- 1Leibniz-Institute of Vegetable and Ornamental Crops (IGZ), Next-Generation Horticultural Systems, Grossbeeren, Germany
- 2Department of Functional Genome and Gene Safety, Chinese Academy of Agricultural Sciences, Beijing, China
Mechanistic models of canopy photosynthesis usually upscale leaf photosynthesis to crop level. A detailed prediction of canopy microclimate with accurate leaf morphological and physiological model parameters is the pre-requisite for accurate predictions. It is well established that certain leaf model parameters (Vcmax, Jmax) of the frequently adopted Farquhar and Caemmerer photosynthesis model change with leaf age and light interception history. Previous approaches to predict Vcmax and Jmax focused primarily on light interception, either by cumulative intercepted photosynthetic photon flux density (PPFD) or by closely related proxy variables such as leaf nitrogen content per leaf area. However, for plants with monopodial growth, such as vertically grown tomatoes or cucumber crops, in greenhouse production, there is a strong relationship between leaf age and light interception, complicating the experimental and mathematical separation of both effects. We propose a modeling framework that separates age and light intensity-related acclimation effects in a crop stand: Improved approximation of intra-leaf light absorption profiles with cumulative chlorophyll content (Chl) is the basis, while parameters are estimated via Gaussian process regression from total Chl, carotenoid content (Car), and leaf mass per area (LMA). The model approximates light absorption profiles within a leaf and links them to leaf capacity profiles of photosynthetic electron transport. Published datasets for Spinacia oleracea and Eucalyptus pauciflora were used to parameterize the relationship between light and capacity profiles and to set the curvature parameter of electron transport rate described by a non-rectangular hyperbola on Cucumis sativus. Using the modified capacity and light absorption profile functions, the new model was then able to predict light acclimation in a 2-month period of a fully grown tomato crop. An age-dependent lower limit of the electron transport capacity per unit Chl was essential in order to capture the decline of Vcmax and Jmax over time and space of the investigated tomato crop. We detected that current leaf photosynthetic capacity in tomato is highly affected by intercepted light-sum of 3–5 previous days.
Introduction
At the heart of most experimental and theoretical plant growth studies are measurements or predictions of primary CO2 assimilation at different spatial and temporal scales. Mathematical or biological integration of instantaneous CO2 assimilation rates over total leaf area and day/night cycle cumulates to daily biomass growth rates excluding certain losses. Therefore, there has been much work on modeling leaf photosynthesis (von Caemmerer et al., 2009), canopy microclimate (Russell et al., 1990; Körner et al., 2007; Myneni and Ross, 2012), and its proper integration (Bonan et al., 2021) over the last decades. In addition, mechanistic models of canopy photosynthesis require for upscaling from leaf photosynthesis rates an accurate description of microclimate and well-estimated leaf-model parameters at different canopy positions.
Certain parameters (e.g., Vcmax and Jmax) of the frequently used Farquhar–Caemmerer–Berry (FCB) leaf photosynthesis model (von Caemmerer et al., 2009) are not constant over time and change with leaf age and past light interception. Photosynthetic acclimation to shade is a well-investigated process both at leaf (Lichtenthaler and Babani, 2004) and intra-leaf levels (Nishio et al., 1993). Focus was often set on light acclimation using either the cumulative intercepted photosynthetic photon flux density (PPFD) or closely related proxy variables, such as the leaf nitrogen content per leaf area, as predictors for Vcmax and Jmax (Meir et al., 2002; Niinemets et al., 2004).
For plants with a monopodial growth habit, such as vertically grown tomatoes or cucumber crops, in greenhouse production (as common in commercial practice), there is a strong relationship between leaf age and light interception (Niinemets, 2016), complicating the experimental and mathematical separation of both effects. This may limit the generality of previously developed acclimation models, especially with the introduction of novel cultivation procedures, e.g., intra-canopy lighting (Joshi et al., 2019). To prevent the concurrent change of leaf age and intercepted light, plants could be grown horizontally (Trouwborst et al., 2011a). This, however, is unpractical and introduces artifacts, e.g., the vertical dominance among plant organs is disturbed.
In this article, we hypothesized that modeling light and age acclimation at the intra-leaf level is a feasible approach for estimating vertical parameter profiles over time, i.e., it enables the separation of age and light intensity-related effects in a crop stand. Besides reanalyzing several datasets from the literature, we performed a greenhouse experiment with a vertical growing tomato crop observing leaf parameters in different canopy depths over time. From that, we assessed the spatial-temporal evolution of Vcmax and Jmax.
Materials and Methods
Model Theory, Extension, and Parameter Estimation
Light Absorption Profiles Within a Leaf
The intra-leaf profile of incident and absorbed radiation can be well described by a two-stream-type approach of simultaneous downward and upward radiation transfer with cumulative chlorophyll (a + b) content c within the leaf mesophyll (Terashima et al., 2009). The absorbed light intensity Ia(c) from both streams can be approximated by a simple exponential profile of incident light I(c) times a two-stream absorption coefficient ka (Badeck, 1995; Buckley and Farquhar, 2004).
With incident irradiance I0 on the upper leaf side, effective extinction coefficient k, scaling parameter p1, and exponent p2. We introduced the exponent p2 to allow for an improved fit of Equation 1 to the two-stream solution.
As neither in nature nor in experimental systems, light incidence is exclusively one-sided, Equation 1 was generalized for a two-sided incidence by Buckley and Farquhar (2004) as follows:
with total chlorophyll content (Chl) per leaf area [c = (0, Chl)] and fractional light incidence wu on the upper leaf side, where I0 here denotes the total incident light on both leaf sides.
To obtain predictive equations for the introduced parameters (p1, p2, ka, and k), we applied the Prospect-D leaf spectra model (Féret et al., 2017) and computed scattering and absorption coefficients (ks and ka) with a two-stream solution within the leaf mesophyll:
with downward and upward propagating diffuse radiation fluxes Id and Iu, respectively. External re (air → epidermis) and internal leaf surface reflectance ri (epidermis → air) are calculated from leaf spectral refraction index (n, Féret et al., 2017) and by solving the Fresnel equations for diffuse incident light (Stern, 1964; Jacquemoud and Baret, 1990). The general solution of Equation 3 was obtained (refer to Jacquemoud and Ustin, 2019) with two free constants (C1 and C2) to be estimated from boundary conditions stated in Equation 3. With given total leaf reflectance and total transmittance (R, T),
The radiation transfer parameters ks and ka are estimated from the solution of Equation 4, and the forward problem (Equation 3) to obtain Id(c) and Iu(c) can be computed.
Parameter Estimation of Light Profile Function
In our approach, Ia(c) was subsequently parameterized (i.e., p1, p2, ka, and k) by a five-step procedure using leaf Chl, leaf carotenoid content (Car), and leaf mass per area (LMA):
1. A set of 470 leaves from the Lopex and Angers leaf spectral dataset (Jacquemoud et al., 2003) were selected (i.e., selected leaves exceed the 5% percentile values of Chl and leaf mass water content over the whole dataset).
2. Solving Equations 3, 4 for those leaves resulted accordingly in i = 1…470 values for ks,i ka,i and corresponding profiles of incident radiation I(c) = Id,i(c) + Iu,i(c).
3. The obtained spectral values of Id,i,(c, λ) + Iu,i(c, λ) between 400 and 700 nm were integrated according to a D55 CIE daylight spectral density distribution (Muschaweck, 2021) characterizing a typical daytime sky. The two-stream spectral absorption coefficients ka(λ) were combined similarly to spectral light intensities. In addition, photosynthetic effective absorption (i.e., assuming 100% for chlorophylls and 70% for carotenoids; Laisk et al., 2014) was accounted for by using the absorption spectra for chlorophyll, carotenoid, leaf dry matter, and water from the Prospect D model.
4. Spectral integrated Id,i(c) + Iu,i(c) were then used to fit p1, p2, and k in Equation 1.
5. All obtained parameter sets (p1, ka, k, n = 470) were analyzed via machine learning (Gaussian process regression, MATLAB R2020a, Regression Learner App) using the leaf parameters (features), namely, Chl, Car, and LMA.
Modeling Photosynthetic Electron Transport
To estimate the whole leaf electron transport rate Jleaf, electron transport rate per unit chlorophyll Jc(c) is integrated over cumulative Chl (i.e., mesophyll thickness; Badeck, 1995; Buckley and Farquhar, 2004) using the Blackman response (linear slope and asymptote, Equation 5). This is a good approximation for the light response of electron transport rate at single cell or chloroplast level (Terashima and Saeki, 1985):
with PSII quantum efficiency of electron transport φ, fractional upper light incidence during measurement wu,m and growth wu,g, respectively, a modified extinction coefficient k’, characteristic leaf irradiance I* during light acclimation (Buckley and Farquhar, 2004), and maximum electron transport rate per unit chlorophyll Jc,max. As a generalization of Equation 5, we apply a non-rectangular hyperbola with curvature parameter θ for Jc(c) with the equation as follows:
Following Buckley and Farquhar (2004), Jc,max is described as a function of absorbed radiation profile with a characteristic light intensity I*. We adopted that approach and extended it in three ways, namely, (1) time-dependent minimum [Jc,max,mn(t)] and (2) maximum [Jc,max,mx(t)] values, respectively, and (3) a modified extinction coefficient k′ = p3k (Equation 7). With p3 = 1, the capacity profile of electron transfer would match the light absorption profile perfectly.
The characteristic light intensity I* is determined from the light intensity history (i.e., past days) of each specific leaf. Besides light-induced changes in Chl, Car, and LMA, which determine the intra-leaf profiles (k′) and optical depth (Chl), I* may be interpreted as a mathematical proxy for light-induced changes of key photosynthetic enzymes or complexes (e.g. cytochrome b6f) to chlorophyll ratios (Evans and Seemann, 1989; Eichelmann et al., 2005; Schöttler and Tóth, 2014).
