- 1State Key Laboratory of Subtropical Silviculture, College of Forestry and Biotechnology, Zhejiang A&F University, Hangzhou, China
- 2Shenzhen Branch, Guangdong Laboratory of Lingnan Modern Agriculture, Genome Analysis Laboratory of the Ministry of Agriculture and Rural Affairs, Agricultural Genomics Institute at Shenzhen, Chinese Academy of Agricultural Sciences, Shenzhen, China
- 3State Key Laboratory of Tree Genetics and Breeding, Research Institute of Tropical Forestry, Chinese Academy of Forestry, Guangzhou, China
- 4Jiangsu Key Laboratory for Bioresources of Saline Soils, Jiangsu Synthetic Innovation Center for Coastal Bio-agriculture, School of Wetlands, Yancheng Teachers University, Yancheng, China
Introduction
Non-coding RNA (ncRNA) is a key regulatory RNA with limited abilities of coding potential (Liu et al., 2015). To date, a variety of ncRNAs have been identified in plants, which can be classified into three groups according to their sequence length: small RNAs <50 nucleotides (nt), such as microRNAs (miRNAs); long non-coding RNAs (lncRNAs) with arbitrarily longer than 200 nt; and intermediate-size ncRNA between small RNAs and lncRNA in length (Wang et al., 2014). In addition, the process of back splicing also produces a class of covalently closed RNA molecules called exonic circular RNAs (circRNAs).
Different types of ncRNAs show diverse mechanisms of action. miRNAs typically degrade their target genes by binding to their transcripts at the post-transcriptional level (Chipman and Pasquinelli, 2019). However, lncRNAs function at transcriptional, post-transcriptional and epigenetic levels by interacting with macromolecules (Wu et al., 2020). Whiles circRNAs can act as miRNA/protein sponges, or regulate alternative splicing or transcription (Lai et al., 2018). These ncRNAs with different mechanisms of action form a complex regulatory network to jointly regulate plant growth, development and stress response. For instance, Vvi-miPEP171d1 regulates adventitious root formation in grapevine (Chen et al., 2020). And miRNA frameworks have been proved to be important for the flower induction in apple (Fan et al., 2018). In addition to regulation of self-life activities, ncRNAs also play a role in plant-to-plant communication. Exogenous miR399 and miR156 can trigger RNA interference to repress the expression of PHOSPHATE OVERACCUMULATOR 2 (PHO2) and SQUAMOSA-PROMOTER BINDING PROTEIN-LIKE 9 (SPL9) in plants (Betti et al., 2021). Moreover, ncRNAs can even act as a bridge between plants and other species. For example, two plant viruses, barley yellow dwarf virus and red clover necrotic mosaic virus, can express small subgenomic (sg) RNAs to attenuate host translation by binding translation initiation factor eIF4G (Miller et al., 2016). Expressing double-strand (ds) RNA in maize can effectively reduce feeding damage of western corn rootworm by triggering its RNA interference (Baum et al., 2007). In summary, ncRNAs play important roles in plant activities, and their regulatory functions are the basis for plant growth, development and survival.
The regulatory roles of ncRNAs make them important tools for adjusting gene expression and studying gene functions. For example, the artificial miRNA pAmiRNA156h-PDSh can effectively decline the expression of phytoene desaturase (MdPDS) in apple (Charrier et al., 2019). Overexpression of amiRNA-319a-HaEcR in tomato can effectively silence the ECDYSONE RECEPTOR (HaEcR) gene to reduce the survival of Helicoverpa armigera (Yogindran and Rajam, 2021). From this point of view, exploring new ncRNAs and elucidating the regulatory mechanism of ncRNAs will have profound impacts on plant research. Here, our emphasis is put on recognition technologies of ncRNAs in plants, including bioinformatic tools and experimental technologies.
Bioinformatics Tools
With the release of more and more genomic information of diverse plants, it provides a key basis for the discovery of novel ncRNAs. In addition, knowledge about the function, structure and conservation of ncRNAs is accumulating, which can help us distinguish different types of ncRNAs. Since ncRNAs were reported, at least 39 bioinformatics websites and softwares have been established, of which 40.5% (13 websites and 4 softwares) were released in the past 5 years (Table 1). Furthermore, 41 deep learning models were developed in the past 3 years (Table 1). These tools can be divided into three classes.
ncRNA Databases
The ncRNA databases collected ncRNA-related information, such as sequences, interactions between ncRNAs and target genes, as well as expression profiles. In addition, some tools contain information that has been supported by experimental evidences. For example, miRbase (Kozomara et al., 2019) integrates published mature sequences of miRNAs and their relevant hairpin precursors of miRNA. miRTarBase (Huang et al., 2020) and NPInter (Teng et al., 2020) not only focus on the interaction information between ncRNAs and other biomolecules, but also provide the relevant experimental evidences. To date, miRNAs, lncRNAs, and circular RNAs (circRNAs) in ncRNAs were collected by different online databases (Chu et al., 2017; Jin et al., 2021). Details of these tools including the application system, input format, and included species are listed in Supplementary Table S1. The development of high-throughput sequencing technologies and the increasing amount of published genomic data have given us the opportunity to collect diverse information from different species, which facilities the analysis of ncRNA evolutional and the construction of conserved models for predicting and exploring novel ncRNAs. However, the application of these databases in cross-species research still faces enormous challenges. On the one hand, there is a lack of identifying species-conserved or species-specific ncRNAs; and on the other hand, it is difficult to determine functional ncRNAs in a predictive manner.
