. C., Fu, H. L., Lin, Y. F., and Rosen, B. P. (2012). Pathways of arsenic uptake and efflux. Curr. Top. Membranes 69, 325–358. doi: 10.1016/B978-0-12-394390-3.00012-4
Yang, M., Lu, K., Zhao, F. J., Xie, W., Ramakrishna, P., Wang, G., et al. (2018). Genome-wide association studies reveal the genetic basis of ionomic variation in rice. Plant Cell 30, 2720–2740. doi: 10.1105/tpc.18.00375
Zhang, J., Zhu, Y. G., Zeng, D. L., Cheng, W. D., Qian, Q., and Duan, G. L. (2008). Mapping quantitative trait loci associated with arsenic accumulation in rice (Oryza sativa). New Phytol. 17RFPR7, 350–356. doi: 10.1111/j.1469-8137.2007.02267.x
Zhao, W., Cho, G. T., Ma, K. H., Chung, J. W., Gwag, J. G., and Park, Y. J. (2010). Development of an allele-mining set in rice using a heuristic algorithm and SSR genotype data with least redundancy for the post-genomic era. Mol. Breed. 26, 639–651. doi: 10.1007/s11032-010-9400-x
Keywords: arsenic, ionomics, eQTLs, co-expression network, transcriptome-wide association studies
Citation: Lee S-B, Kim G-J, Shin J-D, Chung W, Park S-K, Choi G-H, Park S-W and Park Y-J (2022) Genome-Scale Profiling and High-Throughput Analyses Unravel the Genetic Basis of Arsenic Content Variation in Rice. Front. Plant Sci. 13:905842. doi: 10.3389/fpls.2022.905842
Received: 28 March 2022; Accepted: 15 June 2022;
Published: 18 July 2022.
Edited by:
Mukesh Jain, Jawaharlal Nehru University, IndiaReviewed by:
Xin-Yuan Huang, Nanjing Agricultural University, ChinaXuehai Zhang, Henan Agricultural University, China
Copyright © 2022 Lee, Kim, Shin, Chung, Park, Choi, Park and Park. This is an open-access article distributed under the terms of the Creative Commons Attribution License (CC BY). The use, distribution or reproduction in other forums is permitted, provided the original author(s) and the copyright owner(s) are credited and that the original publication in this journal is cited, in accordance with accepted academic practice. No use, distribution or reproduction is permitted which does not comply with these terms.
*Correspondence: Yong-Jin Park, yjpark@kongju.ac.kr; Sang-Won Park, swpark@korea.kr