Equation 2 may be applied to leaf gas exchange measurements obtained from a cuvette system (e.g., LI-6400, LICOR Bioscience) with an actinic light source at one leaf side. For that, leaf transmittance needs to be taken into account. Denoting the reflectance of the lower chamber wall by rch and neglecting multiple reflections, one obtains wu,m = 1/(1 + T⋅rch) and I0’ = I0(1 + T⋅rch). Total leaf transmittance T is also estimated from Chl, Car, and LMA using Gaussian process regression. For the LI-6400 standard lower chamber wall, we assumed rch = 0.5. The quantum efficiency φ of absorbed photons was estimated using an expression given by Yin et al. (2004)
With assumed values for the fraction of cyclic electron flow fcyc (0) and maximum e– transport efficiency of PSII Φ2m [0.88, refer to discussion in Kalaji et al. (2017)]. Equation 8 yields φ = 0.468. Other effects of leaf absorptance αL and non-photosynthetic contributions f are fully accounted for by Ia (Equation 2). This is similar to the approach frequently used for bulk leaves (von Caemmerer et al., 2009)
Mathematically, Jmax is the integral of Jc,max(c) over the cumulative Chl, but in the context of A/Ci curves, the retrieved Jmax should be rather approximated as Jleaf(I0′, Chl) at constant light intensity I0. Assuming a unique proportionality between the capacities of electron transport and the Calvin cycle throughout the leaf, Vcmax is given by
with additional parameter p4.
Empirical Data
Tomato Greenhouse Experiment
Experiment and Crop Management
Tomato seeds (“Pannovy”) were sown on 2 January 2018; 9 days after sowing, 48 seedlings were transplanted to stone-wool cubes and placed in a greenhouse controlled at 18°C at the Leibniz Institute of Vegetable and Ornamental Crops (IGZ), Großbeeren, Germany (52.35 N 13.31 E). On 22 February 2018, 48 tomato plants were selected by uniformity and placed on inert fleece mats with drip irrigation in four rows of each 12 plants in one central compartment (28.8 m2) of the gas-exchange greenhouse (GEGH) at the IGZ (Kläring and Körner, 2020). The remaining seven compartments were equipped in the same way, i.e., border effects were minimized. For a starting period of 12 days, the temperature was controlled to 19°C and 15°C during day and night, respectively; air relative humidity (RH) was set to 80% and air CO2 concentration was maintained at 400 μmol mol–1 during daytime. From 5 March 2018, the greenhouse temperature was set at 23°C, while all other setpoints remained unchanged. During all time, water and nutrients were adequately supplied by an automated non-recirculating system. The nutrient solutions were prepared after de Kreij et al. (2003) and were adjusted daily to constrain electric conductivity (EC) between 2.2 and 2.5 dS m–1 and to a mean pH of 5.6. The canopy was maintained at 4 m heights, and the mean leaf number was 18 leaves per plant (counting leaves > 10 cm in length).
Measurements and Computations
Each plant in the canopy was virtually subdivided into 8 vertical layers. For a leaf residing in layer i, the overlaying Leaf Area Index counted to the top (LAIt,i) was estimated from with total ground area per plant SP (4,167 cm2) and one-sided leaf surface area SL,j (cm2) in layer j. Note that one of the two leaves is included in target layer i. The area of a single leaf was derived from time-dependent length (L) and width (W) of leaves as SL,i = 0.2568⋅W(tL) ⋅ L(tL) + 11.725 where leaf age (tL) dependence was adopted from Yu and Körner (2020).
Using hourly recorded air temperatures from a within canopy-installed psychrometer, we calculated the effective thermal time for tomato phenology using a response function with cardinal temperatures adopted from the CROPGRO-Tomato model (Boote et al., 2012). Outside the greenhouse, recorded and hourly averaged PPFD (I0) was modified for greenhouse structure transmission losses and used to calculate the mean intercepted PPFDi,d for each measured leaf during the last d days.
with crop diffuse extinction coefficient k (0.72, Heuvelink, 1996) and back extrapolated LAIt,i starting from the end of the previous day to d days backward with a total of n daylight hours. Note that the specific value of d is estimated during parameter estimation.
Leaf photosynthesis assessments on marked leaves started on 5th of April that was 42 days after transplanting. Three non-neighboring plants, located in the center of the greenhouse, were selected for measuring CO2 response curves in different vertical canopy levels (1–8). Weekly measurements of photosynthesis CO2-response curves (A-Ci curves, LI-COR 6400; LI-COR Inc., Lincoln, NE, United States) were performed on three plants for all leaves with a length of >10 cm starting with leaf number 9 and terminating with leaf number 39. This corresponded to a leaf-age range from 20 to 57 days at the end of the measurements. All A/Ci curves were obtained on one of the two-second leaflets of each leaf (counted from petiole-base). Leaf temperature was set at 25°C, and CO2 concentration (Ca) was changed stepwise to 400, 350, 300, 300, 250, 200, 100, 400, 450, 500, 550, 600, 800, and 1,000 μmol mol–1 while keeping PPFD constant at 1,500 μmol m–2s–1 at an average leaf vapor pressure deficit of about 2.5 kPa. Several measurements were taken within a period of 10 s and averaged after fluxes had been either stabilized or the maximum measurement time of 120 s was encountered. For obtaining the main biochemical parameters of the FCB model (i.e., Vcmax, Jmax at 25°C) from gas exchange measurements, the fitting approach proposed by Ethier and Livingston (2004) was applied, which implicitly accounts partly for the mesophyll conductance effect. Notably, 2–3 single FCB estimates of Vcmax and Jmax per layer and date were averaged.
A handheld spectrophotometer device (Pigment Analyzer PA-1101, CP, Falkensee, Germany), which measures spectral remission between 320 and 1,120 nm at a spectral resolution (SR) of 3.3 nm (Kläring and Zude, 2009), was used on the same plants and leaves (upper side) as used for gas exchange measurements. We applied the Angers optical dataset (Jacquemoud et al., 2003, SR = 1 nm, dicot leaves) to calibrate the optical output of the Pigment Analyzer according to the following equation:
with estimated total chlorophyll (a + b) content per leaf area and measured remissions (of reflectance) (R*) at wavelengths 713, 709, 703, and 699 nm. For calibration (R2 = 0.955, n = 204), only non-senesced leaves were selected from the dataset while accounting for different SRs between the reference dataset and the device. For noise reduction, we only estimated the mean functions of Chl with the relative insertion level (bottom leaves = 0) for April and May (robust linear regression with bisquare weights, robustfit procedure, MATLAB 2020a, refer to Figure 1).
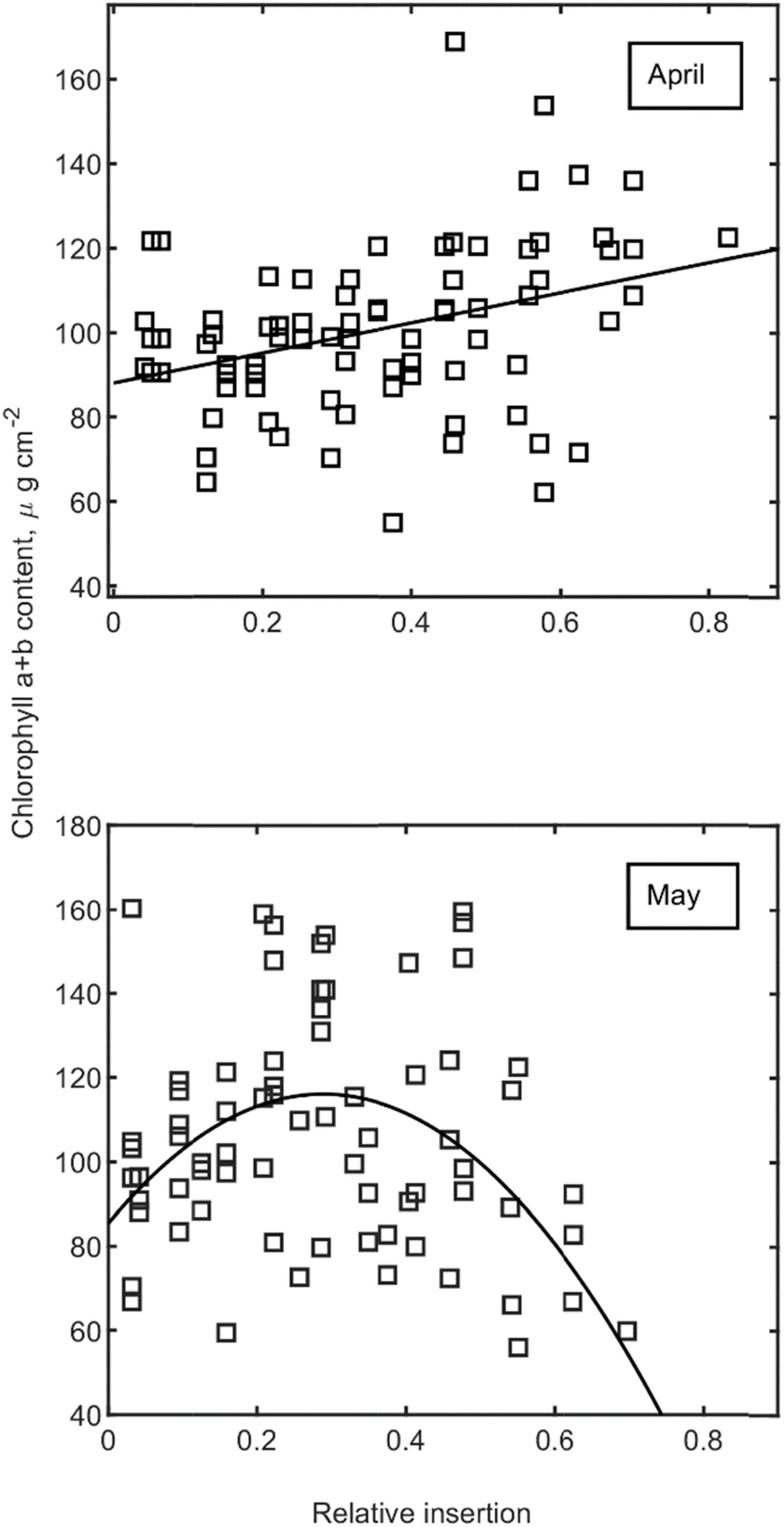
Figure 1. Estimated vertical profiles of chlorophyll content as a function of relative insertion (top leaves = 1, bottom leaves = 0) for the months of April and May. Single estimates (symbols) with linear and quadratic regression functions for April and May, respectively.
