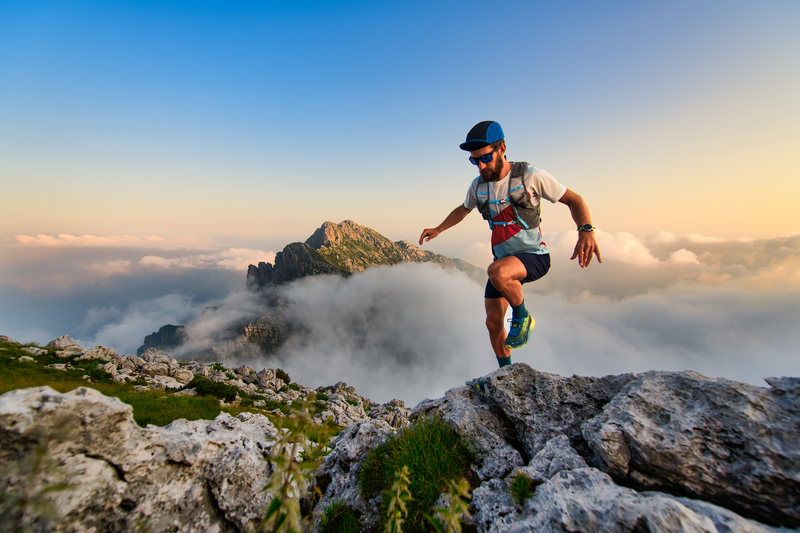
95% of researchers rate our articles as excellent or good
Learn more about the work of our research integrity team to safeguard the quality of each article we publish.
Find out more
ORIGINAL RESEARCH article
Front. Plant Sci. , 25 April 2022
Sec. Plant Abiotic Stress
Volume 13 - 2022 | https://doi.org/10.3389/fpls.2022.818472
This article is part of the Research Topic Identification and Functional Dissection of Stress-responsive Genes in Cotton View all 26 articles
Abiotic stress tolerance is an intricate feature controlled through several genes and networks in the plant system. In abiotic stress, salt, and drought are well known to limit cotton productivity. Transcriptomics meta-analysis has arisen as a robust method to unravel the stress-responsive molecular network in crops. In order to understand drought and salt stress tolerance mechanisms, a meta-analysis of transcriptome studies is crucial. To confront these issues, here, we have given details of genes and networks associated with significant differential expression in response to salt and drought stress. The key regulatory hub genes of drought and salt stress conditions have notable associations with functional drought and salt stress-responsive (DSSR) genes. In the network study, nodulation signaling pathways 2 (NSP2), Dehydration-responsive element1 D (DRE1D), ethylene response factor (ERF61), cycling DOF factor 1 (CDF1), and tubby like protein 3 (TLP3) genes in drought and tubby like protein 1 (TLP1), thaumatin-like proteins (TLP), ethylene-responsive transcription factor ERF109 (EF109), ETS-Related transcription Factor (ELF4), and Arabidopsis thaliana homeodomain leucine-zipper gene (ATHB7) genes in salt showed the significant putative functions and pathways related to providing tolerance against drought and salt stress conditions along with the significant expression values. These outcomes provide potential candidate genes for further in-depth functional studies in cotton, which could be useful for the selection of an improved genotype of Gossypium hirsutum against drought and salt stress conditions.
Gossypium (cotton) species is an excellent model plant system for polyploid plant study (Rong et al., 2005). The Gossypium genus comprises six tetraploids species and 45 diploids (Hawkins et al., 2006; Grover et al., 2015). Approximately two million years ago (MYA), allotetraploid species (Gossypium hirsutum and Gossypium barbadense) were formed from the interspecific hybridization events between Gossypium herbaceum (A1) or Gossypium arboreum (A2) and Gossypium raimondii (D5) or Gossypium gossypioides (D6) followed by polyploidization (Senchina et al., 2003). However, all the polyploid genomes are conserved in terms of gene content but they diversified at the subgenomic level (Chen et al., 2020). The most widely available commercial species of cotton is upland cotton (G. hirsutum) because it provides >90% production of world cotton while G. barbadense gives 3–4% and G. herbaceum and G. arboreum give 2% (Chen et al., 2007). G. hirsutum is the most crucial fiber-producing crop in the world and is grown in more than 80 countries.
Globally, India is the major producer of cotton, although yield productions are low due to crops suffering from one or more abiotic stress factors, such as salt, drought, heat, and waterlogging (Manikandan et al., 2020). Abiotic stresses are a prime factor for limiting plant growth and yield productivity due to constant climate change. Understanding the molecular aspects in plants with response to abiotic stresses is at primacy now (Ambrosino et al., 2020). These responses of plants are crucial to deal with environmental stresses in order to survive. The stress signal passes through the external environment, which continues to move toward the cell to trigger adaptive responses. Among the abiotic stress, drought and salinity show the most acute abiotic stress problems in the production of cotton in subtropic and tropic areas (Abdelraheem et al., 2021). Drought stress majorly affects plant growth and causes morphological, physiochemical, and physiological changes in plants, which hamper plant growth and productivity (Chandra et al., 2021), and salinity of soil also causes significant loss in the production of cotton (Manikandan et al., 2020). Both drought and salt stress have negative impacts in all the stages of growth and can reduce cotton yield by up to 50–67% (Dabbert and Gore, 2014; Ullah et al., 2017; Abdelraheem et al., 2019, 2021). Thus, an understanding of the genomic level of cotton’s response to various environmental stresses would give details on how to improve its genetic makeup with increased resistance to salt and drought stress. The availability of a large amount of transcriptome dataset deposition in Sequence Read Archive (SRA) provides an opportunity to accomplish a comprehensive gene network study (Liao et al., 2003). Understanding these gene networks in the biological insights regarding abiotic stress response in plants is quite challenging (Hartwell et al., 1999; Cramer et al., 2011; Todaka et al., 2012).
For gene regulation and construction of gene networks, transcriptome data is important for functional annotation and discovery of putative genes (Reményi et al., 2004; Ma et al., 2009). Using the genes that were expressed in transcriptome data, gene networks are built based on similarity (correlation) in gene expression (coexpression). The co-expression networks (CNs) of candidate genes help in the study of expression patterns across multiple stress conditions among a large number of genes (Merico et al., 2009; Serin et al., 2016). The Pearson correlation coefficient (PCC) calculated for the similarity score of CNs from genes with significant expression levels, such as sets of genes that have immensely correlated scores tend to group a small module. These correlated genes could be involved in associated signaling pathways and similar functions. Therefore, unknown or poorly characterized co-expressed genes in a module can be predicted by using genes of known function in certain biological processes (Mochida et al., 2011; Rhee and Mutwil, 2014). In CNs of genes, transcription factor hubs are possible contenders for gene regulatory function. Transcription factors help to suggest the co-regulation of subsets of genes that are present in the same co-functionality or signal transduction pathways during the biological processes. At the transcriptional level, co-expression of genes relies upon the promoter region with similar cis-regulatory elements. So, co-expression profiles of genes are integrated into cis-motifs in the respective promoter sequences of a module, which give insights into the regulation of coordinated genes in CNs (Ma and Bohnert, 2007; Todaka et al., 2012; Sharma et al., 2013; Wilkins et al., 2016).
High-throughput comprehensive analysis of expressed genes has become the important approach for showing the putative genes, finding cis-regulatory motifs, predicting gene function, and describing gene regulatory networks (Goda et al., 2008; Brady and Provart, 2009; Mochida et al., 2011). Due to the availability of large-scale transcriptome data in the public domain, we have been able to construct biological networks and pathways. Much effort has been done to make use of co-expression analysis of transcriptome data. These studies used several approaches to find genes and predict the function of genes (Aoki et al., 2007). Later, these pathways and networks have been used to understand functional interaction amongst genes (Moreno-Risueno et al., 2010).
The large-scale availability of transcriptome data in NCBI has given us the chance to build a co-expression network for Gossypium transcriptome in different abiotic stress conditions. These networks might be used to determine the genes that have a significant role in salt and drought stress conditions in specific gene regulatory networks. In this study, we analyzed all the available transcriptome data in NCBI related to salt and drought stress till January 2021 in different tissues and cultivars. For the construction of the co-expression network of cotton, PCC was calculated for drought and salt stress conditions in different tissues (root, leaf, and seed) along with biological processes. Moreover, hub genes were identified from co-expressed modules and also retrieved their functional enrichment and pathways and these identified hub genes were also characterized through expression profiles. These investigations showed that a co-expression module study enables to find the putative genes that could be suitable targets for crop improvement approaches aimed to protect the cotton from drought and salt stress conditions.
A total of 118 (SRA accession number = SRP242577, SRP043419, SRP228010, SRP157859, and SRP192537) and 33 (SRA accession number = SRP192537 and SRP166405) RNA-seq datasets of treated and untreated cotton samples were retrieved from the NCBI SRA database till January 2021 using specific keywords “G. hirsutum” and individual abiotic stress. i.e., “salinity and drought.” These datasets were extracted from the leaf, root, and seed tissues, and 4 cultivars (TM1, TM2, H15, and ZM12) in salinity and drought stress datasets were extracted from the leaf and root tissue that includes TM1 culture.
The parameters for the dataset taken into consideration for further study were the experiments which were conducted for drought and salinity treatment of cotton with at least two replicates (Walia et al., 2005, 2007, 2009; Jain et al., 2007; Hu et al., 2009; Mustroph et al., 2010). In total, our final meta-datasets comprised 151 RNA-seq representing 33 droughts and 118 salt-treated in 7 experiments as summarized in Supplementary Table 1. The quality control of these raw fastq datasets was processed by the Trimmomatic tool (Bolger et al., 2014) with average quality of 20 per base and adapter trimming was also done where it was required. Filtered reads were mapped on the G. hirsutum genome (Wang M. et al., 2019) by using a STAR aligner (Dobin et al., 2013) with the default parameter. The quantification of differentially expressed genes (DEGs) was done through cuffdiff in Cufflinks version 2.2.1 software (Trapnell et al., 2012) with p-values ≤ 0.05, and log2fold-change (log2FC) values ≥ 2 for upregulated and ≤ −2 for downregulated genes. The top-ranked significant DEGs were compared in different tissue under drought and salt stress conditions. Unbiased clustering of drought and salt stress-responsive (DSSR) genes from different tissues with their log2FC were displayed through heatmap using the “Pheatmap” package function in R with normalized scale and euclidean distance method.
Drought and salt stress-responsive genes were selected based on their differential expression in several experiments. Each tissue with higher differential expression was considered a reference in DSSR-GCN (gene co-expression network). The DSSR-GCN was constructed by the FPKM along with < 0.05 p-values. The ‘‘expression correlation networks’’ module in Cytoscape version 3.81 (Smoot et al., 2011) was used to construct the network. This module calculated a positive PCC (r ≥ 0.95) among the interacting members of the network. The co-expressed genes were visualized through Cytoscape.
To identify functionally grouped GO and pathway, the ClueGo module in Cytoscape was used (Bindea et al., 2009). Using ClueGo, a biological process enrichment analysis of co-expressed genes in up and downregulated genes was performed in root and leaf tissue of drought and root, leaf, and seed tissues of salt stress datasets. Moreover, common enriched pathways between drought and salt were identified and represented through the EnrichmentMap module in Cytoscape. Node color shows the normalized enrichment score (NES) from the core enrichment genes. A positive score (red) shows gene set enrichment at the top of the ranked list a negative score (blue) indicates gene set enrichment at the bottom of the ranked list. The color of the border represents the p-value of the pathway enrichment from gene set enrichment analysis (GSEA). The node size shows the number (setSize) of DEGs identified in the pathways.
In a co-expression network, each edge shows an interaction between genes and the node shows a gene. We have estimated closeness connectivity, betweenness centrality (Cb), eccentricity, and degree centrality (k) using the network analyzer (Doncheva et al., 2012) plugin in Cytoscape software. Identification of hub genes was done based on connection or degree or they were shared in DSSR-GCN. Highly connected genes that have a high correlation with other genes in the network are known as hub genes. Any alteration in hub genes expression could lead to affect the major part of the network. Rapid information can be transferred in the network by the genes that have high connectivity compared to the other genes. Significantly highly expressed top-ranked genes that have a higher betweenness centrality value and degree of connectivity (k) were taken as hub genes.