ncRNA Prediction Tools
Prediction of ncRNA, including recognition of ncRNA and prediction of ncRNA function. At present, there are many prediction tools for ncRNA recognition, but relatively few tools for their function prediction. We classify the existing prediction tools according to their categories (Table 1, Supplementary Table S1). How to accurately predict novel ncRNAs and their target genes has always been the focus of researchers. The current development of prediction tools mainly focuses on three aspects: predicting the sequences of miRNAs and their precursors (Wu et al., 2012; Lei and Sun, 2014; Fei et al., 2021), predicting the binding sites of ncRNAs to targets (Bonnet et al., 2010; Brousse et al., 2014), predicting or visualizing the secondary or three-dimensional structure of ncRNAs (Steffen et al., 2006; Byun and Han, 2009; Biesiada et al., 2016). Sequence alignment is the basis for this prediction, and the divergence of ncRNA sequences is an important factor affecting the accuracy of prediction. In general, ancient ncRNAs (especially those related to plant development) remain highly conserved across species (Willmann and Poethig, 2007). However, recently evolved ncRNAs appear to be highly species-specific (Cuperus et al., 2011). Furthermore, there appears to be variability among different classes of ncRNAs in conservation among different species (Wu et al., 2020). Therefore, how to improve the accuracy of ncRNA prediction is an important problem to be solved. With the accumulation of ncRNA data, building conserved models for each ncRNA family may be a solution for this question.
Application of Deep Learning in ncRNA Study
Deep learning developed in recent years has shown a powerful potential capability in addressing bioinformatics problems in ncRNA study. For example, RPITER model can be used to predict interactions between ncRNAs and proteins based on sequence and structure information (Peng et al., 2019). Compared with traditional approaches, more information can be introduced into the computational process of deep learning to ensure the accuracy of the predicted results (Zhang S. W. et al., 2020). So far, at least 41 deep learning models have been built to predict ncRNA classification (Amin et al., 2019), ncRNA-protein interaction (Peng et al., 2019), ncRNA interaction, as well as ncRNA identification and functions (Khan et al., 2020; Zhang P. et al., 2020) (Table 1, Supplementary Table S1).
The basic processes of applying deep learning are showed in Figure 1A. After choosing an appropriate framework, researchers need to input data to generate relevant models. The resulting model can be used for the next prediction step. Compared with other prediction methods, the advantage of deep learning can effectively reduce the prediction bias caused by imperfect design parameters. Its limitation is that the accuracy of predictions heavily depends on the accuracy of the models, which usually require large enough data to build and train. Therefore, the dataset used to build deep learning models seriously affect the accuracy of prediction and analysis. How to obtain a large amount of ncRNA data from different species for building more accurate models is a serious problem that needs to be solved. In addition, most of them are distributed in Linux system, and the proficient computational skills of users are an important precondition to utilize these models. Developing softwares with a user-friendly interface (Xu et al., 2021) is crucial for the application of these models. However, the cross-operating system adaptability of these models and the differences in fit to different data types are the main obstacles facing the use of new models to build user-friendly softwares.
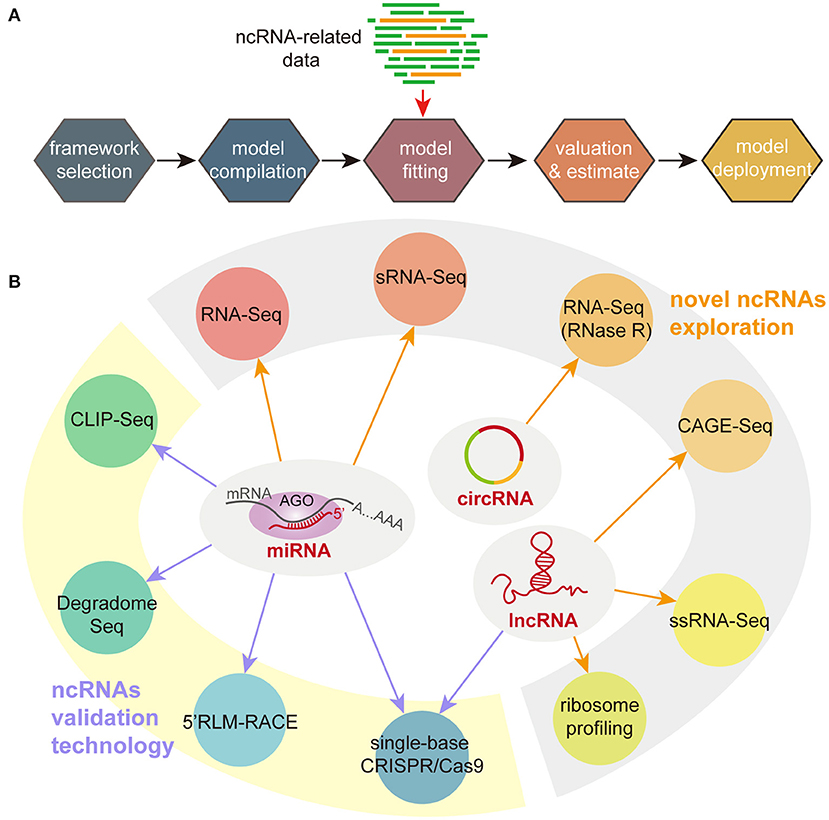
Figure 1. Deep learning processes (A) and experimental techniques (B) for studying ncRNAs. sRNA-Seq, small RNA sequencing; CLIP-Seq, crosslinking and immunoprecipitation with sequencing; 5′RLM-RACE, 5′ RNA ligase mediated amplification of cDNA ends; ssRNA-Seq, strand-specific RNA sequencing; CAGE-Seq, cap analysis gene expression sequencing.