Estimated mean Chl(z) profile functions (Figure 1) were further modified by the received sum of PPFDi (mol m–2) during expansion (21 days) of each leaf i, where the effect was assumed to decrease linearly to zero down to an insertion level (zi) of zi = 0.5, i.e., this initial enhancing effect was assumed to be fully diminished for the lower half of the canopy.
The coefficient (m = 0.0447) was estimated from a regression of corresponding data presented by Trouwborst et al. (2011a) and assumed to apply in an additive manner to the mean profiles in Equation 13. denotes the mean intercepted PPFD during April and May accordingly, while Chl(zi) stands for the expected mean Chl content computed from relative leaf insertion level alone (mean curves in Figure 1).
The vertical profile of LMA, which is also required to estimate leaf optical parameters, was described as an empirical function of leaf position, total leaf number, and bottom value of LMA from a reanalysis of functions provided by Edwards et al. (2010) (Figure 2). Specifically, we considered starch as a source of variation in LMA that does not add useful information for leaf optical properties modeling. Therefore, a starch-free LMA profile was parameterized from a set of published expressions for two cultivars and several months (Edwards et al., 2010). Average LMA values obtained at the end of the experiment over the whole canopy were compared well with the calculated mean LMA over the adopted LMA profile function.
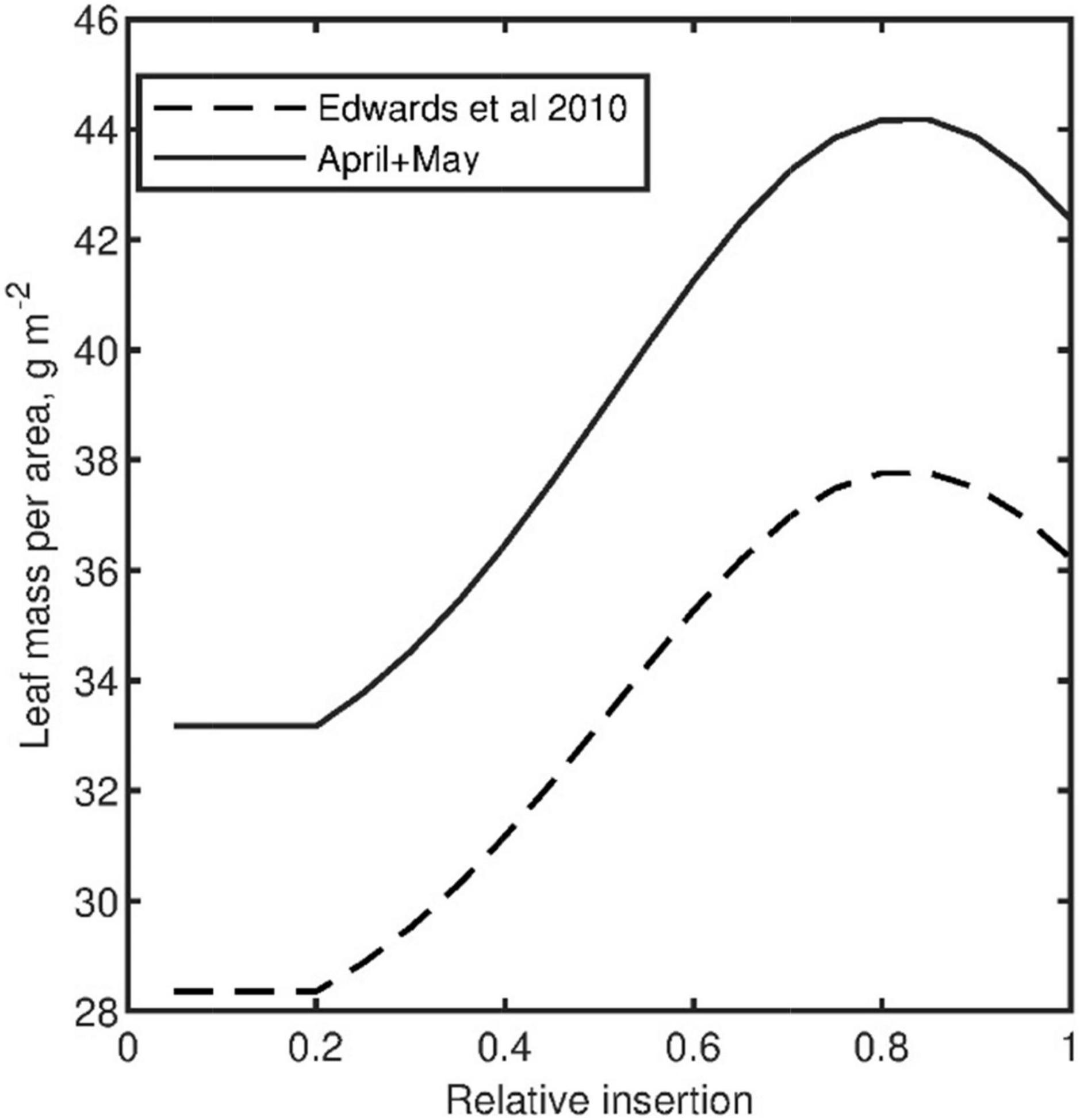
Figure 2. Adopted vertical profile function of leaf mass per area (LMA) (without starch) during the tomato experiment based on LMA measurements of bottom leaves (relative insertion = 0) and an empirical equation form parameterized from Edwards et al. (2010).
Photosynthetic Capacity in Spinach, Eucalyptus, and Cucumber
The profiles of photosynthetic capacity were analyzed with published data of three different crops, i.e., spinach (Spinacia oleracea), eucalyptus (Eucalyptus pauciflora), and cucumber (Cucumis sativus).
Photosynthetic capacity vs. cumulative chlorophyll content for S. oleracea and vertical E. pauciflora leaves were obtained from Nishio et al. (1993) and Evans and Vogelmann (2006), respectively. The effective extinction coefficient k was estimated through Gaussian process regression functions using leaf features Chl, LMA, and Car (refer to Table 2). The measured relative capacity profiles [Cn(c)] were then compared to a normalized form of Equation 7, with estimated φ, p1ka, and I*.
Measured properties of horizontal cucumber leaves and photosynthetic light response 7 days after a step change in growth irradiance at 4 different light transitions were tested (Table 1; Trouwborst et al., 2011b). The provided values of Jmax and net photosynthesis rates An at 25°C were converted to leaf electron transfer rates Jleaf, assuming 50% reduction of dark respiration (Rd) in light,
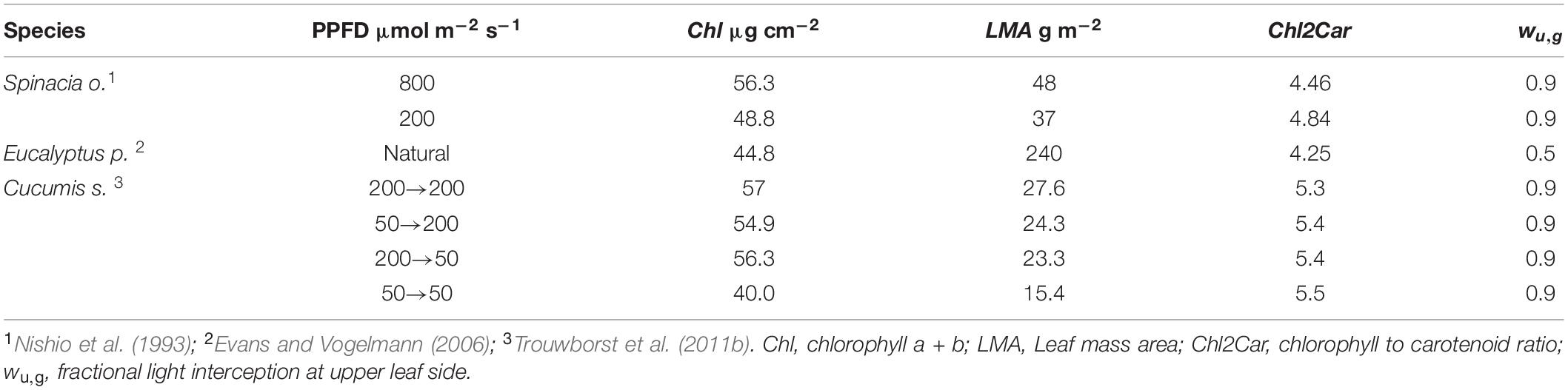
Table 1. Leaf properties used for model testing of photosynthetic capacity profile and electron transport rates.

Table 2. Parameter estimates for the fit of Equation 6 to electron transport rate of differently light acclimated cucumber leaves.
with CO2 compensation point Γ* set to 42.75 ppm (Bernacchi et al., 2001) and leaf internal CO2 concentration Ci (ppm).