Each node is allotted by a specific value based on links held through it, known as degree centrality (k). The degree helps in estimating the significance of node to control the network and it demonstrates the number of interactions retrained through a node in the network with other nodes.
Where Ku denotes the node-set comprising entire neighbors of node u, and w (u,v) indicates the edge weight which connects node v with node u.
The betweenness centrality describes how often a node falls on the shortest path with other nodes in its neighborhood.
Betweenness centrality (Cb) measures how many times a node falls on the shortest path with other neighboring nodes. It refers to the ability of a node to control information flow and signal processing in the network.
Where, p (k,u,f) represents the number of interactions from node k to node f that pass through node u, whereas p (k,f) shows the total number of shortest interactions between nodes k and f (Prasad et al., 2020).
For extensively analyzing the functional annotations of DEGs, we used the GO analysis toolkit for the agricultural community (agriGO version 2) (Tian et al., 2017). The gene ontology provided the annotations at the cellular level, molecular level, and biological level. The GO graph was generated in RStudio using the ggplot package. Further, the functions of DSSR hub genes were confirmed through the GeneMANIA server (Warde-Farley et al., 2010), which predicts the functions of gene sets. The functional study was performed at a 0.05 p-value cutoff, with the Arabidopsis thaliana database as a reference. For KEGG pathways analysis was employed using clusterProfiler package in RStudio with the number of permutation (nPerm) set to 10,000, org.At.tair.db and pAjustMethod set to “none.” P-value ≤ 0.05 was considered for KEGG pathways. The PlantCARE database (Lescot et al., 2002) was searched for cis-regulatory elements in the 2 kb sequences upstream of identified hub genes identified by using the “Signal Scan Search” program.
To check the amplification of the key hub genes of cotton (G. hirsutum), plants were grown in the growth chamber under a controlled temperature of 30–37°C with normal photoperiodic conditions. The seedlings were grown in separate triplicate pots for 1 month, stress treatment was given with, 20% PEG 8,000 (approx 300 ml/kg of soil) in the pots for the drought stress (Patade et al., 2009) and NaCl (300-mM) for salt stress (Li et al., 2017). One time stress treatment was provided to each pot and then after intervals of 12, 24, 48, and 72 h RNA was isolated following the protocol (spectrum™ Plant Total RNA Isolation Kit). Once the RNA was isolated, cDNA was prepared (1 μg/μl) with verso cDNA synthesis kit (Thermo scientific). The amplification of the four putative genes for drought and four genes for salt stress was designed with primer3 Plus and checked with Quantitative Real-Time PCR (qRT-PCR) (HiMedia Insta Q 48 M4). The reaction was prepared with 10 μl of fast the SYBER™ green master mix (Applied Biosystem) and 1 μl of 100 ng cDNA adding 5 picomoles (forward and reverse) primers in a total 20 μl reaction. Ubiquitin was taken as internal control and the program for qRT-PCR was set at 95?C for 2 min, followed by cycling for 40 cycles of denaturation at 95°C for 15 s, annealing at 60 C for 1 min, and extension at 72 C for 30 s. Relative expression of the employed genes was calculated with mean ± SD of biological triplicate samples by the 2^–ΔΔct method (Livak and Schmittgen, 2001).
The statistical analysis of qRT-PCR was carried out, using GraphPad Prism version 8.4.3 software, with two-tailed Student’s t-test in triplicate sample repeats.
A total of 2 SRA accessions (SRP166405 and SRP192537) of drought and 5 SRA accessions (SRP242577, SRP043419, SRP228010, SRP157859, and SRP192537) of salt containing 33 and 118 fastq files were processed through the Trimmomatic tool. These RNA-Seq data included root, leaf, and seed of different cultivars. As a result, 2,168,052,606 clean reads with an average length of 277 bp were produced in salt stress data and 776,795,545 clean reads with an average length of 293 bp were generated in drought stress after removing the adapter sequences and low-quality reads (Table 1). The clean reads were then mapped to the G. hirsutum (cotton) genome, 1,960,725,723 (90.43%) reads in salt (Supplementary Table 1A) and 722984665 (93.06%) reads in drought (Supplementary Table 1B) were mapped to the reference cotton genome.
Table 1a. Top 86 hub genes based on the topological properties in co-expression network of DEGs in drought stress condition, and arranged on the basis of the decreasing degree of connectivity value.
Table 1b. Top 61 hub genes based on the topological properties in co-expression network of DEGs in salt stress condition, and arranged on the basis of the decreasing degree of connectivity value.
Further, 5,962 and 3,510 differentially expressed genes (DEGs) were identified in drought and salt stress data. Total 3,132 and 2,830 up and downregulated genes in drought and 2,265 and 1,245 up and downregulated genes (P-value < 0.05 and log2fold change > 2) in salt stress data. To make the summary of DSSR genes, which were expressed exclusively in drought or salt, DEGs were compared and found that 3,841 were unique in drought and 1,508 were unique in salt stress data (Figure 1). The unbiased clustering of all significant DEGs of drought (leaf and root) and salt (leaf, root, and seed) stress-responsive genes from different tissues with their expression values (log2FC) have shown through heatmap (Supplementary Figure 1) and co-existed differentially expressed genes among drought root, salt root, drought leaf, salt leaf, and salt seed have been presented in Supplementary Figure 2.
Figure 1. Venn diagram showing the number of shared and unique genes in differentially expressed genes (DEGs) of drought and salt stress data. In order to make drought and salt stress-responsive (DSSR) genes set compendium, we focused on 3,841 and 1,508 DSSR uniquely differentially expressed genes in drought and salt.
To build the cotton-DSSR gene network, we have used the analyzed transcriptome data of cotton in two abiotic stress (drought and salt) conditions in different tissues. The protein-protein interaction network was constructed in a circular layout after co-expression calculation using the Expression Correlation module in the Cytoscape tool. Further, among the analyzed transcriptome data, the top 100 DEGs genes with FPKM values and significant p-value (< 0.05) were selected from each tissue for further co-expression network analysis using the PCC method. Based on correlation score calculation drought-responsive gene co-expression network (GCN) was generated which comprised 100 nodes connected by 1,704 and 2,747 co-expressed edges with minimum network density (ND) 0.34 and 0.55 in up- and downregulated genes of leaf tissue at PCC ≥ 0.95. Similarly, drought-responsive GCN of root tissue was generated which comprised 100 nodes connected by 1,505 and 2,314 co-expressed edges with minimum ND 0.30 and 0.46 in up and downregulated genes. Moreover, the salt responsive GCN of leaf tissue was generated which comprised 98 and 9 nodes connected by 4,565 and 45 co-expressed edges in up and downregulated genes, whereas 100 nodes connected with 3,252 and 2,604 co-expressed edges with minimum ND 0.65 and 0.52 in up- and down-regulated genes of salt root tissue. Similarly, salt responsive GCN of seed tissue was generated which comprised 27 and 74 nodes connected with 351 and 2,701 co-expressed edges in up and downregulated genes. These co-expressed genes were further used for network analysis of significant DEGs for drought and salt stress conditions. For this we have estimated closeness connectivity, betweenness centrality (Cb), eccentricity, and degree centrality (k) using network analyzer plugin in Cytoscape software and circular layout obtained network for drought (Figures 2A,C) and salt stress dataset at different tissue levels (Figures 3A,C,E). The genes are ranked based on betweenness centrality scores and degree connectivity in drought (Supplementary Table 2A) and salt stress dataset (Supplementary Table 2B). Among these, SBT, EXPB2, CHS, TLP3, and CML44 have a high degree and betweenness centrality value in the drought stress dataset while WRKY6, ELF4, TLP, EXLB1, AATP1, and PER53 comprises high degree and betweenness centrality values in the salt stress.
Figure 2. The drought-responsive gene network analysis of the top 100 upregulated (A,C) genes with their biological processes (B,D) in leaf and root tissues. Scales are showing from lower to higher degree connectivity.
Figure 3. The salt responsive genes network analysis of the top 100 upregulated (A,C,E) genes with their biological processes (B,D,F) in leaf, root, and seed tissues. Scales are showing from lower to higher degree connectivity.
To acquire an in-depth understanding of the drought and salt stress-responsive gene network, biological processes analysis has been applied on top selected differentially expressed genes using the ClueGo module with a p-value of 0.05 plugin in Cytoscape. The biological functions in drought stress conditions were shown in Figures 2B,D and salt stress conditions were shown in Figures 3B,D,F in different tissue levels. Their detailed functional enrichments are shown in Supplementary Table 3A for drought and Supplementary Table 3B for salt datasets. In drought stress, the interacting genes in biological process (BP) were mainly enriched in the response to water deprivation, cellular response to abiotic stimulus, flavonoid biosynthetic process, phenylpropanoid metabolic process, response to jasmonic acid, and trehalose biosynthetic process in upregulated (Figure 2B) genes of leaf tissue while, flavonoid biosynthetic process, response to decreased oxygen levels, response to hydrogen peroxide and response to water deprivation in upregulated (Figure 2D) genes of root tissue. Moreover, in salt stress condition, upregulated BP were enriched with cellular response to abiotic stimulus, flavonoid metabolic process, isoprenoid metabolic process, hyperosmotic salinity response, response to abscisic acid, response to decreased oxygen levels, response to extracellular stimulus, and response to jasmonic acid in root tissue (Figure 3B) while regulation of secondary metabolite biosynthetic process, induced systemic resistance, oxylipin biosynthetic process and cellular response to boron-containing substance levels were upregulated BP in leaf tissue (Figure 2D). Moreover, upregulated BP in seed tissue were malate metabolic process peptidyl-threonine phosphorylation, basipetalauxin transport, peptidyl-threonine phosphorylation, and maintenance of protein location in the cell (Figure 3F). The co-expression network and their functional enrichment study highlighted the key role of these putative genes in drought and salt stress responses in both (leaf and root) tissues except seed. Therefore seed tissue was dropped from further in-depth studies.
The gene Ontology is used to assign putative functions to all identified DEGs into three categories: biological processes (BP), cellular components (CC), and molecular functions (MF). Significantly differentially expressed genes were taken for further functional and biological pathways investigation. In drought stress conditions, gene ontology analysis in both the tissues (leaf and root) showed a significant presence of peroxidase activity, carboxylesterase, and petinesterase activity in molecular functions (MF). Biological processes in drought were enriched with response to abiotic stimulus, response to stress, and defense response (Supplementary Table 4A). Similarly, in salt stress conditions, both tissues (leaf, and root) showed the significant presence of lipoxygenase activity, oxidoreductase activity, and cytokinin dehydrogenase activity, and phosphoric ester hydrolase activity in MF. BP in salt was enriched with the carboxylic acid biosynthetic process, response to stress, response to abiotic stimulus, response to oxidative stress, and cell redox homeostasis (Supplementary Table 4B). In cellular component, apoplast, cell wall, and extracellular region were enriched in drought and cell wall, extracellular matrix, apoplast, cell cortex, and oxygen-evolving complex were enriched in salt stress data.
Overall, the Kyoto Encyclopedia of Genes and Genomes (KEGG) pathways enrichment analysis reveals the role of drought stress response in Ubiquinone and other terpenoid-quinone biosynthesis and MAPK signaling pathways (Supplementary Table 5A) and salt stress responses in phenylpropanoid biosynthesis and Isoquinoline alkaloid biosynthesis (Supplementary Table 5B). Based on significant log2fold change (≤ -2 or ≥ 2) and p-value ≤ 0.05, TLP3, TLP5, TLP6, TLP, NCED1, EXLB1, PBS1, PCL1, BT2, TAR4, ITN1, ORG2, WRK54, and CAX3 genes were highly upregulated in drought stress condition and TLP1, TLP, GSTXA, NCS2, AKR1, LAC14, PDR1, ERD10, INV1, HSP70, DOX1, AOC, GPAT3, ATHB7, PER1, ELF4, EXLB1, RAV1, PER30, and CIPK6 were highly upregulated in salt stress condition. Overall, this study highlights the key role of these pathways in drought and salt stress responses. Additionally, the common differentially expressed genes (DEGs) were identified in both drought and salt stress conditions, and details of significant DEGs are listed in Supplementary Table 6. By utilizing these genes the common enriched pathways were identified and represented through Cytoscape using the EnrichmentMap module (Figure 4). Biosynthesis of secondary metabolites, metabolic pathways, tryptophan metabolism, MAPK signaling pathways, protein processing in the endoplasmic reticulum, and phenylpropanoid biosynthesis pathways were commonly found in both drought and salt datasets.