Experimental Technologies
Although the results of bioinformatics predictions are becoming more and more accurate with the accumulation of ncRNA knowledge, experimental technologies are still needed to further validate the prediction results. According to the characteristics of ncRNAs, at least three aspects need to be verified: firstly, a functional ncRNA should have transcriptional activity (Bazzini et al., 2009); secondly, there should be an expression correlation between ncRNA and target genes (Bai et al., 2018); thirdly, if the ncRNA functions by degrading its target genes, the cleavage site of the ncRNA on the target genes should be verified (Gao et al., 2018). Moreover, experimental strategies should be varied for different types of ncRNAs. Figure 1B summarized the main sequencing and experimental techniques in ncRNA studies. In detail, the combination of sRNA-Seq and RNA-Seq is commonly used for global identification of novel small ncRNAs (Huang et al., 2019). After removed low-quality reads, high-quality reads are subsequently annotated by several databases (such as miRbase and Rfam) (Deforges et al., 2019; Huang et al., 2019). The length of small RNAs is far shorter than protein-coding genes and lncRNAs. Therefore, the extraction of small RNAs unlike other RNA, can be performed using special RNA-extraction kits (Gao et al., 2019) or TRIzol reagent (Tan et al., 2018). For miRNAs, it is necessary to identify the binding sites between miRNAs and their target genes (Shen W. et al., 2021). Degradome sequencing and crosslinking and immunoprecipitation with sequencing (CLIP-Seq) can be used to analyze binding sites between miRNAs and the target genes (Han et al., 2016; Chipman and Pasquinelli, 2019). Furthermore, 5′-RNA ligase mediated amplification of cDNA ends (5′RLM-RACE) assays are directly used for verifying the predicted binding sites (Cui et al., 2020). The detection of novel lncRNAs can be completed by multiple RNA-Seq strategies, such as isoform-sequencing, strand-specific RNA-Seq (ssRNA-Seq), cap analysis gene expression with polyA-Seq technologies (CAGE-Seq) (Zheng et al., 2021). Although lncRNAs are considered as a part of ncRNAs, some of them still remain a weak ability of translating small peptides. Therefore, ribosome profiling become has become one of the strategies to detect lncRNAs (Wu et al., 2019). Meanwhile, the recently developed high-precision single-base CRISPR/Cas9 technology can effectively create ncRNA-related mutants to explore the relationships between ncRNAs and target genes (Jacobs et al., 2015). Currently, in addition to the research on the function of small RNAs, more and more attention is focused on circRNAs in recent years. However, most studies focus more on the discovery of novel circRNAs by sequencing technologies. One of challenges of circRNA sequencing is to improve the accuracy of detection and quantification of circRNAs due to their lack of poly (A) tails and insufficient expression levels (Wang et al., 2019; Zhao et al., 2019). Treatment of total RNA with ribonuclease R or increasing sequencing depth may resolve these issues (Chen et al., 2017). Meanwhile, it is also necessary to develop functional research techniques to further clarify the biological functions of circRNAs.
Conclusion
In conclusion, although many tools and technologies have been developed to study ncRNAs in plants, there are still opportunities and challenges in this field. In bioinformatics, since there are significant differences in ncRNAs between species, it is beneficial for our research on ncRNAs to collect as much data as possible based on different species. Meanwhile, ncRNAs in a same family exhibit high conservation, it is possible for us to build models to discover novel ncRNAs. Moreover, most prediction tools and deep-learning models are developed based on Linux system, and the development of user-friendly Windows versions will help more researchers to analyze different kinds of ncRNA. As ncRNAs play a regulatory role in plants, how to manipulate ncRNAs through genetic engineering to regulate specific biological processes remains to be resolved.
Author Contributions
JZ conceived the study. DX collected and synthesized the data and draft the manuscript. JZ, WY, CF, BL, and M-ZL revised the manuscript. All authors contributed to the article and approved the final version.
Funding
This work was supported by the Key Scientific and Technological Grant of Zhejiang for Breeding New Agricultural Varieties (2021C02070-1), the National Key Research and Development Program of China (2021YFD2200205 and 2021YFD2200700), the National Science Foundation of China (32171814), the Natural Science Foundation of Zhejiang Province for Distinguished Young Scholars (LR22C160001), and the Zhejiang A&F University Research and Development Fund Talent Startup Project (2021LFR013).