Results
Empirical Description of Simplified Leaf Radiation Transfer Parameters
A major prerequisite for the following analysis is the validity of Equation 1 with profile parameters estimated from bulk leaf properties Chl, Car, and LMA. Setting the coefficient p2 to 0.664 for all leaves improved the fit of Equation 1 to computed profiles of Id(c) + Iu(c) (Equations 3, 4). The root mean squared error (RMSE) decreased from 0.0263 with p2 = 1 (i.e., the standard approach) to an RMSE of 0.01 (p2 = 0.664). Figure 3 shows that the remaining parameters (p1, k, and ka) can be fairly well predicted from leaf properties Chl, Car, and LMA using Gaussian process regression. Due to the two-stream nature of radiation transfer and manifested by the p1 parameter, radiation intensities may exceed 1 (Figure 3A). It is more feasible to estimate the product p1ka (Figure 3D) than its terms separately.
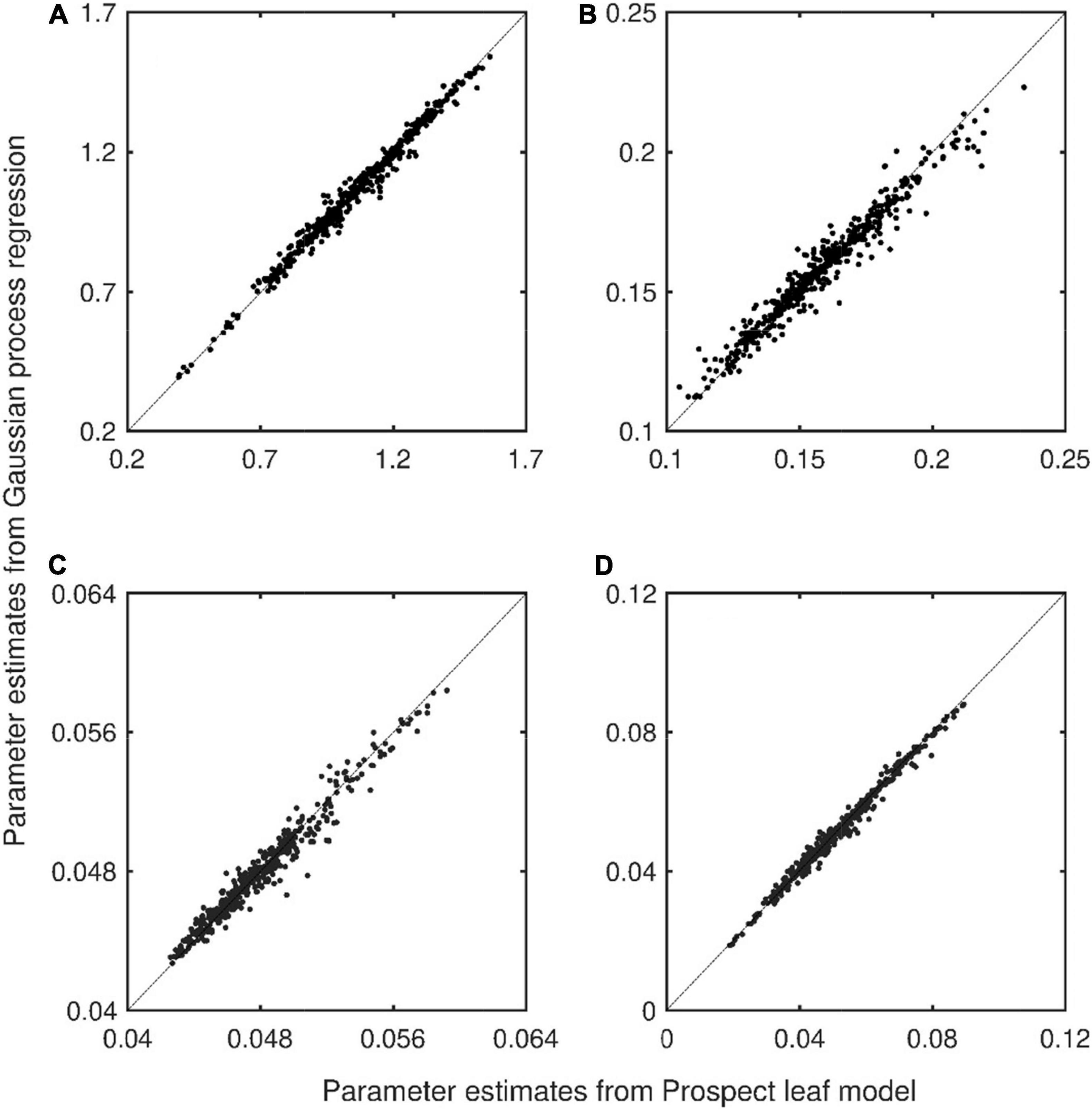
Figure 3. Estimated parameters from the Prospect model (using Equations 1–4) vs. empirical regression estimates using Gaussian process regression y = f(Chl, LMA, Car) with 1:1 line. n = 470, Lopex and Angers leaf optical properties datasets, (A) scaling parameter p1, (B) effective leaf extinction coefficient k, (C) absorption coefficient ka, and (D) the product of p1 and ka. Optical depth is the chlorophyll content (Chl; μg/cm2).
Testing for the Coincidence of Photosynthetic Capacity and Light Absorption
To test Equation 7, we compared the profiles of the normalized light gradient Ia (c, wu,g, I*, k p3)/Ia (0, wu,g, I*, k p3) with published profiles of maximum photosynthetic capacity in Spinach (Nishio et al., 1993; Terashima et al., 2009) and E. pauciflora (Evans and Vogelmann, 2006; Figure 4). While estimating k from given values of Chl, Car, and LMA, we could not justify a perfect match between light absorption and capacity profiles as fitted p3 was always significantly lower than one [5% confidence region for all fitted p3 = (0.156, 0.789)]. As those datasets are most suitable for the identification of p3, we set it in the following to the mean of the obtained 3 estimates (p3 = 0.54).
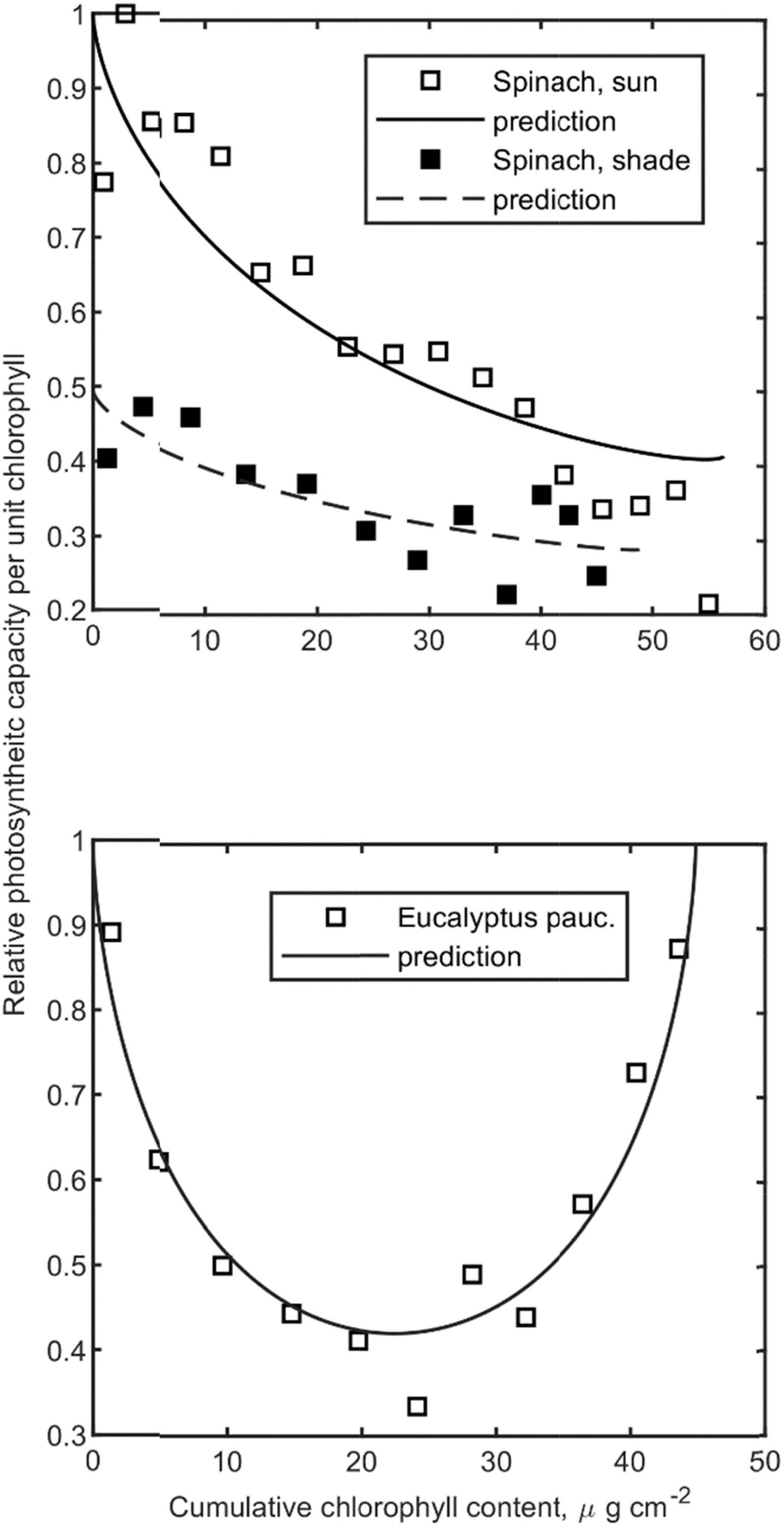
Figure 4. Relative photosynthetic capacity vs. cumulative Chl. (Upper graph) Sun [k = 0.1457, fitted p3 = 0.53 (0.458–0.628)] and shade [k = 0.1455, fitted p3 = 0.36 (0.156–0.559)]-treated horizontal spinach leaves (wu,g = 0.9, Nishio et al., 1993). (Lower graph) Vertical isobilateral leaves of E. pauciflora (k = 0.2535, fitted p3 = 0.73 (0.675–0.789), wu,g = 0.5, Evans and Vogelmann, 2006). Confidence limits are given in parenthesis, p = 0.05.