Figure 4. Common enriched pathways in drought and salt dataset. Node color shows the normalized enrichment score (NES) from the core enrichment genes. A positive score (red) shows gene set enrichment at the top of the ranked list, and a negative score (blue) indicates gene set enrichment at the bottom of the ranked list. The color of the border represents the p-value of the enriched pathway.
In the co-expression network, the genes that have a high correlation with other genes are known as hub genes. Therefore, a topological feature of a network can be used to know the specific drought and salt stress-responsive genes. Using Cytoscape’s Network analyzer tool, candidate hub nodes were determined using two topological features, betweenness (the fraction of all shortest paths that include a node within a network) and degree (number of connections) calculation. Altogether, we have identified 86 and 61 nodes with a high degree of connectivity and betweenness value and were subsequently referred to as hub genes in the network in drought (Table 1A) and salt stress conditions (Table 1B).
A functional enrichment study of 86 and 61 putative drought and salt stress-responsive hub genes was performed by using GeneMania (GM) webserver and GO analysis. Through the GM webserver, we also predicted interactions between hub genes in the network using Arabidopsis thaliana as an additional parameter. The GM outcomes showed their role in response to water, oxidoreductase activity, acting on single donors with incorporation of molecular oxygen, isoprenoid metabolic process, dioxygenase activity, terpenoid biosynthetic process, isoprenoid biosynthetic process, and abscisic acid metabolic process in drought stress conditions (Figure 5A) while response to hypoxia, cellular response to decreased oxygen levels, cellular response to oxygen levels, response to water and aging in salt stress condition (Figure 5B). Furthermore, to confirm the function of GM, gene ontology classification has also been done. The GO analysis showed their role in response to stress, response to stimulus, response to abiotic stimulus, response to abscisic acid, and oxidoreductase activity in drought (Figure 6A). The highest number of genes were involved in response to stimulus, then in response to salt stress, catalytic activity and response to endogenous stimulus in salt stress condition (Figure 6B).
Figure 5. Gene network of hub genes derived from GeneMANIA along with functional enrichment in (A) drought and (B) salt stress conditions.
Figure 6. Gene ontology (GO) classification of hub genes in (A) drought (B) salt stress condition. The x-axis represents significant P-values. The y-axis denotes the enriched functions. Bubble size denotes the number of DEGs enriched in the putative functions.
The KEGG pathways analysis demonstrated the role of drought stress response in phenylpropanoid biosynthesis, biosynthesis of secondary metabolites and diterpenoid biosynthesis, and sulfur metabolism pathways in which the highest number of genes are involved in the biosynthesis of secondary metabolites (Figure 7A) and salt stress, the higher the number of genes is involved in metabolic pathways than in phenylpropanoid biosynthesis and pyruvate metabolism (Figure 7B).
Figure 7. Advanced bubble chart shows putative KEGG pathways in (A) drought (B) salt stress conditions. The x-axis represents GeneRatio, which is the ratio of the number of DEGs and all annotated genes. The y-axis denotes the enriched pathways. Enrichment significance is shown with color and bubble size denotes the number of DEGs enriched in the pathway.
Based on degree centrality cutoff ≥ 30 and significant differential values (log2FC ≥ 2 or ≤ −2 with p-value ≤ 0.05) the expression profiles were illustrated for identified hub genes in both drought and salt stress conditions. The expression pattern revealed that NSP2, CDF1, DRE1D, ERF61, P2C08, HFB2A, TLP3, MYB15, ORG2, and TLP5 genes have significantly higher expression in drought stress conditions (Figure 8A). All the drought-responsive genes were majorly clustered in four groups, three of them showing upregulated genes, in which the TLP5 gene does not cluster with another gene. Moreover, TLP1, TLP, P2C08, EF109, ATHB7, ELF4, bHLH25, DRE1D, WRKY6, bHLH126, and NAC83 genes were expressed significantly higher in salt stress (Figure 8B), in which Tubby-like protein 1 (TLP1) showed the highest expression (>10-fold) among the identified genes also making the separate cluster from other. These outcomes suggested that TLP might have some specific role in drought and salt stress conditions.
Figure 8. Expression profiles of putative hub genes under (A) drought and (B) salt stress conditions. These expressions have been shown with the pheatmap package in R using Log2FC values.
Further, to validate the putative hub genes of G. hirsutum in response to drought and salt stresses, the qRT-PCR validation of four key genes in drought (NSP2, DRE1D, TLP5, and TLP3) and four in salt (TLP1, WRKY6, ATHB7, and EF109) stress conditions was carried out. The corresponding primers are listed in Table 2. The expression of four putative genes was significantly upregulated in salt-stressed conditions, where the majority of the genes showed higher responses at 12, 24, and 72 h (Figures 9A–D) in terms of fold change (FC). TLP1 showed the highest expression at 24 h (>23 FC) and 12 h (>14 FC) compared with control (Figure 9A). Moreover, WRKY6 (>5 FC) and EF109 (>10 FC) also showed the highest expression at 12 h and 24 h compared with control (Figures 9B,D) whereas ATHB7 showed the highest expression at 12 h (>12 FC) in comparison to control (Figure 9C). Likewise, the expression of four putative genes was also upregulated in drought-stressed conditions, where most of the genes showed higher expression at 12 and 72 h (Figures 9E,F), where NSP2 showed the highest expression at 24 h (>16 FC) and 72 h (>29 FC) (Figure 9E) and DRE1D, TLP5, and TLP3 showed relatively higher expression at 12 and 72 h compared with control (Figures 9F–H). The correlation analysis of key drought (NSP2, DRE1D, TLP5, and TLP3) and salt (TLP1, WRKY6, ATHB7, and EF109) stress-responsive hub genes also represented a higher positive correlation between transcriptome and qRT-PCR dataset (Figures 9I,J). Altogether, the differential responses of these putative hub genes in salt and drought stresses suggest that these genes may confer to resist the abiotic stresses in cotton.
Figure 9. The expression pattern of key hub genes in G. hirsutum. qRT-PCR expression pattern of four putative genes in normal (0 h), 12, 24, 48, and 72 h of salt (300 MM) (A–D) and drought (20% PEG solutions PEG8000) stress (E–H) in cotton. Ubiquitin was used as the loading control. Three biological replicates were used for each experiment. The statistical analysis was performed, using two-tailed Student’s t-test. The data are plotted as means ± SD. The error bars represent standard deviations. (I,J) Correlation analysis against the expression values of qRT-PCR and RNA-Seq data in drought and salt stress conditions. The R represents the Pearson correlation coefficient, respectively. Graph showing a significant positive correlation between qRT-PCR and RNA-Seq data values.
The functions of highly expressed hub genes in the expression profiles were further confirmed through cis-regulatory elements analysis. We identified the binding frequency of cis-regulatory elements in 2,000 kb upstream sequences. In drought stress, TLP5, NSP2, DRE1D, NAC29, TLP3, and HFA6B comprises the higher number of abiotic stress-responsive elements (Table 3A), and EF109, MIP1B, ATHB7, and TLP contains the higher number of abiotic stress-responsive elements in salt stress (Table 3B). The upstream region of hub genes showed the abundance of MBS (MYB binding site involved in drought-inducibility) in drought stress conditions (Figure 10A), whereas ABRE (cis-acting element involved in the abscisic acid responsiveness) proximal elements were dominant in salt stress conditions (Figure 10B).
Table 3a. Cis-regulatory elements of identified hub genes in (A) drought and (B) salt stress condition.Cis-regulatory elements of identified hub genes in drought stress condition.
Figure 10. Illustration of cis-regulatory elements of putative hub genes in (A) drought and (B) salt conditions. The micro-parts in diverse colors are the sequence of the putative elements.
Cotton is a very important fiber-producing crop that is being cultivated across the globe. It is grown in semi-arid and arid regions where abiotic stresses namely salt and drought are highly prevalent due to which yields get affected. Among these, scarcity of water is a major limitation for the production of the crop. Water deficit conditions can severely lessen the crop yield and adversely influence the biochemical and physiological processes of plants which further lead to the lint yield reduction. The severity of the drought depends on various factors such as moisture storing ability of soils, rainfall amount and distribution, and evaporative demands. In cotton, the growth of the cell is affected by the reduction in turgor pressure due to drought stress. Drought also affects carbohydrate metabolism and photosynthesis directly or indirectly, changes in photosynthesis lead to the reduction of the boll maintenance in cotton (Leakey et al., 2009). The important physiological defense mechanisms to cope with drought stress conditions are root development, stomata closure, photosynthesis, cellular adaptations, JA and ABA hormone production and ROS scavenging have been determined in cotton (Ullah et al., 2017). Apart from water scarcity, soil salinity also gives rise to significant loss (5–9%) of cotton production (Nirmala et al., 2012). Several defense mechanisms such as hormone regulation, membrane stability maintenance, antioxidants generation, and stress proteins induction, and carbon fixation rate have been found to be involved in plant survival under moisture stress conditions. Drought and salt stress conditions enhance the expression level of stress-related transcription factors and genes which further induces several droughts and salt stress-related pathways to stimulate the tolerance in the plant. There are several transcription factors such as WRKY, NAC, MYB, bHLH, ERF, ORG2, DREB, and TLP which have an important role in DSSR. To know the highly putative genes in drought and stress responses in cotton, comprehensive cotton-DSSR hub genes, and their functional enrichment studies were performed. The overall flow chart and strategy for designing and analyzing drought and salt stress-responsive gene co-expression networks (Figure 11).
Figure 11. The overall flow chart and strategy for designing and analyzing drought and salt stress-responsive genes.