Conflict of Interest
The authors declare that the research was conducted in the absence of any commercial or financial relationships that could be construed as a potential conflict of interest.
Publisher's Note
All claims expressed in this article are solely those of the authors and do not necessarily represent those of their affiliated organizations, or those of the publisher, the editors and the reviewers. Any product that may be evaluated in this article, or claim that may be made by its manufacturer, is not guaranteed or endorsed by the publisher.
Supplementary Material
The Supplementary Material for this article can be found online at: https://www.frontiersin.org/articles/10.3389/fpls.2022.890663/full#supplementary-material
References
Amin, N., McGrath, A., and Chen, Y.-P. P. (2019). Evaluation of deep learning in non-coding RNA classification. Nat. Machine Intellig. 1, 246–256. doi: 10.1038/s42256-019-0051-2
Bai, Q., Wang, X., Chen, X., Shi, G., Liu, Z., Guo, C., et al. (2018). Wheat miRNA TaemiR408 acts as an essential mediator in plant tolerance to pi deprivation and salt stress via modulating stress-associated physiological processes. Front. Plant Sci. 9, 499. doi: 10.3389/fpls.2018.00499
Baum, J. A., Bogaert, T., Clinton, W., Heck, G. R., Feldmann, P., Ilagan, O., et al. (2007). Control of coleopteran insect pests through RNA interference. Nat. Biotechnol. 25, 1322–1326. doi: 10.1038/nbt1359
Bazzini, A. A., Almasia, N. I., Manacorda, C. A., Mongelli, V. C., Conti, G., Maroniche, G. A., et al. (2009). Virus infection elevates transcriptional activity of miR164a promoter in plants. BMC Plant Biol. 9, 152. doi: 10.1186/1471-2229-9-152
Betti, F., Ladera-Carmona, M. J., Weits, D. A., Ferri, G., Iacopino, S., Novi, G., et al. (2021). Exogenous miRNAs induce post-transcriptional gene silencing in plants. Nature Plants 7, 1379–1388. doi: 10.1038/s41477-021-01005-w
Biesiada, M., Purzycka, K. J., Szachniuk, M., Blazewicz, J., and Adamiak, R. W. (2016). “Automated RNA 3D structure prediction with RNAComposer,” in RNA Structure Determination (New York, NY: Humana Press), 199–215. doi: 10.1007/978-1-4939-6433-8_13
Bonnet, E., He, Y., Billiau, K., and Van de Peer, Y. (2010). TAPIR, a web server for the prediction of plant microRNA targets, including target mimics. Bioinformatics 26, 1566–1568. doi: 10.1093/bioinformatics/btq233
Brousse, C., Liu, Q., Beauclair, L., Deremetz, A., Axtell, M. J., and Bouché, N. (2014). A non-canonical plant microRNA target site. Nucleic Acids Res. 42, 5270–5279. doi: 10.1093/nar/gku157
Byun, Y., and Han, K. (2009). PseudoViewer3: generating planar drawings of large-scale RNA structures with pseudoknots. Bioinformatics 25, 1435–1437. doi: 10.1093/bioinformatics/btp252
Chaabane, M., Williams, R. M., Stephens, A. T., and Park, J. W. (2020). circDeep: deep learning approach for circular RNA classification from other long non-coding RNA. Bioinformatics 36, 73–80. doi: 10.1093/bioinformatics/btz537
Chantsalnyam, T., Lim, D. Y., Tayara, H., and Chong, K. T. (2020). ncRDeep: non-coding RNA classification with convolutional neural network. Comput. Biol. Chem. 88, 107364. doi: 10.1016/j.compbiolchem.2020.107364
Chantsalnyam, T., Siraj, A., Tayara, H., and Chong, K. T. (2021). ncRDense: a novel computational approach for classification of non-coding RNA family by deep learning. Genomics 113, 3030–3038. doi: 10.1016/j.ygeno.2021.07.004
Charrier, A., Vergne, E., Joffrion, C., Richer, A., Dousset, N., and Chevreau, E. (2019). An artificial miRNA as a new tool to silence and explore gene functions in apple. Transgenic Res. 28, 611–626. doi: 10.1007/s11248-019-00170-1
Chen, G., Cui, J., Wang, L., Zhu, Y., Lu, Z., and Jin, B. (2017). Genome-wide identification of circular RNAs in Arabidopsis thaliana. Front. Plant Sci. 8, 1678. doi: 10.3389/fpls.2017.01678
Chen, Q. J., Deng, B. H., Gao, J., Zhao, Z. Y., Chen, Z. L., Song, S. R., et al. (2020). A miRNA-encoded small peptide, vvi-miPEP171d1, regulates adventitious root formation. Plant Physiol. 183, 656–670. doi: 10.1104/pp.20.00197
Cheng, S., Zhang, L., Tan, J., Gong, W., Li, C., and Zhang, X. (2019). DM-RPIs: predicting ncRNA-protein interactions using stacked ensembling strategy. Comput. Biol. Chem. 83, 107088. doi: 10.1016/j.compbiolchem.2019.107088