Testing Modified Electron Transfer by Light Acclimation in Cucumber
Published data for electron transport of cucumber leaves (Trouwborst et al., 2011b) could be predicted with fitting parameters to Equation 5 (Table 2 and Figure 5). The estimated empirical model for I* is as follows:
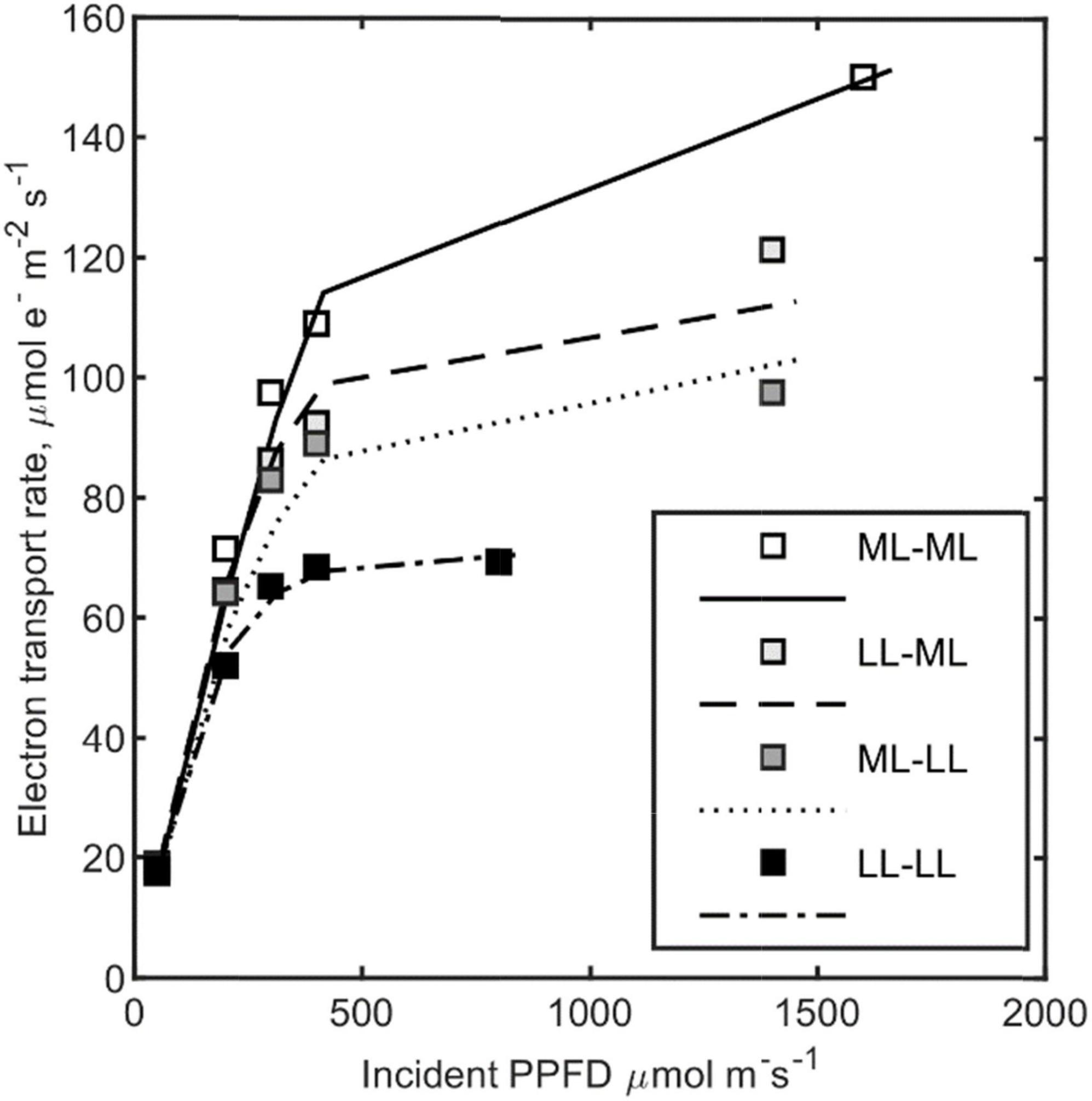
Figure 5. Measured electron transport rates (symbols) and predictions by Equation 6 (lines) vs. incident photosynthetic photon flux density (PPFD) at 7 days after a step change in light intensity for cucumber leaves (Trouwborst et al., 2011b), ML-ML (I1:200 → I2:200 μmol m2 s–1), LL-ML (I1:50 → I2:200 μmol m2 s–1), LL-ML (I1:200 → I2:50 μmol m–2 s–1), and LL-LL (I1:50 → I2:50 μmol m–2 s–1). Plants were measured after 7 days growing at intensity I2. Lines connect computations at assessed PPFD (i.e., symbols). n = 20, root mean squared error = 4.22 μmol e– m–2 s–1.
With pi2 being significantly greater than zero (Table 2), a large influence exists from the preceding light intensity prior to step change. Note that calculated I* is here greater than the mean intensity during growth.
The minimum of Jc,max (Jc,max,mn) was only active at constant low light treatment (LL-LL). The estimated value for θ (0.962) will also be used in subsequent steps.
Vcmax and Jmax in Different Canopy Levels and Leaf Ages in a Tomato Crop
Parameter Estimation
Overall, the tested mechanistic model for photosynthetic light acclimation proved to be successful (Figure 6). The model could explain 68 or 72% of the observed variance for Vcmax and Jmax, respectively (Table 3). The estimated empirical model for I* is as follows:
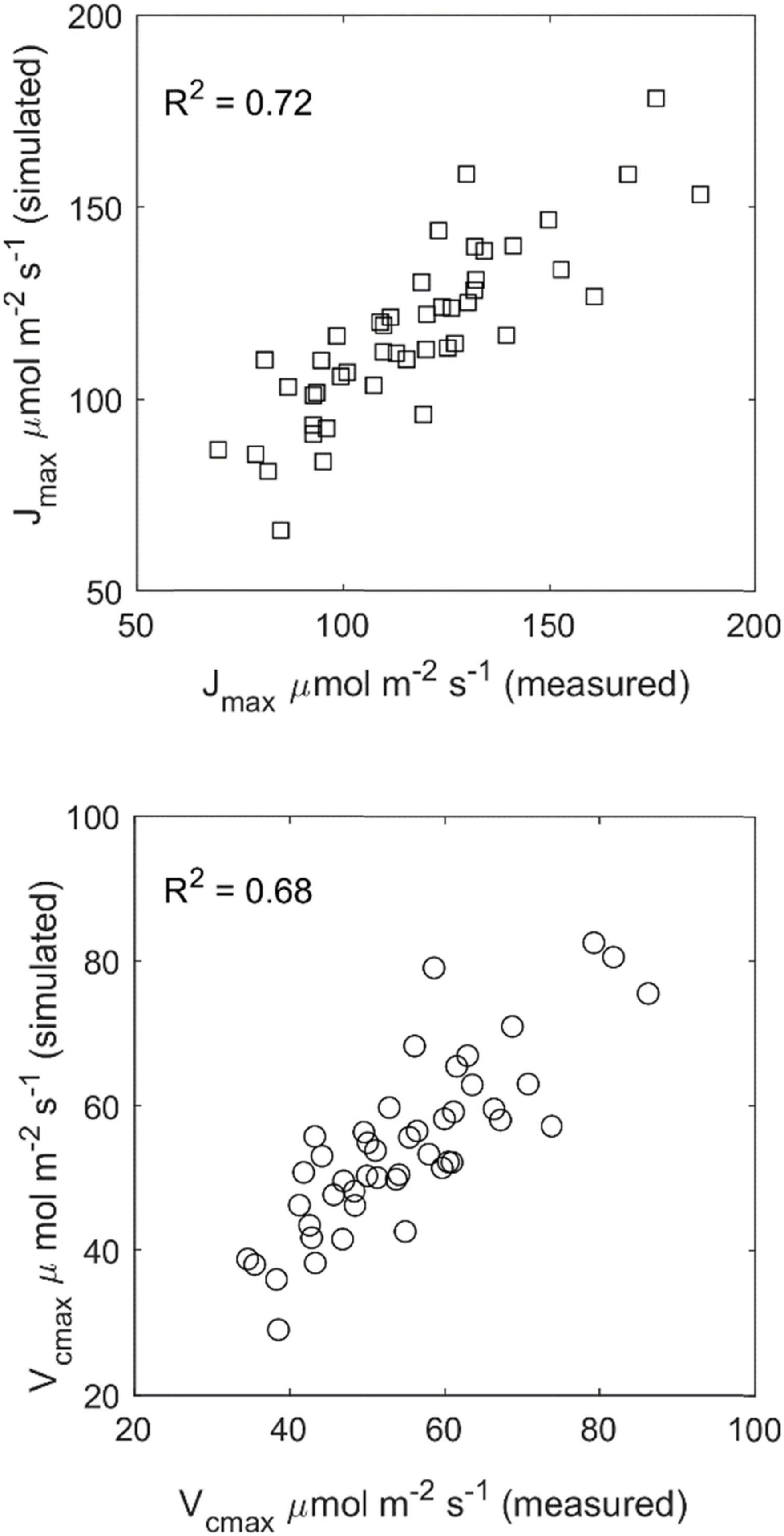
Figure 6. Comparison of predicted vs. measured maximum rates of carboxylation (Vcmax) and electron transport (Jmax) at different canopy depths over 2 months in a tomato canopy. n = 46. Refer to Table 2 for more details.