By using the transcriptome (RNA-Seq) dataset of different tissues of cotton (G. hirsutum) in drought and salt stress conditions, the cotton-DSSR network study was done. Firstly, the GCN with the PCC method was constructed. Further, these GCN were taken for estimating the closeness connectivity, betweenness centrality (Cb), eccentricity, and degree centrality (k) using a network analyzer plugin with a circular layout. Furthermore, the genes are ranked based on betweenness centrality scores and degree connectivity (Figures 3, 4). In drought, SBT, EXPB2, CHS, TLP3, and CML44 genes and in salt stress conditions, WRKY6, ELF4, TLP, EXLB1, AATP1, and PER53 genes were found with a higher degree of connectivity and betweenness centrality scores. Previously, it has been reported that phytosulfokine (PSK) precursor processing by subtilase SBT signaling improves drought stress tolerance in Arabidopsis (Stuhrwohldt et al., 2021), expression of expansin-like B2 (EXPB2) enhanced for cell wall extensibility during drought stress conditions in Solanum pennellii (Egea et al., 2018). Overexpression of Chalcone synthase (CHS) increases the production of flavonoids, which served as antioxidants in response to different stresses, namely drought, salt, and heavy metal (Chen et al., 2015). In Arabidopsis, TLP3 was found to function in response to ABA (Bao et al., 2014) and overexpression of calmodulin-like (CML44) stress-responsive gene from Solanum habrochaites improves tolerance to various abiotic stresses (Munir et al., 2016). These genes (SBT, EXPB2, CHS, TLP3, and CML44) were also detected in drought stress conditions. Therefore, putative drought-responsive genes might be crucial for drought stress responses. In salt stress conditions, the WRKY6 expression was found to be enhanced in response to ROS treatment and it also triggers other plant defense responses and senescence processes (Robatzek and Somssich, 2001; Li et al., 2020). The member of EARLY FLOWERING3 (ELF) have an important role in providing tolerant to high NaCl (Sakuraba et al., 2017; Cheng et al., 2020) and Tubby-like protein (TLP) members have been identified to play a crucial role in several abiotic responses such as drought, salt, oxidative (Wardhan et al., 2012; Xu et al., 2016; Li Z. S. et al., 2021). Furthermore, the expression of expansin-like B1 (EXPB1) was found to be upregulated by salt, oxidative, and osmotic conditions Brassica rapa (Muthusamy et al., 2020). The involvement of these genes (WRKY6, ELF, TLP, and EXLB1) demonstrated an imperative role in salt stress, and these genes, which were also detected in salt stress data, could therefore play an important role in salt stress. For an in-depth understanding of the DSSR network, the biological processes analysis of putative co-expressed responsive genes (Figures 2A,C,E) was carried out with the CluoGo module with a significant p-value. These results demonstrated response to water deprivation, cellular response to abiotic stimulus, flavonoid biosynthetic process, phenylpropanoid metabolic process, response to jasmonic acid and trehalose biosynthesis (Figure 2B) biological process in upregulated genes of leaf tissue while, flavonoid biosynthetic process, response to decreased oxygen levels, response to hydrogen peroxide and response to water deprivation (Figure 2D) biological process in upregulated genes of root tissue in drought stress condition. It has been reported that by regulating the homeostasis of ROS, flavonoids improve drought tolerance of maize seedlings (Li B. Z. et al., 2021) and regulation of phenylpropanoid biosynthesis contributes to drought resistance in apple roots by MYB88 and MYB124, responsible for lignin accumulation under stress conditions (Geng et al., 2020). Moreover, the accumulation of jasmonic acid is required for abscisic acid biosynthesis under drought stress conditions in citrus roots (de Ollas et al., 2013) and enhanced trehalose biosynthesis improves yield production under drought stress so there is a positive correlation between high-yield parameters and trehalose overproduction (Joshi et al., 2020). The outcomes of biological processes in salt stress conditions showed cellular response to abiotic stimulus, flavonoid metabolic process, isoprenoid metabolic process, hyperosmotic salinity response, response to abscisic acid, response to decreased oxygen levels, response to extracellular stimulus, and response to jasmonic acid in upregulated genes of root tissue (Figure 3B) whereas, regulation of secondary metabolite biosynthetic process, induced systemic resistance, oxylipin biosynthetic process and cellular response to boron-containing substance levels were upregulated in leaf tissue (Figure 3D). Seed tissue was enriched with malate metabolic process, peptidyl-threonine phosphorylation, basipetalauxin transport, and maintenance of protein location in cells in upregulated genes (Figure 3F). An earlier report stated that MYB111 is a positive regulator in salt stress response and bioflavonoids addition able to rescue the loss of salt tolerance in myb111 mutants (Li et al., 2019), and salt stress condition also enhances the biosynthesis of isoprenoid (Zuo et al., 2019), indicating their importance in salt stress condition. Furthermore, oxylipin biosynthesis provides adaptation to plants under salt stress conditions (Savchenko et al., 2014) and TCA cycle intermediates (malate, α-ketoglutarate, aconitate, isocitrate, citrate, and succinate) enhanced during salt stress exposure in the barley cultivar Sahara (salt-sensitive) (Bandehagh and Taylor, 2020). These overall outcomes suggest that high-ranked genes and biological processes of co-expressed networks play a crucial role in drought and salt stress responses at both (root and leaf) tissue levels.
Significantly differentially expressed genes were taken for further biological pathways investigation. The KEGG pathways study revealed the role of interacting genes in Ubiquinone and other terpenoid-quinone biosynthesis and MAPK signaling pathways in drought stress response (Supplementary Table 5A) and phenylpropanoid biosynthesis and Isoquinoline alkaloid biosynthesis in salt stress response (Supplementary Table 5B). Ubiquinone (UQ) is an important prenylquinones that acts as an antioxidant, participates in a plant’s response to stress, and regulates gene expression and cell signal transduction (Liu and Lu, 2016). The expression of genes in terpenoids biosynthesis is affected by drought stress and modulated by transcriptional regulation in trichomes of Nicotiana tabacum (Wang et al., 2021). Moreover, enhanced phenylpropanoid concentrations lead to improving the nutritional quality of wheat sprouts under salt stress conditions (Cuong et al., 2020) and increased alkaloid concentration plays an important role in the adaptation to salt conditions (Shi and Gu, 2020). Overall, the KEGG pathway showed that DSSR genes mainly target secondary metabolites-related pathways to provide tolerance against drought and salt stress conditions. Moreover, the common pathways in both drought and salt stress datasets showed that a higher number of genes significantly involved in the biosynthesis of secondary metabolites, metabolic pathways, phenylpropanoid biosynthesis, and tryptophan metabolism pathways (Figure 4). It is reported that tryptophan is a precursor for melatonin and serotonin biosynthesis (Murch et al., 2000), which have a regulatory role in abiotic stress (drought and salt) (Kaur et al., 2015). From outcomes of common enriched pathways, we can hypothesize that the involved genes might have an important role in providing resistance against drought as well as salt stress conditions in cotton.
Based on two topological features, betweenness (the fraction of all shortest paths that include a node within a network) and degree (the number of connections), candidate hub genes were determined using the network analyzer tool. A total of 86 and 61 hub genes were identified with a high degree of connectivity and betweenness value in drought (Table 1A) and salt stress conditions (Table 1B). These identified genes mostly belong to the family of secondary metabolites responsive genes (SMRGs). SMRGs including MYB15 (Marchev et al., 2020), NAC29 (Huang et al., 2015; Wang T. T. et al., 2019), TLP3 (Ibrahim and Jaafar, 2013; Bao et al., 2014), HFB2A (Paupiere et al., 2020), WRKY27, OLE16 (Shao et al., 2019), BBE8 (Daniel et al., 2017), LEA3 (Zhao et al., 2011), CHSY (Dao et al., 2011), DRE1D (Agarwal et al., 2017), Malate synthase (de Kraker and Gershenzon, 2011), cycloartenol synthase (Jin et al., 2017) and CASL1 (Taura et al., 2007) in drought enhances secondary metabolite responsive pathways and exert to provide tolerance in the adverse condition in drought stress condition. In salt, several identified genes such as TLP (Zare-Hassani et al., 2019; de Jesus-Pires et al., 2020), WRKY6 (Robatzek and Somssich, 2001; Afrin et al., 2015), MMP-2 (Guo et al., 2019), peroxidase 53 (Novo-Uzal et al., 2014), bHLH25 (Goossens et al., 2019; Meraj et al., 2020; Yang et al., 2020), malate synthase (Brito et al., 2020), carboxylesterase (Wang et al., 2015; Cao et al., 2019), 1-deoxy-D-xylulose-5-phosphate synthase 2 (Paetzold et al., 2010), NAC (He et al., 2019), DCS2 (George et al., 2017), XTH (Choi et al., 2011; Guerriero et al., 2018), AtHB-7 (Olsson et al., 2004), NCED5 (Udomchalothorn et al., 2017), CAS (Shirazi et al., 2019), ERF (Meraj et al., 2020), galactinol synthase (Patel et al., 2020), EXPA7 (Jadamba et al., 2020) and glutathione S-transferase (Nazar et al., 2011; Czerniawski and Bednarek, 2018) are SMRGs exhibited crucial role in providing resistance under salt stress condition. Overall, these putative hub genes might have putative functions in drought and salt stress responses in cotton.
A functional enrichment study of 86 and 61 candidate drought and salt stress-responsive hub genes was performed by using GeneMania (GM) webserver, further their functions were confirmed through gene ontology (GO) classification. The functional enrichment analysis of 86 candidate hub genes in drought stress by GM demonstrated their role in response to water, oxidoreductase activity, acting on single donors with incorporation of molecular oxygen, isoprenoid metabolic process, dioxygenase activity, terpenoid biosynthetic process, and abscisic acid metabolic process (Figure 5A). In rice, oxidoreductase activity implies a positive role in drought tolerance (Huang et al., 2014), in transgenic tobacco, isoprenoid metabolism has an important function during drought (Tattini et al., 2014) and drought stress also affects the gene expression of terpenoids biosynthesis and control its transcriptional regulation in tobacco (Wang et al., 2021). At the molecular level, several physiological processes such as stomatal closure, germination, seed dormancy, and change in gene expression are regulated through ABA, which is a critical hormone, that serves as the core regulator of several stresses (drought, salt, and low temperature) in plants (Ali et al., 2020). These GM functions were confirmed through GO classification, in which molecular functions and biological processes showed similar outcomes such as response to stress, response to stimulus, response to abiotic stimulus, response to abscisic acid, and oxidoreductase activity in drought (Figure 6A). Moreover, in the functional enrichment study of 61 putative hub genes in salt stress conditions, the GM outcomes showed response to hypoxia, cellular response to decreased oxygen levels, cellular response to oxygen levels, response to water, and aging (Figure 5B). GM results revealed the response to decreased oxygen levels and hypoxia, which were caused by several factors such as excess nutrients, phosphorus, water body stratification (layering), and nitrogen, one of the main reasons is saline gradients and also showing the response to water, indicating their important role during salt stress condition. Salt stress-responsive functions of GM were further affirmed through the GO category, the biological process (BP) of GO demonstrating the significant direct role of these candidate genes in salt stress responses also showing significant catalytic activity in molecular function (MF) (Figure 6B). The catalytic activity has been reported to be involved in abiotic stress tolerances by modulating genes (Chin et al., 2019). Overall, a functional enrichment study in drought and salt stress conditions showed a significant critical role in both the stress conditions.
In drought stress, the KEGG pathways are enriched with phenylpropanoid biosynthesis, biosynthesis of secondary metabolites, diterpenoid biosynthesis, and sulfur metabolism pathways (Figure 7A). The phenylpropanoid biosynthetic pathway is triggered under several abiotic stresses such as drought, heavy metals, salinity, high/low temperatures, and ultraviolet radiations, resulting in the accumulation of several phenolic compounds that have the potential to scavenge harmful reactive oxygen species (Sharma et al., 2019). Terpenoid phytoalexins comprise two zealexins and kauralexins families, accumulate in roots in response to both abiotic and biotic stress such as drought, high salinity, Fusarium verticillioides infection, and diabrotica balteata herbivory. In maize roots, the accumulation of terpenoids is associated with drought tolerance (Vaughan et al., 2015). The previous report stated that sulfur not only acts like other macronutrients but is also imperative during metabolic adaptation reactions to drought stress, indicating sulfur metabolism is required in response to drought stress (Abuelsoud et al., 2016; Ahmad et al., 2016). In salt stress, the KEGG pathways are enriched with metabolic pathways, amino sugar metabolism, phenylpropanoid biosynthesis, and pyruvate metabolism (Figure 7B). The expression profile of phenylpropanoid biosynthetic pathway-related genes and phenylpropanoid compounds levels were investigated and found that enhanced phenylpropanoid concentrations lead to improve nutritional quality of wheat sprouts in the presence of salt treatment (Cuong et al., 2020) and there is a positive correlation of pyruvate pathways under salinity stress condition, as pyruvate pathways get activated under salt stress condition (Che-Othman et al., 2017). Previously it was reported that the amino acid and carbohydrate metabolic pathways play a crucial role during salt stress in S. lycopersicum (Zhang et al., 2017). These results demonstrated important signaling pathways that have crucial functions under drought and salt stress conditions.