Chipman, L. B., and Pasquinelli, A. E. (2019). miRNA targeting: growing beyond the seed. Trends Genet. 35, 215–222. doi: 10.1016/j.tig.2018.12.005
Chu, Q., Zhang, X., Zhu, X., Liu, C., Mao, L., Ye, C., et al. (2017). PlantcircBase: a database for plant circular RNAs. Mol. Plant 10, 1126–1128. doi: 10.1016/j.molp.2017.03.003
Cui, C., Wang, J. J., Zhao, J. H., Fang, Y. Y., He, X. F., Guo, H. S., et al. (2020). A brassica miRNA regulates plant growth and immunity through distinct modes of action. Mol. Plant 13, 231–245. doi: 10.1016/j.molp.2019.11.010
Cuperus, J. T., Fahlgren, N., and Carrington, J. C. (2011). Evolution and functional diversification of MIRNA genes. Plant Cell 23, 431–442. doi: 10.1105/tpc.110.082784
Dai, Q., Guo, M., Duan, X., Teng, Z., and Fu, Y. (2019). Construction of complex features for computational predicting ncRNA-protein interaction. Front. Genet. 10, 18. doi: 10.3389/fgene.2019.00018
Dai, Q., Wang, Z., Song, J., Duan, X., Guo, M., and Tian, Z. (2020). “A stacked ensemble learning framework with heterogeneous feature combinations for predicting ncRNA-protein interaction,” in 2020 IEEE International Conference on Bioinformatics and Biomedicine (Seoul).
Deforges, J., Reis, R. S., Jacquet, P., Sheppard, S., Gadekar, V. P., Hart-Smith, G., et al. (2019). Control of cognate sense mRNA translation by cis-natural antisense RNAs. Plant Physiol. 180, 305–322. doi: 10.1104/pp.19.00043
Fan, S., Zhang, D., Gao, C., Wan, S., Lei, C., Wang, J., et al. (2018). Mediation of flower induction by gibberellin and its inhibitor paclobutrazol: mRNA and miRNA integration comprises complex regulatory cross-talk in apple. Plant Cell Physiol. 59, 2288–2307. doi: 10.1093/pcp/pcy154
Fan, X. N., and Zhang, S. W. (2019). LPI-BLS: Predicting lncRNA?protein interactions with a broad learning system-based stacked ensemble classifier. Neurocomputing 370, 88–93. doi: 10.1016/j.neucom.2019.08.084
Fasold, M., Langenberger, D., Binder, H., Stadler, P. F., and Hoffmann, S. (2011). DARIO: a ncRNA detection and analysis tool for next-generation sequencing experiments. Nucleic Acids Res. 39, W112–W117. doi: 10.1093/nar/gkr357
Fei, Y., Feng, J., Wang, R., Zhang, B., Zhang, H., and Huang, J. (2021). PhasiRNAnalyzer: an integrated analyser for plant phased siRNAs. RNA Biology 18, 1622–1629. doi: 10.1080/15476286.2021.1879543
Fiannaca, A., La Rosa, M., La Paglia, L., Rizzo, R., and Urso, A. (2017). nRC: non-coding RNA Classifier based on structural features. BioData Min. 10, 27. doi: 10.1186/s13040-017-0148-2
Gao, J., Chen, H., Yang, H., He, Y., Tian, Z., and Li, J. (2018). A brassinosteroid responsive miRNA-target module regulates gibberellin biosynthesis and plant development. New Phytol. 220, 488–501. doi: 10.1111/nph.15331
Gao, Z., Li, J., Luo, M., Li, H., Chen, Q., Wang, L., et al. (2019). Characterization and cloning of grape circular RNAs identified the cold resistance-related Vv-circATS1. Plant Physiol. 180, 966–985. doi: 10.1104/pp.18.01331
Han, X., Yin, H., Song, X., Zhang, Y., Liu, M., Sang, J., et al. (2016). Integration of small RNAs, degradome and transcriptome sequencing in hyperaccumulator Sedum alfredii uncovers a complex regulatory network and provides insights into cadmium phytoremediation. Plant Biotechnol. J. 14, 1470–1483. doi: 10.1111/pbi.12512
Hoogstrate, Y., Jenster, G., and Martens-Uzunova, E. S. (2015). FlaiMapper: computational annotation of small ncRNA-derived fragments using RNA-seq high-throughput data. Bioinformatics 31, 665–673. doi: 10.1093/bioinformatics/btu696
Huang, H. Y., Lin, Y. C. D., Li, J., Huang, K. Y., Shrestha, S., et al. (2020). miRTarBase 2020: updates to the experimentally validated microRNA-target interaction database. Nucleic Acids Res. 48, D148–D154, doi: 10.1093/nar/gkz896
Huang, J., Wang, C., Li, X., Fang, X., Huang, N., Wang, Y., et al. (2019). Conservation and divergence in the meiocyte sRNAomes of Arabidopsis, soybean, and cucumber. Plant Physiol. 182, 301–317. doi: 10.1104/pp.19.00807
Huang, L., Jiao, S., Yang, S., Zhang, S., Zhu, X., Guo, R., et al. (2021). LGFC-CNN: prediction of lncRNA-protein interactions by using multiple types of features through deep learning. Genes 12, 1689. doi: 10.3390/genes12111689
Jacobs, T. B., LaFayette, P. R., Schmitz, R. J., and Parrott, W. A. (2015). Targeted genome modifications in soybean with CRISPR/Cas9. BMC Biotechnology 15, 16. doi: 10.1186/s12896-015-0131-2