Best fitting results (in terms of the sum of squares) were obtained manually with d = 3, e.g., 3 previous days were used to compute for each leaf (equally weighted mean calculation). Alternative non-linear time weighting schemes improved the model fit marginally toward d values of 4–5 days.
The proportionality constant pi1 could be well identified for this dataset but at a lower value compared to cucumber (Table 2).
For the time dependence of minimum and maximum Jc,max (Jc,max,mn, Jc,max,mx), which is here considered an aging process, the following relation was adopted.
with an hourly sum of the phenology response since leaf appearance PRsum and empirical parameters pJ0 and pJ1. The factor n was set to 2.6, the mean ratio obtained from experimental estimates (Evans and Seemann, 1989) on bulk leaves of several species.
For about 46% of the tested leaves, the photosynthetic capacity was constrained by PRsum, i.e., Jc,max,mn(t) was set as a lower limit in Equation 7.
Model Simulation
Assuming constant leaf properties and light intensities, different limitation onsets of electron flow by aging and light adaptation were investigated. At low light intensities (PPFD = 250 μmol m–2 s–1, Figure 7A) the computed mean rate of electron transfer (symbols in Figures 7A–C) was almost entirely determined by the ontogenetic prescribed lower limit of electron transfer which decreases monotonically over time. Similarly, the calculated Vcmax (Figure 7D) was decreasing continuously over time. In contrast, at higher PPFD (750 μmol m–2 s–1, Figure 7C), the electron flow could be determined by (constant) light acclimation and was later constrained by the upper limit of the ontogenetic prescribed range of electron flow (Figure 4C). This scenario results in an almost time-invariant behavior of Vcmax (Figure 4D).
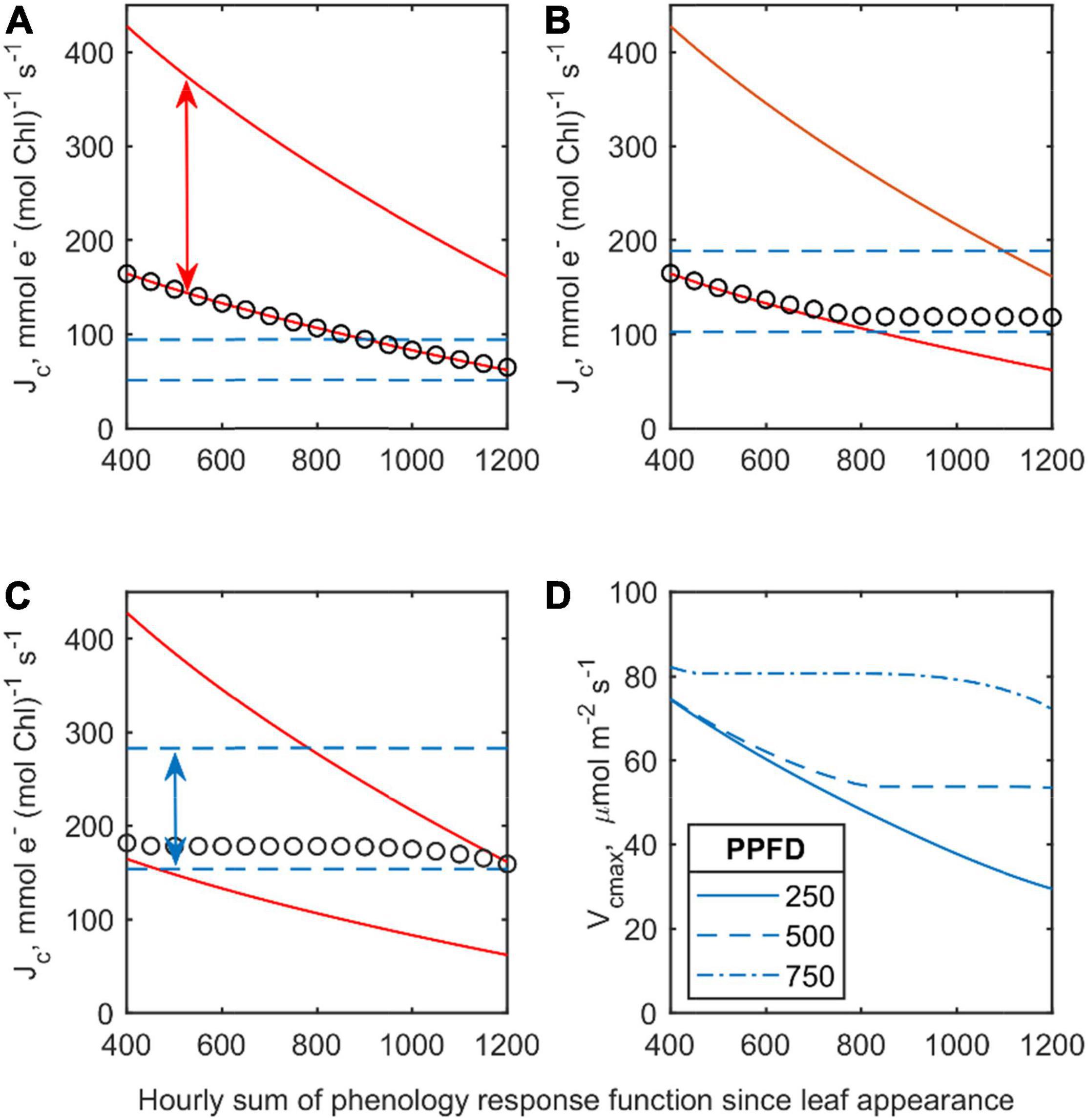
Figure 7. (A–C) Simulated adaptation ranges of electron flow (lines) and final mean leaf rate (symbols) over time at different time constant PPFD: (A) 250 μmol m–2 s–1, (B) 500 μmol m–2 s–1, and (C) 750 μmol m–2 s–1. Lower and upper red lines are Jx,max,mn and Jx,max,mx, respectively. Lower and upper blue dashed lines are minima and maxima set by the profile of absorbed light, respectively. (D) Corresponding calculated rates of Vcmax over time.
Discussion
Model-Framework Validity
We present a novel mathematical framework (Equations 2, 5–10) to describe the time dependency of the FCB photosynthetic model parameters (φ′, θ, Jmax, and Vcmax) caused by progressing leaf phenology and light acclimation. The derived relations build on previous work to model light acclimation (Badeck, 1995) or whole leaf electron transport rates (Buckley and Farquhar, 2004). The proposed model framework requires an accurate specification of the incoming radiation field [PPFD(t), wu,m, and wu,g], additional leaf traits (Chl, Car, and LMA), and further parameters (Jc,max,mx, Jc,max,mn, I*) that are likely functions of perceived temperatures and intercepted light intensities during leaf growth (Equations 16–18).
We tested the capability of the framework to predict published intra-leaf photosynthetic capacity profiles (Figure 4), light response curves for differently light-adapted cucumber leaves (Figure 5), and measured Jmax and Vcmax values at different times and canopy depths in a tomato crop. To limit the degree of freedom for each step, we estimated several parameters hierarchically from independent datasets, e.g., p1ka and k using generated leaf optics data, p3 from capacity profiles, and θ from light response curves.
Clearly, to explore the full validity of our proposed theory, more experimental work with vertically and horizontally grown tomato and cucumber crops is required. An evident key role in this matter was identified in leaf Chl content. Being an integration variable (e.g., Equation 10) it also influences intra-leaf absorption parameters via Gaussian process regression. This fits well with recent observations in various species of Vcmax and Jmax-Chl relations being better predictors than leaf nitrogen (Qian et al., 2021). However, neither its repeatable measurement nor its empirical prediction of Chl in time and space seems to be trivial. For tomato, Chl is dependent on the received light intensity during leaf expansion (Equation 13), Trouwborst et al. (2011a), while it declines with canopy depth (Figure 1).
Model Framework in a Current Scientific Context
Due to multiple and internal reflections (ri) at the leaf epidermis-air interface (Equation 3), the total received irradiance at the topmost mesophyll layer may exceed the incident intensity (Figure 3). This phenomenon has been theoretically predicted and measured (Vogelmann and Björn, 1984). Therefore, the specific parameter p1 was introduced (Figure 3A). A more effective way to predict the profile of absorbed radiation (Equation 2); however, is combining p1 with ka, i.e., p1ka (Figure 4D).
Analogously to the distribution of leaf photosynthetic capacity and leaf nitrogen content with canopy depth, a covariation of photosynthetic capacity profiles with intra-leaf absorbed radiation was observed (Figure 4). Consistently over all three observed capacity profiles, the agreement was imperfect: p3 (on average 0.54) was significantly lower than 1. Earlier studies with whole leaves support our finding: A canopy scale meta-study estimated an analog reduction of the light extinction coefficient by 0.5 (Hikosaka et al., 2016).
The obtained estimate for θ = 0.962 for cucumber leaves (Table 2) corresponds well with an average figure of 0.965 reported by Terashima and Saeki (1985) for chloroplast and cell suspensions. Similarly, Jc,max,mn estimated at 161 was similar to measurements in shaded cucumber leaves of 160 (PPFD = 120 μmol m–2 s–1; Evans, 1989). For dicot plants common bean (Phaseolus vulgaris) and tobacco (Nicotiana tabacum), there is strong evidence that the ratio of the leaf cytochrome b6f complex to chlorophyll content is the major target for both light acclimation and leaf aging (Schöttler and Tóth, 2014), which is linear related to electron flow (Evans and Seemann, 1989). Moreover, this ratio changes for tobacco by a factor of 2.45 from low to high light-adapted leaves (Schöttler and Tóth, 2014), which is close to the adopted value Jc,max,mx/Jc,max,mn = 2.6 (Evans and Seemann, 1989) based on measured electron transport rates.