The transcriptome differential expression profiles of the identified drought and salt stress-responsive hub genes were constructed using significant log2FC and degree centrality values. In drought stress, NSP2, CDF1, DRE1D, ERF61, P2C08, HFB2A, TLP3, MYB15, ORG2, and TLP5 genes show significantly higher expression (Figure 8A). The DEGs in drought were mainly clustered into four groups, in which Group II, III, and IV comprised upregulated genes, while Group I contained downregulated genes. In the upregulated genes, TLP5 does not group with any other genes, as group II only includes the TLP5 gene. In salt stress, the genes TLP1, TLP, P2C08, EF109, ATHB7, ELF4, bHLH25, DRE1D, WRKY6, bHLH126, and NAC83 show a significantly higher expression (Figure 8B). The DEGs in salt were also clustered into four groups, in which Group II, III, and IV comprised upregulated genes while Group I contained downregulated genes. In the upregulated genes, TLP1 does not group with other genes, since Group II only includes the TLP1 gene and showed the highest expression (>10-fold) among the upregulated genes. The TLP gene members have been identified in some dicotyledonous and monocotyledonous plants such as maize (Chen et al., 2016), apple (Xu et al., 2016), and Arabidopsis, poplar, rice (Yang et al., 2008). In Arabidopsis, AtTLP3, and AtTLP9 were found to have a redundant effect in response to osmotic stress treatments and ABA (Lai et al., 2004), while AtTLP9 also responded to salt and drought stress (Lai et al., 2004; Bao et al., 2014). In Malus domestica, several TLP genes showed higher expression during abiotic stress treatments (Xu et al., 2016). It was found that CaTLP1 was expressed in response to dehydration stress in Cicer arietinum, while its expression led to increased tolerance to salt, drought, and oxidative stress in tobacco (Wardhan et al., 2012). Based on the higher expression of hub genes in the transcriptome dataset, four genes (NSP2, DRE1D, TLP5, and TLP3) in drought and four genes (TLP1, WRKY6, ATHB7, and EF109) in salt stress were taken for further qRT-PCR validation (Figure 9). The results showed that tubby-like protein (TLP) showed higher expression in both drought and salt stress responses. Further, correlation analysis also supported the significant positive correlation between qRT-PCR and transcriptome data (Figures 9I,J). As per the previous report, the WRKY6 gene helps in the induction of salicylic acid (Robatzek and Somssich, 2001) in A. thaliana which has reported an important role in mitigating the adverse effects of salt stress on plants (Ma et al., 2017). The higher expression of TLP1 in Cicer arietinum led to increased tolerance to salt, drought, and oxidative stress in tobacco (Wardhan et al., 2012). Moreover, AtTLP3 member has been found to respond to abiotic stresses (Li Z. S. et al., 2021). The overall outcomes suggested that NSP2, DRE1D, TLP5, and TLP3 in drought and TLP1, WRKY6, ATHB7, and EF109 in salt may be crucial in cotton improvement.
Altogether, these studies suggested that TLP gene family members might play an important role in drought as well as in salt stress tolerance and could be suitable targets to protect the cotton from drought and salt stress conditions.
In order to confirm TLP’s functional role and other highly expressed genes in drought and stress conditions, the cis-regulatory element analysis was performed. In drought stress, TLP5, NSP2, DRE1D, NAC29, TLP3, and HFA6B include a higher number of abiotic stress-responsive elements (Table 3A), the upstream region of these putative genes show the abundance of MBS (MYB binding site involved in drought-inducibility) (Figure 10A). This MBS-cis-element is required by the MYB TF for the gene expression of drought-inducible genes (Kaur et al., 2017). Moreover, the TGA-element (auxin-responsive element) shows dominance in the TLP3 gene. The plant can regulate stomatal opening, integrate growth and stomatal regulation in drought conditions by utilizing auxin in Arabidopsis (Salehin et al., 2019). In salt stress, EF109, MIP1B, ATHB7, and TLP contain a higher number of elements that respond to abiotic stress in salt stress (Table 3B), where ABRE elements (cis-acting element involved in the abscisic acid responsiveness) were abundant (Figure 10B). Previously it was stated that ABA is a crucial plant hormone with an important role in regulating salt stress responses (Ha et al., 2014), salinity exposure enhances the accumulation of ABA, resulting in increased tolerance (Zhu, 2002). In addition, TLP1 and WRKY comprise the abundance of P-box (gibberellin-responsive element) in their upstream region. Gibberellic acid is one of the essential plant growth regulators that help improve salt tolerance and also reduce the effects of salt stress on plants (Chauhan et al., 2019) and also induces high salinity tolerance in Pisum sativum through upregulation of antiporter genes, antioxidants, and secondary metabolites (Ahmad et al., 2021). Altogether, these studies suggested that NSP2, DRE1D, TLP5, and TLP3 genes in drought and TLP1, WRKY6, ATHB7, and EF109 genes in salt stress might play an important role in cotton and could be suitable targets to protect the cotton crop from drought and salt stress condition.
Overall, our integrative transcriptome meta-analysis and its network topology analysis revealed that NSP2, DRE1D, ERF61, CDF1, and TLP3 genes induced strong drought stress responses, accentuated by the increased expression levels and the response of TLP1, TLP, EF109, ELF4, and ATHB7 genes enhanced during salt stress condition. The functional enrichment study of candidate hub genes showed the role of oxidoreductase and dioxygenase activity, response to an abiotic stimulus along with several secondary metabolite responsive genes in drought stress conditions, whereas the response to stimulus and salt, response to hypoxia, and decreased oxygen levels with catalytic activity responsive genes in salt stress condition. Moreover pathways analysis also demonstrating the higher numbers of putative hub genes involved in secondary metabolite responsive pathways that have found an important role in drought and salt stress states. These drought and salt stress responsive functions were further confirmed through the higher expression values of putative genes in transcriptome data and qRT-PCR validation and proximal element studies. These droughts (NSP2, DRE1D, TLP5, and TLP3) and salt (TLP1, WRKY6, ATHB7, and EF109) stress-responsive hub genes exert tolerance against unfavorable conditions and protect the cotton crop from drought and stress conditions. These protective actions of hub genes might account to reduce the loss of crop productivity and provide resistivity in adverse states.
The original contributions presented in the study are included in the article/Supplementary Material, further inquiries can be directed to the corresponding author/s.
NB carried out the bioinformatics analysis, designed, and drafted the manuscript. SF performed quantitative expression analysis. CM and SB participated to supervise the study. All authors have read and approved the final manuscript.
This work was supported by a grant (MLP0035) from the Council of Scientific and Industrial Research (CSIR), India. NB acknowledges the financial support from CSIR-UGC SRF.
The authors declare that the research was conducted in the absence of any commercial or financial relationships that could be construed as a potential conflict of interest.
All claims expressed in this article are solely those of the authors and do not necessarily represent those of their affiliated organizations, or those of the publisher, the editors and the reviewers. Any product that may be evaluated in this article, or claim that may be made by its manufacturer, is not guaranteed or endorsed by the publisher.
We acknowledge the HPC facility, CSIR-4PI, and Bangalore. We are also grateful to Mehar Hasan Asif for giving an idea of this work. The manuscript number is provided by the institute is CSIR-NBRI_MS/2021/11/09.
The Supplementary Material for this article can be found online at: https://www.frontiersin.org/articles/10.3389/fpls.2022.818472/full#supplementary-material
Supplementary Figure 1 | Heatmap cluster of expression in different tissues (leaf, root, and seed) under drought and salt stress conditions in cotton using log2FC.
Supplementary Figure 2 | Venn diagram showing the number of shared and unique genes in DEGs of drought and salt stress data in the root, leaf, and seed tissues.
Abdelraheem, A., Esmaeili, N., O’Connell, M., and Zhang, J. F. (2019). Progress and perspective on drought and salt stress tolerance in cotton. Ind. Crop Prod. 130, 118–129. doi: 10.3389/fpls.2021.759245
Abdelraheem, A., Thyssen, G. N., Fang, D. D., Jenkins, J. N., McCarty, J. C., Wedegaertner, T., et al. (2021). GWAS reveals consistent QTL for drought and salt tolerance in a MAGIC population of 550 lines derived from intermating of 11 upland cotton (Gossypium hirsutum) parents. Mol. Genet. Genomics 296, 119–129. doi: 10.1007/s00438-020-01733-2
Abuelsoud, W., Hirschmann, F., and Papenbrock, J. (2016). “Sulfur metabolism and drought stress tolerance in plants,” in Drought Stress Tolerance in Plants: Physiology and Biochemistry, Vol. Vol 1, eds M. A. Hossain, S. H. Wani, S. Bhattacharjee, D. J. Burritt, and L.-S. P. Tran (Berlin: Springer), 227–249. doi: 10.1007/978-3-319-28899-4_9
Afrin, S., Huang, J. J., and Luo, Z. Y. (2015). JA-mediated transcriptional regulation of secondary metabolism in medicinal plants. Sci. Bull. 60, 1062–1072. doi: 10.1007/s11434-015-0813-0
Agarwal, P. K., Gupta, K., Lopato, S., and Agarwal, P. (2017). Dehydration responsive element binding transcription factors and their applications for the engineering of stress tolerance. J. Exp. Bot. 68, 2135–2148. doi: 10.1093/jxb/erx118