Jin, J., Lu, P., Xu, Y., Li, Z., Yu, S., Liu, J., et al. (2021). PLncDB V2. 0: a comprehensive encyclopedia of plant long noncoding RNAs. Nucleic Acids Res. 49, D1489–D1495. doi: 10.1093/nar/gkaa910
Kang, Q., Meng, J., Cui, J., Luan, Y., and Chen, M. (2020). PmliPred: a method based on hybrid model and fuzzy decision for plant miRNA–lncRNA interaction prediction. Bioinformatics 36, 2986–2992. doi: 10.1093/bioinformatics/btaa074
Kang, Q., Meng, J., Shi, W., and Luan, Y. (2021). Ensemble deep learning based on multi-level information enhancement and greedy fuzzy decision for plant miRNA–lncRNA interaction prediction. Interdiscip. Sci. Comput. Life Sci. 13, 603–614. doi: 10.1007/s12539-021-00434-7
Khan, S., Khan, M., Iqbal, N., Hussain, T., Khan, S. A., and Chou, K. C. (2020). A two-level computation model based on deep learning algorithm for identification of piRNA and their functions via Chou's 5-steps rule. Int. J. Peptide Res.and Therap. 26, 795–809. doi: 10.1007/s10989-019-09887-3
Kozomara, A., Birgaoanu, M., and Griffiths-Jones, S. (2019). miRBase: from microRNA sequences to function. Nucleic Acids Res. 47, D155–D162. doi: 10.1093/nar/gky1141
Lai, X. L., Jérémie, B., Stuart, W., Martin, C., Chloe, Z., and Simon, J. C. (2018). CircRNAs in plants. Circular RNAs. Adv. Exp. Med. Biol. (Springer), 1087, 329–343. doi: 10.1007/978-981-13-1426-1_26
Lei, J., and Sun, Y. (2014). miR-PREFeR: an accurate, fast and easy-to-use plant miRNA prediction tool using small RNA-Seq data. Bioinformatics 30, 2837–2839. doi: 10.1093/bioinformatics/btu380
Li, Y., Sun, H., Feng, S., Zhang, Q., Han, S., and Du, W. (2021). Capsule-LPI: a lncRNA–protein interaction predicting tool based on a capsule network. BMC Bioinformatics 22, 246. doi: 10.1186/s12859-021-04171-y
Liu, S., Zhao, X., Zhang, G., Li, W., Liu, F., Liu, S., et al. (2019). PredLnc-GFStack: a global sequence feature based on a stacked ensemble learning method for predicting lncRNAs from transcripts. Genes 10, 672. doi: 10.3390/genes10090672
Liu, X., Hao, L., Li, D., Zhu, L., and Hu, S. (2015). Long non-coding RNAs and their biological roles in plants. Genom. Proteom. Bioinform. 13, 137–147. doi: 10.1016/j.gpb.2015.02.003
Miller, W. A., Shen, R., Staplin, W., and Kanodia, P. (2016). Noncoding RNAs of plant viruses and viroids: sponges of host translation and RNA interference machinery. Mol. Plant Microbe Interact. 29, 156–164. doi: 10.1094/MPMI-10-15-0226-FI
Navamajiti, N., Saethang, T., and Wichadakul, D. (2019). “McBel-Plnc: a deep learning model for multiclass multilabel classification of protein-lncRNA interactions,” in Proceedings of the 2019 6th International Conference on Biomedical and Bioinformatics Engineering. Association for Computing Machinery, Shanghai, China, 21–28.
Pan, X., Fan, Y. X., Yan, J., and Shen, H. B. (2016). IPMiner: hidden ncRNA-protein interaction sequential pattern mining with stacked autoencoder for accurate computational prediction. BMC Genomics 17, 582. doi: 10.1186/s12864-016-2931-8
Peng, C., Han, S., Zhang, H., and Li, Y. (2019). RPITER: a hierarchical deep learning framework for ncRNA–protein interaction prediction. Int. J. Mol. Sci. 20, 1070. doi: 10.3390/ijms20051070
Ramakrishnaiah, Y., Kuhlmann, L., and Tyagi, S. (2021). linc2function: a deep learning model to identify and assign function to long noncoding RNA. bioRxiv [Preprint]. doi: 10.1101/2021.01.29.428785
Shaw, D., Chen, H., Xie, M., and Jiang, T. (2021). DeepLPI: a multimodal deep learning method for predicting the interactions between lncRNAs and protein isoforms. BMC Bioinform. 22, 24. doi: 10.1186/s12859-020-03914-7
Shen, W., Cao, S., Liu, J., Zhang, W., Chen, J., and Li, J. F. (2021). Overexpression of an Osa-miR162a derivative in rice confers cross-kingdom RNA interference-mediated brown planthopper resistance without perturbing host development. Int. J. Mol. Sci. 22, 12652. doi: 10.3390/ijms222312652
Shen, Z. A., Luo, T., Zhou, Y. K., Yu, H., and Du, P. F. (2021). NPI-GNN: predicting ncRNA–protein interactions with deep graph neural networks. Brief. Bioinform. 22, bbab051. doi: 10.1093/bib/bbab051
Song, J., Tian, S., Yu, L., Yang, Q., Xing, Y., Zhang, C., et al. (2020). MD-MLI: prediction of miRNA-lncRNA interaction by using multiple features and hierarchical deep learning. IEEE/ACM Trans. Comput. Biol. Bioinform. doi: 10.1109/TCBB.2020.3034922. [Epub ahead of print].