A strong correlation between Vcmax and Jmax is well known. Wullschleger (1993) presented a Vcmax to Jmax ratio of 0.431 for vegetable crops (17 species), obtained from A/Ci curves assuming implicitly a fixed curvature θ of leaf electron transfer. This ratio evolves automatically as parameter p4 in Equation 10, with an estimated value of p4 = 0.437 for tomato (Table 3), a remarkable agreement of Wullschleger’s result and our estimate.
The bifacial nature of leaf morphology of dicot plants is often accompanied by different leaf reflectance and transmittances measured from the adaxial and abaxial leaf sides (De Lucia et al., 1991; Stuckens et al., 2009). This indicates different effective two-stream parameters depending on whether light is incident on the adaxial or abaxial leaf side. Therefore, additional research would be needed to investigate the necessity of introducing different parameters for the palisade and spongy mesophyll layers (Terashima et al., 2009). Especially for cases with significant light incidence from the lower leaf side, either during acclimation or measurement, this might be of importance.
Extensions to the Model Framework
The major foundation of this analysis is the assumption of the validity of the two-stream approximation of radiation transfer for leaves. This includes the need for identifying two parameters (ks, ka) from total leaf transmittance and reflectance while accounting for diffuse Fresnel reflectance/transmittances at the leaf boundaries (Equations 3, 4). This approach assumes perfectly diffused radiation streams, with equal probability of backward and forward scattering of photons, setting the anisotropy parameter for scattering g to zero. However, an accurate approximation to the radiation transfer equation for a scattering and absorbing slab was recently derived (Liemert et al., 2019). This solution could be a useful asset in improving parameter calibration of Equation 2 or similar functions, which eventually can lead to the derivation of better approximations; even different incidence angles and refraction index changes at the leaf surface can be accounted for Liemert et al. (2019). For that, an independent spectral parameterization of the anisotropy parameter g(λ) (or the scattering phase function) would be required. Measurements on various biological tissues indicate a rather smooth and slow change of g(λ) over the visible wavelength range (Jacques, 2013).
Ways for Practical Application
Both from a theoretical and experimental standpoint, the quantification of received radiation fluxes per leaf (patch) within plant canopies is not straightforward. In real (commercial cultivated) canopies, the leaf-specific and time-dependent estimation could be supported with imaging techniques. One solution would be the combination of a hemispherical gap fractions distribution from fisheye imaging (Eichelmann et al., 2005) with our model framework. Model predictions and accurate specification of the incoming radiation field could be a basis for a powerful monitoring tool in vertical crop stands. In addition, there is a growing number of functional structural plant model (FSPM) codes (Buck-Sorlin et al., 2011; Sarlikioti et al., 2011) and libraries (Bailey, 2019), which are in principle well suited to provide this information even on a leaf patch basis in virtual canopies.
Conclusion
In this study, we extended a previous leaf model for electron transport rate (Buckley and Farquhar, 2004) to account for the phenomenon of non-perfect acclimation of photosynthetic capacity to absorbed radiation within the mesophyll. Adopting the two-stream solution of radiation transfer with cumulative chlorophyll content, we derive the scattering and absorption coefficients from the total reflectance and transmittance of leaves. This allowed the derivation of an improved simplified model for absorbed radiation profile and corresponding lumped parameters, which can be estimated just from total chlorophyll, carotenoid, and dry mass content per leaf area using machine learning methods. A reanalysis of published datasets with this simplified model revealed a significant derivation of measured photosynthetic capacity profiles from calculated absorption profiles, while this deviation can be resolved empirically.
Furthermore, the applicability of the modified model was tested on light acclimation on published experimental data with cucumber (Trouwborst et al., 2011b) and with a self-performed tomato cultivation experiment. These tests revealed that ontogenetic constraints are likely to be superimposed on light intensity effects within the leaf mesophyll.
Data Availability Statement
The raw data supporting the conclusions of this article will be made available by the authors, without undue reservation.
Author Contributions
JG: model development, model conception and realization, manuscript writing, data management, simulations, and figures. WY: experimental measurements, first draft of manuscript, and model conception. OK: experimental design, experimental supervision, and manuscript writing. All authors contributed to the article and approved the submitted version.
Conflict of Interest
The authors declare that the research was conducted in the absence of any commercial or financial relationships that could be construed as a potential conflict of interest.
Publisher’s Note
All claims expressed in this article are solely those of the authors and do not necessarily represent those of their affiliated organizations, or those of the publisher, the editors and the reviewers. Any product that may be evaluated in this article, or claim that may be made by its manufacturer, is not guaranteed or endorsed by the publisher.
Acknowledgments
We thank Angela Schmidt and Robert Klose for their elaborate technical assistance.
References
Badeck, F. W. (1995). Intra-leaf gradient of assimilation rate and optimal allocation of canopy nitrogen: a model on the implications of the use of homogeneous assimilation functions. Aust. J. Plant Physiol. 22, 425–439. doi: 10.1071/PP9950425
Bailey, B. N. (2019). Helios: A Scalable 3D Plant and Environmental Biophysical Modeling Framework. Front. Plant Sci. 10:1185. doi: 10.3389/FPLS.2019.01185/BIBTEX
Bernacchi, C. J., Singsaas, E. L., Pimentel, C., Portis, A. R. Jr., and Long, S. P. (2001). Improved temperature response functions for mod- els of Rubisco-limited photosynthesis. Plant Cell Environ. 24, 253–260. doi: 10.1046/j.1365-3040.2001.00668.x
Bonan, G. B., Patton, E. G., Finnigan, J. J., Baldocchi, D. D., and Harman, I. N. (2021). Moving beyond the incorrect but useful paradigm: reevaluating big-leaf and multilayer plant canopies to model biosphere-atmosphere fluxes – a review. Agric. For. Meteorol. 306:108435. doi: 10.1016/J.AGRFORMET.2021.108435
Boote, K. J., Rybak, M. R., Scholberg, J. M. S., and Jones, J. W. (2012). Improving the CROPGRO-tomato model for predicting growth and yield response to temperature. HortScience 47, 1038–1049. doi: 10.21273/hortsci.47.8.1038
Buckley, T. N., and Farquhar, G. D. (2004). A new analytical model for whole-leaf potential electron transport rate. Plant Cell Environ. 27, 1487–1502. doi: 10.1111/j.1365-3040.2004.01232.x
Buck-Sorlin, G., De Visser, P. H. B., Henke, M., Sarlikioti, V., Van Der Heijden, G. W. A. M., Marcelis, L. F. M., et al. (2011). Towards a functional structural plant model of cut-rose: simulation of light environment, light absorption, photosynthesis and interference with the plant structure. Ann. Bot. 108, 1121–1134. doi: 10.1093/aob/mcr190
de Kreij, C., Voogt, W., and Baas, R. (2003). Nutrient Solutions and Water Quality for Soilless Cultures. Available online at: https://library.wur.nl/WebQuery/wurpubs/fulltext/456342 (accessed June 9, 2022).
De Lucia, E. H., Shenoi, H. D., Naidu, S. L., and Day, T. A. (1991). Photosynthetic symmetry of sun and shade leaves of different orientations. Oecologia 87, 51–57. doi: 10.1007/BF00323779
Edwards, D., Jolliffe, P., and Ehret, D. (2010). Canopy profiles of starch and leaf mass per area in greenhouse tomato and the relationship with leaf area and fruit growth. Sci. Hortic. 125, 637–647. doi: 10.1016/j.scienta.2010.05.019
Eichelmann, H., Oja, V., Rasulov, B., Padu, E., Bichele, I., Pettai, H., et al. (2005). Adjustment of leaf photosynthesis to shade in a natural canopy: reallocation of nitrogen. Plant Cell Environ. 28, 389–401. doi: 10.1111/J.1365-3040.2004.01275.X
Ethier, G. J., and Livingston, N. J. (2004). On the need to incorporate sensitivity to CO2 transfer conductance into the Farquhar-von Caemmerer-Berry leaf photosynthesis model. Plant Cell Environ. 27, 137–153. doi: 10.1111/j.1365-3040.2004.01140.x
Evans, J. R. (1989). Partitioning of Nitrogen Between and Within Leaves Grown under Different Irradiances. Fun. Plant Biol. 16, 533-548.
Evans, J. R., and Seemann, J. R. (1989). “The allocation of protein nitrogen in the photosynthetic apparatus: costs, consequences, and control”. in Photosynthesis, ed W. R. Briggs (New York: A.R. Liss), 183–205.
Evans, J. R., and Vogelmann, T. C. (2006). Photosynthesis within isobilateral Eucalyptus pauciflora leaves. New Phytol. 171, 771–782. doi: 10.1111/j.1469-8137.2006.01789.x
Féret, J. B., Gitelson, A. A., Noble, S. D., Jacquemoud, S., and Féret, J.-B. (2017). PROSPECT-D: towards modeling leaf optical properties through a complete lifecycle PROSPECT-D: towards modeling leaf optical properties through a complete lifecycle 1. Remote Sens. Environ 193, 204–215. doi: 10.1016/j.rse.2017.03.004ï
Heuvelink, E. (1996). Tomato Growth and Yield: Quantitative Analysis and Synthesis. Available online at: https://search.proquest.com/openview/63d3499a7abf92da21725bb0410836dc/1?pq-origsite=gscholar&cbl=2026366&diss=y (accessed on Feb 22, 2022).