Ahmad, F., Kamal, A., Singh, A., Ashfaque, F., Alamri, S., Siddiqui, M. H., et al. (2021). Seed priming with gibberellic acid induces high salinity tolerance in Pisum sativum through antioxidants, secondary metabolites and up-regulation of antiporter genes. Plant Biol. 23, 113–121. doi: 10.1111/plb.13187
Ahmad, N., Malagoli, M., Wirtz, M., and Hell, R. (2016). Drought stress in maize causes differential acclimation responses of glutathione and sulfur metabolism in leaves and roots. BMC Plant Biol. 16:247. doi: 10.1186/s12870-016-0940-z
Ali, S., Hayat, K., Iqbal, A., and Xie, L. N. (2020). Implications of abscisic acid in the drought stress tolerance of plants. Agronomy 10:1323. doi: 10.3390/agronomy10091323
Ambrosino, L., Colantuono, C., Diretto, G., Fiore, A., and Chiusano, M. L. (2020). Bioinformatics resources for plant abiotic stress responses: state of the art and opportunities in the fast evolving -Omics era. Plants 9:591. doi: 10.3390/plants9050591
Aoki, K., Ogata, Y., and Shibata, D. (2007). Approaches for extracting practical information from gene co-expression networks in plant biology. Plant Cell Physiol. 48, 381–390. doi: 10.1093/pcp/pcm013
Bandehagh, A., and Taylor, N. L. (2020). Can alternative metabolic pathways and shunts overcome salinity induced inhibition of central carbon metabolism in crops? Front. Plant Sci. 11:1072. doi: 10.3389/fpls.2020.01072
Bao, Y., Song, W. M., Jin, Y. L., Jiang, C. M., Yang, Y., Li, B., et al. (2014). Characterization of Arabidopsis tubby-like proteins and redundant function of AtTLP3 and AtTLP9 in plant response to ABA and osmotic stress. Plant Mol. Biol. 86, 471–483. doi: 10.1007/s11103-014-0241-6
Bindea, G., Mlecnik, B., Hackl, H., Charoentong, P., Tosolini, M., Kirilovsky, A., et al. (2009). ClueGO: a Cytoscape plug-in to decipher functionally grouped gene ontology and pathway annotation networks. Bioinformatics 25, 1091–1093. doi: 10.1093/bioinformatics/btp101
Bolger, A. M., Lohse, M., and Usadel, B. (2014). Trimmomatic: a flexible trimmer for Illumina sequence data. Bioinformatics 30, 2114–2120. doi: 10.1093/bioinformatics/btu170
Brady, S. M., and Provart, N. J. (2009). Web-queryable large-scale data sets for hypothesis generation in plant biology. Plant Cell 21, 1034–1051. doi: 10.1105/tpc.109.066050
Brito, V. C., de Almeida, C. P., Barbosa, R. R., Carosio, M. G. A., Ferreira, A. G., Fernandez, L. G., et al. (2020). Overexpression of Ricinus communis L. malate synthase enhances seed tolerance to abiotic stress during germination. Ind. Crop Prod. 145:112110. doi: 10.1016/j.indcrop.2020.112110
Cao, X. M., Duan, W. Y., Wei, C. Y., Chen, K. S., Grierson, D., and Zhang, B. (2019). Genome-wide identification and functional analysis of carboxylesterase and methylesterase gene families in peach (Prunus persica L. Batsch). Front. Plant Sci. 10:1511. doi: 10.3389/fpls.2019.01511
Chandra, P., Wunnava, A., Verma, P., Chandra, A., and Sharma, R. K. (2021). Strategies to mitigate the adverse effect of drought stress on crop plants-influences of soil bacteria: a review. Pedosphere 31, 496–509. doi: 10.1016/s1002-0160(20)60092-3
Chauhan, A., AbuAmarah, B. A., Kumar, A., Verma, J. S., Ghramh, H. A., Khan, K. A., et al. (2019). Influence of gibberellic acid and different salt concentrations on germination percentage and physiological parameters of oat cultivars. Saudi J. Biol. Sci. 26, 1298–1304. doi: 10.1016/j.sjbs.2019.04.014
Chen, L. J., Guo, H. M., Lin, Y., and Cheng, H. M. (2015). Chalcone synthase EaCHS1 from Eupatorium adenophorum functions in salt stress tolerance in tobacco. Plant Cell Rep. 34, 885–894. doi: 10.1007/s00299-015-1751-7
Chen, Y. L., Dai, W., Sun, B. M., Zhao, Y., and Ma, Q. (2016). Genome-wide identification and comparative analysis of the TUBBY-like protein gene family in maize. Genes Genomics 38, 25–36. doi: 10.1007/s13258-015-0338-6
Chen, Z. J., Scheffler, B. E., Dennis, E., Triplett, B. A., Zhang, T., Guo, W., et al. (2007). Toward sequencing cotton (Gossypium) genomes. Plant Physiol. 145, 1303–1310. doi: 10.1104/pp.107.107672
Chen, Z. J., Sreedasyam, A., Ando, A., Song, Q. X., De Santiago, L. M., Hulse-Kemp, A. M., et al. (2020). Genomic diversifications of five Gossypium allopolyploid species and their impact on cotton improvement. Nat. Genet. 52, 525–533. doi: 10.1038/s41588-020-0614-5
Cheng, Q., Gan, Z. R., Wang, Y. P., Lu, S. J., Hou, Z. H., Li, H. Y., et al. (2020). The soybean gene j contributes to salt stress tolerance by up-regulating salt-responsive genes. Front. Plant Sci. 11:272. doi: 10.3389/fpls.2020.00272
Che-Othman, M. H., Millar, A. H., and Taylor, N. L. (2017). Connecting salt stress signalling pathways with salinity-induced changes in mitochondrial metabolic processes in C3 plants. Plant Cell Environ. 40, 2875–2905. doi: 10.1111/pce.13034
Chin, D. C., Kumar, R. S., Suen, C. S., Chien, C. Y., Hwang, M. J., Hsu, C. H., et al. (2019). Plant cytosolic ascorbate peroxidase with dual catalytic activity modulates abiotic stress tolerances. Iscience 16, 31–49. doi: 10.1016/j.isci.2019.05.014
Choi, J. Y., Seo, Y. S., Kim, S. J., Kim, W. T., and Shin, J. S. (2011). Constitutive expression of CaXTH3, a hot pepper xyloglucan endotransglucosylase/hydrolase, enhanced tolerance to salt and drought stresses without phenotypic defects in tomato plants (Solanum lycopersicum cv. Dotaerang). Plant Cell Rep. 30, 867–877. doi: 10.1007/s00299-010-0989-3
Cramer, G. R., Urano, K., Delrot, S., Pezzotti, M., and Shinozaki, K. (2011). Effects of abiotic stress on plants: a systems biology perspective. BMC Plant Biol. 11:163. doi: 10.1186/1471-2229-11-163
Cuong, D., Kwon, S. J., Nguyen, B. V., Chun, S. W., Kim, J. K., and Park, S. U. (2020). Effect of salinity stress on phenylpropanoid genes expression and related gene expression in wheat sprout. Agronomy 10:390. doi: 10.3390/agronomy10030390
Czerniawski, P., and Bednarek, P. (2018). Glutathione S-transferases in the biosynthesis of sulfur-containing secondary metabolites in brassicaceae plants. Front. Plant Sci. 9:1639. doi: 10.3389/fpls.2018.01639
Dabbert, T., and Gore, M. A. (2014). Challenges and perspectives on improving heat and drought stress resilience in cotton. J. Cotton Sci. 18, 393–409.
Daniel, B., Konrad, B., Toplak, M., Lahham, M., Messenlehner, J., Winkler, A., et al. (2017). The family of berberine bridge enzyme-like enzymes: a treasure-trove of oxidative reactions. Arch. Biochem. Biophys. 632, 88–103. doi: 10.1016/j.abb.2017.06.023
Dao, T. T. H., Linthorst, H. J. M., and Verpoorte, R. (2011). Chalcone synthase and its functions in plant resistance. Phytochem. Rev. 10, 397–412. doi: 10.1007/s11101-011-9211-7
de Jesus-Pires, C., Ferreira-Neto, J. R. C., Pacifico Bezerra-Neto, J., Kido, E. A., de Oliveira Silva, R. L., Pandolfi, V., et al. (2020). Plant thaumatin-like proteins: function, evolution and biotechnological applications. Curr. Protein Pept. Sci. 21, 36–51. doi: 10.2174/1389203720666190318164905
de Kraker, J. W., and Gershenzon, J. (2011). From amino acid to glucosinolate biosynthesis: protein sequence changes in the evolution of methylthioalkylmalate synthase in Arabidopsis. Plant Cell 23, 38–53. doi: 10.1105/tpc.110.079269
de Ollas, C., Hernando, B., Arbona, V., and Gomez-Cadenas, A. (2013). Jasmonic acid transient accumulation is needed for abscisic acid increase in citrus roots under drought stress conditions. Physiol. Plant. 147, 296–306. doi: 10.1111/j.1399-3054.2012.01659.x
Dobin, A., Davis, C. A., Schlesinger, F., Drenkow, J., Zaleski, C., Jha, S., et al. (2013). STAR: ultrafast universal RNA-seq aligner. Bioinformatics 29, 15–21. doi: 10.1093/bioinformatics/bts635
Doncheva, N. T., Assenov, Y., Domingues, F. S., and Albrecht, M. (2012). Topological analysis and interactive visualization of biological networks and protein structures. Nat. Protoc. 7, 670–685. doi: 10.1038/nprot.2012.004
Egea, I., Albaladejo, I., Meco, V., Morales, B., Sevilla, A., Bolarin, M. C., et al. (2018). The drought-tolerant Solanum pennellii regulates leaf water loss and induces genes involved in amino acid and ethylene/jasmonate metabolism under dehydration. Sci. Rep. 8:2791. doi: 10.1038/s41598-018-21187-2
Geng, D. L., Shen, X. X., Xie, Y. P., Yang, Y. S., Bian, R. L., Gao, Y. Q., et al. (2020). Regulation of phenylpropanoid biosynthesis by MdMYB88 and MdMYB124 contributes to pathogen and drought resistance in apple. Hortic. Res. 7:102. doi: 10.1038/s41438-020-0324-2
George, S., Manoharan, D., Li, J., Britton, M., and Parida, A. (2017). Drought and salt stress in Chrysopogon zizanioides leads to common and specific transcriptomic responses and may affect essential oil composition and benzylisoquinoline alkaloids metabolism. Curr. Plant Biol. 11, 12–22. doi: 10.1016/j.cpb.2017.12.001
Goda, H., Sasaki, E., Akiyama, K., Maruyama-Nakashita, A., Nakabayashi, K., Li, W. Q., et al. (2008). The AtGenExpress hormone and chemical treatment data set: experimental design, data evaluation, model data analysis and data access. Plant J. 55, 526–542. doi: 10.1111/j.0960-7412.2008.03510.x
Goossens, A., Mertens, J., Van Moerkercke, A., Pollier, J., and Memelink, J. (2019). Means and methods for regulating secondary metabolite production in plants. U.S. Patent No. 10,370,672.
Grover, C. E., Zhu, X., Grupp, K. K., Jareczek, J. J., Gallagher, J. P., Szadkowski, E., et al. (2015). Molecular confirmation of species status for the allopolyploid cotton species, Gossypium ekmanianum Wittmack. Genet. Resour. Crop Evol. 62, 103–114. doi: 10.1007/s10722-014-0138-x
Guerriero, G., Berni, R., Munoz-Sanchez, J. A., Apone, F., Abdel-Salam, E. M., Qahtan, A. A., et al. (2018). Production of plant secondary metabolites: examples, tips and suggestions for biotechnologists. Genes 9:309. doi: 10.3390/genes9060309