Steffen, P., Voß, B., Rehmsmeier, M., Reeder, J., and Giegerich, R. (2006). RNAshapes: an integrated RNA analysis package based on abstract shapes. Bioinformatics 22, 500–503. doi: 10.1093/bioinformatics/btk010
Tan, F., Lu, Y., Jiang, W., Wu, T., Zhang, R., Zhao, Y., et al. (2018). DDM1 represses noncoding RNA expression and RNA-directed DNA methylation in heterochromatin. Plant Physiol. 177, 1187–1197. doi: 10.1104/pp.18.00352
Tang, Q., Nie, F., Kang, J., and Chen, W. (2020). ncPro-ML: an integrated computational tool for identifying non-coding RNA promoters in multiple species. Comput. Struct. Biotechnol. J. 18, 2445–2452. doi: 10.1016/j.csbj.2020.09.001
Teng, X., Chen, X., Xue, H., Tang, Y., Zhang, P., Kang, Q., et al. (2020). NPInter v4.0: an integrated database of ncRNA interactions. Nucleic Acids Res. 48, D160–D165. doi: 10.1093/nar/gkz969
Wang, J., Zhao, Y., Gong, W., Liu, Y., Wang, M., Huang, X., et al. (2021a). EDLMFC: an ensemble deep learning framework with multi-scale features combination for ncRNA–protein interaction prediction. BMC Bioinformatics 22, 133. doi: 10.1186/s12859-021-04069-9
Wang, L., Zhong, X., Wang, S., and Liu, Y. (2021b). ncDLRES: a novel method for non-coding RNAs family prediction based on dynamic LSTM and ResNet. BMC Bioinformatics 22, 447. doi: 10.1186/s12859-021-04365-4
Wang, W., Guan, X., Khan, M. T., Xiong, Y., and Wei, D. Q. (2020). LMI-DForest: a deep forest model towards the prediction of lncRNA-miRNA interactions. Comput. Biol. Chem. 89, 107406. doi: 10.1016/j.compbiolchem.2020.107406
Wang, Y., Wang, X., Deng, W., Fan, X., Liu, T. T., He, G., et al. (2014). Genomic features and regulatory roles of intermediate-sized non-coding RNAs in Arabidopsis. Mol. Plant 7, 514–527. doi: 10.1093/mp/sst177
Wang, Y., Xiong, Z., Li, Q., Sun, Y. Y., Jin, J., Chen, H., et al. (2019). Circular RNA profiling of the rice photo-thermosensitive genic male sterile line Wuxiang S reveals circRNA involved in the fertility transition. BMC Plant Biol. 19, 340. doi: 10.1186/s12870-019-1944-2
Wekesa, J. S., Meng, J., and Luan, Y. (2020b). A deep learning model for plant lncRNA-protein interaction prediction with graph attention. Mol. Genet. Genom. 295, 1091–1102. doi: 10.1007/s00438-020-01682-w
Wekesa, J. S., Meng, J., and Luan, Y. (2020c). Multi-feature fusion for deep learning to predict plant lncRNA-protein interaction. Genomics 112, 2928–2936. doi: 10.1016/j.ygeno.2020.05.005
Wekesa, J. S., Luan, Y., and Meng, J. (2020a). “LPI-DL: a recurrent deep learning model for plant lncRNA-protein interaction and function prediction with feature optimization,” in 2020 IEEE International Conference on Bioinformatics and Biomedicine (Seoul).