Hikosaka, K., Anten, N. P. R., Borjigidai, A., Kamiyama, C., Sakai, H., Hasegawa, T., et al. (2016). A meta-analysis of leaf nitrogen distribution within plant canopies. Ann. Bot. 118, 239–247. doi: 10.1093/aob/mcw099
Jacquemoud, S., and Baret, F. (1990). PROSPECT: A Model of Leaf Optical Properties Spectra. Science 91, 75–91. doi: 10.1364/AO.49.001687
Jacquemoud, S., Bidel, L., Francois, C., and Pavan, G. (2003). ANGERS Leaf Optical Properties Database. Available online at: http://opticleaf.ipgp.fr/index.php?page=database (Accessed June 9, 2022).
Jacquemoud, S., and Ustin, S. (2019). Leaf Optical Properties. Cambridge: Cambridge University Press, doi: 10.1017/9781108686457
Jacques, S. L. (2013). Optical properties of biological tissues: a review. Phys. Med. Biol. 58, R37–61. doi: 10.1088/0031-9155/58/14/5007
Joshi, N. C., Ratner, K., Eidelman, O., Bednarczyk, D., Zur, N., Many, Y., et al. (2019). Effects of daytime intra-canopy LED illumination on photosynthesis and productivity of bell pepper grown in protected cultivation. Sci. Hortic. 250, 81–88. doi: 10.1016/j.scienta.2019.02.039
Kalaji, H. M., Schansker, G., Brestic, M., Bussotti, F., Calatayud, A., Ferroni, L., et al. (2017). Frequently asked questions about chlorophyll fluorescence, the sequel. Photosynth Res 132, 13–66. doi: 10.1007/s11120-016-0318-y
Kläring, H. P., and Körner, O. (2020). Design of a real-time gas-exchange measurement system for crop stands in environmental scenarios. Agronomy 10:737. doi: 10.3390/agronomy10050737
Kläring, H. P., and Zude, M. (2009). Sensing of tomato plant response to hypoxia in the root environment. Sci. Hortic. 122, 17–25. doi: 10.1016/J.SCIENTA.2009.03.029
Körner, O., Aaslyng, J. M., Andreassen, A. U., and Holst, N. (2007). Microclimate prediction for dynamic greenhouse climate control. HortScience 42, 272–279. doi: 10.21273/HORTSCI.42.2.272
Laisk, A., Oja, V., Eichelmann, H., and Dall’Osto, L. (2014). Action spectra of photosystems II and i and quantum yield of photosynthesis in leaves in State 1. Biochim. Biophys. Acta Bioenerg. 1837, 315–325. doi: 10.1016/j.bbabio.2013.12.001
Lichtenthaler, H. K., and Babani, F. (2004). “Light adaptation and senescence of the photosynthetic apparatus. Changes in pigment composition, chlorophyll fluorescence parameters and photosynthetic activity,” in Chlorophyll a Fluorescence, eds G. C. Papageorgiou Govindjee (Dordrecht: Springer), 713–736. doi: 10.1007/978-1-4020-3218-9_28
Liemert, A., Martelli, F., Binzoni, T., and Kienle, A. (2019). P 3 solution for the total steady-state and time-resolved reflectance and transmittance from a turbid slab. Appl. Opt. 58, 4143–4148. doi: 10.1364/ao.58.004143
Meir, P., Kruijt, B., Broadmeadow, M., Barbosa, E., Kull, O., Carswell, F., et al. (2002). Acclimation of photosynthetic capacity to irradiance in tree canopies in relation to leaf nitrogen concentration and leaf mass per unit area. Plant Cell Environ. 25, 343–357. doi: 10.1046/j.0016-8025.2001.00811.x
Muschaweck, J. (2021). JMO_Spectrum. Available online at: https://www.mathworks.com/matlabcentral/images/matlab-file-exchange.svg)](https://uk.mathworks.com/matlabcentral/fileexchange/98044-jmo_spectrum) (Accessed June 9, 2022).
Myneni, R. B., and Ross, J. (2012). Photon-Vegetation Interactions: Applications in Optical Remote Sensing and Plant Ecology. Berlin: Springer Science & Business Media.
Niinemets, Ü (2016). Leaf age dependent changes in within-canopy variation in leaf functional traits: a meta-analysis. J. Plant Res. 129, 313–338. doi: 10.1007/s10265-016-0815-2
Niinemets, Ü, Kull, O., and Tenhunen, J. D. (2004). Within-canopy variation in the rate of development of photosynthetic capacity is proportional to integrated quantum flux density in temperate deciduous trees. Plant Cell Environ. 27, 293–313. doi: 10.1111/J.1365-3040.2003.01143.X
Nishio, J. N., Sun, J., and Vogelmann, T. C. (1993). Carbon fixation gradients across spinach leaves do not follow internal light gradients. Plant Cell 5, 953–961. doi: 10.2307/3869663
Qian, X., Liu, L., Croft, H., and Chen, J. (2021). Relationship Between Leaf Maximum Carboxylation Rate and Chlorophyll Content Preserved Across 13 Species. J. Geophys. Res. Biogeosci. 126:e2020JG006076. doi: 10.1029/2020JG006076
Russell, G., Marshall, B., and Jarvis, P. G. (1990). Plant canopies: their growth, form and function. Cambridge: Cambridge University Press.
Sarlikioti, V., De Visser, P. H. B., and Marcelis, L. F. M. (2011). Exploring the spatial distribution of light interception and photosynthesis of canopies by means of a functionalstructural plant model. Ann. Bot. 107, 875–883. doi: 10.1093/aob/mcr006
Schöttler, M. A., and Tóth, S. Z. (2014). Photosynthetic complex stoichiometry dynamics in higher plants: environmental acclimation and photosynthetic flux control. Front. Plant Sci. 5:188. doi: 10.3389/fpls.2014.00188
Stern, F. (1964). Transmission of isotropic radiation across an interface between two dielectrics. Appl. Opt. 3:111. doi: 10.1364/ao.3.000111
Stuckens, J., Verstraeten, W. W., Delalieux, S., Swennen, R., and Coppin, P. (2009). A dorsiventral leaf radiative transfer model: development, validation and improved model inversion techniques. Remote Sens. Environ. 113, 2560–2573. doi: 10.1016/J.RSE.2009.07.014
Terashima, I., Fujita, T., Inoue, T., Chow, W. S., and Oguchi, R. (2009). Green light drives leaf photosynthesis more efficiently than red light in strong white light: revisiting the enigmatic question of why leaves are green. Plant Cell Physiol. 50, 684–697. doi: 10.1093/pcp/pcp034
Terashima, I., and Saeki, T. (1985). A new model for leaf photosynthesis incorporating the gradients of light environment and of photosynthetic properties of chloroplasts within a leaf. Ann. Bot. 56, 489–499. doi: 10.1093/oxfordjournals.aob.a087034
Trouwborst, G., Hogewoning, S. W., Harbinson, J., and van Ieperen, W. (2011a). The influence of light intensity and leaf age on the photosynthetic capacity of leaves within a tomato canopy. J. Hortic. Sci. Biotechnol. 86, 403–407. doi: 10.1080/14620316.2011.11512781
Trouwborst, G., Hogewoning, S. W., Harbinson, J., and Van Ieperen, W. (2011b). Photosynthetic acclimation in relation to nitrogen allocation in cucumber leaves in response to changes in irradiance. Physiol. Plant 142, 157–169. doi: 10.1111/j.1399-3054.2011.01456.x
Vogelmann, T. C., and Björn, L. O. (1984). Measurement of light gradients and spectral regime in plant tissue with a fiber optic probe. Physiol. Plant 60, 361–368. doi: 10.1111/j.1399-3054.1984.tb06076.x
von Caemmerer, S., Farquhar, G., and Berry, J. (2009). “Biochemical Model of C3 Photosynthesis,” in Photosynthesis in Silico, eds A. Laisk and L. Nedbal Govindjee (Springer, Dordrecht), 209–230. doi: 10.1007/978-1-4020-9237-4_9.
Wullschleger, S. D. (1993). Biochemical limitations to carbon assimilation in C3 plants- A retrospective analysis of the A/Ci Curves from 109 species. J. Exp. Bot. 44, 907–920.
Yin, X., Yin, X., Van Oijen, M., and Schapendonk, A. H. C. M. (2004). Extension of a biochemical model for the generalized stoichiometry of electron transport limited C 3 photosynthesis. Plant Cell Environ. 27, 1211–1222.
Keywords: light acclimation, Jmax, chlorophyll, tomato, intra-leaf, age, LMA, Vcmax
Citation: Graefe J, Yu W and Körner O (2022) A Photosynthetic Light Acclimation Model Accounting for the Effects of Leaf Age, Chlorophyll Content, and Intra-Leaf Radiation Transfer. Front. Plant Sci. 13:889709. doi: 10.3389/fpls.2022.889709
Received: 04 March 2022; Accepted: 30 May 2022;
Published: 22 June 2022.
Edited by:
Jung Eek Son, Seoul National University, South KoreaReviewed by:
Nikolaos Katsoulas, University of Thessaly, GreeceQuan Wang, Shizuoka University, Japan
Dae Ho Jung, Cheonan Yonam College, South Korea
Copyright © 2022 Graefe, Yu and Körner. This is an open-access article distributed under the terms of the Creative Commons Attribution License (CC BY). The use, distribution or reproduction in other forums is permitted, provided the original author(s) and the copyright owner(s) are credited and that the original publication in this journal is cited, in accordance with accepted academic practice. No use, distribution or reproduction is permitted which does not comply with these terms.
*Correspondence: Jan Graefe, Z3JhZWZlQGlnemV2LmRl