Guo, M. L., Liu, X., Wang, J. H., Li, L., Zhang, W. D., Gong, B. J., et al. (2019). Investigation on salt-response mechanisms in Arabidopsis thaliana from UniProt protein knowledgebase. J. Plant Interact. 14, 21–29. doi: 10.1080/17429145.2018.1551581
Ha, C. V., Leyva-Gonzalez, M. A., Osakabe, Y., Tran, U. T., Nishiyama, R., Watanabe, Y., et al. (2014). Positive regulatory role of strigolactone in plant responses to drought and salt stress. Proc. Natl. Acad. Sci. U.S.A. 111, 851–856. doi: 10.1073/pnas.1322135111
Hartwell, L. H., Hopfield, J. J., Leibler, S., and Murray, A. W. (1999). From molecular to modular cell biology. Nature 402, C47–C52. doi: 10.1038/35011540
Hawkins, J. S., Kim, H., Nason, J. D., Wing, R. A., and Wendel, J. F. (2006). Differential lineage-specific amplification of transposable elements is responsible for genome size variation in Gossypium. Genome Res. 16, 1252–1261. doi: 10.1101/gr.5282906
He, Z. H., Li, Z. Y., Lu, H. J., Huo, L., Wang, Z. B., Wang, Y. C., et al. (2019). The NAC protein from Tamarix hispida, ThNAC7, confers salt and osmotic stress tolerance by increasing reactive oxygen species scavenging capability. Plants 8:221. doi: 10.3390/plants8070221
Hu, W., Hu, G., and Han, B. (2009). Genome-wide survey and expression profiling of heat shock proteins and heat shock factors revealed overlapped and stress specific response under abiotic stresses in rice. Plant Sci. 176, 583–590. doi: 10.1016/j.plantsci.2009.01.016
Huang, L., Zhang, F., Zhang, F., Wang, W., Zhou, Y., Fu, B., et al. (2014). Comparative transcriptome sequencing of tolerant rice introgression line and its parents in response to drought stress. BMC Genomics 15:1026. doi: 10.1186/1471-2164-15-1026
Huang, Q., Wang, Y., Li, B., Chang, J., Chen, M., Li, K., et al. (2015). TaNAC29, a NAC transcription factor from wheat, enhances salt and drought tolerance in transgenic Arabidopsis. BMC Plant Biol. 15:268. doi: 10.1186/s12870-015-0644-9
Ibrahim, M. H., and Jaafar, H. Z. E. (2013). Abscisic acid induced changes in production of primary and secondary metabolites, photosynthetic capacity, antioxidant capability, antioxidant enzymes and lipoxygenase inhibitory activity of Orthosiphon stamineus Benth. Molecules 18, 7957–7976. doi: 10.3390/molecules18077957
Jadamba, C., Kang, K., Paek, N. C., Lee, S. I., and Yoo, S. C. (2020). Overexpression of rice expansin7 (osexpa7) confers enhanced tolerance to salt stress in rice. Int. J. Mol. Sci. 21:454. doi: 10.3390/ijms21020454
Jain, M., Nijhawan, A., Arora, R., Agarwal, P., Ray, S., Sharma, P., et al. (2007). F-box proteins in rice. Genome-wide analysis, classification, temporal and spatial gene expression during panicle and seed development, and regulation by light and abiotic stress. Plant Physiol. 143, 1467–1483. doi: 10.1104/pp.106.091900
Jin, M. L., Lee, W. M., and Kim, O. T. (2017). Two cycloartenol synthases for phytosterol biosynthesis in Polygala tenuifolia willd. Int. J. Mol. Sci. 18:2426. doi: 10.3390/ijms18112426
Joshi, R., Sahoo, K. K., Singh, A. K., Anwar, K., Pundir, P., Gauta, R. K., et al. (2020). Enhancing trehalose biosynthesis improves yield potential in marker-free transgenic rice under drought, saline, and sodic conditions. J. Exp. Bot. 71, 653–668. doi: 10.1093/jxb/erz462
Kaur, A., Pati, P. K., Pati, A. M., and Nagpal, A. K. (2017). In-silico analysis of cis-acting regulatory elements of pathogenesis-related proteins of Arabidopsis thaliana and Oryza sativa. PLoS One 12:e0184523. doi: 10.1371/journal.pone.0184523
Kaur, H., Mukherjee, S., Baluska, F., and Bhatla, S. C. (2015). Regulatory roles of serotonin and melatonin in abiotic stress tolerance in plants. Plant Signal. Behav. 10:e1049788. doi: 10.1080/15592324.2015.1049788
Lai, C. P., Lee, C. L., Chen, P. H., Wu, S. H., Yang, C. C., and Shaw, J. F. (2004). Molecular analyses of the Arabidopsis TUBBY-like protein gene family. Plant Physiol. 134, 1586–1597. doi: 10.1104/pp.103.037820
Leakey, A. D. B., Ainsworth, E. A., Bernacchi, C. J., Rogers, A., Long, S. P., and Ort, D. R. (2009). Elevated CO2 effects on plant carbon, nitrogen, and water relations: six important lessons from FACE. J. Exp. Bot. 60, 2859–2876. doi: 10.1093/jxb/erp096
Lescot, M., Dehais, P., Thijs, G., Marchal, K., Moreau, Y., Van de Peer, Y., et al. (2002). PlantCARE, a database of plant cis-acting regulatory elements and a portal to tools for in silico analysis of promoter sequences. Nucleic Acids Res. 30, 325–327. doi: 10.1093/nar/30.1.325
Li, B. Z., Fan, R. N., Guo, S. Y., Wang, P. T., Zhu, X. H., Fan, Y. T., et al. (2019). The Arabidopsis MYB transcription factor, MYB111 modulates salt responses by regulating flavonoid biosynthesis. Environ. Exp. Bot. 166:103807. doi: 10.1016/j.envexpbot.2019.103807
Li, B. Z., Fan, R. N., Sun, G. L., Sun, T., Fan, Y. T., Bai, S. L., et al. (2021). Flavonoids improve drought tolerance of maize seedlings by regulating the homeostasis of reactive oxygen species. Plant Soil 461, 389–405. doi: 10.1007/s11104-020-04814-8
Li, Z. S., Wang, X. Y., Cao, X. C., Chen, B. Z., Ma, C. K., Lv, J. Y., et al. (2021). GhTULP34, a member of tubby-like proteins, interacts with GhSKP1A to negatively regulate plant osmotic stress. Genomics 113, 462–474. doi: 10.1016/j.ygeno.2020.09.024
Li, H., Chang, J., Chen, H., Wang, Z., Gu, X., Wei, C., et al. (2017). Exogenous melatonin confers salt stress tolerance to watermelon by improving photosynthesis and redox homeostasis. Front. Plant Sci. 8:295. doi: 10.3389/fpls.2017.00295
Li, W. X., Pang, S. Y., Lu, Z. G., and Jin, B. (2020). Function and mechanism of WRKY transcription factors in abiotic stress responses of plants. Plants 9:1515. doi: 10.3390/plants9111515
Liao, J. C., Boscolo, R., Yang, Y. L., Tran, L. M., Sabatti, C., and Roychowdhury, V. P. (2003). Network component analysis: reconstruction of regulatory signals in biological systems. Proc. Natl. Acad. Sci. U.S.A. 100, 15522–15527. doi: 10.1073/pnas.2136632100
Liu, M. M., and Lu, S. F. (2016). Plastoquinone and ubiquinone in plants: biosynthesis, physiological function and metabolic engineering. Front. Plant Sci. 7:1898. doi: 10.3389/fpls.2016.01898
Livak, K. J., and Schmittgen, T. D. (2001). Analysis of relative gene expression data using real-time quantitative PCR and the 2- ΔΔCT method. Methods 25, 402–408. doi: 10.1006/meth.2001.1262
Ma, Q. B., Dai, X. Y., Xu, Y. Y., Guo, J., Liu, Y. J., Chen, N., et al. (2009). Enhanced tolerance to chilling stress in OsMYB3R-2 transgenic rice is mediated by alteration in cell cycle and ectopic expression of stress genes. Plant Physiol. 150, 244–256. doi: 10.1104/pp.108.133454
Ma, S., and Bohnert, H. J. (2007). Integration of Arabidopsis thaliana stress-related transcript profiles, promoter structures, and cell-specific expression. Genome Biol. 8:R49. doi: 10.1186/gb-2007-8-4-r49
Ma, X. H., Zheng, J., Zhang, X., Hu, Q. D., and Qian, R. J. (2017). Salicylic acid alleviates the adverse effects of salt stress on Dianthus superbus (Caryophyllaceae) by activating photosynthesis, protecting morphological structure, and enhancing the antioxidant system. Front. Plant Sci. 8:600. doi: 10.3389/fpls.2017.00600
Manikandan, A., Sahu, DK., Blaise, D., and Shukla, PK. (2020). Identification of relationship among exogenous NaCl with cotton leaves on cation uptake, nutrient ratios and status in rhizosphere soil. Chem. Sci. Rev. Lett. 9, 956–965.
Marchev, A. S., Yordanova, Z. P., and Georgiev, M. I. (2020). Green (cell) factories for advanced production of plant secondary metabolites. Crit. Rev. Biotechnol. 40, 443–458. doi: 10.1080/07388551.2020.1731414
Meraj, T. A., Fu, J. Y., Raza, M. A., Zhu, C. Y., Shen, Q. Q., Xu, D. B., et al. (2020). Transcriptional factors regulate plant stress responses through mediating secondary metabolism. Genes 11:346. doi: 10.3390/genes11040346
Merico, D., Gfeller, D., and Bader, G. D. (2009). How to visually interpret biological data using networks. Nat. Biotechnol. 27, 921–924. doi: 10.1038/nbt.1567
Mochida, K., Uehara-Yamaguchi, Y., Yoshida, T., Sakurai, T., and Shinozaki, K. (2011). Global landscape of a co-expressed gene network in barley and its application to gene discovery in Triticeae crops. Plant Cell Physiol. 52, 785–803. doi: 10.1093/pcp/pcr035
Moreno-Risueno, M. A., Busch, W., and Benfey, P. N. (2010). Omics meet networks - using systems approaches to infer regulatory networks in plants. Curr. Opin. Plant Biol. 13, 126–131. doi: 10.1016/j.pbi.2009.11.005
Munir, S., Liu, H., Xing, Y. L., Hussain, S., Ouyang, B., Zhang, Y. Y., et al. (2016). Overexpression of calmodulin-like (ShCML44) stress-responsive gene from Solanum habrochaites enhances tolerance to multiple abiotic stresses. Sci. Rep. 6:31772. doi: 10.1038/srep31772
Murch, S. J., KrishnaRaj, S., and Saxena, P. K. (2000). Tryptophan is a precursor for melatonin and serotonin biosynthesis in in vitro regenerated St. John’s Wort (Hypericum perforatum L. cv. Anthos) plants. Plant Cell Rep. 19, 698–704. doi: 10.1007/s002990000206
Mustroph, A., Lee, S. C., Oosumi, T., Zanetti, M. E., Yang, H., Ma, K., et al. (2010). Cross-kingdom comparison of transcriptomic adjustments to low-oxygen stress highlights conserved and plant-specific responses. Plant Physiol. 152, 1484–1500. doi: 10.1104/pp.109.151845
Muthusamy, M., Kim, J. Y., Yoon, E. K., Kim, J. A., and Lee, S. I. (2020). BrEXLB1, a Brassica rapa expansin-like B1 gene is associated with root development, drought stress response, and seed germination. Genes 11:404. doi: 10.3390/genes11040404
Nazar, R., Iqbal, N., Masood, A., Syeed, S., and Khan, N. A. (2011). Understanding the significance of sulfur in improving salinity tolerance in plants. Environ. Exp. Bot. 70, 80–87. doi: 10.1038/s41598-021-92317-6
Nirmala, B., Vamadevaih, H., Katageri, I., Uppinal, N., and Mirajkar, K. (2012). In vitro screening for salinity stress at seedling stage of cotton. Karnataka J. Agric. Sci. 25, 39–42.