Willmann, M. R., and Poethig, R. S. (2007). Conservation and evolution of miRNA regulatory programs in plant development. Curr. Opin. Plant Biol. 10, 503–511. doi: 10.1016/j.pbi.2007.07.004
Wu, H. J., Ma, Y. K., Chen, T., Wang, M., and Wang, X. J. (2012). PsRobot: a web-based plant small RNA meta-analysis toolbox. Nucleic Acids Res. 40, W22–W28. doi: 10.1093/nar/gks554
Wu, H. Y. L., Song, G., Walley, J. W., and Hsu, P. Y. (2019). The tomato translational landscape revealed by transcriptome assembly and ribosome profiling. Plant Physiol. 181, 367–380. doi: 10.1104/pp.19.00541
Wu, L., Liu, S., Qi, H., Cai, H., and Xu, M. (2020). Research progress on plant long non-coding RNA. Plants 9, 408. doi: 10.3390/plants9040408
Xu, D., Lu, Z., Jin, K., Qiu, W., Qiao, G., Han, X., et al. (2021). SPDE: a multi-functional software for sequence processing and data extraction. Bioinformatics 37, 3686–3687. doi: 10.1093/bioinformatics/btab235
Yang, C., Yang, L., Zhou, M., Xie, H., Zhang, C., Wang, M. D., et al. (2018). LncADeep: an ab initio lncRNA identification and functional annotation tool based on deep learning. Bioinformatics 34, 3825–3834. doi: 10.1093/bioinformatics/bty428
Yang, S., Wang, Y., Lin, Y., Shao, D., He, K., and Huang, L. (2020). LncMirNet: predicting lncRNA–miRNA interaction based on deep learning of ribonucleic acid sequences. Molecules 25, 4372. doi: 10.3390/molecules25194372
Yi, H. C., You, Z. H., Wang, M. N., Guo, Z. H., Wang, Y. B., and Zhou, J. R. (2020). RPI-SE: a stacking ensemble learning framework for ncRNA-protein interactions prediction using sequence information. BMC Bioinformatics 21, 60. doi: 10.1186/s12859-020-3406-0
Yogindran, S., and Rajam, M. V. (2021). Host-derived artificial miRNA-mediated silencing of ecdysone receptor gene provides enhanced resistance to Helicoverpa armigera in tomato. Genomics 113, 736–747. doi: 10.1016/j.ygeno.2020.10.004
Yu, H., Shen, Z. A., and Du, P. F. (2021). NPI-RGCNAE: fast predicting ncRNA-protein interactions using the relational graph convolutional network auto-encoder. IEEE J. Biomed. Health Inform. doi: 10.1109/JBHI.2021.3122527. [Epub ahead of print].
Zhan, Z. H., Jia, L. N., Zhou, Y., Li, L. P., and Yi, H. C. (2019). BGFE: a deep learning model for ncRNA-protein interaction predictions based on improved sequence Information. Int. J. Mol. Sci. 20, 978. doi: 10.3390/ijms200409783
Zhan, Z. H., You, Z. H., Li, L. P., Zhou, Y., and Yi, H. C. (2018). Accurate prediction of ncRNA-protein interactions from the integration of sequence and evolutionary information. Front. Genet. 9, e00458. doi: 10.3389/fgene.2018.00458
Zhang, P., Meng, J., Luan, Y., and Liu, C. (2020). Plant miRNA–lncRNA interaction prediction with the ensemble of CNN and IndRNN. Interdiscipl. Sci. Comput. Life Sci. 12, 82–89. doi: 10.1007/s12539-019-00351-w
Zhang, S. W., Zhang, X. X., Fan, X. N., and Li, W. N. (2020). LPI-CNNCP: prediction of lncRNA-protein interactions by using convolutional neural network with the copy-padding trick. Anal. Biochem. 601, 113767. doi: 10.1016/j.ab.2020.113767
Zhang, Y., Jia, C., and Kwoh, C. K. (2021). Predicting the interaction biomolecule types for lncRNA: an ensemble deep learning approach. Brief. Bioinform. 22, bbaa228. doi: 10.1093/bib/bbaa228
Zhao, W., Chu, S. S., and Jiao, Y. Q. (2019). Present scenario of circular RNAs (circRNAs) in plants. Front. Plant Sci. 10, 379. doi: 10.3389/fpls.2019.00379
Zheng, X., Chen, Y., Zhou, Y., Shi, K., Hu, X., Li, D., et al. (2021). Full-length annotation with multistrategy RNA-seq uncovers transcriptional regulation of lncRNAs in cotton. Plant Physiol. 185, 179–195. doi: 10.1093/plphys/kiaa003
Zhou, H., Wekesa, J. S., Luan, Y., and Meng, J. (2021). PRPI-SC: an ensemble deep learning model for predicting plant lncRNA-protein interactions. BMC Bioinformatics 22, 415. doi: 10.1186/s12859-021-04328-9
Keywords: non-coding RNA, bioinformatics tools, experimental technology, deep learning, miRNA
Citation: Xu D, Yuan W, Fan C, Liu B, Lu M-Z and Zhang J (2022) Opportunities and Challenges of Predictive Approaches for the Non-coding RNA in Plants. Front. Plant Sci. 13:890663. doi: 10.3389/fpls.2022.890663
Received: 06 March 2022; Accepted: 28 March 2022;
Published: 14 April 2022.
Edited by:
Enhui Shen, Zhejiang University, ChinaCopyright © 2022 Xu, Yuan, Fan, Liu, Lu and Zhang. This is an open-access article distributed under the terms of the Creative Commons Attribution License (CC BY). The use, distribution or reproduction in other forums is permitted, provided the original author(s) and the copyright owner(s) are credited and that the original publication in this journal is cited, in accordance with accepted academic practice. No use, distribution or reproduction is permitted which does not comply with these terms.
*Correspondence: Jin Zhang, zhangj@zafu.edu.cn; orcid.org/0000-0002-8397-5078