Novo-Uzal, E., Gutierrez, J., Martinez-Cortes, T., and Pomar, F. (2014). Molecular cloning of two novel peroxidases and their response to salt stress and salicylic acid in the living fossil Ginkgo biloba. Ann. Bot. 114, 923–936. doi: 10.1093/aob/mcu160
Olsson, A., Engström, P., and Söderman, E. (2004). The homeobox genes ATHB12 and ATHB7encode potential regulators of growth in response to water deficit in Arabidopsis. Plant Mol. Biol. 55, 663–677. doi: 10.1007/s11103-004-1581-4
Paetzold, H., Garms, S., Bartram, S., Wieczorek, J., Uros-Gracia, E. M., Rodriguez-Concepcion, M., et al. (2010). The isogene 1-Deoxy-D-Xylulose 5-Phosphate Synthase 2 controls isoprenoid profiles, precursor pathway allocation, and density of tomato trichomes. Mol. Plant 3, 904–916. doi: 10.1093/mp/ssq032
Patade, V. Y., Bhargava, S., and Suprasanna, P. (2009). Halopriming imparts tolerance to salt and PEG induced drought stress in sugarcane. Agric. Ecosyst. Environ. 134, 24–28. doi: 10.1016/j.agee.2009.07.003
Patel, M. K., Kumar, M., Li, W. Q., Luo, Y., Burritt, D. J., Alkan, N., et al. (2020). Enhancing salt tolerance of plants: from metabolic reprogramming to exogenous chemical treatments and molecular approaches. Cells 9:2492. doi: 10.3390/cells9112492
Paupiere, M. J., Tikunov, Y., Schleiff, E., Bovy, A., and Fragkostefanakis, S. (2020). Reprogramming of tomato leaf metabolome by the activity of heat stress transcription factor HsfB1. Front. Plant Sci. 11:610599. doi: 10.3389/fpls.2020.610599
Prasad, K., Khatoon, F., Rashid, S., Ali, N., AlAsmari, AF., Ahmed, MZ., et al. (2020). Targeting hub genes and pathways of innate immune response in COVID-19: A network biology perspective. Int. J. Biol. Macromol. 163, 1–8. doi: 10.1016/j.ijbiomac.2020.06.228
Reményi, A., Schöler, H. R., and Wilmanns, M. (2004). Combinatorial control of gene expression. Nat. Struct. Mol. Biol. 11, 812–815. doi: 10.1038/nsmb820
Rhee, S. Y., and Mutwil, M. (2014). Towards revealing the functions of all genes in plants. Trends Plant Sci. 19, 212–221. doi: 10.1016/j.tplants.2013.10.006
Robatzek, S., and Somssich, I. E. (2001). A new member of the Arabidopsis WRKY transcription factor family, AtWRKY6, is associated with both senescence- and defence-related processes. Plant J. 28, 123–133. doi: 10.1046/j.1365-313x.2001.01131.x
Rong, J., Bowers, J. E., Schulze, S. R., Waghmare, V. N., Rogers, C. J., Pierce, G. J., et al. (2005). Comparative genomics of Gossypium and Arabidopsis: unraveling the consequences of both ancient and recent polyploidy. Genome Res. 15, 1198–1210. doi: 10.1101/gr.3907305
Sakuraba, Y., Bulbul, S., Piao, W. L., Choi, G., and Paek, N. C. (2017). Arabidopsis EARLY FLOWERING3 increases salt tolerance by suppressing salt stress response pathways. Plant J. 92, 1106–1120. doi: 10.1111/tpj.13747
Salehin, M., Li, B. H., Tang, M., Katz, E., Song, L., Ecker, J. R., et al. (2019). Auxin-sensitive Aux/IAA proteins mediate drought tolerance in Arabidopsis by regulating glucosinolate levels. Nat. Commun. 10:4021. doi: 10.1038/s41467-019-12002-1
Savchenko, T. V., Zastrijnaja, O. M., and Klimov, V. V. (2014). Oxylipins and plant abiotic stress resistance. Biochemistry 79, 362–375. doi: 10.1134/S0006297914040051
Senchina, D. S., Alvarez, I., Cronn, R. C., Liu, B., Rong, J. K., Noyes, R. D., et al. (2003). Rate variation among nuclear genes and the age of polyploidy in Gossypium. Mol. Biol. Evol. 20, 633–643. doi: 10.1093/molbev/msg065
Serin, E. A. R., Nijveen, H., Hilhorst, H. W. M., and Ligterink, W. (2016). Learning from Co-expression networks: possibilities and challenges. Front. Plant Sci. 7:444. doi: 10.3389/fpls.2016.00444
Shao, Q., Liu, X. F., Su, T., Ma, C. L., and Wang, P. P. (2019). New insights into the role of seed oil body proteins in metabolism and plant development. Front. Plant Sci. 10:1568. doi: 10.3389/fpls.2019.01568
Sharma, A., Shahzad, B., Rehman, A., Bhardwaj, R., Landi, M., and Zheng, B. S. (2019). Response of phenylpropanoid pathway and the role of polyphenols in plants under abiotic stress. Molecules 24:2452. doi: 10.3390/molecules24132452
Sharma, R., De Vleesschauwer, D., Sharma, M. K., and Ronald, P. C. (2013). Recent advances in dissecting stress-regulatory crosstalk in rice. Mol. Plant 6, 250–260. doi: 10.1093/mp/sss147
Shi, P., and Gu, M. (2020). Transcriptome analysis and differential gene expression profiling of two contrasting quinoa genotypes in response to salt stress. BMC Plant Biol 20:568. doi: 10.1186/s12870-020-02753-1
Shirazi, Z., Aalami, A., Tohidfar, M., and Sohani, M. M. (2019). Triterpenoid gene expression and phytochemical content in Iranian licorice under salinity stress. Protoplasma 256, 827–837. doi: 10.1007/s00709-018-01340-4
Smoot, M. E., Ono, K., Ruscheinski, J., Wang, P. L., and Ideker, T. (2011). Cytoscape 2.8: new features for data integration and network visualization. Bioinformatics 27, 431–432. doi: 10.1093/bioinformatics/btq675
Stuhrwohldt, N., Buhler, E., Sauter, M., and Schaller, A. (2021). Phytosulfokine (PSK) precursor processing by subtilase SBT3.8 and PSK signaling improve drought stress tolerance in Arabidopsis. J. Exp. Bot. 72, 3427–3440. doi: 10.1093/jxb/erab017
Tattini, M., Velikova, V., Vickers, C., Brunetti, C., Di Ferdinando, M., Trivellini, A., et al. (2014). Isoprene production in transgenic tobacco alters isoprenoid, non-structural carbohydrate and phenylpropanoid metabolism, and protects photosynthesis from drought stress. Plant Cell Environ. 37, 1950–1964. doi: 10.1111/pce.12350
Taura, F., Sirikantaramas, S., Shoyama, Y., Yoshikai, K., Shoyama, Y., and Morimoto, S. (2007). Cannabidiolic-acid synthase, the chemotype-determining enzyme in the fiber-type Cannabis sativa. FEBS Lett. 581, 2929–2934. doi: 10.1016/j.febslet.2007.05.043
Tian, T., Liu, Y., Yan, H., You, Q., Yi, X., Du, Z., et al. (2017). agriGO v2.0: a GO analysis toolkit for the agricultural community, 2017 update. Nucleic Acids Res. 45, W122–W129. doi: 10.1093/nar/gkx382
Todaka, D., Nakashima, K., Shinozaki, K., and Yamaguchi-Shinozaki, K. (2012). Toward understanding transcriptional regulatory networks in abiotic stress responses and tolerance in rice. Rice 5:6. doi: 10.1186/1939-8433-5-6
Trapnell, C., Roberts, A., Goff, L., Pertea, G., Kim, D., Kelley, D. R., et al. (2012). Differential gene and transcript expression analysis of RNA-seq experiments with TopHat and Cufflinks. Nat. Protoc. 7, 562–578. doi: 10.1038/nprot.2012.016
Udomchalothorn, T., Plaimas, K., Sripinyowanich, S., Boonchai, C., Kojonna, T., Chutimanukul, P., et al. (2017). OsNucleolin1-L expression in Arabidopsis enhances photosynthesis via transcriptome modification under salt stress conditions. Plant Cell Physiol. 58, 717–734. doi: 10.1093/pcp/pcx024
Ullah, A., Sun, H., Yang, X. Y., and Zhang, X. L. (2017). Drought coping strategies in cotton: increased crop per drop. Plant Biotechnol. J. 15, 271–284. doi: 10.1111/pbi.12688
Vaughan, M. M., Christensen, S., Schmelz, E. A., Huffaker, A., Mcauslane, H. J., Alborn, H. T., et al. (2015). Accumulation of terpenoid phytoalexins in maize roots is associated with drought tolerance. Plant Cell Environ. 38, 2195–2207. doi: 10.1111/pce.12482
Walia, H., Wilson, C., Condamine, P., Liu, X., Ismail, A. M., Zeng, L., et al. (2005). Comparative transcriptional profiling of two contrasting rice genotypes under salinity stress during the vegetative growth stage. Plant Physiol. 139, 822–835.
Walia, H., Wilson, C., Ismail, A. M., Close, T. J., and Cui, X. (2009). Comparing genomic expression patterns across plant species reveals highly diverged transcriptional dynamics in response to salt stress. BMC Genomics 10:398. doi: 10.1104/pp.105.065961
Walia, H., Wilson, C., Zeng, L., Ismail, A. M., Condamine, P., and Close, T. J. (2007). Genome-wide transcriptional analysis of salinity stressed japonica and indica rice genotypes during panicle initiation stage. Plant Mol. Biol. 63, 609–623. doi: 10.1007/s11103-006-9112-0
Wang, J., Wang, H. Y., Fu, Y. P., Huang, T. T., Liu, Y. B., and Wang, X. W. (2021). Genetic variance and transcriptional regulation modulate terpenoid biosynthesis in trichomes of Nicotiana tabacum under drought. Ind. Crop Prod. 167:113501. doi: 10.1016/j.indcrop.2021.113501
Wang, M., Tu, L., Yuan, D., Zhu, D., Shen, C., Li, J., et al. (2019). Reference genome sequences of two cultivated allotetraploid cottons, Gossypium hirsutum and Gossypium barbadense. Nat. Genet. 51, 224–229. doi: 10.1038/s41588-018-0282-x
Wang, T. T., Yang, B. X., Guan, Q. J., Chen, X., Zhong, Z. H., Huang, W., et al. (2019). Transcriptional regulation of Lonicera japonica Thunb. during flower development as revealed by comprehensive analysis of transcription factors. BMC Plant Biol. 19:198. doi: 10.1186/s12870-019-1803-1
Wang, Q., Eneji, A. E., Kong, X. Q., Wang, K. Y., and Dong, H. Z. (2015). Salt stress effects on secondary metabolites of cotton in relation to gene expression responsible for aphid development. PLoS One 10:e0129541. doi: 10.1371/journal.pone.0129541
Warde-Farley, D., Donaldson, S. L., Comes, O., Zuberi, K., Badrawi, R., Chao, P., et al. (2010). The GeneMANIA prediction server: biological network integration for gene prioritization and predicting gene function. Nucleic Acids Res. 38, W214–W220. doi: 10.1093/nar/gkq537
Wardhan, V., Jahan, K., Gupta, S., Chennareddy, S., Datta, A., Chakraborty, S., et al. (2012). Overexpression of CaTLP1, a putative transcription factor in chickpea (Cicer arietinum L.), promotes stress tolerance. Plant Mol. Biol. 79, 479–493. doi: 10.1007/s11103-012-9925-y
Wilkins, O., Hafemeister, C., Plessis, A., Holloway-Phillips, M. M., Pham, G. M., Nicotra, A. B., et al. (2016). EGRINs (environmental gene regulatory influence networks) in rice that function in the response to water deficit, high temperature, and agricultural environments. Plant Cell 28, 2365–2384. doi: 10.1105/tpc.16.00158
Xu, J. N., Xing, S. S., Zhang, Z. F., Chen, X. S., and Wang, X. Y. (2016). Genome-wide identification and expression analysis of the tubby-like protein family in the Malus domestica genome. Front. Plant Sci. 7:1693. doi: 10.3389/fpls.2016.01693
Yang, Z., Li, J. L., Liu, L. N., Xie, Q., and Sui, N. (2020). Photosynthetic regulation under salt stress and salt-tolerance mechanism of sweet sorghum. Front. Plant Sci. 10:1722. doi: 10.3389/fpls.2019.01722
Yang, Z. F., Zhou, Y., Wang, X. F., Gu, S. L., Yu, J. M., Liang, G. H., et al. (2008). Genomewide comparative phylogenetic and molecular evolutionary analysis of tubby-like protein family in Arabidopsis, rice, and poplar. Genomics 92, 246–253. doi: 10.1016/j.ygeno.2008.06.001
Zare-Hassani, E., Motafakkerazad, R., Razeghi, J., and Kosari-Nasab, M. (2019). The effects of methyl jasmonate and salicylic acid on the production of secondary metabolites in organ culture of Ziziphora persica. Plant Cell Tissue Organ Cult. 138, 437–444. doi: 10.1007/s11240-019-01639-x
Zhang, Z., Mao, C. Y., Shi, Z., and Kou, X. H. (2017). The amino acid metabolic and carbohydrate metabolic pathway play important roles during salt-stress response in tomato. Front. Plant Sci. 8:1231. doi: 10.3389/fpls.2017.01231
Zhao, P. S., Liu, F., Zheng, G. C., and Liu, H. (2011). Group 3 late embryogenesis abundant protein in Arabidopsis: structure, regulation, and function. Acta Physiol. Plant. 33, 1063–1073. doi: 10.1007/s11738-010-0678-4
Zhu, J. K. (2002). Salt and drought stress signal transduction in plants. Annu. Rev. Plant Biol. 53, 247–273. doi: 10.1146/annurev.arplant.53.091401.143329
Keywords: stress, meta-analysis, cotton, hub genes, network
Citation: Bano N, Fakhrah S, Mohanty CS and Bag SK (2022) Transcriptome Meta-Analysis Associated Targeting Hub Genes and Pathways of Drought and Salt Stress Responses in Cotton (Gossypium hirsutum): A Network Biology Approach. Front. Plant Sci. 13:818472. doi: 10.3389/fpls.2022.818472
Received: 19 November 2021; Accepted: 21 March 2022;
Published: 25 April 2022.
Edited by:
Qing Liu, Commonwealth Scientific and Industrial Research Organisation (CSIRO), AustraliaReviewed by:
Jelli Venkatesh, Seoul National University, South KoreaCopyright © 2022 Bano, Fakhrah, Mohanty and Bag. This is an open-access article distributed under the terms of the Creative Commons Attribution License (CC BY). The use, distribution or reproduction in other forums is permitted, provided the original author(s) and the copyright owner(s) are credited and that the original publication in this journal is cited, in accordance with accepted academic practice. No use, distribution or reproduction is permitted which does not comply with these terms.
*Correspondence: Sumit Kumar Bag, c3VtaXQuYmFnQG5icmkucmVzLmlu
Disclaimer: All claims expressed in this article are solely those of the authors and do not necessarily represent those of their affiliated organizations, or those of the publisher, the editors and the reviewers. Any product that may be evaluated in this article or claim that may be made by its manufacturer is not guaranteed or endorsed by the publisher.
Research integrity at Frontiers
Learn more about the work of our research integrity team to safeguard the quality of each article we publish.